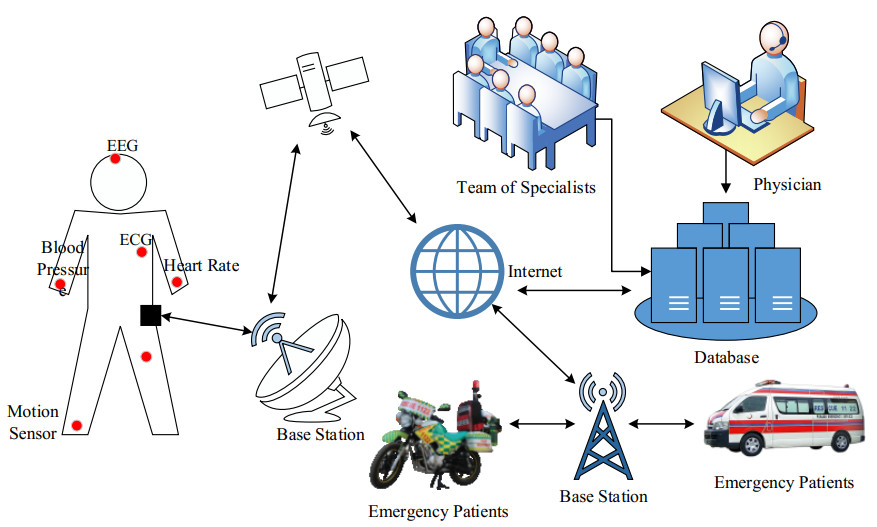
Artificial Intelligence (AI) driven adaptive techniques are viable to optimize the resources in the Internet of Things (IoT) enabled wearable healthcare devices. Due to the miniature size and ability of wireless data transfer, Body Sensor Networks (BSNs) have become the center of attention in current medical media technologies. For a long-term and reliable healthcare system, high energy efficiency, transmission reliability, and longer battery lifetime of wearable sensors devices are required. There is a dire need for empowering sensor-based wearable techniques in BSNs from every aspect i.e., data collection, healthcare monitoring, and diagnosis. The consideration of protocol layers, data routing, and energy optimization strategies improves the efficiency of healthcare delivery. Hence, this work presents some key contributions. Firstly, it proposes a novel avant-garde framework to simultaneously optimize the energy efficiency, battery lifetime, and reliability for smart and connected healthcare. Secondly, in this study, an Adaptive Transmission Data Rate (ATDR) mechanism is proposed, which works on the average constant energy consumption by varying the active time of the sensor node to optimize the energy over the dynamic wireless channel. Moreover, a Self-Adaptive Routing Algorithm (SARA) is developed to adopt a dynamic source routing mechanism with an energy-efficient and shortest possible path, unlike the conventional routing methods. Lastly, real-time datasets are adopted for intensive experimental setup for revealing pervasive and cost-effective healthcare through wearable devices. It is observed and analysed that proposed algorithms outperform in terms of high energy efficiency, better reliability, and longer battery lifetime of portable devices.
Citation: Noman Zahid, Ali Hassan Sodhro, Usman Rauf Kamboh, Ahmed Alkhayyat, Lei Wang. AI-driven adaptive reliable and sustainable approach for internet of things enabled healthcare system[J]. Mathematical Biosciences and Engineering, 2022, 19(4): 3953-3971. doi: 10.3934/mbe.2022182
[1] | Yanxu Zhu, Hong Wen, Jinsong Wu, Runhui Zhao . Online data poisoning attack against edge AI paradigm for IoT-enabled smart city. Mathematical Biosciences and Engineering, 2023, 20(10): 17726-17746. doi: 10.3934/mbe.2023788 |
[2] | Nawaz Ali Zardari, Razali Ngah, Omar Hayat, Ali Hassan Sodhro . Adaptive mobility-aware and reliable routing protocols for healthcare vehicular network. Mathematical Biosciences and Engineering, 2022, 19(7): 7156-7177. doi: 10.3934/mbe.2022338 |
[3] | Roman Gumzej, Bojan Rosi . Open interoperability model for Society 5.0's infrastructure and services. Mathematical Biosciences and Engineering, 2023, 20(9): 17096-17115. doi: 10.3934/mbe.2023762 |
[4] | Ridha Ouni, Kashif Saleem . Secure smart home architecture for ambient-assisted living using a multimedia Internet of Things based system in smart cities. Mathematical Biosciences and Engineering, 2024, 21(3): 3473-3497. doi: 10.3934/mbe.2024153 |
[5] | Abdullah Lakhan, Mazhar Ali Dootio, Ali Hassan Sodhro, Sandeep Pirbhulal, Tor Morten Groenli, Muhammad Saddam Khokhar, Lei Wang . Cost-efficient service selection and execution and blockchain-enabled serverless network for internet of medical things. Mathematical Biosciences and Engineering, 2021, 18(6): 7344-7362. doi: 10.3934/mbe.2021363 |
[6] | Muhammad Zia Ur Rahman, Ali Hassan Raza, Abeer Abdulaziz AlSanad, Muhammad Azeem Akbar, Rabia Liaquat, Muhammad Tanveer Riaz, Lulwah AlSuwaidan, Halah Abdulaziz Al-Alshaikh, Hatoon S Alsagri . Real-time artificial intelligence based health monitoring, diagnosing and environmental control system for COVID-19 patients. Mathematical Biosciences and Engineering, 2022, 19(8): 7586-7605. doi: 10.3934/mbe.2022357 |
[7] | Ching-Chun Chang, Chang-Tsun Li . Algebraic secret sharing using privacy homomorphisms for IoT-based healthcare systems. Mathematical Biosciences and Engineering, 2019, 16(5): 3367-3381. doi: 10.3934/mbe.2019168 |
[8] | Faten S. Alamri, Khalid Haseeb, Tanzila Saba, Jaime Lloret, Jose M. Jimenez . Multimedia IoT-surveillance optimization model using mobile-edge authentic computing. Mathematical Biosciences and Engineering, 2023, 20(11): 19174-19190. doi: 10.3934/mbe.2023847 |
[9] | Gaurav Tripathi, Kuldeep Singh, Dinesh Kumar Vishwakarma . Applied convolutional neural network framework for tagging healthcare systems in crowd protest environment. Mathematical Biosciences and Engineering, 2021, 18(6): 8727-8757. doi: 10.3934/mbe.2021431 |
[10] | Anichur Rahman, Muaz Rahman, Dipanjali Kundu, Md Razaul Karim, Shahab S. Band, Mehdi Sookhak . Study on IoT for SARS-CoV-2 with healthcare: present and future perspective. Mathematical Biosciences and Engineering, 2021, 18(6): 9697-9726. doi: 10.3934/mbe.2021475 |
Artificial Intelligence (AI) driven adaptive techniques are viable to optimize the resources in the Internet of Things (IoT) enabled wearable healthcare devices. Due to the miniature size and ability of wireless data transfer, Body Sensor Networks (BSNs) have become the center of attention in current medical media technologies. For a long-term and reliable healthcare system, high energy efficiency, transmission reliability, and longer battery lifetime of wearable sensors devices are required. There is a dire need for empowering sensor-based wearable techniques in BSNs from every aspect i.e., data collection, healthcare monitoring, and diagnosis. The consideration of protocol layers, data routing, and energy optimization strategies improves the efficiency of healthcare delivery. Hence, this work presents some key contributions. Firstly, it proposes a novel avant-garde framework to simultaneously optimize the energy efficiency, battery lifetime, and reliability for smart and connected healthcare. Secondly, in this study, an Adaptive Transmission Data Rate (ATDR) mechanism is proposed, which works on the average constant energy consumption by varying the active time of the sensor node to optimize the energy over the dynamic wireless channel. Moreover, a Self-Adaptive Routing Algorithm (SARA) is developed to adopt a dynamic source routing mechanism with an energy-efficient and shortest possible path, unlike the conventional routing methods. Lastly, real-time datasets are adopted for intensive experimental setup for revealing pervasive and cost-effective healthcare through wearable devices. It is observed and analysed that proposed algorithms outperform in terms of high energy efficiency, better reliability, and longer battery lifetime of portable devices.
Abbreviations: BSNs: Body Sensor Networks; IoT: Internet of Things; AI: Artificial Intelligence; SARA: Self-Adaptive Routing Algorithm; ATDR: Adaptive Transmission Data Rate; IoMT: Internet of Medical Things; QoS: Quality of Service; TPC: Transmission Power Control; V2V: Vehicle-to-Vehicle; WSN: Wireless Sensor Network; PLR: Packet Loss Ratio; TP: Transmission Power; RSSI: Received Signal Strength Indicator; NIB: Network In Box; QoE: Quality of Experience; AEOA: Adaptive Energy Optimization Algorithm; NSGA: Nondominated Sorting Genetic Algorithm; CPS: Cyber Physical Systems; RF: Random Forest; DSR: Dynamic Source Routing; RREQ: Route Request; RREP: Route Reply
AI-based intelligent IoT devices have decision making power and this unique feature has entirely changed the landscape of the healthcare system. Modern research brings a revolution in wireless communication and sensor devices by developing miniature-sized wearable and implanted sensors. This took the research towards the development of network architecture, addressed as Body Sensor Network (BSN). Sensor networks are the most promising technology for pervasive health care systems in near future due to their high efficiency and great potential in health. The main role of BSNs in pervasive healthcare is to provide non-invasive, cost-effective, and anytime health care facilities over long-distance remote areas. The BSNs also facilitate the old age peoples at home and continuously monitor their health condition.
In the recent research trends, the BSNs are inspired by the patients, soldiers, astronauts' preferences to carry tiny size portable devices as wearable with the bodies to get vital sign signals for monitoring the health conditions, location, and body nutrients. The major weakness to implementing sensor nodes is the consumption of much higher energy with limited battery life. Energy is the most serious problem among all ranges of portable wearable devices. For a longer and continuous data collection & transmission process, the energy will not be sufficient and helpful for the physicians to monitor the patients 24/7 due to a shorter battery lifetime. Henceforth, obtaining the longer battery life with acceptable reliability and high energy efficiency in connected and pervasive healthcare are the key threats to be tackled smartly. Moreover, due to emerging trends in wearable devices, it is critical to managing with their battery lifetime, energy efficiency, and charge drain for achieving pervasive and connected healthcare. According to the study, the transmitter node consumes more than 50% of the power, so, most of the battery charge is consumed during data transmission as compared to the sensing, processing, and receiver nodes. Optimizing the battery lifetime on Internet of Medical Things (IoMT) devices is vital for emergency patients at remote locations [1].
Figure 1 shows the proposed smart and connected health architecture allowing physicians to access the patient remotely. On-body sensing nodes transfer data wirelessly to the data centers from which doctors may monitor the patient health status and analyze the condition with the prior recorded data. It creates an evident and prominent path for the specialists and physicians that they can access and diagnose the critical condition patients by removing the prior hurdles. The battery lifetime of nodes, transmission energy consumption, and changes in the body motion and postures in a highly dynamic environment create a huge challenge in the BSNs. Moreover, the latest battery technology does not assure longtime battery-driven BSN nodes. In the near future due to the slow-going progress in the improvement of batteries and replacement of batteries is not a simple and sufficient task in most cases like implanted sensor nodes.
The main aim of this research is to develop green and battery-friendly data transmission technical solutions to minimize energy consumption and prolong the battery lifetime of BSNs.
The four novel key contributions are made;
● First, this paper proposes a novel avant-garde framework to simultaneously optimize the energy efficiency, battery lifetime, and reliability for smart and connected healthcare.
● Second, an Adaptive Transmission Data Rate (ATDR) mechanism is proposed, which works on the average constant energy consumption by varying the active-time of sensor nodes to optimize the energy over dynamic wireless channels.
● Third, a Self-Adaptive Routing Algorithm (SARA) is developed, basic idea behind this approach is to adopt a dynamic source routing mechanism with an energy-efficient and shortest possible path, unlike the conventional routing methods.
● Forth, Real-time data sets are used for the setup of extensive theoretical and experimental testbeds using Monte Carlo simulation in MATLAB.
The remaining of the paper is organized as Section 2 extensively sheds light on the related research. The novel adaptive, intelligent, and reliable approaches are proposed in Section 3. Section 4, develops the experimental test-bed with detailed results and presents detailed evaluation and analysis. Finally, the paper is concluded in Section 5.
This section describes the existing work having different techniques for Transmission Power Control (TPC) and energy efficiency in BSNs. A lot of researchers have already worked on TPC and routing techniques to fulfill the requirements of BSNs in terms of Quality of Service (QoS), reliability, and battery lifetime. The required QoS may be achieved in a variety of BSN applications by adopting a hybrid design approach to increase the system's performance. The use of a hybrid architecture can improve network efficiency while also lowering energy usage and increasing throughput.
Authors in [2] proposed a cross-layer routing method to enhance QoS in terms of energy consumption, packet delivery, throughput, and network lifetime. This study firstly suggested an optimal routing policy at the network layer for energy-efficient and reliable link; secondly, to enhance the QoS adjusts the contention widow size at the data link layer. But it takes into count the number of nodes in-network and their distances. Researchers in [3] proposed a cross-layer design-based solution that optimizes energy efficiency, transmission reliability, and lifetime of BSNs from several layers simultaneously. This method chooses the relay node to ensure the balance of network energy consumption, and all nodes transmit with the same packet size and transmission power. A synthesized hybrid method to handle the unbalanced energy consumption due to the different locations of the nodes has been proposed. Moreover, to enhance the residual energy of nodes considering the different links in the network suitable packet size is determined. But this approach only works well with already defined relay nodes, can't choose the relay node on run time, and also no policy for packet loss. In [4] researchers designed a mathematical model and jointly optimized network considering relay nodes location and cross-layer.
Authors in [5] developed an algorithm for video transmission in vehicular networks for empowering future IoT driven intelligent transportation system. This algorithm optimizes QoS by novel green, sustainable, reliable, and available framework to improve the performance of vehicle-to-vehicle (V2V) networks during multimedia transmission. Application of pervasive healthcare has been considered to validate the performance of the proposed algorithm and used real-time healthcare data sets to compare the performance of conventional and proposed methods. Authors in [6,7] propose three different techniques for the edge computing-based industrial applications that work in three different ways; a first central dynamic approach which efficiently processes the sensing and transmission process in IoT based portable devices; second novel battery model which evaluates the energy dissipation in IoT devices; third data reliability model for AI-based IoT devices. Hongan Li et al. [8] proposed a neural network-based image reconstruction method for data-efficient and secure transmission in an IoT environment. Data secure transmission has been considered in this research and the transmission energy problem remained unsolved. These schemes save more energy, give less packet loss ratio (PLR) and also fulfil the main requirements of the system. [9,10] provided a technique for reducing the overall energy consumption of the wireless sensor network (WSN) using MAC, Network, and Physical layer-based cross-layer optimization strategy. The impact of varying modulation levels and transmission time on energy usage was examined for WSN due to known distance value between nodes, size of data packets and routing approaches.
Authors in [11,12] developed a unique algorithm for effectively managing energy in BSNs for various body positions, and the entire system was tested on hardware. Researchers in [13,14] proposed four distinct critical methods for energy optimization in medical care. These approaches contribute in many ways, such as identifying energy drain, TP level selection, duty-cycle optimization through feedback control mechanism. In addition, energy harvesting strategies for energy optimization can be a better option [15,16]. Their energy and battery-aware techniques are suitable for body sensors. Researchers in [17,18] developed an energy-efficient routing approach with dynamic features to address the existing problems like link reliability, throughput, nodes energy, and energy-efficient routing path selection. But only the source node can decide the routing path by seeing the table of metrics. To increase reliability and to decrease the cost and energy consumption, wearable devices based human motion monitoring system has been designed in [19]. The module is designed with nine-axis wireless motion considering the walking body posture but the trade-off between reliability and power usage still needs to be improved. The sensor node adaptively adjusts the TP level in this method based on the channel state. It gets Received Signal Strength Indicator (RSSI) input from the base station (BS) and adjusts the TP level for the next transmission to adapt the channel variations for maximum energy savings [20].
Ali Hassan Sodhro et al. [21] propose an AI-based novel framework for the 6G enabled industrial network in box (NIB) system that optimizes energy efficiency, quality of experience (QoE), and Quality of Service (QoS) while taking duty cycle and transmission power into consideration. Authors in [22,23] present machine learning-based methods for the classification and recognition of different body postures (i.e., walking, hand gesture). Blockchain-enabled cost-efficient service selection and execution method used for the distributed networks to minimize the service cost and security risk by neglecting the cost of energy [24]. Authors in [25] presented reliable data transmission methods based on the matrix decomposition and ensemble recovery mechanisms for the WSNs. The proposed methods have a significant impact on data reliability and recovery, however, the impact of data decomposition and transmission on energy has not been examined. Abdullah Lakhan et al. [26] devised an algorithm based on different schemes to minimize the energy consumption of resource-constrained health care devices. However, this study only focused on energy consumption and widely ignored reliability/data transmission. While authors in [27] propose a novel scheme to deal with the latency and to fairly allocate the workload in mobile sensors cloud networks. Laizhong Cui et al. [28] proposed a novel coding scheme by integrating the coding schemes and genetic operations with a nondominated sorting genetic algorithm (NSGA-II) to optimize energy efficiency and latency for various IoT applications while considering user demands. The integration of the Physical and MAC layers allowed this system to fine-tune the duty cycle, modulation level, and transmission power. This method, however, is not suitable for tiny sensing devices due to their small battery size and energy-intensive transmission. Celestine Iwendi et al. [29] proposed a machine learning support system combined Random Forest (RF) and a genetic algorithm for developing a novel intrusion detection approach that optimizes detection rate, accuracy, and false alarm rate. This research paper illustrates how to overcome the security issues in smart healthcare. The authors, on the other hand, did not take into account energy depletion or examine the impact of the proposed approaches on energy consumption.
To manage the transmission power of sensing nodes, the authors of [30] devised a Machine Learning driven regression algorithm. Since channel temporal fluctuations have a direct impact on reliability and transmission power, this approach outperforms in predicting channel dynamics. The issue is that it compromises data reliability, which is critical in healthcare. The authors of [31] offer a joint framework for IoT-driven cyber physical systems (CPS) and include a fuzzy-based intelligent decision-making approach that considers convergence and interoperability. In addition, designed a hardware platform with an analogue front end (AFE) chip model to gather electrocardiogram (ECG) data from patients. This article's main focus is on reliability, convergence, and interoperability, while energy losses have been ignored. Ahmed Alkhayyat et al. [32] provide an energy-efficient approach for transmitting images over the WSN that takes into account the image quality and intensity but ignores network delay. An energy-efficient transmission solution for wearable sensing devices has been proposed [33], but QoS and reliability are ignored which is crucial in healthcare applications. Authors in [34] analyze the performance and determine the total power required for end-to-end communication in WSNs considering the various transmission modes. Ali Hassan Sodhro et al. [35] provide energy-efficient transmission power control techniques to optimize energy depletion while simultaneously increasing battery lifetime and throughput. Research article [36] discusses the significance of sensing devices in remote healthcare applications such as remote diagnostics, telemedicine, and chronic heart disease monitoring. Authors in [37] present a thorough review of power management strategies used in healthcare applications. Moreover, article [38] analyses and compares the data rate control and transmission power control methods to illustrate how they affect energy efficiency.
The examined review reveals that most of the researchers explored various techniques and algorithms for healthcare, but very few have focused on the data rate aware intelligent energy optimization techniques. The proposed algorithms in this research article simultaneously focus on the data transmission rate and energy optimization.
Two distinct algorithms for energy-efficient, battery-aware, and ubiquitous healthcare are presented in this section. To avoid any risk, these algorithms enable ubiquitous and reliable communication and continually check the node's remaining energy.
In BSNs, we can take advantage of channel dynamics due to its large temporal variations. This algorithm keeps track of the overall energy drain until the completion of the task. The main concept behind the proposed ATDR is that it works on the average constant energy consumption by varying the active-time of a sensor node to optimize the energy over a dynamic wireless channel, while for channel estimation and calculation of dynamics, an AI-driven regression method is used, which intelligently predicts the channel dynamics for the next time slot (for more details please see [30]). Besides, the proposed ATDR works very well if the channel is changing with large temporal variations. It optimizes the active time of the node when the transmission power of the sensor node goes towards the peak and requests the transport layer to adjust the packet size based on active time. While remaining data bits will be stored in the buffer for the next transmission with a large time slot. Figure 2 shows how different layers interact and shares information to carry out ATDR.
Proposed Adaptive Transmission Data Rate |
Input: RSSI, DC, Tact Output: Energy Optimization (EO) If RSSI showing large variations Select average TP Calculate Eavg_const Select appropriate TP level Tact=Eavg_const−(Pslp.Tslp+Ptran.Ttran)(Pe+Pa) W=Tact×Rs×b DC=TactTtotal end |
Here, we have found the average energy consumption from the extensive data sets to accomplish the data streams with minimum bit loss and to avoid the buffer overflow. TP level and active time are inversely related. An increase in the former decreases the latter which leads to constant average energy drain as shown in Eqs (1) and (3).
Eavg_const−(Pslp.Tslp+Ptran.Ttran)(Pe+Pa)=Tact | (1) |
While,
Eavg_const=(Pe+Pa)Tact+(Pslp.Tslp+Ptran.Ttran) | (2) |
Tact≥min(numberofbits)Rs.Rc.b | (3) |
In this algorithm Network, Transport, MAC and Physical layers share the information to optimize the transmission energy and to attain the better QoS with minimum data loss. Node senses the channel condition through the TP and RSSI levels, if TP is greater than the -15 dBm and RSSI is less than the target value, then data will be transmitted through the path followed by relay nodes. Proposed SARA works without routing table and updated routing information. The basic idea behind the proposed SARA is to adopt a dynamic source routing (DSR) strategy with an energy-efficient and shortest possible path, unlike the conventional routing methods. Proposed SARA explores the path from source to destination by broadcasting the initial packet containing the information about source and destination. For the suitable and energy-efficient routing path selection following parameters have been considered for four entities i.e., residual energy of relay node, acceptable RSSI, minimum transmission power, and shortest path from source to sink node.
The alluring speciality of SARA is that it operates without any external intervention and doesn't demand any prior connectivity with the previous networks, because its self-adaptive and dynamic features make it is easy to integrate. It is a reactive and sympathetic algorithm and from all aspects, this algorithm runs on-demand basis with the concept of DSR. In source routing sender sends a packet and determines the routing path by the complete sequence of the intermediate nodes through which the packet is forwarded. The distribution of sensor nodes with a proper network is depicted in Figure 3.
When any source node takes the decision of routing through SARA, it relays on two Route Request (RREQ) and Route Reply (RREP) packets. First of all, to find out any routing path of the destination, the transmitter source node checks its route cache and if it hasn't found any information then broadcasts the packet of RREQ. The RREQ packet will be received by all the neighbour nodes who are in the wireless range of the transmitter sensor node and this flow carry on in order to dynamically discover a new route. The RREQ packet contains; First, a unique ID to avoid duplicate reception, as if any node receives multiple copies of the same packet then this node will discard them. Second, a list of nodes that contains the intermediate nodes information. Third, Source node information, and the fourth one is the information about the destination. The main advantage of our proposed SARA is that if the second last and last node, both are approaching the BS then the BS will choose the node from which it has received first on the basis of RSSI. Thus, according to this method, the selection of routing will be contained on the shortest path with minimum nodes. SARA is also helpful in energy saving as it chooses the suitable and shortest path and does not select some intermediate node if the next node is receiving the acceptable RSSI from the previous node.
Proposed Self-Adaptive Routing Algorithm |
Input: TP, Packet loss (Pl), Packet loss threshold (Pl-thr), Residual Energy (RE), TP Supper (TPsup) Output: Energy-efficient Route If Pl < = Pl-thr & & TP > = TPth Generate RREQ TP = TPmin →Relay Node If RE & RSSI = Target Add location & Broadcast elseif it is destination RREP else No action end →Source Node If Receives back RREQ Discard else TP = TPmin + 1 If TP < = TPsup Continue Direct transmission else Re-Broadcast RREQ end end end |
Figure 4 shows that the source node broadcasts the first packet with minimum TP and then waits for the reply of the same packet with relay/intermediate node ID, if it does not receive then re-broadcast the RREQ packet with one step higher TP and perform this task continuously by the adjustment of TP until the reception of RREQ from another node. First of all, the intermediate node checks RSSI and its residual energy if both are acceptable then it broadcasts the RREQ packet with its ID otherwise doesn't take any action. If an intermediate node has enough residual energy with acceptable RSSI then it inspects whether it is the destination node or not, if yes then it will transmit its location via RREP otherwise nodes endure the process of RREQ broadcasting with the insertion of ID.
Before the rebroadcasting of the RREQ packet, each node maintains a route cache and also confirms the routing information from the cache. The crucial advantage of maintaining a route cache is that it reduces route discovery generated overhead and also if any intermediate node has the routing path of the destination in its cache then it will generate an RREP packet towards the source node instead of RREQ. The destination node or BS generates the RREP packet which has the route information to the BS and sends it to the transmitter sensor node. The transmitter sensor node saves the routing path information in its cache. The route obtained by the SARA is considered the shortest routing path as if the two nodes having different distances are in the range of the transmitter sensor node then both will be received the RREQ packet. So, when the RREQ will be rebroadcast by the node having a small distance then the node at a large distance will cancel it as it has already the RREQ packet with the same ID. And if any node breaks the route, it notifies the source node about this and the source node will remove the routing information from its cache.
This section intensively establishes the experimental setup with specific performance metrics for instance, packet loss ratio (PLR), the energy consumption of routing path, transmitter node energy consumption, and standard deviation (std. dev). Second, presents a detailed discussion on the results acquired by MATLAB and a comparison with the conventional methods.
This research has been carried out by considering body posture i.e., walking, and two scenarios such as 'Right Wrist to Chest' and 'Right Ankle to Chest', and information-rich datasets [39][BZM1]. It is supposed that the walking speed of the old-aged patients 2.5 km/h, is the reasonable entity to analyze the reliability in terms of RSSI at the base station.
To calculate the total energy consumption on routing path for the completion of one task the following equation is used in which Etotal_task is the total energy consumed to complete one task;
Etotaltask=∑pj=1Ej,j=1,2,3,…,p(pnumberofpackets) | (4) |
First, the channel is classified and examined in bad and good on the basis of packet loss ratio (PLR) and energy drain by theoretical approaches then carefully choosing the different tunable parameters to carry out this research. Adjustment of data rate and active time depend on the energy-constrained Eqs (1) and (2). This empowers a proposed ATDR to adaptively adjust according to the dynamic features of the wireless channel to mitigate packet loss and energy consumption. Moreover, to enhance the alive time of the node, and to avoid further losses, the transmitter sensor node continuously inspects PLR seeing the TP level and RSSI respectively, and takes the decision about routing. The beauty of the proposed SARA is that it can find the routing path without any previous data.
The performance of the entire testbed is evaluated and theoretically analyzed for proposed algorithms and Baseline adaptive power control (APC) through Monte Carlo simulations in MATLAB. The channel is categorized in good and poor states as a result of walking body posture. High interworking of layers, battery power management, interoperable, and TP traits improves the performance of the BSN. A hybrid approach is important for dynamic channel-based BSNs operating in a diverse environment.
The proposed hybrid approach gives a strong relationship between data rate (Kbps), channel dynamics, and energy dissipation (mJ) in comparison to conventional methods. The above-mentioned graphs illustrate the experimental results of the proposed adaptive transmission data rate mechanism (ATDR) and conventional APC method. Figure 5(a), (b) presents that with the varying channel, the proposed ATDR mechanism adaptively adjusts transmission data rate and carried out channel dynamics for its advantage. As it transmits more data whenever finds the better channel and lesser in bad channel conditions by looking at the RSSI and tries to overcome the energy consumption at the transmitter. During this transport layer controls data rate while MAC and PHY layers control active time and TP/modulation level accordingly. The results indicate that the node's overall energy consumption remains constant while the active time and data rate are varied. As a result, the proposed approach is suitable in situations where the channel's temporal variations are high.
Figure 6 draws a connection between the transmitted data bits and varying channel over time. It can be seen clearly that at the best channel it transmits more data with less TP, while at the bad channel it transmits fewer data and saves energy by the adjustment of duty-cycle and TP. Furthermore, as compared to conventional approaches, the proposed scheme enables the sensing node to transmit more data.
Figure 7 illustrates that the proposed method delivered more data bits with less PLR and reduced energy usage. It is interpreted that energy can be saved by taking advantage of channel fluctuations. Moreover, fewer data bits have been lost by the proposed method because it carefully chose the data rate and carried out data transmission considering the good and bad channels.
Experimental results are showing that the proposed ATDR saves more energy with more data bits transmission and gives less PLR than the APC algorithm. Figure 8 illustrates the TP and RSSI levels of transmitter sensor node for APC and proposed SARA, while continuous fluctuations in TP and RSSI reveal that channel promptly varies between good and bad channel. Figure 8(a), (c) depicts the adaptive adjustment of TP level in response to channel variations, indicating that the transmitter sensor node makes a routing decision after a few transmissions due to continuous packet loss, despite the fact that TP is also high. It can be seen clearly that the proposed SARA takes self-decision about routing and determines the optimum routing path to control the PLR and reduce energy consumption at the transmitter side. For experimental setup, two experimental scenarios have been considered, 'Right Wrist to Chest', and 'Right Ankle to Chest' for walking body postures with real-time data sets. Figure 8(b), (d) depicts RSSI values associated with specific TP levels, which demonstrates that BS receives the best RSSI after the routing. It can be examined that after the continuous monitoring of RSSI/PLR and TP, the proposed SARA finds out the best routing path and carry out the next transmissions at the newly selected path with minimum TP.
Figure 9 presents the energy consumption per packet at the transmitter in both scenarios of the body and gives a clear comparison between the proposed SARA and conventional APC. Figure 9 illustrates the transmission energy consumption by the proposed SARA after the selection of the best routing path and reveals that the proposed method consumes less power at the transmitter than the APC. Furthermore, the proposed SARA gives less PLR as compared to the PLR of the APC. The experimental results show that the proposed routing scheme outperforms as it adaptively finds the routing path and saves more energy for the transmitter sensor node with a minimum packet loss ratio. The limitation of the routing algorithm is that the total energy consumption of the path is increased.
This research has been focused on the green and battery-friendly communication solutions in BSNs, by taking into account the tradeoff between the energy-saving, channel reliability, and battery lifetime extension during the transmission of vital sign signals as an incredible option for creating autonomous, self-sufficient, and cost-effective medical healthcare platform for patients. Thus, in this context, green, reliable, and battery-friendly communication solutions are proposed by our research which can build reliable and sustainable BSNs. The alluring functionality of our proposed system is that it can take self-decision about routing or can shift on the hop topology and find the best energy-efficient, low routing cost, and shortest path. As a result of the research in this area, the two innovative approaches are developed, i) Proposed Adaptive Transmission Data Rate (ATDR) algorithm, which consumes the same energy in every transmission by the adjustment of the active time and packet size and exploits the dynamic nature of the channel. ii) Proposed Self Adaptive Routing Algorithm (SARA), which takes the self-decision for routing to overcome the energy transmission consumption and to enhance the reliability. Theoretical analysis and the experimental results of Monte Carlo simulation in MATLAB show that the proposed algorithms perform better in terms of energy efficiency, reliability and enhance the lifetime of BSN. In near future, we shall focus on the intelligent AI-based techniques for IoT-5G pervasive healthcare applications.
Highlights of the manuscripts
● A novel avant-garde framework to simultaneously optimize the energy efficiency, battery lifetime, and reliability for smart and connected healthcare.
● Adaptive Transmission Data Rate (ATDR) algorithm is proposed, which consumes the same energy in every transmission by the adjustment of the active time and packet size and exploits the dynamic nature of the channel.
● Self-Adaptive Routing Algorithm (SARA) is proposed.
This work is funded by Research grant of PIFI 2020 (2020VBC0002), China.
There is no conflict of interest between all authors.
[1] |
Y. Gu, C. Budati, Energy-aware workflow scheduling and optimization in clouds using bat algorithm, Future Gener. Comput. Syst., 113 (2020), 106–112. https://doi.org/10.1016/j.future.2020.06.031 doi: 10.1016/j.future.2020.06.031
![]() |
[2] |
L. Mishra, S. Varma, Performance evaluation of real-time stream processing systems for Internet of Things applications, Future Gener. Comput. Syst., 113 (2020), 207–217. https://doi.org/10.1016/j.future.2020.07.012 doi: 10.1016/j.future.2020.07.012
![]() |
[3] |
X. Chen, Y. Xu, A. Liu, Cross layer design for optimizing transmission reliability, energy efficiency, and lifetime in body sensor networks, Sensors, 17 (2017), 900. https://doi.org/10.3390/s17040900 doi: 10.3390/s17040900
![]() |
[4] |
S. L. Chen, M. C. Tuan, H. Y. Lee, T. L. Lin, VLSI implementation of a cost-efficient micro control unit with an asymmetric encryption for wireless body sensor networks, IEEE Access, 5 (2017), 4077–4086. https://doi.org/10.1109/ACCESS.2017.2679123 doi: 10.1109/ACCESS.2017.2679123
![]() |
[5] |
A. H. Sodhro, M. S. Obaidat, Q. H. Abbasi, P. Pace, S. Pirbhulal, G. Fortino, et al., Quality of service optimization in an IoT-driven intelligent transportation system, IEEE Wireless Commun., 26 (2019), 10–17. https://doi.org/10.1109/MWC.001.1900085 doi: 10.1109/MWC.001.1900085
![]() |
[6] |
K. G. Mkongwa, Q. Liu, C. Zhang, Link reliability and performance optimization in wireless body area networks, IEEE Access, 7 (2019), 155392–155404. https://doi.org/10.1109/ACCESS.2019.2944573 doi: 10.1109/ACCESS.2019.2944573
![]() |
[7] |
A. H. Sodhro, S. Pirbhulal, V. H. C. De Albuquerque, Artificial intelligence-driven mechanism for edge computing-based industrial applications, IEEE Trans. Ind. Inf., 15 (2019), 4235–4243. https://doi.org/10.1109/TII.2019.2902878 doi: 10.1109/TII.2019.2902878
![]() |
[8] |
H. Li, Q. Zheng, W. Yan, R. Tao, X. Qi, Z. Wen, Image super-resolution reconstruction for secure data transmission in Internet of Things environment, Math. Biosci. Eng., 18 (2021), 6652–6671. https://doi.org/10.3934/mbe.2021330 doi: 10.3934/mbe.2021330
![]() |
[9] |
K. Babber, R. Randhawa, A cross-layer optimization framework for energy efficiency in wireless sensor networks, Wireless Sensor Network, 9 (2017), 189. https://doi.org/10.4236/wsn.2017.96011 doi: 10.4236/wsn.2017.96011
![]() |
[10] |
A. H. Sodhro, S. Pirbhulal, M. Qaraqe, S. Lohano, G. H. Sodhro, N. Ur R. Junejo, et al., Power control algorithms for media transmission in remote healthcare systems, IEEE Access, 6 (2018), 42384–42393. https://doi.org/10.1109/ACCESS.2018.2859205 doi: 10.1109/ACCESS.2018.2859205
![]() |
[11] |
Y. Zhou, Z. Sheng, C. Mahapatra, V. CM Leung, P. Servati, Topology design and cross-layer optimization for wireless body sensor networks, Ad Hoc Networks, 59 (2017), 48–62. https://doi.org/10.1016/j.adhoc.2017.01.005 doi: 10.1016/j.adhoc.2017.01.005
![]() |
[12] |
A. R. Bhangwar, A. Ahmed, U. A. Khan, T. Saba, K. Almustafa, K. Haseeb, et al., WETRP: Weight based energy & temperature aware routing protocol for wireless body sensor networks, IEEE Access, 7 (2019), 87987–87995. https://doi.org/10.1109/ACCESS.2019.2925741 doi: 10.1109/ACCESS.2019.2925741
![]() |
[13] |
I. Saidu, S. Subramaniam, A. Jaafar, Z. A. Zukarnain, An efficient battery lifetime aware power saving (EBLAPS) mechanism in IEEE 802.16 e networks, Wireless Pers. Commun., 80 (2015), 29–49. https://doi.org/10.1007/s11277-014-1993-7 doi: 10.1007/s11277-014-1993-7
![]() |
[14] |
R. Gravina, P. Alinia, H. Ghasemzadeh, G. Fortino, Multi-sensor fusion in body sensor networks: State-of-the-art and research challenges, Inf. Fusion, 35 (2017), 68–80. https://doi.org/10.1016/j.inffus.2016.09.005 doi: 10.1016/j.inffus.2016.09.005
![]() |
[15] | A. Sangwan, P. P. Bhattacharya, An optimization to routing approach under WBAN architectural constraints, In Intelligent Systems Technologies and Applications, Springer, Cham, (2016), 75–89. |
[16] |
H. Karvonen, J. Iinatti, M. Hämäläinen, A cross-layer energy efficiency optimization model for WBAN using IR-UWB transceivers, Telecommun. Syst., 58 (2015), 165–177. https://doi.org/10.1007/s11235-014-9900-9 doi: 10.1007/s11235-014-9900-9
![]() |
[17] |
R. Talat, M. S. Obaidat, M. Muzammal, A. H. Sodhro, Z. Luo, S. Pirbhulal, A decentralised approach to privacy preserving trajectory mining, Future Gener. Comput. Syst., 102 (2020), 382–392. https://doi.org/10.1016/j.future.2019.07.068 doi: 10.1016/j.future.2019.07.068
![]() |
[18] |
A. H. Sodhro, S. Pirbhulal, A. Sangaiah, Convergence of IoT and product lifecycle management in medical health care, Future Gener. Comput. Syst., 86 (2018), 380–391. https://doi.org/10.1016/j.future.2018.03.052 doi: 10.1016/j.future.2018.03.052
![]() |
[19] |
J. Zhao, G. Li, Study on real-time wearable sport health device based on body sensor networks, Comput. Commun., 154 (2020), 40–47. https://doi.org/10.1016/j.comcom.2020.02.045 doi: 10.1016/j.comcom.2020.02.045
![]() |
[20] |
Y. Lin, X. Jin, J. Chen, A. H. Sodhro, Z. Pan, An analytic computation-driven algorithm for Decentralized Multicore Systems, Future Gener. Comput. Syst., 96 (2019), 101–110. https://doi.org/10.1016/j.future.2019.01.031 doi: 10.1016/j.future.2019.01.031
![]() |
[21] |
A. H. Sodhro, N. Zahid, L. Wang, S. Pirbhulal, Y. Ouzrout, A. Sekhari, et al., Towards ML-based Energy-Efficient Mechanism for 6G Enabled Industrial Network in Box Systems, IEEE Trans. Ind. Inf., 17 (2020). https://doi.org/10.1109/TII.2020.3026663 doi: 10.1109/TII.2020.3026663
![]() |
[22] |
W. Jiang, X. Ye, R. Chen, F. Su, M. Lin, Y. Ma, et al., Wearable on-device deep learning system for hand gesture recognition based on FPGA accelerator, Math. Biosci. Eng., 18 (2021), 132–153. https://doi.org/10.3934/mbe.2021007 doi: 10.3934/mbe.2021007
![]() |
[23] |
W. Aziz, L. Hussain, I. R. Khan, J. S. Alowibdi, M. H. Alkinani, Machine learning based classification of normal, slow and fast walking by extracting multimodal features from stride interval time series, Math. Biosci. Eng., 18 (2021), 495–517. https://doi.org/10.3934/mbe.2021027 doi: 10.3934/mbe.2021027
![]() |
[24] |
A. Lakhan, M. A. Dootio, A. H. Sodhro, S. Pirbhulal, T. M. Groenli, M. S. Khokhar, et al., Cost-efficient service selection and execution and blockchain-enabled serverless network for internet of medical things, Math. Biosci. Eng., 18(2021), 7344–7362. https://doi.org/10.3934/mbe.2021363 doi: 10.3934/mbe.2021363
![]() |
[25] |
F. Li, G. Zhou, J. Lei, Reliable data transmission in wireless sensor networks with data decomposition and ensemble recovery, Math. Biosci. Eng., 16 (2019), 4526–4545. https://doi.org/10.3934/mbe.2019226 doi: 10.3934/mbe.2019226
![]() |
[26] |
A. Lakhan, J. Li, T. M. Groenli, A. H. Sodhro, N. A. Zardari, A. S. Imran, et al., Dynamic application partitioning and task-scheduling secure schemes for biosensor healthcare workload in mobile edge cloud, Electronics, 10 (2021), 2797. https://doi.org/10.3390/electronics10222797 doi: 10.3390/electronics10222797
![]() |
[27] |
A. Lakhan, M. A. Dootio, T. M. Groenli, A. H. Sodhro, M. S. Khokhar, Multi-layer latency aware workload assignment of e-transport iot applications in mobile sensors cloudlet cloud networks, Electronics, 10 (2021), 1719. https://doi.org/10.3390/electronics10141719 doi: 10.3390/electronics10141719
![]() |
[28] |
L. Cui, C. Xu, S. Yang, J. Z. Huang, J. Li, X. Wang, et al., Joint optimization of energy consumption and latency in mobile edge computing for Internet of Things, IEEE Internet Things J., 6 (2018), 4791–4803. https://doi.org/10.1109/JIOT.2018.2869226 doi: 10.1109/JIOT.2018.2869226
![]() |
[29] |
C. Iwendi, J. H. Anajemba, C. Biamba, D. Ngabo, Security of things intrusion detection system for smart healthcare, Electronics, 10 (2021), 1375. https://doi.org/10.3390/electronics10121375 doi: 10.3390/electronics10121375
![]() |
[30] | N. Zahid, A. H. Sodhro, R. F. Zafar, B. Zahid, S. A. Khan, F. Akhter, Regression-based transmission power control for green healthcare, in 2019 2nd international conference on computing, mathematics and engineering technologies (iCoMET), IEEE, 2019. https://doi.org/10.1109/ICOMET.2019.8673532 |
[31] |
A. H. Sodhro, N. Zahid, AI-enabled framework for fog computing driven e-healthcare applications, Sensors, 21 (2021), 8039. https://doi.org/10.3390/s21238039 doi: 10.3390/s21238039
![]() |
[32] |
S. T. Abbas, H. J. Mohammed, J. S. Ahmed, A. S. Rashid, B. Alhayani, A. Alkhayyat, The optimization efficient energy cooperative communication image transmission over WSN, Appl. Nanosci., (2021), 1–13. https://doi.org/10.1007/s13204-021-02100-2 doi: 10.1007/s13204-021-02100-2
![]() |
[33] |
A. H. Sodhro, Y. Li, M. A. Shah, Energy-efficient adaptive transmission power control for wireless body area networks, IET Commun., 10 (2016), 81–90. https://doi.org/10.1049/iet-com.2015.0368 doi: 10.1049/iet-com.2015.0368
![]() |
[34] | A. Alkhayyat, S. F. Jawad, S. B. Sadkhan, Cooperative communication based: Efficient power allocation for wireless body area networks, in 2019 1st AL-Noor International Conference for Science and Technology (NICST), IEEE, (2019), 106–111. https://doi.org/10.1109/NICST49484.2019.9043843 |
[35] | A. H. Sodhro, M. S. Al-Rakhami, L. Wang, H. Magsi, N. Zahid, S. Pirbhulal, et al., Decentralized energy efficient model for data transmission in IoT-based healthcare system, in 2021 IEEE 93rd Vehicular Technology Conference (VTC2021-Spring), IEEE, (2021), 1–5. https://doi.org/10.1109/VTC2021-Spring51267.2021.9448886 |
[36] |
J. F. A.Rida, A. Alkhayyat, Remote Health Care based on mobile wireless communication Networks, J. Appl. Sci. Eng., 24 (2021), 799–805. https://doi.org/10.6180/jase.202110_24(5).0016 doi: 10.6180/jase.202110_24(5).0016
![]() |
[37] |
A. H. Sodhro, S. Pirbhulal, G. H. Sodhro, A. Gurtov, M. Muzammal, Z. Luo, A joint transmission power control and duty-cycle approach for smart healthcare system, IEEE Sensors J., 19 (2018), 8479–8486. https://doi.org/10.1109/JSEN.2018.2881611 doi: 10.1109/JSEN.2018.2881611
![]() |
[38] | A. H. Sodhro, L. Chen, A. Sekhari, Y. Ouzrout, W. Wu, Energy efficiency comparison between data rate control and transmission power control algorithms for wireless body sensor networks, Int. J. Distrib. Sensor Networks, 14 (2018), 1550147717750030. https://doi.org/10.1177/1550147717750030 |
[39] | L. Hanlen, V. Chaganti, B. Gilbert, D. Rodda, T. Lamahewa, D. Smith, Open-source testbed for body area networks: 200 sample/sec, 12 hrs continuous measurement, in 2010 IEEE 21st International Symposium on Personal, Indoor and Mobile Radio Communications Workshops, (2010), 66–71. https://doi.org/10.1109/PIMRCW.2010.5670518 |
1. | Noman Zahid, Ahmed Alkhayyat, Muhammad Ismail, Ali Hassan Sodhro, 2022, An Effective Traffic Management Approach For Decentralized BSNs, 978-1-6654-5468-1, 1, 10.1109/VTC2022-Fall57202.2022.10012931 | |
2. | Nawaz Ali Zardari, Razali Ngah, Omar Hayat, Ali Hassan Sodhro, Adaptive mobility-aware and reliable routing protocols for healthcare vehicular network, 2022, 19, 1551-0018, 7156, 10.3934/mbe.2022338 | |
3. | Harsh S. Dhiman, Dipankar Deb, Josep M. Guerrero, On wavelet transform based convolutional neural network and twin support vector regression for wind power ramp event prediction, 2022, 36, 22105379, 100795, 10.1016/j.suscom.2022.100795 | |
4. | Ali Hassan Sodhro, Charlotte Sennersten, Awais Ahmad, Towards Cognitive Authentication for Smart Healthcare Applications, 2022, 22, 1424-8220, 2101, 10.3390/s22062101 | |
5. | Alessandro Leone, Gabriele Rescio, Giovanni Diraco, Andrea Manni, Pietro Siciliano, Andrea Caroppo, Ambient and Wearable Sensor Technologies for Energy Expenditure Quantification of Ageing Adults, 2022, 22, 1424-8220, 4893, 10.3390/s22134893 | |
6. | Barbaros Preveze, Ahmed Alkhayyat, Firas Abedi, Aqeel Mahmood Jawad, Ali S. Abosinnee, Ghanshyam Singh, SDN-Driven Internet of Health Things: A Novel Adaptive Switching Technique for Hospital Healthcare Monitoring System, 2022, 2022, 1530-8677, 1, 10.1155/2022/3150756 | |
7. | Mariusz Nowak, Rafał Różycki, Grzegorz Waligóra, Joanna Szewczyk, Adrian Sobiesierski, Grzegorz Sot, Data Processing with Predictions in LoRaWAN, 2022, 16, 1996-1073, 411, 10.3390/en16010411 | |
8. | Min Su, Pei Li, Xueqin Liu, Dapeng Wei, Jun Yang, Textile-Based Flexible Capacitive Pressure Sensors: A Review, 2022, 12, 2079-4991, 1495, 10.3390/nano12091495 | |
9. | Te-Wei Chiang, Dai-Lun Chiang, Tzer-Shyong Chen, Frank Yeong-Sung Lin, Victor R. L. Shen, Min-Chien Wang, Novel Lagrange interpolation polynomials for dynamic access control in a healthcare cloud system, 2022, 19, 1551-0018, 9200, 10.3934/mbe.2022427 | |
10. | Somasundaram Muthuvel, Sivakumar Rajagopal, Shamala K. Subramaniam, Analysis of Security Issues in Wireless Body Area Networks in Heterogeneous Networks, 2022, 22, 1424-8220, 7588, 10.3390/s22197588 | |
11. | Mehmet B. Unver, Governing fiduciary relationships or building up a governance model for trust in AI? Review of healthcare as a socio-technical system, 2023, 1360-0869, 1, 10.1080/13600869.2023.2192569 | |
12. | Nam-Phuong Tran, Thanh Phung Truong, Quang Tuan Do, Nhu-Ngoc Dao, Sungrae Cho, Joint wireless resource allocation and bitrate adaptation for QoE improvement in IRS-aided RSMA-enabled IoMT streaming systems, 2024, 25, 25426605, 101145, 10.1016/j.iot.2024.101145 | |
13. | Marius Minea, Viviana Laetitia Minea, Augustin Semenescu, Smart Preventive Maintenance of Hybrid Networks and IoT Systems Using Software Sensing and Future State Prediction, 2023, 23, 1424-8220, 6012, 10.3390/s23136012 | |
14. | Guolun Zhong, Lei Deng, ACPScanner: Prediction of Anticancer Peptides by Integrated Machine Learning Methodologies, 2024, 1549-9596, 10.1021/acs.jcim.3c01860 | |
15. | Yagmur Yigit, Kubra Duran, Naghmeh Moradpoor, Leandros Maglaras, Nguyen Van Huynh, Berk Canberk, 2024, Chapter 4, 978-981-97-5623-0, 135, 10.1007/978-981-97-5624-7_4 | |
16. | Subasis Mohapatra, Mannava Yesubabu, Amlan Sahoo, Subhadarshini Mohanty, Sachi Nandan Mohanty, IoT-based Ubiquitous Healthcare System with Intelligent Approach to an Epidemic, 2024, 18, 18722121, 10.2174/0118722121240884230926092316 | |
17. | Walid Osamy, Ahmed M. Khedr, Bader Alwasel, Ahmed Salim, DGTTSSA: Data Gathering Technique Based on Trust and Sparrow Search Algorithm for WSNs, 2023, 23, 1424-8220, 5433, 10.3390/s23125433 | |
18. | Mohammed Salem Basingab, Hatim Bukhari, Suhail H. Serbaya, Georgios Fotis, Vasiliki Vita, Stylianos Pappas, Ali Rizwan, AI-Based Decision Support System Optimizing Wireless Sensor Networks for Consumer Electronics in E-Commerce, 2024, 14, 2076-3417, 4960, 10.3390/app14124960 | |
19. | Muhammad Irfan Younas, Muhammad Jawed Iqbal, Abdul Aziz, Ali Hassan Sodhro, Toward QoS Monitoring in IoT Edge Devices Driven Healthcare—A Systematic Literature Review, 2023, 23, 1424-8220, 8885, 10.3390/s23218885 | |
20. | Padma Vijetha Dev. B, K. Venkata Prasad, Revolutionary of secure lightweight energy efficient routing protocol for internet of medical things: a review, 2023, 83, 1573-7721, 37247, 10.1007/s11042-023-17069-z | |
21. | Mario José Diván, Dmitry Shchemelinin, Marcos E. Carranza, Cesar Ignacio Martinez-Spessot, Mikhail Buinevich, Real-Time Reliability Monitoring on Edge Computing: a Systematic Mapping, 2023, 22, 2713-3206, 1243, 10.15622/ia.22.6.1 | |
22. | Bimal Kumar Meher, Ruhul Amin, Mohammad Abdussami, Venkatasamy Sureshkumar, Md Ajaharul Hossain, Efficient Certificateless Anonymous Mutual Authentication in WBANs for Smart Healthcare, 2024, 25, 1524-9050, 17666, 10.1109/TITS.2024.3413321 | |
23. | Jyoti Srivastava, Sidheswar Routray, 2024, Energy Preserving Techniques for CPS in Smart Healthcare System, 979-8-3503-8056-9, 362, 10.1109/SMART63812.2024.10882476 | |
24. | Yu Xing, Zhidong Xia, Wei Zhou, Peng Fu, Chen Zhao, Jia Luo, Fabric Resistive and Capacitive Sensors using Wet-Spinning Wire of Conductive Silicone Rubber, 2025, 09244247, 116426, 10.1016/j.sna.2025.116426 | |
25. | Sujeet Kumar, Sachin Kumar Gupta, Papita Chaudhary, Sumit Kumar, 2024, Real-Time Prediction of Patient Recovery Time using AI-driven Optimization Techniques in Healthcare, 979-8-3503-6472-9, 1111, 10.1109/AECE62803.2024.10911851 |
Proposed Adaptive Transmission Data Rate |
Input: RSSI, DC, Tact Output: Energy Optimization (EO) If RSSI showing large variations Select average TP Calculate Eavg_const Select appropriate TP level Tact=Eavg_const−(Pslp.Tslp+Ptran.Ttran)(Pe+Pa) W=Tact×Rs×b DC=TactTtotal end |
Proposed Self-Adaptive Routing Algorithm |
Input: TP, Packet loss (Pl), Packet loss threshold (Pl-thr), Residual Energy (RE), TP Supper (TPsup) Output: Energy-efficient Route If Pl < = Pl-thr & & TP > = TPth Generate RREQ TP = TPmin →Relay Node If RE & RSSI = Target Add location & Broadcast elseif it is destination RREP else No action end →Source Node If Receives back RREQ Discard else TP = TPmin + 1 If TP < = TPsup Continue Direct transmission else Re-Broadcast RREQ end end end |
Proposed Adaptive Transmission Data Rate |
Input: RSSI, DC, Tact Output: Energy Optimization (EO) If RSSI showing large variations Select average TP Calculate Eavg_const Select appropriate TP level Tact=Eavg_const−(Pslp.Tslp+Ptran.Ttran)(Pe+Pa) W=Tact×Rs×b DC=TactTtotal end |
Proposed Self-Adaptive Routing Algorithm |
Input: TP, Packet loss (Pl), Packet loss threshold (Pl-thr), Residual Energy (RE), TP Supper (TPsup) Output: Energy-efficient Route If Pl < = Pl-thr & & TP > = TPth Generate RREQ TP = TPmin →Relay Node If RE & RSSI = Target Add location & Broadcast elseif it is destination RREP else No action end →Source Node If Receives back RREQ Discard else TP = TPmin + 1 If TP < = TPsup Continue Direct transmission else Re-Broadcast RREQ end end end |