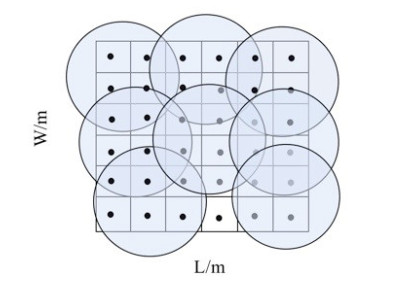
The aim of this paper is to introduce a discrete mixture model from the point of view of reliability and ordered statistics theoretically and practically for modeling extreme and outliers' observations. The base distribution can be expressed as a mixture of gamma and Lindley models. A wide range of the reported model structural properties are investigated. This includes the shape of the probability mass function, hazard rate function, reversed hazard rate function, min-max models, mean residual life, mean past life, moments, order statistics and L-moment statistics. These properties can be formulated as closed forms. It is found that the proposed model can be used effectively to evaluate over- and under-dispersed phenomena. Moreover, it can be applied to analyze asymmetric data under extreme and outliers' notes. To get the competent estimators for modeling observations, the maximum likelihood approach is utilized under conditions of the Newton-Raphson numerical technique. A simulation study is carried out to examine the bias and mean squared error of the estimators. Finally, the flexibility of the discrete mixture model is explained by discussing three COVID-19 data sets.
Citation: Mohamed S. Eliwa, Buthaynah T. Alhumaidan, Raghad N. Alqefari. A discrete mixed distribution: Statistical and reliability properties with applications to model COVID-19 data in various countries[J]. Mathematical Biosciences and Engineering, 2023, 20(5): 7859-7881. doi: 10.3934/mbe.2023340
[1] | Haitao Huang, Min Tian, Jie Zhou, Xiang Liu . Reliable task allocation for soil moisture wireless sensor networks using differential evolution adaptive elite butterfly optimization algorithm. Mathematical Biosciences and Engineering, 2023, 20(8): 14675-14698. doi: 10.3934/mbe.2023656 |
[2] | Shuming Sun, Yijun Chen, Ligang Dong . An optimization method for wireless sensor networks coverage based on genetic algorithm and reinforced whale algorithm. Mathematical Biosciences and Engineering, 2024, 21(2): 2787-2812. doi: 10.3934/mbe.2024124 |
[3] | Hao Yuan, Qiang Chen, Hongbing Li, Die Zeng, Tianwen Wu, Yuning Wang, Wei Zhang . Improved beluga whale optimization algorithm based cluster routing in wireless sensor networks. Mathematical Biosciences and Engineering, 2024, 21(3): 4587-4625. doi: 10.3934/mbe.2024202 |
[4] | Xiang Liu, Min Tian, Jie Zhou, Jinyan Liang . An efficient coverage method for SEMWSNs based on adaptive chaotic Gaussian variant snake optimization algorithm. Mathematical Biosciences and Engineering, 2023, 20(2): 3191-3215. doi: 10.3934/mbe.2023150 |
[5] | Zhonghua Lu, Min Tian, Jie Zhou, Xiang Liu . Enhancing sensor duty cycle in environmental wireless sensor networks using Quantum Evolutionary Golden Jackal Optimization Algorithm. Mathematical Biosciences and Engineering, 2023, 20(7): 12298-12319. doi: 10.3934/mbe.2023547 |
[6] | Noman Zahid, Ali Hassan Sodhro, Usman Rauf Kamboh, Ahmed Alkhayyat, Lei Wang . AI-driven adaptive reliable and sustainable approach for internet of things enabled healthcare system. Mathematical Biosciences and Engineering, 2022, 19(4): 3953-3971. doi: 10.3934/mbe.2022182 |
[7] | Guohao Sun, Sen Yang, Shouming Zhang, Yixing Liu . A hybrid butterfly algorithm in the optimal economic operation of microgrids. Mathematical Biosciences and Engineering, 2024, 21(1): 1738-1764. doi: 10.3934/mbe.2024075 |
[8] | Tingting Yang, Yi He . Design of intelligent robots for tourism management service based on green computing. Mathematical Biosciences and Engineering, 2023, 20(3): 4798-4815. doi: 10.3934/mbe.2023222 |
[9] | Zhiyang Ju, Hui Zhang, Ying Tan, Xiang Chen . Coverage control of mobile sensor networks with directional sensing. Mathematical Biosciences and Engineering, 2022, 19(3): 2913-2934. doi: 10.3934/mbe.2022134 |
[10] | Zhenao Yu, Peng Duan, Leilei Meng, Yuyan Han, Fan Ye . Multi-objective path planning for mobile robot with an improved artificial bee colony algorithm. Mathematical Biosciences and Engineering, 2023, 20(2): 2501-2529. doi: 10.3934/mbe.2023117 |
The aim of this paper is to introduce a discrete mixture model from the point of view of reliability and ordered statistics theoretically and practically for modeling extreme and outliers' observations. The base distribution can be expressed as a mixture of gamma and Lindley models. A wide range of the reported model structural properties are investigated. This includes the shape of the probability mass function, hazard rate function, reversed hazard rate function, min-max models, mean residual life, mean past life, moments, order statistics and L-moment statistics. These properties can be formulated as closed forms. It is found that the proposed model can be used effectively to evaluate over- and under-dispersed phenomena. Moreover, it can be applied to analyze asymmetric data under extreme and outliers' notes. To get the competent estimators for modeling observations, the maximum likelihood approach is utilized under conditions of the Newton-Raphson numerical technique. A simulation study is carried out to examine the bias and mean squared error of the estimators. Finally, the flexibility of the discrete mixture model is explained by discussing three COVID-19 data sets.
Wireless sensor networks (WSN) are widely used in the automation, production, transport, healthcare, and agricultural fields [1,2,3]. As one core technology in the area of the Internet, wireless sensor networks are composed of multiple sensors in different ways to complete signal collection, signal transmission, data processing, and other operations [4,5,6]. WSN coverage problem is one of the basic problems in sensor networks [7,8]. Coverage capability is a criterion for evaluating the monitoring capability of WSN to a monitoring area, that is, how to deploy nodes to reach the maximum networks coverage, so as to improve the stability and effectiveness of information transmission.
Much work has been done to improve the maximum coverage of WSN. Yang et al. [9] improve the traditional centroid location algorithm, design a weighting strategy according to the reference anchor node and raise the location accuracy through multiple weights. From the view of repairing coverage gaps, based on graph theory and geometric calculation, Lu et al. [10] propose a Voronoi polygon strategy to repair local gaps for higher coverage. Based on game theory, Hajjej et al. [11] propose a new topology control method using reinforcement learning (RL) to repair coverage holes in a distributed way, so as to repair coverage vulnerabilities more effectively.
In recent years, an increasing number of scholars have consistently tried to apply intelligent algorithms to solve the WSN coverage problem [12] and have obtained specific results [13]. Kannan et al. [14] introduce the simulated annealing (SA) into the positioning research of wireless sensor networks. The algorithm has strong global optimization ability and robustness by jumping out of local optimal solution with a certain probability. However, the simulated annealing relies excessively on the parameters setting, which leads to the plight that better solution and search time cannot have both Kulkarni et al. [15] introduce particle swarm optimization (PSO) to improve node deployment and data fusion tasks. There is still a disadvantage that it is easy to fall into local optimum. Thus, the classical evolutionary computation cannot meet the needs of the problem. ZainEldin et al. [16] propose a novel improved dynamic deployment technique based-on genetic algorithm (IDDT-GA) to solve this problem. Similarly, aiming at how to minimize the number of nodes and maximize the coverage at the same time, Rebai et al. [17] construct an integer programming model and a special genetic algorithm (GA) is proposed for this model. Shivalingegowda et al. [18] introduce a new algorithm called hybrid gravitational search algorithm with social ski-driver (GSA-SSD). It proves that the hybrid algorithm model has certain advantages in the application of wireless sensor networks. Binh et al. [19] optimize several metaheuristic algorithms for WSN coverage in restricted areas with obstacles. Raj Priyadarshini et al. [20] extend the problem to underwater environment and proposed an energy prediction algorithm based on Markov chain Monte Carlo (MCMC) process for underwater acoustic WSN to reduce coverage vulnerabilities. Khalaf et al. [21] introduce a coverage optimization method for WSN based on the bee algorithm (BA) and compare with the genetic algorithm (GA), the results show BA can spend less time and use less resources to achieve higher coverage. Wang et al. [22] propose a Virtual Force Algorithm-Lévy-Embedded Grey Wolf Optimization (VFLGWO) to solve the WSN coverage problem. The results under different nodes and different monitoring area are discussed respectively in this paper. Feng et al. [23] combine K-means algorithm with artificial fish swarm algorithm, so that a WSN clustering method is proposed, which has better performance compared with traditional methods.
Arora et al. [24] propose a node location scheme using a natural heuristic meta-heuristic algorithm called butterfly optimization algorithm (BOA), which is simulated on sensor networks of different scales and compared to the performance of PSO and firefly algorithm (FA). The results show that BOA can obtain more accurate and stable node positioning. However, I think this work is still not perfect, because the butterfly algorithm has the disadvantages of low optimization accuracy and easy to fall into local optimization. Therefore, a series of work has down to improve the butterfly optimization algorithm and make BOA better with hybrid strategies. Arora et al. [24] combine the artificial bee colony algorithm (ABC) with the butterfly optimization algorithm and add the Lévy flights strategy to the butterfly optimization algorithm, so that a BOA/ABC algorithm is proposed. When the search phase of BOA is completed, the ABC algorithm start to explore in the search space. To some extent, it speeds up the convergence speed and widens the search space. The core idea of hybrid strategy still lies in accelerating convergence speed and jumping out of local optimization. Utama et al. [25] propose a new hybrid butterfly optimization algorithm for solving green vehicle routing problem (G-VRP). This algorithm is discretized by LRV method and applied to solve discrete optimization problems. By combining the tabu search (TS) algorithm and local search swap and flip strategies, the algorithm gradually approaches the global optimum by continuously flipping and exchanging nodes. Although it can solve the G-VRP well, it still has high time complexity and needs a lot of computing time than BOA. To overcome the problems faced by classical evolutionary computation. Our contributions lie in two aspects:
1) We make a new attempt to integrate five different improvement strategies with BOA, which help to accelerate the convergence speed and improve the optimization accuracy while ensuring the calculation time. We introduce Kent chaotic map in the initialization stage, improve the local search and mutation strategy of butterflies, construct a new inertia weight factor, and propose a simple simulated annealing process based on Standard normal distribution disturbance and Metropolis criterion, which gives full play to the advantages of meta heuristic algorithm.
2) The time complexity, convergence and asymptotic of the algorithm are analyzed in this paper. Through simulation test, the results show the hybrid strategies perform well.
In this paper, we use Boolean perception model to research the problem. There is a set of N sensor nodes S={si,i=1,2,3,⋯n} in a 2D monitoring area of size L×W. Each node takes (xi,yi) as the center of a circle and takes R as the radius. Suppose the monitoring area which is to be covered is discretized into L×W pixels, if the target pixel is within the sensor node coverage radius, it is successfully covered. The position of pixel pj can be expressed as (xj,yj), so the distance can be expressed as
d(si,pj)=√(xi− xj)2+(yi− yj)2 | (2.1) |
when the pixel pj is within the radius of the nodes, pj is covered successfully. So, we can get the probability which the pixel pj is covered successfully, as shown in Eq (2.2).
p(si,pj)={1d(si,pj)≤R0d(si,pj)>R | (2.2) |
In particular, the target point only needs to be covered by any node in S={si,i=1,2,3,⋯n}, then the information of this pixel can be detected. The joint perception probability of target point pj is
p(S,pj)=1−∏ni=1[1−p(si,pj)] | (2.3) |
In Eq (2.3), if pj is out of the range of any node si in S, then ∏ni=1[1−p(si,pj)]=1. So p(S,pj)=0, that is, this pixel is out of WSN coverage range. If pj is in the range of any node si in S, then p(si,pj)=1,∏ni=1[1−p(si,pj)]=0, so P(S,pj)=1, that is, the pixel pj is perceived by S.
We use coverage rate to express the monitoring ability of sensor nodes. The formula of coverage rate is the ratio of sensor nodes coverage monitoring area Ac to the whole area A, which can be expressed by Eq (2.4).
f=AcA=∑L×Wj=1p(S,pj)L×W | (2.4) |
We take Eq (2.4) as the objective function. We give a simple example to illustrate the problem vividly, as shown in Figure 1. Assume that the nodes deployment scheme is shown as the figure, the shaded areas represent covered areas and white areas represent uncovered areas. Only one pixel is not covered so the coverage area of sensor nodes Ac = 35. The number of sensor nodes is 8 and the monitoring area A=6×6=36. So the coverage rate is
coverage=AcA=3536=0.972. | (2.5) |
The butterfly optimization algorithm (BOA) [26] based on the butterfly foraging behavior, was first proposed by Professor Arora et al. in 2018. In nature, butterflies use multiple sensory organs to find food, such as vision, touch, etc. The most important one is the smell. In BOA, each butterfly can emit a certain concentration of fragrance and smell the fragrance nearby. The butterfly moves towards the most fragrant direction. This phase is known as the global search phase. Suppose one butterfly cannot smell the fragrance from other butterflies. It will move randomly. This phase is known as the local search phase. For the above behaviors, we make a range of assumptions as follows:
a) All the butterflies can disseminate a particular concentration of fragrance.
b) Each butterfly moves only at random or towards the direction of the highest concentration.
c) The intensity of the stimulus is only affected by the objective function.
d) The butterfly controls the local search and the global search by switching the probability p.
The fragrance concentration is closely related to the population's fitness, and related to the following three factors: stimulus intensity I, perceived form c, and power index α. The relationship among them can be expressed as
f=cIα | (3.1) |
In the population iteration process, for butterflies moving through search space, the first thing to do is to calculate the fitness of all butterflies and then calculate the fragrance that the group produced at that location through Eq (3.2).
xit+1=xit+(r2×g∗−xit)×fi | (3.2) |
where xt+1i is the location xi for ith butterfly in iteration t+1, xti is the location xi for ith butterfly in iteration t.Here g∗ represents the current best location among all the locations in the current stage. The fragrance of ith butterfly is represented by fi. r, a random number in [0, 1]. The local search phase can be expressed as Eq (3.3).
xit+1=xit+(r2×xjt−xkt)×fi | (3.3) |
where xtjand xtk are the random butterflies in the solution space from the current population. r, is a random number in [0, 1]. Based on the above description, the process of BOA is as follows:
Step 1: Set each parameter and initialize the population position.
Step 2: Calculate fitness function and find the best population.
Step 3: If rand > p, , do global search using Eq (3.2).
Step 4: If rand < p do local search using Eq (3.3).
Step 5: Judge whether butterfly exceeds the search boundary and do boundary treatment.
Step 6: Calculate the fitness of the new population in Step 3 or Step 4, and if it is better than the current solution, update the global optimal solution and the global optimal fitness.
Step 7: If the algorithm meets the maximum iteration, jump out of the program, else jump to Step3.
Like other classical evolutionary computation algorithms, BOA randomly initializes population, which may cause population unequally distribution at the initial stage. It will enormously affect the speed of searching. Chaotic map is one of the methods to solve this problem. In this paper, we choose Kent chaotic map, which can improve the search speed and increase population diversity.
Chaos phenomenon is one of the main manifestations of complex dynamics of non-linear dynamic mapping, which has great randomness, non-periodicity and other characteristics. So, it is widely used by scholars in the fields of information security, communication encryption and control theory [27,28,29]. In the field of evolutionary computation, chaotic mapping is often used to replace random number generator to initialize population [30,31,32].
Logistic chaotic map [33] is a commonly used chaotic map model, which is famous for its simple structure. However, it has a natural disadvantage, that is, it depends too much on the setting of parameters and initial values [34]. Because the chaotic interval is limited and the output chaotic sequence values are not uniformly distributed, the effect is still not ideal when the model is in a full mapping state. Kent chaotic map [35] is designed by
xk+1={xkα0≤x≤α1−xk1−αα<x≤1 | (4.1) |
which combines the advantages of simple structure and uniform distribution of sequence values.
As shown in Figures 2 and 3, when iterating 200 times, there is still a part of the value aggregation extremes under the Logistic chaotic map model compared with the Kent map. To show the advantages of Kent map more clearly, we iterate 1000 times to get the value distribution of the Logistic map and the Kent map as shown in Figure 4, where the X-axis represents the range of values generated, the Y-axis represents the type of chaotic model, and the Z-axis represents the frequency of sequence values generated by the chaotic model in the relevant range.
It is clear from the graph that the Kent chaotic map has better randomness and chaotic characteristics than Logistic chaotic map. So, it can utilize as much information as possible in the solution space.
BOA is similar to other heuristic swarm intelligence algorithms, facing the balance of the capacities between the global and the local search. A host of scholars use the inertial weight strategy, including linearity weight and nonlinearity weight to solve this problem. Both linearity and nonlinearity weight strategies have been widely used in the improvement of intelligent algorithms.
Sigmoid output=11+e−x | (4.2) |
We consider a better balance between linearity and nonlinearity of Sigmoid function [36], mathematical description of Sigmoid function is shown as Eq (4.2).
We design a new inertial weight based on the Sigmoid function. Inspired by the Paper [37], we add a self-adaptive property to make population search more flexible. The new function is shown as follows
w=1(1+μe10tM−5)2 | (4.3) |
where μ∈ [0, 1], tis the current iteration number, M is the maximum iteration number. At the beginning of the iteration, the weight is relatively large. The weight is slowly reduced as the number of iterations increases, which is expected to search in the most extensive possible range. In the middle of the iteration, the weight reduces at a relatively rapid rate to ensure a faster search speed. In the later iteration, the weight slowly decreases so that the local search ability increases, and it gradually approaches the global optimal solution within the solution space. The weight evolution curve depicts as Figure 5.
Equation (4.4) which is modified from Eq (3.2) described as follows:
xt+1i=w×xti+(r2×g∗−xti)×fi. | (4.4) |
In the local search phase of BOA, when a butterfly cannot feel the fragrance of the best butterfly, it can only move towards the butterfly nearby. At this time, the blindness of population limits the search range to some extent, which would lead individuals to fall into the local optimum. In order to enhance the ability of local search, in this paper, we draw on the idea of genetic algorithm (GA) [38] and differential evolution (DE) [39], propose elite-fusion mutation and elite-oriented mutation strategies, which enlarge the population on the one hand and reduce the possibility of algorithm falling into the local optimum at the end of the iteration on the other hand. Equation (4.5), which is modified from Eq (3.3) described as follows:
xt+1i=β×[xti+(r2×xtj−xtk)]+(1−β)×g∗ | (4.5) |
xt+1i=g∗+θ×(xtj−xtk)+γ×(xtm−xtn). | (4.6) |
where xti is the position xi for tth butterfly in iteration number, xt+1i is the position xi for t+1th butterfly in iteration number, xtj,xtk,xtm and xtn are the random butterflies in the solution space from the current population. θ, β, γ is a number in [0, 1]. Equation (4.6) retains part of the elite solution and merges it with the population generated from the local search phase. They compose a new solution through a certain weight, thus enriching the population diversity.
In the search process, H-BOA relies on the information of elite butterflies, so the direction of elite butterflies, that is, the direction with the strongest fragrance, is very important. Normal distribution disturbance is a way of random disturbance term, which is usually used in optimization problem [40,41]. In nature, normal distribution is one of the objective laws of nature. Introducing positive distribution to disturb the population is in line with the objective law of swarm intelligence algorithm. Therefore, adding a normal distribution disturbance term to the new location individuals generated in the search stage will not destroy the search law and expand the search space to a certain extent.
Simulated annealing (SA) is a heuristic algorithm proposed by Professor Metropolis in 1953. It is based on the principle of solid annealing [42]. The most commonly used is the Metropolis criterion, which says that the algorithm dynamically generates a probability to determine whether to accept an inferior solution at each iteration.so it will help the algorithm jump out of the local optimum effectively. This shows excellent global optimization.
If the complete simulated annealing algorithm is combined with BOA, it will increase the time complexity of the algorithm and reduce the convergence speed of the algorithm. Therefore, we adopt the core idea of Metropolis criterion to help the algorithm jump out of the local optimal solution while ensuring the convergence speed. In this paper, the initial solution of the simulated annealing process is the population after the search stage. We add the population subject to the disturbance of standard normal distribution to replace the process of generating random solutions in the simulated annealing process, as well as combining it with the simulated annealing process. The new solution is explored around the optimal individual through Metropolis criterion, so the population can make full use of the characteristic information reflected by the best butterfly and guide the whole group to evolve towards the optimal solution. At the same time, it has the ability to jump out of the local optimum and great convergence speed. It can constantly modify and refine the evolution direction.
Assume that Tt is the current temperature, T0 is the initial temperature, the maximum number of iterations is M, and the current iteration is t. We parallel it to the search phase. The flow chart of this stage is shown in Figure 6.
Based on the above description, the Pseudocode of H-BOA can be described as follows:
Algorithm 1 A Hybrid-Strategy-Improved Butterfly Optimization Algorithm |
Input: Population size N, switch probability p, pc, sensor modality c, power exponent α, maxi-mum iterations M and parameters β,γ,θ,μ,T0 Output: Best solution X∗ 1 Initialize the butterfly population F with Kent chaotic map 2 for t← 1 to M do 3 for i← 1 to N do 4 Calculate fitness of F 5 end for 6 Find the best solution as Xbest 7 for i← 1 to N do 8 Generate a random number r from [0, 1] 9 if r≤p then 10 Do global search using Eq (4.4) 11 else 12 If r>pcthen 13 Do elite-fusion local mutation strategy using Eq (4.5) 14 else 15 Do elite-oriented local mutation strategy using Eq (4.6) 16 end if 17 end if 18 end for 19 Perform disturbance with Standard Normal Distribution 20 Perform simulated annealing process according to Metropolis criterion 21 Update the best solution 22 end for |
Time complexity of an algorithm is one of the criteria for evaluating the performance of an algorithm. In BOA, assume that the number of populations is N, the objective function is f(x), and the dimension is n. According to the analysis of BOA, the time complexity of BOA is O(n + f(n)).
For H-BOA, in the initial population phase, assume the time for initializing each parameter is t1, the time for initializing the population position using Kent chaotic map is t2, the time for calculating fitness according to the population position is f(n), and the time to save the current optimal solution is t3, so the total time complexity in this phase is
O(N(nt2+t3+f(n)+t1)=O(n+f(n)). |
When entering the iteration, assume the time for updating new inertia weight is t4. The time to calculate the individual fragrance concentration is t5.
1) Global search phase: Assume that the time used to update each dimension of the butterfly position is t6 according to Eq (4.4), the time to calculate the fitness of the new population is f(n), the time to compare the fitness of the latest and old butterfly is t7. Then the total time complexity of the global search phase is
O(N(t4+t5+nt6+t7+f(n))=O(n+f(n)). |
2) Local search phase: The population uses two mutation methods to perform local wandering. The time to generate a random number p c is t8, the time used to update each dimension of the butterfly is t9 and t10 according to Eqs (4.5) and (4.6), the time to calculate new population fitness is f(n). The time for comparing and replacing the fitness of the old and new population is the same as that of the global search phase, which is t7. Then the total time complexity of this stage is
O(N(t4+t7+n(t8+t9+t10)+f(n)))=O(n+f(n)). |
Standard normal disturbance and simulated annealing stage: Assume that the time to generate a normal distribution of random numbers is t12, the time used to calculate each dimension of the butterfly after disturbance is t13, the fitness of the new population is calculated as f(n) and the time complexity of the simulated annealing stage is O(Nn), then the total time complexity of this stage is
O(N(t12+t13)+f(n))+O(Nn)=O(n+f(n)). |
Record the optimal solution stage: Assume that the comparison time of each butterfly's fitness with the current optimal solution is t14 and the time to replace the new solution in the simulated annealing phase is t15.so the total time complexity of this stage is
O(N(t14+t15))=O(n). |
Therefore, the total time complexity of H-BOA is O(n+f(n)), which does not increase the extra time complexity compared with BOA.
The space complexity is mainly affected by dimensionality and population size, so the space complexity of the two algorithms is O(Nn).
In this section, we analyze the asymptotic and convergence of H-BOA. In addition, the definitions and theorems are provided.
Definition 1. The objective function f:Rn→R is a continuous function on the non-empty feasible region S and there is an optimal solution set Ω={x∗|x∗∈S,f(x∗)<δ} in S, δ is an acceptable fitness.
Theorem 1. H-BOA has progressive fitness. Assume that the optimal butterfly position found in the tth is x∗t, the non-negative random process generated by H-BOA is{dt∣=f(x∗t)−f(x∗),1≤t≤T}. When f(x∗t)>f(x∗), there is a normal number τ, which makes E(dt+1)≤E(dt)−τ. Then we can say the optimization algorithm has progressive fitness [43].
Proof. According to the analysis in Section 4.4, the new solution generated by normal distribution disturbance and simulated annealing process is not necessarily closer to the global optimal solution, but the previous optimal position will be recorded during optimization, that is, the original better position will not be covered. Therefore, the distance between the new solution and the global optimal solution will not increase after annealing, that is, the direction of population evolution is monotonous.
Because the core purpose of H-BOA is to find the most fragrant butterfly so that P(f(x∗t+1)−f(x∗t)>0)=0. The butterfly population updates individual positions in three ways and all of them have random factors. SoP(f(x∗t+1)=f(x∗t))≠1. From the above derivations we can get
P(f(x∗t+1)−f(x∗t)<0)>0. |
Let E[f(x∗t+1)−f(x∗t)]=−τt+1,where τt+1>0. So
E(dt+1−dt)=E[(f(x∗t+1)−f(x∗))−(f(x∗t)−f(x∗))]=E(f(x∗t+1)−f(x∗t) |
=−τt+1. |
That is E(dt+1)=E(dt)−τt+1. Finally, let τ=min{τ1,τ2,···,τT}, so we can get
E(dt+1)≤E(dt)−τ. |
So, the algorithm has progressive fitness.
In the next step, we will analyze the convergence of H-BOA and the definitions and theorems are provided [44,45].
Definition 2. There is a set of random sequences ξt(t=1,2,···) in the probability space, if there is a random variable ξ when any ϵ>0 is satisfied, it makes
limt→∞P{|ξt−ξ|<ϵ}=1 |
or the following equation is qualified, namely
P{⋃∞n=1⋂∞k≥n[|ξt−ξ|≥ϵ]}=0. |
Then the random sequence {ϵt} is said to converge to Random variable ε with probability 1.
Lemma 1: (Borel-Cantelli Lemmas): Let A1,A2,···F
1) If ∑∞n=1P(An)<∞, then P(limsupnAn)=0.
2) If∑∞n=1P(An)=∞ and {An} are independent, then P(limsupnAn)=1.
Theorem 2. Let {X(t)} be the position sequence of H-BOA. {Xg(t)}∈X(t) be the best solution sequence. Assume that ΔE generated in the simulated annealing stage satisfies ΔE∼N(μ,σ2) If Definition 2 is satisfied, namely
P{limt→∞f(xg(t))=X∗}=1. |
We can call that H-BOA converges to the global optimum with probability 1.
Proof. Let p(k)=∏kt=1P{|f(Xg(t))−X∗|≥ϵ} where ϵ > 0. Here X∗ is the global optimal solution. SupposeX∗=min{f(x),x∈Ω}, then
p(k)=k∏t=1P{|f(Xg(t))−X∗|≥ϵ}=∏kt=1P{f(Xg(t))−X∗≥ϵ} |
=∏kt=1P{Δf(Xg(t))≥ϵ+X∗−f(Xg(t−1))}. |
So, we can get an equation from Δf(Xg(t))∼N(μ1,σ21) shown as
P(k)=∏kt=1∫∞ϵ+X∗−f(Xg(t−1))1√2πσ1e−x22πσ21dt, |
then let
b=max{∫∞ϵ+X∗−f(Xg(t−1))1√2πσ1e−x22πσ21dt}. |
According to the relevant knowledge of the infinite series, we can get
∑∞k=1p(k)≤∑∞k=1bk=11−b<∞, |
From Lemma 1 we can get
P{⋃∞n=1⋂∞k≥n|f(Xg(t))−X∗|≥ϵ}=0. |
Finally, it can be seen that f(Xg(t) converges to X∗ with probability 1 from Definition 2.
The experimental environment is Windows10, 64-bit operating system, CPU is Intel Core i510400H, main frequency is 3.2 GHz, memory is 8 G, and the algorithm is based on MATLAB2020b.
To verify the performance of H-BOA, we select ten international benchmark functions shown in Table 2, where f1,f2,f3,f4,f5,f6 are unimodal functions, f7,f8,f9,f10 are multimodal functions. We compared H-BOA with particle swarm optimization (PSO), BOA, a new inertia weight proposed in Section 4.2 improved butterfly optimization algorithm (IBOA) and butterfly optimization algorithm of Cauchy mutation and adaptive weight optimization (CWBOA) [46]. To keep the fairness and objectivity, the initial population size is all uniformly set to 30, the number of iterations is set to 500, and the part of parameters are the same as those in CWBOA. To reduce the randomness, we conducted 30 experiments and took the average and standard. The specific algorithm parameter settings are shown in Table 1. The data of CWBOA is directly derived from the paper [46].
Algorithm | parameter settings |
H-BOA | p=pc=0.8,β=0.3,θ=γ=0.1,μ=1,T0=1000 p=0.8,I=0.01,α=0.1 p=0.8,I=0.01,α=0.1 p=0.8,I=0.01,α=0.1 c1=c2=2,Vmax=1,Vmin=−1 |
IBOA | |
BOA CWBOA PSO |
Function | dim | range | best value |
f1=n∑i=1x2i | 30 | (-100,100) | 0 |
f2=max(|xi|,1<i<n) | 30 | (-100,100) | 0 |
f3=n∑i=1|xi|+n∏i=1|xi| | 30 | (-10, 10) | 0 |
f4=n∑i=1(n∑j=1xj)2 | 30 | (-100,100) | 0 |
f5=n∑i=1ix4+random(0,1) | 30 | (-1.28, 1.28) | 0 |
f6=0.26(x21+x22)−0.48x1x2 | 30 | (-10, 10) | 0 |
f7=n∑i=1[x2i−10cos(2πxi)+10] | 30 | (-5.12, 5.12) | 0 |
f8=d∑i=1x2i+(d∑i=10.5ixi)2+(d∑i=10.5ixi)4 | 30 | (-5, 10) | 0 |
f9=14000d∑i=1x2i−d∏i=1cos(xi√i)+1 | 30 | (-600,600) | 0 |
f10=−20exp(−0.2×√1nn∑i=1x2i)−exp(1nn∑i=1cos(2πxi))+20+e | 30 | (-32, 32) | 0 |
To measure the convergence and accuracy of H-BOA, Table 3 shows the optimization results of the five algorithms on the test functions. "-" represents the test function is not included in the original paper. Figure 7 shows the iterative convergence curves of the four algorithms where the X-axis represents the number of iterations and the Y-axis represents the fitness.
Function | HBOA | IBOA | CWBOA | BOA | PSO | |
f1 | Mean | 0 | 1.36E-11 | 0 | 5.70E-11 | 8.81E+02 |
Std | 0 | 5.90E-13 | 0 | 6.59E-12 | 1.72E+02 | |
f2 | Mean | 0 | 1.17E-11 | 3.29E-134 | 5.53E-11 | 1.08E+03 |
Std | 0 | 8.53E-13 | 1.80E-133 | 5.30E-12 | 6.54E+02 | |
f3 | Mean | 0 | 5.99E-09 | 3.86E-134 | 1.94E-08 | 5.49E+00 |
Std | 0 | 4.70E-10 | 1.52E-133 | 2.52E-09 | 8.36E-01 | |
f4 | Mean | 0 | 2.91E-09 | - | 1.75E-08 | 1.05E+01 |
Std | 0 | 1.69E-09 | - | 2.59E-09 | 2.87E+00 | |
f5 | Mean | 8.71E-05 | 1.15E-03 | 1.57E-04 | 1.63E-03 | 2.47E-01 |
Std | 7.24E-05 | 2.89E-04 | 1.35E-04 | 7.81E-04 | 1.40E-01 | |
f6 | Mean | 0 | 9.79E-13 | 0 | 1.78E-12 | 5.43E-84 |
Std | 0 | 3.18E-13 | 0 | 2.50E-13 | 9.22E-84 | |
f7 | Mean | 0 | 2.27E-14 | 0 | 1.30E+02 | 1.04E+02 |
Std | 0 | 3.11E-14 | 0 | 7.49E+01 | 1.62E+01 | |
f8 | Mean | 0 | 1.12E-11 | 0 | 5.39E-11 | 1.25E+04 |
Std | 0 | 1.27E-12 | 0 | 7.27E-12 | 9.39E+03 | |
f9 | Mean | 0 | 8.04E-12 | 0 | 3.28E-11 | 9.14E+00 |
Std | 0 | 6.77E-13 | 0 | 1.14E-11 | 4.32E+00 | |
f10 | Mean | 8.88E-16 | 5.72E-09 | 8.88E-16 | 1.93E-08 | 1.02E+01 |
Std | 0 | 7.95E-11 | 0 | 1.12E-09 | 8.39E-01 |
It can be seen from Table 3 that H-BOA has found the optimal function value 0 except f5 and f10 and the optimization effect reaches 100%. For function f5, the average accuracy of H-BOA is two orders of magnitude better than BOA, and one order of magnitude better than CWBOA. As for the function f10, the average accuracy of H-BOA is seven orders of magnitude better than that of BOA, which is the same as the result of CWBOA and the variance is 0. The algorithm has good stability and robustness. In addition, as can be seen from the results that the performance of IBOA is generally better than that of BOA, which shows the new inertia weight strategy is feasible.
As can be seen from Figure 7, when solving different functions, the curve of the H-BOA is smoother, the convergence speed is faster, the optimization accuracy is higher and the curve inflection point appears firstly, which indicating that H-BOA has a stronger ability to overcome the local optimum. Further prove the effectiveness of hybrid strategies.
The simulation experiment uses MATLAB, and the environment settings are the same with international function test. We choose H-BOA, BOA, the single-strategy improved butterfly optimization algorithm (IBOA) and the sparrow search algorithm (SSA) for comparison. The algorithm parameters are shown as Table 4, where the maximum number of iterations is M=100, and the population size is N=30.
Algorithm | parameter settings |
H-BOA | p=pc=0.8,β=0.3,θ=γ=0.1,μ=1,T0=1000 |
IBOA | p=0.8,I=0.01,α=0.1 |
BOA SSA |
p=0.8,I=0.01,α=0.1 ST=0.6,PD=0.7,SD=0.2 |
To show the effectiveness of H-BOA, in this paper, we tried to do multiple experiments with different numbers of nodes. The experimental parameter settings are shown in Table 5. Figure 8 shows the simulation coverage figure. Figure 9 describes the maximum coverage percent that various algorithm can achieve and Table 6 shows the specific maximum coverage value. Figure 10 shows the evolution curve of the maximum coverage of WSN with the number of population iterations when the number of nodes is 25, as can be seen from the figure that H-BOA has more uniform distribution and less gaps. This fully shows higher convergence and better robustness. In particular, for some wireless sensor node deployment tasks that require significantly higher coverage, the optimization strategy of the HBOA algorithm can achieve more excellent optimization with fewer nodes in less time.
Parameter settings | value |
Region Area | 100m × 100m |
Pixels | 100 × 100 |
Nodes Perceived Radius |
N = 10, 15, 20, 25, 30 R = 15m |
Algorithm | Number of nodes | Maximum coverage (%) | |||
N = 10 | N = 15 | N = 20 | N = 25 | N = 30 | |
SSA | 62.14 | 78.51 | 83.42 | 92.60 | 94.50 |
H-BOA | 64.61 | 80.05 | 91.21 | 95.78 | 98.34 |
BOA | 56.82 | 71.81 | 82.47 | 90.91 | 94.13 |
IBOA | 56.68 | 74.42 | 83.07 | 91.60 | 94.37 |
In summary, H-BOA has better performance in WSN node coverage application and can improve energy effectiveness and real-time schedule.
Based on BOA, we propose a hybrid-strategy-improved butterfly optimization algorithm (HBOA). First, H-BOA uses Kent chaotic map to initialize the population to keep a more balanced search space. Next, we introduce a new inertial weight modified from the Sigmoid function to increase the fixability of global search and local search. Then the introduction of elite-fusion and elite-oriented strategy aims to improve population diversity. Finally, we adopt the disturbance based on standard normal distribution to prevent the algorithm from falling into precociousness and use simulated annealing process to ensure the quality of the solution. The above improvement points observably improve the performance of the algorithm. We selected the international test functions for performance testing and compared them with BOA, a single-strategy-improved butterfly optimization algorithm (IBOA) and particle swarm optimization (PSO). The results show that H-BOA is better than other algorithms, together with higher accuracy and robustness. We apply H-BOA in WSN coverage research, which proves that this algorithm can effectively solve this type of problem and broaden the application field. However, the algorithm still has some shortcomings. Our next work is how to optimize the strategy to make the algorithm more expressive and propose more effective hybrid heuristic algorithms for different and more complex problems.
All sources of funding of the study must be disclosed.
The authors declare there is no conflict of interest.
[1] |
A. El-Gohary, A. Alshamrani, A. N. Al-Otaibi, The generalized Gompertz distribution, Appl. Math. Model., 37 (2013), 13–24. https://doi.org/10.1016/j.apm.2011.05.017 doi: 10.1016/j.apm.2011.05.017
![]() |
[2] |
A. Saboor, H. S. Bakouch, M. N. Khan, Beta sarhan–zaindin modified Weibull distribution, Appl. Math. Model., 40 (2016), 6604–6621. https://doi.org/10.1016/j.apm.2016.01.033 doi: 10.1016/j.apm.2016.01.033
![]() |
[3] |
X. Jia, S. Nadarajah, B. Guo. Bayes estimation of P (Y < X) for the Weibull distribution with arbitrary parameters, Appl. Math. Model., 47 (2017), 249–259. https://doi.org/10.1016/j.apm.2017.03.020 doi: 10.1016/j.apm.2017.03.020
![]() |
[4] |
A. J. Fernández, Optimal lot disposition from Poisson–Lindley count data, Appl. Math. Model., 70 (2019), 595–604. https://doi.org/10.1016/j.apm.2019.01.045 doi: 10.1016/j.apm.2019.01.045
![]() |
[5] |
M. Alizadeh, A. Z. Afify, M. S. Eliwa, S. Ali, The odd log-logistic Lindley-G family of distributions: Properties, Bayesian and non-Bayesian estimation with applications, Comput. Stat., 35 (2020), 281–308. https://doi.org/10.1007/s00180-019-00932-9 doi: 10.1007/s00180-019-00932-9
![]() |
[6] |
S. Kumar, A. S. Yadav, S. Dey, M. Saha, Parametric inference of generalized process capability index Cpyk for the power Lindley distribution, Qual. Technol. Quant. Manage., 19 (2022), 153–186. https://doi.org/10.1080/16843703.2021.1944966 doi: 10.1080/16843703.2021.1944966
![]() |
[7] |
S. Nedjar, H. Zeghdoudi, On gamma Lindley distribution: Properties and simulations, J. Comput. Appl. Math., 15 (2016), 167–174. https://doi.org/10.1016/j.cam.2015.11.047 doi: 10.1016/j.cam.2015.11.047
![]() |
[8] | H. Messaadia, H. Zeghdoudi, Around gamma Lindley distribution, J. Mod. Appl. Stat. Methods., 16 (2017), 23. |
[9] |
D. Roy, The discrete normal distribution, Commun. Stat. Theory Methods, 32 (2003), 1871–1883. https://doi.org/10.1081/STA-120023256 doi: 10.1081/STA-120023256
![]() |
[10] |
E. Gómez-Déniz, E. Calderín-Ojeda, The discrete Lindley distribution: Properties and applications, J. Stat. Comput. Simul., 81 (2011), 1405–1416. https://doi.org/10.1080/00949655.2010.487825 doi: 10.1080/00949655.2010.487825
![]() |
[11] |
M. Bebbington, C. D. Lai, M. Wellington, R. Zitikis, The discrete additive Weibull distribution: A bathtub-shaped hazard for discontinuous failure data, Reliab. Eng. Syst. Saf., 106 (2012), 37–44. https://doi.org/10.1016/j.ress.2012.06.009 doi: 10.1016/j.ress.2012.06.009
![]() |
[12] |
V. Nekoukhou, M. H. Alamatsaz, H. Bidram, Discrete generalized exponential distribution of a second type, Statistics, 47 (2013), 876–887. https://doi.org/10.1080/02331888.2011.633707 doi: 10.1080/02331888.2011.633707
![]() |
[13] | M. H. Alamatsaz, S. Dey, T. Dey, S. S. Harandi, Discrete generalized Rayleigh distribution, Pak. J. Stat., 32 (2016). |
[14] |
M. El-Morshedy, M. S. Eliwa, H. Nagy, A new two-parameter exponentiated discrete Lindley distribution: Properties, estimation and applications, J. Appl. Stat., 47 (2020), 354–375. https://doi.org/10.1080/02664763.2019.1638893 doi: 10.1080/02664763.2019.1638893
![]() |
[15] |
J. Gillariose, O. S. Balogun, E. M. Almetwally, R. A. Sherwani, F. Jamal, J. Joseph, On the discrete Weibull Marshall–Olkin family of distributions: Properties, characterizations, and applications, Axioms, 10 (2021), 287. https://doi.org/10.3390/axioms10040287 doi: 10.3390/axioms10040287
![]() |
[16] |
B. Singh, R. P. Singh, A. S. Nayal, A. Tyagi, Discrete inverted Nadarajah-Haghighi distribution: Properties and classical estimation with application to complete and censored data, Stat. Optim. Inform. Comput., 10 (2022), 1293–1313. https://doi.org/10.19139/soic-2310-5070-1365 doi: 10.19139/soic-2310-5070-1365
![]() |
[17] |
B. Singh, V. Agiwal, A. S. Nayal, A. Tyagi, A discrete analogue of Teissier distribution: Properties and classical estimation with application to count data, Relia. Theory Appl., 17 (2022), 340–355. https://doi.org/10.24412/1932-2321-2022-167-340-355 doi: 10.24412/1932-2321-2022-167-340-355
![]() |
[18] |
M. S. Eliwa, M. El-Morshedy, A one-parameter discrete distribution for over-dispersed data: Statistical and reliability properties with applications, J. Appl. Stat., 49 (2022), 2467–2487. https://doi.org/10.1080/02664763.2021.1905787 doi: 10.1080/02664763.2021.1905787
![]() |
[19] |
E. Altun, M. El-Morshedy, M. S. Eliwa, A study on discrete Bilal distribution with properties and applications on integervalued autoregressive process, Stat. J., 20 (2022), 501–528. https://doi.org/10.57805/revstat.v20i4.384 doi: 10.57805/revstat.v20i4.384
![]() |
[20] | M. El-Morshedy, E. Altun, M. S. Eliwa, A new statistical approach to model the counts of novel coronavirus cases, Math. Sci., (2021), 1–4. https://doi.org/10.1007/s40096-021-00390-9 |
[21] |
J. R. Hosking, L-moments: Analysis and estimation of distributions using linear combinations of order statistics, J. Royal Stat. Soc. Series B, 52 (1990), 105–124. https://doi.org/10.1111/j.2517-6161.1990.tb01775.x doi: 10.1111/j.2517-6161.1990.tb01775.x
![]() |
1. | Jicheng Yao, Xiaonan Luo, Fang Li, Yizhou Feng, Jundi Dou, Lixiang Dai, Songhua Xu, Ruiai Chen, 2022, Butterfly intelligent optimization algorithm based on Good Point Set and adaptive weight factor, 978-1-6654-5478-0, 194, 10.1109/ICDH57206.2022.00037 | |
2. | Maosong Peng, Xiuxi Wei, Huajuan Huang, A chaotic adaptive butterfly optimization algorithm, 2023, 1864-5909, 10.1007/s12065-023-00832-4 | |
3. | Qing He, Zhouxin Lan, Damin Zhang, Liu Yang, Shihang Luo, Improved Marine Predator Algorithm for Wireless Sensor Network Coverage Optimization Problem, 2022, 14, 2071-1050, 9944, 10.3390/su14169944 | |
4. | Wen Long, Jianjun Jiao, Tiebin Wu, Ming Xu, Shaohong Cai, A balanced butterfly optimization algorithm for numerical optimization and feature selection, 2022, 26, 1432-7643, 11505, 10.1007/s00500-022-07389-x | |
5. | Khaoula Zaimen, Mohamed-El-Amine Brahmia, Laurent Moalic, Abdelhafid Abouaissa, Lhassane Idoumghar, A Survey of Artificial Intelligence Based WSNs Deployment Techniques and Related Objectives Modeling, 2022, 10, 2169-3536, 113294, 10.1109/ACCESS.2022.3217200 | |
6. | Zhenzhen Geng, 2023, Chapter 78, 978-981-19-9372-5, 693, 10.1007/978-981-19-9373-2_78 | |
7. | Yan Liu, 2024, Refining Machine Learning for Employee Turnover Classification: A CAP-BOA-Based Probability Neural Network Approach, 979-8-3503-8098-9, 741, 10.1109/EEBDA60612.2024.10486002 | |
8. | Junqi Geng, Haihua Wang, Jie Su, Xiaoyu Zheng, Xianming Sun, Xu Wu, Yue Zhang, 2023, Coverage optimization of wireless sensor networks with improved golden jackal optimization, 979-8-3503-1449-6, 1, 10.1109/ICECAI58670.2023.10176640 | |
9. | J. David Sukeerthi Kumar, M. V. Subramanyam, A. P. Siva Kumar, Hybrid Sand Cat Swarm Optimization Algorithm-based reliable coverage optimization strategy for heterogeneous wireless sensor networks, 2024, 2511-2104, 10.1007/s41870-024-02163-8 | |
10. | V. Saravanan, Indhumathi G, Ramya Palaniappan, Narayanasamy P, M. Hema Kumar, K. Sreekanth, Navaneethan S, A novel approach to node coverage enhancement in wireless sensor networks using walrus optimization algorithm, 2024, 24, 25901230, 103143, 10.1016/j.rineng.2024.103143 | |
11. | Xuejun Chen, Ying Wang, Haitao Zhang, Jianzhou Wang, A novel hybrid forecasting model with feature selection and deep learning for wind speed research, 2024, 43, 0277-6693, 1682, 10.1002/for.3098 | |
12. | Ateq Alsaadi, Fazal Dayan, Nauman Ahmed, Dumitru Baleanu, Muhammad Rafiq, Ali Raza, A novel method for the dynamics of worms in wireless sensor networks with fuzzy partition, 2023, 13, 2158-3226, 10.1063/5.0165342 | |
13. | Chengwang Lin, Hoiman Cheng, Parameter Optimization and Solution Performance Analysis of Multi-Modal Butterfly Optimization Algorithm, 2024, 12, 2169-3536, 143163, 10.1109/ACCESS.2024.3470845 | |
14. | Haixu Niu, Yonghai Li, Chunyu Zhang, Tianfei Chen, Lijun Sun, Muhammad Irsyad Abdullah, Multi-Strategy Bald Eagle Search Algorithm Embedded Orthogonal Learning for Wireless Sensor Network (WSN) Coverage Optimization, 2024, 24, 1424-8220, 6794, 10.3390/s24216794 | |
15. | Zhixiong Liu, Wei Zhou, Energy-Efficient Algorithms for Path Coverage in Sensor Networks, 2023, 23, 1424-8220, 5026, 10.3390/s23115026 | |
16. | Mostafa Basirnezhad, Mahboobeh Houshmand, Seyyed Abed Hosseini, Mehrdad Jalali, 2023, Optimizing Coverage in Wireless Sensor Networks Using the Cheetah Meta-Heuristic Algorithm, 979-8-3503-6941-0, 1, 10.1109/IoT60973.2023.10365363 | |
17. | Ojonukpe S. Egwuche, Abhilash Singh, Absalom E. Ezugwu, Japie Greeff, Micheal O. Olusanya, Laith Abualigah, Machine learning for coverage optimization in wireless sensor networks: a comprehensive review, 2023, 0254-5330, 10.1007/s10479-023-05657-z | |
18. | Aiguo Li, Jie Yang, 2022, Multi-objective optimization algorithm for indoor positioning sensor deployment based on wireless network, 9781450397520, 110, 10.1145/3586102.3586138 | |
19. | Aiguo Li, Yunfei Jia, A Base Station Deployment Algorithm for Wireless Positioning Considering Dynamic Obstacles, 2025, 82, 1546-2226, 4573, 10.32604/cmc.2025.059184 |
Algorithm 1 A Hybrid-Strategy-Improved Butterfly Optimization Algorithm |
Input: Population size N, switch probability p, pc, sensor modality c, power exponent α, maxi-mum iterations M and parameters β,γ,θ,μ,T0 Output: Best solution X∗ 1 Initialize the butterfly population F with Kent chaotic map 2 for t← 1 to M do 3 for i← 1 to N do 4 Calculate fitness of F 5 end for 6 Find the best solution as Xbest 7 for i← 1 to N do 8 Generate a random number r from [0, 1] 9 if r≤p then 10 Do global search using Eq (4.4) 11 else 12 If r>pcthen 13 Do elite-fusion local mutation strategy using Eq (4.5) 14 else 15 Do elite-oriented local mutation strategy using Eq (4.6) 16 end if 17 end if 18 end for 19 Perform disturbance with Standard Normal Distribution 20 Perform simulated annealing process according to Metropolis criterion 21 Update the best solution 22 end for |
Algorithm | parameter settings |
H-BOA | p=pc=0.8,β=0.3,θ=γ=0.1,μ=1,T0=1000 p=0.8,I=0.01,α=0.1 p=0.8,I=0.01,α=0.1 p=0.8,I=0.01,α=0.1 c1=c2=2,Vmax=1,Vmin=−1 |
IBOA | |
BOA CWBOA PSO |
Function | dim | range | best value |
f1=n∑i=1x2i | 30 | (-100,100) | 0 |
f2=max(|xi|,1<i<n) | 30 | (-100,100) | 0 |
f3=n∑i=1|xi|+n∏i=1|xi| | 30 | (-10, 10) | 0 |
f4=n∑i=1(n∑j=1xj)2 | 30 | (-100,100) | 0 |
f5=n∑i=1ix4+random(0,1) | 30 | (-1.28, 1.28) | 0 |
f6=0.26(x21+x22)−0.48x1x2 | 30 | (-10, 10) | 0 |
f7=n∑i=1[x2i−10cos(2πxi)+10] | 30 | (-5.12, 5.12) | 0 |
f8=d∑i=1x2i+(d∑i=10.5ixi)2+(d∑i=10.5ixi)4 | 30 | (-5, 10) | 0 |
f9=14000d∑i=1x2i−d∏i=1cos(xi√i)+1 | 30 | (-600,600) | 0 |
f10=−20exp(−0.2×√1nn∑i=1x2i)−exp(1nn∑i=1cos(2πxi))+20+e | 30 | (-32, 32) | 0 |
Function | HBOA | IBOA | CWBOA | BOA | PSO | |
f1 | Mean | 0 | 1.36E-11 | 0 | 5.70E-11 | 8.81E+02 |
Std | 0 | 5.90E-13 | 0 | 6.59E-12 | 1.72E+02 | |
f2 | Mean | 0 | 1.17E-11 | 3.29E-134 | 5.53E-11 | 1.08E+03 |
Std | 0 | 8.53E-13 | 1.80E-133 | 5.30E-12 | 6.54E+02 | |
f3 | Mean | 0 | 5.99E-09 | 3.86E-134 | 1.94E-08 | 5.49E+00 |
Std | 0 | 4.70E-10 | 1.52E-133 | 2.52E-09 | 8.36E-01 | |
f4 | Mean | 0 | 2.91E-09 | - | 1.75E-08 | 1.05E+01 |
Std | 0 | 1.69E-09 | - | 2.59E-09 | 2.87E+00 | |
f5 | Mean | 8.71E-05 | 1.15E-03 | 1.57E-04 | 1.63E-03 | 2.47E-01 |
Std | 7.24E-05 | 2.89E-04 | 1.35E-04 | 7.81E-04 | 1.40E-01 | |
f6 | Mean | 0 | 9.79E-13 | 0 | 1.78E-12 | 5.43E-84 |
Std | 0 | 3.18E-13 | 0 | 2.50E-13 | 9.22E-84 | |
f7 | Mean | 0 | 2.27E-14 | 0 | 1.30E+02 | 1.04E+02 |
Std | 0 | 3.11E-14 | 0 | 7.49E+01 | 1.62E+01 | |
f8 | Mean | 0 | 1.12E-11 | 0 | 5.39E-11 | 1.25E+04 |
Std | 0 | 1.27E-12 | 0 | 7.27E-12 | 9.39E+03 | |
f9 | Mean | 0 | 8.04E-12 | 0 | 3.28E-11 | 9.14E+00 |
Std | 0 | 6.77E-13 | 0 | 1.14E-11 | 4.32E+00 | |
f10 | Mean | 8.88E-16 | 5.72E-09 | 8.88E-16 | 1.93E-08 | 1.02E+01 |
Std | 0 | 7.95E-11 | 0 | 1.12E-09 | 8.39E-01 |
Algorithm | parameter settings |
H-BOA | p=pc=0.8,β=0.3,θ=γ=0.1,μ=1,T0=1000 |
IBOA | p=0.8,I=0.01,α=0.1 |
BOA SSA |
p=0.8,I=0.01,α=0.1 ST=0.6,PD=0.7,SD=0.2 |
Parameter settings | value |
Region Area | 100m × 100m |
Pixels | 100 × 100 |
Nodes Perceived Radius |
N = 10, 15, 20, 25, 30 R = 15m |
Algorithm | Number of nodes | Maximum coverage (%) | |||
N = 10 | N = 15 | N = 20 | N = 25 | N = 30 | |
SSA | 62.14 | 78.51 | 83.42 | 92.60 | 94.50 |
H-BOA | 64.61 | 80.05 | 91.21 | 95.78 | 98.34 |
BOA | 56.82 | 71.81 | 82.47 | 90.91 | 94.13 |
IBOA | 56.68 | 74.42 | 83.07 | 91.60 | 94.37 |
Algorithm 1 A Hybrid-Strategy-Improved Butterfly Optimization Algorithm |
Input: Population size N, switch probability p, pc, sensor modality c, power exponent α, maxi-mum iterations M and parameters β,γ,θ,μ,T0 Output: Best solution X∗ 1 Initialize the butterfly population F with Kent chaotic map 2 for t← 1 to M do 3 for i← 1 to N do 4 Calculate fitness of F 5 end for 6 Find the best solution as Xbest 7 for i← 1 to N do 8 Generate a random number r from [0, 1] 9 if r≤p then 10 Do global search using Eq (4.4) 11 else 12 If r>pcthen 13 Do elite-fusion local mutation strategy using Eq (4.5) 14 else 15 Do elite-oriented local mutation strategy using Eq (4.6) 16 end if 17 end if 18 end for 19 Perform disturbance with Standard Normal Distribution 20 Perform simulated annealing process according to Metropolis criterion 21 Update the best solution 22 end for |
Algorithm | parameter settings |
H-BOA | p=pc=0.8,β=0.3,θ=γ=0.1,μ=1,T0=1000 p=0.8,I=0.01,α=0.1 p=0.8,I=0.01,α=0.1 p=0.8,I=0.01,α=0.1 c1=c2=2,Vmax=1,Vmin=−1 |
IBOA | |
BOA CWBOA PSO |
Function | dim | range | best value |
f1=n∑i=1x2i | 30 | (-100,100) | 0 |
f2=max(|xi|,1<i<n) | 30 | (-100,100) | 0 |
f3=n∑i=1|xi|+n∏i=1|xi| | 30 | (-10, 10) | 0 |
f4=n∑i=1(n∑j=1xj)2 | 30 | (-100,100) | 0 |
f5=n∑i=1ix4+random(0,1) | 30 | (-1.28, 1.28) | 0 |
f6=0.26(x21+x22)−0.48x1x2 | 30 | (-10, 10) | 0 |
f7=n∑i=1[x2i−10cos(2πxi)+10] | 30 | (-5.12, 5.12) | 0 |
f8=d∑i=1x2i+(d∑i=10.5ixi)2+(d∑i=10.5ixi)4 | 30 | (-5, 10) | 0 |
f9=14000d∑i=1x2i−d∏i=1cos(xi√i)+1 | 30 | (-600,600) | 0 |
f10=−20exp(−0.2×√1nn∑i=1x2i)−exp(1nn∑i=1cos(2πxi))+20+e | 30 | (-32, 32) | 0 |
Function | HBOA | IBOA | CWBOA | BOA | PSO | |
f1 | Mean | 0 | 1.36E-11 | 0 | 5.70E-11 | 8.81E+02 |
Std | 0 | 5.90E-13 | 0 | 6.59E-12 | 1.72E+02 | |
f2 | Mean | 0 | 1.17E-11 | 3.29E-134 | 5.53E-11 | 1.08E+03 |
Std | 0 | 8.53E-13 | 1.80E-133 | 5.30E-12 | 6.54E+02 | |
f3 | Mean | 0 | 5.99E-09 | 3.86E-134 | 1.94E-08 | 5.49E+00 |
Std | 0 | 4.70E-10 | 1.52E-133 | 2.52E-09 | 8.36E-01 | |
f4 | Mean | 0 | 2.91E-09 | - | 1.75E-08 | 1.05E+01 |
Std | 0 | 1.69E-09 | - | 2.59E-09 | 2.87E+00 | |
f5 | Mean | 8.71E-05 | 1.15E-03 | 1.57E-04 | 1.63E-03 | 2.47E-01 |
Std | 7.24E-05 | 2.89E-04 | 1.35E-04 | 7.81E-04 | 1.40E-01 | |
f6 | Mean | 0 | 9.79E-13 | 0 | 1.78E-12 | 5.43E-84 |
Std | 0 | 3.18E-13 | 0 | 2.50E-13 | 9.22E-84 | |
f7 | Mean | 0 | 2.27E-14 | 0 | 1.30E+02 | 1.04E+02 |
Std | 0 | 3.11E-14 | 0 | 7.49E+01 | 1.62E+01 | |
f8 | Mean | 0 | 1.12E-11 | 0 | 5.39E-11 | 1.25E+04 |
Std | 0 | 1.27E-12 | 0 | 7.27E-12 | 9.39E+03 | |
f9 | Mean | 0 | 8.04E-12 | 0 | 3.28E-11 | 9.14E+00 |
Std | 0 | 6.77E-13 | 0 | 1.14E-11 | 4.32E+00 | |
f10 | Mean | 8.88E-16 | 5.72E-09 | 8.88E-16 | 1.93E-08 | 1.02E+01 |
Std | 0 | 7.95E-11 | 0 | 1.12E-09 | 8.39E-01 |
Algorithm | parameter settings |
H-BOA | p=pc=0.8,β=0.3,θ=γ=0.1,μ=1,T0=1000 |
IBOA | p=0.8,I=0.01,α=0.1 |
BOA SSA |
p=0.8,I=0.01,α=0.1 ST=0.6,PD=0.7,SD=0.2 |
Parameter settings | value |
Region Area | 100m × 100m |
Pixels | 100 × 100 |
Nodes Perceived Radius |
N = 10, 15, 20, 25, 30 R = 15m |
Algorithm | Number of nodes | Maximum coverage (%) | |||
N = 10 | N = 15 | N = 20 | N = 25 | N = 30 | |
SSA | 62.14 | 78.51 | 83.42 | 92.60 | 94.50 |
H-BOA | 64.61 | 80.05 | 91.21 | 95.78 | 98.34 |
BOA | 56.82 | 71.81 | 82.47 | 90.91 | 94.13 |
IBOA | 56.68 | 74.42 | 83.07 | 91.60 | 94.37 |