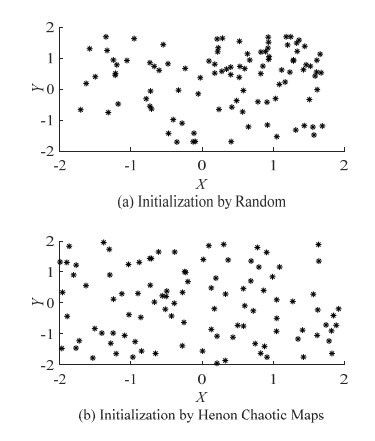
Defect detection on magnetic tile surfaces is of great significance for the production monitoring of permanent magnet motors. However, it is challenging to detect the surface defects from the magnetic tile due to these issues: 1) Defects appear randomly on the surface of the magnetic tile; 2) the defects are tiny and often overwhelmed by the background. To address such problems, an Adaptive Rotation Attention Network (ARA-Net) is proposed for defect detection on the magnetic tile surface, where the Adaptive Rotation Convolution (ARC) module is devised to capture the random defects on the magnetic tile surface by learning multi-view feature maps, and then the Rotation Region Attention (RAA) module is designed to locate the small defects from the complicated background by focusing more attention on the defect features. Experiments conducted on the MTSD3C6K dataset demonstrate the proposed ARA-Net outperforms the state-of-the-art methods, further providing assistance for permanent magnet motor monitoring.
Citation: Fang Luo, Yuan Cui, Xu Wang, Zhiliang Zhang, Yong Liao. Adaptive rotation attention network for accurate defect detection on magnetic tile surface[J]. Mathematical Biosciences and Engineering, 2023, 20(9): 17554-17568. doi: 10.3934/mbe.2023779
[1] | Shuang Wang, Heming Jia, Qingxin Liu, Rong Zheng . An improved hybrid Aquila Optimizer and Harris Hawks Optimization for global optimization. Mathematical Biosciences and Engineering, 2021, 18(6): 7076-7109. doi: 10.3934/mbe.2021352 |
[2] | Yuheng Wang, Yongquan Zhou, Qifang Luo . Parameter optimization of shared electric vehicle dispatching model using discrete Harris hawks optimization. Mathematical Biosciences and Engineering, 2022, 19(7): 7284-7313. doi: 10.3934/mbe.2022344 |
[3] | Cuicui Cai, Maosheng Fu, Xianmeng Meng, Chaochuan Jia, Mingjing Pei . Indoor high-precision visible light positioning system using Jaya algorithm. Mathematical Biosciences and Engineering, 2023, 20(6): 10358-10375. doi: 10.3934/mbe.2023454 |
[4] | Shenghan Li, Linlin Ye . Multi-level thresholding image segmentation for rubber tree secant using improved Otsu's method and snake optimizer. Mathematical Biosciences and Engineering, 2023, 20(6): 9645-9669. doi: 10.3934/mbe.2023423 |
[5] | Peng-Yeng Yin, Chih-Chun Tsai, Rong-Fuh Day, Ching-Ying Tung, Bir Bhanu . Ensemble learning of model hyperparameters and spatiotemporal data for calibration of low-cost PM2.5 sensors. Mathematical Biosciences and Engineering, 2019, 16(6): 6858-6873. doi: 10.3934/mbe.2019343 |
[6] | Yongquan Zhou, Yanbiao Niu, Qifang Luo, Ming Jiang . Teaching learning-based whale optimization algorithm for multi-layer perceptron neural network training. Mathematical Biosciences and Engineering, 2020, 17(5): 5987-6025. doi: 10.3934/mbe.2020319 |
[7] | Xiaohao Chen, Maosheng Fu, Zhengyu Liu, Chaochuan Jia, Yu Liu . Harris hawks optimization algorithm and BP neural network for ultra-wideband indoor positioning. Mathematical Biosciences and Engineering, 2022, 19(9): 9098-9124. doi: 10.3934/mbe.2022423 |
[8] | Li-zhen Du, Shanfu Ke, Zhen Wang, Jing Tao, Lianqing Yu, Hongjun Li . Research on multi-load AGV path planning of weaving workshop based on time priority. Mathematical Biosciences and Engineering, 2019, 16(4): 2277-2292. doi: 10.3934/mbe.2019113 |
[9] | Huangjing Yu, Yuhao Wang, Heming Jia, Laith Abualigah . Modified prairie dog optimization algorithm for global optimization and constrained engineering problems. Mathematical Biosciences and Engineering, 2023, 20(11): 19086-19132. doi: 10.3934/mbe.2023844 |
[10] | Xiangyang Ren, Shuai Chen, Kunyuan Wang, Juan Tan . Design and application of improved sparrow search algorithm based on sine cosine and firefly perturbation. Mathematical Biosciences and Engineering, 2022, 19(11): 11422-11452. doi: 10.3934/mbe.2022533 |
Defect detection on magnetic tile surfaces is of great significance for the production monitoring of permanent magnet motors. However, it is challenging to detect the surface defects from the magnetic tile due to these issues: 1) Defects appear randomly on the surface of the magnetic tile; 2) the defects are tiny and often overwhelmed by the background. To address such problems, an Adaptive Rotation Attention Network (ARA-Net) is proposed for defect detection on the magnetic tile surface, where the Adaptive Rotation Convolution (ARC) module is devised to capture the random defects on the magnetic tile surface by learning multi-view feature maps, and then the Rotation Region Attention (RAA) module is designed to locate the small defects from the complicated background by focusing more attention on the defect features. Experiments conducted on the MTSD3C6K dataset demonstrate the proposed ARA-Net outperforms the state-of-the-art methods, further providing assistance for permanent magnet motor monitoring.
Meta-heuristic (MH) optimization algorithms are extremely popular in engineering applications, because of superiority about simple concepts, easy implementation, no requirement for gradient information as well as capability of bypassing local optima, which can be treat as competitive solutions for various of problems covering different research fields, such as pattern recognition, target tracking, artificial intelligence and system control. By imitating social behaviors, biological principles or physical phenomena in nature, meta-heuristic algorithms can be categorized into 3 categories: evolution-based, physics-based and swarm-based approaches. A large number of scholars and researchers have successively proposed a variety of algorithms with specific strategies, for example, inspired by foraging behavior of Escherichia coli in human intestine, Passino [1] summarized the Bacterial Foraging Optimization (BFO) algorithm. Kennedy and Eberhart [2] proposed Particle Swarm Optimization (PSO) algorithm to find the optimal solution through cooperation and information sharing among individuals in the group. Differential Evolution (DE) algorithm based on differential simple mutation operation and one-to-one competitive survival strategy was presented by Storn and Price [3] in 1995. Moreover, Whale Optimization Algorithm (WOA) mimicking hunting behavior along with helix-shaped movement of humpbacks and Ant Colony Optimization (ACO) algorithm inspired by social intelligence of ants in finding the closest path from the nest to a source of food both attract considerable attention [4,5]. Besides, there are so many applications of meta-heuristic optimization algorithms for practical purposes. Yıldız wt al. [6] incorporated the chaotic maps in the elementary Lévy flight distribution and dubbed it as Chaotic Lévy Flight Distribution (CLFD) algorithm to address physical world engineering optimization problems. Wansasueb integrated Grey Wolf Optimizer (GWO), Genetic Algorithm (GA), Population Base Increment Learning (PBIL) and Water Cycle Algorithm (WCA) to form a new meta-heuristic named as Ensemble of Genetic algorithm (E-GGWP-W) to optimize design issue of composite wing [7,8,9,10,11]. Winyangkul et al. [12] presented multi-objective topology and sizing optimization of a morphing wing structure. Kumar et al. [13] enhanced 5 MH algorithms based on random migration search phase and simulated annealing-based selection for solving size and topology optimization of the trusses. Sparrow Search Algorithm (SSA) is employed to manage the operation of microgrid for minimizing either the total operating cost or the total emission [14]. Long et al. [15] proposed a novel hybrid seagull optimization algorithm (HSOA) based on cosine function and differential mutation strategy for estimating the parameters of photovoltaic models.
Harris Hawks Optimization (HHO) algorithm is a new kind of swarm intelligence algorithm proposed by Ali Asghar Heidari in 2019, which imitates the cooperative behavior of Harris hawks during predation [16]. The entire optimization process consists of three phases: exploration, exploitation and conversion. It enjoys the characteristics of simplicity in structure, concision in control parameters and excellent capability in global search. However, it suffers the shortcomings of low optimization accuracy, slow convergence speed and easy to fall into local optima. In order to settle the aforementioned issues, experts in different fields carried out a range of improvement method and put them into application. Tang et al. [17] promoted the convergence speed and accuracy of algorithm by introducing Tent Chaotic Map and elite hierarchy strategy into Chaos Elite Harris Hawks Optimization (CEHHO). Yin et al. [18] made use of nonlinear control parameter strategy and random inverse learning method to improve HHO (NOL-HHO). Attiya et al. [19] proposed an improved HHO algorithm (HHOSA) based on simulated annealing to solve problem of task scheduling in cloud computing. Ismael et al. [20] proposed Harris Hawks Optimization algorithm based on Opposition-Based Learning (HHOA-OBL) for hyperparameter estimation and feature selection. Qu et al. [21] used information exchange mechanism and nonlinear escape energy factors to improve population diversity and performance of algorithm. Ma et al. [22] mended fitness function through maximum likelihood estimation to get the solution for nonlinear equations about indoor positioning relate to difference of arrival time. Turabieh et al. [23] optimized HHO by controlling distribution of population and realized prediction of students' potential. ElSayed and Elattar [24] combined HHO with sequential quadratic programming to achieve optimal coordination for directional overcurrent relays of power. The Adaptive Harris Hawks Optimization (ADHHO) is proposed by Song for parameter identification of photovoltaic systems [25]. The persistent-trigonometric-differences mechanism and improved energy factor are helpful for balancing exploration and exploitation. With the help of comprehensive learning, equilibrium optimizer and terminal replacement mechanism, an improved algorithm named as comprehensive Learning Harris Hawks-Equilibrium Optimization (CLHHEO) is presented by Zhong for solving constrained optimization problems [26]. Hu et al. [27] used Specular Reflection Learning to improve HHO (HHOSRL) algorithm for assessing COVID-19. Dynamic Multi-Swarm Differential Learning Harris Hawks Optimizer (DMSDL-HHO) that divides population into many sub-swarms and introduces differential mutation operator candidate pool strategy is applied to the dispatch problem of hydropower stations [28]. Luo et al. [29] enhanced automatic epilepsy diagnosis method by using time-frequency analysis and Improved Harris Hawks Optimization (IHHO) with a hierarchical mechanism. Bardhan et al. [30] proposed ELM-IHHO by integrating the standard HHO algorithm with mutation-based search mechanism and Extreme Learning Machine for predicting soil compression index. Choi et al. [31] proposed an unsupervised intelligent system named as HHO-SVM for predicting the performance of a truck-haulage system using a combination of HHO and Support Vector Machine. Golafshani et al. [32] extended multi-layer neural network and radial basis function neural network by HHO to develop predictive models for the compressive strength of concretes containing supplementary cementitious materials.
As mentioned above, many scholars have applied variants of original HHO inspired by previous work in practical application, demonstrating the excellent performance and optimization capability of HHO. However, the original HHO still has potential for further improvement of convergence speed and solution accuracy. Moreover, there are serval defects belong to all or part of aforementioned algorithms. Firstly, the improved means for original HHO are not comprehensive, only focus on 2-3 aspects. There are opportunities to promote HHO in future. Secondly, significance of population's initial distribution is neglected, which directly affect the quality of subsequent optimization. Thirdly, there are some subjective factors in settings of parameters and constants, the reasons are not clearly stated, universality and robustness of the system may be reduced. Fourthly, according to a certain trigonometric function, individual takes movement in fixed pattern, it leads to the decrease of ergodicity and accuracy of search. Fifthly, the energy factor E used by the former studies does not accord with the law of physical consumption for animals. Finally, the influence between individuals in population is not taken into the consideration.
This paper takes efforts to improve original HHO and avoid above-mentioned problems as far as possible. First, change the generation mode of initial population. Chaos is a quite unique phenomenon caused by nonlinear effect, which has the characteristics of sensitivity to initial value, non-periodicity, long-term unpredictability and universality. So, chaos can exhibit the phenomenon of randomness and connection to the "random process" in system. Generating initial population by Hénon Chaotic Map based on conception of chaos can promote population's randomness and ergodicity in distribution. Second, improve the population diversity and local search capability of HHO. Quantum computing uses quantum bits code to store data, for the same size of population, compared with other methods, quantum code can contain much more information. So, introducing quantum correction in exploitation phase under certain condition can make use of features of quantum computing to promote diversity and increase the opportunities of taking precise movement of individual, under the premise of size of population is no changed. Third, enhance global search ability of HHO. Modified Nelder-Mead simplex method is widely used to solve parameter estimation and similar statistical problems, which requires only one or two function evaluations per iteration and quickly produces satisfactory results in first few iterations. So, modified Nelder-Mead simplex method is used to boost search performance and breadth in exploration phase, empower individual to move in wide range as needed in the search space, reducing the probability of falling into local optima. At the same time, it will not excessively increase computational complexity. Fourth, group communication factor belong to BFO can express relationship between individuals effectively. Attraction and repellent power are introduced into the search process as parameters can optimize function evaluation, and then, population regeneration will be more accurate. Final, when animal is running or jumping, with energy consumption, muscle cells will produce lactic acid, then muscles show fatigue. At this time, the speed or jumping distance will be declined, animal need to stop and have a rest. Integrate the conception of cycle about "run—consume physical strength—stop to take rest—restore energy" into update of escape energy factor E can better balance exploitation and exploration of HHO in accordant with biological laws.
The improved HHO studied in this paper is named as Quantum Correction Harris Hawks Optimization (QC-HHO) algorithm. It is tested by 10 classic benchmark functions and 30 CEC2014 benchmark functions, experimental results are recorded. In order to provide accurate and reliable conclusion, Wilcoxon rank-sum test with 5% degree is carefully performed. Compared with other optimizers, it can be generalized that the QC-HHO outperforms others in most cases. Moreover, for the application of gas leakage source localization, QC-HHO can provide excellent experimental result, search accuracy and speed are satisfactory.
Harris Hawks Optimization algorithm is a meta-heuristic algorithm derived from Harris hawk's predation action. This section briefly describes the basic principles and mathematical models of it.
The exploration phase of HHO algorithm is global search process. When hawks find the target prey in the air, all individuals coordinate their action to find a favorable position around the prey and form siege.
In initial state, the individual Hawk appears in a certain position belong to search space according to the principle of randomness, then gradually moves to the optimal solution. Set q as a random number located between 0 and 1, when q < 0.5, each individual will take movement by reference the position of other ones and prey. As q ≥ 0.5, the Harris hawk will stay in a tree that is in the scope of activity of population. The mathematical model can be described as follows:
X(t+1)={Xrand(t)−r1⋅|Xrand(t)−2⋅r2⋅X(t)|, q⩾0.5(XPrey(t)−Xm(t))−r3⋅(lb+r4⋅(ub−lb)), q<0.5 | (2.1) |
Xm=1N∑Ni=1Xi(t) | (2.2) |
In Eq (2.1), X(t) is position of population after the tth round of iteration. Xrand is location of a random individual in population. XPrey is position of the optimal solution, Xm is the average position of population. r1, r2, r3 and r4 are the random numbers in the interval of (0, 1). Ub and lb are the upper and lower bounds of search space respectively, N is population size.
The exploitation phase is a process of local search. When the prey is surrounded, the hawks will take attack. The HHO algorithm simulates escape behavior of prey and hunting strategy of hawks through different combinations of escape energy factor E and random numbers r ∈ (0, 1). r is escape opportunity for prey. r < 0.5 means that the escape is successful, but r ≥ 0.5 denotes an unsuccessful escape.
1) Soft besiege
As |E| ≥ 0.5 and r ≥ 0.5, prey is energetic and try to escape by jump, but eventually it is caught, a mathematical formula is as follows:
X(t+1)=ΔX(t)−E⋅|J⋅XPrey(t)−X(t)| | (2.3) |
ΔX(t)=XPrey(t)−X(t) | (2.4) |
J=2⋅(1−r5) | (2.5) |
In Eq (2.3), ΔX(t) represents the distance between optimal solution and current individual after the tth time of iteration, r5 is a random number between 0 and 1. J is jumping distance of prey during running for life.
2) Hard besiege
As |E| < 0.5 and r ≥ 0.5, prey is lack of physical strength and captured directly, formula is as follows:
X(t+1)=XPrey(t)−E⋅|ΔX(t)| | (2.6) |
3) Soft besiege with progressive rapid dives
As |E| ≥ 0.5 and r < 0.5, prey is energetic and has chance to escape, hawk will make a more intelligent soft encirclement, the implementation is as follows:
Y=XPrey(t)−E⋅|J⋅XPrey(t)−X(t)| | (2.7) |
Z=Y+S×LF(D) | (2.8) |
X(t+1)={Y,f(Y)<f(X(t))Z,f(Z)<f(X(t)) | (2.9) |
In Eq (2.8), D is the dimension of problem, S is a D-dimensional random vector, and LF is the Levy flight function as follows:
LF(x)=0.01×ru×σ|rv|1β | (2.10) |
σ=(Γ(1+β)×sin(πβ2)Γ(1+β2)×β×2(β−12))1β | (2.11) |
In Eqs (2.10) and (2.11), ru and rv are random numbers between 0 and 1, β is a constant numbered as 1.5.
4) Hard besiege with progressive rapid dives
As |E| < 0.5 and r < 0.5, prey has insufficient physical energy, but it still has a chance to escape. In order to reduce the average distance from prey, hawks form a new hard encirclement, the strategy is as follows:
Y=XPrey(t)−E⋅|J⋅XPrey(t)−Xm(t)| | (2.12) |
Z=Y+S×LF(D) | (2.13) |
X(t+1)={Y,f(Y)<f(X(t))Z,f(Z)<f(X(t)) | (2.14) |
The HHO algorithm controls the conversion between global search and local search through the escape energy factor E, which is defined as follows:
E=2⋅E0⋅(1−tT) | (2.15) |
In Eq (2.15), T is the maximum rounds of iterations, E0 as (-1, 1) is the initial value of the energy in iterations. When |E| ≥ 1, the HHO algorithm enter into the exploration phase, which represents individuals in population move towards the prey within whole search space. When |E| < 1, search will convert into the exploitation phase.
In order to overcome the shortcomings of original HHO, as well as improving the searching performance, this section will propose the optimization methods in 5 aspects include Hénon Chaotic Map, quantum correction, modified Nelder-Mead simplex method, group communication factor and amended escape energy factor E.
This section will give the definition and mathematical model of the Hénon Chaotic Map, as well as compare the distribution of initial population generated by random number and Hénon Chaotic Map.
High-quality initial population can help to improve the accuracy and speed of convergence. If the distribution of initial population is far from the target solution, it is impossible to effectively solve the problem [33]. Therefore, in the process of initialization, the initial values should be distributed as evenly as possible in the solution space in order to get better results. The original HHO algorithm is initialized by random numbers, which cannot guarantee the diversity and ergodicity of population.
As complex behavior of nonlinear systems, chaos exhibits phenomenon that is similar to randomness. This feature can be used to improve the performance of the algorithm [34]. Hénon Chaotic Map is proposed by Michel Hénon in 1976, who is a French mathematician [35]. It is discrete-time dynamic system which can generate chaotic phenomenon in 2-Dimensional space, the mathematical expression is as follows:
{xn+1=1−a⋅x2n+ynyn+1=b⋅xn | (3.1) |
In Eq (3.1), when a takes 1.4, b takes 0.3, system will enter into chaotic state. The initial state of Harris hawk population is as follows:
X={xji},i∈{1,2,⋯,N},j∈{1,2,⋯,D} | (3.2) |
In Eq (3.2), N is scale of population, D is dimension of problem. Figure 1 shows the initial population generated by two methods. (a) is the population generated by random numbers, and (b) is generated by Hénon Chaotic Map. It can be seen that (b) is better than (a) in both ergodicity and randomness.
This section uses quantum bits code and quantum rotation gate to improve the population diversity and local search capability of HHO. In 1981, quantum computing was proposed by Richard Feynman who is an American theoretical physicist. The basic unit of information in storage is called as a quantum bit. Each quantum bit may represent 1 or 0, even the superimposed state of 0 and 1, which can be expressed by a linear superposition of two orthogonal basis vectors:
|Ψ⟩=α|0⟩+β|1⟩ | (3.3) |
α and β are probability amplitudes, which represent the linear probability of individual states |0⟩ and |1⟩ respectively and satisfy following conditions:
|α|2+|β|2=1 | (3.4) |
One quantum bit can be expressed as [αβ]. n quantum bits can describe 2n state at the same time. Therefore, for the same size of population, compared with other methods, quantum code can contain more information. Because evaluating optimization algorithm through fitness function have to use a certain value, it is necessary to perform a measurement for each quantum bit that will collapse into a certain value. The mathematical formula is as follows:
|Ψ⟩={|0⟩,r⩽|α|2|1⟩,r>|α|2,r∈(0,1) | (3.5) |
For the purpose of enhancing the population diversity and search accuracy, the position X of individual in population is converted into a quantum bit that can be updated by quantum rotation gate, the process is as follows:
1) Obtain parameter P that is the ratio of individual position X to search space.
P=X(t)−lbub−lb | (3.6) |
For example, position of individual xi ∈ X is (7, 9), ub and lb are 15 and 6 respectively. So, the value of P is as follow:
Pi=xi−lbub−lb=[1913] | (3.7) |
2) Convert a decimal integer IPji = ⌊Pji×1000⌋, i = {1, 2, ..., N}, j = {1, 2, ..., D} into a 10-bit binary number BPji=(b1b2⋯b10), N is population size and D is dimension of problem. So Eq (3.7) can deduce as follow:
IPi=Pi×1000=[111333] | (3.8) |
BPi=[00011011110101001101] | (3.9) |
3) Take a random number r ∈ (0, 1), calculating αk and βk according to Eqs (3.4) and (3.5) respectively, BPji will be converted into a quantum bit sequence that contain 10 quantum bits as follows:
QBji=[α1α2⋯α10β1β2⋯β10] | (3.10) |
The entire population X={xji} can be expressed as {QBji}, i ∈ {1, 2, ..., N}, j ∈ {1, 2, ..., D}. Based on assumption of r = 0.4, Eq (3.9) can be expressed as follow:
QB1i=[0.770.890.840.450.320.950.430.550.220.390.630.450.550.890.950.320.900.840.970.92] | (3.11) |
QB2i=[0.680.590.720.550.780.820.500.450.950.390.730.810.690.840.630.570.870.890.310.92] | (3.12) |
4) Quantum rotation gate is used to change the distribution domain of α and β, and its form is as follows:
U=[cosθ−sinθsinθcosθ] | (3.13) |
θ in Eq (3.13) is rotation angle, and the update process of quantum bit is as follows:
[α′β′]=U[αβ] | (3.14) |
For example, set θ as 0.2, after one time of rotation, QBji in Eqs (3.11) and (3.12) will be as follow:
QB1i=[0.630.780.710.260.120.870.240.370.020.200.770.620.710.960.990.500.970.930.990.98] | (3.15) |
QB2i=[0.520.420.570.370.640.690.320.260.870.200.850.910.820.930.770.720.950.960.490.98] | (3.16) |
5) According to the quantum state updated by Eqs (3.14) and (3.5), the quantum bit QBji is collapsed into individual position X′={xji}′, the fitness of function f(X′) is calculated, as well as update XPrey(t). So, QBji in Eqs (3.15) and (3.16) can be convert to BPi as follow:
BPi=[10011011111111001101] | (3.17) |
6) If the count of rotations does not reach the maximum m, return to Step 4).
Since the measurement, collapse and rotation of the quantum bit will increase the time consumption, in order to balance the accuracy and efficiency of optimization, the quantum rotation operation is only performed in the situations that adopting soft besiege strategies or hard besiege strategies, meanwhile, the curve convergence state is as smooth state. Compared with HHO, this method gives the search agent more opportunities to take delicate movement, so it is more likely to get the better results.
Nelder-Mead technique is proposed by John Nelder and Mead Roger in 1965, it is a kind of heuristic search method with simplex concept that can converge to a non-stable point for the problem that can be solved by an alternative method. It is usually used for the nonlinear optimization problem of which derivative is unknown and issues for finding the minimum or maximum value of objective function in a multi-dimensional space [36].
The NM method constructs an initial simplex containing a given point, and then the point with the worst value of function is replaced by way of reflection, expansion and contraction. If three methods mentioned above are all failed, contraction reduce the radius of the simplex to small enough. The modified NM method is introduced in global search phase to enhance search ability of HHO. The steps are as follows:
1) Set XNM-Optimal is the optimal point in population, XNM-Suboptimal is the suboptimal point, and XNM-Worst and XNM-Subworst are the worst point and the point that is next to the worst point respectively.
2) Generate a group of new positions XNM-Elite [17] with parameters of XNM-Optimal, XNM-Suboptimal, XNM-Subworst and XNM-Worst, the formula is as follows:
XNM - Elite=[f(XNM - Suboptimal )f(XNM - Suboptimal )+f(XNM - Optimal )⋅XNM - Suboptimal+f(XNM - Optimal )f(XNM - Suboptimal )+f(XNM - Optimal )⋅XNM - Suboptimalf(XNM - Suboptimal )f(XNM - Suboptimal )+f(XNM - Worst )⋅XNM - Suboptimal+f(XNM - Worst )f(XNM - Suboptimal )+f(XNM - Worst )⋅XNM - Suboptimalf(XNM - Subworst )f(XNM - Subworst )+f(XNM - Optimal )⋅XNM - Subworst+f(XNM - Optimal )f(XNM - Subworst )+f(XNM - Optimal )⋅XNM - Subworstf(XNM - Subworst )f(XNM - Subworst )+f(XNM - Worst )⋅XNM - Subworst+f(XNM - Worst )f(XNM - Subworst )+f(XNM - Worst )⋅XNM - Subworst] | (3.18) |
3) Let XNM = {XNM-EliteT, XNM-Optimal, XNM-Suboptimal, XNM-Worst, XNM-Subworst} denote the list of points in the simplex.
4) Sort the points XNM in the simplex from lowest function value to highest one. Update XNM-Optimal, XNM-Subworst, XNM-Worst at each step in the iteration.
5) Set reflected point XNMr and expanded point XNMe as follows:
XNMr=2⋅Xm−XNM−worst | (3.19) |
XNMe=Xm+2⋅(Xm−XNM−worst) | (3.20) |
In Eqs (3.19) and (3.20), Xm is the average position of population in Eq (2.2).
6) If f(XNMr) < f(XNM-Optimal), XNM-Worst is replaced according to follow rules:
a. If f(XNMe) < f(XNMr), accept XNMe, named as "Expansion".
b. Otherwise, accept XNMr, named as "Reflection <2> ".
7) If f(XNM-Optimal) ≤ f(XNMr) < f(XNM-Subworst), XNM-Worst is replaced by XNMr, named as "Reflection <1> ",
8) If f(XNM-Subworst) ≤ f(XNMr), perform "Contraction" by follow rules:
a. If f(XNMr) < f(XNM-Worst), calculate the contracted point XNMc1 as follows:
XNMc1=Xm+(XNMr−Xm)×0.5 | (3.21) |
If f(XNMc1) < f(XNMr), XNM-Worst is replaced by XNMc1, named as "Outside contraction", otherwise, continue with Step 9).
b. If f(XNMr) ≥ f(XNM-Worst), set the contracted point XNMc2 as follows:
XNMc2=Xm+(XNM−worst−Xm)×0.5 | (3.22) |
If f(XNMc2) < f(XNM-Worst), accept XNMc2, named as "Inside contraction", otherwise, continue with Step 9). The individual Xworst corresponding to XNM-Worst in population is updated.
9) Update list of XNM:
v(i)=XNM(1)−XNM(i)−XNM(1)2 | (3.23) |
Calculate f(v(i)), i = 2, 3, ..., 8. The simplex at the next iteration is {XNM(1), v(2), ..., v(8)}, named as "Shrink".
10) Go back to Step 4), until the stop condition is met.
11) If f(XNM-Optimal) < f(XPrey(t)), set Fitness(t) = f(XNM-Optimal), and update XPrey(t) as XNM-Optimal.
Take an example, set cost function as:
f(x,y)=100×(y−x2)2+(1−x)2 | (3.24) |
For convenience of understanding and expressing the procedure of NM method, the simplex in this example contains only 3 points, XNM = {XNM-Optimal, XNM-Worst, XNM-Subworst}, initial values of them are [-2.9723, 0.1679], [-1.9723, 0.1679] and [-2.9723, 1.1679]. From Step 1) to Step 3) are skipped, after 19 times of iteration, data are shown in Table 1.
Iteration No. | f(XNM-Optimal) | f(XNM-Subworst) | f(XNM-Worst) | f(XNMe) | f(XNMr) | f(XNMc1) | f(XNMc2) | Action |
1 | 1394.21 | 5893.56 | 7526.89 | — | — | — | — | Sort |
1394.21 | 5893.56 | 31.09 | 31.09 | 749.80 | — | — | Expansion | |
2 | 31.09 | 1394.21 | 5893.56 | 31.09 | 749.80 | — | — | Sort |
31.09 | 1394.21 | 3.54 | 3.54 | 21.96 | — | — | Expansion | |
3 | 3.54 | 31.09 | 1394.21 | 3.54 | 21.96 | — | — | Sort |
3.54 | 31.09 | 8.22 | 3.54 | 8.22 | — | — | Reflection <1> | |
4 | 3.54 | 8.22 | 31.09 | 3.54 | 8.22 | — | — | Sort |
3.54 | 1.25 | 86.88 | 3.54 | 1.39e+4 | — | 188.77 | Shrink | |
5 | 1.25 | 3.54 | 86.88 | 3.54 | 1.39e+4 | — | 188.77 | Sort |
1.25 | 3.54 | 71.21 | 3.54 | 1675.13 | — | 71.21 | Inside contraction | |
...... | ...... | |||||||
12 | 0.07 | 0.19 | 0.26 | 3.54 | 3.10 | — | 0.19 | Sort |
0.07 | 0.19 | 0.14 | 3.54 | 0.26 | 0.14 | 0.19 | Outside contraction | |
...... | ...... | |||||||
19 | 0.0103 | 0.0263 | 0.0263 | 3.5432 | 0.0263 | 0.1447 | 0.0263 | Sort |
0.0103 | 0.0263 | 0.0084 | 0.0331 | 0.0084 | 0.1447 | 0.0263 | Reflection <2> |
As shown in Table 1, each iteration possesses 2 lines of data, the top line is the status of f(XNM) after execution of Step 4), the bottom one is the result of Step 9).
1) At the 2nd iteration, fit for Step 6a), so action of "Expansion" is executed, XNM-Worst is changed and f(XNM-Worst) is 3.54 that is shown in the bottom line of 2nd iteration.
2) At the 3rd iteration, fit for Step 7), action of "Reflection <1> " is executed, f(XNM-Worst) is changed to 3.54.
3) At the 4th iteration, fit for Step 9), XNM is updated.
4) At the 5th iteration, fit for Step 8b), action of "Inside contraction" is executed, f(XNM-Worst) will be equal to f(XNMc2).
5) At the 12th iteration, fit for Step 8a), action of "Outside contraction" is executed, f(XNM-Worst) is changed as the values of f(XNMc1).
6) At the 19th iteration, fit for Step 6b), action of "Reflection <2> " is executed, XNM-Worst is equal to XNMr.
Harris hawk is a kind of animals hunting in group. When they encircle prey, there are 2 or 3 hawks stop in one tree at most, it means that position of each hawk is affected by another one. Original HHO does not take the relationship between individuals into consideration. The attraction and repulsion between creatures are introduced into HHO. The attractive force makes hawks to move toward to same prey. The repulsive force keeps hawks at a certain distance and not gather in a single position. The group communication factor gcf is added to the fitness of cost function in order to generate a new fitness for comparison and optimization. The group communication factor gcf of the ith search agent is defined as follows:
gcfi=∑Nk=1[−dattract⋅exp(−ωattract⋅vd)]+∑Nk=1[−hrepel⋅exp(−ωrepel⋅vd)] | (3.25) |
In Eq (3.25), N is population size, dattract is the attraction released by hawk, and ωattract indicates the diffusivity of attraction. hrepel and ωrepel are the influence and width of repellent respectively. The variable vd is the distance parameter between different variables of the ith search agent, which is defined as follows:
vd=∑Dm=1(Xm−Xmi)2 | (3.26) |
In Eq (3.26), D is the dimension of search agent. Xmi is the position of the mth variable of the ith search agent.
Fitnessgcf=Fitnessi+gcfi|i=1,2,…N | (3.27) |
In Eq (3.27), N is population size, Fitnessgcf is fitness value of function that integrated with group communication factor after iteration. Therefore, introduction of gcf improves comprehensiveness and completeness of fitness.
In HHO algorithm, escape energy factor E reflects the search ability for the optimal solution, determines the conversion between global search and local search as well as affects the strategy adopted in exploitation phase. As shown in Eq (2.15), the value of E is depended on the random number E0, it appears as linear decreasing trend including many times of fluctuation during optimization process, which can hardly describe actual change tendency of creatures' energy. Some scholars improved factor E from various of aspects, such as the m-HHO algorithm proposed by Gupta et al. [37] set nonlinear energy factor E as follows in order to obtain more opportunities for exploitation.
E=(Estart - Eend)×exp(−t2(mi×T)2)+Eend | (3.28) |
In Eq (3.28), t is count of iteration, T is the maximum number of iterations, mi is the nonlinear modulation index, and Estart and Eend are the initial and final energy parameter values respectively.
Although m-HHO get better results in exploration phase, the universality and robustness of algorithm are reduced because of abandoning random parameter. So HHO and m-HHO are both not in line with the energy consumption and recovery of prey during escape or actual situation of predator and prey. Prey's physical fitness decreases rapidly with the increase of running distance in escape. It can recover to a certain extent through a short rest. QC-HHO separates the change process of E in HHO into several groups, each group contains corresponding data of E in iteration, that is as following formula:
E1_Sawi=r−i,r∈[50,100],i∈{1,2,...,r} | (3.29) |
E1_Partj = (E1_Saw−r2)×4max(abs(E1_Saw)),j∈{1,2,...,Tr} | (3.30) |
E1={E1_Partj},j∈{1,2,...,Tr} | (3.31) |
E=E0×E1 | (3.32) |
J=E−0.51.5+1 | (3.33) |
In Eq (3.29), r is a random number in the interval of [50, 100] that means the size of each group. In Eq (3.30), j determines the frequency of physical recovery of prey in escape, namely, how many times of rest the prey takes for. E1_Partj is the escape energy factor of each group in whole search process. In Eq (3.32), E0 is in the interval of [-1, 1] that is as same as it of HHO in Eq (2.15). In Eq (2.5) relate to HHO, jump distance of prey is irrelevant to energy. However, according to Eq (3.33), the more energy the prey has, the longer distance it can jump.
As shown in Figure 2, (a) is the change trend of escape energy factor E of original HHO, which is relevant linear, (b) is the curve of E in m-HHO algorithm, and (c) is E of QC-HHO algorithm. It can be seen that the fluctuation of E in each group is getting smaller and smaller. The energy of prey is recovery after short break when it is exhausted. The physical consumption of prey in the process of being chased is decreasing, the upper limit of physical will be lower and lower as time goes on. That is conform to the feature of bio-energy.
This section describes the application of methods mentioned in former sections as form of pseudo code, the corresponding flow chart is shown in Figure 3.
Input: N is population size, T is maximum number of iterations
Output: Position of prey and corresponding fitness value
Execute Eqs (3.1) and (3.2) to initialize the population Xi(i = 1, 2, ..., N).
Initialize the parameters of Nelder-Mead simplex method
While (stopping condition is not met) do
Check state for convergence curve
Execute Eq (3.27) to calculate the fitness values integrating with the group communication factor gcf
for (each hawk Xi) do
Execute Eq (3.25) to calculate the group communication factor gcf
Execute Eq (3.32) to update the escape energy factor E
Execute Eq (3.33) to update the jump strength J
if1 the state of convergence curve is rapid convergence and iteration is in later stage
set E = 1 to force the search enter into exploration
endif1
if2 (|E| ≥ 1) then exploration phase
if3 convergence curve is rapid convergence
Execute Eq (2.1) to update the location
else
Execute Eqs (3.18)–(3.23) to use Nelder-Mead simplex method
endif3
endif2
if4 (|E| < 1) then exploitation phase
if5 (r ≥ 0.5 and |E| ≥ 0.5) then soft besiege
Execute Eq (2.3) to update the location
endif5
if6 (r ≥ 0.5 and |E| < 0.5) then hard besiege
Execute Eq (2.6) to update the location
endif6
if7 (r ≥ 0.5 and it is possible to drop into local optima)
Execute Eqs (3.6), (3.10), (3.13) and (3.14) to execute quantum correction
endif7
if8 (r < 0.5 and |E| ≥ 0.5) then soft besiege with progressive rapid dives
Execute Eq (2.9) to update the location
endif8
if9 (r < 0.5 and |E| < 0.5) then hard besiege with progressive rapid dives
Execute Eq (2.14) to update the location
endif9
endif4
endfor
endwhile
Return XPrey
Set the population size of the QC-HHO is N and iteration rounds is T, D is dimension of problem, the time complexity of algorithm according to the execution steps of the algorithm is analyzed.
Step 1, initialization of hawk population, the time complexity is O(2N × D).
Step 2, initialize the parameters of Nelder-Mead simplex method, the time complexity is O(1).
Step 3, calculate the convergence trend, the time complexity is O(1).
Step 4, calculate the function fitness, and the time complexity is O(1).
Step 5, perform group behavior.
Step 5.1, calculate the group communication factor gcf, the time complexity is O(N).
Step 5.2, calculate the escape energy factor E and jump strength J, the time complexity is O(2).
Step 5.3, judge whether to force search enter into exploration according to the convergence trend, make comparison once, make calculation once, the time complexity is O(2).
Step 5.4, confirm to enter into exploration or exploitation, make comparison once, the time complexity is O(1).
Step 5.5, in exploration phase, compare twice, move once, update parameters of Nelder-Mead simplex method, and the time complexity is O(4).
Step 5.6, in the exploitation phase, compare once to determine the attack strategy, move once, the time complexity is O(1).
Step 5.7, in soft besiege, move once, execute quantum correction, the time complexity is O(20 × D + 12).
Step 5.8, in hard besiege, move once, execute quantum correction, the time complexity is O(20 × D + 12).
Step 5.9, in soft and hard besiege with progressive rapid dives, move once, the time complexity is O(1).
Step 5 will repeat for N times, so its time complexity is O((10 + N + 20 × D + 12) × N).
After the above steps, the time complexity of QC-HHO after T iterations is O(T ×((10 + N + 20 × D + 12) × N)).
The spatial complexity is evaluating indicator of through storage space used by algorithm and analyzed according to the procedures. Set the scale of population is N, the number of iterations is T, and the dimension of function is D. X[N][D] stores the position of population, XPrey[1][D] saves the optimized variable, Fitness keeps the optimal result for fitness of function, gcf is the relationship between individuals in population, NM_X[7][1] stores the parameters of Nelder-Mead simplex method, NM_X_Fitness[2][1] is fitness of function belong to NM simplex method, P[N][D] keeps ratio of individual position X to search space, IP[N][D] stores decimal integer of P, BP[10] are binary data of individual, QB[N][D][2][10] stores quantum bits of population, X'[N][D] stores the position of population after quantum correction. Therefore, the spatial complexity of QC-HHO algorithm is: O(N × D × 20) + 4 × O(N × D) + 2 × O(D) + O(21).
This section makes use of 10 classic benchmark functions and 30 CEC2014 benchmark functions to test the performance of QC-HHO on the platform of intel i7-4790, 8GB DRAM and MatLab 2012b, and presents the experimental results and analysis.
This section is to evaluate the performance of QC-HHO, taking comparison between QC-HHO and BFO [1], PSO [2], DE [3], HHO [16] as well as other 4 improved HHO algorithms including CEHHO [17], ADHHO [25], CLHHEO [26], DMSDL-HHO [28] on classic benchmark functions.
Classic benchmark functions are grouped into three categories: F1−F4 are uni-modal functions that used to evaluate the capability of exploitation. F5−F8 are multi-modal functions, F9 and F10 are fixed-dimensional multi-modal functions. F5−F10 have more than one local optima, the number of variables increases exponentially, which are used to evaluate the ability of exploration and avoiding the local optima. The figures, names, variable number, variable boundary and theoretical optimal solution of functions are shown in Table 2.
Figure | Function No. | Function Name | Number of Variables | Boundary | Optimal Solution |
![]() |
F1 | Sphere | 30 | [-100,100] | 0 |
![]() |
F2 | Schwefel 1.2 | 30 | [-100,100] | 0 |
![]() |
F3 | Schwefel 2.21 | 30 | [-100,100] | 0 |
![]() |
F4 | Quartic with Noise | 30 | [−1.28, 1.28] | 0 |
![]() |
F5 | Schwefel | 30 | [−500,500] | −418.9829 × 5 |
![]() |
F6 | Rastrigin | 30 | [−5.12, 5.12] | 0 |
![]() |
F7 | Ackley | 30 | [−32, 32] | 0 |
![]() |
F8 | Penalized 1.1 | 30 | [−50, 50] | 0 |
![]() |
F9 | Goldstein-Price | 2 | [−2, 2] | 3 |
![]() |
F10 | Shekel 5 | 4 | [0, 10] | −10.1532 |
In detail, for the tests on classic benchmark functions, the termination criterion is the maximum number of iterations T is 500, the number of populations is 30, the rounds of runs on each function is 30, the maximum number m of quantum rotations is 3, means and standard deviations are collected as support for evaluation and shown in Tables 3 and 4.
Function No. | metrics | BFO | PSO | DE | HHO | QC-HHO |
F1 | mean | 5.67E-05 | 3.89E-01 | 1.01E-13 | 1.13E-97 | 0.00E+00 |
std | 8.46E-06 | 1.29E-01 | 7.61E-14 | 2.48E-97 | 0.00E+00 | |
F2 | mean | 2.66E-04 | 3.54E+01 | 8.50E-11 | 5.89E-79 | 0.00E+00 |
std | 4.50E-05 | 1.46E+01 | 9.14E-11 | 1.81E-78 | 0.00E+00 | |
F3 | mean | 4.34E-03 | 2.61E+00 | 0.00E+00 | 3.11E-50 | 0.00E+00 |
std | 5.68E-04 | 1.29E+00 | 0.00E+00 | 6.55E-50 | 1.01E-101 | |
F4 | mean | 2.50E-04 | 3.55E+00 | 5.63E-03 | 1.97E-04 | 4.23E-05 |
std | 2.16E-04 | 8.49E+00 | 1.48E-03 | 2.05E-04 | 4.18E-05 | |
F5 | mean | -2.01E+03 | -2.74E+03 | -1.12E+04 | -1.26E+04 | -1.44E+04 |
std | 4.40E+02 | 3.61E+02 | 5.75E+02 | 1.68E+00 | 1.85E-12 | |
F6 | mean | 1.11E-02 | 9.82E+01 | 6.92E+01 | 0.00E+00 | 0.00E+00 |
std | 1.72E-03 | 1.26E+01 | 3.88E+01 | 0.00E+00 | 0.00E+00 | |
F7 | mean | 5.85E-03 | 2.56E+00 | 1.18E-07 | 8.88E-16 | 8.88E-16 |
std | 4.33E-04 | 1.13E+00 | 4.96E-08 | 0.00E+00 | 0.00E+00 | |
F8 | mean | 1.56E+00 | 3.43E+00 | 9.76E-15 | 1.03E-05 | 1.57E-22 |
std | 7.01E-03 | 1.10E+00 | 9.85E-15 | 1.33E-05 | 5.57E-48 | |
F9 | mean | 8.40E+00 | 3.00E+00 | 3.73E+00 | 3.00E+00 | 3.00E+00 |
std | 1.14E+01 | 5.91E-04 | 2.57E-15 | 2.29E-07 | 4.38E-02 | |
F10 | mean | -7.91E-01 | -4.15E+00 | -1.17E+01 | -5.05E+00 | -9.97E+00 |
std | 5.20E-01 | 2.40E+00 | 2.89E-06 | 1.64E-02 | 1.56E+00 |
Function No. | metrics | CEHHO | DMSDL-HHO | ADHHO | CLHHEO | QC-HHO |
f1 | ave | 1.0600E-111 | 0.0000E+00 | 2.5300E-196 | 2.9200E-187 | 0.0000E+00 |
std | 5.2400E-111 | 0.0000E+00 | 8.8820E-63 | 0.0000E+00 | 0.0000E+00 | |
rank | 5 | 1 | 3 | 4 | 1 | |
f2 | ave | 2.3400E-89 | 0.0000E+00 | 2.2653E-102 | 9.3000E-116 | 0.0000E+00 |
std | 1.2700E-88 | 0.0000E+00 | 1.1250E-98 | 4.9100E-115 | 0.0000E+00 | |
rank | 5 | 1 | 4 | 3 | 1 | |
f3 | ave | 8.3500E-58 | 0.0000E+00 | 8.9240E-74 | 4.0400E-74 | 0.0000E+00 |
std | 1.9700E-67 | 0.0000E+00 | 1.1230E-70 | 1.5900E-73 | 1.0100E-101 | |
rank | 5 | 1 | 4 | 3 | 1 | |
f4 | ave | 1.4900E-04 | 1.3380E-04 | 4.2924E-05 | 4.0479E-05 | 4.2300E-05 |
std | 1.2800E-05 | 1.4544E-04 | 4.3058E-05 | 4.3225E-05 | 4.1800E-05 | |
rank | 5 | 4 | 3 | 1 | 2 | |
f5 | ave | -1.3506E+04 | -1.3803E+04 | -1.2730E+04 | -1.3511E+04 | -1.4443E+04 |
std | -1.2600E+04 | 1.7569E+03 | 5.0093E-04 | -1.2691E+04 | 1.8500E-12 | |
rank | 4 | 2 | 5 | 3 | 1 | |
f6 | ave | 0.0000E+00 | 0.0000E+00 | 0.0000E+00 | 0.0000E+00 | 0.0000E+00 |
std | 0.0000E+00 | 0.0000E+00 | 0.0000E+00 | 0.0000E+00 | 0.0000E+00 | |
rank | 1 | 1 | 1 | 1 | 1 | |
f7 | ave | 8.8800E-16 | 8.8800E-16 | 8.8800E-16 | 8.8800E-16 | 8.8800E-16 |
std | 0.0000E+00 | 0.0000E+00 | 0.0000E+00 | 0.0000E+00 | 0.0000E+00 | |
rank | 1 | 1 | 1 | 1 | 1 | |
f8 | ave | 8.4400E-06 | 4.1151E-13 | 1.7461E-24 | 2.8600E-12 | 1.5700E-22 |
std | 2.9600E-09 | 2.7293E-13 | 3.3065E-07 | 3.5400E-12 | 5.5700E-48 | |
rank | 5 | 3 | 1 | 4 | 2 | |
f9 | ave | 3.0000E+00 | 3.0000E+00 | 3.0000E+00 | 3.0000E+00 | 3.0000E+00 |
std | 1.6400E-07 | 3.2183E-15 | 5.9349E-06 | 7.6682E-07 | 4.3800E-02 | |
rank | 1 | 1 | 1 | 1 | 1 | |
f10 | ave | -5.3600E+00 | -1.0151E+01 | -5.4742E+00 | -1.0133E+01 | -9.9654E+00 |
std | 1.1700E+00 | 8.5892E-15 | 2.3396E-01 | 1.0048E-03 | 1.5600E+00 | |
rank | 5 | 1 | 4 | 2 | 3 |
As shown in Table 3, the performance of QC-HHO is significantly better than the other 4 classic optimization algorithms, especially for F1, F2, F3, F6, the theoretical optimal solution can be found. For uni-modal functions F1−F4, compared with the other algorithms, the means and standard deviations of QC-HHO are not only good at final result, but are more stable. In terms of multi-modal functions and fixed-dimensional multi-modal functions, the mean of F5, F7, F9, QC-HHO is similar to HHO and superior to BFO and DE. For F10, QC-HHO obtains satisfactory results, and have a slight advantage over HHO.
As shown in Table 4, theoretical optimal value of F1, F2, F3 are found by QC-HHO and DMSDL -HHO that completely suppress other 3 improved HHO algorithms about best value and mean, they are both ranked first. stability of DMSDL-HHO in F3 is the best among all 5 algorithms. For F4, QC-HHO is ranked second and slightly behind CLHHEO, but it is more stable. For F5, the result of QC-HHO is not only outstanding, but also is more stable substantially. For F6 and F9, 5 optimizers get theoretical optimal values without exception. F7, results of 5 algorithms are identical, but they are not theoretical optimal value. That is means that model of original HHO is not suitable for this function. For F8 and F10, QC-HHO get ranking the second and third. QC-HHO, it can be seen that the capability of solving multi-modal problems is not as good as this of uni-modal ones.
The convergence curves of classic benchmark functions are shown in Figures 4−6. The blue solid line with rectangle is the QC-HHO, the purple solid line is CEHHO, the green solid line is ADHHO, the black dashed line is DMSDL-HHO and the red dot dashed line is CLHHEO.
Figure 4 is convergence curves of uni-modal benchmark functions. Among the 5 improved HHO algorithms, QC-HHO has the best final optimal result. Convergence speed of F1 is the fastest, better than the other 4 algorithms, and finds the theoretical optimal solution. For F2 and F3, although QC-HHO is slower in convergence speed in the early phase of search, speed is significantly faster in the later phase, and a better result is found through quantum rotation correction in the final phase. For F4, QC-HHO fell into the local optima at least 2 times, but all of them successfully jumped out and keep searching the search space to find good solutions.
Figure 5 is comparison of multi-modal benchmark functions. For F5 and F6, QC-HHO has a slightly slower convergence rate than ADHHO and CLHHEO, and achieves a jump out of the local optima in initial phase of F5. For F7, under the premise of identical final result, the convergence speed of QC-HHO is the fastest among 5 algorithms. F8, QC-HHO's convergence speed is not outstanding, and the final result is worse than this of ADHHO.
Figure 6 shows convergence curves of 5 improved HHO in fixed-dimensional multi-modal functions F9 and F10. The final optimization results are at the same order of magnitude, but QC-HHO jumps out of local optima for several times. For F9, the final results of all optimizes are as same as theoretical optimal value, the only differences between them are convergence rate. QC-HHO takes one time of jumping out of local optima to get better result.
This section compares QC-HHO to CEHHO [17], ADHHO [25], CLHHEO [26] and DMSDL-HHO [28] on 30 CEC2014 benchmark functions for further tests of QC-HHO's performance.
For CEC2014 test, population size N is set to 100, the search range is in [−100,100]D, for each function, each algorithm independently run 50 times to get experimental results that composed of mean and standard deviation values on 30, 50, and 100-dimensional CEC2014 benchmark functions in Tables 5, 7 and 9, respectively. Moreover, for convenience of making analysis, ranking statistics for each category are recorded in Tables 6, 8 and 10.
metrics | HHO | CEHHO | DMSDL- HHO | AD-HHO | CLHHEO | QC-HHO | |
f1 | ave | 3.4388E+07 | 6.7119E+07 | 9.2986E+00 | 9.3981E+00 | 6.0859E+04 | 8.2197E+00 |
std | 1.5816E+07 | 1.7777E+07 | 1.3081E+01 | 1.2775E+01 | 2.0189E+07 | 1.5221E+01 | |
f2 | ave | 3.4053E+07 | 1.4616E+08 | 1.9284E-07 | 1.8058E-07 | 7.4597E+05 | 1.7759E-07 |
std | 1.1072E+07 | 5.5324E+07 | 1.0708E-07 | 8.9939E-08 | 4.8320E+07 | 1.1139E-07 | |
f3 | ave | 2.4042E+04 | 3.7078E+04 | 2.6431E-01 | 4.4136E-01 | 5.6404E+02 | 3.6351E-01 |
std | 5.4667E+03 | 1.3501E+04 | 3.0418E-01 | 2.9975E-01 | 1.0972E+04 | 3.4800E-01 | |
f4 | ave | 6.2954E+02 | 8.1557E+02 | 3.0498E-10 | 3.0772E-10 | 1.2677E-10 | 3.3697E-10 |
std | 6.7784E+01 | 2.0063E+02 | 1.0968E-10 | 1.1019E-10 | 1.0309E+02 | 7.3327E-11 | |
f5 | ave | 5.2052E+02 | 5.2059E+02 | 5.1904E+02 | 5.1888E+02 | 5.0843E+02 | 5.1453E+02 |
std | 1.2532E-01 | 1.4775E-01 | 2.7139E-04 | 3.0074E-04 | 1.4283E-01 | 2.3809E-04 | |
f6 | ave | 6.3334E+02 | 6.3626E+02 | 1.0219E+02 | 1.3807E+02 | 4.4244E+02 | 3.9525E+01 |
std | 3.3253E+00 | 2.9463E+00 | 2.4023E+00 | 2.8022E+00 | 3.3249E+00 | 2.3540E+00 | |
f7 | ave | 7.0130E+02 | 7.0243E+02 | 9.3954E-11 | 1.6007E-10 | 9.3062E-07 | 9.4327E-12 |
std | 8.5586E-02 | 6.3357E-01 | 1.8047E-14 | 1.8914E-14 | 1.0667E+00 | 1.5569E-14 | |
f8 | ave | 9.3159E+02 | 9.4180E+02 | 2.2990E+02 | 2.7292E+02 | 1.6638E+02 | 1.6360E+02 |
std | 1.7349E+01 | 2.2440E+01 | 1.0357E+01 | 9.9155E+00 | 1.6189E+01 | 1.8853E+01 | |
f9 | ave | 1.0874E+03 | 1.1007E+03 | 2.8799E+02 | 4.0555E+02 | 3.5026E+02 | 2.7859E+02 |
std | 2.0406E+01 | 2.0994E+01 | 1.3628E+01 | 1.3421E+01 | 1.7502E+01 | 1.4507E+01 | |
f10 | ave | 4.0048E+03 | 4.1507E+03 | 8.6068E+02 | 7.3350E+02 | 1.9039E+03 | 7.7819E+02 |
std | 7.8162E+02 | 6.1751E+02 | 2.8306E+02 | 2.7512E+02 | 2.3224E+02 | 3.0040E+02 | |
f11 | ave | 5.6507E+03 | 5.7285E+03 | 3.1228E+03 | 3.8417E+03 | 5.4454E+03 | 6.0754E+03 |
std | 7.8910E+02 | 5.5742E+02 | 7.7495E+02 | 6.1912E+02 | 5.1104E+02 | 6.9601E+02 | |
f12 | ave | 1.2019E+03 | 1.2021E+03 | 2.5912E+02 | 2.8480E+02 | 1.6350E+02 | 4.3482E+01 |
std | 4.7933E-01 | 6.9743E-01 | 2.9394E-01 | 2.7541E-01 | 6.4237E-01 | 2.7386E-01 | |
f13 | ave | 1.3005E+03 | 1.3005E+03 | 6.2438E+02 | 6.6377E+02 | 1.0466E+03 | 6.1473E+02 |
std | 1.5096E-01 | 1.2744E-01 | 1.0724E-01 | 8.1349E-02 | 1.0359E-01 | 7.3202E-02 | |
f14 | ave | 1.4003E+03 | 1.4004E+03 | 1.0463E+03 | 1.1425E+03 | 1.1245E+03 | 8.6137E+02 |
std | 1.0112E-01 | 2.7234E-01 | 2.5115E-02 | 2.2234E-02 | 3.0647E-01 | 1.5575E-02 | |
f15 | ave | 1.5453E+03 | 1.5601E+03 | 1.5535E+02 | 1.4709E+02 | 1.3600E+02 | 1.2897E+03 |
std | 8.9250E+00 | 1.7244E+01 | 1.1863E+00 | 9.3748E-01 | 2.0126E+01 | 1.2965E+00 | |
f16 | ave | 1.6124E+03 | 1.6123E+03 | 1.1359E+03 | 1.3203E+03 | 7.7656E+02 | 9.7576E+02 |
std | 4.8097E-01 | 5.9799E-01 | 6.7343E-01 | 6.8893E-01 | 6.3623E-01 | 7.4550E-01 | |
f17 | ave | 4.4967E+06 | 5.7408E+06 | 8.6200E+03 | 7.0382E+03 | 5.6425E+04 | 1.0090E+04 |
std | 3.6931E+06 | 3.3646E+06 | 5.5689E+03 | 4.7452E+03 | 2.9065E+06 | 5.0348E+03 | |
f18 | ave | 1.9322E+05 | 7.8069E+05 | 2.5421E+03 | 2.3654E+03 | 7.8574E+03 | 2.8281E+03 |
std | 3.4242E+05 | 7.2558E+05 | 5.2332E+03 | 3.7030E+03 | 7.7741E+05 | 3.5642E+03 | |
f19 | ave | 1.9518E+03 | 1.9926E+03 | 1.8122E+02 | 2.2464E+02 | 1.3694E+03 | 1.5917E+02 |
std | 3.6942E+01 | 3.4779E+01 | 8.3276E-01 | 8.9098E-01 | 3.9001E+01 | 8.6310E-01 | |
f20 | ave | 2.5130E+04 | 4.7393E+04 | 1.1201E+03 | 1.0525E+03 | 1.0623E+03 | 1.6085E+03 |
std | 8.9647E+03 | 7.8229E+03 | 5.6089E+02 | 4.8117E+02 | 8.9803E+03 | 4.6628E+02 | |
f21 | ave | 7.8219E+05 | 7.8782E+05 | 1.0874E+03 | 1.1780E+03 | 6.7445E+04 | 7.9901E+03 |
std | 7.2130E+05 | 7.2787E+05 | 8.7689E+02 | 8.5450E+02 | 8.4029E+05 | 9.6452E+02 | |
f22 | ave | 3.1687E+03 | 3.2757E+03 | 1.3253E+03 | 7.9046E+02 | 5.8064E+02 | 1.4654E+03 |
std | 2.9168E+02 | 1.6299E+02 | 1.0242E+01 | 9.8666E+01 | 1.8406E+02 | 1.0312E+01 | |
f23 | ave | 2.5000E+03 | 2.5000E+03 | 2.5000E+03 | 2.5000E+03 | 2.5000E+03 | 2.5000E+03 |
std | 0.0000E+00 | 0.0000E+00 | 0.0000E+00 | 0.0000E+00 | 0.0000E+00 | 0.0000E+00 | |
f24 | ave | 2.6000E+03 | 2.6000E+03 | 2.6000E+03 | 2.6000E+03 | 2.6000E+03 | 2.6000E+03 |
std | 4.0537E-04 | 0.0000E+00 | 0.0000E+00 | 0.0000E+00 | 0.0000E+00 | 0.0000E+00 | |
f25 | ave | 2.7000E+03 | 2.7000E+03 | 2.7000E+03 | 2.7000E+03 | 2.7000E+03 | 2.7000E+03 |
std | 0.0000E+00 | 0.0000E+00 | 0.0000E+00 | 0.0000E+00 | 0.0000E+00 | 0.0000E+00 | |
f26 | ave | 2.7622E+03 | 2.7403E+03 | 2.0384E+03 | 1.9048E+03 | 1.9035E+03 | 2.5068E+03 |
std | 4.8819E+01 | 5.1410E+01 | 7.3958E-02 | 5.8292E-02 | 5.0192E+01 | 5.5044E-02 | |
f27 | ave | 2.9000E+03 | 2.9000E+03 | 2.9000E+03 | 2.9000E+03 | 2.9000E+03 | 2.9000E+03 |
std | 0.0000E+00 | 0.0000E+00 | 0.0000E+00 | 0.0000E+00 | 0.0000E+00 | 0.0000E+00 | |
f28 | ave | 3.0000E+03 | 3.0000E+03 | 3.0000E+03 | 3.0000E+03 | 3.0000E+03 | 3.0000E+03 |
std | 0.0000E+00 | 0.0000E+00 | 0.0000E+00 | 0.0000E+00 | 0.0000E+00 | 0.0000E+00 | |
f29 | ave | 6.2109E+05 | 2.6613E+06 | 3.2662E+05 | 3.0237E+05 | 2.8801E+05 | 3.0168E+05 |
std | 2.7211E+06 | 0.0000E+00 | 0.0000E+00 | 0.0000E+00 | 0.0000E+00 | 0.0000E+00 | |
f30 | ave | 8.2238E+04 | 4.5278E+04 | 4.4532E+03 | 4.1717E+03 | 4.2684E+04 | 5.9009E+03 |
std | 1.4431E+05 | 0.0000E+00 | 0.0000E+00 | 0.0000E+00 | 0.0000E+00 | 0.0000E+00 |
Unimodal Functions | Simple Multimodal Functions | Hybrid Functions | Composition Functions | All Functions | |
HHO | 5.00 | 5.08 | 5.00 | 2.75 | 4.43 |
CEHHO | 6.00 | 5.77 | 6.00 | 2.63 | 5.00 |
DMSDL-HHO | 2.00 | 2.46 | 2.17 | 1.75 | 2.17 |
ADHHO | 2.67 | 3.08 | 1.67 | 1.38 | 2.30 |
CLHHEO | 4.00 | 2.54 | 3.17 | 1.38 | 2.50 |
QC-HHO | 1.33 | 2.08 | 3.00 | 1.75 | 2.10 |
metrics | HHO | CEHHO | DMSDL- HHO | ADHHO | CLHHEO | QC-HHO | |
f1 | ave | 8.8085E+07 | 1.5267E+08 | 1.6076E+00 | 1.5998E+00 | 1.3999E+00 | 1.3853E+00 |
std | 3.3967E+07 | 6.2054E+07 | 2.3140E+00 | 2.0577E+00 | 2.6593E+00 | 1.6336E-01 | |
f2 | ave | 6.5654E+08 | 2.2991E+09 | 1.3291E+03 | 1.1442E+03 | 1.5104E+03 | 1.0161E+03 |
std | 2.5436E+08 | 7.6478E+08 | 6.7502E+02 | 6.2056E+02 | 6.7748E+02 | 6.5509E+02 | |
f3 | ave | 5.7216E+04 | 8.6216E+04 | 1.5200E-01 | 1.6903E-01 | 1.8400E-01 | 1.8167E-01 |
std | 1.0290E+04 | 1.4523E+04 | 1.6414E-02 | 1.9100E-02 | 2.2674E-02 | 1.7807E-02 | |
f4 | ave | 9.2274E+02 | 1.1512E+03 | 1.1415E-01 | 8.8438E-02 | 7.2032E-02 | 6.6518E-02 |
std | 1.3692E+02 | 1.3042E+02 | 1.4276E+00 | 1.7794E+00 | 1.4689E+00 | 1.4655E+00 | |
f5 | ave | 5.2084E+02 | 5.2079E+02 | 5.4338E+03 | 5.1954E+03 | 4.5483E+03 | 5.9474E+02 |
std | 1.2934E-01 | 1.5010E-01 | 1.7678E-05 | 1.9315E-05 | 2.8812E-05 | 1.6617E-05 | |
f6 | ave | 6.6089E+02 | 6.6458E+02 | 2.9099E+02 | 2.5444E+02 | 2.2542E+02 | 2.6008E+02 |
std | 4.8858E+00 | 3.4152E+00 | 4.4083E+00 | 4.1760E+00 | 4.6698E+00 | 3.1034E+00 | |
f7 | ave | 7.0626E+02 | 7.2412E+02 | 1.5010E+00 | 1.3966E+00 | 1.2825E+00 | 1.7613E+00 |
std | 1.9491E+00 | 6.5846E+00 | 9.1075E-02 | 9.1857E-02 | 7.6984E-02 | 1.0520E-01 | |
f8 | ave | 1.0830E+03 | 1.0884E+03 | 5.9830E+02 | 5.6709E+02 | 2.1714E+02 | 4.8943E+02 |
std | 2.4935E+01 | 2.8755E+01 | 2.2672E+01 | 2.6441E+01 | 2.6903E+01 | 5.4955E+00 | |
f9 | ave | 1.2985E+03 | 1.3268E+03 | 5.3727E+02 | 6.7579E+02 | 6.6299E+02 | 5.4358E+02 |
std | 4.1898E+01 | 3.8579E+01 | 4.4425E+01 | 3.5146E+01 | 5.6706E+01 | 4.3760E+01 | |
f10 | ave | 7.4406E+03 | 8.3494E+03 | 1.5085E+03 | 3.1313E+03 | 2.7626E+03 | 1.8091E+03 |
std | 1.3736E+03 | 9.9589E+02 | 8.3676E+02 | 7.3305E+02 | 8.4150E+02 | 8.0097E+02 | |
f11 | ave | 1.0140E+04 | 1.1356E+04 | 6.5184E+03 | 7.7585E+03 | 9.2076E+03 | 6.2393E+02 |
std | 1.1277E+03 | 1.4072E+03 | 9.3317E+02 | 7.8877E+02 | 7.5315E+02 | 1.0175E+03 | |
f12 | ave | 1.2027E+03 | 1.2029E+03 | 1.1083E+02 | 1.3577E+02 | 2.0733E+02 | 8.4096E+01 |
std | 5.6534E-01 | 7.1506E-01 | 1.2631E-01 | 1.8406E-01 | 1.7612E-01 | 1.0291E-01 | |
f13 | ave | 1.3006E+03 | 1.3006E+03 | 1.1865E+03 | 1.0192E+03 | 1.2148E+03 | 1.9979E+03 |
std | 1.1094E-01 | 1.5253E-01 | 6.3924E-02 | 5.5773E-02 | 5.2991E-03 | 6.2082E-02 | |
f14 | ave | 1.4004E+03 | 1.4004E+03 | 9.4218E+02 | 1.1219E+03 | 2.5971E+02 | 9.4118E+02 |
std | 1.0639E-01 | 1.5366E-01 | 8.4510E-02 | 6.8426E-02 | 5.3345E-03 | 7.6521E-02 | |
f15 | ave | 1.6353E+03 | 2.0286E+03 | 1.6569E+02 | 1.4874E+02 | 1.5910E+02 | 1.6023E+02 |
std | 4.9995E+01 | 4.0222E+02 | 3.8812E+00 | 4.6768E+00 | 3.8753E+00 | 3.5583E+00 | |
f16 | ave | 1.6222E+03 | 1.6222E+03 | 1.5002E+03 | 1.4165E+03 | 1.7790E+03 | 1.3866E+03 |
std | 4.2170E-01 | 5.1725E-01 | 2.9190E-01 | 6.5435E-01 | 6.4365E-01 | 4.4801E-01 | |
f17 | ave | 1.4557E+07 | 3.9310E+07 | 1.8954E+04 | 1.8389E+04 | 2.1112E+04 | 1.6400E+04 |
std | 8.7585E+06 | 1.6772E+07 | 1.0117E+04 | 1.0110E+04 | 8.0640E+03 | 7.4718E+03 | |
f18 | ave | 6.4198E+06 | 2.9993E+07 | 3.6657E+05 | 4.5792E+05 | 4.2545E+05 | 4.7209E+05 |
std | 2.1913E+07 | 5.3636E+07 | 1.8446E+06 | 3.5932E+06 | 3.8297E+06 | 1.6075E+06 | |
f19 | ave | 1.9772E+03 | 2.0031E+03 | 1.2075E+03 | 6.1665E+02 | 6.7387E+02 | 2.0142E+03 |
std | 2.5168E+01 | 3.4978E+01 | 1.4342E+01 | 1.3839E+01 | 1.2625E+01 | 1.5203E+01 | |
f20 | ave | 3.9538E+04 | 6.3072E+04 | 1.8738E+03 | 2.2161E+03 | 2.4283E+03 | 1.8695E+03 |
std | 1.3456E+04 | 1.8238E+04 | 1.1249E+03 | 1.2644E+03 | 1.1763E+03 | 1.2064E+03 | |
f21 | ave | 5.4741E+06 | 9.3026E+06 | 7.8663E+03 | 9.1266E+03 | 1.0157E+04 | 8.7394E+03 |
std | 2.5403E+06 | 5.1269E+06 | 5.8327E+03 | 5.4384E+03 | 4.0745E+03 | 4.4298E+03 | |
f22 | ave | 4.0893E+03 | 4.1887E+03 | 3.3122E+03 | 1.6926E+03 | 1.2617E+03 | 3.4122E+03 |
std | 4.1644E+02 | 4.0711E+02 | 2.9382E+02 | 2.7958E+02 | 3.1192E+02 | 1.4846E+01 | |
f23 | ave | 2.5000E+03 | 2.5000E+03 | 2.5000E+03 | 2.5000E+03 | 2.5000E+03 | 2.5000E+03 |
std | 0.0000E+00 | 0.0000E+00 | 0.0000E+00 | 0.0000E+00 | 0.0000E+00 | 0.0000E+00 | |
f24 | ave | 2.6000E+03 | 2.6000E+03 | 2.6000E+03 | 2.6000E+03 | 2.6000E+03 | 2.6000E+03 |
std | 1.2144E-04 | 0.0000E+00 | 0.0000E+00 | 0.0000E+00 | 0.0000E+00 | 0.0000E+00 | |
f25 | ave | 2.7000E+03 | 2.7000E+03 | 2.7000E+03 | 2.7000E+03 | 2.9068E+03 | 2.7000E+03 |
std | 0.0000E+00 | 0.0000E+00 | 0.0000E+00 | 0.0000E+00 | 0.0000E+00 | 0.0000E+00 | |
f26 | ave | 2.7801E+03 | 2.7900E+03 | 1.6534E+03 | 2.0271E+03 | 1.6382E+03 | 1.7901E+03 |
std | 4.0219E+01 | 3.1478E+01 | 4.7512E+01 | 4.7105E+01 | 5.4754E+01 | 4.0077E+01 | |
f27 | ave | 2.9000E+03 | 2.9000E+03 | 2.9000E+03 | 2.9000E+03 | 2.9000E+03 | 2.9000E+03 |
std | 0.0000E+00 | 0.0000E+00 | 0.0000E+00 | 0.0000E+00 | 0.0000E+00 | 0.0000E+00 | |
f28 | ave | 3.0000E+03 | 3.0000E+03 | 3.0000E+03 | 3.0000E+03 | 3.0000E+03 | 3.0000E+03 |
std | 4.5936E-13 | 0.0000E+00 | 0.0000E+00 | 0.0000E+00 | 0.0000E+00 | 0.0000E+00 | |
f29 | ave | 3.1000E+03 | 3.1000E+03 | 3.1000E+03 | 3.1000E+03 | 3.1000E+03 | 3.1000E+03 |
std | 0.0000E+00 | 0.0000E+00 | 0.0000E+00 | 0.0000E+00 | 0.0000E+00 | 0.0000E+00 | |
f30 | ave | 3.9043E+04 | 8.2553E+04 | 2.1650E+03 | 2.2834E+03 | 2.5747E+03 | 2.7625E+03 |
std | 1.2152E+05 | 0.0000E+00 | 0.0000E+00 | 0.0000E+00 | 0.0000E+00 | 0.0000E+00 |
Unimodal Functions | Simple Multimodal Functions | Hybrid Functions | Composition Functions | All Functions | |
HHO | 5.00 | 4.69 | 4.83 | 2.00 | 4.03 |
CEHHO | 6.00 | 5.38 | 5.83 | 2.25 | 4.70 |
DMSDL-HHO | 2.67 | 3.00 | 2.17 | 1.13 | 2.30 |
ADHHO | 2.33 | 2.85 | 2.33 | 1.50 | 2.33 |
CLHHEO | 3.33 | 2.69 | 2.83 | 1.88 | 2.57 |
QC-HHO | 1.67 | 2.38 | 3.00 | 1.63 | 2.23 |
Metrics | HHO | CEHHO | DMSDL- HHO | AD-HHO | CLHHEO | QC-HHO | |
f1 | ave | 2.9532E+08 | 4.5244E+08 | 2.2065E-01 | 2.3133E-01 | 1.8079E-01 | 2.0389E-01 |
std | 5.9293E+07 | 1.0812E+08 | 4.0428E-01 | 4.2021E-01 | 4.6860E-01 | 3.8815E-01 | |
f2 | ave | 1.4056E+10 | 2.3634E+10 | 4.0215E+01 | 3.2914E+01 | 2.4421E+01 | 2.3105E+01 |
std | 3.4046E+09 | 3.1652E+09 | 1.2254E+02 | 1.3453E+02 | 1.0388E+02 | 1.4275E+02 | |
f3 | ave | 1.5690E+05 | 1.9603E+05 | 4.3587E-01 | 4.0324E-01 | 1.2412E-01 | 3.8466E-01 |
std | 1.8735E+04 | 3.3780E+04 | 9.0178E-02 | 5.3367E-02 | 8.9880E-02 | 6.4643E-02 | |
f4 | ave | 2.5476E+03 | 4.2424E+03 | 1.3242E+01 | 1.1286E+01 | 1.3599E+01 | 8.2205E+00 |
std | 4.0800E+02 | 1.0406E+03 | 1.2069E+01 | 8.2972E+00 | 1.6341E+01 | 9.8653E+00 | |
f5 | ave | 5.2111E+02 | 5.2107E+02 | 5.1999E+02 | 6.5981E+02 | 8.4588E+02 | 5.2108E+02 |
std | 9.4023E-02 | 8.6480E-02 | 3.3689E-06 | 3.8421E-06 | 3.1172E-06 | 3.6386E-06 | |
f6 | ave | 7.4008E+02 | 7.5001E+02 | 4.3865E+02 | 5.1949E+02 | 5.0975E+02 | 5.3346E+02 |
std | 6.5795E+00 | 6.8974E+00 | 6.9352E+00 | 6.4936E+00 | 7.6965E+00 | 8.2792E+00 | |
f7 | ave | 8.4469E+02 | 9.8020E+02 | 1.2370E+00 | 1.0825E+00 | 1.2535E+00 | 1.1447E+00 |
std | 3.1984E+01 | 6.4082E+01 | 5.4277E-01 | 5.3984E-01 | 4.0310E-01 | 4.8305E-01 | |
f8 | ave | 1.5063E+03 | 1.5608E+03 | 1.0793E+03 | 1.0420E+03 | 1.0178E+03 | 3.9801E+02 |
std | 3.2668E+01 | 3.0244E+01 | 5.3529E+01 | 4.3120E+01 | 4.8401E+01 | 4.2877E+01 | |
f9 | ave | 1.8268E+03 | 1.8914E+03 | 1.5135E+03 | 2.6553E+03 | 3.3141E+02 | 1.9302E+03 |
std | 4.5102E+01 | 4.4228E+01 | 7.4349E+01 | 5.5063E+01 | 6.7256E+01 | 4.5389E+01 | |
f10 | ave | 1.7487E+04 | 1.9500E+04 | 8.3093E+03 | 1.9446E+03 | 9.1671E+03 | 1.8913E+03 |
std | 1.8860E+03 | 1.7014E+03 | 8.5620E+02 | 5.3573E+02 | 8.2863E+02 | 6.3636E+02 | |
f11 | ave | 2.2358E+04 | 2.3184E+04 | 1.6779E+04 | 1.5132E+04 | 1.8203E+04 | 2.1426E+04 |
std | 2.1316E+03 | 2.7566E+03 | 1.0591E+03 | 1.0470E+03 | 1.8372E+03 | 9.7728E+02 | |
f12 | ave | 1.2036E+03 | 1.2038E+03 | 1.7679E+02 | 3.4298E+02 | 1.7758E+02 | 2.7543E+02 |
std | 5.1525E-01 | 4.3964E-01 | 1.8670E-01 | 1.7408E-01 | 3.1450E-01 | 2.0032E-01 | |
f13 | ave | 1.3006E+03 | 1.3011E+03 | 1.2036E+03 | 6.9965E+02 | 1.0099E+03 | 5.4023E+02 |
std | 7.3180E-02 | 9.3521E-01 | 6.1230E-02 | 1.0670E-01 | 5.8115E-02 | 5.5391E-02 | |
f14 | ave | 1.4397E+03 | 1.4759E+03 | 1.2487E+03 | 1.4576E+03 | 1.3813E+03 | 1.3914E+03 |
std | 1.0803E+01 | 1.0229E+01 | 3.0977E+00 | 2.7375E+00 | 2.7636E+00 | 2.9033E+00 | |
f15 | ave | 8.0315E+03 | 3.0563E+04 | 1.0476E+03 | 7.5402E+02 | 1.2366E+03 | 6.8471E+02 |
std | 3.4553E+03 | 1.4417E+04 | 4.7003E+02 | 1.7529E+02 | 5.4379E+02 | 1.4072E+02 | |
f16 | ave | 1.6461E+03 | 1.6460E+03 | 1.5723E+03 | 4.4884E+02 | 1.3370E+03 | 3.3899E+02 |
std | 5.3948E-01 | 6.5221E-01 | 6.7085E+00 | 6.9260E+00 | 7.7507E+00 | 6.7595E+00 | |
f17 | ave | 5.9354E+07 | 9.1421E+07 | 4.3274E+04 | 5.5973E+04 | 4.1236E+04 | 5.8317E+04 |
std | 2.0834E+07 | 4.7987E+07 | 8.9080E+03 | 8.2787E+03 | 1.0579E+04 | 1.4855E+04 | |
f18 | ave | 2.5553E+07 | 4.2986E+07 | 3.1724E+04 | 2.6405E+04 | 3.6162E+04 | 4.6724E+04 |
std | 4.0091E+07 | 2.0825E+07 | 1.7080E+04 | 1.8732E+04 | 2.0411E+04 | 5.2549E+03 | |
f19 | ave | 2.2215E+03 | 2.3154E+03 | 1.2729E+03 | 1.1020E+03 | 1.1081E+03 | 1.4420E+03 |
std | 8.0641E+01 | 4.4544E+01 | 7.0559E+01 | 5.6971E+01 | 3.8067E+01 | 4.3033E+01 | |
f20 | ave | 1.1887E+05 | 1.7736E+05 | 1.1082E+04 | 1.1422E+04 | 1.2123E+04 | 9.4987E+03 |
std | 2.4661E+04 | 4.7633E+04 | 4.6661E+03 | 5.1722E+03 | 6.0422E+03 | 6.2754E+03 | |
f21 | ave | 1.9119E+07 | 2.9989E+07 | 1.1519E+04 | 9.7435E+03 | 1.2092E+04 | 9.7915E+03 |
std | 6.3314E+06 | 1.4428E+07 | 3.0529E+03 | 4.5540E+03 | 3.2256E+03 | 4.0206E+03 | |
f22 | ave | 5.8287E+03 | 6.5909E+03 | 3.2905E+03 | 2.8780E+03 | 3.1277E+03 | 3.0934E+03 |
std | 5.7346E+02 | 7.5484E+02 | 4.3025E+02 | 4.2572E+02 | 4.9619E+02 | 4.7700E+02 | |
f23 | ave | 2.5000E+03 | 2.5000E+03 | 2.5000E+03 | 2.5000E+03 | 2.5000E+03 | 2.5000E+03 |
std | 0.0000E+00 | 0.0000E+00 | 0.0000E+00 | 0.0000E+00 | 0.0000E+00 | 0.0000E+00 | |
f24 | ave | 2.6000E+03 | 2.6000E+03 | 2.6000E+03 | 2.6000E+03 | 2.6000E+03 | 2.6000E+03 |
std | 7.2310E-05 | 0.0000E+00 | 0.0000E+00 | 0.0000E+00 | 0.0000E+00 | 0.0000E+00 | |
f25 | ave | 2.7000E+03 | 2.7000E+03 | 2.7000E+03 | 2.7000E+03 | 2.7000E+03 | 2.7000E+03 |
std | 0.0000E+00 | 0.0000E+00 | 0.0000E+00 | 0.0000E+00 | 0.0000E+00 | 0.0000E+00 | |
f26 | ave | 2.8000E+03 | 2.8000E+03 | 2.8000E+03 | 2.8000E+03 | 2.8000E+03 | 2.8000E+03 |
std | 0.0000E+00 | 0.0000E+00 | 0.0000E+00 | 0.0000E+00 | 0.0000E+00 | 0.0000E+00 | |
f27 | ave | 2.9000E+03 | 2.9000E+03 | 2.9000E+03 | 2.9000E+03 | 2.9000E+03 | 2.9000E+03 |
std | 1.3781E-12 | 0.0000E+00 | 0.0000E+00 | 0.0000E+00 | 0.0000E+00 | 0.0000E+00 | |
f28 | ave | 3.0000E+03 | 3.0000E+03 | 3.0000E+03 | 3.0000E+03 | 3.0000E+03 | 3.0000E+03 |
std | 9.1873E-13 | 0.0000E+00 | 0.0000E+00 | 0.0000E+00 | 0.0000E+00 | 0.0000E+00 | |
f29 | ave | 3.1000E+03 | 3.1000E+03 | 3.1000E+03 | 3.1000E+03 | 3.1000E+03 | 3.1000E+03 |
std | 0.0000E+00 | 0.0000E+00 | 0.0000E+00 | 0.0000E+00 | 0.0000E+00 | 0.0000E+00 | |
f30 | ave | 3.2000E+03 | 3.2000E+03 | 5.3231E+02 | 1.3189E+02 | 4.7449E+02 | 4.1959E+02 |
std | 0.0000E+00 | 0.0000E+00 | 0.0000E+00 | 0.0000E+00 | 0.0000E+00 | 0.0000E+00 |
Unimodal Functions | Simple Multimodal Functions | Hybrid Functions | Composition Functions | All Functions | |
HHO | 5.00 | 4.77 | 5.00 | 1.50 | 3.97 |
CEHHO | 6.00 | 5.46 | 6.00 | 1.50 | 4.57 |
DMSDL-HHO | 3.67 | 2.46 | 2.67 | 1.38 | 2.33 |
ADHHO | 3.33 | 2.92 | 1.67 | 1.00 | 2.20 |
CLHHEO | 1.33 | 3.08 | 2.83 | 1.25 | 2.37 |
QC-HHO | 1.67 | 2.31 | 2.83 | 1.13 | 2.03 |
As shown in Tables 5 and 6, the results obtained in 30-dimensional CEC2014 benchmark functions show that QC-HHO obtains 15 times ranking the first, 5 times ranking the second, 4 times ranking the third, 5 times ranking the fourth and 1 time ranking the sixth, and no ranking the fifth, its average ranking is 2.1 which is less than this of any other optimizers. DMSDL-HHO and ADHHO compute the average rankings are 2.17 and 2.30 respectively, which are also relatively competitive. The reason why QC-HHO can be the top one is that it is extremely good at Unimodal Functions and Simple Multimodal Functions. QC-HHO is outstanding in average ranking of these two categories, it is means quantum correction mechanism and modified Nelder-Mead simplex method guarantee QC-HHO can find better solution in exploration and exploitation phase by precise or long-distance movement, even if other optimizers are incapable of get better results. Also, the modified escape energy factor E makes the conversion between global and local search at right time. Distribution of initial population generated by Hénon Chaotic Map makes search more accurate. Uniform and moderate distance between individuals is good for group communication factor play a more effective role in the process of population regeneration. On the other side, intermediate level optimization ability result in QC-HHO is surpassed by DMSDL-HHO and ADHHO in categories of Hybrid Functions and Composition Functions.
As shown in Tables 7 and 8, on the 50-dimensional CEC2014 benchmark functions, top one is also QC-HHO that obtains 14 times ranking the first, 5 times ranking the second, 5 times ranking the third, 4 times ranking the fourth and 2 time ranking the sixth, and no ranking the fifth, its average ranking is 3.23 that is more than this of 30-dimension to some extent. No.2 to No.4 positions are also DMSDL-HHO, ADHHO and CLHHEO. The competitiveness of HHO and CEHHO are relatively weak in comparison to the others.
As shown in Tables 9 and 10, on the 100-dimensional CEC2014 benchmark functions, QC-HHO obtains 15 times ranking the first, 6 times ranking the second, 3 times ranking the third, 5 times ranking the fourth and 1 time ranking the fifth, and no ranking the sixth, its average ranking is 2.03 that is better than this of 30 and 50-dimension. Increase of dimension makes QC-HHO take the advantage of distribution of initial population effectively. Results of Composition Functions are obviously promoted. Higher dimension makes taking long-distance movement of individuals by Nelder-Mead simplex method easier, be instrumental in bypass the local optima. As the result, the final solution is better than lower-dimensional population.
According to the analysis aforementioned, QC-HHO has good performance in solution accuracy and more reliable scalability than other competitors, be able to get the most times on ranking the first, and its average rankings are always the highest in category of all functions. Specifically, QC-HHO achieves the highest average ranking on categories of Unimodal Functions and Simple Multimodal Function, however, for Hybrid Functions and Composition Functions, QC-HHO is incapable of achieving the top one, just position in the second or the third, that proves that QC-HHO has a robust exploration and exploitation capability for finding an optimal solution, at the same time, it need to be enhanced in future study.
This section takes analysis about efficiency and time consumption of QC-HHO on classic benchmark functions and CEC2014 benchmark functions. The results are shown in Tables 11 and 12 that correspond with the testing data in Tables 3−10.
Function No. | HHO | CEHHO | DMSDL-HHO | ADHHO | CLHHEO | QC-HHO |
F1 | 0.1711 (1) | 0.1898 (4) | 0.1979 (5) | 0.1994 (6) | 0.1855 (3) | 0.1851 (2) |
F2 | 1.0354 (4) | 1.0333 (3) | 1.0402 (5) | 1.0536 (6) | 1.0280 (2) | 1.0240 (1) |
F3 | 0.2127 (2) | 0.2134 (4) | 0.2130 (3) | 0.2252 (6) | 0.2161 (5) | 0.2120 (1) |
F4 | 0.3470 (1) | 0.3969 (5) | 0.3489 (3) | 0.3998 (6) | 0.3732 (4) | 0.3483 (2) |
F5 | 0.3200 (2) | 0.3387 (4) | 0.3172 (1) | 0.3529 (6) | 0.3415 (5) | 0.3380 (3) |
F6 | 0.2748 (2) | 0.2853 (4) | 0.2854 (5) | 0.2900 (6) | 0.2839 (3) | 0.2740 (1) |
F7 | 0.2775 (2) | 0.3013 (5) | 0.2782 (3) | 0.2967 (4) | 0.3072 (6) | 0.2770 (1) |
F8 | 0.8146 (1) | 0.8694 (6) | 0.8476 (5) | 0.8259 (2) | 0.8385 (4) | 0.8381 (3) |
F9 | 0.1752 (1) | 0.1950 (6) | 0.1947 (4) | 0.1909 (3) | 0.1889 (2) | 0.1948 (5) |
F10 | 0.4427 (1) | 0.4727 (5) | 0.4679 (3) | 0.4762 (6) | 0.4427 (1) | 0.4720 (4) |
ave | 0.4071 | 0.4296 | 0.4191 | 0.4311 | 0.4206 | 0.4163 |
rank | 1 | 5 | 3 | 6 | 4 | 2 |
HHO | CEHHO | DMSDL-HHO | ADHHO | CLHHEO | QC-HHO | |
f1 | 2.5712 (1) | 3.8191 (6) | 3.1869 (4) | 3.7112 (5) | 2.9971 (3) | 2.9932 (2) |
f2 | 2.2815 (1) | 3.3411 (4) | 3.0629 (3) | 3.6919 (6) | 3.5918 (5) | 3.0009 (2) |
f3 | 2.3558 (1) | 3.3897 (3) | 4.0936 (4) | 4.2559 (5) | 4.2625 (6) | 3.0685 (2) |
f4 | 2.2709 (1) | 3.4221 (6) | 3.1982 (3) | 3.2618 (4) | 3.3069 (5) | 3.0166 (2) |
f5 | 2.6414 (1) | 3.8369 (4) | 3.4979 (3) | 3.9471 (5) | 3.9995 (6) | 3.4643 (2) |
f6 | 15.0265 (1) | 16.5892 (6) | 15.3747 (3) | 15.4918 (4) | 15.7313 (5) | 15.1352 (2) |
f7 | 2.4981 (1) | 3.6428 (3) | 3.8173 (5) | 3.8433 (6) | 3.7917 (4) | 3.5909 (2) |
f8 | 2.3186 (2) | 3.4396 (5) | 2.1830 (1) | 2.3421 (3) | 3.5208 (6) | 3.3858 (4) |
f9 | 2.5208 (1) | 3.6129 (4) | 3.8699 (5) | 3.8792 (6) | 3.5934 (2) | 3.5991 (3) |
f10 | 2.5110 (1) | 3.5748 (5) | 3.5401 (4) | 3.8220 (6) | 3.4978 (2) | 3.5326 (3) |
f11 | 2.7039 (1) | 3.8528 (3) | 4.1015 (6) | 3.9709 (5) | 3.9666 (4) | 3.8243 (2) |
f12 | 5.9112 (3) | 8.4490 (4) | 5.6371 (1) | 9.0890 (6) | 5.7282 (2) | 8.5186 (5) |
f13 | 2.3970 (1) | 3.4216 (4) | 4.7038 (5) | 5.1111 (6) | 3.1425 (2) | 3.3602 (3) |
f14 | 2.3843 (1) | 3.4155 (5) | 2.4024 (3) | 2.4702 (4) | 3.4258 (6) | 2.3998 (2) |
f15 | 2.5474 (1) | 3.7753 (3) | 4.1422 (5) | 3.9425 (4) | 3.7215 (2) | 4.6415 (6) |
f16 | 2.6729 (3) | 4.2012 (5) | 2.4023 (2) | 4.4965 (6) | 2.3619 (1) | 2.9426 (4) |
f17 | 2.6649 (1) | 3.9537 (3) | 4.2704 (5) | 4.3114 (6) | 3.9855 (4) | 3.8294 (2) |
f18 | 2.4950 (1) | 3.5390 (2) | 4.4723 (5) | 4.5069 (6) | 4.3060 (4) | 3.8235 (3) |
f19 | 4.7688 (1) | 7.0241 (4) | 7.1387 (5) | 7.5082 (6) | 6.9847 (2) | 7.0149 (3) |
f20 | 2.5339 (1) | 3.6043 (2) | 4.3699 (5) | 4.4119 (6) | 4.2767 (4) | 3.7216 (3) |
f21 | 2.6110 (1) | 3.6399 (4) | 3.9836 (6) | 3.7167 (5) | 3.4723 (3) | 3.3203 (2) |
f22 | 2.9906 (1) | 4.2424 (4) | 4.2634 (5) | 4.2992 (6) | 4.2040 (3) | 4.1862 (2) |
f23 | 3.6790 (1) | 5.3735 (4) | 4.3400 (2) | 5.4874 (5) | 5.6162 (6) | 5.2882 (3) |
f24 | 3.1085 (1) | 4.3348 (4) | 5.1338 (5) | 5.1898 (6) | 4.2848 (3) | 4.2692 (2) |
f25 | 3.5959 (1) | 5.0386 (2) | 6.7478 (6) | 5.1213 (3) | 5.2570 (5) | 5.2076 (4) |
f26 | 16.3414 (1) | 21.0678 (4) | 17.5273 (2) | 21.8697 (5) | 22.3253 (6) | 20.3958 (3) |
f27 | 16.4166 (1) | 21.0634 (4) | 19.1805 (2) | 19.5642 (3) | 21.5892 (5) | 22.3870 (6) |
f28 | 4.2274 (1) | 5.9362 (2) | 8.2345 (6) | 6.0785 (3) | 6.4602 (4) | 8.0234 (5) |
f29 | 5.6623 (1) | 7.8752 (5) | 7.5715 (4) | 7.9956 (6) | 7.4263 (3) | 7.3215 (2) |
f30 | 3.6760 (1) | 5.3156 (6) | 4.7602 (3) | 4.8526 (4) | 4.6273 (2) | 5.1187 (5) |
Ave | 4.3461 | 5.9264 | 5.7069 | 6.0747 | 5.8485 | 5.8127 |
rank | 1 | 5 | 2 | 6 | 4 | 3 |
In order to assess the computational complexity of QC-HHO, the mean values of the runtime over all runs of each algorithm on classic benchmark functions and 30-dimensional CEC2014 benchmark function are recorded in Tables 11 and 12. The numbers in the brackets behind the time values are the rank of computational complexity for every function.
The runtime taken by 6 kinds of optimizers to find the solutions for F1–F10 of classic benchmark functions are listed in Table 11. As per results in the table, QC-HHO shows a reasonably fast and competitive performance in finding the best solutions compared to most of optimizers for uni-modal cases. It takes 4 ranking the first, 2 ranking the second, 3 ranking the third, 1 ranking the fourth, 1 rank fifth, which is belong to moderate levels. F4 is an exception, all 5 improved HHO take more time than HHO, but QC-HHO has relatively good performance. For multi-modal and fixed-dimensional multi-modal cases F5−F10, the search efficiency of QC-HHO is slightly lower than this of HHO, excluding for F7. This result imputes to execution of quantum correction in exploitation phase. Quantum correction can take more exact and accurate search to bypass optima, but it is a kind of time-consuming operation. On the other side, QC-HHO is superior to most of other 4 improved HHO algorithms in most cases. As a whole, although QC-HHO takes less computational cost only for part of uni-modal functions, it performs faster than HHO, CEHHO, DMSDL-HHO, ADHHO, CLHHEO. Because of quantum correction mechanism and modified Nelder-Mead simplex method, time consumption of QC-HHO on multi-modal functions is very high.
Each algorithm's average runtime results on all functions, and the ranking results according to the average runtime are shown in the last two rows. The results in Table 12. Because procedures of CEHHO, DMSDL-HHO, ADHHO, CLHHEO and QC-HHO are all more complicated than original HHO, the runtime of most cases are longer than these of HHO. Average runtime of DMSDL-HHO and QC-HHO are 5.7069 and 5.8127 respectively, the rank of QC-HHO is 3 that is lower than this of DMSDL-HHO. However, it is just lower in 2%, at the same time, the mean for QC-HHO on the 30-dimensional CEC2014 benchmark functions is higher than this DMSDL-HHO in 8%. It is worth of exchange 2% of the time cost to 8% of the performance improvement. Furthermore, QC-HHO spend more time than other optimizers in Hybrid Functions and Composition Functions, QC-HHO just rank third and fourth. Because the functions in these two categories are much more complicated than Unimodal Functions and Simple Multimodal Functions, quantum correction as a kind of time-consuming operation will be executed more frequently. Also, in order to bypass the local optima, individual need to move in long distance by modified Nelder-Mead simplex method. These two optimization mechanisms are both increase the computational complexity.
In order to investigate the significant differences between the results of proposed QC-HHO versus other optimizers and provide more accurate and reliable conclusions, Wilcoxon rank-sum test with 5% degree is carefully performed. Tables 13 and 14 shows the attained p-values of the Wilcoxon rank-sum test with 5% significance.
HHO | BFO | WOA | PSO | DE | CEHHO | DMSDL- HHO | ADHHO | CLHHEO | |
F1 | 9.40E-12 | 9.40E-12 | 9.40E-12 | 9.40E-12 | 9.40E-12 | 9.40E-12 | 9.40E-12 | 9.40E-12 | 9.40E-12 |
F2 | 2.26E-11 | 2.26E-11 | 2.26E-11 | 2.26E-11 | 2.26E-11 | 2.26E-11 | 2.26E-11 | 2.26E-11 | 2.26E-11 |
F3 | 3.02E-11 | 3.02E-11 | 3.02E-11 | 3.02E-11 | 3.02E-11 | 3.02E-11 | 3.02E-11 | 3.02E-11 | 3.02E-11 |
F4 | 4.91E-11 | 3.03E-11 | 1.17E-11 | 3.02E-11 | 3.02E-11 | 4.71E-11 | 4.79E-11 | 4.66E-11 | 4.97E-11 |
F5 | 1.72E-12 | 1.72E-12 | 1.72E-12 | 1.72E-12 | 1.72E-12 | 1.72E-12 | 1.72E-12 | 1.72E-12 | 1.72E-12 |
F6 | 1.21E-12 | 1.21E-12 | 1.21E-12 | 1.21E-12 | 1.21E-12 | 1.21E-12 | 1.21E-12 | 1.21E-12 | 1.21E-12 |
F7 | 1.21E-12 | 1.21E-12 | 3.63E-09 | 1.21E-12 | 1.21E-12 | 1.21E-12 | 1.21E-12 | 1.21E-12 | 1.21E-12 |
F8 | 1.21E-12 | 1.21E-12 | 1.21E-12 | 1.21E-12 | 1.21E-12 | 1.21E-12 | 1.21E-12 | 1.21E-12 | 1.21E-12 |
F9 | 3.02E-11 | 1.07E-07 | 3.69E-11 | 1.46E-10 | NaN | 2.99E-11 | 2.98E-11 | 2.92E-11 | 2.80E-11 |
F10 | 3.69E-11 | 3.02E-11 | 9.76E-10 | 4.62E-10 | 9.92E-10 | 3.76E-11 | 3.77E-11 | 3.81E-11 | 3.53E-11 |
HHO | CEHHO | DMSDL-HHO | ADHHO | CLHHEO | |
f1 | 1.7705E-08 | 5.2425E-09 | 4.6262E-05 | 1.1551E-03 | 4.6508E-05 |
f2 | 3.5327E-09 | 2.7663E-09 | 4.7363E-05 | 4.7113E-05 | 4.3478E-05 |
f3 | 8.7391E-07 | 6.3286E-06 | 4.7284E-05 | 1.1551E-03 | 4.7527E-05 |
f4 | 1.0382E-06 | 1.2266E-05 | 4.8506E-05 | 4.7113E-05 | 4.8058E-05 |
f5 | 2.6731E-01 | 9.0608E-01 | 2.5354E-01 | 2.5354E-01 | 2.5354E-01 |
f6 | 2.9196E-05 | 4.7397E-05 | 4.5470E-04 | 4.9405E-04 | 4.5264E-04 |
f7 | 2.0927E-07 | 9.0425E-06 | 4.7493E-05 | 6.0475E-05 | 5.0695E-05 |
f8 | 4.8206E-04 | 3.0793E-03 | 3.8648E-05 | 9.1699E-03 | 3.8831E-05 |
f9 | 1.6326E-04 | 2.2196E-04 | 5.7790E-04 | 4.6421E-04 | 5.7327E-04 |
f10 | 2.3410E-05 | 1.4001E-03 | 9.3708E-04 | 6.5117E-03 | 9.6393E-04 |
f11 | 5.1528E-04 | 8.9460E-03 | 5.3768E-05 | 1.1551E-03 | 5.3884E-05 |
f12 | 2.8497E-04 | 5.5144E-04 | 4.7290E-05 | 4.7113E-05 | 4.7754E-05 |
f13 | 7.1943E-03 | 1.8116E-03 | 9.5190E-03 | 4.2925E-01 | 9.5909E-03 |
f14 | 2.1220E-03 | 5.1121E-03 | 5.4286E-05 | 1.4169E-03 | 5.5851E-05 |
f15 | 2.6195E-03 | 2.1976E-04 | 4.6889E-05 | 4.7113E-05 | 4.7320E-05 |
f16 | 1.1162E-04 | 2.4663E-03 | 5.6092E-04 | 4.9265E-04 | 5.5844E-04 |
f17 | 2.4221E-05 | 9.3334E-05 | 4.7033E-05 | 1.1551E-03 | 3.8066E-05 |
f18 | 4.1052E-04 | 4.0984E-02 | 5.2596E-05 | 1.5099E-02 | 5.2665E-05 |
f19 | 1.9152E-03 | 4.8671E-03 | 4.9178E-05 | 4.2925E-04 | 5.2957E-05 |
f20 | 8.5933E-05 | 4.4240E-04 | 4.6667E-05 | 4.7113E-05 | 4.6744E-05 |
f21 | 9.6968E-05 | 1.5948E-03 | 4.7318E-05 | 4.7113E-05 | 5.3494E-05 |
f22 | 1.1347E-04 | 8.9985E-03 | 4.6991E-05 | 4.7113E-05 | 4.6674E-05 |
f23 | 6.0998E-03 | 3.6646E-04 | 1.7514E-02 | 1.2542E-04 | 1.8586E-02 |
f24 | 4.7882E-04 | 2.1092E-05 | 3.0202E-03 | 4.7113E-05 | 3.2206E-03 |
f25 | 3.5328E-03 | 7.4526E-03 | 2.4097E-05 | 9.8325E-05 | 2.4222E-05 |
f26 | 8.3156E-04 | 5.4782E-03 | 2.5678E-02 | 4.7113E-05 | 2.5918E-02 |
f27 | 1.1901E-03 | 1.1267E-02 | 2.2684E-05 | 1.5890E-04 | 2.2524E-05 |
f28 | 1.0185E-03 | 7.8966E-03 | 2.6043E-03 | 4.7113E-05 | 2.2437E-03 |
f29 | 7.1010E-05 | 5.6701E-03 | 1.1766E-02 | 4.7113E-05 | 1.0596E-02 |
f30 | 1.4942E-02 | 4.1432E-02 | 4.7113E-05 | 4.7113E-05 | 5.1618E-05 |
This section is applied to problem of gas leakage source localization. The results of QC-HHO are compared to various modified optimizers proposed in previous studies.
This problem can be described mathematically as follows:
Consider: z = [x, y, q]
Minimize: f(z) = 9∑i = 1[CiSersorData − CiTryDataMax(C SersorData i, CiTryData )]2 | (5.1) |
Variable range:
0⩽x⩽50⩽y⩽50<q<1000 |
Where
CTryData(x,y)=q2πuDyDzexp[(ySensor−y)22D2y]×ξ | (5.2) |
{Dy=0.04xSensor(1+0.0001xSensor)−0.5Dz=0.016xSensor(1+0.0003xSensor)−0.5 | (5.3) |
In Eq (5.1), CiSensorData is the data of gas concentration collected by the ith sensor. CiTryData is the data that is predicted by Gaussian puff model in Eqs (5.2) and (5.3). f(z) means the deviation of concentration value between predicted by Gaussian puff model and measured by sensor. The smaller f(z), the closer to actual gas leakage position. ξ is Eq (5.2) is random error simulated by white Gaussian noise with standard deviation of σ2wge. XSensor and YSensor are the location of sensor.
The experimental site is set as Figure 7, there are 9 sensors set in it. Assuming the coordinate of gas leakage sources is (2, 3), gas release rate q is 30ml/min. the mean velocity of wind speed is 12.50 cm/s. The standard deviation σwge of measurement error is set to 0.5, the final positioning results are shown in Table 15.
(x, y) | q | Optimal Cost (s) | Position deviation (%) | Release rate deviation (%) | |
QC-HHO | (1.98, 2.97) | 30.21 | 193.49 | 0.036 | 0.7 |
CLHHEO | (2.05, 3.02) | 31.04 | 194.05 | 0.042 | 3.47 |
ADHHO | (1.97, 3.04) | 29.56 | 202.01 | 0.041 | 1.47 |
DMSDL-HHO | (2.02, 2.96) | 30.28 | 200.21 | 0.05 | 0.93 |
CEHHO | (1.93, 2.92) | 28.97 | 196.77 | 0.092 | 3.43 |
HHO | (1.93, 2.92) | 28.39 | 190.21 | 0.117 | 5.37 |
Although the index of optimal cost of QC-HHO is not outstanding in all optimizer, the deviations of position and release rate are excellent and smaller than these of others. Good results are obtained at an acceptable cost of time.
This paper briefly explains the principles of classic HHO and proposes QC-HHO that includes 5 aspects of improvement: optimizing the distribution of initial populations by Hénon Chaotic Map, promoting local search and population diversity by quantum correction, enhancing global search performance by Nelder-Mead simplex method, describing the relationship between individuals by group communication factor, improving the selection of strategies in exploitation phase as well as occasion of conversion between global and local search by modified escape energy factor E based on biological energy consumption. A test was conducted on 10 classic benchmark functions and 30 CEC2014 benchmark functions to analyze exploration, exploitation, capability of jumping out of local optima, and convergence feature of the proposed algorithm. The data and convergence curve show that QC-HHO is competitive with other optimization algorithms in finding the theoretical optimal solution, it improves the efficiency and robustness of original HHO. On the other hand, the performance of the multi-modal benchmark function is not as good as that of uni-modal benchmark function, which needs to be further studied.
For application of indoor gas leakage source localization through wireless sensor networks, the experimental data shows that the accuracy of position and gas release rate are excellent, but index of time consumption is just in the middle level. As the whole, the performance corresponds to the result of testing of benchmark function. Limited by objective conditions and project requirements, QC-HHO is not testing in outdoor environment. Also, for multiple leakage source, if distance between leakage sources is too close and the diffusion concentration fields are overlapped, there will be large deviation in the locating results. Furthermore, quantum correction is helpful for jumping local optima, but it is a kind of extremely time-consuming operation, so the time when to execute it should be more accurate.
This article is funded by: Research on Key Issues of Gas Leakage Source Location in Compressed Sensing Sensor Network in Three-dimensional Complex Flow Field Project of Tianjin Natural Science Foundation (20JCYBJC00320).
All authors declare there is no conflicts of interest.
[1] |
D. Zhang, X. Huang, J. Fei, Defect reconstruction from magnetic flux leakage measurements employing modified cuckoo search algorithm, Math. Biosci. Eng., 18 (2021), 1898–1925. https://doi.org/10.3934/mbe.2021099 doi: 10.3934/mbe.2021099
![]() |
[2] |
X. Yan, X. Huang, G. Liang, A feature extraction and classification algorithm based on improved sparse auto-encoder for round steel surface defects, Math. Biosci. Eng., 17 (2020), 5369–5394. https://doi.org/10.3934/mbe.2020290 doi: 10.3934/mbe.2020290
![]() |
[3] |
G. Dong, A pixel-wise framework based on convolutional neural network for surface defect detection, Math. Biosci. Eng., 19 (2022), 8786–8803. https://doi.org/10.3934/mbe.2022408 doi: 10.3934/mbe.2022408
![]() |
[4] |
Q. Wan, L. Gao, X. Li, Logit inducing with abnormality capturing for semi-supervised image anomaly detection, IEEE Trans. Instrum. Meas., 71 (2022), 1–12. https://doi.org/10.1109/TIM.2022.3205674 doi: 10.1109/TIM.2022.3205674
![]() |
[5] |
D. Wang, Y. Pan, Numerical sensing and simulation analysis of three-dimensional flow field and temperature field of submersible motor, Jour. Sen., 2023 (2023), 1–7. https://doi.org/10.21603/2542-1840-2023-7-1-1-7 doi: 10.21603/2542-1840-2023-7-1-1-7
![]() |
[6] | Q. Li, Q. Huang, T. Yang, Y. Zhou, K. Yang, H. Song, Internal defects inspection of arc magnets using multi-head attention-based CNN, Measurement, 202 (2022), 1–13. |
[7] | Y. Zhang, W. Wang, Z. Li, S. Shu, X. Lang, T. Zhang, et al., Development of a cross-scale weighted feature fusion network for hot-rolled steel surface defect detection, Eng. Appl. Art. Int., 117 (2023), 1–11. |
[8] |
X, Ling, Y. Wu, R, Ali, H. Zhu, Magnetic tile surface defect detection methodology based on self-attention and self-supervised learning, Comput. Int. Neural, 2022 (2022), 1–12. https://doi.org/10.1155/2022/3003810 doi: 10.1155/2022/3003810
![]() |
[9] | T, Liu, Z. He, Z, Lin, G. Cao, W. Su, S. Xie, An adaptive image segmentation network for surface defect detection, IEEE Trans. Neural Networks Learn. Syst., 2022 (2022), 1–14. |
[10] |
L. Xie, L. Lin, M. Yin, L. Meng, G. Yin, A novel surface defect inspection algorithm for magnetic tile, Appl. Surf. Sci., 375 (2016), 118–126. https://doi.org/10.1016/j.apsusc.2016.03.013 doi: 10.1016/j.apsusc.2016.03.013
![]() |
[11] |
C. Yang, P. Liu, G. Yin, H. Jiang, X. Li, Defect detection in magnetic tile images based on stationary wavelet transform, NDT E Int., 83 (2016), 78–87. https://doi.org/10.1016/j.ndteint.2016.04.006 doi: 10.1016/j.ndteint.2016.04.006
![]() |
[12] |
X. Cao, B. Chen, W. He, Unsupervised defect segmentation of magnetic tile based on attention enhanced flexible u-net, IEEE Trans. Instrum. Meas., 71 (2022), 1–10. https://doi.org/10.1109/TIM.2022.3170989 doi: 10.1109/TIM.2022.3170989
![]() |
[13] |
L. Xie, X. Xiang, H. Xu, L. Wang, L. Lin, G. Yin, FFCNN: A deep neural network for surface defect detection of magnetic tile, IEEE Trans. Ind. Electron., 68 (2020), 3506–3516. https://doi.org/10.1109/TIE.2020.2982115 doi: 10.1109/TIE.2020.2982115
![]() |
[14] |
W. Liang, Y. Sun ELCNN: A deep neural network for small object defect detection of magnetic tile, IEEE Trans. Instrum. Meas., 71 (2022), 1–10. https://doi.org/10.1109/TIM.2021.3132999 doi: 10.1109/TIM.2021.3132999
![]() |
[15] |
Y. Huang, C. Qiu, K. Yuan, Surface defect saliency of magnetic tile, Vision Comput., 36 (2020), 85–96. https://doi.org/10.1007/s00371-019-01734-2 doi: 10.1007/s00371-019-01734-2
![]() |
[16] |
Z. Zhong, H. Wang, D. Xiang, Small defect detection based on local structure similarity for magnetic tile surface, Electronics, 12 (2022), 1–17. https://doi.org/10.3390/electronics12010001 doi: 10.3390/electronics12010001
![]() |
[17] | C. Li, H. Yan, X. Qian, S. Zhu, P. Zhu, C. Liao, A domain adaptation YOLOv5 model for industrial defect inspection, Measurement, 213 (2023), 1–9. |
[18] | Q. Lin, J. Zhou, Q. Ma, Y. Ma, L. Kang, J. Wang, EMRA-Net: A pixel-wise network fusing local and global features for tiny and low-contrast surface defect detection, IEEE Trans. Instrum. Meas., 71 (2022), 1–14. |
[19] | Y. Pu, Y. Wang, Z. Xia, Y. Han, Y. Wang, W. Gan, Adaptive rotated convolution for rotated object detection, preprint, arXiv: 2303.07820. |
[20] | X. Chen, F. Zhou, G. Trajcevski, M. Bonsangue, Multi-view learning with distinguishable feature fusion for rumor detection, Knowl. Based. Syst., 240 (2022), 1–17. |
[21] |
B. Liu, X. Chen, Y. Xiao, W. Li, L. Liu, C. Liu, An efficient dictionary-based multi-view learning method, Inf. Sci., 576 (2021), 157–172. https://doi.org/10.1016/j.ins.2021.06.069 doi: 10.1016/j.ins.2021.06.069
![]() |
[22] |
X. Tao, J. Zhang, W. Ma, Z. Hou, Z. Lu, C. Adak, Unsupervised anomaly detection for surface defects with dual-siamese network, IEEE. Trans. Ind. Inf., 18 (2022), 7707–7717. https://doi.org/10.1109/TII.2022.3142326 doi: 10.1109/TII.2022.3142326
![]() |
[23] |
E. Gu, G. Xiao, F. Lian, T. Mu, Jie. Hong, J. Liu, Segmentation and evaluation of crack image from aircraft fuel tank via atrous spatial pyramid fusion and hybrid attention network, IEEE Trans. Instrum. Meas., 72 (2023), 1–14. https://doi.org/10.1109/TIM.2022.3223075 doi: 10.1109/TIM.2022.3223075
![]() |
[24] | Y. Deng, X. Wang, Y. Liao, ASA-Net: Adaptive sparse attention network for robust electric load forecasting, IEEE Int. Things J., (2023), 1–12. |
[25] |
X. Wang, Z. He, C. Liu, B. Zhang, Z. Lin, J. Guo, S. Xie, CGA-UNet: Category-guide attention U-Net for dental abnormality detection and segmentation from dental-maxillofacial images, IEEE Trans. Instrum. Meas., 72 (2023), 1–11. https://doi.org/10.1109/TIM.2023.3234080 doi: 10.1109/TIM.2023.3234080
![]() |
[26] | A. Paszke, S. Gross, F. Massa, A. Lerer, J. Bradbury, G. Chanan, et al., Pytorch: An imperative style, high-performance deep learning library, in Proceedings of the Advances in Neural Information Processing Systems (NIPS), (2019), 8026–8037. |
[27] |
L. Bottou, F. E. Curtis, J. Nocedal, Optimization methods for large-scale machine learning, Siam Rev., 60 (2018), 223–311. https://doi.org/10.1137/16M1080173 doi: 10.1137/16M1080173
![]() |
[28] | G. Huang, Z. Liu, L. Van Der Maaten, K. Q. Weinberger, Densely connected convolutional networks, in Proceedings of the IEEE Conference on Computer Vision and Pattern Recognition (CVPR), 2017, 4700–4708. |
[29] | A. Howard, S. Sandler, G. Chu, L. Chen, Searching for mobilenetv3, in Proceedings of the IEEE Conference on Computer Vision and Pattern Recognition (CVPR), 2019, 1314–1324. |
[30] | M. Tan, Q. Le, Efficientnet: Rethinking model scaling for convolutional neural networks, in Proceedings of the International Conference on Machine Learning (ICLR), 2019, 6105–6114. |
[31] | K. Han, Y. Wang, Q. Tian, J. Guo, Ghostnet: More features from cheap operations, in Proceedings of the IEEE Conference on Computer Vision and Pattern Recognition (CVPR), 2020, 1580–1589. |
[32] | K. Simonyan, A. Zisserman, Very deep convolutional networks for large-scale image recognition, preprint, tarXiv: 1409.1556. |
[33] | Z. Chen, L. Xie, J. Niu, X. Liu, Visformer: The vision-friendly transformer, in Proceedings of the IEEE Conference on Computer Vision and Pattern Recognition (CVPR), 2021,589–598. |
[34] |
J. Wang, K. Sun, T. Cheng, B. Jiang, Deep high-resolution representation learning for visual recognition, IEEE Trans. Pattern Anal. Mach. Intell., 43 (2020), 3349–3364. https://doi.org/10.1109/TPAMI.2020.2983686 doi: 10.1109/TPAMI.2020.2983686
![]() |
[35] |
Y. Zhu, L. Xie, M. Yin, G. Yin, Convolution with rotation invariance for online detection of tiny defects on magnetic tile surface, IEEE Trans. Instrum. Meas., 72 (2023), 1–12. https://doi.org/10.1109/TIM.2023.3248084 doi: 10.1109/TIM.2023.3248084
![]() |
1. | Shuhao Jiang, Mengyuan Wang, Jichang Guo, Mengqian Wang, K-means clustering algorithm based on improved flower pollination algorithm, 2022, 32, 1017-9909, 10.1117/1.JEI.32.3.032003 | |
2. | Xiaolong Zhang, Yuan Chen, Jiawei Liang, Jiawei Zhang, Tianyang Lu, Chuanqing Wang, 2022, Research on Anti-crawler and Anti-Anti-crawler Technology, 979-8-3503-0969-0, 35, 10.1109/ICINC58035.2022.00015 | |
3. | Lin Huang, Qiang Fu, Nan Tong, An Improved Harris Hawks Optimization Algorithm and Its Application in Grid Map Path Planning, 2023, 8, 2313-7673, 428, 10.3390/biomimetics8050428 | |
4. | Ming-Wei Li, Yi-Zhang Lei, Zhong-Yi Yang, Hsin-Pou Huang, Wei-Chiang Hong, An optimization method for maintenance routing and scheduling in offshore wind farms based on chaotic quantum Harris hawks optimization, 2024, 308, 00298018, 118306, 10.1016/j.oceaneng.2024.118306 | |
5. | Guoping You, Yudan Hu, Chao Lian, Zhen Yang, Mixed-Strategy Harris Hawk Optimization Algorithm for UAV Path Planning and Engineering Applications, 2024, 14, 2076-3417, 10581, 10.3390/app142210581 | |
6. | Hainan Wang, Junjie Hou, Haining Wang, Meng Wang, Yuting Zhu, Wenyu Zhang, An Improved Chaotic Quantum Multi-Objective Harris Hawks Optimization Algorithm for Emergency Centers Site Selection Decision Problem, 2025, 82, 1546-2226, 2177, 10.32604/cmc.2024.057441 | |
7. | Jiahao Li, Tao Hu, Xinyu Lian, Lan Jiang, Liyan Pan, Huaxia Deng, Shuaishuai Sun, Xinglong Gong, Constitutive optimization modeling of magnetorheological dampers under multiple influencing factors, 2025, 00207403, 110284, 10.1016/j.ijmecsci.2025.110284 |
Iteration No. | f(XNM-Optimal) | f(XNM-Subworst) | f(XNM-Worst) | f(XNMe) | f(XNMr) | f(XNMc1) | f(XNMc2) | Action |
1 | 1394.21 | 5893.56 | 7526.89 | — | — | — | — | Sort |
1394.21 | 5893.56 | 31.09 | 31.09 | 749.80 | — | — | Expansion | |
2 | 31.09 | 1394.21 | 5893.56 | 31.09 | 749.80 | — | — | Sort |
31.09 | 1394.21 | 3.54 | 3.54 | 21.96 | — | — | Expansion | |
3 | 3.54 | 31.09 | 1394.21 | 3.54 | 21.96 | — | — | Sort |
3.54 | 31.09 | 8.22 | 3.54 | 8.22 | — | — | Reflection <1> | |
4 | 3.54 | 8.22 | 31.09 | 3.54 | 8.22 | — | — | Sort |
3.54 | 1.25 | 86.88 | 3.54 | 1.39e+4 | — | 188.77 | Shrink | |
5 | 1.25 | 3.54 | 86.88 | 3.54 | 1.39e+4 | — | 188.77 | Sort |
1.25 | 3.54 | 71.21 | 3.54 | 1675.13 | — | 71.21 | Inside contraction | |
...... | ...... | |||||||
12 | 0.07 | 0.19 | 0.26 | 3.54 | 3.10 | — | 0.19 | Sort |
0.07 | 0.19 | 0.14 | 3.54 | 0.26 | 0.14 | 0.19 | Outside contraction | |
...... | ...... | |||||||
19 | 0.0103 | 0.0263 | 0.0263 | 3.5432 | 0.0263 | 0.1447 | 0.0263 | Sort |
0.0103 | 0.0263 | 0.0084 | 0.0331 | 0.0084 | 0.1447 | 0.0263 | Reflection <2> |
Figure | Function No. | Function Name | Number of Variables | Boundary | Optimal Solution |
![]() |
F1 | Sphere | 30 | [-100,100] | 0 |
![]() |
F2 | Schwefel 1.2 | 30 | [-100,100] | 0 |
![]() |
F3 | Schwefel 2.21 | 30 | [-100,100] | 0 |
![]() |
F4 | Quartic with Noise | 30 | [−1.28, 1.28] | 0 |
![]() |
F5 | Schwefel | 30 | [−500,500] | −418.9829 × 5 |
![]() |
F6 | Rastrigin | 30 | [−5.12, 5.12] | 0 |
![]() |
F7 | Ackley | 30 | [−32, 32] | 0 |
![]() |
F8 | Penalized 1.1 | 30 | [−50, 50] | 0 |
![]() |
F9 | Goldstein-Price | 2 | [−2, 2] | 3 |
![]() |
F10 | Shekel 5 | 4 | [0, 10] | −10.1532 |
Function No. | metrics | BFO | PSO | DE | HHO | QC-HHO |
F1 | mean | 5.67E-05 | 3.89E-01 | 1.01E-13 | 1.13E-97 | 0.00E+00 |
std | 8.46E-06 | 1.29E-01 | 7.61E-14 | 2.48E-97 | 0.00E+00 | |
F2 | mean | 2.66E-04 | 3.54E+01 | 8.50E-11 | 5.89E-79 | 0.00E+00 |
std | 4.50E-05 | 1.46E+01 | 9.14E-11 | 1.81E-78 | 0.00E+00 | |
F3 | mean | 4.34E-03 | 2.61E+00 | 0.00E+00 | 3.11E-50 | 0.00E+00 |
std | 5.68E-04 | 1.29E+00 | 0.00E+00 | 6.55E-50 | 1.01E-101 | |
F4 | mean | 2.50E-04 | 3.55E+00 | 5.63E-03 | 1.97E-04 | 4.23E-05 |
std | 2.16E-04 | 8.49E+00 | 1.48E-03 | 2.05E-04 | 4.18E-05 | |
F5 | mean | -2.01E+03 | -2.74E+03 | -1.12E+04 | -1.26E+04 | -1.44E+04 |
std | 4.40E+02 | 3.61E+02 | 5.75E+02 | 1.68E+00 | 1.85E-12 | |
F6 | mean | 1.11E-02 | 9.82E+01 | 6.92E+01 | 0.00E+00 | 0.00E+00 |
std | 1.72E-03 | 1.26E+01 | 3.88E+01 | 0.00E+00 | 0.00E+00 | |
F7 | mean | 5.85E-03 | 2.56E+00 | 1.18E-07 | 8.88E-16 | 8.88E-16 |
std | 4.33E-04 | 1.13E+00 | 4.96E-08 | 0.00E+00 | 0.00E+00 | |
F8 | mean | 1.56E+00 | 3.43E+00 | 9.76E-15 | 1.03E-05 | 1.57E-22 |
std | 7.01E-03 | 1.10E+00 | 9.85E-15 | 1.33E-05 | 5.57E-48 | |
F9 | mean | 8.40E+00 | 3.00E+00 | 3.73E+00 | 3.00E+00 | 3.00E+00 |
std | 1.14E+01 | 5.91E-04 | 2.57E-15 | 2.29E-07 | 4.38E-02 | |
F10 | mean | -7.91E-01 | -4.15E+00 | -1.17E+01 | -5.05E+00 | -9.97E+00 |
std | 5.20E-01 | 2.40E+00 | 2.89E-06 | 1.64E-02 | 1.56E+00 |
Function No. | metrics | CEHHO | DMSDL-HHO | ADHHO | CLHHEO | QC-HHO |
f1 | ave | 1.0600E-111 | 0.0000E+00 | 2.5300E-196 | 2.9200E-187 | 0.0000E+00 |
std | 5.2400E-111 | 0.0000E+00 | 8.8820E-63 | 0.0000E+00 | 0.0000E+00 | |
rank | 5 | 1 | 3 | 4 | 1 | |
f2 | ave | 2.3400E-89 | 0.0000E+00 | 2.2653E-102 | 9.3000E-116 | 0.0000E+00 |
std | 1.2700E-88 | 0.0000E+00 | 1.1250E-98 | 4.9100E-115 | 0.0000E+00 | |
rank | 5 | 1 | 4 | 3 | 1 | |
f3 | ave | 8.3500E-58 | 0.0000E+00 | 8.9240E-74 | 4.0400E-74 | 0.0000E+00 |
std | 1.9700E-67 | 0.0000E+00 | 1.1230E-70 | 1.5900E-73 | 1.0100E-101 | |
rank | 5 | 1 | 4 | 3 | 1 | |
f4 | ave | 1.4900E-04 | 1.3380E-04 | 4.2924E-05 | 4.0479E-05 | 4.2300E-05 |
std | 1.2800E-05 | 1.4544E-04 | 4.3058E-05 | 4.3225E-05 | 4.1800E-05 | |
rank | 5 | 4 | 3 | 1 | 2 | |
f5 | ave | -1.3506E+04 | -1.3803E+04 | -1.2730E+04 | -1.3511E+04 | -1.4443E+04 |
std | -1.2600E+04 | 1.7569E+03 | 5.0093E-04 | -1.2691E+04 | 1.8500E-12 | |
rank | 4 | 2 | 5 | 3 | 1 | |
f6 | ave | 0.0000E+00 | 0.0000E+00 | 0.0000E+00 | 0.0000E+00 | 0.0000E+00 |
std | 0.0000E+00 | 0.0000E+00 | 0.0000E+00 | 0.0000E+00 | 0.0000E+00 | |
rank | 1 | 1 | 1 | 1 | 1 | |
f7 | ave | 8.8800E-16 | 8.8800E-16 | 8.8800E-16 | 8.8800E-16 | 8.8800E-16 |
std | 0.0000E+00 | 0.0000E+00 | 0.0000E+00 | 0.0000E+00 | 0.0000E+00 | |
rank | 1 | 1 | 1 | 1 | 1 | |
f8 | ave | 8.4400E-06 | 4.1151E-13 | 1.7461E-24 | 2.8600E-12 | 1.5700E-22 |
std | 2.9600E-09 | 2.7293E-13 | 3.3065E-07 | 3.5400E-12 | 5.5700E-48 | |
rank | 5 | 3 | 1 | 4 | 2 | |
f9 | ave | 3.0000E+00 | 3.0000E+00 | 3.0000E+00 | 3.0000E+00 | 3.0000E+00 |
std | 1.6400E-07 | 3.2183E-15 | 5.9349E-06 | 7.6682E-07 | 4.3800E-02 | |
rank | 1 | 1 | 1 | 1 | 1 | |
f10 | ave | -5.3600E+00 | -1.0151E+01 | -5.4742E+00 | -1.0133E+01 | -9.9654E+00 |
std | 1.1700E+00 | 8.5892E-15 | 2.3396E-01 | 1.0048E-03 | 1.5600E+00 | |
rank | 5 | 1 | 4 | 2 | 3 |
metrics | HHO | CEHHO | DMSDL- HHO | AD-HHO | CLHHEO | QC-HHO | |
f1 | ave | 3.4388E+07 | 6.7119E+07 | 9.2986E+00 | 9.3981E+00 | 6.0859E+04 | 8.2197E+00 |
std | 1.5816E+07 | 1.7777E+07 | 1.3081E+01 | 1.2775E+01 | 2.0189E+07 | 1.5221E+01 | |
f2 | ave | 3.4053E+07 | 1.4616E+08 | 1.9284E-07 | 1.8058E-07 | 7.4597E+05 | 1.7759E-07 |
std | 1.1072E+07 | 5.5324E+07 | 1.0708E-07 | 8.9939E-08 | 4.8320E+07 | 1.1139E-07 | |
f3 | ave | 2.4042E+04 | 3.7078E+04 | 2.6431E-01 | 4.4136E-01 | 5.6404E+02 | 3.6351E-01 |
std | 5.4667E+03 | 1.3501E+04 | 3.0418E-01 | 2.9975E-01 | 1.0972E+04 | 3.4800E-01 | |
f4 | ave | 6.2954E+02 | 8.1557E+02 | 3.0498E-10 | 3.0772E-10 | 1.2677E-10 | 3.3697E-10 |
std | 6.7784E+01 | 2.0063E+02 | 1.0968E-10 | 1.1019E-10 | 1.0309E+02 | 7.3327E-11 | |
f5 | ave | 5.2052E+02 | 5.2059E+02 | 5.1904E+02 | 5.1888E+02 | 5.0843E+02 | 5.1453E+02 |
std | 1.2532E-01 | 1.4775E-01 | 2.7139E-04 | 3.0074E-04 | 1.4283E-01 | 2.3809E-04 | |
f6 | ave | 6.3334E+02 | 6.3626E+02 | 1.0219E+02 | 1.3807E+02 | 4.4244E+02 | 3.9525E+01 |
std | 3.3253E+00 | 2.9463E+00 | 2.4023E+00 | 2.8022E+00 | 3.3249E+00 | 2.3540E+00 | |
f7 | ave | 7.0130E+02 | 7.0243E+02 | 9.3954E-11 | 1.6007E-10 | 9.3062E-07 | 9.4327E-12 |
std | 8.5586E-02 | 6.3357E-01 | 1.8047E-14 | 1.8914E-14 | 1.0667E+00 | 1.5569E-14 | |
f8 | ave | 9.3159E+02 | 9.4180E+02 | 2.2990E+02 | 2.7292E+02 | 1.6638E+02 | 1.6360E+02 |
std | 1.7349E+01 | 2.2440E+01 | 1.0357E+01 | 9.9155E+00 | 1.6189E+01 | 1.8853E+01 | |
f9 | ave | 1.0874E+03 | 1.1007E+03 | 2.8799E+02 | 4.0555E+02 | 3.5026E+02 | 2.7859E+02 |
std | 2.0406E+01 | 2.0994E+01 | 1.3628E+01 | 1.3421E+01 | 1.7502E+01 | 1.4507E+01 | |
f10 | ave | 4.0048E+03 | 4.1507E+03 | 8.6068E+02 | 7.3350E+02 | 1.9039E+03 | 7.7819E+02 |
std | 7.8162E+02 | 6.1751E+02 | 2.8306E+02 | 2.7512E+02 | 2.3224E+02 | 3.0040E+02 | |
f11 | ave | 5.6507E+03 | 5.7285E+03 | 3.1228E+03 | 3.8417E+03 | 5.4454E+03 | 6.0754E+03 |
std | 7.8910E+02 | 5.5742E+02 | 7.7495E+02 | 6.1912E+02 | 5.1104E+02 | 6.9601E+02 | |
f12 | ave | 1.2019E+03 | 1.2021E+03 | 2.5912E+02 | 2.8480E+02 | 1.6350E+02 | 4.3482E+01 |
std | 4.7933E-01 | 6.9743E-01 | 2.9394E-01 | 2.7541E-01 | 6.4237E-01 | 2.7386E-01 | |
f13 | ave | 1.3005E+03 | 1.3005E+03 | 6.2438E+02 | 6.6377E+02 | 1.0466E+03 | 6.1473E+02 |
std | 1.5096E-01 | 1.2744E-01 | 1.0724E-01 | 8.1349E-02 | 1.0359E-01 | 7.3202E-02 | |
f14 | ave | 1.4003E+03 | 1.4004E+03 | 1.0463E+03 | 1.1425E+03 | 1.1245E+03 | 8.6137E+02 |
std | 1.0112E-01 | 2.7234E-01 | 2.5115E-02 | 2.2234E-02 | 3.0647E-01 | 1.5575E-02 | |
f15 | ave | 1.5453E+03 | 1.5601E+03 | 1.5535E+02 | 1.4709E+02 | 1.3600E+02 | 1.2897E+03 |
std | 8.9250E+00 | 1.7244E+01 | 1.1863E+00 | 9.3748E-01 | 2.0126E+01 | 1.2965E+00 | |
f16 | ave | 1.6124E+03 | 1.6123E+03 | 1.1359E+03 | 1.3203E+03 | 7.7656E+02 | 9.7576E+02 |
std | 4.8097E-01 | 5.9799E-01 | 6.7343E-01 | 6.8893E-01 | 6.3623E-01 | 7.4550E-01 | |
f17 | ave | 4.4967E+06 | 5.7408E+06 | 8.6200E+03 | 7.0382E+03 | 5.6425E+04 | 1.0090E+04 |
std | 3.6931E+06 | 3.3646E+06 | 5.5689E+03 | 4.7452E+03 | 2.9065E+06 | 5.0348E+03 | |
f18 | ave | 1.9322E+05 | 7.8069E+05 | 2.5421E+03 | 2.3654E+03 | 7.8574E+03 | 2.8281E+03 |
std | 3.4242E+05 | 7.2558E+05 | 5.2332E+03 | 3.7030E+03 | 7.7741E+05 | 3.5642E+03 | |
f19 | ave | 1.9518E+03 | 1.9926E+03 | 1.8122E+02 | 2.2464E+02 | 1.3694E+03 | 1.5917E+02 |
std | 3.6942E+01 | 3.4779E+01 | 8.3276E-01 | 8.9098E-01 | 3.9001E+01 | 8.6310E-01 | |
f20 | ave | 2.5130E+04 | 4.7393E+04 | 1.1201E+03 | 1.0525E+03 | 1.0623E+03 | 1.6085E+03 |
std | 8.9647E+03 | 7.8229E+03 | 5.6089E+02 | 4.8117E+02 | 8.9803E+03 | 4.6628E+02 | |
f21 | ave | 7.8219E+05 | 7.8782E+05 | 1.0874E+03 | 1.1780E+03 | 6.7445E+04 | 7.9901E+03 |
std | 7.2130E+05 | 7.2787E+05 | 8.7689E+02 | 8.5450E+02 | 8.4029E+05 | 9.6452E+02 | |
f22 | ave | 3.1687E+03 | 3.2757E+03 | 1.3253E+03 | 7.9046E+02 | 5.8064E+02 | 1.4654E+03 |
std | 2.9168E+02 | 1.6299E+02 | 1.0242E+01 | 9.8666E+01 | 1.8406E+02 | 1.0312E+01 | |
f23 | ave | 2.5000E+03 | 2.5000E+03 | 2.5000E+03 | 2.5000E+03 | 2.5000E+03 | 2.5000E+03 |
std | 0.0000E+00 | 0.0000E+00 | 0.0000E+00 | 0.0000E+00 | 0.0000E+00 | 0.0000E+00 | |
f24 | ave | 2.6000E+03 | 2.6000E+03 | 2.6000E+03 | 2.6000E+03 | 2.6000E+03 | 2.6000E+03 |
std | 4.0537E-04 | 0.0000E+00 | 0.0000E+00 | 0.0000E+00 | 0.0000E+00 | 0.0000E+00 | |
f25 | ave | 2.7000E+03 | 2.7000E+03 | 2.7000E+03 | 2.7000E+03 | 2.7000E+03 | 2.7000E+03 |
std | 0.0000E+00 | 0.0000E+00 | 0.0000E+00 | 0.0000E+00 | 0.0000E+00 | 0.0000E+00 | |
f26 | ave | 2.7622E+03 | 2.7403E+03 | 2.0384E+03 | 1.9048E+03 | 1.9035E+03 | 2.5068E+03 |
std | 4.8819E+01 | 5.1410E+01 | 7.3958E-02 | 5.8292E-02 | 5.0192E+01 | 5.5044E-02 | |
f27 | ave | 2.9000E+03 | 2.9000E+03 | 2.9000E+03 | 2.9000E+03 | 2.9000E+03 | 2.9000E+03 |
std | 0.0000E+00 | 0.0000E+00 | 0.0000E+00 | 0.0000E+00 | 0.0000E+00 | 0.0000E+00 | |
f28 | ave | 3.0000E+03 | 3.0000E+03 | 3.0000E+03 | 3.0000E+03 | 3.0000E+03 | 3.0000E+03 |
std | 0.0000E+00 | 0.0000E+00 | 0.0000E+00 | 0.0000E+00 | 0.0000E+00 | 0.0000E+00 | |
f29 | ave | 6.2109E+05 | 2.6613E+06 | 3.2662E+05 | 3.0237E+05 | 2.8801E+05 | 3.0168E+05 |
std | 2.7211E+06 | 0.0000E+00 | 0.0000E+00 | 0.0000E+00 | 0.0000E+00 | 0.0000E+00 | |
f30 | ave | 8.2238E+04 | 4.5278E+04 | 4.4532E+03 | 4.1717E+03 | 4.2684E+04 | 5.9009E+03 |
std | 1.4431E+05 | 0.0000E+00 | 0.0000E+00 | 0.0000E+00 | 0.0000E+00 | 0.0000E+00 |
Unimodal Functions | Simple Multimodal Functions | Hybrid Functions | Composition Functions | All Functions | |
HHO | 5.00 | 5.08 | 5.00 | 2.75 | 4.43 |
CEHHO | 6.00 | 5.77 | 6.00 | 2.63 | 5.00 |
DMSDL-HHO | 2.00 | 2.46 | 2.17 | 1.75 | 2.17 |
ADHHO | 2.67 | 3.08 | 1.67 | 1.38 | 2.30 |
CLHHEO | 4.00 | 2.54 | 3.17 | 1.38 | 2.50 |
QC-HHO | 1.33 | 2.08 | 3.00 | 1.75 | 2.10 |
metrics | HHO | CEHHO | DMSDL- HHO | ADHHO | CLHHEO | QC-HHO | |
f1 | ave | 8.8085E+07 | 1.5267E+08 | 1.6076E+00 | 1.5998E+00 | 1.3999E+00 | 1.3853E+00 |
std | 3.3967E+07 | 6.2054E+07 | 2.3140E+00 | 2.0577E+00 | 2.6593E+00 | 1.6336E-01 | |
f2 | ave | 6.5654E+08 | 2.2991E+09 | 1.3291E+03 | 1.1442E+03 | 1.5104E+03 | 1.0161E+03 |
std | 2.5436E+08 | 7.6478E+08 | 6.7502E+02 | 6.2056E+02 | 6.7748E+02 | 6.5509E+02 | |
f3 | ave | 5.7216E+04 | 8.6216E+04 | 1.5200E-01 | 1.6903E-01 | 1.8400E-01 | 1.8167E-01 |
std | 1.0290E+04 | 1.4523E+04 | 1.6414E-02 | 1.9100E-02 | 2.2674E-02 | 1.7807E-02 | |
f4 | ave | 9.2274E+02 | 1.1512E+03 | 1.1415E-01 | 8.8438E-02 | 7.2032E-02 | 6.6518E-02 |
std | 1.3692E+02 | 1.3042E+02 | 1.4276E+00 | 1.7794E+00 | 1.4689E+00 | 1.4655E+00 | |
f5 | ave | 5.2084E+02 | 5.2079E+02 | 5.4338E+03 | 5.1954E+03 | 4.5483E+03 | 5.9474E+02 |
std | 1.2934E-01 | 1.5010E-01 | 1.7678E-05 | 1.9315E-05 | 2.8812E-05 | 1.6617E-05 | |
f6 | ave | 6.6089E+02 | 6.6458E+02 | 2.9099E+02 | 2.5444E+02 | 2.2542E+02 | 2.6008E+02 |
std | 4.8858E+00 | 3.4152E+00 | 4.4083E+00 | 4.1760E+00 | 4.6698E+00 | 3.1034E+00 | |
f7 | ave | 7.0626E+02 | 7.2412E+02 | 1.5010E+00 | 1.3966E+00 | 1.2825E+00 | 1.7613E+00 |
std | 1.9491E+00 | 6.5846E+00 | 9.1075E-02 | 9.1857E-02 | 7.6984E-02 | 1.0520E-01 | |
f8 | ave | 1.0830E+03 | 1.0884E+03 | 5.9830E+02 | 5.6709E+02 | 2.1714E+02 | 4.8943E+02 |
std | 2.4935E+01 | 2.8755E+01 | 2.2672E+01 | 2.6441E+01 | 2.6903E+01 | 5.4955E+00 | |
f9 | ave | 1.2985E+03 | 1.3268E+03 | 5.3727E+02 | 6.7579E+02 | 6.6299E+02 | 5.4358E+02 |
std | 4.1898E+01 | 3.8579E+01 | 4.4425E+01 | 3.5146E+01 | 5.6706E+01 | 4.3760E+01 | |
f10 | ave | 7.4406E+03 | 8.3494E+03 | 1.5085E+03 | 3.1313E+03 | 2.7626E+03 | 1.8091E+03 |
std | 1.3736E+03 | 9.9589E+02 | 8.3676E+02 | 7.3305E+02 | 8.4150E+02 | 8.0097E+02 | |
f11 | ave | 1.0140E+04 | 1.1356E+04 | 6.5184E+03 | 7.7585E+03 | 9.2076E+03 | 6.2393E+02 |
std | 1.1277E+03 | 1.4072E+03 | 9.3317E+02 | 7.8877E+02 | 7.5315E+02 | 1.0175E+03 | |
f12 | ave | 1.2027E+03 | 1.2029E+03 | 1.1083E+02 | 1.3577E+02 | 2.0733E+02 | 8.4096E+01 |
std | 5.6534E-01 | 7.1506E-01 | 1.2631E-01 | 1.8406E-01 | 1.7612E-01 | 1.0291E-01 | |
f13 | ave | 1.3006E+03 | 1.3006E+03 | 1.1865E+03 | 1.0192E+03 | 1.2148E+03 | 1.9979E+03 |
std | 1.1094E-01 | 1.5253E-01 | 6.3924E-02 | 5.5773E-02 | 5.2991E-03 | 6.2082E-02 | |
f14 | ave | 1.4004E+03 | 1.4004E+03 | 9.4218E+02 | 1.1219E+03 | 2.5971E+02 | 9.4118E+02 |
std | 1.0639E-01 | 1.5366E-01 | 8.4510E-02 | 6.8426E-02 | 5.3345E-03 | 7.6521E-02 | |
f15 | ave | 1.6353E+03 | 2.0286E+03 | 1.6569E+02 | 1.4874E+02 | 1.5910E+02 | 1.6023E+02 |
std | 4.9995E+01 | 4.0222E+02 | 3.8812E+00 | 4.6768E+00 | 3.8753E+00 | 3.5583E+00 | |
f16 | ave | 1.6222E+03 | 1.6222E+03 | 1.5002E+03 | 1.4165E+03 | 1.7790E+03 | 1.3866E+03 |
std | 4.2170E-01 | 5.1725E-01 | 2.9190E-01 | 6.5435E-01 | 6.4365E-01 | 4.4801E-01 | |
f17 | ave | 1.4557E+07 | 3.9310E+07 | 1.8954E+04 | 1.8389E+04 | 2.1112E+04 | 1.6400E+04 |
std | 8.7585E+06 | 1.6772E+07 | 1.0117E+04 | 1.0110E+04 | 8.0640E+03 | 7.4718E+03 | |
f18 | ave | 6.4198E+06 | 2.9993E+07 | 3.6657E+05 | 4.5792E+05 | 4.2545E+05 | 4.7209E+05 |
std | 2.1913E+07 | 5.3636E+07 | 1.8446E+06 | 3.5932E+06 | 3.8297E+06 | 1.6075E+06 | |
f19 | ave | 1.9772E+03 | 2.0031E+03 | 1.2075E+03 | 6.1665E+02 | 6.7387E+02 | 2.0142E+03 |
std | 2.5168E+01 | 3.4978E+01 | 1.4342E+01 | 1.3839E+01 | 1.2625E+01 | 1.5203E+01 | |
f20 | ave | 3.9538E+04 | 6.3072E+04 | 1.8738E+03 | 2.2161E+03 | 2.4283E+03 | 1.8695E+03 |
std | 1.3456E+04 | 1.8238E+04 | 1.1249E+03 | 1.2644E+03 | 1.1763E+03 | 1.2064E+03 | |
f21 | ave | 5.4741E+06 | 9.3026E+06 | 7.8663E+03 | 9.1266E+03 | 1.0157E+04 | 8.7394E+03 |
std | 2.5403E+06 | 5.1269E+06 | 5.8327E+03 | 5.4384E+03 | 4.0745E+03 | 4.4298E+03 | |
f22 | ave | 4.0893E+03 | 4.1887E+03 | 3.3122E+03 | 1.6926E+03 | 1.2617E+03 | 3.4122E+03 |
std | 4.1644E+02 | 4.0711E+02 | 2.9382E+02 | 2.7958E+02 | 3.1192E+02 | 1.4846E+01 | |
f23 | ave | 2.5000E+03 | 2.5000E+03 | 2.5000E+03 | 2.5000E+03 | 2.5000E+03 | 2.5000E+03 |
std | 0.0000E+00 | 0.0000E+00 | 0.0000E+00 | 0.0000E+00 | 0.0000E+00 | 0.0000E+00 | |
f24 | ave | 2.6000E+03 | 2.6000E+03 | 2.6000E+03 | 2.6000E+03 | 2.6000E+03 | 2.6000E+03 |
std | 1.2144E-04 | 0.0000E+00 | 0.0000E+00 | 0.0000E+00 | 0.0000E+00 | 0.0000E+00 | |
f25 | ave | 2.7000E+03 | 2.7000E+03 | 2.7000E+03 | 2.7000E+03 | 2.9068E+03 | 2.7000E+03 |
std | 0.0000E+00 | 0.0000E+00 | 0.0000E+00 | 0.0000E+00 | 0.0000E+00 | 0.0000E+00 | |
f26 | ave | 2.7801E+03 | 2.7900E+03 | 1.6534E+03 | 2.0271E+03 | 1.6382E+03 | 1.7901E+03 |
std | 4.0219E+01 | 3.1478E+01 | 4.7512E+01 | 4.7105E+01 | 5.4754E+01 | 4.0077E+01 | |
f27 | ave | 2.9000E+03 | 2.9000E+03 | 2.9000E+03 | 2.9000E+03 | 2.9000E+03 | 2.9000E+03 |
std | 0.0000E+00 | 0.0000E+00 | 0.0000E+00 | 0.0000E+00 | 0.0000E+00 | 0.0000E+00 | |
f28 | ave | 3.0000E+03 | 3.0000E+03 | 3.0000E+03 | 3.0000E+03 | 3.0000E+03 | 3.0000E+03 |
std | 4.5936E-13 | 0.0000E+00 | 0.0000E+00 | 0.0000E+00 | 0.0000E+00 | 0.0000E+00 | |
f29 | ave | 3.1000E+03 | 3.1000E+03 | 3.1000E+03 | 3.1000E+03 | 3.1000E+03 | 3.1000E+03 |
std | 0.0000E+00 | 0.0000E+00 | 0.0000E+00 | 0.0000E+00 | 0.0000E+00 | 0.0000E+00 | |
f30 | ave | 3.9043E+04 | 8.2553E+04 | 2.1650E+03 | 2.2834E+03 | 2.5747E+03 | 2.7625E+03 |
std | 1.2152E+05 | 0.0000E+00 | 0.0000E+00 | 0.0000E+00 | 0.0000E+00 | 0.0000E+00 |
Unimodal Functions | Simple Multimodal Functions | Hybrid Functions | Composition Functions | All Functions | |
HHO | 5.00 | 4.69 | 4.83 | 2.00 | 4.03 |
CEHHO | 6.00 | 5.38 | 5.83 | 2.25 | 4.70 |
DMSDL-HHO | 2.67 | 3.00 | 2.17 | 1.13 | 2.30 |
ADHHO | 2.33 | 2.85 | 2.33 | 1.50 | 2.33 |
CLHHEO | 3.33 | 2.69 | 2.83 | 1.88 | 2.57 |
QC-HHO | 1.67 | 2.38 | 3.00 | 1.63 | 2.23 |
Metrics | HHO | CEHHO | DMSDL- HHO | AD-HHO | CLHHEO | QC-HHO | |
f1 | ave | 2.9532E+08 | 4.5244E+08 | 2.2065E-01 | 2.3133E-01 | 1.8079E-01 | 2.0389E-01 |
std | 5.9293E+07 | 1.0812E+08 | 4.0428E-01 | 4.2021E-01 | 4.6860E-01 | 3.8815E-01 | |
f2 | ave | 1.4056E+10 | 2.3634E+10 | 4.0215E+01 | 3.2914E+01 | 2.4421E+01 | 2.3105E+01 |
std | 3.4046E+09 | 3.1652E+09 | 1.2254E+02 | 1.3453E+02 | 1.0388E+02 | 1.4275E+02 | |
f3 | ave | 1.5690E+05 | 1.9603E+05 | 4.3587E-01 | 4.0324E-01 | 1.2412E-01 | 3.8466E-01 |
std | 1.8735E+04 | 3.3780E+04 | 9.0178E-02 | 5.3367E-02 | 8.9880E-02 | 6.4643E-02 | |
f4 | ave | 2.5476E+03 | 4.2424E+03 | 1.3242E+01 | 1.1286E+01 | 1.3599E+01 | 8.2205E+00 |
std | 4.0800E+02 | 1.0406E+03 | 1.2069E+01 | 8.2972E+00 | 1.6341E+01 | 9.8653E+00 | |
f5 | ave | 5.2111E+02 | 5.2107E+02 | 5.1999E+02 | 6.5981E+02 | 8.4588E+02 | 5.2108E+02 |
std | 9.4023E-02 | 8.6480E-02 | 3.3689E-06 | 3.8421E-06 | 3.1172E-06 | 3.6386E-06 | |
f6 | ave | 7.4008E+02 | 7.5001E+02 | 4.3865E+02 | 5.1949E+02 | 5.0975E+02 | 5.3346E+02 |
std | 6.5795E+00 | 6.8974E+00 | 6.9352E+00 | 6.4936E+00 | 7.6965E+00 | 8.2792E+00 | |
f7 | ave | 8.4469E+02 | 9.8020E+02 | 1.2370E+00 | 1.0825E+00 | 1.2535E+00 | 1.1447E+00 |
std | 3.1984E+01 | 6.4082E+01 | 5.4277E-01 | 5.3984E-01 | 4.0310E-01 | 4.8305E-01 | |
f8 | ave | 1.5063E+03 | 1.5608E+03 | 1.0793E+03 | 1.0420E+03 | 1.0178E+03 | 3.9801E+02 |
std | 3.2668E+01 | 3.0244E+01 | 5.3529E+01 | 4.3120E+01 | 4.8401E+01 | 4.2877E+01 | |
f9 | ave | 1.8268E+03 | 1.8914E+03 | 1.5135E+03 | 2.6553E+03 | 3.3141E+02 | 1.9302E+03 |
std | 4.5102E+01 | 4.4228E+01 | 7.4349E+01 | 5.5063E+01 | 6.7256E+01 | 4.5389E+01 | |
f10 | ave | 1.7487E+04 | 1.9500E+04 | 8.3093E+03 | 1.9446E+03 | 9.1671E+03 | 1.8913E+03 |
std | 1.8860E+03 | 1.7014E+03 | 8.5620E+02 | 5.3573E+02 | 8.2863E+02 | 6.3636E+02 | |
f11 | ave | 2.2358E+04 | 2.3184E+04 | 1.6779E+04 | 1.5132E+04 | 1.8203E+04 | 2.1426E+04 |
std | 2.1316E+03 | 2.7566E+03 | 1.0591E+03 | 1.0470E+03 | 1.8372E+03 | 9.7728E+02 | |
f12 | ave | 1.2036E+03 | 1.2038E+03 | 1.7679E+02 | 3.4298E+02 | 1.7758E+02 | 2.7543E+02 |
std | 5.1525E-01 | 4.3964E-01 | 1.8670E-01 | 1.7408E-01 | 3.1450E-01 | 2.0032E-01 | |
f13 | ave | 1.3006E+03 | 1.3011E+03 | 1.2036E+03 | 6.9965E+02 | 1.0099E+03 | 5.4023E+02 |
std | 7.3180E-02 | 9.3521E-01 | 6.1230E-02 | 1.0670E-01 | 5.8115E-02 | 5.5391E-02 | |
f14 | ave | 1.4397E+03 | 1.4759E+03 | 1.2487E+03 | 1.4576E+03 | 1.3813E+03 | 1.3914E+03 |
std | 1.0803E+01 | 1.0229E+01 | 3.0977E+00 | 2.7375E+00 | 2.7636E+00 | 2.9033E+00 | |
f15 | ave | 8.0315E+03 | 3.0563E+04 | 1.0476E+03 | 7.5402E+02 | 1.2366E+03 | 6.8471E+02 |
std | 3.4553E+03 | 1.4417E+04 | 4.7003E+02 | 1.7529E+02 | 5.4379E+02 | 1.4072E+02 | |
f16 | ave | 1.6461E+03 | 1.6460E+03 | 1.5723E+03 | 4.4884E+02 | 1.3370E+03 | 3.3899E+02 |
std | 5.3948E-01 | 6.5221E-01 | 6.7085E+00 | 6.9260E+00 | 7.7507E+00 | 6.7595E+00 | |
f17 | ave | 5.9354E+07 | 9.1421E+07 | 4.3274E+04 | 5.5973E+04 | 4.1236E+04 | 5.8317E+04 |
std | 2.0834E+07 | 4.7987E+07 | 8.9080E+03 | 8.2787E+03 | 1.0579E+04 | 1.4855E+04 | |
f18 | ave | 2.5553E+07 | 4.2986E+07 | 3.1724E+04 | 2.6405E+04 | 3.6162E+04 | 4.6724E+04 |
std | 4.0091E+07 | 2.0825E+07 | 1.7080E+04 | 1.8732E+04 | 2.0411E+04 | 5.2549E+03 | |
f19 | ave | 2.2215E+03 | 2.3154E+03 | 1.2729E+03 | 1.1020E+03 | 1.1081E+03 | 1.4420E+03 |
std | 8.0641E+01 | 4.4544E+01 | 7.0559E+01 | 5.6971E+01 | 3.8067E+01 | 4.3033E+01 | |
f20 | ave | 1.1887E+05 | 1.7736E+05 | 1.1082E+04 | 1.1422E+04 | 1.2123E+04 | 9.4987E+03 |
std | 2.4661E+04 | 4.7633E+04 | 4.6661E+03 | 5.1722E+03 | 6.0422E+03 | 6.2754E+03 | |
f21 | ave | 1.9119E+07 | 2.9989E+07 | 1.1519E+04 | 9.7435E+03 | 1.2092E+04 | 9.7915E+03 |
std | 6.3314E+06 | 1.4428E+07 | 3.0529E+03 | 4.5540E+03 | 3.2256E+03 | 4.0206E+03 | |
f22 | ave | 5.8287E+03 | 6.5909E+03 | 3.2905E+03 | 2.8780E+03 | 3.1277E+03 | 3.0934E+03 |
std | 5.7346E+02 | 7.5484E+02 | 4.3025E+02 | 4.2572E+02 | 4.9619E+02 | 4.7700E+02 | |
f23 | ave | 2.5000E+03 | 2.5000E+03 | 2.5000E+03 | 2.5000E+03 | 2.5000E+03 | 2.5000E+03 |
std | 0.0000E+00 | 0.0000E+00 | 0.0000E+00 | 0.0000E+00 | 0.0000E+00 | 0.0000E+00 | |
f24 | ave | 2.6000E+03 | 2.6000E+03 | 2.6000E+03 | 2.6000E+03 | 2.6000E+03 | 2.6000E+03 |
std | 7.2310E-05 | 0.0000E+00 | 0.0000E+00 | 0.0000E+00 | 0.0000E+00 | 0.0000E+00 | |
f25 | ave | 2.7000E+03 | 2.7000E+03 | 2.7000E+03 | 2.7000E+03 | 2.7000E+03 | 2.7000E+03 |
std | 0.0000E+00 | 0.0000E+00 | 0.0000E+00 | 0.0000E+00 | 0.0000E+00 | 0.0000E+00 | |
f26 | ave | 2.8000E+03 | 2.8000E+03 | 2.8000E+03 | 2.8000E+03 | 2.8000E+03 | 2.8000E+03 |
std | 0.0000E+00 | 0.0000E+00 | 0.0000E+00 | 0.0000E+00 | 0.0000E+00 | 0.0000E+00 | |
f27 | ave | 2.9000E+03 | 2.9000E+03 | 2.9000E+03 | 2.9000E+03 | 2.9000E+03 | 2.9000E+03 |
std | 1.3781E-12 | 0.0000E+00 | 0.0000E+00 | 0.0000E+00 | 0.0000E+00 | 0.0000E+00 | |
f28 | ave | 3.0000E+03 | 3.0000E+03 | 3.0000E+03 | 3.0000E+03 | 3.0000E+03 | 3.0000E+03 |
std | 9.1873E-13 | 0.0000E+00 | 0.0000E+00 | 0.0000E+00 | 0.0000E+00 | 0.0000E+00 | |
f29 | ave | 3.1000E+03 | 3.1000E+03 | 3.1000E+03 | 3.1000E+03 | 3.1000E+03 | 3.1000E+03 |
std | 0.0000E+00 | 0.0000E+00 | 0.0000E+00 | 0.0000E+00 | 0.0000E+00 | 0.0000E+00 | |
f30 | ave | 3.2000E+03 | 3.2000E+03 | 5.3231E+02 | 1.3189E+02 | 4.7449E+02 | 4.1959E+02 |
std | 0.0000E+00 | 0.0000E+00 | 0.0000E+00 | 0.0000E+00 | 0.0000E+00 | 0.0000E+00 |
Unimodal Functions | Simple Multimodal Functions | Hybrid Functions | Composition Functions | All Functions | |
HHO | 5.00 | 4.77 | 5.00 | 1.50 | 3.97 |
CEHHO | 6.00 | 5.46 | 6.00 | 1.50 | 4.57 |
DMSDL-HHO | 3.67 | 2.46 | 2.67 | 1.38 | 2.33 |
ADHHO | 3.33 | 2.92 | 1.67 | 1.00 | 2.20 |
CLHHEO | 1.33 | 3.08 | 2.83 | 1.25 | 2.37 |
QC-HHO | 1.67 | 2.31 | 2.83 | 1.13 | 2.03 |
Function No. | HHO | CEHHO | DMSDL-HHO | ADHHO | CLHHEO | QC-HHO |
F1 | 0.1711 (1) | 0.1898 (4) | 0.1979 (5) | 0.1994 (6) | 0.1855 (3) | 0.1851 (2) |
F2 | 1.0354 (4) | 1.0333 (3) | 1.0402 (5) | 1.0536 (6) | 1.0280 (2) | 1.0240 (1) |
F3 | 0.2127 (2) | 0.2134 (4) | 0.2130 (3) | 0.2252 (6) | 0.2161 (5) | 0.2120 (1) |
F4 | 0.3470 (1) | 0.3969 (5) | 0.3489 (3) | 0.3998 (6) | 0.3732 (4) | 0.3483 (2) |
F5 | 0.3200 (2) | 0.3387 (4) | 0.3172 (1) | 0.3529 (6) | 0.3415 (5) | 0.3380 (3) |
F6 | 0.2748 (2) | 0.2853 (4) | 0.2854 (5) | 0.2900 (6) | 0.2839 (3) | 0.2740 (1) |
F7 | 0.2775 (2) | 0.3013 (5) | 0.2782 (3) | 0.2967 (4) | 0.3072 (6) | 0.2770 (1) |
F8 | 0.8146 (1) | 0.8694 (6) | 0.8476 (5) | 0.8259 (2) | 0.8385 (4) | 0.8381 (3) |
F9 | 0.1752 (1) | 0.1950 (6) | 0.1947 (4) | 0.1909 (3) | 0.1889 (2) | 0.1948 (5) |
F10 | 0.4427 (1) | 0.4727 (5) | 0.4679 (3) | 0.4762 (6) | 0.4427 (1) | 0.4720 (4) |
ave | 0.4071 | 0.4296 | 0.4191 | 0.4311 | 0.4206 | 0.4163 |
rank | 1 | 5 | 3 | 6 | 4 | 2 |
HHO | CEHHO | DMSDL-HHO | ADHHO | CLHHEO | QC-HHO | |
f1 | 2.5712 (1) | 3.8191 (6) | 3.1869 (4) | 3.7112 (5) | 2.9971 (3) | 2.9932 (2) |
f2 | 2.2815 (1) | 3.3411 (4) | 3.0629 (3) | 3.6919 (6) | 3.5918 (5) | 3.0009 (2) |
f3 | 2.3558 (1) | 3.3897 (3) | 4.0936 (4) | 4.2559 (5) | 4.2625 (6) | 3.0685 (2) |
f4 | 2.2709 (1) | 3.4221 (6) | 3.1982 (3) | 3.2618 (4) | 3.3069 (5) | 3.0166 (2) |
f5 | 2.6414 (1) | 3.8369 (4) | 3.4979 (3) | 3.9471 (5) | 3.9995 (6) | 3.4643 (2) |
f6 | 15.0265 (1) | 16.5892 (6) | 15.3747 (3) | 15.4918 (4) | 15.7313 (5) | 15.1352 (2) |
f7 | 2.4981 (1) | 3.6428 (3) | 3.8173 (5) | 3.8433 (6) | 3.7917 (4) | 3.5909 (2) |
f8 | 2.3186 (2) | 3.4396 (5) | 2.1830 (1) | 2.3421 (3) | 3.5208 (6) | 3.3858 (4) |
f9 | 2.5208 (1) | 3.6129 (4) | 3.8699 (5) | 3.8792 (6) | 3.5934 (2) | 3.5991 (3) |
f10 | 2.5110 (1) | 3.5748 (5) | 3.5401 (4) | 3.8220 (6) | 3.4978 (2) | 3.5326 (3) |
f11 | 2.7039 (1) | 3.8528 (3) | 4.1015 (6) | 3.9709 (5) | 3.9666 (4) | 3.8243 (2) |
f12 | 5.9112 (3) | 8.4490 (4) | 5.6371 (1) | 9.0890 (6) | 5.7282 (2) | 8.5186 (5) |
f13 | 2.3970 (1) | 3.4216 (4) | 4.7038 (5) | 5.1111 (6) | 3.1425 (2) | 3.3602 (3) |
f14 | 2.3843 (1) | 3.4155 (5) | 2.4024 (3) | 2.4702 (4) | 3.4258 (6) | 2.3998 (2) |
f15 | 2.5474 (1) | 3.7753 (3) | 4.1422 (5) | 3.9425 (4) | 3.7215 (2) | 4.6415 (6) |
f16 | 2.6729 (3) | 4.2012 (5) | 2.4023 (2) | 4.4965 (6) | 2.3619 (1) | 2.9426 (4) |
f17 | 2.6649 (1) | 3.9537 (3) | 4.2704 (5) | 4.3114 (6) | 3.9855 (4) | 3.8294 (2) |
f18 | 2.4950 (1) | 3.5390 (2) | 4.4723 (5) | 4.5069 (6) | 4.3060 (4) | 3.8235 (3) |
f19 | 4.7688 (1) | 7.0241 (4) | 7.1387 (5) | 7.5082 (6) | 6.9847 (2) | 7.0149 (3) |
f20 | 2.5339 (1) | 3.6043 (2) | 4.3699 (5) | 4.4119 (6) | 4.2767 (4) | 3.7216 (3) |
f21 | 2.6110 (1) | 3.6399 (4) | 3.9836 (6) | 3.7167 (5) | 3.4723 (3) | 3.3203 (2) |
f22 | 2.9906 (1) | 4.2424 (4) | 4.2634 (5) | 4.2992 (6) | 4.2040 (3) | 4.1862 (2) |
f23 | 3.6790 (1) | 5.3735 (4) | 4.3400 (2) | 5.4874 (5) | 5.6162 (6) | 5.2882 (3) |
f24 | 3.1085 (1) | 4.3348 (4) | 5.1338 (5) | 5.1898 (6) | 4.2848 (3) | 4.2692 (2) |
f25 | 3.5959 (1) | 5.0386 (2) | 6.7478 (6) | 5.1213 (3) | 5.2570 (5) | 5.2076 (4) |
f26 | 16.3414 (1) | 21.0678 (4) | 17.5273 (2) | 21.8697 (5) | 22.3253 (6) | 20.3958 (3) |
f27 | 16.4166 (1) | 21.0634 (4) | 19.1805 (2) | 19.5642 (3) | 21.5892 (5) | 22.3870 (6) |
f28 | 4.2274 (1) | 5.9362 (2) | 8.2345 (6) | 6.0785 (3) | 6.4602 (4) | 8.0234 (5) |
f29 | 5.6623 (1) | 7.8752 (5) | 7.5715 (4) | 7.9956 (6) | 7.4263 (3) | 7.3215 (2) |
f30 | 3.6760 (1) | 5.3156 (6) | 4.7602 (3) | 4.8526 (4) | 4.6273 (2) | 5.1187 (5) |
Ave | 4.3461 | 5.9264 | 5.7069 | 6.0747 | 5.8485 | 5.8127 |
rank | 1 | 5 | 2 | 6 | 4 | 3 |
HHO | BFO | WOA | PSO | DE | CEHHO | DMSDL- HHO | ADHHO | CLHHEO | |
F1 | 9.40E-12 | 9.40E-12 | 9.40E-12 | 9.40E-12 | 9.40E-12 | 9.40E-12 | 9.40E-12 | 9.40E-12 | 9.40E-12 |
F2 | 2.26E-11 | 2.26E-11 | 2.26E-11 | 2.26E-11 | 2.26E-11 | 2.26E-11 | 2.26E-11 | 2.26E-11 | 2.26E-11 |
F3 | 3.02E-11 | 3.02E-11 | 3.02E-11 | 3.02E-11 | 3.02E-11 | 3.02E-11 | 3.02E-11 | 3.02E-11 | 3.02E-11 |
F4 | 4.91E-11 | 3.03E-11 | 1.17E-11 | 3.02E-11 | 3.02E-11 | 4.71E-11 | 4.79E-11 | 4.66E-11 | 4.97E-11 |
F5 | 1.72E-12 | 1.72E-12 | 1.72E-12 | 1.72E-12 | 1.72E-12 | 1.72E-12 | 1.72E-12 | 1.72E-12 | 1.72E-12 |
F6 | 1.21E-12 | 1.21E-12 | 1.21E-12 | 1.21E-12 | 1.21E-12 | 1.21E-12 | 1.21E-12 | 1.21E-12 | 1.21E-12 |
F7 | 1.21E-12 | 1.21E-12 | 3.63E-09 | 1.21E-12 | 1.21E-12 | 1.21E-12 | 1.21E-12 | 1.21E-12 | 1.21E-12 |
F8 | 1.21E-12 | 1.21E-12 | 1.21E-12 | 1.21E-12 | 1.21E-12 | 1.21E-12 | 1.21E-12 | 1.21E-12 | 1.21E-12 |
F9 | 3.02E-11 | 1.07E-07 | 3.69E-11 | 1.46E-10 | NaN | 2.99E-11 | 2.98E-11 | 2.92E-11 | 2.80E-11 |
F10 | 3.69E-11 | 3.02E-11 | 9.76E-10 | 4.62E-10 | 9.92E-10 | 3.76E-11 | 3.77E-11 | 3.81E-11 | 3.53E-11 |
HHO | CEHHO | DMSDL-HHO | ADHHO | CLHHEO | |
f1 | 1.7705E-08 | 5.2425E-09 | 4.6262E-05 | 1.1551E-03 | 4.6508E-05 |
f2 | 3.5327E-09 | 2.7663E-09 | 4.7363E-05 | 4.7113E-05 | 4.3478E-05 |
f3 | 8.7391E-07 | 6.3286E-06 | 4.7284E-05 | 1.1551E-03 | 4.7527E-05 |
f4 | 1.0382E-06 | 1.2266E-05 | 4.8506E-05 | 4.7113E-05 | 4.8058E-05 |
f5 | 2.6731E-01 | 9.0608E-01 | 2.5354E-01 | 2.5354E-01 | 2.5354E-01 |
f6 | 2.9196E-05 | 4.7397E-05 | 4.5470E-04 | 4.9405E-04 | 4.5264E-04 |
f7 | 2.0927E-07 | 9.0425E-06 | 4.7493E-05 | 6.0475E-05 | 5.0695E-05 |
f8 | 4.8206E-04 | 3.0793E-03 | 3.8648E-05 | 9.1699E-03 | 3.8831E-05 |
f9 | 1.6326E-04 | 2.2196E-04 | 5.7790E-04 | 4.6421E-04 | 5.7327E-04 |
f10 | 2.3410E-05 | 1.4001E-03 | 9.3708E-04 | 6.5117E-03 | 9.6393E-04 |
f11 | 5.1528E-04 | 8.9460E-03 | 5.3768E-05 | 1.1551E-03 | 5.3884E-05 |
f12 | 2.8497E-04 | 5.5144E-04 | 4.7290E-05 | 4.7113E-05 | 4.7754E-05 |
f13 | 7.1943E-03 | 1.8116E-03 | 9.5190E-03 | 4.2925E-01 | 9.5909E-03 |
f14 | 2.1220E-03 | 5.1121E-03 | 5.4286E-05 | 1.4169E-03 | 5.5851E-05 |
f15 | 2.6195E-03 | 2.1976E-04 | 4.6889E-05 | 4.7113E-05 | 4.7320E-05 |
f16 | 1.1162E-04 | 2.4663E-03 | 5.6092E-04 | 4.9265E-04 | 5.5844E-04 |
f17 | 2.4221E-05 | 9.3334E-05 | 4.7033E-05 | 1.1551E-03 | 3.8066E-05 |
f18 | 4.1052E-04 | 4.0984E-02 | 5.2596E-05 | 1.5099E-02 | 5.2665E-05 |
f19 | 1.9152E-03 | 4.8671E-03 | 4.9178E-05 | 4.2925E-04 | 5.2957E-05 |
f20 | 8.5933E-05 | 4.4240E-04 | 4.6667E-05 | 4.7113E-05 | 4.6744E-05 |
f21 | 9.6968E-05 | 1.5948E-03 | 4.7318E-05 | 4.7113E-05 | 5.3494E-05 |
f22 | 1.1347E-04 | 8.9985E-03 | 4.6991E-05 | 4.7113E-05 | 4.6674E-05 |
f23 | 6.0998E-03 | 3.6646E-04 | 1.7514E-02 | 1.2542E-04 | 1.8586E-02 |
f24 | 4.7882E-04 | 2.1092E-05 | 3.0202E-03 | 4.7113E-05 | 3.2206E-03 |
f25 | 3.5328E-03 | 7.4526E-03 | 2.4097E-05 | 9.8325E-05 | 2.4222E-05 |
f26 | 8.3156E-04 | 5.4782E-03 | 2.5678E-02 | 4.7113E-05 | 2.5918E-02 |
f27 | 1.1901E-03 | 1.1267E-02 | 2.2684E-05 | 1.5890E-04 | 2.2524E-05 |
f28 | 1.0185E-03 | 7.8966E-03 | 2.6043E-03 | 4.7113E-05 | 2.2437E-03 |
f29 | 7.1010E-05 | 5.6701E-03 | 1.1766E-02 | 4.7113E-05 | 1.0596E-02 |
f30 | 1.4942E-02 | 4.1432E-02 | 4.7113E-05 | 4.7113E-05 | 5.1618E-05 |
(x, y) | q | Optimal Cost (s) | Position deviation (%) | Release rate deviation (%) | |
QC-HHO | (1.98, 2.97) | 30.21 | 193.49 | 0.036 | 0.7 |
CLHHEO | (2.05, 3.02) | 31.04 | 194.05 | 0.042 | 3.47 |
ADHHO | (1.97, 3.04) | 29.56 | 202.01 | 0.041 | 1.47 |
DMSDL-HHO | (2.02, 2.96) | 30.28 | 200.21 | 0.05 | 0.93 |
CEHHO | (1.93, 2.92) | 28.97 | 196.77 | 0.092 | 3.43 |
HHO | (1.93, 2.92) | 28.39 | 190.21 | 0.117 | 5.37 |
Iteration No. | f(XNM-Optimal) | f(XNM-Subworst) | f(XNM-Worst) | f(XNMe) | f(XNMr) | f(XNMc1) | f(XNMc2) | Action |
1 | 1394.21 | 5893.56 | 7526.89 | — | — | — | — | Sort |
1394.21 | 5893.56 | 31.09 | 31.09 | 749.80 | — | — | Expansion | |
2 | 31.09 | 1394.21 | 5893.56 | 31.09 | 749.80 | — | — | Sort |
31.09 | 1394.21 | 3.54 | 3.54 | 21.96 | — | — | Expansion | |
3 | 3.54 | 31.09 | 1394.21 | 3.54 | 21.96 | — | — | Sort |
3.54 | 31.09 | 8.22 | 3.54 | 8.22 | — | — | Reflection <1> | |
4 | 3.54 | 8.22 | 31.09 | 3.54 | 8.22 | — | — | Sort |
3.54 | 1.25 | 86.88 | 3.54 | 1.39e+4 | — | 188.77 | Shrink | |
5 | 1.25 | 3.54 | 86.88 | 3.54 | 1.39e+4 | — | 188.77 | Sort |
1.25 | 3.54 | 71.21 | 3.54 | 1675.13 | — | 71.21 | Inside contraction | |
...... | ...... | |||||||
12 | 0.07 | 0.19 | 0.26 | 3.54 | 3.10 | — | 0.19 | Sort |
0.07 | 0.19 | 0.14 | 3.54 | 0.26 | 0.14 | 0.19 | Outside contraction | |
...... | ...... | |||||||
19 | 0.0103 | 0.0263 | 0.0263 | 3.5432 | 0.0263 | 0.1447 | 0.0263 | Sort |
0.0103 | 0.0263 | 0.0084 | 0.0331 | 0.0084 | 0.1447 | 0.0263 | Reflection <2> |
Figure | Function No. | Function Name | Number of Variables | Boundary | Optimal Solution |
![]() |
F1 | Sphere | 30 | [-100,100] | 0 |
![]() |
F2 | Schwefel 1.2 | 30 | [-100,100] | 0 |
![]() |
F3 | Schwefel 2.21 | 30 | [-100,100] | 0 |
![]() |
F4 | Quartic with Noise | 30 | [−1.28, 1.28] | 0 |
![]() |
F5 | Schwefel | 30 | [−500,500] | −418.9829 × 5 |
![]() |
F6 | Rastrigin | 30 | [−5.12, 5.12] | 0 |
![]() |
F7 | Ackley | 30 | [−32, 32] | 0 |
![]() |
F8 | Penalized 1.1 | 30 | [−50, 50] | 0 |
![]() |
F9 | Goldstein-Price | 2 | [−2, 2] | 3 |
![]() |
F10 | Shekel 5 | 4 | [0, 10] | −10.1532 |
Function No. | metrics | BFO | PSO | DE | HHO | QC-HHO |
F1 | mean | 5.67E-05 | 3.89E-01 | 1.01E-13 | 1.13E-97 | 0.00E+00 |
std | 8.46E-06 | 1.29E-01 | 7.61E-14 | 2.48E-97 | 0.00E+00 | |
F2 | mean | 2.66E-04 | 3.54E+01 | 8.50E-11 | 5.89E-79 | 0.00E+00 |
std | 4.50E-05 | 1.46E+01 | 9.14E-11 | 1.81E-78 | 0.00E+00 | |
F3 | mean | 4.34E-03 | 2.61E+00 | 0.00E+00 | 3.11E-50 | 0.00E+00 |
std | 5.68E-04 | 1.29E+00 | 0.00E+00 | 6.55E-50 | 1.01E-101 | |
F4 | mean | 2.50E-04 | 3.55E+00 | 5.63E-03 | 1.97E-04 | 4.23E-05 |
std | 2.16E-04 | 8.49E+00 | 1.48E-03 | 2.05E-04 | 4.18E-05 | |
F5 | mean | -2.01E+03 | -2.74E+03 | -1.12E+04 | -1.26E+04 | -1.44E+04 |
std | 4.40E+02 | 3.61E+02 | 5.75E+02 | 1.68E+00 | 1.85E-12 | |
F6 | mean | 1.11E-02 | 9.82E+01 | 6.92E+01 | 0.00E+00 | 0.00E+00 |
std | 1.72E-03 | 1.26E+01 | 3.88E+01 | 0.00E+00 | 0.00E+00 | |
F7 | mean | 5.85E-03 | 2.56E+00 | 1.18E-07 | 8.88E-16 | 8.88E-16 |
std | 4.33E-04 | 1.13E+00 | 4.96E-08 | 0.00E+00 | 0.00E+00 | |
F8 | mean | 1.56E+00 | 3.43E+00 | 9.76E-15 | 1.03E-05 | 1.57E-22 |
std | 7.01E-03 | 1.10E+00 | 9.85E-15 | 1.33E-05 | 5.57E-48 | |
F9 | mean | 8.40E+00 | 3.00E+00 | 3.73E+00 | 3.00E+00 | 3.00E+00 |
std | 1.14E+01 | 5.91E-04 | 2.57E-15 | 2.29E-07 | 4.38E-02 | |
F10 | mean | -7.91E-01 | -4.15E+00 | -1.17E+01 | -5.05E+00 | -9.97E+00 |
std | 5.20E-01 | 2.40E+00 | 2.89E-06 | 1.64E-02 | 1.56E+00 |
Function No. | metrics | CEHHO | DMSDL-HHO | ADHHO | CLHHEO | QC-HHO |
f1 | ave | 1.0600E-111 | 0.0000E+00 | 2.5300E-196 | 2.9200E-187 | 0.0000E+00 |
std | 5.2400E-111 | 0.0000E+00 | 8.8820E-63 | 0.0000E+00 | 0.0000E+00 | |
rank | 5 | 1 | 3 | 4 | 1 | |
f2 | ave | 2.3400E-89 | 0.0000E+00 | 2.2653E-102 | 9.3000E-116 | 0.0000E+00 |
std | 1.2700E-88 | 0.0000E+00 | 1.1250E-98 | 4.9100E-115 | 0.0000E+00 | |
rank | 5 | 1 | 4 | 3 | 1 | |
f3 | ave | 8.3500E-58 | 0.0000E+00 | 8.9240E-74 | 4.0400E-74 | 0.0000E+00 |
std | 1.9700E-67 | 0.0000E+00 | 1.1230E-70 | 1.5900E-73 | 1.0100E-101 | |
rank | 5 | 1 | 4 | 3 | 1 | |
f4 | ave | 1.4900E-04 | 1.3380E-04 | 4.2924E-05 | 4.0479E-05 | 4.2300E-05 |
std | 1.2800E-05 | 1.4544E-04 | 4.3058E-05 | 4.3225E-05 | 4.1800E-05 | |
rank | 5 | 4 | 3 | 1 | 2 | |
f5 | ave | -1.3506E+04 | -1.3803E+04 | -1.2730E+04 | -1.3511E+04 | -1.4443E+04 |
std | -1.2600E+04 | 1.7569E+03 | 5.0093E-04 | -1.2691E+04 | 1.8500E-12 | |
rank | 4 | 2 | 5 | 3 | 1 | |
f6 | ave | 0.0000E+00 | 0.0000E+00 | 0.0000E+00 | 0.0000E+00 | 0.0000E+00 |
std | 0.0000E+00 | 0.0000E+00 | 0.0000E+00 | 0.0000E+00 | 0.0000E+00 | |
rank | 1 | 1 | 1 | 1 | 1 | |
f7 | ave | 8.8800E-16 | 8.8800E-16 | 8.8800E-16 | 8.8800E-16 | 8.8800E-16 |
std | 0.0000E+00 | 0.0000E+00 | 0.0000E+00 | 0.0000E+00 | 0.0000E+00 | |
rank | 1 | 1 | 1 | 1 | 1 | |
f8 | ave | 8.4400E-06 | 4.1151E-13 | 1.7461E-24 | 2.8600E-12 | 1.5700E-22 |
std | 2.9600E-09 | 2.7293E-13 | 3.3065E-07 | 3.5400E-12 | 5.5700E-48 | |
rank | 5 | 3 | 1 | 4 | 2 | |
f9 | ave | 3.0000E+00 | 3.0000E+00 | 3.0000E+00 | 3.0000E+00 | 3.0000E+00 |
std | 1.6400E-07 | 3.2183E-15 | 5.9349E-06 | 7.6682E-07 | 4.3800E-02 | |
rank | 1 | 1 | 1 | 1 | 1 | |
f10 | ave | -5.3600E+00 | -1.0151E+01 | -5.4742E+00 | -1.0133E+01 | -9.9654E+00 |
std | 1.1700E+00 | 8.5892E-15 | 2.3396E-01 | 1.0048E-03 | 1.5600E+00 | |
rank | 5 | 1 | 4 | 2 | 3 |
metrics | HHO | CEHHO | DMSDL- HHO | AD-HHO | CLHHEO | QC-HHO | |
f1 | ave | 3.4388E+07 | 6.7119E+07 | 9.2986E+00 | 9.3981E+00 | 6.0859E+04 | 8.2197E+00 |
std | 1.5816E+07 | 1.7777E+07 | 1.3081E+01 | 1.2775E+01 | 2.0189E+07 | 1.5221E+01 | |
f2 | ave | 3.4053E+07 | 1.4616E+08 | 1.9284E-07 | 1.8058E-07 | 7.4597E+05 | 1.7759E-07 |
std | 1.1072E+07 | 5.5324E+07 | 1.0708E-07 | 8.9939E-08 | 4.8320E+07 | 1.1139E-07 | |
f3 | ave | 2.4042E+04 | 3.7078E+04 | 2.6431E-01 | 4.4136E-01 | 5.6404E+02 | 3.6351E-01 |
std | 5.4667E+03 | 1.3501E+04 | 3.0418E-01 | 2.9975E-01 | 1.0972E+04 | 3.4800E-01 | |
f4 | ave | 6.2954E+02 | 8.1557E+02 | 3.0498E-10 | 3.0772E-10 | 1.2677E-10 | 3.3697E-10 |
std | 6.7784E+01 | 2.0063E+02 | 1.0968E-10 | 1.1019E-10 | 1.0309E+02 | 7.3327E-11 | |
f5 | ave | 5.2052E+02 | 5.2059E+02 | 5.1904E+02 | 5.1888E+02 | 5.0843E+02 | 5.1453E+02 |
std | 1.2532E-01 | 1.4775E-01 | 2.7139E-04 | 3.0074E-04 | 1.4283E-01 | 2.3809E-04 | |
f6 | ave | 6.3334E+02 | 6.3626E+02 | 1.0219E+02 | 1.3807E+02 | 4.4244E+02 | 3.9525E+01 |
std | 3.3253E+00 | 2.9463E+00 | 2.4023E+00 | 2.8022E+00 | 3.3249E+00 | 2.3540E+00 | |
f7 | ave | 7.0130E+02 | 7.0243E+02 | 9.3954E-11 | 1.6007E-10 | 9.3062E-07 | 9.4327E-12 |
std | 8.5586E-02 | 6.3357E-01 | 1.8047E-14 | 1.8914E-14 | 1.0667E+00 | 1.5569E-14 | |
f8 | ave | 9.3159E+02 | 9.4180E+02 | 2.2990E+02 | 2.7292E+02 | 1.6638E+02 | 1.6360E+02 |
std | 1.7349E+01 | 2.2440E+01 | 1.0357E+01 | 9.9155E+00 | 1.6189E+01 | 1.8853E+01 | |
f9 | ave | 1.0874E+03 | 1.1007E+03 | 2.8799E+02 | 4.0555E+02 | 3.5026E+02 | 2.7859E+02 |
std | 2.0406E+01 | 2.0994E+01 | 1.3628E+01 | 1.3421E+01 | 1.7502E+01 | 1.4507E+01 | |
f10 | ave | 4.0048E+03 | 4.1507E+03 | 8.6068E+02 | 7.3350E+02 | 1.9039E+03 | 7.7819E+02 |
std | 7.8162E+02 | 6.1751E+02 | 2.8306E+02 | 2.7512E+02 | 2.3224E+02 | 3.0040E+02 | |
f11 | ave | 5.6507E+03 | 5.7285E+03 | 3.1228E+03 | 3.8417E+03 | 5.4454E+03 | 6.0754E+03 |
std | 7.8910E+02 | 5.5742E+02 | 7.7495E+02 | 6.1912E+02 | 5.1104E+02 | 6.9601E+02 | |
f12 | ave | 1.2019E+03 | 1.2021E+03 | 2.5912E+02 | 2.8480E+02 | 1.6350E+02 | 4.3482E+01 |
std | 4.7933E-01 | 6.9743E-01 | 2.9394E-01 | 2.7541E-01 | 6.4237E-01 | 2.7386E-01 | |
f13 | ave | 1.3005E+03 | 1.3005E+03 | 6.2438E+02 | 6.6377E+02 | 1.0466E+03 | 6.1473E+02 |
std | 1.5096E-01 | 1.2744E-01 | 1.0724E-01 | 8.1349E-02 | 1.0359E-01 | 7.3202E-02 | |
f14 | ave | 1.4003E+03 | 1.4004E+03 | 1.0463E+03 | 1.1425E+03 | 1.1245E+03 | 8.6137E+02 |
std | 1.0112E-01 | 2.7234E-01 | 2.5115E-02 | 2.2234E-02 | 3.0647E-01 | 1.5575E-02 | |
f15 | ave | 1.5453E+03 | 1.5601E+03 | 1.5535E+02 | 1.4709E+02 | 1.3600E+02 | 1.2897E+03 |
std | 8.9250E+00 | 1.7244E+01 | 1.1863E+00 | 9.3748E-01 | 2.0126E+01 | 1.2965E+00 | |
f16 | ave | 1.6124E+03 | 1.6123E+03 | 1.1359E+03 | 1.3203E+03 | 7.7656E+02 | 9.7576E+02 |
std | 4.8097E-01 | 5.9799E-01 | 6.7343E-01 | 6.8893E-01 | 6.3623E-01 | 7.4550E-01 | |
f17 | ave | 4.4967E+06 | 5.7408E+06 | 8.6200E+03 | 7.0382E+03 | 5.6425E+04 | 1.0090E+04 |
std | 3.6931E+06 | 3.3646E+06 | 5.5689E+03 | 4.7452E+03 | 2.9065E+06 | 5.0348E+03 | |
f18 | ave | 1.9322E+05 | 7.8069E+05 | 2.5421E+03 | 2.3654E+03 | 7.8574E+03 | 2.8281E+03 |
std | 3.4242E+05 | 7.2558E+05 | 5.2332E+03 | 3.7030E+03 | 7.7741E+05 | 3.5642E+03 | |
f19 | ave | 1.9518E+03 | 1.9926E+03 | 1.8122E+02 | 2.2464E+02 | 1.3694E+03 | 1.5917E+02 |
std | 3.6942E+01 | 3.4779E+01 | 8.3276E-01 | 8.9098E-01 | 3.9001E+01 | 8.6310E-01 | |
f20 | ave | 2.5130E+04 | 4.7393E+04 | 1.1201E+03 | 1.0525E+03 | 1.0623E+03 | 1.6085E+03 |
std | 8.9647E+03 | 7.8229E+03 | 5.6089E+02 | 4.8117E+02 | 8.9803E+03 | 4.6628E+02 | |
f21 | ave | 7.8219E+05 | 7.8782E+05 | 1.0874E+03 | 1.1780E+03 | 6.7445E+04 | 7.9901E+03 |
std | 7.2130E+05 | 7.2787E+05 | 8.7689E+02 | 8.5450E+02 | 8.4029E+05 | 9.6452E+02 | |
f22 | ave | 3.1687E+03 | 3.2757E+03 | 1.3253E+03 | 7.9046E+02 | 5.8064E+02 | 1.4654E+03 |
std | 2.9168E+02 | 1.6299E+02 | 1.0242E+01 | 9.8666E+01 | 1.8406E+02 | 1.0312E+01 | |
f23 | ave | 2.5000E+03 | 2.5000E+03 | 2.5000E+03 | 2.5000E+03 | 2.5000E+03 | 2.5000E+03 |
std | 0.0000E+00 | 0.0000E+00 | 0.0000E+00 | 0.0000E+00 | 0.0000E+00 | 0.0000E+00 | |
f24 | ave | 2.6000E+03 | 2.6000E+03 | 2.6000E+03 | 2.6000E+03 | 2.6000E+03 | 2.6000E+03 |
std | 4.0537E-04 | 0.0000E+00 | 0.0000E+00 | 0.0000E+00 | 0.0000E+00 | 0.0000E+00 | |
f25 | ave | 2.7000E+03 | 2.7000E+03 | 2.7000E+03 | 2.7000E+03 | 2.7000E+03 | 2.7000E+03 |
std | 0.0000E+00 | 0.0000E+00 | 0.0000E+00 | 0.0000E+00 | 0.0000E+00 | 0.0000E+00 | |
f26 | ave | 2.7622E+03 | 2.7403E+03 | 2.0384E+03 | 1.9048E+03 | 1.9035E+03 | 2.5068E+03 |
std | 4.8819E+01 | 5.1410E+01 | 7.3958E-02 | 5.8292E-02 | 5.0192E+01 | 5.5044E-02 | |
f27 | ave | 2.9000E+03 | 2.9000E+03 | 2.9000E+03 | 2.9000E+03 | 2.9000E+03 | 2.9000E+03 |
std | 0.0000E+00 | 0.0000E+00 | 0.0000E+00 | 0.0000E+00 | 0.0000E+00 | 0.0000E+00 | |
f28 | ave | 3.0000E+03 | 3.0000E+03 | 3.0000E+03 | 3.0000E+03 | 3.0000E+03 | 3.0000E+03 |
std | 0.0000E+00 | 0.0000E+00 | 0.0000E+00 | 0.0000E+00 | 0.0000E+00 | 0.0000E+00 | |
f29 | ave | 6.2109E+05 | 2.6613E+06 | 3.2662E+05 | 3.0237E+05 | 2.8801E+05 | 3.0168E+05 |
std | 2.7211E+06 | 0.0000E+00 | 0.0000E+00 | 0.0000E+00 | 0.0000E+00 | 0.0000E+00 | |
f30 | ave | 8.2238E+04 | 4.5278E+04 | 4.4532E+03 | 4.1717E+03 | 4.2684E+04 | 5.9009E+03 |
std | 1.4431E+05 | 0.0000E+00 | 0.0000E+00 | 0.0000E+00 | 0.0000E+00 | 0.0000E+00 |
Unimodal Functions | Simple Multimodal Functions | Hybrid Functions | Composition Functions | All Functions | |
HHO | 5.00 | 5.08 | 5.00 | 2.75 | 4.43 |
CEHHO | 6.00 | 5.77 | 6.00 | 2.63 | 5.00 |
DMSDL-HHO | 2.00 | 2.46 | 2.17 | 1.75 | 2.17 |
ADHHO | 2.67 | 3.08 | 1.67 | 1.38 | 2.30 |
CLHHEO | 4.00 | 2.54 | 3.17 | 1.38 | 2.50 |
QC-HHO | 1.33 | 2.08 | 3.00 | 1.75 | 2.10 |
metrics | HHO | CEHHO | DMSDL- HHO | ADHHO | CLHHEO | QC-HHO | |
f1 | ave | 8.8085E+07 | 1.5267E+08 | 1.6076E+00 | 1.5998E+00 | 1.3999E+00 | 1.3853E+00 |
std | 3.3967E+07 | 6.2054E+07 | 2.3140E+00 | 2.0577E+00 | 2.6593E+00 | 1.6336E-01 | |
f2 | ave | 6.5654E+08 | 2.2991E+09 | 1.3291E+03 | 1.1442E+03 | 1.5104E+03 | 1.0161E+03 |
std | 2.5436E+08 | 7.6478E+08 | 6.7502E+02 | 6.2056E+02 | 6.7748E+02 | 6.5509E+02 | |
f3 | ave | 5.7216E+04 | 8.6216E+04 | 1.5200E-01 | 1.6903E-01 | 1.8400E-01 | 1.8167E-01 |
std | 1.0290E+04 | 1.4523E+04 | 1.6414E-02 | 1.9100E-02 | 2.2674E-02 | 1.7807E-02 | |
f4 | ave | 9.2274E+02 | 1.1512E+03 | 1.1415E-01 | 8.8438E-02 | 7.2032E-02 | 6.6518E-02 |
std | 1.3692E+02 | 1.3042E+02 | 1.4276E+00 | 1.7794E+00 | 1.4689E+00 | 1.4655E+00 | |
f5 | ave | 5.2084E+02 | 5.2079E+02 | 5.4338E+03 | 5.1954E+03 | 4.5483E+03 | 5.9474E+02 |
std | 1.2934E-01 | 1.5010E-01 | 1.7678E-05 | 1.9315E-05 | 2.8812E-05 | 1.6617E-05 | |
f6 | ave | 6.6089E+02 | 6.6458E+02 | 2.9099E+02 | 2.5444E+02 | 2.2542E+02 | 2.6008E+02 |
std | 4.8858E+00 | 3.4152E+00 | 4.4083E+00 | 4.1760E+00 | 4.6698E+00 | 3.1034E+00 | |
f7 | ave | 7.0626E+02 | 7.2412E+02 | 1.5010E+00 | 1.3966E+00 | 1.2825E+00 | 1.7613E+00 |
std | 1.9491E+00 | 6.5846E+00 | 9.1075E-02 | 9.1857E-02 | 7.6984E-02 | 1.0520E-01 | |
f8 | ave | 1.0830E+03 | 1.0884E+03 | 5.9830E+02 | 5.6709E+02 | 2.1714E+02 | 4.8943E+02 |
std | 2.4935E+01 | 2.8755E+01 | 2.2672E+01 | 2.6441E+01 | 2.6903E+01 | 5.4955E+00 | |
f9 | ave | 1.2985E+03 | 1.3268E+03 | 5.3727E+02 | 6.7579E+02 | 6.6299E+02 | 5.4358E+02 |
std | 4.1898E+01 | 3.8579E+01 | 4.4425E+01 | 3.5146E+01 | 5.6706E+01 | 4.3760E+01 | |
f10 | ave | 7.4406E+03 | 8.3494E+03 | 1.5085E+03 | 3.1313E+03 | 2.7626E+03 | 1.8091E+03 |
std | 1.3736E+03 | 9.9589E+02 | 8.3676E+02 | 7.3305E+02 | 8.4150E+02 | 8.0097E+02 | |
f11 | ave | 1.0140E+04 | 1.1356E+04 | 6.5184E+03 | 7.7585E+03 | 9.2076E+03 | 6.2393E+02 |
std | 1.1277E+03 | 1.4072E+03 | 9.3317E+02 | 7.8877E+02 | 7.5315E+02 | 1.0175E+03 | |
f12 | ave | 1.2027E+03 | 1.2029E+03 | 1.1083E+02 | 1.3577E+02 | 2.0733E+02 | 8.4096E+01 |
std | 5.6534E-01 | 7.1506E-01 | 1.2631E-01 | 1.8406E-01 | 1.7612E-01 | 1.0291E-01 | |
f13 | ave | 1.3006E+03 | 1.3006E+03 | 1.1865E+03 | 1.0192E+03 | 1.2148E+03 | 1.9979E+03 |
std | 1.1094E-01 | 1.5253E-01 | 6.3924E-02 | 5.5773E-02 | 5.2991E-03 | 6.2082E-02 | |
f14 | ave | 1.4004E+03 | 1.4004E+03 | 9.4218E+02 | 1.1219E+03 | 2.5971E+02 | 9.4118E+02 |
std | 1.0639E-01 | 1.5366E-01 | 8.4510E-02 | 6.8426E-02 | 5.3345E-03 | 7.6521E-02 | |
f15 | ave | 1.6353E+03 | 2.0286E+03 | 1.6569E+02 | 1.4874E+02 | 1.5910E+02 | 1.6023E+02 |
std | 4.9995E+01 | 4.0222E+02 | 3.8812E+00 | 4.6768E+00 | 3.8753E+00 | 3.5583E+00 | |
f16 | ave | 1.6222E+03 | 1.6222E+03 | 1.5002E+03 | 1.4165E+03 | 1.7790E+03 | 1.3866E+03 |
std | 4.2170E-01 | 5.1725E-01 | 2.9190E-01 | 6.5435E-01 | 6.4365E-01 | 4.4801E-01 | |
f17 | ave | 1.4557E+07 | 3.9310E+07 | 1.8954E+04 | 1.8389E+04 | 2.1112E+04 | 1.6400E+04 |
std | 8.7585E+06 | 1.6772E+07 | 1.0117E+04 | 1.0110E+04 | 8.0640E+03 | 7.4718E+03 | |
f18 | ave | 6.4198E+06 | 2.9993E+07 | 3.6657E+05 | 4.5792E+05 | 4.2545E+05 | 4.7209E+05 |
std | 2.1913E+07 | 5.3636E+07 | 1.8446E+06 | 3.5932E+06 | 3.8297E+06 | 1.6075E+06 | |
f19 | ave | 1.9772E+03 | 2.0031E+03 | 1.2075E+03 | 6.1665E+02 | 6.7387E+02 | 2.0142E+03 |
std | 2.5168E+01 | 3.4978E+01 | 1.4342E+01 | 1.3839E+01 | 1.2625E+01 | 1.5203E+01 | |
f20 | ave | 3.9538E+04 | 6.3072E+04 | 1.8738E+03 | 2.2161E+03 | 2.4283E+03 | 1.8695E+03 |
std | 1.3456E+04 | 1.8238E+04 | 1.1249E+03 | 1.2644E+03 | 1.1763E+03 | 1.2064E+03 | |
f21 | ave | 5.4741E+06 | 9.3026E+06 | 7.8663E+03 | 9.1266E+03 | 1.0157E+04 | 8.7394E+03 |
std | 2.5403E+06 | 5.1269E+06 | 5.8327E+03 | 5.4384E+03 | 4.0745E+03 | 4.4298E+03 | |
f22 | ave | 4.0893E+03 | 4.1887E+03 | 3.3122E+03 | 1.6926E+03 | 1.2617E+03 | 3.4122E+03 |
std | 4.1644E+02 | 4.0711E+02 | 2.9382E+02 | 2.7958E+02 | 3.1192E+02 | 1.4846E+01 | |
f23 | ave | 2.5000E+03 | 2.5000E+03 | 2.5000E+03 | 2.5000E+03 | 2.5000E+03 | 2.5000E+03 |
std | 0.0000E+00 | 0.0000E+00 | 0.0000E+00 | 0.0000E+00 | 0.0000E+00 | 0.0000E+00 | |
f24 | ave | 2.6000E+03 | 2.6000E+03 | 2.6000E+03 | 2.6000E+03 | 2.6000E+03 | 2.6000E+03 |
std | 1.2144E-04 | 0.0000E+00 | 0.0000E+00 | 0.0000E+00 | 0.0000E+00 | 0.0000E+00 | |
f25 | ave | 2.7000E+03 | 2.7000E+03 | 2.7000E+03 | 2.7000E+03 | 2.9068E+03 | 2.7000E+03 |
std | 0.0000E+00 | 0.0000E+00 | 0.0000E+00 | 0.0000E+00 | 0.0000E+00 | 0.0000E+00 | |
f26 | ave | 2.7801E+03 | 2.7900E+03 | 1.6534E+03 | 2.0271E+03 | 1.6382E+03 | 1.7901E+03 |
std | 4.0219E+01 | 3.1478E+01 | 4.7512E+01 | 4.7105E+01 | 5.4754E+01 | 4.0077E+01 | |
f27 | ave | 2.9000E+03 | 2.9000E+03 | 2.9000E+03 | 2.9000E+03 | 2.9000E+03 | 2.9000E+03 |
std | 0.0000E+00 | 0.0000E+00 | 0.0000E+00 | 0.0000E+00 | 0.0000E+00 | 0.0000E+00 | |
f28 | ave | 3.0000E+03 | 3.0000E+03 | 3.0000E+03 | 3.0000E+03 | 3.0000E+03 | 3.0000E+03 |
std | 4.5936E-13 | 0.0000E+00 | 0.0000E+00 | 0.0000E+00 | 0.0000E+00 | 0.0000E+00 | |
f29 | ave | 3.1000E+03 | 3.1000E+03 | 3.1000E+03 | 3.1000E+03 | 3.1000E+03 | 3.1000E+03 |
std | 0.0000E+00 | 0.0000E+00 | 0.0000E+00 | 0.0000E+00 | 0.0000E+00 | 0.0000E+00 | |
f30 | ave | 3.9043E+04 | 8.2553E+04 | 2.1650E+03 | 2.2834E+03 | 2.5747E+03 | 2.7625E+03 |
std | 1.2152E+05 | 0.0000E+00 | 0.0000E+00 | 0.0000E+00 | 0.0000E+00 | 0.0000E+00 |
Unimodal Functions | Simple Multimodal Functions | Hybrid Functions | Composition Functions | All Functions | |
HHO | 5.00 | 4.69 | 4.83 | 2.00 | 4.03 |
CEHHO | 6.00 | 5.38 | 5.83 | 2.25 | 4.70 |
DMSDL-HHO | 2.67 | 3.00 | 2.17 | 1.13 | 2.30 |
ADHHO | 2.33 | 2.85 | 2.33 | 1.50 | 2.33 |
CLHHEO | 3.33 | 2.69 | 2.83 | 1.88 | 2.57 |
QC-HHO | 1.67 | 2.38 | 3.00 | 1.63 | 2.23 |
Metrics | HHO | CEHHO | DMSDL- HHO | AD-HHO | CLHHEO | QC-HHO | |
f1 | ave | 2.9532E+08 | 4.5244E+08 | 2.2065E-01 | 2.3133E-01 | 1.8079E-01 | 2.0389E-01 |
std | 5.9293E+07 | 1.0812E+08 | 4.0428E-01 | 4.2021E-01 | 4.6860E-01 | 3.8815E-01 | |
f2 | ave | 1.4056E+10 | 2.3634E+10 | 4.0215E+01 | 3.2914E+01 | 2.4421E+01 | 2.3105E+01 |
std | 3.4046E+09 | 3.1652E+09 | 1.2254E+02 | 1.3453E+02 | 1.0388E+02 | 1.4275E+02 | |
f3 | ave | 1.5690E+05 | 1.9603E+05 | 4.3587E-01 | 4.0324E-01 | 1.2412E-01 | 3.8466E-01 |
std | 1.8735E+04 | 3.3780E+04 | 9.0178E-02 | 5.3367E-02 | 8.9880E-02 | 6.4643E-02 | |
f4 | ave | 2.5476E+03 | 4.2424E+03 | 1.3242E+01 | 1.1286E+01 | 1.3599E+01 | 8.2205E+00 |
std | 4.0800E+02 | 1.0406E+03 | 1.2069E+01 | 8.2972E+00 | 1.6341E+01 | 9.8653E+00 | |
f5 | ave | 5.2111E+02 | 5.2107E+02 | 5.1999E+02 | 6.5981E+02 | 8.4588E+02 | 5.2108E+02 |
std | 9.4023E-02 | 8.6480E-02 | 3.3689E-06 | 3.8421E-06 | 3.1172E-06 | 3.6386E-06 | |
f6 | ave | 7.4008E+02 | 7.5001E+02 | 4.3865E+02 | 5.1949E+02 | 5.0975E+02 | 5.3346E+02 |
std | 6.5795E+00 | 6.8974E+00 | 6.9352E+00 | 6.4936E+00 | 7.6965E+00 | 8.2792E+00 | |
f7 | ave | 8.4469E+02 | 9.8020E+02 | 1.2370E+00 | 1.0825E+00 | 1.2535E+00 | 1.1447E+00 |
std | 3.1984E+01 | 6.4082E+01 | 5.4277E-01 | 5.3984E-01 | 4.0310E-01 | 4.8305E-01 | |
f8 | ave | 1.5063E+03 | 1.5608E+03 | 1.0793E+03 | 1.0420E+03 | 1.0178E+03 | 3.9801E+02 |
std | 3.2668E+01 | 3.0244E+01 | 5.3529E+01 | 4.3120E+01 | 4.8401E+01 | 4.2877E+01 | |
f9 | ave | 1.8268E+03 | 1.8914E+03 | 1.5135E+03 | 2.6553E+03 | 3.3141E+02 | 1.9302E+03 |
std | 4.5102E+01 | 4.4228E+01 | 7.4349E+01 | 5.5063E+01 | 6.7256E+01 | 4.5389E+01 | |
f10 | ave | 1.7487E+04 | 1.9500E+04 | 8.3093E+03 | 1.9446E+03 | 9.1671E+03 | 1.8913E+03 |
std | 1.8860E+03 | 1.7014E+03 | 8.5620E+02 | 5.3573E+02 | 8.2863E+02 | 6.3636E+02 | |
f11 | ave | 2.2358E+04 | 2.3184E+04 | 1.6779E+04 | 1.5132E+04 | 1.8203E+04 | 2.1426E+04 |
std | 2.1316E+03 | 2.7566E+03 | 1.0591E+03 | 1.0470E+03 | 1.8372E+03 | 9.7728E+02 | |
f12 | ave | 1.2036E+03 | 1.2038E+03 | 1.7679E+02 | 3.4298E+02 | 1.7758E+02 | 2.7543E+02 |
std | 5.1525E-01 | 4.3964E-01 | 1.8670E-01 | 1.7408E-01 | 3.1450E-01 | 2.0032E-01 | |
f13 | ave | 1.3006E+03 | 1.3011E+03 | 1.2036E+03 | 6.9965E+02 | 1.0099E+03 | 5.4023E+02 |
std | 7.3180E-02 | 9.3521E-01 | 6.1230E-02 | 1.0670E-01 | 5.8115E-02 | 5.5391E-02 | |
f14 | ave | 1.4397E+03 | 1.4759E+03 | 1.2487E+03 | 1.4576E+03 | 1.3813E+03 | 1.3914E+03 |
std | 1.0803E+01 | 1.0229E+01 | 3.0977E+00 | 2.7375E+00 | 2.7636E+00 | 2.9033E+00 | |
f15 | ave | 8.0315E+03 | 3.0563E+04 | 1.0476E+03 | 7.5402E+02 | 1.2366E+03 | 6.8471E+02 |
std | 3.4553E+03 | 1.4417E+04 | 4.7003E+02 | 1.7529E+02 | 5.4379E+02 | 1.4072E+02 | |
f16 | ave | 1.6461E+03 | 1.6460E+03 | 1.5723E+03 | 4.4884E+02 | 1.3370E+03 | 3.3899E+02 |
std | 5.3948E-01 | 6.5221E-01 | 6.7085E+00 | 6.9260E+00 | 7.7507E+00 | 6.7595E+00 | |
f17 | ave | 5.9354E+07 | 9.1421E+07 | 4.3274E+04 | 5.5973E+04 | 4.1236E+04 | 5.8317E+04 |
std | 2.0834E+07 | 4.7987E+07 | 8.9080E+03 | 8.2787E+03 | 1.0579E+04 | 1.4855E+04 | |
f18 | ave | 2.5553E+07 | 4.2986E+07 | 3.1724E+04 | 2.6405E+04 | 3.6162E+04 | 4.6724E+04 |
std | 4.0091E+07 | 2.0825E+07 | 1.7080E+04 | 1.8732E+04 | 2.0411E+04 | 5.2549E+03 | |
f19 | ave | 2.2215E+03 | 2.3154E+03 | 1.2729E+03 | 1.1020E+03 | 1.1081E+03 | 1.4420E+03 |
std | 8.0641E+01 | 4.4544E+01 | 7.0559E+01 | 5.6971E+01 | 3.8067E+01 | 4.3033E+01 | |
f20 | ave | 1.1887E+05 | 1.7736E+05 | 1.1082E+04 | 1.1422E+04 | 1.2123E+04 | 9.4987E+03 |
std | 2.4661E+04 | 4.7633E+04 | 4.6661E+03 | 5.1722E+03 | 6.0422E+03 | 6.2754E+03 | |
f21 | ave | 1.9119E+07 | 2.9989E+07 | 1.1519E+04 | 9.7435E+03 | 1.2092E+04 | 9.7915E+03 |
std | 6.3314E+06 | 1.4428E+07 | 3.0529E+03 | 4.5540E+03 | 3.2256E+03 | 4.0206E+03 | |
f22 | ave | 5.8287E+03 | 6.5909E+03 | 3.2905E+03 | 2.8780E+03 | 3.1277E+03 | 3.0934E+03 |
std | 5.7346E+02 | 7.5484E+02 | 4.3025E+02 | 4.2572E+02 | 4.9619E+02 | 4.7700E+02 | |
f23 | ave | 2.5000E+03 | 2.5000E+03 | 2.5000E+03 | 2.5000E+03 | 2.5000E+03 | 2.5000E+03 |
std | 0.0000E+00 | 0.0000E+00 | 0.0000E+00 | 0.0000E+00 | 0.0000E+00 | 0.0000E+00 | |
f24 | ave | 2.6000E+03 | 2.6000E+03 | 2.6000E+03 | 2.6000E+03 | 2.6000E+03 | 2.6000E+03 |
std | 7.2310E-05 | 0.0000E+00 | 0.0000E+00 | 0.0000E+00 | 0.0000E+00 | 0.0000E+00 | |
f25 | ave | 2.7000E+03 | 2.7000E+03 | 2.7000E+03 | 2.7000E+03 | 2.7000E+03 | 2.7000E+03 |
std | 0.0000E+00 | 0.0000E+00 | 0.0000E+00 | 0.0000E+00 | 0.0000E+00 | 0.0000E+00 | |
f26 | ave | 2.8000E+03 | 2.8000E+03 | 2.8000E+03 | 2.8000E+03 | 2.8000E+03 | 2.8000E+03 |
std | 0.0000E+00 | 0.0000E+00 | 0.0000E+00 | 0.0000E+00 | 0.0000E+00 | 0.0000E+00 | |
f27 | ave | 2.9000E+03 | 2.9000E+03 | 2.9000E+03 | 2.9000E+03 | 2.9000E+03 | 2.9000E+03 |
std | 1.3781E-12 | 0.0000E+00 | 0.0000E+00 | 0.0000E+00 | 0.0000E+00 | 0.0000E+00 | |
f28 | ave | 3.0000E+03 | 3.0000E+03 | 3.0000E+03 | 3.0000E+03 | 3.0000E+03 | 3.0000E+03 |
std | 9.1873E-13 | 0.0000E+00 | 0.0000E+00 | 0.0000E+00 | 0.0000E+00 | 0.0000E+00 | |
f29 | ave | 3.1000E+03 | 3.1000E+03 | 3.1000E+03 | 3.1000E+03 | 3.1000E+03 | 3.1000E+03 |
std | 0.0000E+00 | 0.0000E+00 | 0.0000E+00 | 0.0000E+00 | 0.0000E+00 | 0.0000E+00 | |
f30 | ave | 3.2000E+03 | 3.2000E+03 | 5.3231E+02 | 1.3189E+02 | 4.7449E+02 | 4.1959E+02 |
std | 0.0000E+00 | 0.0000E+00 | 0.0000E+00 | 0.0000E+00 | 0.0000E+00 | 0.0000E+00 |
Unimodal Functions | Simple Multimodal Functions | Hybrid Functions | Composition Functions | All Functions | |
HHO | 5.00 | 4.77 | 5.00 | 1.50 | 3.97 |
CEHHO | 6.00 | 5.46 | 6.00 | 1.50 | 4.57 |
DMSDL-HHO | 3.67 | 2.46 | 2.67 | 1.38 | 2.33 |
ADHHO | 3.33 | 2.92 | 1.67 | 1.00 | 2.20 |
CLHHEO | 1.33 | 3.08 | 2.83 | 1.25 | 2.37 |
QC-HHO | 1.67 | 2.31 | 2.83 | 1.13 | 2.03 |
Function No. | HHO | CEHHO | DMSDL-HHO | ADHHO | CLHHEO | QC-HHO |
F1 | 0.1711 (1) | 0.1898 (4) | 0.1979 (5) | 0.1994 (6) | 0.1855 (3) | 0.1851 (2) |
F2 | 1.0354 (4) | 1.0333 (3) | 1.0402 (5) | 1.0536 (6) | 1.0280 (2) | 1.0240 (1) |
F3 | 0.2127 (2) | 0.2134 (4) | 0.2130 (3) | 0.2252 (6) | 0.2161 (5) | 0.2120 (1) |
F4 | 0.3470 (1) | 0.3969 (5) | 0.3489 (3) | 0.3998 (6) | 0.3732 (4) | 0.3483 (2) |
F5 | 0.3200 (2) | 0.3387 (4) | 0.3172 (1) | 0.3529 (6) | 0.3415 (5) | 0.3380 (3) |
F6 | 0.2748 (2) | 0.2853 (4) | 0.2854 (5) | 0.2900 (6) | 0.2839 (3) | 0.2740 (1) |
F7 | 0.2775 (2) | 0.3013 (5) | 0.2782 (3) | 0.2967 (4) | 0.3072 (6) | 0.2770 (1) |
F8 | 0.8146 (1) | 0.8694 (6) | 0.8476 (5) | 0.8259 (2) | 0.8385 (4) | 0.8381 (3) |
F9 | 0.1752 (1) | 0.1950 (6) | 0.1947 (4) | 0.1909 (3) | 0.1889 (2) | 0.1948 (5) |
F10 | 0.4427 (1) | 0.4727 (5) | 0.4679 (3) | 0.4762 (6) | 0.4427 (1) | 0.4720 (4) |
ave | 0.4071 | 0.4296 | 0.4191 | 0.4311 | 0.4206 | 0.4163 |
rank | 1 | 5 | 3 | 6 | 4 | 2 |
HHO | CEHHO | DMSDL-HHO | ADHHO | CLHHEO | QC-HHO | |
f1 | 2.5712 (1) | 3.8191 (6) | 3.1869 (4) | 3.7112 (5) | 2.9971 (3) | 2.9932 (2) |
f2 | 2.2815 (1) | 3.3411 (4) | 3.0629 (3) | 3.6919 (6) | 3.5918 (5) | 3.0009 (2) |
f3 | 2.3558 (1) | 3.3897 (3) | 4.0936 (4) | 4.2559 (5) | 4.2625 (6) | 3.0685 (2) |
f4 | 2.2709 (1) | 3.4221 (6) | 3.1982 (3) | 3.2618 (4) | 3.3069 (5) | 3.0166 (2) |
f5 | 2.6414 (1) | 3.8369 (4) | 3.4979 (3) | 3.9471 (5) | 3.9995 (6) | 3.4643 (2) |
f6 | 15.0265 (1) | 16.5892 (6) | 15.3747 (3) | 15.4918 (4) | 15.7313 (5) | 15.1352 (2) |
f7 | 2.4981 (1) | 3.6428 (3) | 3.8173 (5) | 3.8433 (6) | 3.7917 (4) | 3.5909 (2) |
f8 | 2.3186 (2) | 3.4396 (5) | 2.1830 (1) | 2.3421 (3) | 3.5208 (6) | 3.3858 (4) |
f9 | 2.5208 (1) | 3.6129 (4) | 3.8699 (5) | 3.8792 (6) | 3.5934 (2) | 3.5991 (3) |
f10 | 2.5110 (1) | 3.5748 (5) | 3.5401 (4) | 3.8220 (6) | 3.4978 (2) | 3.5326 (3) |
f11 | 2.7039 (1) | 3.8528 (3) | 4.1015 (6) | 3.9709 (5) | 3.9666 (4) | 3.8243 (2) |
f12 | 5.9112 (3) | 8.4490 (4) | 5.6371 (1) | 9.0890 (6) | 5.7282 (2) | 8.5186 (5) |
f13 | 2.3970 (1) | 3.4216 (4) | 4.7038 (5) | 5.1111 (6) | 3.1425 (2) | 3.3602 (3) |
f14 | 2.3843 (1) | 3.4155 (5) | 2.4024 (3) | 2.4702 (4) | 3.4258 (6) | 2.3998 (2) |
f15 | 2.5474 (1) | 3.7753 (3) | 4.1422 (5) | 3.9425 (4) | 3.7215 (2) | 4.6415 (6) |
f16 | 2.6729 (3) | 4.2012 (5) | 2.4023 (2) | 4.4965 (6) | 2.3619 (1) | 2.9426 (4) |
f17 | 2.6649 (1) | 3.9537 (3) | 4.2704 (5) | 4.3114 (6) | 3.9855 (4) | 3.8294 (2) |
f18 | 2.4950 (1) | 3.5390 (2) | 4.4723 (5) | 4.5069 (6) | 4.3060 (4) | 3.8235 (3) |
f19 | 4.7688 (1) | 7.0241 (4) | 7.1387 (5) | 7.5082 (6) | 6.9847 (2) | 7.0149 (3) |
f20 | 2.5339 (1) | 3.6043 (2) | 4.3699 (5) | 4.4119 (6) | 4.2767 (4) | 3.7216 (3) |
f21 | 2.6110 (1) | 3.6399 (4) | 3.9836 (6) | 3.7167 (5) | 3.4723 (3) | 3.3203 (2) |
f22 | 2.9906 (1) | 4.2424 (4) | 4.2634 (5) | 4.2992 (6) | 4.2040 (3) | 4.1862 (2) |
f23 | 3.6790 (1) | 5.3735 (4) | 4.3400 (2) | 5.4874 (5) | 5.6162 (6) | 5.2882 (3) |
f24 | 3.1085 (1) | 4.3348 (4) | 5.1338 (5) | 5.1898 (6) | 4.2848 (3) | 4.2692 (2) |
f25 | 3.5959 (1) | 5.0386 (2) | 6.7478 (6) | 5.1213 (3) | 5.2570 (5) | 5.2076 (4) |
f26 | 16.3414 (1) | 21.0678 (4) | 17.5273 (2) | 21.8697 (5) | 22.3253 (6) | 20.3958 (3) |
f27 | 16.4166 (1) | 21.0634 (4) | 19.1805 (2) | 19.5642 (3) | 21.5892 (5) | 22.3870 (6) |
f28 | 4.2274 (1) | 5.9362 (2) | 8.2345 (6) | 6.0785 (3) | 6.4602 (4) | 8.0234 (5) |
f29 | 5.6623 (1) | 7.8752 (5) | 7.5715 (4) | 7.9956 (6) | 7.4263 (3) | 7.3215 (2) |
f30 | 3.6760 (1) | 5.3156 (6) | 4.7602 (3) | 4.8526 (4) | 4.6273 (2) | 5.1187 (5) |
Ave | 4.3461 | 5.9264 | 5.7069 | 6.0747 | 5.8485 | 5.8127 |
rank | 1 | 5 | 2 | 6 | 4 | 3 |
HHO | BFO | WOA | PSO | DE | CEHHO | DMSDL- HHO | ADHHO | CLHHEO | |
F1 | 9.40E-12 | 9.40E-12 | 9.40E-12 | 9.40E-12 | 9.40E-12 | 9.40E-12 | 9.40E-12 | 9.40E-12 | 9.40E-12 |
F2 | 2.26E-11 | 2.26E-11 | 2.26E-11 | 2.26E-11 | 2.26E-11 | 2.26E-11 | 2.26E-11 | 2.26E-11 | 2.26E-11 |
F3 | 3.02E-11 | 3.02E-11 | 3.02E-11 | 3.02E-11 | 3.02E-11 | 3.02E-11 | 3.02E-11 | 3.02E-11 | 3.02E-11 |
F4 | 4.91E-11 | 3.03E-11 | 1.17E-11 | 3.02E-11 | 3.02E-11 | 4.71E-11 | 4.79E-11 | 4.66E-11 | 4.97E-11 |
F5 | 1.72E-12 | 1.72E-12 | 1.72E-12 | 1.72E-12 | 1.72E-12 | 1.72E-12 | 1.72E-12 | 1.72E-12 | 1.72E-12 |
F6 | 1.21E-12 | 1.21E-12 | 1.21E-12 | 1.21E-12 | 1.21E-12 | 1.21E-12 | 1.21E-12 | 1.21E-12 | 1.21E-12 |
F7 | 1.21E-12 | 1.21E-12 | 3.63E-09 | 1.21E-12 | 1.21E-12 | 1.21E-12 | 1.21E-12 | 1.21E-12 | 1.21E-12 |
F8 | 1.21E-12 | 1.21E-12 | 1.21E-12 | 1.21E-12 | 1.21E-12 | 1.21E-12 | 1.21E-12 | 1.21E-12 | 1.21E-12 |
F9 | 3.02E-11 | 1.07E-07 | 3.69E-11 | 1.46E-10 | NaN | 2.99E-11 | 2.98E-11 | 2.92E-11 | 2.80E-11 |
F10 | 3.69E-11 | 3.02E-11 | 9.76E-10 | 4.62E-10 | 9.92E-10 | 3.76E-11 | 3.77E-11 | 3.81E-11 | 3.53E-11 |
HHO | CEHHO | DMSDL-HHO | ADHHO | CLHHEO | |
f1 | 1.7705E-08 | 5.2425E-09 | 4.6262E-05 | 1.1551E-03 | 4.6508E-05 |
f2 | 3.5327E-09 | 2.7663E-09 | 4.7363E-05 | 4.7113E-05 | 4.3478E-05 |
f3 | 8.7391E-07 | 6.3286E-06 | 4.7284E-05 | 1.1551E-03 | 4.7527E-05 |
f4 | 1.0382E-06 | 1.2266E-05 | 4.8506E-05 | 4.7113E-05 | 4.8058E-05 |
f5 | 2.6731E-01 | 9.0608E-01 | 2.5354E-01 | 2.5354E-01 | 2.5354E-01 |
f6 | 2.9196E-05 | 4.7397E-05 | 4.5470E-04 | 4.9405E-04 | 4.5264E-04 |
f7 | 2.0927E-07 | 9.0425E-06 | 4.7493E-05 | 6.0475E-05 | 5.0695E-05 |
f8 | 4.8206E-04 | 3.0793E-03 | 3.8648E-05 | 9.1699E-03 | 3.8831E-05 |
f9 | 1.6326E-04 | 2.2196E-04 | 5.7790E-04 | 4.6421E-04 | 5.7327E-04 |
f10 | 2.3410E-05 | 1.4001E-03 | 9.3708E-04 | 6.5117E-03 | 9.6393E-04 |
f11 | 5.1528E-04 | 8.9460E-03 | 5.3768E-05 | 1.1551E-03 | 5.3884E-05 |
f12 | 2.8497E-04 | 5.5144E-04 | 4.7290E-05 | 4.7113E-05 | 4.7754E-05 |
f13 | 7.1943E-03 | 1.8116E-03 | 9.5190E-03 | 4.2925E-01 | 9.5909E-03 |
f14 | 2.1220E-03 | 5.1121E-03 | 5.4286E-05 | 1.4169E-03 | 5.5851E-05 |
f15 | 2.6195E-03 | 2.1976E-04 | 4.6889E-05 | 4.7113E-05 | 4.7320E-05 |
f16 | 1.1162E-04 | 2.4663E-03 | 5.6092E-04 | 4.9265E-04 | 5.5844E-04 |
f17 | 2.4221E-05 | 9.3334E-05 | 4.7033E-05 | 1.1551E-03 | 3.8066E-05 |
f18 | 4.1052E-04 | 4.0984E-02 | 5.2596E-05 | 1.5099E-02 | 5.2665E-05 |
f19 | 1.9152E-03 | 4.8671E-03 | 4.9178E-05 | 4.2925E-04 | 5.2957E-05 |
f20 | 8.5933E-05 | 4.4240E-04 | 4.6667E-05 | 4.7113E-05 | 4.6744E-05 |
f21 | 9.6968E-05 | 1.5948E-03 | 4.7318E-05 | 4.7113E-05 | 5.3494E-05 |
f22 | 1.1347E-04 | 8.9985E-03 | 4.6991E-05 | 4.7113E-05 | 4.6674E-05 |
f23 | 6.0998E-03 | 3.6646E-04 | 1.7514E-02 | 1.2542E-04 | 1.8586E-02 |
f24 | 4.7882E-04 | 2.1092E-05 | 3.0202E-03 | 4.7113E-05 | 3.2206E-03 |
f25 | 3.5328E-03 | 7.4526E-03 | 2.4097E-05 | 9.8325E-05 | 2.4222E-05 |
f26 | 8.3156E-04 | 5.4782E-03 | 2.5678E-02 | 4.7113E-05 | 2.5918E-02 |
f27 | 1.1901E-03 | 1.1267E-02 | 2.2684E-05 | 1.5890E-04 | 2.2524E-05 |
f28 | 1.0185E-03 | 7.8966E-03 | 2.6043E-03 | 4.7113E-05 | 2.2437E-03 |
f29 | 7.1010E-05 | 5.6701E-03 | 1.1766E-02 | 4.7113E-05 | 1.0596E-02 |
f30 | 1.4942E-02 | 4.1432E-02 | 4.7113E-05 | 4.7113E-05 | 5.1618E-05 |
(x, y) | q | Optimal Cost (s) | Position deviation (%) | Release rate deviation (%) | |
QC-HHO | (1.98, 2.97) | 30.21 | 193.49 | 0.036 | 0.7 |
CLHHEO | (2.05, 3.02) | 31.04 | 194.05 | 0.042 | 3.47 |
ADHHO | (1.97, 3.04) | 29.56 | 202.01 | 0.041 | 1.47 |
DMSDL-HHO | (2.02, 2.96) | 30.28 | 200.21 | 0.05 | 0.93 |
CEHHO | (1.93, 2.92) | 28.97 | 196.77 | 0.092 | 3.43 |
HHO | (1.93, 2.92) | 28.39 | 190.21 | 0.117 | 5.37 |