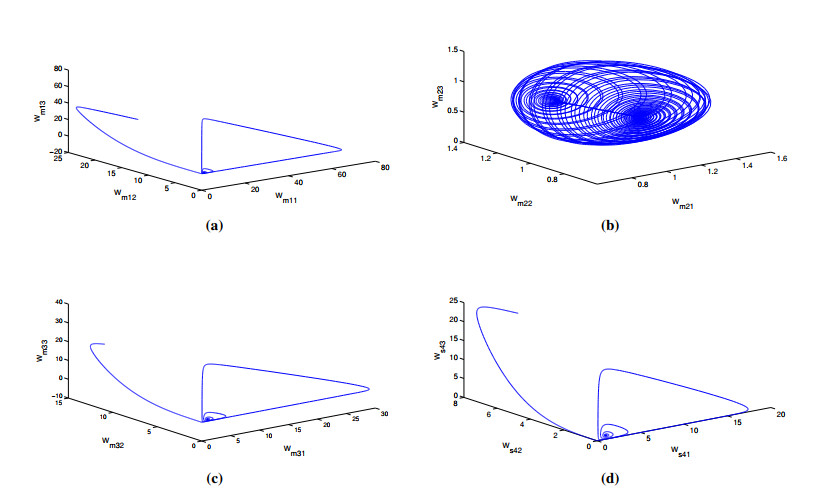
Coronary microvascular dysfunction (CMD) is one of the basic mechanisms of myocardial ischemia. Myocardial contrast echocardiography (MCE) is a bedside technique that utilises microbubbles which remain entirely within the intravascular space and denotes the status of microvascular perfusion within that region. Some pilot studies suggested that MCE may be used to diagnose CMD, but without further validation. This study is aimed to investigate the diagnostic performance of MCE for the evaluation of CMD. MCE was performed at rest and during adenosine triphosphate stress. ECG triggered real-time frames were acquired in the apical 4-chamber, 3-chamber, 2-chamber, and long-axis imaging planes. These images were imported into Narnar for further processing. Eighty-two participants with suspicion of coronary disease and absence of significant epicardial lesions were prospectively investigated. Thermodilution was used as the gold standard to diagnose CMD. CMD was present in 23 (28%) patients. Myocardial blood flow reserve (MBF) was assessed using MCE. CMD was defined as MBF reserve < 2. The MCE method had a high sensitivity (88.1%) and specificity (95.7%) in the diagnosis of CMD. There was strong agreement with thermodilution (Kappa coefficient was 0.727; 95% CI: 0.57–0.88, p < 0.001). However, the correlation coefficient (r = 0.376; p < 0.001) was not high.
Citation: Jucheng Zhang, Minwen Ma, Huajun Li, Zhaoxia Pu, Haipeng Liu, Tianhai Huang, Huan Cheng, Yinglan Gong, Yonghua Chu, Zhikang Wang, Jun Jiang, Ling Xia. Early diagnosis of coronary microvascular dysfunction by myocardial contrast stress echocardiography[J]. Mathematical Biosciences and Engineering, 2023, 20(5): 7845-7858. doi: 10.3934/mbe.2023339
[1] | Honglei Wang, Wenliang Zeng, Xiaoling Huang, Zhaoyang Liu, Yanjing Sun, Lin Zhang . MTTLm6A: A multi-task transfer learning approach for base-resolution mRNA m6A site prediction based on an improved transformer. Mathematical Biosciences and Engineering, 2024, 21(1): 272-299. doi: 10.3934/mbe.2024013 |
[2] | Shanzheng Wang, Xinhui Xie, Chao Li, Jun Jia, Changhong Chen . Integrative network analysis of N6 methylation-related genes reveal potential therapeutic targets for spinal cord injury. Mathematical Biosciences and Engineering, 2021, 18(6): 8174-8187. doi: 10.3934/mbe.2021405 |
[3] | Pingping Sun, Yongbing Chen, Bo Liu, Yanxin Gao, Ye Han, Fei He, Jinchao Ji . DeepMRMP: A new predictor for multiple types of RNA modification sites using deep learning. Mathematical Biosciences and Engineering, 2019, 16(6): 6231-6241. doi: 10.3934/mbe.2019310 |
[4] | Yong Zhu, Zhipeng Jiang, Xiaohui Mo, Bo Zhang, Abdullah Al-Dhelaan, Fahad Al-Dhelaan . A study on the design methodology of TAC3 for edge computing. Mathematical Biosciences and Engineering, 2020, 17(5): 4406-4421. doi: 10.3934/mbe.2020243 |
[5] | Atefeh Afsar, Filipe Martins, Bruno M. P. M. Oliveira, Alberto A. Pinto . A fit of CD4+ T cell immune response to an infection by lymphocytic choriomeningitis virus. Mathematical Biosciences and Engineering, 2019, 16(6): 7009-7021. doi: 10.3934/mbe.2019352 |
[6] | Tamás Tekeli, Attila Dénes, Gergely Röst . Adaptive group testing in a compartmental model of COVID-19*. Mathematical Biosciences and Engineering, 2022, 19(11): 11018-11033. doi: 10.3934/mbe.2022513 |
[7] | Wenli Cheng, Jiajia Jiao . An adversarially consensus model of augmented unlabeled data for cardiac image segmentation (CAU+). Mathematical Biosciences and Engineering, 2023, 20(8): 13521-13541. doi: 10.3934/mbe.2023603 |
[8] | Tongmeng Jiang, Pan Jin, Guoxiu Huang, Shi-Cheng Li . The function of guanylate binding protein 3 (GBP3) in human cancers by pan-cancer bioinformatics. Mathematical Biosciences and Engineering, 2023, 20(5): 9511-9529. doi: 10.3934/mbe.2023418 |
[9] | Xin Yu, Jun Liu, Ruiwen Xie, Mengling Chang, Bichun Xu, Yangqing Zhu, Yuancai Xie, Shengli Yang . Construction of a prognostic model for lung squamous cell carcinoma based on seven N6-methylandenosine-related autophagy genes. Mathematical Biosciences and Engineering, 2021, 18(5): 6709-6723. doi: 10.3934/mbe.2021333 |
[10] | Tahir Rasheed, Faran Nabeel, Muhammad Bilal, Yuping Zhao, Muhammad Adeel, Hafiz. M. N. Iqbal . Aqueous monitoring of toxic mercury through a rhodamine-based fluorescent sensor. Mathematical Biosciences and Engineering, 2019, 16(4): 1861-1873. doi: 10.3934/mbe.2019090 |
Coronary microvascular dysfunction (CMD) is one of the basic mechanisms of myocardial ischemia. Myocardial contrast echocardiography (MCE) is a bedside technique that utilises microbubbles which remain entirely within the intravascular space and denotes the status of microvascular perfusion within that region. Some pilot studies suggested that MCE may be used to diagnose CMD, but without further validation. This study is aimed to investigate the diagnostic performance of MCE for the evaluation of CMD. MCE was performed at rest and during adenosine triphosphate stress. ECG triggered real-time frames were acquired in the apical 4-chamber, 3-chamber, 2-chamber, and long-axis imaging planes. These images were imported into Narnar for further processing. Eighty-two participants with suspicion of coronary disease and absence of significant epicardial lesions were prospectively investigated. Thermodilution was used as the gold standard to diagnose CMD. CMD was present in 23 (28%) patients. Myocardial blood flow reserve (MBF) was assessed using MCE. CMD was defined as MBF reserve < 2. The MCE method had a high sensitivity (88.1%) and specificity (95.7%) in the diagnosis of CMD. There was strong agreement with thermodilution (Kappa coefficient was 0.727; 95% CI: 0.57–0.88, p < 0.001). However, the correlation coefficient (r = 0.376; p < 0.001) was not high.
The constituent members in a system mainly found in nature can be interacting with each other through cooperation and competition. Demonstrations for such systems involve biological species, countries, businesses, and many more. It's very much intriguing to investigate in a comprehensive manner numerous social as well as biological interactions existent in dissimilar species/entities utilizing mathematical modeling. The predation and the competition species are the most famous interactions among all such types of interactions. Importantly, Lotka [1] and Volterra [2] in the 1920s have announced individually the classic equations portraying population dynamics. Such illustrious equations are notably described as predator-prey (PP) equations or Lotka-Volterra (LV) equations. In this structure, PP/LV model represents the most influential model for interacting populations. The interplay between prey and predator together with additional factors has been a prominent topic in mathematical ecology for a long period. Arneodo et al. [3] have established in 1980 that a generalized Lotka-Volterra biological system (GLVBS) would depict chaos phenomena in an ecosystem for some explicitly selected system parameters and initial conditions. Additionally, Samardzija and Greller [4] demonstrated in 1988 that GLVBS would procure chaotic reign from the stabled state via rising fractal torus. LV model was initially developed as a biological concept, yet it is utilized in enormous diversified branches for research [5,6,7,8]. Synchronization essentially is a methodology of having different chaotic systems (non-identical or identical) following exactly a similar trajectory, i.e., the dynamical attributes of the slave system are locked finally into the master system. Specifically, synchronization and control have a wide spectrum for applications in engineering and science, namely, secure communication [9], encryption [10,11], ecological model [12], robotics [13], neural network [14], etc. Recently, numerous types of secure communication approaches have been explored [15,16,17,18] such as chaos modulation [18,19,20,21], chaos shift keying [22,23] and chaos masking [9,17,20,24]. In chaos communication schemes, the typical key idea for transmitting a message through chaotic/hyperchaotic models is that a message signal is nested in the transmitter system/model which originates a chaotic/ disturbed signal. Afterwards, this disturbed signal has been emitted to the receiver through a universal channel. The message signal would finally be recovered by the receiver. A chaotic model has been intrinsically employed both as receiver and transmitter. Consequently, this area of chaotic synchronization & control has sought remarkable considerations among differential research fields.
Most prominently, synchronization theory has been in existence for over 30 years due to the phenomenal research of Pecora and Carroll [25] established in 1990 using drive-response/master-slave/leader-follower configuration. Consequently, many authors and researchers have started introducing and studying numerous control and synchronization methods [9,26,27,28,29,30,31,32,33,34,35,36] etc. to achieve stabilized chaotic systems for possessing stability. In [37], researchers discussed optimal synchronization issues in similar GLVBSs via optimal control methodology. In [38,39], the researchers studied the adaptive control method (ACM) to synchronize chaotic GLVBSs. Also, researchers [40] introduced a combination difference anti-synchronization scheme in similar chaotic GLVBSs via ACM. In addition, authors [41] investigated a combination synchronization scheme to control chaos existing in GLVBSs using active control strategy (ACS). Bai and Lonngren [42] first proposed ACS in 1997 for synchronizing and controlling chaos found in nonlinear dynamical systems. Furthermore, compound synchronization using ACS was first advocated by Sun et al. [43] in 2013. In [44], authors discussed compound difference anti-synchronization scheme in four chaotic systems out of which two chaotic systems are considered as GLVBSs using ACS and ACM along with applications in secure communications of chaos masking type in 2019. Some further research works [45,46] based on ACS have been reported in this direction. The considered chaotic GLVBS offers a generalization that allows higher-order biological terms. As a result, it may be of interest in cases where biological systems experience cataclysmic changes. Unfortunately, some species will be under competitive pressure in the coming years and decades. This work may be comprised as a step toward preserving as many currently living species as possible by using the proposed synchronization approach which is based on master-slave configuration and Lyapunov stability analysis.
In consideration of the aforementioned discussions and observations, our primary focus here is to develop a systematic approach for investigating compound difference anti-synchronization (CDAS) approach in 4 similar chaotic GLVBSs via ACS. The considered ACS is a very efficient yet theoretically rigorous approach for controlling chaos found in GLVBSs. Additionally, in view of widely known Lyapunov stability analysis (LSA) [47], we discuss actively designed biological control law & convergence for synchronization errors to attain CDAS synchronized states.
The major attributes for our proposed research in the present manuscript are:
● The proposed CDAS methodology considers four chaotic GLVBSs.
● It outlines a robust CDAS approach based active controller to achieve compound difference anti-synchronization in discussed GLVBSs & conducts oscillation in synchronization errors along with extremely fast convergence.
● The construction of the active control inputs has been executed in a much simplified fashion utilizing LSA & master-salve/ drive-response configuration.
● The proposed CDAS approach in four identical chaotic GLVBSs of integer order utilizing ACS has not yet been analyzed up to now. This depicts the novelty of our proposed research work.
This manuscript is outlined as follows: Section 2 presents the problem formulation of the CDAS scheme. Section 3 designs comprehensively the CDAS scheme using ACS. Section 4 consists of a few structural characteristics of considered GLVBS on which CDAS is investigated. Furthermore, the proper active controllers having nonlinear terms are designed to achieve the proposed CDAS strategy. Moreover, in view of Lyapunov's stability analysis (LSA), we have examined comprehensively the biological controlling laws for achieving global asymptotical stability of the error dynamics for the discussed model. In Section 5, numerical simulations through MATLAB are performed for the illustration of the efficacy and superiority of the given scheme. Lastly, we also have presented some conclusions and the future prospects of the discussed research work in Section 6.
We here formulate a methodology to examine compound difference anti-synchronization (CDAS) scheme viewing master-slave framework in four chaotic systems which would be utilized in the coming up sections.
Let the scaling master system be
˙wm1= f1(wm1), | (2.1) |
and the base second master systems be
˙wm2= f2(wm2), | (2.2) |
˙wm3= f3(wm3). | (2.3) |
Corresponding to the aforementioned master systems, let the slave system be
˙ws4= f4(ws4)+U(wm1,wm2,wm3,ws4), | (2.4) |
where wm1=(wm11,wm12,...,wm1n)T∈Rn, wm2=(wm21,wm22,...,wm2n)T∈Rn, wm3=(wm31,wm32,...,wm3n)T∈Rn, ws4=(ws41,ws42,...,ws4n)T∈Rn are the state variables of the respective chaotic systems (2.1)–(2.4), f1,f2,f3,f4:Rn→Rn are four continuous vector functions, U=(U1,U2,...,Un)T:Rn×Rn×Rn×Rn→Rn are appropriately constructed active controllers.
Compound difference anti-synchronization error (CDAS) is defined as
E=Sws4+Pwm1(Rwm3−Qwm2), |
where P=diag(p1,p2,.....,pn),Q=diag(q1,q2,.....,qn),R=diag(r1,r2,.....,rn),S=diag(s1,s2,.....,sn) and S≠0.
Definition: The master chaotic systems (2.1)–(2.3) are said to achieve CDAS with slave chaotic system (2.4) if
limt→∞‖E(t)‖=limt→∞‖Sws4(t)+Pwm1(t)(Rwm3(t)−Qwm2(t))‖=0. |
We now present our proposed CDAS approach in three master systems (2.1)–(2.3) and one slave system (2.4). We next construct the controllers based on CDAS approach by
Ui= ηisi−(f4)i−KiEisi, | (3.1) |
where ηi=pi(f1)i(riwm3i−qiwm2i)+piwm1i(ri(f3)i−qi(f2)i), for i=1,2,...,n.
Theorem: The systems (2.1)–(2.4) will attain the investigated CDAS approach globally and asymptotically if the active control functions are constructed in accordance with (3.1).
Proof. Considering the error as
Ei= siws4i+piwm1i(riwm3i−qiwm2i),fori=1,2,3,.....,n. |
Error dynamical system takes the form
˙Ei= si˙ws4i+pi˙wm1i(riwm3i−qiwm2i)+piwm1i(ri˙wm3i−qi˙wm2i)= si((f4)i+Ui)+pi(f1)i(riwm3i−qiwm2i)+piwm1i(ri(f3)i−qi(f2)i)= si((f4)i+Ui)+ηi, |
where ηi=pi(f1)i(riwm3i−qiwm2i)+piwm1i(ri(f3)i−qi(f2)i), i=1,2,3,....,n. This implies that
˙Ei= si((f4)i−ηisi−(f4)i−KiEisi)+ηi= −KiEi | (3.2) |
The classic Lyapunov function V(E(t)) is described by
V(E(t))= 12ETE= 12ΣE2i |
Differentiation of V(E(t)) gives
˙V(E(t))=ΣEi˙Ei |
Using Eq (3.2), one finds that
˙V(E(t))=ΣEi(−KiEi)= −ΣKiE2i). | (3.3) |
An appropriate selection of (K1,K1,.......,Kn) makes ˙V(E(t)) of eq (3.3), a negative definite. Consequently, by LSA [47], we obtain
limt→∞Ei(t)=0,(i=1,2,3). |
Hence, the master systems (2.1)–(2.3) and slave system (2.4) have attained desired CDAS strategy.
We now describe GLVBS as the scaling master system:
{˙wm11=wm11−wm11wm12+b3w2m11−b1w2m11wm13,˙wm12=−wm12+wm11wm12,˙wm13=b2wm13+b1w2m11wm13, | (4.1) |
where (wm11,wm12,wm13)T∈R3 is state vector of (4.1). Also, wm11 represents the prey population and wm12, wm13 denote the predator populations. For parameters b1=2.9851, b2=3, b3=2 and initial conditions (27.5,23.1,11.4), scaling master GLVBS displays chaotic/disturbed behaviour as depicted in Figure 1(a).
The base master systems are the identical chaotic GLVBSs prescribed respectively as:
{˙wm21=wm21−wm21wm22+b3w2m21−b1w2m21wm23,˙wm22=−wm22+wm21wm22,˙wm23=b2wm23+b1w2m21wm23, | (4.2) |
where (wm21,wm22,wm23)T∈R3 is state vector of (4.2). For parameter values b1=2.9851, b2=3, b3=2, this base master GLVBS shows chaotic/disturbed behaviour for initial conditions (1.2,1.2,1.2) as displayed in Figure 1(b).
{˙wm31=wm31−wm31wm32+b3w2m31−b1w2m31wm33,˙wm32=−wm32+wm31wm32,˙wm33=b2wm33+b1w2m31wm33, | (4.3) |
where (wm31,wm32,wm33)T∈R3 is state vector of (4.3). For parameters b1=2.9851, b2=3, b3=2, this second base master GLVBS displays chaotic/disturbed behaviour for initial conditions (2.9,12.8,20.3) as shown in Figure 1(c).
The slave system, represented by similar GLVBS, is presented by
{˙ws41=ws41−ws41ws42+b3w2s41−b1w2s41ws43+U1,˙ws42=−ws42+ws41ws42+U2,˙ws43=b2ws43+b1w2s41ws43+U3, | (4.4) |
where (ws41,ws42,ws43)T∈R3 is state vector of (4.4). For parameter values, b1=2.9851, b2=3, b3=2 and initial conditions (5.1,7.4,20.8), the slave GLVBS exhibits chaotic/disturbed behaviour as mentioned in Figure 1(d).
Moreover, the detailed theoretical study for (4.1)–(4.4) can be found in [4]. Further, U1, U2 and U3 are controllers to be determined.
Next, the CDAS technique has been discussed for synchronizing the states of chaotic GLVBS. Also, LSA-based ACS is explored & the necessary stability criterion is established.
Here, we assume P=diag(p1,p2,p3), Q=diag(q1,q2,q3), R=diag(r1,r2,r3), S=diag(s1,s2,s3). The scaling factors pi,qi,ri,si for i=1,2,3 are selected as required and can assume the same or different values.
The error functions (E1,E2,E3) are defined as:
{E1=s1ws41+p1wm11(r1wm31−q1wm21),E2=s2ws42+p2wm12(r2wm32−q2wm22),E3=s3ws43+p3wm13(r3wm33−q3wm23). | (4.5) |
The major objective of the given work is the designing of active control functions Ui,(i=1,2,3) ensuring that the error functions represented in (4.5) must satisfy
limt→∞Ei(t)=0for(i=1,2,3). |
Therefore, subsequent error dynamics become
{˙E1=s1˙ws41+p1˙wm11(r1wm31−q1wm21)+p1wm11(r1˙wm31−q1˙wm21),˙E2=s2˙ws42+p2˙wm12(r2wm32−q2wm22)+p2wm12(r2˙wm32−q2˙wm22),˙E3=s3˙ws43+p3˙wm13(r3wm33−q3wm23)+p3wm13(r3˙wm33−q3˙wm23). | (4.6) |
Using (4.1), (4.2), (4.3), and (4.5) in (4.6), the error dynamics simplifies to
{˙E1=s1(ws41−ws41ws42+b3w2s41−b1w2s41ws43+U1)+p1(wm11−wm11wm12+b3w2m11−b1w2m11wm13)(r1wm31−q1wm21)+p1wm11(r1(wm31−wm31wm32+b3w2m31−b1w2m31wm33)−q1(wm21−wm21wm22+b3w2m21−b1w2m21wm23),˙E2=s2(−ws42+ws41ws42+U2)+p2(−wm12+wm11wm12)(r2wm32−q2wm22)+p2wm12(r2(−wm32+wm31wm32)−q2(−wm22+wm21wm22)),˙E3=s3(b2ws43+b1w2s41ws43+U3)+p3(b2wm13+b1w2m11wm13)(r3wm33−q3wm23)+p3wm13(r3(b2wm33+b1w2m31wm33)−q3(b2wm23+b1w2m21wm23)). | (4.7) |
Let us now choose the active controllers:
U1= η1s1−(f4)1−K1E1s1, | (4.8) |
where η1=p1(f1)1(r1wm31−q1wm21)+p1wm11(r1(f3)1−q1(f2)1), as described in (3.1).
U2= η2s2−(f4)2−K2E2s2, | (4.9) |
where η2=p2(f1)2(r2wm32−q2wm22)+p2wm12(r2(f3)2−q2(f2)2).
U3= η3s3−(f4)3−K3E3s3, | (4.10) |
where η3=p3(f1)3(r3wm33−q3wm23)+p3wm13(r3(f3)3−q3(f2)3) and K1>0,K2>0,K3>0 are gaining constants.
By substituting the controllers (4.8), (4.9) and (4.10) in (4.7), we obtain
{˙E1=−K1E1,˙E2=−K2E2,˙E3=−K3E3. | (4.11) |
Lyapunov function V(E(t)) is now described by
V(E(t))= 12[E21+E22+E23]. | (4.12) |
Obviously, the Lyapunov function V(E(t)) is +ve definite in R3. Therefore, the derivative of V(E(t)) as given in (4.12) can be formulated as:
˙V(E(t))= E1˙E1+E2˙E2+E3˙E3. | (4.13) |
Using (4.11) in (4.13), one finds that
˙V(E(t))= −K1E21−K2E22−K3E23<0, |
which displays that ˙V(E(t)) is -ve definite.
In view of LSA [47], we, therefore, understand that CDAS error dynamics is globally as well as asymptotically stable, i.e., CDAS error E(t)→0 asymptotically for t→∞ to each initial value E(0)∈R3.
This section conducts a few simulation results for illustrating the efficacy of the investigated CDAS scheme in identical chaotic GLVBSs using ACS. We use 4th order Runge-Kutta algorithm for solving the considered ordinary differential equations. Initial conditions for three master systems (4.1)–(4.3) and slave system (4.4) are (27.5,23.1,11.4), (1.2,1.2,1.2), (2.9,12.8,20.3) and (14.5,3.4,10.1) respectively. We attain the CDAS technique among three masters (4.1)–(4.3) and corresponding one slave system (4.4) by taking pi=qi=ri=si=1, which implies that the slave system would be entirely anti-synchronized with the compound of three master models for i=1,2,3. In addition, the control gains (K1,K2,K3) are taken as 2. Also, Figure 2(a)–(c) indicates the CDAS synchronized trajectories of three master (4.1)–(4.3) & one slave system (4.4) respectively. Moreover, synchronization error functions (E1,E2,E3)=(51.85,275.36,238.54) approach 0 as t tends to infinity which is exhibited via Figure 2(d). Hence, the proposed CDAS strategy in three masters and one slave models/systems has been demonstrated computationally.
In this work, the investigated CDAS approach in similar four chaotic GLVBSs using ACS has been analyzed. Lyapunov's stability analysis has been used to construct proper active nonlinear controllers. The considered error system, on the evolution of time, converges to zero globally & asymptotically via our appropriately designed simple active controllers. Additionally, numerical simulations via MATLAB suggest that the newly described nonlinear control functions are immensely efficient in synchronizing the chaotic regime found in GLVBSs to fitting set points which exhibit the efficacy and supremacy of our proposed CDAS strategy. Exceptionally, both analytic theory and computational results are in complete agreement. Our proposed approach is simple yet analytically precise. The control and synchronization among the complex GLVBSs with the complex dynamical network would be an open research problem. Also, in this direction, we may extend the considered CDAS technique on chaotic systems that interfered with model uncertainties as well as external disturbances.
The authors gratefully acknowledge Qassim University, represented by the Deanship of Scientific Research, on the financial support for this research under the number 10163-qec-2020-1-3-I during the academic year 1441 AH/2020 AD.
The authors declare there is no conflict of interest.
[1] |
P. G. Camici, F. Crea, Coronary microvascular dysfunction, N. Engl. J. Med., 356 (2007), 830–840. https://doi.org/10.1056/NEJMra061889 doi: 10.1056/NEJMra061889
![]() |
[2] |
G. Montalescot, U. Sechtem, S. Achenbach, F. Andreotti, C. Arden, A. Budaj, et al., 2013 ESC guidelines on the management of stable coronary artery disease: the Task Force on the management of stable coronary artery disease of the European Society of Cardiology, Eur. Heart J., 34 (2013), 2949–3003. https://doi.org/10.1093/eurheartj/eht296 doi: 10.1093/eurheartj/eht296
![]() |
[3] |
T. J. Ford, E. Yii, N. Sidik, R. Good, P. Rocchiccioli, M. McEntegart, et al., Ischemia and no obstructive coronary artery disease: prevalence and correlates of coronary vasomotion disorders, Circ. Cardiovasc. Interventions, 12 (2019), e008126. https://doi.org/10.1161/CIRCINTERVENTIONS.119.008126 doi: 10.1161/CIRCINTERVENTIONS.119.008126
![]() |
[4] |
C. L. Schumann, R. C. Mathew, J. L. Dean, Y. Yang, P. C. Balfour, P. W. Shaw, et al., Functional and economic impact of INOCA and influence of coronary microvascular dysfunction, JACC Cardiovasc. Imaging, 14 (2021), 1369–1379. https://doi.org/10.1016/j.jcmg.2021.01.041 doi: 10.1016/j.jcmg.2021.01.041
![]() |
[5] |
J. C. Kaski, F. Crea, B. J. Gersh, P. G. Camici, Reappraisal of ischemic heart disease, Circulation, 138 (2018), 1463–1480. https://doi.org/10.1161/CIRCULATIONAHA.118.031373 doi: 10.1161/CIRCULATIONAHA.118.031373
![]() |
[6] |
H. Shimokawa, A. Suda, J. Takahashi, C. Berry, P. G. Camici, F. Crea, et al., Clinical characteristics and prognosis of patients with microvascular angina: an international and prospective cohort study by the Coronary Vasomotor Disorders International Study (COVADIS) Group, Eur. Heart J., 42, (2021), 4592–4600. https://doi.org/10.1093/eurheartj/ehab282 doi: 10.1093/eurheartj/ehab282
![]() |
[7] |
L. J. Shaw, C. N. Merz, C. J. Pepine, S. E. Reis, V. Bittner, K. E. Kip, et al., The economic burden of angina in women with suspected ischemic heart disease, Circulation, 114 (2006), 894–904. https://doi.org/10.1161/CIRCULATIONAHA.105.609990 doi: 10.1161/CIRCULATIONAHA.105.609990
![]() |
[8] |
G. A. Lanza, D. Morrone, C. Pizzi, I. Tritto, L. Bergamaschi, A. De Vita, et al., Diagnostic approach for coronary microvascular dysfunction in patients with chest pain and no obstructive coronary artery disease, Trends Cardiovasc. Med., 32 (2022), 448–453. https://doi.org/10.1016/j.tcm.2021.08.005 doi: 10.1016/j.tcm.2021.08.005
![]() |
[9] |
P. Ong, B. Safdar, A. Seitz, A. Hubert, J. F. Beltrame, E. Prescott, Diagnosis of coronary microvascular dysfunction in the clinic, Cardiovasc. Res., 116 (2020), 841–855. https://doi.org/10.1093/cvr/cvz339 doi: 10.1093/cvr/cvz339
![]() |
[10] |
T. Padro, O. Manfrini, R. Bugiardini, J. Canty, E. Cenko, G. De Luca, et al., ESC working group on coronary pathophysiology and microcirculation position paper on 'coronary microvascular dysfunction in cardiovascular disease', Cardiovasc. Res., 116 (2020), 741–755. https://doi.org/10.1093/cvr/cvaa003 doi: 10.1093/cvr/cvaa003
![]() |
[11] |
F. Vancheri, G. Longo, S. Vancheri, M. Henein, Coronary microvascular dysfunction, J. Clin. Med., 9 (2020). https://doi.org/10.3390/jcm9092880 doi: 10.3390/jcm9092880
![]() |
[12] |
L. M. Gan, J. Wikstrom, R. Fritsche-Danielson, Coronary flow reserve from mouse to man-from mechanistic understanding to future interventions, J. Cardiovasc. Transl. Res., 6 (2013), 715–728. https://doi.org/10.1007/s12265-013-9497-5 doi: 10.1007/s12265-013-9497-5
![]() |
[13] |
J. M. Lee, J. H. Jung, D. Hwang, J. Park, Y. Fan, S. H. Na, et al., Coronary flow reserve and microcirculatory resistance in patients with intermediate coronary stenosis, J. Am. Coll. Cardiol., 67 (2016), 1158–1169. https://doi.org/10.1016/j.jacc.2015.12.053 doi: 10.1016/j.jacc.2015.12.053
![]() |
[14] |
S. G. Ahn, J. Suh, O. Y. Hung, H. S. Lee, Y. H. Bouchi, W. Zeng, et al., Discordance between fractional flow reserve and coronary flow reserve: insights from intracoronary imaging and physiological assessment, JACC Cardiovasc. Interventions, 10 (2017), 999–1007. https://doi.org/10.1016/j.jcin.2017.03.006 doi: 10.1016/j.jcin.2017.03.006
![]() |
[15] |
T. J. Ford, B. Stanley, R. Good, P. Rocchiccioli, M. McEntegart, S. Watkins, et al., Stratified medical therapy using invasive coronary function testing in angina: the CorMicA trial, J. Am. Coll. Cardiol., 72 (2018), 2841–2855. https://doi.org/10.1016/j.jacc.2018.09.006 doi: 10.1016/j.jacc.2018.09.006
![]() |
[16] |
S. S. Abdelmoneim, A. Dhoble, M. Bernier, P. J. Erwin, G. Korosoglou, R. Senior, et al., Quantitative myocardial contrast echocardiography during pharmacological stress for diagnosis of coronary artery disease: a systematic review and meta-analysis of diagnostic accuracy studies. Eur. J. Echocardiography, 10 (2009), 813–825. https://doi.org/10.1093/ejechocard/jep084 doi: 10.1093/ejechocard/jep084
![]() |
[17] |
S. M. Bierig, P. Mikolajczak, S. C. Herrmann, N. Elmore, M. Kern, A. J. Labovitz, Comparison of myocardial contrast echocardiography derived myocardial perfusion reserve with invasive determination of coronary flow reserve, Eur. J. Echocardiography, 10 (2009), 250–255. https://doi.org/10.1093/ejechocard/jen217 doi: 10.1093/ejechocard/jen217
![]() |
[18] |
R. Vogel, A. Indermuhle, J. Reinhardt, P. Meier, P. T. Siegrist, M. Namdar, et al., The quantification of absolute myocardial perfusion in humans by contrast echocardiography: algorithm and validation, J. Am. Coll. Cardiol., 45 (2005), 754–762. https://doi.org/10.1016/j.jacc.2004.11.044 doi: 10.1016/j.jacc.2004.11.044
![]() |
[19] |
M. A. Al-Mohaissen, Echocardiographic assessment of primary microvascular angina and primary coronary microvascular dysfunction, Trends Cardiovasc. Med., 2022 (2022). https://doi.org/10.1016/j.tcm.2022.02.007 doi: 10.1016/j.tcm.2022.02.007
![]() |
[20] |
F. Rigo, R. Sicari, S. Gherardi, A. Djordjevic-Dikic, L. Cortigiani, E. Picano, The additive prognostic value of wall motion abnormalities and coronary flow reserve during dipyridamole stress echo, Eur. Heart J., 29 (2008), 79–88. https://doi.org/10.1093/eurheartj/ehm527 doi: 10.1093/eurheartj/ehm527
![]() |
[21] |
J. Zhan, L. Zhong, J. Wu, Assessment and treatment for coronary microvascular dysfunction by contrast enhanced ultrasound, Front. Cardiovasc. Med., 9 (2022). https://doi.org/10.3389/fcvm.2022.899099 doi: 10.3389/fcvm.2022.899099
![]() |
[22] |
J. H. Lam, J. X. Quah, T. Davies, C. J. Boos, K. Nel, C. M. Anstey, et al., Relationship between coronary microvascular dysfunction and left ventricular diastolic function in patients with chest pain and unobstructed coronary arteries, Echocardiography, 37 (2020), 1199–1204. https://doi.org/10.1111/echo.14794 doi: 10.1111/echo.14794
![]() |
[23] |
R. Senior, H. Becher, M. Monaghan, L. Agati, J. Zamorano, J. L. Vanoverschelde, et al., Clinical practice of contrast echocardiography: recommendation by the European Association of Cardiovascular Imaging (EACVI) 2017, Eur. Heart J. Cardiovasc. Imaging, 18 (2017), 1205–1205. https://doi.org/10.1093/ehjci/jex182 doi: 10.1093/ehjci/jex182
![]() |
[24] |
J. R. Lindner, Contrast echocardiography: current status and future directions, Heart, 107 (2021), 18–24. https://doi.org/10.1136/heartjnl-2020-316662 doi: 10.1136/heartjnl-2020-316662
![]() |
[25] |
S. Masi, D. Rizzoni, S. Taddei, R. J. Widmer, A. C. Montezano, T. F. Luscher, et al., Assessment and pathophysiology of microvascular disease: recent progress and clinical implications, Eur. Heart J., 42 (2021), 2590–2604. https://doi.org/10.1093/eurheartj/ehaa857 doi: 10.1093/eurheartj/ehaa857
![]() |
[26] |
D. Rinkevich, T. Belcik, N. C. Gupta, E. Cannard, N. J. Alkayed, S. Kaul, Coronary autoregulation is abnormal in syndrome X: insights using myocardial contrast echocardiography, J. Am. Soc. Echocardiography, 26 (2013), 290–296. https://doi.org/10.1016/j.echo.2012.12.008 doi: 10.1016/j.echo.2012.12.008
![]() |
[27] |
H. Everaars, G. A. de Waard, R. S. Driessen, I. Danad, P. M. van de Ven, P. G. Raijmakers, et al., Doppler flow velocity and thermodilution to assess coronary flow reserve: a head-to-head comparison with[15O]H2O PET, JACC Cardiovasc. Interventions, 11 (2018), 2044–2054. https://doi.org/10.1016/j.jcin.2018.07.011 doi: 10.1016/j.jcin.2018.07.011
![]() |
[28] |
Y. Li, C. P. Ho, M. Toulemonde, N. Chahal, R. Senior, M. X. Tang, Fully automatic myocardial segmentation of contrast echocardiography sequence using random forests guided by shape model, IEEE Trans. Med. Imaging, 37 (2018), 1081–1091. https://doi.org/10.1109/TMI.2017.2747081 doi: 10.1109/TMI.2017.2747081
![]() |
[29] |
M. Li, D. Zeng, Q. Xie, R. Xu, Y. Wang, D. Ma, et al., A deep learning approach with temporal consistency for automatic myocardial segmentation of quantitative myocardial contrast echocardiography, Int. J. Cardiovasc. Imaging, 37 (2021), 1967–1978. https://doi.org/10.1007/s10554-021-02181-8 doi: 10.1007/s10554-021-02181-8
![]() |
1. | Muhammad Zubair Mehboob, Arslan Hamid, Jeevotham Senthil Kumar, Xia Lei, Comprehensive characterization of pathogenic missense CTRP6 variants and their association with cancer, 2025, 25, 1471-2407, 10.1186/s12885-025-13685-0 |