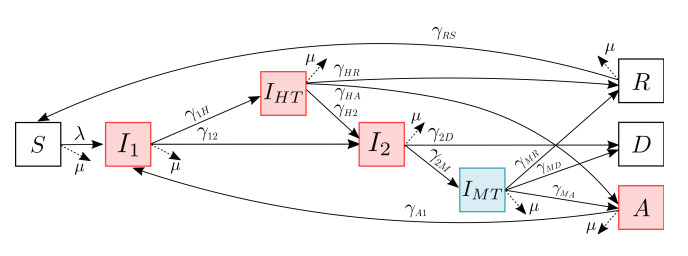
Citation: Vinh Huy Chau. Powerlifting score prediction using a machine learning method[J]. Mathematical Biosciences and Engineering, 2021, 18(2): 1040-1050. doi: 10.3934/mbe.2021056
[1] | Darja Kalajdzievska, Michael Yi Li . Modeling the effects of carriers on transmission dynamics of infectious diseases. Mathematical Biosciences and Engineering, 2011, 8(3): 711-722. doi: 10.3934/mbe.2011.8.711 |
[2] | Martin Luther Mann Manyombe, Joseph Mbang, Jean Lubuma, Berge Tsanou . Global dynamics of a vaccination model for infectious diseases with asymptomatic carriers. Mathematical Biosciences and Engineering, 2016, 13(4): 813-840. doi: 10.3934/mbe.2016019 |
[3] | Yijun Lou, Li Liu, Daozhou Gao . Modeling co-infection of Ixodes tick-borne pathogens. Mathematical Biosciences and Engineering, 2017, 14(5&6): 1301-1316. doi: 10.3934/mbe.2017067 |
[4] |
Shuoer Wang, Yidi Sun, Songjiao Zhao, Feng Wei, Gong Yang .
Breast conserving surgery (BCS) with adjuvant radiation therapy showed improved prognosis compared with mastectomy for early staged triple negative breast cancer patients . Mathematical Biosciences and Engineering, 2020, 17(1): 92-104. doi: 10.3934/mbe.2020005 |
[5] | Yanfeng Liang, David Greenhalgh . Estimation of the expected number of cases of microcephaly in Brazil as a result of Zika. Mathematical Biosciences and Engineering, 2019, 16(6): 8217-8242. doi: 10.3934/mbe.2019416 |
[6] | Kai Wang, Zhidong Teng, Xueliang Zhang . Dynamical behaviors of an Echinococcosis epidemic model with distributed delays. Mathematical Biosciences and Engineering, 2017, 14(5&6): 1425-1445. doi: 10.3934/mbe.2017074 |
[7] | Biao Tang, Weike Zhou, Yanni Xiao, Jianhong Wu . Implication of sexual transmission of Zika on dengue and Zika outbreaks. Mathematical Biosciences and Engineering, 2019, 16(5): 5092-5113. doi: 10.3934/mbe.2019256 |
[8] | Chunyang Qin, Yuming Chen, Xia Wang . Global dynamics of a delayed diffusive virus infection model with cell-mediated immunity and cell-to-cell transmission. Mathematical Biosciences and Engineering, 2020, 17(5): 4678-4705. doi: 10.3934/mbe.2020257 |
[9] | Eugene Kashdan, Svetlana Bunimovich-Mendrazitsky . Hybrid discrete-continuous model of invasive bladder cancer. Mathematical Biosciences and Engineering, 2013, 10(3): 729-742. doi: 10.3934/mbe.2013.10.729 |
[10] | Zongwei Ma, Hongying Shu . Viral infection dynamics in a spatial heterogeneous environment with cell-free and cell-to-cell transmissions. Mathematical Biosciences and Engineering, 2020, 17(3): 2569-2591. doi: 10.3934/mbe.2020141 |
Typhoidal Salmonella and nontyphoidal Salmonella (NTS) are intracellular bacteria that cause significant global morbidity and mortality [1]. In industrialized countries, NTS is normally associated with zoonotic reservoirs and is transferred between humans and other animals through the fecal-oral route. Most infected individuals experience mild gastrointestinal illness, such as nausea, vomiting, profuse watery diarrhea, and abdominal pain. The infections are usually self-limiting, and antimicrobial treatment is not recommended for uncomplicated illness.
In Sub-Saharan Africa, in contrast to the rest of the world, a strain of NTS (ST-313) appears to be human-adapted, becoming a predominant cause of invasive nontyphoidal Salmonella (iNTS) bloodstream infections [2,3]. The clinical features of iNTS disease are diverse and non-specific, and diarrhea is often absent. The symptoms range from hepatosplenomegaly to respiratory symptoms. These symptoms overlap with pneumonia and malaria clinical presentations in Sub-Saharan Africa, and iNTS is often misdiagnosed. Empirical diagnosis often fails to identify and treat iNTS disease [4]. Even when the microbiologically confirmed cases are treated with appropriate antimicrobial drugs, there is a high case fatality of 22-47% [2,5,6].
The emergence of iNTS ST-313 in sub-Saharan Africa is thought to be due to the large proportion of the African population with some degree of immune suppression or impairment caused by malnutrition or comorbidity with untreated HIV and other infections [7,8]. The risk factors in children include HIV infection, malaria, and malnutrition, and the main risk factor in adults is the advanced HIV infection. Among the HIV-infected adults, before the antiretroviral therapy era, 20-40% of survivors had recurrence; despite the treatment, up to 25% of patients had several recurrences [5,9].
There is some evidence showingthat iNTSmay have evolved to transmitfrom person to person [10,11,12]. Moreover, asymptomatic carriers can shed NTS into the environment and infect other children in nearby homes. These asymptomatic carriers create a reservoir of iNTS infections for community-acquired iNTS bacteremia in children [13,14].
Multidrug-resistant iNTS is a challenge for the local healthcare system and can be a major factor in the high prevalence [12]. Salmonella was once susceptible to a broad range of affordable and effective antimicrobial drugs. Recently, multidrug-resistant strains have emerged, and a large proportion of the infection are resistant to three or more commonly available antibiotics.
Mathematical models are tools to help understand the dynamics of epidemics and guide the mitigation efforts [15,16]. Both compartmental differential equation models and stochastic individual-based models have been developed to clarify the zoonotic Salmonella transmission dynamics. These models can inform public health workers and help eradicate the infection from a population. For example, in [17], a compartmental model simulates a Salmonella Typhimurium infection in swine in Great Britain. In this model, the infected pigs are grouped into susceptible, latent, infectious (shedder), and carrier stage compartments. In [18,19], Susceptible-Infectious-Recovered (SIRS) type compartmental models were used to simulate the transmission dynamics of Salmonella infection in dairy herds. In [20], a multidisciplinary approach involves quantitative PCR, and probabilistic models are proposed to study the spatial and stochastic nature of within-in host dynamics for Salmonella Typhimurium infection.
Although there are many Salmonella models in animals, there are very few models for iNTS within human populations in the developing world, such as Sub-Saharan Africa. In [21], a Susceptible-Infectious-Recovered type differential equation model is proposed as the first model for iNTS dynamics in this high-risk population, with the focus on the cost-benefit analysis for different scenarios of diagnostic deployment and their impact on antimicrobial treatment for the patients.
We propose a staged progression ODE model to help understand the transmission dynamics of iNTS and inform effective public health control policy. We formulate the model for an immunocompromised population in Sub-Saharan Africa and account for different risk factors, including untreated HIV/AIDS infections. The model accounts for multiple infection stages, including asymptomatic carriers.
After describing the proposed model (Section 2), we derive the disease basic reproduction number and analyze how much each infection stage contributes to the spread of the iNTS (Section 3). We then simulate a baseline scenario in Siaya County, Kenya (Section 4.1), characterize the impact of the different risk factors towards the local epidemic through sensitivity analysis, and inform potential mitigation strategies (Section 4.2).
Our staged progression compartmental model (Figure 1) accounts for two infection stages and two treatment stages with different outcome statuses. The susceptible population, S, is infected at a rate of infection λ and progresses to an early-stage infection, I1, with mild symptoms. Some of the infected individuals receive home treatment, entering stage IHT (at rate γ1H). The outcome of home treatment include recovered R (at rate γHR), asymptomatic A (at rate γHA), or severe infection status I2 (at rate γH2) with acute/serious symptoms. The infected people not receiving home treatment enter severe infection I2 directly at rate γ12.
Some of the severely sick population I2 are treated in a medical facility and enter the compartment IMT at the rate γ2M. They then either recover and enter R at the rate γMR, die and enter D at rate γMD, or become an asymptomatic carrier A at rate γMA. We assume that those with severe symptoms who do not receive medical treatment will suffer mortality at the rate γ2D. The recovered population, R, obtains some temporary immunity from the infection and becomes susceptible S again at the rate γRS.
Home treatment IHT is common in Sub-Saharan Africa, and antibiotics are easily available over the counter without a prescription for self-treatment. Inappropriate use of these drugs may contribute to the emergence of multidrug-resistant phenotypes of iNTS [13]. Also, less expensive generic drugs of variable quality for treating bacterial infections could also be contributing to the increasing resistance. Although we do not explicitly model the emergence of resistant strains in the current model, we will be considering these factors in future versions of the model.
In Sub-Saharan Africa, iNTS infection rates peak first in young children ≤5 years old and then again in adults 25-40 years old [22]. The first peak is related to malaria, malnutrition, and HIV for young children, while the second peak is due to advanced HIV infection in the adults.
We account for the immunocompromised adults with advanced HIV infection who become asymptomatic carriers A. These asymptomatic carriers feel well but are still transmitting the pathogen. They are often receiving effective home treatment in early-stage infection IHT or medical treatment in severe infection IMT, and they still retain a relatively low pathogen load after the treatment. If these infected carriers are on Antiretroviral Therapy (ART) HIV treatment, then their immune system is usually strong enough to fight off the iNTS bacteria. However, if they are not on ART, then eventually the bacterial load increases until it breaks down their immune system, and they become sick again (at rate γA1). Although these asymptomatic carriers feel well, they are still infectious and constantly shedding the disease into the environment without being detected. Thus, these asymptomatic carriers act as a reservoir for the iNTS emergence and transmission [8].
Because the iNTS prevalence is highest in age groups ≤5 and 25-40 years old, we explicitly account for these age groups in the model. The older age group also can capture the impact of asymptomatic HIV-infected adults. We divide the population into the following groups, denoted by upper index κ: HIV- adults (κ=1), HIV+ with ART adults (κ=2), HIV+ not treated adults (κ=3), and young children ≤5 years old (κ=4). For each group κ, we model the disease dynamics using the same framework shown in Figure 1 but with different progression rates between the compartments. For simplicity of notation, we omit the group index κ when the formulas are the same for all the groups unless there could be some ambiguity in the notation. A description of all the state variables is in Table 1.
Compartment | Description |
Sκ | Susceptible |
Iκ1 | Infected people with mild symptoms |
IκHT | Infected people who do home treatment |
Iκ2 | Infected people with severe symptoms |
IκMT | Infected people who are admitted to medical facilities |
Rκ | People recover from the infection (with temporary immunity) |
Aκ | People with asymptomatic carriage of the infection (go back home) |
Dκ | People die from the infection |
Group index κ | |
κ=1 | HIV- adults, 25—40 years old |
κ=2 | HIV+ adults, on ART, 25—40 years old |
κ=3 | HIV+ adults, not on ART, 25—40 years old |
κ=4 | Children, ≤ 5 years old |
The rates that people advance between the compartments in Figure 1 depend on the average time people spend in each compartment and the branching probabilities which are distributed across all possible downstream compartments (buted across all possible down).
For each group κ, we first define the branching probabilities, Pκij, as the fraction of people who progress from state i to state j. These exit probabilities sum to unity (Eq (2.1)) and, even though this constraint could reduce the number of variables, we keep all of them in the equations to simplify the notation. Then, we define τκi as the average time spent in a state i. Assuming an exponential distribution for the time spent in a stage i, we can derive the progression rates γκ$ij$ in terms of the ratios of the branching probabilities and time spent in the upstream stage τκi, as shown in Figure 1.
We find that this approach is more intuitive and less prone to errors than defining the progression rates directly, especially when having multiple pathways from one compartment to another.
PS1+PSN=1,P12+P1H+P1N=1,P2D+P2M+P2N=1,PA1+PAN=1,PMR+PMD+PMA+PMN=1,PHR+PHA+PH2+PHN=1,PRS+PRN=1. | (2.1) |
γ1H=P1H/τ1,γHR=PHR/τH,γMR=PMR/τM,γ12=P12/τ1,γHA=PHA/τH,γMD=PMD/τM,γ2D=P2D/τ2,γH2=PH2/τH,γMA=PMA/τM,γ2M=P2M/τ2,γRS=PRS/τR,γA1=PA1/τA, | (2.2) |
μ=P1N/τ1=PHN/τH=P2N/τ2=PMN/τM=PAN/τA=PRN/τR | (2.3) |
We model the force of infection rates for all the susceptible groups, λκ, as the summation of the sources of infection from each infectious stage among all the groups:
λκ=cκβκ∑4ℓ=1cℓ(Iℓ1+IℓHT+Iℓ2+Aℓ)∑4ℓ=1cℓ(Sℓ+Iℓ1+IℓHT+Iℓ2+Aℓ+Rℓ),κ=1,…4, | (2.4) |
where cκ is the number of contacts that an individual in group κ has per day. We assume a homogenously mixing population and that the proportion of the contacts with an infectious person is approximated by the last term in the Eq (2.4). This proportion is defined by dividing the total number of contacts from the infectious population per day (the numerator) by the total number of contacts from all the active population per day (the denominator). We have assumed that the infectious people do not change their behavior until being admitted to the medical facility (stage IMT). Once in a medical facility, then we assume they no longer have contacts with the general population and are not part of force of infection.
For simplicity and the lack of data, in our study, we assume that contacts in different groups are equal, c=cκ for κ=1,…4. Under this assumption, the force of infection in Eq (2.4) can be reduced to
λκ=cβκ∑4ℓ=1Iℓ1+IℓHT+Iℓ2+Aℓ∑4ℓ=1Sℓ+Iℓ1+IℓHT+Iℓ2+Aℓ+Rℓ,κ=1,…4. |
We assume that the transmissibility per contact βκ for each subpopulation is approximately constant through different infection stages. For different groups κ=1,⋯,4, the transmissibility βκ varies. This difference reflects the average level of immune-competency of susceptible adults or children in the corresponding group.
Following the analysis in Feasey et al. [23], within the young child group (κ=4), since the risk factors, such as malaria and HIV, are at endemic state in the studying region, we formulate the averaged susceptibilities as the contribution of prevalences of these factors,
β4=β40(0.56σMA+0.25σCHIV+0.2σMN), |
where σMA and σMN are the prevalence of malaria and malnutrition, respectively, in children under age five. The coefficient σCHIV is the HIV prevalence in the child group, which depends on the fraction of non-ART-treated HIV adults (1−σART) and the maternal transmission rate (σMAT),
σCHIV=σAHIV(1−σART)σMAT. |
This approach results in an estimated prevalence of 2.4% in children in the baseline scenario. There is very little correlation between iNTS incidence and ART treatment in children [7,24], and it is neglected in the model.
The resulting model for iNTS transmission among high-risk cohorts in Sub-Saharan Africa is expressed as a system of differential equations for each population group κ,
˙Sκ=μκ(Sκ0−Sκ)−λκSκ+γκRSRκ,˙Iκ1=λκSκ+γκA1Aκ−(γκ1H+γκ12)Iκ1−μκIκ1,˙IκHT=γκ1HIκ1−(γκHR+γκHA+γκH2)IκHT−μκIκHT,˙Iκ2=γκH2IκHT+γκ12Iκ1−(γκ2D+γκ2M)Iκ2−μκIκ2,κ=1,…4.˙IκMT=γκ2MIκ2−(γκMR+γκMD+γκMA)IκMT−μκIκMT,˙Aκ=γκHAIκHT+γκMAIκMT−γκA1Aκ−μκAκ,˙Rκ=γκHRIκHT+γκMRIκMT−γκRSRκ−μκRκ,˙Dκ=γκ2DIκ2+γκMDIκMT, | (2.5) |
all the progression rates are defined in Eqs (2.2) and (2.3), and all the model parameters are summarized in Table 2.
Description | HIV- Adults (κ=1) | HIV+ ART (κ=2) | HIV+ no ART (κ=3) | Children (κ=4) | Reference | |
cβκ | Transmission rate | 4.6×10−3 | 0.092 | 0.257 | 0.232 | Calibrated |
μκ | Migration rate (mortality+aging) per day | 2.0×10−4 | 3.7×10−4 | 9.2×10−4 | 5.8×10−4 | Derived |
- Mortality rate per 1000 people per year | 5.02 | 70 | 270 | 10.4 | [25,26] | |
- Aging rate per year | 1/15 | 1/15 | 1/15 | 1/5 | Derived | |
Sκ0 | Population size (Siaya County) | 106202 | 19171 | 10784 | 141752 | [27] |
- | Incidence, per 100, 000 population per year | 37 | 739 | 2070 | 1870 | [28,29] |
σMA | Prevalence of malaria epidemic in children | - | - | - | 2% | [30] |
σMN | Prevalence of malnutrition in children | - | - | - | 40% | [26] |
σHIV | Prevalence of HIV epidemic | σAHIV=22% | σAHIV=22% | σAHIV=22% | σCHIV=2% | EO∗ |
σART | Coverage of ART for HIV adults | 64% | - | EO∗ | ||
σMAT | Maternal trans. rate for non-ART adults | - | - | 30% | - | [31] |
Without the effect of migration, the raw estimates for disease parameters... | ||||||
P01H | Fraction of I1 do self-treatment | 20% | 20% | 20% | 50% | EO∗ |
P012 | Fraction of I1 do not do self-treatment | 80% | 80% | 80% | 50% | EO∗ |
P0HR | Fraction of IHT recover | 50% | 40% | 10% | 50% | EO∗ |
P0HA | Fraction of IHT become asymp. | - | 4% [32] | 35%* | - | EO∗ |
P0H2 | Fraction of IHT become I2 | 50% | 56% | 55% | 50% | EO∗ |
P02M | Fraction of I2 do medical-treatment | 90% | 90% | 90% | 90% | EO∗ |
P02D | Fraction of I2 do not get medical-treatment | 10% | 10% | 10% | 10% | EO∗ |
P0MD | Fraction of IMT die | 11% [33] | 11% [33] | 47% [6] | 22% [34] | EO∗ |
P0MA | Fraction of IMT become asymp. | - | 4% | 35%* | - | EO∗ |
P0MR | Fraction of IMT recover | 89% | 85% | 18% | 78% | EO∗ |
τ01 | Time spent in I1 (infected → lightly sick) | 1 d | 1 d | 1 d | 1 d | EO∗ |
τ0H | Time spent in self-treatment | 3 d | 3 d | 3 d | 3 d | EO∗ |
τ02 | Time spent in I2 (lightly sick → heavily sick) | 4 d | 4 d | 4 d | 4 d | EO∗ |
τ0M | Time spent in medical-treatment | 7 d | 7 d | 7 d | 7 d | EO∗ |
τ0R | Time of immunity period after recovery | 1 yr | 1 yr | 1 yr | 2 m | EO∗ |
τ0A | Time of being asymptomatic | - | 59 d [32] | 45 d [32] (60 d [35]) |
- | EO∗ |
The adjusted parameter values for migration... | ||||||
τ∗ | Time spent in stage ∗ | (1/τ0∗+μκ)−1 | Derived | |||
P∗N | Fraction of migration at stage ∗ | μκ×τ∗ | Derived | |||
Pij | Fraction of people at stage i move to stage j | P0ij∗(1−P∗N) | Derived |
When there is no iNTS epidemic present in the population, the susceptible population Sκ is balanced at a steady state level Sκ0 through a constant birth and natural removing rate μκ. We consider the migration (removal) rate μκ in the equations to account for non-iNTS mortality and the natural aging out of the age cohort.
We use the next generation matrix approach to define the basic reproduction number R0 for the system Eq (2.5). The resulting formula can be expressed as a weighted sum of the subetaoup basic reproduction numbers, Rκ0,κ=1,…,4. The subetaoup basic reproduction numbers, Rκ0, must account for recurring infections, and we are able to explain the formula in terms of the sum of terms in an infinite recurrence relationship.
Following the next generation matrix approach, we consider the equations in Eq (2.5) that are associated with the infected groups: X=(X1,X2,X3,X4)T, where Xκ=(Iκ1,IκHT,Iκ2,IκMT,Aκ). Next, the right-hand sides of equations for the infected groups are split into the infection part, Fκ, and transition part Vκ:
ddt(Iκ1IκHTIκ2IκMTAκ)=(cβκ4∑ℓ=1Iℓ1+IℓHT+Iℓ2+Aℓ4∑ℓ=1Sℓ+Iℓ1+IℓHT+Iℓ2+Aℓ+RℓSκ0000)−((γκ1H+γκ12)Iκ1−γκA1Aκ+μκIκ1(γκHR+γκHA+γκH2)IκHT−γκ1HIκ1+μκIκHT(γκ2D+γκ2M)Iκ2−γκH2IκHT−γκ12Iκ1+μκIκ2(γκMR+γκMD+γκMA)IκMT−γκ2MIκ2+μκIκMTγκA1Aκ−γκHAIκHT−γκMAIκMT+μκAκ)=:Fκ−Vκ,κ=1,…,4. |
These equations are then linearized at the disease-free equilibrium (DFE) Xκ0=(Sκ0,0,0,0,0,0,0),κ=1,2,3,4. That is, we define Jacobian matrices of F:=(F1;F2;F3;F4) and V:=(V1;V2;V3;V4) evaluate them at the DFE to obtain
JF:=∂F∂X=(D1FD1FD1FD1FD2FD2FD2FD2FD3FD3FD3FD3FD4FD4FD4FD4F)andJV:=∂V∂X=(D1VD2VD3VD4V), |
where
DκF=(φκφκφκ0φκ00000000000000000000),φκ=cβκSκ0N0,κ=1,⋯,4,N0=4∑κ=1Sκ0, |
and
DκV=(γκ12+γκ1H+μκ000−γκA1−γκ1HγκH2+γκHA+γκHR+μκ000−γκ12−γκH2γκ2D+γκ2M+μκ0000−γκ2MγκMA+γκMD+γκMR+μκ00−γκHA0−γκMAγκA1+μκ). |
The basic reproduction number is calculated as the spectral radius of the next generation matrix JFJ−1V. Note that the matrix JV is defined in terms of the transition rates γ∗, and the next generation matrix is defined in terms of its inverse, J−1V. Thus, the resulting formulas for R0 are better understood in terms of τ∗ and P∗ as defined in Eqs (2.1) to (2.3).
After long algebraic manipulations, we obtain
R0:=Spectral Radius of (JFJ−1V)=4∑κ=1Sκ0N0Rκ0, | (3.1) |
where
Rκ0=cβκτκ1+Pκ1HτκH+(Pκ12+Pκ1HPκH2)τκ2+(Pκ1HPκHA+Pκ1HPκH2Pκ2MPκMA+Pκ12Pκ2MPκMA)τκA1−PκA1(Pκ1HPκHA+Pκ1HPκH2Pκ2MPκMA+Pκ12Pκ2MPκMA). | (3.2) |
The obtained basic reproduction number R0 Eq (3.1) for the entire population is a weighted average of the contributions from each population group (Rκ0) with the weights being the fractions of the population in the corresponding group. Since we partition the population into groups of subpopulation with different transmission parameters, our model structure is comparable to the differential infectivity model studied in [40], where the basic reproduction number has a similar pattern of weighted average.
We have written the basic reproduction number Rκ0 in terms of branching probabilities rather than progression rates, and this allows us to give more intuitive interpretations of the quantity. The factor cβκ in the reproduction number represents the number of contacts per day times the probability of infection per contact with someone in age-group κ. Together with the terms in the numerator of Eq (3.2), it gives the total contribution of infection from different infectious stages (detailed later).
The denominator in the formula Eq (3.2) for Rκ0, however, can look perplexing at first. This can be interpreted as a scaling factor to account for the possibility that the asymptomatic individuals can have recurrent infection (A→I1) and continue shedding to the population.
From Eq (3.2), we recognize that the coefficient factors for τκ∗ represent the probabilities of transitions between the initial infected stage Iκ1 and the corresponding infectious stage, that is
Rκ0=cβκτκ1+Prob(Iκ1→IκHT)τκH+Prob(Iκ1→Iκ2)τκ2+Prob(Iκ1→Aκ)τκA1−Prob(Iκ1→Aκ)PκA1, | (3.3) |
where
Prob(Iκ1→IκHT)=Pκ1H,Prob(Iκ1→Iκ2)=Pκ12+Pκ1HPκH2,Prob(Iκ1→Aκ)={Route #1Iκ1→IκHT→Aκ,Pκ1HPκHA,Route #2Iκ1→IκHT→Iκ2→IκMT→Aκ,Pκ1HPκH2Pκ2MPκMA,Route #3Iκ1→Iκ2→IκMT→Aκ,Pκ12Pκ2MPκMA,=Pκ1HPκHA+Pκ1HPκH2Pκ2MPκMA+Pκ12Pκ2MPκMA. | (3.4) |
When being infectious, one first spends τκ1 amount of time in stage Iκ1, then, with probability Prob(Iκ1→IκHT), one progresses to IκHT stage and spend τκH amount of time there. Similarly, with probability Prob(Iκ1→Iκ2) and Prob(Iκ1→Aκ), one progresses to stages Iκ2 and Aκ and spends τκ2 and τκA amount of time, respectively. For convenience, we define the expected duration of time one spends in a stage Δ as
ˉτκΔ=Prob(Iκ1→Δ)τκΔ,Δ∈{Iκ1,IκHT,Iκ2,IκMT,Rκ,Aκ}, | (3.5) |
where the probabilities Prob(Iκ1→IκHT), Prob(Iκ1→Iκ2), and Prob(Iκ1→Aκ) are defined in Eq (3.4), and
Prob(Iκ1→Iκ1)=1,Prob(Iκ1→IκMT)=Prob(Iκ1→Iκ2)Pκ2M=(Pκ12+Pκ1HPκH2)Pκ2M,Prob(Iκ1→Rκ)=Pκ1HPκHR+Pκ1HPκH2Pκ2MPκMR+Pκ12Pκ2MPκMR. | (3.6) |
Then the average infectious time in one disease cycle, ˉτκI, is
ˉτκI:=ˉτκ1+ˉτκH+ˉτκ2+ˉτκA. | (3.7) |
Thus, the average number of new secondary cases that an infectious individual could produce in one disease cycle is the summation of all the new cases in four infectious compartments
(Rκ0)cycle:=cβκˉτκI. |
Since the basic reproduction number accounts for all the secondary infections that happened during the entire infection duration, we need to include the recurrent infection when an asymptomatic person Aκ becomes Iκ1 again. Note that this is different from being reinfected after recovery (Rκ→Iκ1), which is considered as a separate infectious period. The probability of having a recurrent infection is
Pκγ=Prob(I cycle 11→A)⋅Prob(A→I cycle 21)=Prob(Iκ1→Aκ)⋅PκA1. | (3.8) |
Then, with probability Pκγ, the infected individual would have another infectious onset and create another (Rκ0)cycle number of new infections in the second cycle. This process may repeat again and again with diminishing probability, and the R0 of the entire process is the summation of the resulting infinite geometric series,
Rκ0=(Rκ0)cycle⏟1st cycle of infection+Pκγ(Rκ0)cycle⏟2nd cycle+⋯+(Pκγ)n−1(Rκ0)cycle⏟nth cycle+⋯=(Rκ0)cycle1−Pκγ=Eq(3.3). |
This recovers the basic reproduction number obtained through the next generation matrix approach in Eq (3.3).
Setting the right-hand side of the system Eq (2.5) and substituting all the rates by probabilities as defined in Eqs (2.1) to (2.3), we obtain the endemic steady state for each subpopulation group:
(Iκ1)EE=μκ(Sκ0−SκEE)1−Pκθˉτκ1,(IκHT)EE=μκ(Sκ0−SκEE)1−PκθˉτκH,(Iκ2)EE=μκ(Sκ0−SκEE)1−Pκθˉτκ2,(IκMT)EE=μκ(Sκ0−SκEE)1−PκθˉτκM,(Aκ)EE=μκ(Sκ0−SκEE)1−PκθˉτκA,(Rκ)EE=μκ(Sκ0−SκEE)1−PκθˉτκR, | (3.9) |
where ˉτκΔ's are defined in Eq (3.5) and
Pκθ=Prob(Iκ1→Aκ)PκA1+Prob(Iκ1→Rκ)PκRS |
is the probability of an infected individual to survive the infection and becomes either asymptomatic (first term) or recovered (second term), and the susceptible subpopulation at endemic state, (S1EE,S2EE,S3EE,S4EE), is the non-trivial and positive solution to the following system
ai(Si0−SiEE)Ri0SiEE=aj(Sj0−SjEE)Rj0SjEE,1≤i≠j≤4,4∑κ=1SκEE(1−Rκ0−dκ)+dκSκ0=0, | (3.10) |
where the coefficients
aκ=ˉτκIμκ1−Pκθ,dκ=(ˉτκI+ˉτκR)μκ1−Pκθ,κ=1,⋯,4, |
the coefficient Rκ0 is defined in Eq (3.2), and Sκ0 is the DFE.
By using a combination of probabilities rather than rates, we interpret the obtained endemic steady states: At the endemic steady state, the migration-in rate, μκ(Sκ0−SκEE), needs to balance out the migration-out rate, which is a product of the total infected population, IκEE, and averaged death rate, (1−Pκθ)/(ˉτκI+ˉτκM+ˉτκR). Thus, at the steady state, we obtain the balanced equation
μκ(Sκ0−SκEE)=1−PκθˉτκI+ˉτκM+ˉτκRIκEE, |
and the total balanced infected population (people who has been infected) is
IκEE=μκ(Sκ0−SκEE)1−Pκθ(ˉτκI+ˉτκM+ˉτκR). |
This population IκEE is then distributed to different infected compartments, proportional to the expected infection time span spent (ˉτκΔ) in that compartment as defined in Eq (3.5).
The disease transmission rates, cβκ, are difficult to quantify directly and need to be estimated based on more observable quantities. We calibrate the model using the incidence information in Table 2, which describes the situation in Siaya County, Kenya.
The migration rate, μκ (per capita per day), includes both the baseline (non-iNTS related) death rate and the aging rate, and we assume there is no significant spatial migration in/out of the studied region in this age range [41]. For example, for the children under age 5 (κ=4), μ4=(10.4/1000+1/5)/365≈5.8×10−4.
To estimate the transmission rate, cβκ, we match the incidences at the endemic state. To this end, we setup a nonlinear system,
(λκ)EE=cβκ4∑κ=1(Iκ1)EE+(Iκ2)EE+(IκHT)EE+(Aκ)EE4∑κ=1SκEE+(Iκ1)EE+(Iκ2)EE+(IκHT)EE+(Aκ)EE+(Rκ)EE=cβκ4∑κ=1aκ(S0−SκEE)4∑κ=1SκEE+dκ(S0−SκEE)=incidence for group κ365×100,000,κ=1,⋯,4, |
and the four equations from the system Eq (3.10), and solve for the eight unknowns: the transmission rates cβκ (product as one variable) and endemic states for susceptible SκEE, κ=1,⋯,4.
In 2002-2003, an emerging iNTS epidemic took about three years to peak in a naive West African population [34,42]. Although the reported estimates for the iNTS disease burden are known to be gross underestimates of the actual incidence, they probably capture the underlying trend of the epidemic. We assumed that approximately 20% of the cases were reported. We considered other underreporting factors to verify that the qualitative aspects of predictions were fairly insensitive to this assumption.
We initialized the system of equations by infecting one person and letting the infection spread until 0.1% of the population was infected. We then used this balanced distribution of infected and recovered populations for the t=0 initial conditions for the model. This balanced initial condition gives a naturally distributed infection across different compartments as if the epidemic had emerged from a single infection. The initialization also avoids nonphysical oscillations that can occur when the population is not realistically distributed among the different compartments in the initial conditions.
To study the population cohort that are most impacted by the pathogen, we simulate the infection and death-count curves for different groups in one epidemic. At the baseline scenario, the child group (κ=4) suffers the most from the epidemic as it has the most infection cases throughout the course of the epidemic (Figure 3 top left) and the most accumulative deaths (top right) among all the population cohorts.
On the other hand, the HIV adult without ART group (κ=3) has the highest per capita infection rate (bottom left), which is about four times higher than the rate in children. The incidence rates are comparable between the HIV non-ART adults and child groups (bottom right), which suggests that HIV no-ART adults have a longer infection course than the children have. In fact, we can estimate the average lengths of infection periods, ˉτκI+ˉτκM (defined in Eqs (3.5) and (3.7)), for HIV no-ART adults and children, which are about 26 days and 10 days, respectively. The major difference is due to the substantial period that HIV no-ART adults spend in the asymptomatic stage, which is about 15 days on average.
We then study the pathogen shedding at different stages to understand the major source of infection. Among all the population cohorts, the child group has the most infectious counts in all its stages (Figure 4 left). However, when we also consider the infectious time spent at different stages, then the asymptomatic HIV+ adults without ART gives the most time-weighted infectious counts(Figure 4 right).
The critical role that HIV+ adults without ART play can also be seen in the basic reproduction numbers for different subetaoups (Table 3). We see that among all the population cohorts (column 2 of Table 3), HIV+ no ART adults contribute the most in terms of Rκ0. Furthermore, if breaking this contribution to different infectious stages (row 4 of Table 3), the asymptomatic stage of the HIV+ no ART adult group (column 6) has the largest value (6.31). This indicates that these asymptomatic untreated HIV adults serve as the main driving force of the iNTS epidemic.
Rκ0 | I1 | IHT | I2 | A | |
HIV- adults (κ=1) | 0.03 | 5×10−3 | 3×10−3 | 0.02 | - |
HIV+ART adults (κ=2) | 0.77 | 0.10 | 0.06 | 0.37 | 0.24 |
HIV+ no ART adults (κ=3) | 8.47 | 0.41 | 0.25 | 1.50 | 6.31 |
Children (κ=4) | 1.35 | 0.25 | 0.37 | 0.74 | - |
We are interested in understanding how the risk factors could affect the epidemic course and exploring potential mitigation strategies for iNTS disease control. The sensitivity analysis identifies impactful model parameters and quantifies the relative changes in the quantities of interest (QOIs) with respect to the perturbation on the model parameters of interest (POIs) [43]. We define the normalized sensitivity index of a QOI, q(p), with respect to a POI, p, as
Sqp=pq×∂q∂p. |
This index measures a percentage response: if the parameter p changes by x%, then the quantity q changes by Sqp×x%. The sign of the index indicates if the response is positively or negatively correlated.
We first consider the QOIs that are related to the source of the infection: the R0 and the population size of asymptomatic non-ART adults, as suggested by the baseline simulations in Section 4.1. The first two columns of the sensitivity indices in Table 4 show that the coverage of ART for the HIV+ adults (σART) is the most sensitive risk factor: a 1% increase in the ART coverage would reduce the R0 by 0.56% (from R0=1.08→1.074), and the total number of asymptomatic HIV without ART would be reduced by 7.59% (8 fewer people). This suggests that it would be the most effective to control the source of the infection by improving the coverage of the ART among the HIV-infected adults.
Normalized sensitivity indices | ||||||
![]() |
R0 | Asymp. no-ART adults (peak) | Peaking time | Total infect. at peak | Total infect. at endemic | Accum. deaths |
σMN | 0.53 | 5.84 | −5.94 | 10.86 | 7.12 | 7.67 |
σART | −0.56 | −7.59 | 5.01 | −9.98 | −6.70 | −7.25 |
σHIV | 0.39 | 5.21 | −3.54 | 7.18 | 4.88 | 5.20 |
σMA | 0.07 | 0.82 | −1.24 | 1.52 | 1.00 | 1.07 |
σMAT | 0.04 | 0.43 | −0.42 | 0.81 | 0.53 | 0.57 |
Trend | Linear | Linear | Nonlinear | Linear | Linear | Linear |
![]() |
The changes in R0 (0.56%) and asymptomatic HIV+ no-ART (A3 group) adults (7.59%) may look like a small perturbation of the baseline scenario. However, each of these A3 adults may create a super spreading event: the A3 group consists around 12% of the infected population and are responsible for 74% of the infection (infection cases weighted by the R0's in Table 3). Thus, it is critical to reduce this highly infectious group and control the silent shedding.
Among the QOIs related to the disease dynamics and outcomes (columns 3-5 in Table 4), the prevalence of malnutrition in children (σMN) is the most sensitive factor: by lowering 1% of the malnutrition prevalence, the total infected population at the peak would be reduced by 10.86% (81 fewer cases, including 68 children), and the accumulative disease-induced deaths through one outbreak season (the endemic state reached around year 8) could be reduced by 7.67% (2450, including 488 adults and 1962 children). Moreover, σMN is the second most sensitive parameter for the other two QOIs (columns 1-2). This suggests that it would be the most effective to reduce malnutrition prevalence in children, who are the largest high-risk population in our baseline simulation, in order to both control the additional shedding to the community and limit human mortality from the epidemic.
Moreover, the peaking time of the epidemic (column 3 in Table 4) has highly sensitive and nonlinear responses to the changes in all the model parameters (see figure below the table). The large magnitudes of the indices suggest that even a small reduction of the risk factors can delay the start of an epidemic, which can be considered as potential strategies to relieve pressure on the healthcare system. The nonlinearity in the response curves indicates that the peaking time of an epidemic will be hard to predict.
We create and analyze a stage-progression compartmental model to investigate the iNTS epidemic emerging in sub-Saharan Africa. Our model considers multiple age groups in a population with different levels of immune-competence that are associated with the complex risk factors circulating in the region, including the HIV infection, availability of antiretroviral therapy, and high prevalence of malaria infection and malnutrition in children under age 5.
We defined the progression between the infection stages as a function of the branching probabilities at each decision stage and the average time spent within the stage. This approach allows a more straightforward parameter estimation based on the epidemiology literature than directly defining the progression rates. It also gives a more intuitive interpretation during the model analysis.
We derived the basic reproduction number R0 as a weighted average of the contribution from each population cohort (Rκ0). Within each population, the contributing Rκ0 is the summation of the number of secondary cases generated at each infectious stage weighted by the expected probability of entering the infectious stage. In particular, since asymptomatic adults can have recurrent infections, they create an infinite chain of infection with diminishing probability.
Both the basic reproduction number and the numerical simulations suggest that the HIV+ adults with no ART are the driving force of infection for the epidemic. At the peaking time, they form 12% of the infected population and are responsible for 74% of the infection. Thus, it would be critical and most effective to design the intervention program that targets this particular cohort to control the chain of the infection. On the other hand, our simulations show that largest disease burden is among the children group in terms of the highest infection and death counts. This suggests that reducing the susceptibility among this cohort would reduce the disease burden the most.
The sensitivity analysis identifies that the coverage of ART for the HIV-infected adults, σART, and the malnutrition prevalence in children, σMN, are the two most important risk factors that may inform effective disease mitigation. To control the source of the infection, it would the most impactful to improve ART coverage among the HIV+ adults, which would reduce the asymptomatic cohort that drives the epidemic. Children are the largest high-risk population, and it would be most productive to reduce the overall disease burden by lowering the malnutrition prevalence in this cohort. This would improve their immune competence and thus make them less susceptible to the pathogen. To better inform the public health efforts, it will be useful to combine our model with a cost-benefit analysis to optimize mitigation strategies.
This model offers important insights into iNTS dynamics and control. We recognize that our conclusions are based on assumptions, biases, and uncertainties in our model and parameters. Many of these limitations are related to the choice of model parameters. For example, we have assumed that all the parameters are constant, and there is no seasonal variation. In reality, malaria prevalence among children is correlated with the seasonal peak of the rainy season, and the local temperature is also playing a vital role in the mosquito-borne disease. Moreover, the malnutrition prevalence also increases during the rainy season when the household food supplies decrease, and the new season's crops are growing.
Moreover, by having constant parameters rather than distributions, we didn't consider the potential super spreading events that may cause small outbreaks in a local area. We have made the assumption that these events are uniformly distributed in time and the mean-field approximation can capture their average impact over the time scales we are considering. It would be worthwhile to validate this assumption in the future by comparing our simple model with a more complex simulation.
Our results will guide us in developing a more detailed individual agent-based model. The agent-based model will account for each individual's age, treatment history, family unit, and local community spatial mixing. The sensitivity analysis has identified the importance of including comorbidities, such as HIV infection and malnutrition, in our simulation. We will also investigate the impact of different mitigation strategies, seasonality, and local superspreading events.
Thus, before using any model to guide policy, the model parameters need to be carefully reviewed for the local setting. Also, the uncertainty of model predictions must be quantified with respect to the model assumptions. The current model is a preliminary study to investigate the iNTS epidemic, yet it provides a robust framework that could be further extended to incorporate more practical scenarios. We hope that models similar to the one presented here can help inform public health workers to mitigate the disease burden.
This research was partially supported by the NSF award 1563531 and the NIH-NIGMS Models of Infectious Disease Agent Study (MIDAS) award U01GM097661. Support also came from R01 AI130473-01A1, D43 TW005884, and D43 TW010543 (PI: Perkins). The content is solely the responsibility of the authors and does not necessarily represent the official views of the National Science Foundation or the National Institutes of Health. The authors gratefully acknowledge the assistance and dedication of the University of New Mexico-Kenya Programs faculty, staff, and trainees. We are also grateful to all of the parents and guardians who participated in our research studies over the last 18 years by entrusting us to care for their children and investigate pediatric infectious diseases in the Siaya Community.
All authors declare no conflicts of interest in this paper.
[1] | D. J. V. D. Windt, V. Sud, H. Zhang, A. Tsung, H. Huang, The effects of physical exercise on fatty liver disease, J. Liver Res., 18 (2018), 89–101. |
[2] |
D. Song, D. S. F. Yu, P. W. C. Li, Y. Lei, The effectiveness of physical exercise on cognitive and psychological outcomes in individuals with mild cognitive impairment: A systematic review and meta-analysis, Int. J. Nurs. Stud., 79 (2018), 155–164. doi: 10.1016/j.ijnurstu.2018.01.002
![]() |
[3] | M. G. Benedetti, G. Furlini, A. Zati, G. L. Mauro, The effectiveness of physical exercise on bone density in osteoporotic patients, Bio. Med. Res. Int., (2018), 4840531. |
[4] |
M. M. Ziaaldini, E. Marzetti, A. Picca, Z. Murlasits, Biochemical pathways of sarcopenia and their modulation by physical exercise: A narrative review, Front. Med., 4 (2017), 167. doi: 10.3389/fmed.2017.00167
![]() |
[5] |
C. M. Hearing, W. C. Chang, K. L. Szuhany, T. Deckersbach, A. A. Nierenberg, L. G. Sylvia, Physical exercise for treatment of mood disorders: A critical review, Curr. Behav. Neurosci. Rep., 3 (2016), 350–359. doi: 10.1007/s40473-016-0089-y
![]() |
[6] | L. W. Judge, L. J. Urbina, D. L. Hoover, B. W. Craig, L. M. Judge, B. M. Leitzelar, et al., The impact of competitive trait anxiety on collegiate powerlifting performance, J. Strength Cond. Res., 30 (2016), 2399–2405. |
[7] | M. Mottaghi, A. Atarodi, Z. Rohani, The relationship between coaches' and athletes' competitive anxiety and their performance, Iran. J. Psychiatry Behav. Sci., 7 (2013), 68–76. |
[8] |
H. J. Pritchard, D. A. Tod, M. J. Barnes, J. W. Keogh, M. R. McGuigan, Tapering practices of New Zealand's elite raw powerlifters, J. Strength Cond. Res., 30 (2016), 1796–1804. doi: 10.1519/JSC.0000000000001292
![]() |
[9] |
M. Zawadka, M. S. Paszkowska, P. Gawda, E. Lukasik, J. Smolka, M. Jablonski, What factors can affect lumbopelvic flexion-extension motion in the sagittal plane?: A literature review, Hum. Mov. Sci., 58 (2018), 205–218. doi: 10.1016/j.humov.2018.02.008
![]() |
[10] | A. C. Fry, W. J. Kraemer, M. H. Stone, L. P. Koziris, J. T. Thrush, S. J. Fleck, Relationships between serum testosterone, cortisol and weightlifting performance, J. Strength Cond. Res., 14 (2000), 338–343. |
[11] | I. Sandau, M. Witt, The influence of stress factors on joint loading of lower extremities in weightlifting, Ger. J. Sports Med./Deutsch. Z. Sportmed., 69 (2018), 1–10. |
[12] | P. A. Solberg, W. G. Hopkins, G. Paulsen, T. A. Haugen, Peak age and performance progression in world-class weightlifting and powerlifting athletes, Int. J. Sports Physiol. Perform., (2019), 1–24. |
[13] |
C. Latella, W. P. Teo, J. Spathis, D. V. D. Hoek, Long-term strength adaptation: A 15-year analysis of powerlifting athletes, J. Strength Cond. Res., 34 (2020), 2412. doi: 10.1519/JSC.0000000000003657
![]() |
[14] |
C. Latella, D. V. D. Hoek, W. P. Teo, Factors affecting powerlifting performance: an analysis of age-and weight-based determinants of relative strength, Int. J. Perform. Anal. Sport, 18 (2018), 532–544. doi: 10.1080/24748668.2018.1496393
![]() |
[15] | M. M. Anton, W. W. Spirduso, H. Tanaka, Age-related declines in anaerobic muscular performance: Weightlifting and powerlifting, Med. Sci. Sports Exercise, 36 (2014), 143–147. |
[16] |
L. P. James, P. Comfort, T. J. Suchomel, V. G. Kelly, E. M. Beckman, G. G. Haff, The impact of power clean ability and training age on adaptations to weightlifting-style training, J. Strength Cond. Res., 33 (2019), 2936–2944. doi: 10.1519/JSC.0000000000002534
![]() |
[17] |
J. Pearson, J. G. Spathis, D. J. V. D. Hoek, P. J. Owen, J. Weakley, C. Latella, Effect of competition frequency on strength performance of powerlifting athletes, J. Strength Cond. Res., 34 (2020), 1213–1219. doi: 10.1519/JSC.0000000000003563
![]() |
[18] |
N. A. Coker, A. N. Varanoske, K. M. Baker, D. L. H. Vaughn, A. J. Wells, Predictors of competitive success of national-level powerlifters: A multilevel analysis, Int. J. Perform. Anal. Sport, 18 (2018), 796–805. doi: 10.1080/24748668.2018.1519751
![]() |
[19] | S. K. Travis, M. C. Zourdos, C. D. Bazyler, Weight selection attempts of elite classic powerlifters, Percept. Mot. Skills, 2020. |
[20] | M. Z. Naser, A. Alavi, Insights into performance fitness and error metrics for machine learning, preprint, arXiv: 2006.00887. |
[21] | A. Botchkarev, Performance metrics (error measures) in machine learning regression, forecasting and prognostics: Properties and typology, preprint, arXiv: 1809.03006. |
[22] |
G. B. Huang, Q. Y. Zhu, C. K. Siew, Extreme learning machine: Theory and applications, Neurocomputing, 70 (2006), 489–501. doi: 10.1016/j.neucom.2005.12.126
![]() |
[23] | M. Rocca, C. Perna, Nonlinear autoregressive sieve bootstrap based on extreme learning machines, Math. Biosci. Eng., 17 (2019), 636–653. |
[24] | G. B. Huang, H. Zhou, X. Ding, R. Zhang, Extreme learning machine for regression and multiclass classification, IEEE Trans. Syst., Man, Cybern., Part B (Cybern.), 42 (2011), 513–529. |
[25] | J. Tang, C. Deng, G. B. Huang, Extreme learning machine for multilayer perceptron, IEEE Trans. Neural Networks Learn. Syst., 27 (2015), 809–821. |
[26] | W. Deng, Q. Zheng, L. Chen, Regularized extreme learning machine, 2009 IEEE Symp. Comput. Intel. Data Min., (2009), 389–395. |
[27] | Y. Zheng, B. Chen, S. Wang, W. Wang, W. Qin, Mixture correntropy-based kernel extreme learning machines, IEEE Trans. Neural Networks Learn. Syst., 2020. |
[28] | J. Cao, H. Dai, B. Lei, C. Yin, H. Zeng, A. Kummert, Maximum correntropy criterion-based hierarchical one-class classification, IEEE Trans. Neural Networks Learn. Syst., 2020. |
[29] |
O. F. Ertugrul, A novel randomized machine learning approach: Reservoir computing extreme learning machine. Appl. Soft Comput., 94 (2020), 106433. doi: 10.1016/j.asoc.2020.106433
![]() |
[30] |
S. Mirjalili, A. Lewis, The whale optimization algorithm. Adv. Eng. Software, 95 (2016), 51–67. doi: 10.1016/j.advengsoft.2016.01.008
![]() |
[31] |
L. L. Li, J. Sun, M. L. Tseng, Z. G. Li, Extreme learning machine optimized by whale optimization algorithm using insulated gate bipolar transistor module aging degree evaluation, Expert Syst. Appl., 127 (2019), 58–67. doi: 10.1016/j.eswa.2019.03.002
![]() |
[32] | https://www.powerlifting.sport/championships/results.html |
[33] | https://www.kaggle.com/open-powerlifting/powerlifting-database |
[34] | T. Yang, Study on the growth rule of the olympic men's weightlifting champion and winning factors. Sichuan Sports Sci., 38 (2019), 54–58. |
[35] |
B. T. Le, D. Xiao, Y. Mao, D. He, Coal analysis based on visible-infrared spectroscopy and a deep neural network, Infrared Phys. Technol., 93 (2018), 34–40. doi: 10.1016/j.infrared.2018.07.013
![]() |
[36] | D. Xiao, B. T. Le, Rapid analysis of coal characteristics based on deep learning and visible-infrared spectroscopy, Microchem. J., (2020), 104880. |
1. | Ylenia Russotto, Cristina Micali, Giovanni Francesco Pellicanò, Giuseppe Nunnari, Emmanuele Venanzi Rullo, HIV and Mediterranean Zoonoses: A Review of the Literature, 2022, 14, 2036-7449, 694, 10.3390/idr14050075 | |
2. | Claire E. Depew, Stephen J. McSorley, The role of tissue resident memory CD4 T cells in Salmonella infection: Implications for future vaccines, 2023, 41, 0264410X, 6426, 10.1016/j.vaccine.2023.09.011 |
Compartment | Description |
Sκ | Susceptible |
Iκ1 | Infected people with mild symptoms |
IκHT | Infected people who do home treatment |
Iκ2 | Infected people with severe symptoms |
IκMT | Infected people who are admitted to medical facilities |
Rκ | People recover from the infection (with temporary immunity) |
Aκ | People with asymptomatic carriage of the infection (go back home) |
Dκ | People die from the infection |
Group index κ | |
κ=1 | HIV- adults, 25—40 years old |
κ=2 | HIV+ adults, on ART, 25—40 years old |
κ=3 | HIV+ adults, not on ART, 25—40 years old |
κ=4 | Children, ≤ 5 years old |
Description | HIV- Adults (κ=1) | HIV+ ART (κ=2) | HIV+ no ART (κ=3) | Children (κ=4) | Reference | |
cβκ | Transmission rate | 4.6×10−3 | 0.092 | 0.257 | 0.232 | Calibrated |
μκ | Migration rate (mortality+aging) per day | 2.0×10−4 | 3.7×10−4 | 9.2×10−4 | 5.8×10−4 | Derived |
- Mortality rate per 1000 people per year | 5.02 | 70 | 270 | 10.4 | [25,26] | |
- Aging rate per year | 1/15 | 1/15 | 1/15 | 1/5 | Derived | |
Sκ0 | Population size (Siaya County) | 106202 | 19171 | 10784 | 141752 | [27] |
- | Incidence, per 100, 000 population per year | 37 | 739 | 2070 | 1870 | [28,29] |
σMA | Prevalence of malaria epidemic in children | - | - | - | 2% | [30] |
σMN | Prevalence of malnutrition in children | - | - | - | 40% | [26] |
σHIV | Prevalence of HIV epidemic | σAHIV=22% | σAHIV=22% | σAHIV=22% | σCHIV=2% | EO∗ |
σART | Coverage of ART for HIV adults | 64% | - | EO∗ | ||
σMAT | Maternal trans. rate for non-ART adults | - | - | 30% | - | [31] |
Without the effect of migration, the raw estimates for disease parameters... | ||||||
P01H | Fraction of I1 do self-treatment | 20% | 20% | 20% | 50% | EO∗ |
P012 | Fraction of I1 do not do self-treatment | 80% | 80% | 80% | 50% | EO∗ |
P0HR | Fraction of IHT recover | 50% | 40% | 10% | 50% | EO∗ |
P0HA | Fraction of IHT become asymp. | - | 4% [32] | 35%* | - | EO∗ |
P0H2 | Fraction of IHT become I2 | 50% | 56% | 55% | 50% | EO∗ |
P02M | Fraction of I2 do medical-treatment | 90% | 90% | 90% | 90% | EO∗ |
P02D | Fraction of I2 do not get medical-treatment | 10% | 10% | 10% | 10% | EO∗ |
P0MD | Fraction of IMT die | 11% [33] | 11% [33] | 47% [6] | 22% [34] | EO∗ |
P0MA | Fraction of IMT become asymp. | - | 4% | 35%* | - | EO∗ |
P0MR | Fraction of IMT recover | 89% | 85% | 18% | 78% | EO∗ |
τ01 | Time spent in I1 (infected → lightly sick) | 1 d | 1 d | 1 d | 1 d | EO∗ |
τ0H | Time spent in self-treatment | 3 d | 3 d | 3 d | 3 d | EO∗ |
τ02 | Time spent in I2 (lightly sick → heavily sick) | 4 d | 4 d | 4 d | 4 d | EO∗ |
τ0M | Time spent in medical-treatment | 7 d | 7 d | 7 d | 7 d | EO∗ |
τ0R | Time of immunity period after recovery | 1 yr | 1 yr | 1 yr | 2 m | EO∗ |
τ0A | Time of being asymptomatic | - | 59 d [32] | 45 d [32] (60 d [35]) |
- | EO∗ |
The adjusted parameter values for migration... | ||||||
τ∗ | Time spent in stage ∗ | (1/τ0∗+μκ)−1 | Derived | |||
P∗N | Fraction of migration at stage ∗ | μκ×τ∗ | Derived | |||
Pij | Fraction of people at stage i move to stage j | P0ij∗(1−P∗N) | Derived |
Rκ0 | I1 | IHT | I2 | A | |
HIV- adults (κ=1) | 0.03 | 5×10−3 | 3×10−3 | 0.02 | - |
HIV+ART adults (κ=2) | 0.77 | 0.10 | 0.06 | 0.37 | 0.24 |
HIV+ no ART adults (κ=3) | 8.47 | 0.41 | 0.25 | 1.50 | 6.31 |
Children (κ=4) | 1.35 | 0.25 | 0.37 | 0.74 | - |
Normalized sensitivity indices | ||||||
![]() |
R0 | Asymp. no-ART adults (peak) | Peaking time | Total infect. at peak | Total infect. at endemic | Accum. deaths |
σMN | 0.53 | 5.84 | −5.94 | 10.86 | 7.12 | 7.67 |
σART | −0.56 | −7.59 | 5.01 | −9.98 | −6.70 | −7.25 |
σHIV | 0.39 | 5.21 | −3.54 | 7.18 | 4.88 | 5.20 |
σMA | 0.07 | 0.82 | −1.24 | 1.52 | 1.00 | 1.07 |
σMAT | 0.04 | 0.43 | −0.42 | 0.81 | 0.53 | 0.57 |
Trend | Linear | Linear | Nonlinear | Linear | Linear | Linear |
![]() |
Compartment | Description |
Sκ | Susceptible |
Iκ1 | Infected people with mild symptoms |
IκHT | Infected people who do home treatment |
Iκ2 | Infected people with severe symptoms |
IκMT | Infected people who are admitted to medical facilities |
Rκ | People recover from the infection (with temporary immunity) |
Aκ | People with asymptomatic carriage of the infection (go back home) |
Dκ | People die from the infection |
Group index κ | |
κ=1 | HIV- adults, 25—40 years old |
κ=2 | HIV+ adults, on ART, 25—40 years old |
κ=3 | HIV+ adults, not on ART, 25—40 years old |
κ=4 | Children, ≤ 5 years old |
Description | HIV- Adults (κ=1) | HIV+ ART (κ=2) | HIV+ no ART (κ=3) | Children (κ=4) | Reference | |
cβκ | Transmission rate | 4.6×10−3 | 0.092 | 0.257 | 0.232 | Calibrated |
μκ | Migration rate (mortality+aging) per day | 2.0×10−4 | 3.7×10−4 | 9.2×10−4 | 5.8×10−4 | Derived |
- Mortality rate per 1000 people per year | 5.02 | 70 | 270 | 10.4 | [25,26] | |
- Aging rate per year | 1/15 | 1/15 | 1/15 | 1/5 | Derived | |
Sκ0 | Population size (Siaya County) | 106202 | 19171 | 10784 | 141752 | [27] |
- | Incidence, per 100, 000 population per year | 37 | 739 | 2070 | 1870 | [28,29] |
σMA | Prevalence of malaria epidemic in children | - | - | - | 2% | [30] |
σMN | Prevalence of malnutrition in children | - | - | - | 40% | [26] |
σHIV | Prevalence of HIV epidemic | σAHIV=22% | σAHIV=22% | σAHIV=22% | σCHIV=2% | EO∗ |
σART | Coverage of ART for HIV adults | 64% | - | EO∗ | ||
σMAT | Maternal trans. rate for non-ART adults | - | - | 30% | - | [31] |
Without the effect of migration, the raw estimates for disease parameters... | ||||||
P01H | Fraction of I1 do self-treatment | 20% | 20% | 20% | 50% | EO∗ |
P012 | Fraction of I1 do not do self-treatment | 80% | 80% | 80% | 50% | EO∗ |
P0HR | Fraction of IHT recover | 50% | 40% | 10% | 50% | EO∗ |
P0HA | Fraction of IHT become asymp. | - | 4% [32] | 35%* | - | EO∗ |
P0H2 | Fraction of IHT become I2 | 50% | 56% | 55% | 50% | EO∗ |
P02M | Fraction of I2 do medical-treatment | 90% | 90% | 90% | 90% | EO∗ |
P02D | Fraction of I2 do not get medical-treatment | 10% | 10% | 10% | 10% | EO∗ |
P0MD | Fraction of IMT die | 11% [33] | 11% [33] | 47% [6] | 22% [34] | EO∗ |
P0MA | Fraction of IMT become asymp. | - | 4% | 35%* | - | EO∗ |
P0MR | Fraction of IMT recover | 89% | 85% | 18% | 78% | EO∗ |
τ01 | Time spent in I1 (infected → lightly sick) | 1 d | 1 d | 1 d | 1 d | EO∗ |
τ0H | Time spent in self-treatment | 3 d | 3 d | 3 d | 3 d | EO∗ |
τ02 | Time spent in I2 (lightly sick → heavily sick) | 4 d | 4 d | 4 d | 4 d | EO∗ |
τ0M | Time spent in medical-treatment | 7 d | 7 d | 7 d | 7 d | EO∗ |
τ0R | Time of immunity period after recovery | 1 yr | 1 yr | 1 yr | 2 m | EO∗ |
τ0A | Time of being asymptomatic | - | 59 d [32] | 45 d [32] (60 d [35]) |
- | EO∗ |
The adjusted parameter values for migration... | ||||||
τ∗ | Time spent in stage ∗ | (1/τ0∗+μκ)−1 | Derived | |||
P∗N | Fraction of migration at stage ∗ | μκ×τ∗ | Derived | |||
Pij | Fraction of people at stage i move to stage j | P0ij∗(1−P∗N) | Derived |
Rκ0 | I1 | IHT | I2 | A | |
HIV- adults (κ=1) | 0.03 | 5×10−3 | 3×10−3 | 0.02 | - |
HIV+ART adults (κ=2) | 0.77 | 0.10 | 0.06 | 0.37 | 0.24 |
HIV+ no ART adults (κ=3) | 8.47 | 0.41 | 0.25 | 1.50 | 6.31 |
Children (κ=4) | 1.35 | 0.25 | 0.37 | 0.74 | - |
Normalized sensitivity indices | ||||||
![]() |
R0 | Asymp. no-ART adults (peak) | Peaking time | Total infect. at peak | Total infect. at endemic | Accum. deaths |
σMN | 0.53 | 5.84 | −5.94 | 10.86 | 7.12 | 7.67 |
σART | −0.56 | −7.59 | 5.01 | −9.98 | −6.70 | −7.25 |
σHIV | 0.39 | 5.21 | −3.54 | 7.18 | 4.88 | 5.20 |
σMA | 0.07 | 0.82 | −1.24 | 1.52 | 1.00 | 1.07 |
σMAT | 0.04 | 0.43 | −0.42 | 0.81 | 0.53 | 0.57 |
Trend | Linear | Linear | Nonlinear | Linear | Linear | Linear |
![]() |