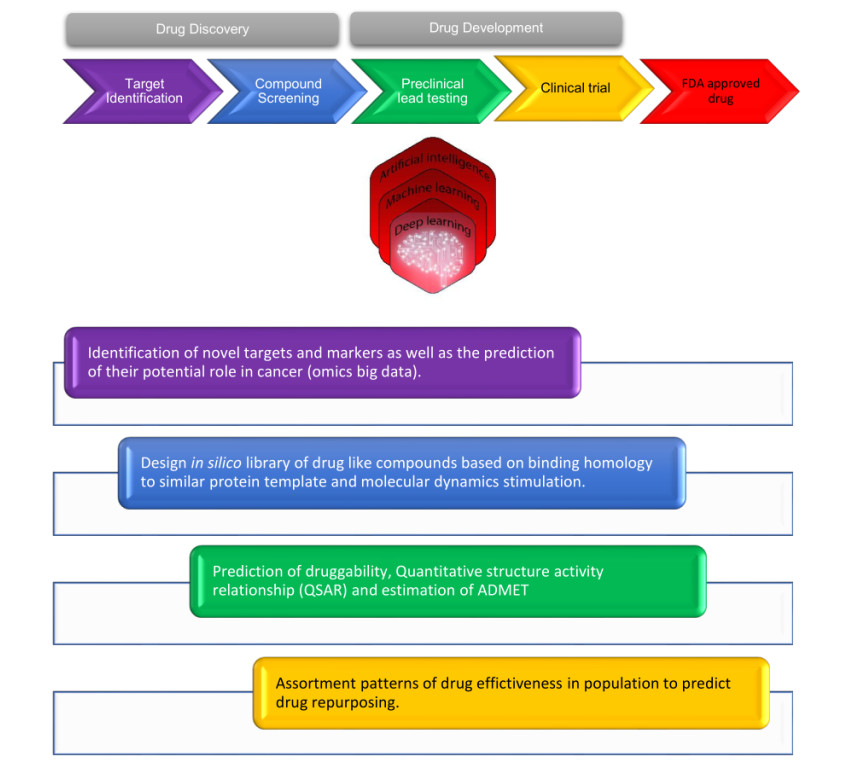
Citation: Rachael C. Adams, Behnam Rashidieh. Can computers conceive the complexity of cancer to cure it? Using artificial intelligence technology in cancer modelling and drug discovery[J]. Mathematical Biosciences and Engineering, 2020, 17(6): 6515-6530. doi: 10.3934/mbe.2020340
[1] | Juan Zhou, Xiong Li, Yuanting Ma, Zejiu Wu, Ziruo Xie, Yuqi Zhang, Yiming Wei . Optimal modeling of anti-breast cancer candidate drugs screening based on multi-model ensemble learning with imbalanced data. Mathematical Biosciences and Engineering, 2023, 20(3): 5117-5134. doi: 10.3934/mbe.2023237 |
[2] | Huiqing Wang, Xiao Han, Jianxue Ren, Hao Cheng, Haolin Li, Ying Li, Xue Li . A prognostic prediction model for ovarian cancer using a cross-modal view correlation discovery network. Mathematical Biosciences and Engineering, 2024, 21(1): 736-764. doi: 10.3934/mbe.2024031 |
[3] | Anastasia-Maria Leventi-Peetz, Kai Weber . Probabilistic machine learning for breast cancer classification. Mathematical Biosciences and Engineering, 2023, 20(1): 624-655. doi: 10.3934/mbe.2023029 |
[4] | Muhammad Bilal Shoaib Khan, Atta-ur-Rahman, Muhammad Saqib Nawaz, Rashad Ahmed, Muhammad Adnan Khan, Amir Mosavi . Intelligent breast cancer diagnostic system empowered by deep extreme gradient descent optimization. Mathematical Biosciences and Engineering, 2022, 19(8): 7978-8002. doi: 10.3934/mbe.2022373 |
[5] | Delong Cui, Hong Huang, Zhiping Peng, Qirui Li, Jieguang He, Jinbo Qiu, Xinlong Luo, Jiangtao Ou, Chengyuan Fan . Next-generation 5G fusion-based intelligent health-monitoring platform for ethylene cracking furnace tube. Mathematical Biosciences and Engineering, 2022, 19(9): 9168-9199. doi: 10.3934/mbe.2022426 |
[6] | Swati Shinde, Madhura Kalbhor, Pankaj Wajire . DeepCyto: a hybrid framework for cervical cancer classification by using deep feature fusion of cytology images. Mathematical Biosciences and Engineering, 2022, 19(7): 6415-6434. doi: 10.3934/mbe.2022301 |
[7] | Yiqin Bao, Hongbing Lu, Qiang Zhao, Zhongxue Yang, Wenbin Xu . Detection system of dead and sick chickens in large scale farms based on artificial intelligence. Mathematical Biosciences and Engineering, 2021, 18(5): 6117-6135. doi: 10.3934/mbe.2021306 |
[8] | Bo An . Construction and application of Chinese breast cancer knowledge graph based on multi-source heterogeneous data. Mathematical Biosciences and Engineering, 2023, 20(4): 6776-6799. doi: 10.3934/mbe.2023292 |
[9] | Vinh Huy Chau . Powerlifting score prediction using a machine learning method. Mathematical Biosciences and Engineering, 2021, 18(2): 1040-1050. doi: 10.3934/mbe.2021056 |
[10] | Saied M. Abd El-Atty, Konstantinos A. Lizos, Osama Alfarraj, Faird Shawki . Internet of Bio Nano Things-based FRET nanocommunications for eHealth. Mathematical Biosciences and Engineering, 2023, 20(5): 9246-9267. doi: 10.3934/mbe.2023405 |
Cancer is a highly dynamic, heterogeneous, and complex disease underlain by diverse aberrations at both the molecular and cellular level. Consequently, cancerous cells induce abnormal changes at the organ and tissue level, with potentially lethal outcomes. As one of the leading causes of death worldwide, cancers pose a significant challenge for the healthcare system, encompassing burdens on research, resource development and acquisition, and patient care [1]. Positive impacts on patient well-being and outcome are at the forefront of cancer research aims, meaning the demand for effective therapies in conjunction with a personalized medicine approach has never been more apparent [2]. However, novel cancer therapeutics comprise a large proportion of failed drug trials, resulting in very limited new treatment options for large cohorts of patients, many of whom are refractory to current treatments [3,4]. With the use of current technologies such as RNA-sequencing and extensive proteomic analyses allowing access to enormous amounts of highly informative data, it is essential to employ techniques which allow us to capitalize on this information. Therefore, this highlights the urgent need to take advantage of automation and computer-based modelling in order to efficiently process extensive datasets in order to streamline the drug development process. This approach enables data mining on an ever-increasing scale, and high throughput screening with the potential for identification and validation of new drug targets or active compounds whilst gaining further insight into the complexities of disease phenotypes and mechanisms [5].
AI is the computer-driven simulation of human thought patterns to mimic behaviour and problem-solving strategies. AI technology extracts relationships and concepts from a data set and learns from available patterns independently [4,5]. This technology includes machine learning (ML), and deep learning (DL). As a subfield of AI, ML automates model development by iteratively learning from data sets through statistical methods, advantageously identifying patterns within the data without requiring manual coding to detect them. In other words, ML is computer algorithms that can predict future outcomes through learning from unstructured or structured data, identification and classification of hidden patterns [4,5]. This tool has garnered significant interest in the pharmacological industry in recent years to improve all aspects of the drug discovery pipeline, from target identification up to pre-clinical and clinical development phases [5]. ML holds great predictive power with algorithms capable of independently adapting as the data available to learn from increases [5]. DL is an ML based method utilizing a logical structure of artificial neural networks which is inspired by the biological neural networks to recognise and discriminate different patterns [5]. These approaches have revolutionized the context of systems biology from diagnosis, to recognizing the patterns of diseases and identifying novel drug targets (Figure 1). Given the non-linear and heterogenous composition and communication of structures and pathways that define biological functions, these methods can be particularly useful to search for modifiable targets and their correlations to the specific disease [6]. It is evident that the wealth of literature information and available data pertaining to the study of cancer biology appropriately poises this field for investigation with AI models to derive outputs with high translational capacity. Synergistically, the recent advancements in training DL algorithms to detect levels of error, and back-propagation through the model to determine the most informative features have driven the implementation of these models in related fields such as radiology and pathology [7]. The ability to automatically and repetitively process high volumes of clinical data, especially with various types of images being a primary data type, represents a significant clinical advantage and has led to the recent popularization of AI methods for cancer diagnosis [7]. Subsequently, large ‘omic’ data sets that typically provide directionality for identifying novel drug targets or testing responses to therapeutic agents can be rapidly screened with in silico analyses, thus providing an opportunity to implement AI models throughout the cancer diagnosis and treatment process [8].
Given these considerations, here we aim to review the current standing of computational modelling and ML approaches to cancer research and drug discovery. We seek to highlight the major ML techniques used in cancer modelling interrelated with the importance of understanding the underlying biology and physiology which directs research advances. Additionally, we provide an overview of the drug development pipeline with consideration of ML methods being used to streamline this process, and the associated challenges—both biological and technical. The scope of this review cannot explore all machine learning approaches in depth, particularly in the setting of cancer therapies, and for this we refer the reader to several excellent reviews which highlight the importance of this rapidly evolving field [7,8,9].
Disease modelling may be articulated as a problem-solving challenge, whereby the model facilitates our understanding of an issue by incorporating the essential aspects, functions, and interactions of a system [10]. The research question driving an investigation must be appropriately matched by the resolution of the model, such that the level of detail being investigated will be sufficient to answer the question [10]. Ultimately, the model should provide new and reproducible insights into molecular mechanisms underlying disease phenotypes [10], and ideally show strong predictive potential in order to enhance preclinical experimental design [3]. Pertinent in the case of cancer therapy is seeking to understand how modulation of a validated target may alter, and preferentially improve, a patient’s disease state [5]. Using ML algorithms to survey databases, data sets, or relevant literature [5] to initially identify targets provides increased flexibility compared to in vitro or in vivo target screening and can reduce bias and uncertainty that results from both biological variation and certain experimental techniques [6]. For example, Kumari and colleagues developed a prediction model to identify candidate drug target proteins based on amino-acid sequence features, through the use of databases documenting human protein sequences [11]. Such studies inform model-driven experimentation to test hypotheses in a pre-clinical setting to validate the target [6]. Additionally, the feedback to further optimize computer models and expand the parameters they consider [3]. Iteratively, a greater information output can be gained from in silico models with increased perturbations to replicate the complexity of cancer cells and tumors [3], producing functional responses as the algorithm continues to learn cellular characteristics and adapt to heterogenous environmental changes within a cancer system [12].
Designing computational models to investigate various aspects of cancer pathophysiology requires a deep understanding of the interplay between single cells and the tumour microenvironment. From this foundation, discrete in silico cell-based models can be derived to study single cells at a level that conserves the intracellular dynamics that progressively transform in cancer after genetic mutation [12,13]. In conjunction, it is often beneficial to model the tissue microenvironment as a continuum, a format which typically encompasses features such as transport of oxygen, nutrients, waste, and drugs [12,14]. Combining different methods strengthens the biological relevance of the model, connecting cellular phenotypes to local conditions, and whole tumour changes to fluctuations at the cellular level—concepts that are particularly crucial when investigating drug effects [15]. Metzcar et al. accurately describe this approach as the creation of a ‘virtual laboratory’, given discrete cell-based models track specific cellular parameters that may be perturbed during tumorigenesis [12]. Additionally, these approaches provide information about a cell’s behaviour within given environmental constraints, cell-cell interactions, and responses to selective pressures [12]. Of further benefit is the fact that validated computational models allow deep and thoughtful exploration of certain paradigms that cannot be tested on laboratory animals and/or humans due to economic, ethical, welfare, or practical restrictions [3]. Implementing ML models, heavily informed by available preclinical data and extensive databases as previously mentioned, can explore diverse hypotheses and improve our understanding of the system in question as the model iteratively updates.
Agent-based models are commonly employed in cancer studies, as each cell can be modelled as an independent autonomous entity, referred to as an agent, which makes decisions based on the simulated environment and the learned rules of the model [16]. This captures the inherent variability and inhomogeneity between cells within a population, which is essential when considering the abnormal development and signalling pathways exhibited by tumour cells [13,17]. Lattice-based models spatially restrict cells to a grid network [18] allowing evaluation of their spatial resolution [17] with respect to fluid dynamics and other physical forces, as well as interaction with other cells [19]. Firstly, as a form of lattice-based modelling, cellular automaton (CA) models are often employed to replicate monolayers or multi-cell solid tumour structures, where the volume of one agent represents a single cell [12,17]. Cai et al [20] implemented a CA model to investigate tumour growth and angiogenesis with consideration for vasculature remodeling and blood flow dynamics, whereby the tumour cells would proliferate dependent on oxygen levels and space constraints, and vessel growth was contingent on substrate availability and mechanical stress. This model produced evidence for a strong association between highly proliferative tumour cells and the microvasculature, supporting previous observations that increased angiogenesis is linked with tumour growth and further refining our understanding of the use of tumour-associated vasculature as a drug-delivery system [20,21]. Secondly, lattice-gas CA (LGCA) models are most recognised for demonstrating the invasive capacity of tumour cells, with a single agent containing multiple cells which grow to great densities over time to illustrate cell population dynamics [12,17,22]. An influential study by Hatzikirou et al. [23] investigating the mechanism driving invasive tumour phenotypes and uncontrolled cell division used an LGCA model to demonstrate the cellular response, i.e., propagation, migration, or apoptosis, to varying degrees of hypoxia. The advantage of characterizing cell positioning and velocity with the LGCA model allowed this group to conclude that tumour cells exhibit phenotypic plasticity and adapt to local environmental changes [23]. LGCA models are typically favored when considering tumour invasion, given they have been shown to predict the motion-dependent velocity of the discrete cells at the helm of the invasion as they pass through channels in lattice agent sites [12,24]. In contrast, increased resolution of tumour behaviour can be accessed with the complex probabilistic Cellular Potts model (CPM), the third form of lattice-based modelling whereby each cell is represented by multiple lattice sites [12,25,26]. Designing targeted therapies requires an understanding of the irregularity and heterogeneity of tumour cell morphology; insight produced by a CPM as it tracks dynamic and fluctuating cell shapes and volumes in response to environmental interactions under the principle of free energy minimization [26,27,28].
Alternatively, off-lattice models can be used to depict cellular spatial organisation given each cell is not restricted to a grid for mapping their movement [29]. Centre-based off-lattice approaches represent cells as points or spheres, allowing the model to track the cell’s centre of mass to understand forces, such as adhesion and repulsion, endured by the cell within a growing tumour [12,26]. Although such models require consideration of cell collisions due to the lack of grid guidance, they aid in the study of tumour morphology with respect to cell-cell and cell-extracellular matrix interactions, which may be further enhanced by modelling each cell as a cluster of multiple subcompartments [29,30]. Off-lattice models also offer boundary-based options in which cells are represented as polygons to identify the cellular adjustments to external forces, which has proven informative in the study of tissue confluency, protrusion and contraction for motility, and tissue morphogenesis [31,32].
With applications of AI to biomedical sciences, we must be cognizant of properly training models to avoid bias, and to ensure algorithms are cross-validated. Proper model development is contingent on establishing sensitivity with relevant data and populations [33] and appropriate decision boundaries applied to unseen data [34]. Shirin et al. [34] investigated the efficiency of various cross-validation methods to optimize artificial neural network structures for cancer prognosis. The purpose of this is to assess how the results of a model can be applied to an independent data set to prevent over-fitting [34,35]. It was determined that stratified rather than simple k-fold cross validation is preferable for evaluating a network. Stratification of the data through rearrangement into folds, each representing the whole dataset, as opposed to a single partition, prevents biased outputs as the network is tested. Reviewing the accuracy of the model to provide reliable data validates the output patterns and provides information about the model’s performance.
To capitalise on the benefits of computational modelling approaches, significant efforts have been made recently in order to make these technologies accessible and readily available, without the pre-requisite of extensive experience. Various programs and software packages are easily available to assist in dissecting cellular phenotypes and microenvironment interactions, with specific programs of interest summarized in Table 1. The interaction of tumours with adjacent cells create a tumour microenvironment (TME) which induces tumour-derived signalling. TME affects several immune effector and mediator cells generally to suppress tumour infiltration and anti-tumour functions as well as evading immune surveillance as a hallmark of cancer [36,37]. Many web-based tools have been developed to estimate the abundances of immune cells in tumour infiltration, using gene expression data such as CIBERSORT developed by Alizadeh laboratory in Java and R platform [38]. Later, they expanded the application of this tool by ML methods like support vector regression (SVR) to profile tumour infiltrating immune cells using RNA-from bulk RNA sequencing data without physical single cell isolation [39]. Multiple computational methods have also been developed to utilise gene expression profiles (GEPs) based on linear regression to find, understand and correct the patterns of expressed genes in specific cell types. As an example, Scaden is a deep neural network (DNN) based method which quantifies GEPs from RNA-seq data to predict cell fraction profiles [40].
Resource | Description | Application |
PhysiCell | Agent-based (lattice-free) multicellular simulation platform designed for use on standard computers with computational cost related linearly to the number of cells being modelled [12]. | Understanding the contribution of cell growth and interaction to tissue-scale changes. Encompasses cellular processes including division, apoptosis, and necrosis, in the context of heterogeneous cell populations and dynamic microenvironments [80,81]. |
BioFVM (Finite Volume Method for Biological Problems) | Widely accessible code for modelling three-dimensional transport system in a biological context using partial differential equations [46]. | The substrate surrounding cells varies widely and is subject to various processes including diffusion, secretion, uptake, and decay as cellular phenotypes changes in response to the environment. BioVFM allows for multicellular modelling with consideration of three-dimensional substrate changes to better understand the interactions of cells with their immediate surroundings, with implications for signalling molecules and drugs within the substrate. |
CellPD: Cell Phenotype Digitizer | An accessible, user-friendly program designed to quantify features of a cell’s phenotype. Designed with consideration for experimental variability and irregularities [47]. | The complexity of biological systems can be increasingly characterised with the quantification of various cellular parameters, such as proliferation and death. This allows for measuring cellular changes in particular conditions over multiple time points whilst removing the variability associated with laboratory-based experiments. This also provides the opportunity to quantitatively assess phenotypic changes with environmental disturbances, such as the introduction of a novel drug. |
multicellDS (Multicellular Data Standard) | A platform designed to curate public, standardised datasets to maximise the output of experimental and clinical information. Facilitates model refinement and streamlining datasets from high-throughput studies with an array of data processing techniques [48]. | Collaborative, data-sharing efforts may introduce new insights and strengthen our ability to generate and test novel hypotheses. The fusion of data from different groups and research focuses can only help in deepening our understanding of the cellular biology underlying the complexities of cancer. |
Tumour resistance to chemotherapy poses a significant and complicated challenge to successful treatment and limits the span of effective clinical approaches. Resistance may be attributable to a variety of mechanisms, experimentally revealed to include genetic, cellular, pharmacokinetic contributions, among others [41]. The combination of extensive data sets generated from in vivo and in vitro experiments and clinical cohorts has encouraged a new era of studying molecular dynamics through computational simulations, which has improved our understanding of resistance mechanisms but also advanced the tools available for disease prognosis [42,43]. Additionally, high-throughput approaches allow for the incorporation of the pharmacokinetics and pharmacodynamics of drug treatments; consideration of which may be limited in experiment-based studies [44]. Ultimately, the goal of implementing modelling techniques in the study of molecular resistance is to facilitate more effective drug design by overcoming the intricacies associated with cancer biology [45].
Drug resistance models can be generalised as either mechanistic-based or data-driven prediction-based [49]. Mechanistic-based models produce quantitative readouts at both the molecular and cellular level. This may originate at the fundamental level of drug-target binding relationships, which have been shown to be altered by conformational target protein changes caused by mutation [49]. Agent-based models are also employed in this context to address cellular signalling responses to drugs, with consideration of the microenvironment and dynamic effects of drug concentration [42]. Such interactions may be further complicated by changes in cellular behaviour and population composition, which are commonly represented by ordinary differential equations to include cell cycle changes and dynamic interactions with the immune system in order to identify critical parameters for drug efficacy [50,51]. Drug resistance is correlated with multiple factors including cellular phenotypes and activity, and responses to extracellular signals. To assess the interaction of these varying components, Jackson et al. [51] developed a hybrid discrete-continuous model using partial differential equations, whereby individual cells were exposed to DNA damage-inducing drugs and altered oxygen concentrations in a fixed vasculature landscape [52]. Here, resistance was modelled as either pre-existing or as a consequence of drug treatment, permitting comparison of resistance variation in the context of tumour growth and microenvironmental conditions [52]. Ultimately, this model indicated the overt importance of the microenvironment only when cells acquire resistance. Such studies improve our understanding of the techniques to appropriately target various tumour cell populations and assist in identifying drug combination schedules which may lead to more effective tumour control.
The drug discovery and development pipeline are complex multi-stage processes dependent on identifying a therapeutically targetable facet of disease and using extensive high-quality data sets to produce a safe and efficacious drug. The opportunity to take advantage of machine learning capabilities is one that has gained increased interest recently. Not only does this provide a high-throughput biological approach with exorbitant potential for data analysis and storage, but it also increases the likelihood of developing a successful product and avoiding the poor drug approval rate that plagues the pharmaceutical industry [5].
Developing methods to predict the mechanisms of action of new drugs early screening processes using neural network automation and evolutionary algorithms may profoundly impact the pharmaceutical industry. ML-based recognition of patterns and integrated learning for drug discovery represents the capability of AI to solve problems by learning from experience and novel conditions. Various ML algorithms have been developed and/or adopted for drug discovery, such as supervised learning algorithms of support vector machine (SVM) and random forest (RF) that can be utilized for ligand or structure-based virtual screening and quantitative structure-activity relationship (QSAR) methods. These algorithms, alongside others for de novo drug design and Absorption, Distribution, Metabolism, Excretion and Toxicity (ADMET) analysis are extensively discussed in Yang et al. review [53]. Here, we will briefly describe the application of machine learning techniques in the context of the various stages of drug-discovery which summarised in Figure 1, to assess how the artificial intelligence approach is revolutionizing therapeutic development and overcoming typical limitations in this field.
Currently, the role of machine learning algorithms in pre-clinical drug development lies in biomarker prediction and high-throughput screening of cancer responses to novel therapeutics [5]. One of the most prominent issues in the clinical management of cancer is the accurate prediction of people who may develop a malignant disease [54]. Current risk stratification protocols are dependent on prognostic biomarkers, defined as a particular property of diseased tissue which, when analysed, correlates with the possibility of cancer development [54]. Existing biomarkers often show limited predictive potential and commonly only identified through long and arduous surveillance processes, thus warranting the application of computational models to streamline and improve biomarker discovery [55]. Fischer et al. validated this premise by devising computational models to represent various cell populations, such as myeloid and lymphoid cells, which were then combined in a multi-cell model scheme to predict the cytokine and chemokine signatures in inflamed tissue [55]. This approach inherently incorporated time-dependent fluctuations in signalling pathways and kinetics of cell interactions. Ultimately, resembling tissue processes in a computational model provides information about cell-cell interactions and resulting signalling and inflammatory changes, showing great potential as a method to screen putative therapeutics and assess their effects on biomarker profiles [54]. Additionally, biomarker prediction allows for progress in the direction of personalized medicine, as information about the expression of particular markers is informative about how a drug will affect individual patients. Seemingly, various limitations are associated with the predictive capacity of biomarkers, including reproducibility, model selection, and data quality, meaning their application is often limited to simulations or pre-clinical settings, rather than progressing into clinical practice [5].
Furthermore, developing techniques to understand the metastatic potential of a tumour is an active research topic in relation to cancer prognosis, primarily to avoid patient exposure to unnecessary treatments [56]. Considering this, Mojarad et al. [57] investigated a number of pathological prognostic breast cancer markers including tumour size, expression of estrogen and progesterone receptors, and levels of Ki-67 and p53 in an artificial neural network model to test their reliability for predicting metastasis. Using this platform, various combinations of the markers, and their inherent biological interaction, could be considered to assess the effectiveness of their use for prognosis. The predictive capacity of artificial neural networks proves them to be important in dissecting the non-linear relationship between the wealth of biomarkers and disease progression and outcome.
An additional aspect where AI models have proven beneficial is the prediction of cancer cell sensitivity to therapeutics, particularly in the context of drug repurposing and personalized medicine. Menden et al. [58] developed a high-throughput screening method to assess the response of genetically variable cancer cell lines to active compounds, with readouts of the drug’s effectiveness (IC50) dependent on the genomic heterogeneity and the chemical structure and on-target interactions of the drug. Such models are fundamental to implementing AI screening methods to assess the anti-tumour efficacy of novel therapeutics. By basing the model on the cellular genetic landscape, this poises the framework for application in drug repurposing, as existing therapies could be incorporated and their effect on various genetic targets assessed, thus potentially increasing their treatment applicability [59].
Two fundamental questions immediately arise in the development of new drugs. Firstly, whether modifying a certain molecular or cellular target will have a noticeable and beneficial effect on the disease state, and secondly, whether these targets can legitimately be altered with a deliverable drug [5]. To answer these questions, there must be sufficient evidence to suggest that the target plays a causal role in disease development or progression. AI models of natural language processing for text mining have been employed to survey the literature to identify disease targets and associated genetic markers with high confidence [60,61]. In support of this, the abundance of data available in public databases from both pre-clinical and clinical settings provides homeostatic and disease-state genetic profiles, expanding into transcriptomic, metabolic, and proteomic landscapes [51]. Additionally, chemical libraries may be screened and in silico models developed to predict the feasibility of structural interactions between potential drug molecules and biological targets with implications for the drug-target association, and pharmacokinetics and pharmacodynamics [63,64]. The depth and multi-dimensionality of such datasets require application carefully selected models so as to gain the most insight with optimal efficiency. The aim of these data mining and high-throughput screening approaches is to identify a ‘hit compound’ which can then be tested in biological assays, usually beginning with cell-based experiments followed by in vivo models, with tentative hypotheses about their activity in association with the target [65]. Due to the predictive capacity of ML models, a significant advantage of implementing computational techniques is the refinement and prioritisation of promising targets which progress to testing in in vivo or in vitro systems, thus reducing attrition rates and expenditure [4].
Extensive compound libraries are screened using both virtual and experimental methods to determine the efficacy of the compound in modifying the disease target [5]. This is followed by systematic modifications to refine hit compounds, aiming to improve their target affinity and selectivity whilst minimising off-target effects and toxicity [4,5]. This may be aided by the computational screening of compounds with similar structure and properties to the lead compound, improving the understanding of the activity of the small molecule and enhancements that may induce greater efficacy [66]. De novo drug design has also proven informative, with deep learning models used to generate compounds with essentially optimal parameters such as solubility and bioactivity, thus providing additional insights as to how best to modify a lead compound [67]. Additionally, deep learning simulations are employed to model the most efficient chemical synthesis processes, thus streamlining the laboratory-based production of the target [5]. Importantly, AI models may improve confidence in the safety profiling of novel drugs due to their capacity for prediction of mechanisms of action, inherently considering cell signalling pathways and downstream effects of drug-target interactions. A variety of AI tools and platforms have been developed for the purpose of predicting the toxicity of new compounds, exploiting chemical databases to link chemical structures with known toxic properties [68].
Translation of preclinical findings into clinical treatment through controlled trials in the context of cancer therapeutics shows a poor success response, with as few as 8% of novel drugs approved for use [64]. It has been suggested that implementing computational models to identify appropriate patient populations for drug trials could improve trial outcomes [69]. This approach would allow a more accurate assessment of the effect of novel drugs on patients expressing relevant phenotypes based on biomarker prediction who would likely respond to the treatment [4]. Additionally, enrolling cancer patients in trials predicted to benefit them based on such analyses may aid in improving enrolment statistics and medication adherence [70].
The application of AI in assessing disease pathology after drug treatment is an area where quantitative results would be extremely beneficial, as opposed to qualitative descriptions [17]. Whilst the use of algorithms for efficient quantitation of tissue morphological changes and in histopathology samples is necessary before human trials to understand drug effects on cellular and tissue changes, there are obvious applications for such models after drug treatment to assess patient response [5]. Parameters such as cellular heterogeneity, cell-cell interactions, marker expression, and cell morphology have been quantified have been investigated with machine learning models [65,66]. This would; however, require larger cohort sizes to obtain sufficient information to understand the cancer response to the tumour, alongside conventional analysis to ensure appropriate classification and interpretable results [5].
In efforts to progress our understanding of cancer biology and pioneer new treatments, AI offers a sophisticated tool for processing biomedical literature and mining extensive datasets. Already, large pharmaceutical companies and technology groups are investing in these approaches to fast-track drug discovery [72], relying on collaboration between various scientific disciplines, and pharmaceutical and technology companies. High-throughput screening has already been implemented widely and integrated into laboratory settings such as with the recently established ‘cancer-on-a-chip’ platform [73]. Using cost-effective miniaturized platforms to accelerate testing whilst recapitulating the tumour microenvironment, lymphatics, angiogenesis, metastasis, and immune infiltrate, provides a powerful tool for generating large amounts of data and quickly testing possible treatments [73]. Additional prospects involve further personalization of treatments and improving the ability to predict tumour and patient responses to therapy.
We have identified many drugs for targeting disease throughout our publications [74,75,76,77,78,79,80]. However, according to practical experience, this is a time-consuming procedure that can be accelerated by utilizing modern technologies and automation. The recent crisis of COVID19 pandemic divulged the emerging prerequisite of applying AI in finding new therapeutics and vaccines urgently. While the significance of AI is already evident in all aspects of cancer prognosis, diagnosis, and drug development pipeline; it remains important to consider and maintain the balance between the curation of reproducible, trustworthy biological data with computation skills and the expertise to appropriately select and develop simulations to model scientific phenomena. ML approaches show great promise in their predictive capacity for enhancing and fast-tracking necessary novel therapeutics. Harnessing computational power for advances in healthcare and patient welfare using solid data as the foundation introduces vast possibilities for new therapies, with great potential for progress in the field of personalized medicine [81,82]. Researchers argue that a series of experiments can solve problems and address hypothetical questions. However, attempting to approach similar problems amongst different contexts with the same methodology may be biased and produce sub-optimal outcomes. Concepts of modern biology which carefully define the cell as a system can be revolutionized by converting communications, signalling pathways and interactions as algorithms, thus allowing us to harness computer power to better understand biological processes. With this approach, we may more directly investigate biological systems by reducing the complexity of the fundamental information. Defining user-friendly languages to analyse and predict biological functions rapidly and accurately will aid in converting new targets to opportunities for therapeutic intervention. This approach will enhance both the quality of decision making with unbiased and unsupervised means, and the quantity of data that can be analysed, thereby accelerating experimental processes with machines thinking and deciding to find therapeutics for terminal diseases such as cancer. The main question that should be answered in the future is whether AI-based, ML algorithms and DL methods can conceive the heterogeneity of cancer and combine multiple types of data and multi-omics big data from different resources to find their biological relation to enhance the prediction of effective drugs for targeting cancer.
The authors disclose no potential conflicts of interest.
[1] | R. Mahumad, K. Alam, J. Dunn, J. Gow, Emerging cancer incidence, mortality, hospitalisation and associated burden among Australian cancer patients, 1982-2014: An incidence-based approach in terms of trends, determinants and inequality, BMJ Open, 5 (2019). |
[2] | M. Breitenbach, J. Hoffmann, Editorial: Cancer models, Front. Oncol., 8 (2018), 401-401. |
[3] | L. Ogilvie, A. Kovachev, C. Wierling, B. Lange, H. Lehrach, Models of models: A translational Route for cancer treatment and drug development, Front. Oncol., 7 (2017). |
[4] | K. Mak, M. Pichika, Artificial intelligence in drug development: Present status and future prospects, Drug Discovery Today, 24 (2019), 773-780. |
[5] | J. Vamathevan, D. Clark, P. Czodrowski, I. Dunham, E. Ferran, G. Lee, et al., Applications of machine learning in drug discovery and development, Nat. Rev. Drug Discovery, 18 (2018), 463-477. |
[6] | O. Wolkenhauer, Why model? Front. Phys., 5 (2014). |
[7] | A. Levine, C. Schlosser, J. Grewal., R. Coope, S. Jones, S. Yip, Rise of the machines: Advances in deep learning for cancer diagnosis, Trends Cancer, 5 (2019), 157-169. |
[8] | K. Vougas, T. Sakellaropolous, A. Kotsina, G. R. P. Foukas, A. Ntargaras, F. Koinis, et al., Machine learning and data mining frameworks for predicting drug response in cancer: An overview and a novel in silico screening process based on association rule mining. Pharmacol. Ther., 203 (2019), 107395. |
[9] | G. V. Sherbet, W. L. Woo, S. Dlay, Application of artificial intelligence-based technology in cancer management: A commentary on the deployment of artificial neural networks, Anticancer Res., 38 (2018), 6607-6613. |
[10] | R. M. Thomas, T. Van Dyke, G. Merlino, C. P. Day, Concepts in cancer modeling: A brief history, Cancer Res., 76 (2016), 5921-5925. |
[11] | P. Kumari, A. Nath, R. Chaube, Identification of human drug targets using machine-learning algorithms, Comput. Biol. Med., 56 (2014), 175-181. |
[12] | J. Metzcar, Y. Wang, R. Heiland, P. Macklin, A Review of cell-based computational modeling in cancer biology, JCO Clin. Cancer Inform., 3 (2019), 1-13. |
[13] | D. Hanahan, R. A. Weinberg, The hallmarks of cancer, Cell, 100 (2000), 57-70. |
[14] | A. Ghaffarizadeh, S. H. Friedman, P. Macklin, BioFVM: An efficient, parallelized diffusive transport solver for 3-D biological simulations, Bioinformatics, 32 (2016), 1256-1258. |
[15] | A. Ghaffarizadeh, R. Heiland, S. H. Friedman, S. M. Mumethaler, P. Macklin, PhysiCell: An open source physics-based cell simulator for 3-D multicellular systems, PLOS Comput. Biol., 14 (2018), e1005991. |
[16] | E. Bonabeau, Agent-based modeling: Methods and techniques for simulating human systems, Proc. Natl. Acad. Sci. USA, 99 (2002), 7280-7287. |
[17] | P. Van Liedekerke, M. M. Palm, N. Jagiella, D. Drasdo, Simulating tissue mechanics with agent-based models: Concepts, perspectives and some novel results, Comput. Particle Mech., 2 (2015), 401-444. |
[18] | R. C. Kennedy, G. E. Ropella, C. A. Hunt, A cell-centered, agent-based framework that enables flexible environment granularities, Theor. Biol. Med. Model. 13 (2016). |
[19] | J. Poleszczuk, P. Macklin, H. Enderling, Agent-based modeling of cancer stem cell driven solid tumor growth, Methods Mol. Biol., 1516 (2016), 335-346. |
[20] | Y. Cai, S. Xu, J. Wu, Q. Long, Coupled modelling of tumour angiogenesis, tumour growth and blood perfusion, J. Theor. Biol., 279 (2011), 90-101. |
[21] | A. Shirinifard, J. S. Gens, B. L. Zaitlen, N. J. Poplawski, M. Swat, J. A. Glazier, 3D multi-cell simulation of tumor growth and angiogenesis, PLOS One, 4 (2009), e7190. |
[22] | B. Chopard, R. Ouared, A. Deustch, H. Hatzikirou, D. Wolf-Gladrow, Lattice-gas cellular automaton models for biology: From fluids to cells, Acta Biotheor., 58 (2010), 329-340. |
[23] | H. Hatzikirou, D. Basanta, M. Simon, K. Schaller, A. Deustch, 'Go or Grow': The key to the emergence of invasion in tumour progression?, Math. Med. Biol.: A J. IMA, 29 (2010), 49-65. |
[24] | H. N. Weerasinghe, P. M. Burragem, K. Burrage, D. V. Nicolau, Mathematical models of cancer cell plasticity, J. Oncol., 2019. |
[25] | M. S. Alber, M. A. Kiskowski, J. A. Glazier, Y. Jiang, On cellular automaton approaches to modeling biological cells, in Mathematical Systems Theory in Biology, Communications, Computation, and Finance, Springer, New York, (2003), 1-39. |
[26] | A. Szabó, R. M. Merks, Cellular potts modeling of tumor growth, tumor invasion, and tumor evolution, Front. Oncol., 3 (2013). |
[27] | N. Guisoni, K. I. Mazzitello, L. Diambra, Modeling active cell movement with the potts model, Front. Phys., 6 (2018). |
[28] | E. G. Rens, L. Edelstein-Keshet, From energy to cellular forces in the cellular potts model: An algorithmic approach, PLOS Comput. Biol., 15 (2019), e1007459. |
[29] | K. A. Rejniak, A. R. A. Anderson, Hybrid models of tumor growth, Wiley Interdiscip. Rev.: Syst. Biol. Med., 3 (2011), 115-125. |
[30] | J. Jeon, V. Quaranta, P. T. Cummings, An off-lattice hybrid discrete-continuum model of tumor growth and invasion, Biophys. J., 98 (2010), 37-47. |
[31] | S. Koride, A. J. Loza, S. X. Sun, Epithelial vertex models with active biochemical regulation of contractility can explain organized collective cell motility, APL Bioeng., 2 (2018). |
[32] | S. Alt, P. Ganguly, G. Salbreux, Vertex models: from cell mechanics to tissue morphogenesis, Philos. Tran. R. Soc. London. Ser. B, Biol. Sci., 372 (2017). |
[33] | K. R. Foster, R. Koprowski, J. D. Skufca, Machine learning, medical diagnosis, and biomedical engineering research-commentary, Biomed. Eng. Online, 13 (2014), 94. |
[34] | A. M. Shirin, S. S. Dlay, W. L. Woo, G. V. Sherbet, Cross validation evaluation for breast cancer prediction using multilayer perceptron neural networks, Am. J. Eng. Appl. Sci., 4 (2012). |
[35] | T. Dietterich, Overfitting and undercomputing in machine learning, ACM Comput. Surveys, 27 (1995), 326-327. |
[36] | T. L. Whiteside, The tumor microenvironment and its role in promoting tumor growth, Oncogene, 27 (2018), 5904-5912. |
[37] | D. Hanahan, R. A. Weinberg, Hallmarks of cancer: the next generation, Cell, 144 (2011), 646-674. |
[38] | A. M. Newman, C. B. Steen, C. L. Liu, A. J. Gentles, A. A. Chaudhuri, F. Scherer, et al., Determining cell type abundance and expression from bulk tissues with digital cytometry. Nat. Biotech., 37 (2019), 773-782. |
[39] | B. Chen, M. S. Khodadoust, C. L. Liu, A. M. Newman, A. A. Alizadeh, Profiling tumor infiltrating immune cells with CIBERSORT, Methods Mol. Biol., 1711 (2018), 243-259. |
[40] | K. Menden, M. Marouf, S. Oller, A. Dalmia, D. S. Magruder, K. Kloiber, et al., Deep learning-based cell composition analysis from tissue expression profiles, Sci. Adv., 6 (20100), eaba2619. |
[41] | X. Sun, B. Hu, Mathematical modeling and computational prediction of cancer drug resistance, Brief Bioinf., 19 (2018), 1382-1399. |
[42] | J. Cosgrove, J. Butler, K. Alden, M. Read, V. Kumar, L. Cucurull-Sanchez, et al., Agent-based modeling in systems pharmacology, CPT: Pharmacometrics Syst. Pharmacol., 4 (2015), 615-629. |
[43] | R. L. Dedrick, D. S. Zaharko, R. A. Bender, W. A. Bleyer, R. J. Lutz, Pharmacokinetic considerations on resistance to anticancer drugs, Cancer Chemother Rep., 59 (1975), 795-804. |
[44] | H. B. Frieboes, M. E. Edgerton, J. P. Fruehauf, F. R. A. J. Rose, L. K. Worrall, R. A. Gatenby, et al., Prediction of drug response in breast cancer using integrative experimental/computational modeling, Cancer Res., 69 (2009, 4484-4492. |
[45] | B. G. Birkhead, E. M. Rankin, S. Gallivan, L. Dones, R. D. Rubens, A mathematical model of the development of drug resistance to cancer chemotherapy, Eur. J. Cancer Clin. Oncol., 23 (1987), 1421-1427. |
[46] | A. Ghaffarizadeh, S. H. Friedman, P. Macklin, BioFVM: an efficient, parallelized diffusive transport solver for 3-D biological simulations, Bioinformatics, 32 (2015), 1256-1258. |
[47] | E. F. Juarez, R. Lau, S. H. Friedman, A. Ghaffarizadeh, E. Jonckheere, D. B. Agus, et al., Quantifying differences in cell line population dynamics using CellPD, BMC Syst. Biol., 10 (2016). |
[48] | S. H. Friedman, A. R. A. Anderson, D. M. Bortz, A. G. Fletcher, H. B. Frieboes, A. Ghaffarizadeh, et al., MultiCellDS: a standard and a community for sharing multicellular data, preprint, bioRxiv 090696. |
[49] | H. C. Tang, Y. C. Chen, Insight into molecular dynamics simulation of BRAF(V600E) and potent novel inhibitors for malignant melanoma, Int. J. Nanomedicine, 10 (2015), 3131-3146. |
[50] | K. J. Mahasa, R. Ouifki, A. Eladdadi, L. de Pillis, Mathematical model of tumor-immune surveillance, J. Theor. Biol., 404 (2016), 312-330. |
[51] | T. Jackson, A. Radunskaya, Applications of Dynamical Systems in Biology and Medicine, 2015. |
[52] | A. Dhawan, T. A. Graham, A. G. Fletcher, A computational modeling approach for deriving biomarkers to predict cancer risk in premalignant disease, Cancer Prev. Res., 9 (2016), 283-295. |
[53] | X. Yang, Y. Wang, R. Byrne, G. Schneider, S. Yang, Concepts of artificial intelligence for computer-assisted drug discovery, Chem. Rev., 119 (2019), 10520-10594. |
[54] | W. Kolch, D. Fey, Personalized computational models as biomarkers, J. Pers. Med., 7 (2017), 9. |
[55] | C. L. Fischer, A. M. Bates, E. A. Lanzel, J. M. Guthmiller, G. K. Johnson, N. K. Singh, et al., Computational models accurately predict multi-cell biomarker profiles in inflammation and cancer, Sci. Rep., 9 (2019), 10877. |
[56] | F. J. Esteva, G. N. Hortobagyi, Prognostic molecular markers in early breast cancer, Breast Cancer Res., 6 (2004), 109-118. |
[57] | S. Mojarad, B. Venturini, P. Fulgenzi, R. Papaleo, M. Brisigotti, F. Monti, et al., Prediction of nodal metastasis and prognosis of breast cancer by ANN-based assessment of tumour size and p53, Ki-67 and steroid receptor expression, Anticancer Res., 33 (2013), 3925-3933. |
[58] | M. P. Menden, F. Iorio, M. Garnett, U. McDermott, C. H. Benes, P. J. Ballester, et al., Machine learning prediction of cancer cell sensitivity to drugs based on genomic and chemical properties, Plos One, 8 (2013), e61318. |
[59] | A. Bravo, J. Pinero, N. Queralt-Rosinach, M. Rautschka, L. I. Furlong, Extraction of relations between genes and diseases from text and large-scale data analysis: implications for translational research, BMC Bioinfor., 16 (2015). |
[60] | J. Kim, J-j. Kim, H. Lee, An analysis of disease-gene relationship from medline abstracts by DigSee, Sci. Rep., 7 (2017), 40154. |
[61] | P. Mamoshina, M. Volosnikova, I. V. Ozerov, E. Putin, E. Skibina, F. Cortese, et al., Machine learning on human muscle transcriptomic data for biomarker discovery and tissue-specific drug target identification, Front. Genet., 9 (2018). |
[62] | T. Zhu, S, Cao, P. C. Su, R. Patel, D. Shah, H. B. Chokshi, et al., Hit identification and optimization in virtual screening: Practical recommendations based on a critical literature analysis, J. Med. Chem., 56 (2013), 6560-6572. |
[63] | G. Klopman, S. K. Chakravarti, H, Zhu, J. M. Ivanov, R. D. Saiakhov, ESP: A method to predict toxicity and pharmacological properties of chemicals using multiple MCASE databases, J. Chem. Infor. Comput. Sci., 44 (2004), 704-715. |
[64] | I. W. Mak, N. Evaniew, M. Ghert, Lost in translation: animal models and clinical trials in cancer treatment, Am. J. Trans. Res., 6 (2014), 114-118. |
[65] | B. Ramsundar, B. Liu, Z. Wu, A. Verra, M. Tudor, R. P. Sherridan, et al., Is multitask deep learning practical for pharma?, J. Chem. Infor. Model., 57 (2017), 2068-2076. |
[66] | M. Olivecrona, T. Blashcke, O. Engvist, H. Chen, Molecular de-novo design through deep reinforcement learning, J. Cheminfor., 9 (2017). |
[67] | T. Luechtefeld, D. Marsh, C. Rowlands, T. Hartung, Machine learning of toxicological big data enables read-across structure activity relationships (RASAR) outperforming animal test reproducibility, Toxicol. Sci., 165 (2018), 198-212. |
[68] | J. L. Perez-Gracia, M. F. Sanmamed, A. Bosch, A. Patino-Garcia, K. A. Schalper, V. Segura, et al., Strategies to design clinical studies to identify predictive biomarkers in cancer research, Cancer Treat. Rev., 53 (2017), 79-97. |
[69] | E. E. Bain, L. SHafner, D. P. Walling, A. A. Othman, C. C. Stein, J. Hinkle, et al., Use of a novel artificial intelligence platform on mobile devices to assess dosing compliance in a Phase 2 clinical trial in subjects with schizophrenia, JMIR mHealth uHealth, 5 (2017), e18. |
[70] | A. H. Beck, A. R. Sangoi, S. Leung, R. K. Marinelli, T. O. Nielsen, M. J. van de Vijver, et al., Systematic analysis of breast cancer morphology uncovers stromal features associated with survival, Sci. Trans. Med., 3 (2011). |
[71] | N. L. Mani, K. A. Schalper, C. Hatzis, O. Saglam, F. Tavassoli, M. Butler, et al., Quantitative assessment of the spatial heterogeneity of tumor-infiltrating lymphocytes in breast cancer, Breast Cancer Res., 18 (2016). |
[72] | F. Lake, Artificial intelligence in drug discovery: what is new, and what is next?, Future Drug Discovery, 1 (2019). |
[73] | K. L. Fetah, B. J. DiPArdo, E. M. Kongadzem, J. S. Tomlinson, A. Elzaghied, M. Elmusrati, et al., Cancer modeling-on-a-chip with future artificial intelligence integration, Small, 15 (2019). |
[74] | V. Assadollahi, B. Rashidieh, M. Alasvand, A. Abdolahi, J. A. Lopez, Interaction and molecular dynamics simulation study of Osimertinib (AstraZeneca 9291) anticancer drug with the EGFR kinase domain in native protein and mutated L844V and C797S, J. Cell. Biochem., 120 (2019), 13046-13055. |
[75] | S. Ghafari, M. Komeilian, M. Hashemi, S. Oushani, G. Rigi, B. Rashidieh, et al., Molecular docking based screening of Listeriolysin-O for improved inhibitors, Bioinformation, 13 (2017), 160-163. |
[76] | V. Assadollahi, B. Rashidieh, Molecular dynamics simulation of EFGR L844V mutant sensitive to AZD9291 in non-small cell lung cancer, J. Thorac. Oncol., 12 (2017), 1210. |
[77] | M. M. Ranbar, V. Assadolahi, M. Yazdani, D. Nikaein, B. Rashidieh, Virtual dual inhibition of COX-2/5-LOX enzymes based on binding properties of alpha-amyrins, the anti-inflammatory compound as a promising anti-cancer drug, EXCLI J., 15 (2016), 238-245. |
[78] | B. Rashidieh, M. Valizadeh, V. Assadollahi, M. M. Ranjbar, Molecular dynamics simulation on the low sensitivity of mutants of NEDD-8 activating enzyme for MLN4924 inhibitor as a cancer drug, Am. J. Cancer Res., 5 (2015), 3400-3406. |
[79] | B. Rashidieh, Z. Madani. M. K. Azam, S. K. Maklavani, N. R. Akbari, S. Tavakoli, et al., Molecular docking based virtual screening of compounds for inhibiting sortase A in L. monocytogenes, Bioinformation, 11 (2015), 501-505. |
[80] | B. Rashidieh, S. Etemadiafshar, G. Memari, M. Mirzaeichegeni, S. Yazdi, F. Farsimadan, et al., A molecular modeling based screening for potential inhibitors to alpha hemolysin from Staphylococcus aureus, Bioinformation, 11 (2015), 373-377. |
[81] | J. Ozik, N. Collier, R. Heiland, G. An, P. Macklin, Learning-accelerated discovery of immune-tumour interactions, Mol. Syst. Des. Eng., 4 (2019), 747-760. |
[82] | J. Ozik, N. Collier, J. M. Wozniak, C. Macal, C. Cockrell, S. F. Friedman, et al., High-throughput cancer hypothesis testing with an integrated PhysiCell-EMEWS workflow, BMC Bioinfor., 19 (2018). |
1. | Cong Du, Sang-Bing Tsai, Step-Counting Function of Adolescent Physical Training APP Based on Artificial Intelligence, 2021, 2021, 1563-5147, 1, 10.1155/2021/5582598 | |
2. | Qiaoling Ma, 2021, Chapter 76, 978-3-030-74813-5, 539, 10.1007/978-3-030-74814-2_76 | |
3. | Davinder Paul Singh, , DWUT-MLP: Classification of anticancer drug response using various feature selection and classification techniques, 2022, 225, 01697439, 104562, 10.1016/j.chemolab.2022.104562 | |
4. | Mei Yang, 2021, Chapter 89, 978-3-030-79196-4, 619, 10.1007/978-3-030-79197-1_89 | |
5. | Xiaojie Min, 2021, Creativity of Zen Tao Graphic Design Based on Artificial Intelligence Technology, 9781450385046, 1265, 10.1145/3495018.3495379 | |
6. | Zhenxing Bian, 2022, Chapter 19, 978-981-16-8051-9, 173, 10.1007/978-981-16-8052-6_19 | |
7. | Davinder Paul Singh, Baijnath Kaushik, CTDN (Convolutional Temporal Based Deep- Neural Network): An Improvised Stacked Hybrid Computational Approach for Anticancer Drug Response Prediction, 2023, 14769271, 107868, 10.1016/j.compbiolchem.2023.107868 | |
8. | Simon Stephan, Stéphane Galland, Ouassila Labbani Narsis, Kenji Shoji, Sébastien Vachenc, Stéphane Gerart, Christophe Nicolle, Agent-based approaches for biological modeling in oncology: A literature review, 2024, 152, 09333657, 102884, 10.1016/j.artmed.2024.102884 | |
9. | Rosalvo Ferreira de Oliveira Neto, Sérgio Ruschi Bergamachi Silva, Cintia Emi Yanaguibashi Leal, Edilson Beserra de Alencar Filho, Machine learning based QSAR and Molecular Dynamics simulations in the structural design and mechanism of action of imidazole derivatives with anti-melanoma activity, 2025, 61, 2175-9790, 10.1590/s2175-97902025e24510 |
Resource | Description | Application |
PhysiCell | Agent-based (lattice-free) multicellular simulation platform designed for use on standard computers with computational cost related linearly to the number of cells being modelled [12]. | Understanding the contribution of cell growth and interaction to tissue-scale changes. Encompasses cellular processes including division, apoptosis, and necrosis, in the context of heterogeneous cell populations and dynamic microenvironments [80,81]. |
BioFVM (Finite Volume Method for Biological Problems) | Widely accessible code for modelling three-dimensional transport system in a biological context using partial differential equations [46]. | The substrate surrounding cells varies widely and is subject to various processes including diffusion, secretion, uptake, and decay as cellular phenotypes changes in response to the environment. BioVFM allows for multicellular modelling with consideration of three-dimensional substrate changes to better understand the interactions of cells with their immediate surroundings, with implications for signalling molecules and drugs within the substrate. |
CellPD: Cell Phenotype Digitizer | An accessible, user-friendly program designed to quantify features of a cell’s phenotype. Designed with consideration for experimental variability and irregularities [47]. | The complexity of biological systems can be increasingly characterised with the quantification of various cellular parameters, such as proliferation and death. This allows for measuring cellular changes in particular conditions over multiple time points whilst removing the variability associated with laboratory-based experiments. This also provides the opportunity to quantitatively assess phenotypic changes with environmental disturbances, such as the introduction of a novel drug. |
multicellDS (Multicellular Data Standard) | A platform designed to curate public, standardised datasets to maximise the output of experimental and clinical information. Facilitates model refinement and streamlining datasets from high-throughput studies with an array of data processing techniques [48]. | Collaborative, data-sharing efforts may introduce new insights and strengthen our ability to generate and test novel hypotheses. The fusion of data from different groups and research focuses can only help in deepening our understanding of the cellular biology underlying the complexities of cancer. |
Resource | Description | Application |
PhysiCell | Agent-based (lattice-free) multicellular simulation platform designed for use on standard computers with computational cost related linearly to the number of cells being modelled [12]. | Understanding the contribution of cell growth and interaction to tissue-scale changes. Encompasses cellular processes including division, apoptosis, and necrosis, in the context of heterogeneous cell populations and dynamic microenvironments [80,81]. |
BioFVM (Finite Volume Method for Biological Problems) | Widely accessible code for modelling three-dimensional transport system in a biological context using partial differential equations [46]. | The substrate surrounding cells varies widely and is subject to various processes including diffusion, secretion, uptake, and decay as cellular phenotypes changes in response to the environment. BioVFM allows for multicellular modelling with consideration of three-dimensional substrate changes to better understand the interactions of cells with their immediate surroundings, with implications for signalling molecules and drugs within the substrate. |
CellPD: Cell Phenotype Digitizer | An accessible, user-friendly program designed to quantify features of a cell’s phenotype. Designed with consideration for experimental variability and irregularities [47]. | The complexity of biological systems can be increasingly characterised with the quantification of various cellular parameters, such as proliferation and death. This allows for measuring cellular changes in particular conditions over multiple time points whilst removing the variability associated with laboratory-based experiments. This also provides the opportunity to quantitatively assess phenotypic changes with environmental disturbances, such as the introduction of a novel drug. |
multicellDS (Multicellular Data Standard) | A platform designed to curate public, standardised datasets to maximise the output of experimental and clinical information. Facilitates model refinement and streamlining datasets from high-throughput studies with an array of data processing techniques [48]. | Collaborative, data-sharing efforts may introduce new insights and strengthen our ability to generate and test novel hypotheses. The fusion of data from different groups and research focuses can only help in deepening our understanding of the cellular biology underlying the complexities of cancer. |