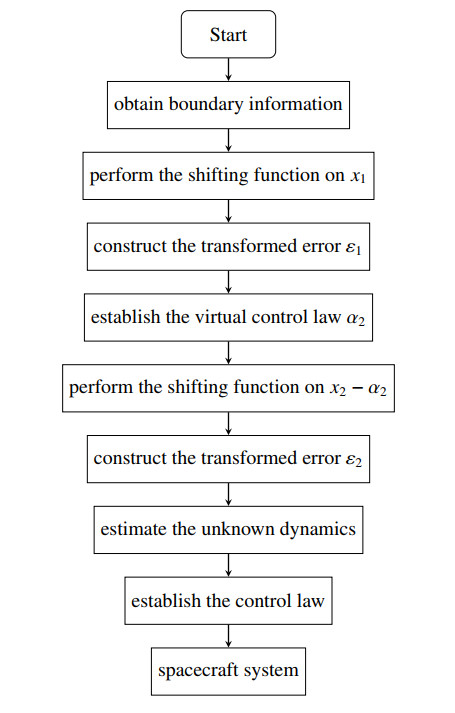
The high-accuracy attitude maneuvering problem for spacecraft systems is investigated. A prescribed performance function and a shifting function are first employed to ensure the predefined-time stability of attitude errors and eliminate the constraints on tracking errors at the incipient stage. Subsequently, a novel predefined-time control scheme is developed by combining prescribed performance control and backstepping control procedures. Radial basis function neural network and minimum learning parameter techniques are introduced to model the function of lumped uncertainty including inertial uncertainties, actuator faults and virtual control law derivatives. According to the rigorous stability analysis, the preset tracking precision can be achieved within a predefined time and the fixed-time boundedness of all closed-loop signals is established. Finally, the efficacy of the propounded control scheme is manifested through numerical simulation results.
Citation: Yuhan Su, Shaoping Shen. Adaptive predefined-time prescribed performance control for spacecraft systems[J]. Mathematical Biosciences and Engineering, 2023, 20(3): 5921-5948. doi: 10.3934/mbe.2023256
[1] | Dongxiang Gao, Yujun Zhang, Libing Wu, Sihan Liu . Fixed-time command filtered output feedback control for twin-roll inclined casting system with prescribed performance. Mathematical Biosciences and Engineering, 2024, 21(2): 2282-2301. doi: 10.3934/mbe.2024100 |
[2] | Gaowang Zhang, Feng Wang, Jian Chen, Huayi Li . Fixed-time sliding mode attitude control of a flexible spacecraft with rotating appendages connected by magnetic bearing. Mathematical Biosciences and Engineering, 2022, 19(3): 2286-2309. doi: 10.3934/mbe.2022106 |
[3] | Yun Liu, Yuhong Huo . Predefined-time sliding mode control of chaotic systems based on disturbance observer. Mathematical Biosciences and Engineering, 2024, 21(4): 5032-5046. doi: 10.3934/mbe.2024222 |
[4] | Jiao Wu, Shi Qiu, Ming Liu, Huayi Li, Yuan Liu . Finite-time velocity-free relative position coordinated control of spacecraft formation with dynamic event triggered transmission. Mathematical Biosciences and Engineering, 2022, 19(7): 6883-6906. doi: 10.3934/mbe.2022324 |
[5] | Hui Wang . Prescribed-time control of stochastic high-order nonlinear systems. Mathematical Biosciences and Engineering, 2022, 19(11): 11399-11408. doi: 10.3934/mbe.2022531 |
[6] | Tianqi Yu, Lei Liu, Yan-Jun Liu . Observer-based adaptive fuzzy output feedback control for functional constraint systems with dead-zone input. Mathematical Biosciences and Engineering, 2023, 20(2): 2628-2650. doi: 10.3934/mbe.2023123 |
[7] | Zhenqi He, Lu Yao . Improved successive approximation control for formation flying at libration points of solar-earth system. Mathematical Biosciences and Engineering, 2021, 18(4): 4084-4100. doi: 10.3934/mbe.2021205 |
[8] | Rinaldo M. Colombo, Mauro Garavello . Optimizing vaccination strategies in an age structured SIR model. Mathematical Biosciences and Engineering, 2020, 17(2): 1074-1089. doi: 10.3934/mbe.2020057 |
[9] | Na Zhang, Jianwei Xia, Tianjiao Liu, Chengyuan Yan, Xiao Wang . Dynamic event-triggered adaptive finite-time consensus control for multi-agent systems with time-varying actuator faults. Mathematical Biosciences and Engineering, 2023, 20(5): 7761-7783. doi: 10.3934/mbe.2023335 |
[10] | Jia Tan, ShiLong Chen, ZhengQiang Li . Robust tracking control of a flexible manipulator with limited control input based on backstepping and the Nussbaum function. Mathematical Biosciences and Engineering, 2023, 20(12): 20486-20509. doi: 10.3934/mbe.2023906 |
The high-accuracy attitude maneuvering problem for spacecraft systems is investigated. A prescribed performance function and a shifting function are first employed to ensure the predefined-time stability of attitude errors and eliminate the constraints on tracking errors at the incipient stage. Subsequently, a novel predefined-time control scheme is developed by combining prescribed performance control and backstepping control procedures. Radial basis function neural network and minimum learning parameter techniques are introduced to model the function of lumped uncertainty including inertial uncertainties, actuator faults and virtual control law derivatives. According to the rigorous stability analysis, the preset tracking precision can be achieved within a predefined time and the fixed-time boundedness of all closed-loop signals is established. Finally, the efficacy of the propounded control scheme is manifested through numerical simulation results.
The attitude stabilization problem has attracted extensive attention in recent years for its significant applications in spacecraft navigation, satellite formation flying and the recycling of space debris. To achieve satisfactory tracking performance, system uncertainties and other nonlinear dynamics of the controlled spacecraft should be handled effectively. A wide range of control techniques have been proposed to address these issues, including adaptive control [1], output feedback control [2] and robust control [3]. What is more, spacecraft systems commonly experience actuator constraints such as saturation and degradation, which would severely undermine their practicality and reliability. To deal with these actuator nonlinearity problems, several methods have been developed in [4,5,6]. However, the majority of above-mentioned strategies can only realize asymptotic stabilization of the system, implying that the convergence time of tracking errors is infinite and cannot be determined by users, which is contrary to the requirement that some real-time space missions require a rapid convergence.
To improve the convergence rate of the aforementioned strategies, the concept of finite-time control was initially proposed in [7] and continuously applied to a variety of nonlinear systems[8,9,10]. However, the convergence time cannot be predefined as desired, and its upper bound is an infinite function of original states. This deficiency was addressed by developing a fixed-time controller [11], which has the appealing merit that its time is independent of initial configurations. Sliding mode methods are widely employed to realize control performance. In [12], a nonsingular terminal sliding mode surface (NTSMS) is designed by using a piecewise continuous function. And based on the NTSMS, an adaptive controller is proposed for spacecraft formation, allowing for fixed-time coordinated attitude tracking. In [13], a robust fixed-time attitude controller is established through the use of a faster fixed-time sliding mode surface and a fixed-time observer is designed for lumped uncertainty. In addition to sliding mode control, a backstepping technique can also be used in the construction of fixed-time controllers. In [14], a fixed-time backstepping controller is constructed by virtue of a command filter for a class of nonstrict-feedback nonlinear systems, and the fuzzy logic system is introduced to approximate uncertainties, input saturation and dead zones. In [15], a fixed-time control protocol is proposed for hyper sonic vehicles by using a tracking differentiator for the calculation of the derivatives of virtual control law. In [16], a command filter based backstepping control scheme is introduced to avoid the computational complexity of the derivative of virtual control law in conventional backstepping schemes. In [17], the backstepping control technique is combined with a dynamic surface method to light the computational burden. Notwithstanding, no explicit functions between tunable parameters and settling time can be obtained via the above-mentioned methods.
Recently, the stabilization of systems with predefined-time convergence has become a hotpot and many meaningful studies have been conducted on this topic because of its enhanced property as compared to fixed/finite-time control schemes when it comes to rendering states into the origin with the settling time that is explicitly equal to a user-tuning parameter. The sliding mode control and backstepping are two of the most commonly used methods for designing predefined-time controllers, and some representative work of predefined-time control of spacecraft systems has been reported in [18,19,20,21]. However, the estimate of the convergence time bound in these works is somewhat conservative, resulting in the actual settling time being several times shorter than the estimate.
Another important control goal of designed controllers is to achieve desirable transient performance. To this end, prescribed performance control (PPC) protocol was first developed in [22] and heavily implemented in spacecraft systems[23,24,25] in recent years. In [25], combining PPC and NTSMS, a fixed-time sliding mode attitude controller is designed for flexible spacecraft systems. Unlike the conventional exponential convergence prescribed performance function (PPF), a novel predefined-time PPF is designed in [26], and it has the more appealing property that the convergence time is prescribed by users. Chen et al. [27] utilized a polynomial function to design the PPF with predefined time convergence; it can mitigate the chattering problem caused by an exponential function. Bu et al. [28] constructed a finite-time prescribed performance controller for waverider vehicles, and no fuzzy/neural systems are required to estimate the unknown dynamics. In [29] and [30], two brand-new types of finite-time PPFs are explored for the purpose of minimizing the overshoot and overcoming the fragility problem caused by actuator saturation. Notwithstanding, the condition that the PPFs should be larger than the tracking errors at the incipient stage needs to be satisfied for the execution of most of the above PPF-based controllers.
To the best of the authors' knowledge, developing a predefined-time controller for spacecraft systems with inertia perturbation, space-environment disturbances and actuator faults is an open topic. Inspired by the existing work, we have designed a radial basis function neural network (RBFNN)-based controller with prescribed performance and appointed-time convergence which ensures that the tracking error will converge to a prescribed small region in the vicinity of the origin within the predefined time. The main contributions can be summarized as follows.
1) By employing the proposed PPC control scheme, both the convergence time and tracking accuracy can be arbitrarily predefined by users. The designed controller presents great robustness against input saturation, actuator misalignment and unexpected disturbance.
2) By applying a novel shifting function to conventional PPC, we release the constraints for the initial tracking errors to be smaller than the initial PPF values. Additionally, the shifting function also allows for an improved handling of input saturation.
3) Following the representative backstepping design methodology, we propose an attitude controller with prescribed performance and appointed-time convergence for spacecraft systems. The singularity problem associated with virtual control law is avoided via the design of a piecewise continuous function.
4) RBFNN and minimum learning parameter (MLP) techniques are combined to estimate the system uncertainty and the derivative of virtual control law. Moreover, the fixed-time convergence of the learning parameter is ensured by constructing the adaptive law.
Lemma 1. [11] Considering a nonlinear system
˙x=f(x,t) | (2.1) |
suppose that there exists a positive-definite Lyapunov function V and scalars γ1>0, γ2>0, p>1, 0<q<1 and Δ>0 such that the following property holds:
˙V≤−γ1Vp−γ2Vq+Δ | (2.2) |
Then, the equilibrium of (2.1) is practically fixed-time stable with the settling time Tf≤1γ1κ(1−p)+1γ2κ(q−1), where 0<κ<1. The solution of (2.1) will converge to a residual set that is given as
{x∣V≤min{(Δγ1(1−κ))1p,(Δγ2(1−κ))1q}} | (2.3) |
Lemma 2. [31] For x,y∈R, the following relationship holds:
|x|m|y|n≤mm+nc|x|m+n+nm+nc−mn|y|m+n | (2.4) |
where m>0, n>0 and c>0.
Lemma 3. [32] For y>x and l>0, we have
x(y−x)l≤l1+l(y1+l−x1+l) | (2.5) |
Lemma 4. [33] For the variables x1,x2,…xn>0, 0<y1≤1 and y2>1, the following inequalities hold:
n∑i=1xy1i≥(n∑i=1xi)y1 | (2.6) |
n∑i=1xy2i≥n1−y2(n∑i=1xi)y2 | (2.7) |
Notation 1. In this paper, sigα(β)=[|ξ1|αsgn(β1),|β2|αsgn(β2),…,|βn|αsgn(βn)]T, where β=[β1,β2,…,βn]T; sgn(⋅) denotes the sign function. The definition of the skew-symmetric matrix is given by β×=[0,−β3,β2;β3,0,−β1;−β2,β1,0], when n=3.
The kinematic equation and dynamics of a spacecraft can be presented as
[˙q0˙qv]=[−12qTv12(q×v+q0I3)]ω | (2.8) |
J˙ω+ω×Jω=τ+d | (2.9) |
where q=[q0,q1,q2,q3]T=[q0,qv]T∈R4 denotes the unit quaternion vector which is used to parameterize the orientation of spacecraft satisfying the identity q20+qTvqv=1; ω∈R3 represents the angular velocity of spacecraft; d∈R3 denotes the unknown environment disturbance torque; J=J0+ΔJ∈R3×3 denotes the inertia matrix of the spacecraft system, with J0 and ΔJ being the nominal and perturbed components, respectively; τ∈R3 is the control torque acting on spacecraft.
The relationship between the actual control torque and the command input can be given by [34]:
τ=Esat(uc)+σ | (2.10) |
where uc=[uc1,uc2,uc3]T∈R3 is the command torque generated by controllers; E=diag{e1(t),e2(t),e3(t)}∈R3×3 represents the failure coefficient matrix indicating the effectiveness condition of the actuator with 0≤ei(t)≤1; σ=[σ1,σ2,σ3]T∈R3 is the bias faults vector. The saturation characteristic of an actuator [35] can be formulated as sat(uci)=sgn(uci)⋅min{|uci|,umaxi}, where umaxi denotes the maximum permissible torque generated by actuators.
Define qd=[qd0,qd1,qd2,qd3]T=[qd0,qdv]T∈R4 as the expected attitude vector. The attitude error described in unit quaternion format [36] is given by qe=[qe0,qe1,qe2,qe3]T=[qe0,qev]T, qev=qd0qv−q×dvqv−q0qdv, qe0=q×dvqv+q0qd0 and q2e0+qTevqev=1. Considering the rest-to-rest attitude maneuvering case, we have that ωe=ω in this paper.
The attitude error dynamics of the spacecraft systems is given by
[˙qe0˙qev]=[−12qTev12(q×ev+qe0I3)]ωe | (2.11) |
˙ωe=J−10(M+τ+N+d) | (2.12) |
where M=−ωe×J0ωe and N=−ΔJ˙ωe−ωe×ΔJωe.
Define x1=qev and x2=˙qev; (2.11) and (2.12) can be reconstructed as
{˙x1=x2˙x2=G+Π+d2+F(qev)J0−1uc | (2.13) |
where G=˙F(qev)ωe+F(qev)J0−1M, Π=F(qev)J0−1N+F(qev)J0−1(E−I3)uc+F(qev)J0−1σ+F(qev)J0−1(E(sat(uc)−uc)), d2=F(qev)J0−1d and F(qev)=12(q×ev+qe0I3).
Assumption 1. The perturbed part of the inertia matrix, the external disturbance and the faulty torque are unknown but bounded. The lumped disturbance is bounded and satisfies ‖d2‖≤ˉd, where ˉd is a positive constant.
The primary objective is to design an adaptive controller for spacecraft systems in the presence of actuator fault so as to achieve the prespecified tracking accuracy within a predefined time and satisfy the prescribed performance boundaries throughout the entire process, as well as to ensure the fixed-time boundedness of other closed-loop signals. Both the settling time and tracking precision can be defined according to the specific requirements of users, irrespective of the initial conditions.
To guarantee that attitude trajectory of the spacecraft remains within the prescribed boundaries with desirable transient and static performance, the following constraints are constructed first:
−δ_iρi(t)<qevi(t)<¯δiρi(t) | (3.1) |
where ¯δi,δ_i>0, (i=1,2,3) are two adjustable parameters and ρi(t) is the PPF.
In this paper, we propose a novel predefined-time PPF as
ρi(t)={(ρi0−ρi∞)cos(πt2Tc)ai+ρi∞,t<Tcρi∞,t≥Tc | (3.2) |
where ρi0>ρi∞>0 are constants, Tc>0 is the predefined maximum allowable convergence time that can be arbitrarily defined by users and ai>0 represents a preset constant that can adjust the convergence rate. ρi(t) is a monotonically decreasing smooth function that can converge from ρi0 to ρi∞ within Tc.
The traditional PPC method requires that the initial tracking errors satisfy the condition (3.1). Based on this constraint, the values of ¯δi, δ_i and even ρi need to be reassigned when the original tracking error exceeds the initial value of the PPF, which is challenging considering that the initial configurations are unavailable. To overcome this weakness, we introduce a shifting function to map the value of the initial tracking error into the interval [−δ_iρi0,¯δiρi0] as
ηi=2kiπarctan(qevi) | (3.3) |
where ki={¯δiρi0,qevi≥0,δ_iρi0,qevi<0.. Note that we set ¯δi=δ_i=1 in the following paper for the simplicity of analysis.
Remark 1. From (3.3), it can be seen that limqevi→−∞ηi=−ρi0, limqevi→+∞ηi=ρi0, which indicates that, regardless of the largeness of the attitude errors, they will not violate the prescribed boundary requirements defined in (3.1) at the outset. Moreover, when limt→Tcηi=0, we can obtain limt→Tcqevi=0, meaning that the predefined-time attitude maneuvering can be achieved by rendering ηi to zero within a prescribed interval.
Since ηi satisfies the boundary conditions, we have
−ρi(t)<ηi<ρi(t) | (3.4) |
From (3.3), the prescribed boundary for the attitude error qevi is shifted into
−hi(t)<qevi<hi(t) | (3.5) |
where hi(t)=tan(π2kiρi(t)),(i=1,2,3). hi(t) is a monotonically decreasing function with limt→Tchi=tan(π2kiρi(∞)).
To convert the constraint on qevi into its unconstrained counterpart, the transformation function is defined as
T(εi)=2πarctan(εi) | (3.6) |
Obviously, T(εi) is a monotonically increasing function with the following properties: (1) −1<T(εi)<1; (2) limεi→+∞T(εi)=1; (3) limεi→−∞T(εi)=−1; (4) T(0)=0.
In what follows, we define
ηi=ρiT(εi) | (3.7) |
Therefore, the transformed error εi is introduced as
εi=T−1(ξi)=tan(π2ξi) | (3.8) |
where ξi=ηiρi is the normalized error.
During the period of actuator saturation, the attitude errors may grow to exceed the prescribed envelopes, which can result in the transformed error εi approaching infinity. Consequently, the actuator will be kept saturated, compromising the stability and reliability of the system. To this end, we redesign the coefficient of our proposed shifting function as follows:
ki={ρi,|sat(uci)−uci|>0si,|sat(uci)−uci|=0 | (3.9) |
where 0<si<π2 is a positive constant.
Remark 2. According to the properties of T(εi), it is obvious that the desired performance for the shifted attitude errors ηi prescribed in (3.4) can be achieved when the boundedness of εi is ensured. In this respect, the problem of (3.4) is converted into its equivalent of stabilizing the transformed state εi by designing the controller.
Remark 3. Unlike the previous finite-time PPFs proposed in [26,27,28,37], the settling of ρi0 is independent of qevi(0). With the assistance of the shifting function provided in (3.3), the PPF defined in (3.2) does not require prior knowledge of initial errors to design the parameters. The removal of restrictions on initial conditions simplifies the design process and contributes to the reliability and practicality of the proposed PPC scheme.
Remark 4. When there is input saturation, the shifting function ensures that the shifted error ηi remains within the appointed boundary [−ρi,ρi]. With a smaller ηi, the value of the normalized error ξi will be reduced, resulting in a smaller transformed error εi and a decline in the control input. When the actuator exits its saturation zone, the coefficient of the shifting function changes to si. Compared with the existing strategies[37,38,39], this method can reduce the control output by minimizing the absolute value of the normalized error ηi. (The proof can be seen in Appendix.)
To facilitate the implementation of backstepping methods, we can define
{z1=x1z2=x2−α2 | (4.1) |
From (2.13), the time derivative of z1 is
˙z1=x2 | (4.2) |
To remove the initial value constraints, we impose the following shifted function on z1 and obtain the shifted error signals:
η1i=2k1iπarctan(z1i) | (4.3) |
Define the first normalized tracking error ξ1i=η1iρ1i, and using the transformation function defined in (3.6), we can obtain
ε1i=tan(π2ξ1i) | (4.4) |
The time derivative of ε1i is
˙ε1i=∂ε1i∂ξ1i⋅˙ξ1i=π2sec(π2ξ1i)2⋅˙η1iρ1i−η1i˙ρ1iρ21i=π2ρ1isec(π2ξ1i)2⋅(2k1iπ√1+z21i˙z1i−η1i˙ρ1iρ1i)=ψ1i(g1ix2i−f1i) | (4.5) |
where ψ1i=π2ρ1isec(π2ξ1i)2, g1i=2k1iπ√1+z21i and f1i=η1i˙ρ1ρ1i.
We can rewrite ε1i in vector form as
˙ε1=ψ1(g1x2−f1) | (4.6) |
where ψ1=diag{ψ1i}, g1=diag{g1i} and f1=[f11,f12,f13]T.
Then, the virtual control law α2=[α21,α22,α23]T can be established as
α2=−(g1)−1(ψ1)−1(k1sigp(ε1)+k2ϕ1−ψ1f1) | (4.7) |
where k1>0, k2>0, p>1 and ϕ1=[ϕ11,ϕ12,ϕ13]T is a piecewise continuous function designed as
ϕ1i={sigq(ε1i),if|ε1i|>μl1sig(ε1i)(μ2)q−12+l2sig2(ε1i)(μ2)q2−1+l3sig2(ε1i)(μ2)q−32,if|ε1i|≤μ | (4.8) |
where 0<q<1, l1=0.5q2−2.5q+3, l2=−q2+4q−3, l3=0.5q2−1.5q+1 and μ is a tiny positive constant.
Remark 5. If α2 is designed as α2=−(g1)−1(ψ1)−1(k1sigp(ε1)+k2sigq(ε1)−ψ1f1), then its derivative will be ˙α2=−(g1)−1(ψ1)−1(k1˙ε1sigp−1(ε1)+k2˙ε1sigq−1(ε1)−ψ1˙f1). The singularity problem may happen in ˙α2 because of 0<q<1 when ε1i=0 and ˙ε1i≠0. To avoid the problem, we design the above piecewise function at the switching point μ. The values of l1, l2 and l3 are selected to ensure the continuity of ϕ1i and its first and second derivative.
The candidate of the first Lyapunov function is defined as
V1=12εT1ε1 | (4.9) |
Differentiating V1 yields
˙V1=εT1˙ε1=εT1ψ1[g1(z2+α2)−f1] | (4.10) |
Substituting (4.7) into (4.10), when |ε1i|>μ, we have
˙V1=εT1ψ1[g1(z2−(g1)−1(ψ1)−1(k1sigp(ε1)+k2sigq(ε1)−ψ1f1))−f1]=εT1ψ1g1z2−k13∑i=1|ε1i|p+1−k23∑i=1|ε1i|q+1 | (4.11) |
When |ε1i|≤μ, we can obtain
˙V1=εT1ψ1g1z2−k13∑i=1|ε1i|p+1−k2(l1(μ2)q−123∑i=1|ε1i|2+l2(μ2)q2−13∑i=1|ε1i|3+l3(μ2)q−323∑i=1|ε1i|4)≤εT1ψ1g1z2−k13∑i=1|ε1i|p+1−k23∑i=1|ε1i|q+1+k2(3∑i=1|ε1i|q+1+l1(μ2)q−123∑i=1|ε1i|2+l2(μ2)q2−13∑i=1|ε1i|3+l3(μ2)q−323∑i=1|ε1i|4)≤εT1ψ1g1z2−k13∑i=1|ε1i|p+1−k23∑i=1|ε1i|q+1+3k2((μ2)q+12+(l1+l2+l3)(μ2)q+12)≤εT1ψ1g1z2−k13∑i=1|ε1i|p+1−k23∑i=1|ε1i|q+1+6k2(μ2)q+12 | (4.12) |
Note that, when |ε1i|≤μ, there is only a bounded term 6k2(μ2)q+12 added to the structure of (4.11).
Taking the derivative of z2, we can obtain
˙z2=G+Π+d2+F(qev)J0−1uc−˙α2 | (4.13) |
Similarly, we can relax the feasibility condition by introducing the shifting function to z2 and obtain the shifted error signals:
η2i=2k2iπarctan(z2i) | (4.14) |
Define the second normalized tracking error ξ2i=η2iρ2i, and the ith component of the transformed error vector can be defined as
ε2i=tan(π2ξ2i) | (4.15) |
The time derivative of ε2i is
˙ε2i=∂ε2i∂ξ2i⋅˙ξ2i=π2sec(π2ξ2i)2⋅˙η2iρ2i−η2i˙ρ2iρ22i=π2ρ2isec(π2ξ2i)2⋅(2k2iπ√1+z22i˙z2i−η2i˙ρ2iρ2i)=ψ2i(g2i˙z2i−f2i) | (4.16) |
where ψ2i=π2ρ2isec(π2ξ2i)2, g2i=2k2iπ√1+z22i and f2i=η2i˙ρ2iρ2i.
The vector form can be rewritten as
˙ε2=ψ2(g2˙z2−f2) | (4.17) |
where ψ2=diag{ψ2i}, g2=diag{g2i} and f2=[f21,f22,f23]T.
Substituting (4.13) into (4.17), we have
˙ε2=ψ2(g2(G+Π+d2+F(qev)J0−1uc−˙α2)−f2) | (4.18) |
Choose the second Lyapunov function candidate as
V2=12εT2ε2 | (4.19) |
Differentiating V2 with respect of time yields
˙V2=εT2ψ2[g2(G+Π+d2+F(qev)J0−1uc−˙α2)−f2]=εT2D+εT2ψ2[g2(G+d2+F(qev)J0−1uc)−f2] | (4.20) |
where D=ψ2g2(Π−˙α2)=[D1,D2,D3]T is the lumped disturbance which can be approximated with the aid of the following RBFNN:
D(Z)=WTS(Z)+ζ | (4.21) |
where W∈Rn×3 denotes the optimal weight matrix, n represents the number of network neurons, Z=[qev,ωe,ε1]T is the input vector, ζ∈R3 is the approximation error vector with ‖ζ‖≤ζm and S(Z)=[S1(Z),S2(Z),...,Sn(Z)]Tn∑i=1Si(Z)∈Rn is the basis function vector with
Si=exp(−(Z−βi)T(Z−βi)H2),i=1,2,...n | (4.22) |
where βi and H are the receptive field center and width of the neural cell, respectively.
By defining θ=‖W‖2, we use the MLP technique. In this way, we regulate the norm of the ideal weight matrix rather than its elements and only one learning parameter is required to be updated for the execution of the neural network. Therefore, the computational burden and the complexity of the propounded strategy can be significantly reduced.
The RBFNN-based adaptive controller can be designed as
uc=−J0F(qev)−1(g2)−1(ψ2)−1(r1sigp(ε2)+r2sigq(ε2)+r3ε2+ψ2g2G−ψ2f2+ε2‖ε2‖2εT1ψ1g1z2+ˆθε22h2ST(Z)S(Z)) | (4.23) |
where r1>0, r2>0, r3>R+12 and R=‖ψ2‖2‖g2‖2. The adaptive law of the learning parameter θ is developed as
˙ˆθ=−w1ˆθ−w2ˆθq+λ‖ε2‖22h2ST(Z)S(Z) | (4.24) |
where w1>0, w2>0, λ>0 and h>0.
Substituting (4.23) into (4.20), we have
˙V2≤εT2ψ2g2d2+εT2D−r13∑i=1|ε2i|p+1−r23∑i=1|ε2i|q+1−r3‖ε2‖2−εT1ψ1g1z2−ˆθ‖ε2‖22h2ST(Z)S(Z) | (4.25) |
With the help of Young's inequality and the property that 0≤STS≤1, we have
εT2ψ2g2d2≤‖ε2‖2‖ψ2‖2‖g2‖22+‖d2‖22≤R‖ε2‖22+ˉd22 | (4.26) |
εT2D=εT2WTS(Z)+εT2ζ≤‖ε2‖‖W‖‖S(Z)‖+3∑i=1ε2iζi≤θ‖ε2‖2ST(Z)S(Z)2h2+h22+‖ε2‖22+3ζ2m2≤θ‖ε2‖22h2ST(Z)S(Z)+h22+‖ε2‖22+3ζ2m2 | (4.27) |
Theorem 1. Considering the spacecraft system (2.13), the controller (4.23) and the adaptive law (4.24), one can ensure the practical fixed-time boundedness of all of the closed-loop signals. Besides, for any constants ν and T, if the PPF (3.2) parameters are respectively set as ρ1i∞=2s1iπarctan(ν) and Tc=T, the tracking error will converge into the predefined region |qevi|≤ν within the predefined time T, irrespective of the initial conditions.
Proof. Choose the third Lyapunov function for the whole system:
V3=V1+V2+12λ˜θ2 | (4.28) |
where ˜θ=θ−ˆθ.
Differentiating V3 yields
˙V3=˙V1+˙V2−1λ˜θ˙ˆθ | (4.29) |
Together with (4.11), (4.12), (4.24), (4.25), (4.26) and (4.27), one has
˙V3≤εT1ψ1g1z2−k13∑i=1|ε1i|p+1−k23∑i=1|ε1i|q+1+6k2(μ2)q+12+R‖ε2‖22+ˉd22+θ‖ε2‖22h2ST(Z)S(Z)+h22+‖ε2‖22+3ζ2m2−r13∑i=1|ε2i|p+1−r23∑i=1|ε2i|q+1−r3‖ε2‖2−εT1ψ1g1z2−ˆθ‖ε2‖22h2ST(Z)S(Z)−˜θλ(−w1ˆθ−w2ˆθq+λ‖ε2‖22h2ST(Z)S(Z))≤−k13∑i=1|ε1i|p+1−k23∑i=1|ε1i|q+1−r13∑i=1|ε2i|p+1−r23∑i=1|ε2i|q+1+w1λ˜θˆθ+w2λ˜θˆθq+6k2(μ2)q+12+ˉd22+h22+3ζ2m2 | (4.30) |
With the help of Young's inequality, the following inequality is true.
w1λ˜θˆθ=w1λ˜θ(θ−˜θ)=−w1λ˜θ2+w1λ˜θθ≤−w12λ˜θ2+w12λθ2 | (4.31) |
By invoking (4.31), (4.30) can be rewritten as
˙V3≤−k13∑i=1|ε1i|p+1−k23∑i=1|ε1i|q+1−r13∑i=1|ε2i|p+1−r23∑i=1|ε2i|q+1−w12λ˜θ2+w12λθ2+w2λ˜θˆθq+Δ1 | (4.32) |
where Δ1=6k2(μ2)q+12+ˉd22+h22+3ζ2m2.
Applying Lemma 2 and selecting x=w12λ˜θ2, y=1, m=1+p2, n=1−p2 and c=2p+1 yields
(w12λ˜θ2)p+12≤w12λ˜θ2+1−p2(2p+1)−1+p1−p | (4.33) |
In view of Lemma 3, one has
w2λ˜θˆθq=w2λ˜θ(θ−˜θ)q≤w2qλ(1+q)(θq+1−˜θq+1) | (4.34) |
Hence, substituting (4.33) and (4.34) into (4.32), we can obtain
˙V3≤−k13∑i=1|ε1i|p+1−k23∑i=1|ε1i|q+1−r13∑i=1|ε2i|p+1−r23∑i=1|ε2i|q+1−(w12λ˜θ2)p+12−w2qλ(1+q)˜θq+1+w12λθ2+1−p2(2p+1)−1+p1−p+w2qλ(1+q)θq+1+Δ1≤−a1(εT1ε12)p+12−b1(εT1ε12)q+12−a2(εT2ε22)p+12−b2(εT2ε22)q+12−a3(˜θ22λ)p+12−b3(˜θ22λ)q+12+Δ≤−γ1Vp+123−γ2Vq+123+Δ | (4.35) |
where a1=k12p+12, b1=k12q+1231−q2, a2=r12p+12, b2=r22q+1231−q2, a3=wp+121, b3=2q+12w2qλq+12λ(1+q), γ1=min{a1,a2,a3}, γ2=min{31−q2b1,31−q2b2,31−q2b3} and Δ=w12λθ2+1−p2(2p+1)−1+p1−p+w2qλ(1+q)θq+1+Δ1.
(1) In light of Lemma 1, V3 will converge to the region Ωv=min{(Δγ1(1−κ))2p+1,(Δγ2(1−κ))2q+1} within the fixed time Tf. The settling time is bounded by Tf≤Tmax=2γ1κ(1−p)+2γ2κ(q−1).
Apparently, ε1i and ε2i will converge to the following regions, respectively:
Ωε1i=min{√2(Δγ1(1−κ))2p+1,√2(Δγ2(1−κ))2q+1} | (4.36) |
Ωε2i=min{√2(Δγ1(1−κ))2p+1,√2(Δγ2(1−κ))2q+1} | (4.37) |
Based on (4.4) and (4.15), we can further obtain the residual sets that η1i and η2i will respectively converge to Ωη1i and Ωη2i within Tf.
Ωη1i={η1i∣|η1i|≤ρ1i(Tf)2πarctan(Ωε1i)} | (4.38) |
Ωη2i={η2i∣|η2i|≤ρ2i(Tf)2πarctan(Ωε2i)} | (4.39) |
(2) In view of (3.4) and the property of ρ1i(t), the inequality −ρ1i(∞)<η1i<ρ1i(∞) is satisfied when t≥T. By designing ρ1i(∞)=ρ1i∞=2s1iπarctan(ν) and Tc=T, we have
|qevi|≤ν | (4.40) |
Therefore, the attitude error can converge to a prescribed region Ω={qevi∣|qevi|≤ν} within the predefined time T. The flowchart that manifests the process for generating the proposed control action is shown in Figure 1.
Remark 6. The tracking accuracy and convergence time can be explicitly and arbitrarily settled in advance, irrespective of the initial conditions, by tuning ν and T respectively. A smaller ν and T contribute to improved precision, as well as a shorter convergence period. However, it is noted that the setting of the parameters ν and T is exactly based on a trade-off between ambitious aims and allowable practices.
Remark 7. The control parameters p, q, r1, r2, k1 and k2 can be selected by trial-and-error methods to ensure that all other closed-loop signals are fixed-time bounded. The setting of these parameters does not necessarily require taking the values of ν and T into consideration.
The nominal component of the inertia matrix is defined as
J0=[201.20.91.2171.40.91.415]kg⋅m2. |
The uncertain part of the inertia matrix is
ΔJ=[4.20.90.60.9−72.50.62.55.89]kg⋅m2. |
The external disturbance is set to be
d=[−4+4cos(0.2t)−cos(0.4t)3+3sin(0.2t)−2cos(0.4t)−3+4sin(0.2t)−3cos(0.4t)]×10−2N⋅m. |
The actuator misalignment takes the form of
e1={10.6ift≤2ift>2e2={10.4ift≤4ift>4e3={10.5ift≤5ift>5 |
σ1={0−0.2ift≤3ift>3σ2={00.1ift≤4ift>4σ3={0−0.1ift≤6ift>6 |
The desired attitude is qd=[1,0,0,0]T. We consider two groups of different initial values to perform the simulation. Case 1: q(0)=[0.6698,−0.5158,0.4716,0.2508]T; Case 2: q(0)=[0.1737,−0.2632,0.7896,−0.5264]T. The maximum control torque is considered to be umaxi=7.5 N ⋅ m.
For the virtual control law (4.7) and the actual control law (4.23), the parameters are selected as k1=1, k2=2, p=1.2, q=0.8, r1=10, r2=5 and μ=0.01. The parameters of the update law (4.24) are chosen as w1=2, w2=1, λ=10 and h=1. The shifting function parameters are given as s1i=s2i=0.4. The initial PPFs are set as ρ1i0=ρ2i0=0.4. It is noteworthy that the initial condition has been violated since the initial errors qe2(0) and qe3(0) in Case 1 and qe2(0) and qe3(0) in Case 2 are bigger than ρ1i0.
In this section, Cases 1 and 2 are considered to demonstrate the efficacy of our proposed approach when it comes to handling attitude tracking problems with a predefined convergence time independent of the original states. The predefined-time PPF parameters are given as ν=0.01, T=10, ρ1i∞=2s1iπarctan(ν)=0.0025, ρ2i∞=0.1 and a1i=a2i=1.2.
The simulation results are shown in Figures 2–7. Figures 2 and 3 show that the proposed controller performs fairly well under different initial conditions, and that the actual settling time is 7.5 s, which is shorter than the predefined one. With the implementation of the shifting function, the proposed controller is able to maintain attitude errors within prescribed envelopes despite the tracking errors exceeding the PPFs at the beginning. As shown in Figure 4, different control torques are required under different initial conditions to provide the desired performance. It can be seen in Figures 5 and 6 that the transformed errors ε1i and ε2i are fixed-time bounded. The boundedness of the adaptive parameter is shown in Figure 7.
To further illustrate that the attitude maneuvering performance of spacecraft systems can be prescribed with our proposed method in terms of convergence time, we present the results of the simulation with two different convergence times T=10 and T=15. Case 1 is considered for the initial attitude value. Other parameters of the PPFs and the proposed controller remain unchanged from Simulation one.
The corresponding results are shown in Figures 8–10. It is observed in Figure 8 that the proposed controller will render attitude error into the predefined region |qevi|≤0.01 within T. Figures 8 and 9 also show that the convergence time of attitude errors with our proposed methods can be directly and arbitrarily set by selecting different values of T. In general, a smaller T indicates a shorter stabilization period but a greater control burden as shown in Figure 10.
To demonstrate that the tracking performance of spacecraft systems can be prescribed with our propounded controller in terms of control accuracy, we present the simulation with ν as ν=0.01 and ν=0.001 and the same value of prescribed settling time T=5. Hence, ρ1i∞ values are set as ρ1∞i=0.0025 and ρ1∞i=2.5×10−4, while other adjustable parameters remain the same as those in Simulation one. We consider the example in Case 2 as the initial value of the quaternion.
The results are depicted in Figures 11 and 12. It is shown in Figure 11 that the attitude error is stabilized into the prescribed region |qevi|≤ν within T no matter whether the initial values of the quaternion violate the prescribed constraints. Generally, a smaller ν contributes to improved precision in attitude maneuvering at the expense of a heavier burden on the controller, as shown in Figure 12.
To illustrate the advantage of our propounded controller, the fault-tolerant fast fixed-time convergent attitude control (FTFFTCAC) proposed in [38] is considered to perform the comparative study. The preset convergence time and prescribed accuracy are respectively given as T=10, v=0.01. Other control parameters remain unchanged. We select Case 2 for the initial tracking errors.
For the following modified prescribed performance function (MPPF) developed in [38], the MPPF parameters are chosen as k=0.4, Tm=10, ρ0=1 and ρ∞=0.01. Other control parameters are chosen as [38].
ρi(t)={(ρ0−ρ∞(1+t/Tm))exp(−ktTm−t)+ρ∞,t<Tmρ∞,t≥Tm | (5.1) |
It can be seen in Figures 13 and 14 that our proposed control scheme exhibits better tracking performance than the FTFFTCAC scheme, with faster convergence and higher accuracy. Figure 15 shows that the control consumption of the designed controller is significantly less than that of FTFFTCAC, and that the control action is smoother.
To demonstrate the robustness of our proposed control scheme, an additional disturbance is imposed on the spacecraft during the period of 13–18 s with the term dsud=[2+0.5sin(0.2t)2+0.5sin(0.2t)2+0.5sin(0.2t)]N⋅m.
Figure 16 shows that the proposed controller can guarantee the tracking errors with performance in terms of convergence time and steady-state precision in the presence of an unexpected disturbance. The shifted errors are always kept within the constraints, which verifies the robustness of our proposed controller. It is depicted in Figure 17 that the control torque is bounded and not chattering when a sudden change in disturbance occurs. As shown in Figure 18, the attitude error qe1 reaches the guaranteed performance boundary at around t=14 s, resulting in a loss of efficacy for the FTFFTCAC controller.
In this article, a novel adaptive predefined-time prescribed performance controller is presented for spacecraft systems. By employing a predefined-time PPF, we guarantee that the attitude errors will satisfy the prescribed tracking accuracy within a predefined time. By introducing a novel shifting function, we eliminate the constraints on initial errors, enabling the proposed method to be implemented even if the attitude errors violate the prescribed boundaries initially. RBFNN and MLP techniques have been introduced to approximate the lumped uncertain dynamics, and the adaptive law has been designed to ensure the fixed-time convergence of the learning parameter. Our proposed method has the notable merit of allowing the settling time and the tracking precision to be directly prespecified by setting two adjustable parameters. The proposed control scheme exhibits excellent performance against input saturation, actuator misalignment and unexpected disturbances.
This research was supported by the National Natural Science Foundation (NNSF) of China under Grants 61333008, 61603320, 61733017 and 61673327 and Xiamen Key Laboratory Of Big Data Intelligent Analysis and Decision.
The authors declare that there is no conflict of interest.
To achieve |e|≤v, the PPF parameter should be designed as ρ∞=v according to previous PPC schemes [37,38,39].
The PPF defined in (3.2) and in [37,38,39] can be rewritten in a general form as follows:
ρ(t)=rρ0+(1−r)v | (A1) |
where 0≤r≤1 refers to the monotonically decreasing component of the PPFs. For a given time, r is a constant.
For our proposed PPC control strategy, according to Theorem 4.1, we need to design ρ∞=2sπarctan(ν) to guarantee that the tracking error converges to the region |e|≤v. Therefore, the PPF defined in (3.2) can be rewritten as
ρ(t)=rρ0+(1−r)karctan(v) | (A2) |
where 0<k=2sπ<1.
The traditional formulation of normalized error in [37,38,39] can be written as
ξ1=eρ=erρ0+(1−r)v | (A3) |
In our proposed scheme, the new normalized error is defined as
ξ2=ηρ=karctan(e)rρ0+(1−r)karctan(v) | (A4) |
Letting f(e)=|ξ2|−|ξ1|=ξ2(|e|)−ξ1(|e|) yields
f=karctan(|e|)rρ0+(1−r)karctan(v)−|e|rρ0+(1−r)v=karctan(|e|)(rρ0+(1−r)v)−|e|(rρ0+(1−r)karctan(v))(rρ0+(1−r)karctan(v))(rρ0+(1−r)v) | (A5) |
Define g(|e|)=karctan(|e|)(rρ0+(1−r)v)−|e|(rρ0+(1−r)karctan(v)). Differentiating g with respect to e, we can obtain
˙g=sgn(e)k(rρ0+(1−r)v)√1+e2−sgn(e)(rρ0+(1−r)karctan(v))=sgn(e)k(rρ0+(1−r)v)−sgn(e)(rρ0+(1−r)karctan(v))√1+e2√1+e2 | (A6) |
When v→0, we have arctan(v)=v. Thus, ˙g can be rewritten as
˙g=sgn(e)rρ0(k−√1+e2)+sgn(e)k(1−r)v(1−√1+e2)√1+e2 | (A7) |
Given that 0<k<1, when e<0, it is obvious that ˙g>0. Similarly, when e>0, we can obtain ˙g<0. Therefore, we have that f(e)≤f(0)=0 for any e∈R. From this perspective, the absolute value of normalized error is reduced by our method. In addition, due to the property that the transformed function is monotonically increasing, a decrease in the transformed error and the control torque output can be achieved with the same error e.
[1] |
K. Xia, Y. Eun, T. Lee, S. Y. Park, Integrated adaptive control for spacecraft attitude and orbit tracking using disturbance observer, Int. J. Aeronaut. Space Sci., 22 (2021), 936–947. https://doi.org/10.1007/s42405-021-00359-x doi: 10.1007/s42405-021-00359-x
![]() |
[2] |
X. Chen, L. Zhao, Observer-based finite-time attitude containment control of multiple spacecraft systems, IEEE Trans. Circuits Syst. II Express Briefs, 68 (2020), 1273–1277. https://doi.org/10.1109/TCSII.2020.3024941 doi: 10.1109/TCSII.2020.3024941
![]() |
[3] |
R. Nadafi, M. Kabganian, Robust nonlinear attitude tracking control of an underactuated spacecraft under saturation and time-varying uncertainties, Eur. J. Control, 63 (2022), 133–142. https://doi.org/10.1016/j.ejcon.2021.09.003 doi: 10.1016/j.ejcon.2021.09.003
![]() |
[4] |
Q. Hu, B. Li, M. I. Friswell, Observer-based fault diagnosis incorporating online control allocation for spacecraft attitude stabilization under actuator failures, J. Astronaut. Sci., 60 (2013), 211–236. https://doi.org/10.1007/s40295-014-0021-1 doi: 10.1007/s40295-014-0021-1
![]() |
[5] |
M. Z. Dai, B. Xiao, C. Zhang, J. Wu, Event-triggered policy to spacecraft attitude stabilization with actuator output nonlinearities, IEEE Trans. Circuits Syst. II Express Briefs, 68 (2021), 2855–2859. https://doi.org/10.1109/TCSII.2021.3056761 doi: 10.1109/TCSII.2021.3056761
![]() |
[6] |
Q. Liu, M. Liu, G. Duan, Adaptive fuzzy backstepping control for attitude stabilization of flexible spacecraft with signal quantization and actuator faults, Sci. China Inf. Sci., 64 (2021), 1–16. https://doi.org/10.1007/s11432-020-2949-5 doi: 10.1007/s11432-020-2949-5
![]() |
[7] |
S. P. Bhat, D. S. Bernstein, Finite-time stability of continuous autonomous systems, SIAM J. Control Optim., 38 (2000), 751–766. https://doi.org/10.1137/S0363012997321358 doi: 10.1137/S0363012997321358
![]() |
[8] |
Z. Xiong, X. Li, M. Ye, Q. Zhang, Finite-time stability and optimal control of an impulsive stochastic reaction-diffusion vegetation-water system driven by lévy process with time-varying delay, Math. Biosci. Eng., 18 (2021), 8462–8498. https://doi.org/10.3934/mbe.2021419 doi: 10.3934/mbe.2021419
![]() |
[9] |
Y. Wang, B. Zhu, H. Zhang, W. X. Zheng, Functional observer-based finite-time adaptive ismc for continuous systems with unknown nonlinear function, Automatica, 125 (2021), 109468. https://doi.org/10.1016/j.automatica.2020.109468 doi: 10.1016/j.automatica.2020.109468
![]() |
[10] |
F. Wang, B. Chen, Y. Sun, Y. Gao, C. Lin, Finite-time fuzzy control of stochastic nonlinear systems, IEEE Trans. Cybern., 50 (2019), 2617–2626. https://doi.org/10.1109/TCYB.2019.2925573 doi: 10.1109/TCYB.2019.2925573
![]() |
[11] |
A. Polyakov, Nonlinear feedback design for fixed-time stabilization of linear control systems, IEEE Trans. Autom. Control, 57 (2011), 2106–2110. https://doi.org/10.1109/TAC.2011.2179869 doi: 10.1109/TAC.2011.2179869
![]() |
[12] |
M. L. Zhuang, S. M. Song, Fixed-time coordinated attitude tracking control for spacecraft formation flying considering input amplitude constraint, Int. J. Control Autom. Syst., 20 (2022), 2129–2147. https://doi.org/10.1007/s12555-021-0366-8 doi: 10.1007/s12555-021-0366-8
![]() |
[13] |
S. M. Esmaeilzadeh, M. Golestani, A. Fekih, Adaptive attitude stabilization of flexible spacecraft with fast fixed-time convergence, Iran. J. Sci. Technol. Trans. Mech. Eng., 46 (2022), 195–208. https://doi.org/10.1007/s40997-020-00415-z doi: 10.1007/s40997-020-00415-z
![]() |
[14] |
S. Kang, P. X. Liu, H. Wang, Fixed-time adaptive fuzzy command filtering control for a class of uncertain nonlinear systems with input saturation and dead zone, Nonlinear Dyn., 110 (2022), 2401–2414. https://doi.org/10.1007/s11071-022-07731-w doi: 10.1007/s11071-022-07731-w
![]() |
[15] |
H. Yang, D. Ye, Adaptive fixed-time bipartite tracking consensus control for unknown nonlinear multi-agent systems: An information classification mechanism, Inf. Sci., 459 (2018), 238–254. https://doi.org/10.1016/j.ins.2018.04.016 doi: 10.1016/j.ins.2018.04.016
![]() |
[16] |
L. Zhao, J. Yu, X. Chen, Neural-network-based adaptive finite-time output feedback control for spacecraft attitude tracking, IEEE Trans. Neural Networks Learn. Syst., 2022 (2022), 1–8. https://doi.org/10.1109/TNNLS.2022.3144493 doi: 10.1109/TNNLS.2022.3144493
![]() |
[17] |
X. Liu, D. Tong, Q. Chen, W. Zhou, K. Liao, Observer-based adaptive nn tracking control for nonstrict-feedback systems with input saturation, Neural Process. Lett., 53 (2021), 3757–3781. https://doi.org/10.1007/s11063-021-10575-x doi: 10.1007/s11063-021-10575-x
![]() |
[18] |
E. Jiménez-Rodríguez, J. D. Sánchez-Torres, A. G. Loukianov, Predefined-time backstepping control for tracking a class of mechanical systems, IFAC-PapersOnLine, 50 (2017), 1680–1685. https://doi.org/10.1016/j.ifacol.2017.08.492 doi: 10.1016/j.ifacol.2017.08.492
![]() |
[19] |
S. Xie, Q. Chen, Adaptive nonsingular predefined-time control for attitude stabilization of rigid spacecrafts, IEEE Trans. Circuits Syst. II Express Briefs, 69 (2021), 189–193. https://doi.org/10.1109/TCSII.2021.3078708 doi: 10.1109/TCSII.2021.3078708
![]() |
[20] |
J. Ni, L. Liu, Y. Tang, C. Liu, Predefined-time consensus tracking of second-order multiagent systems, IEEE Trans. Syst. Man Cybern. Syst., 51 (2021), 2550–2560. https://doi.org/10.1109/TSMC.2019.2916257 doi: 10.1109/TSMC.2019.2916257
![]() |
[21] |
E. Jiménez-Rodríguez, A. J. Muñoz-Vázquez, J. D. Sánchez-Torres, M. Defoort, A. G. Loukianov, A lyapunov-like characterization of predefined-time stability, IEEE Trans. Autom. Control, 65 (2020), 4922–4927. https://doi.org/10.1109/TAC.2020.2967555 doi: 10.1109/TAC.2020.2967555
![]() |
[22] |
C. P. Bechlioulis, G. A. Rovithakis, Robust adaptive control of feedback linearizable mimo nonlinear systems with prescribed performance, IEEE Trans. Autom. Control, 53 (2008), 2090–2099. https://doi.org/10.1109/TAC.2008.929402 doi: 10.1109/TAC.2008.929402
![]() |
[23] |
C. Wei, J. Luo, H. Dai, G. Duan, Learning-based adaptive attitude control of spacecraft formation with guaranteed prescribed performance, IEEE Trans. Cybern., 49 (2019), 4004–4016. https://doi.org/10.1109/TCYB.2018.2857400 doi: 10.1109/TCYB.2018.2857400
![]() |
[24] |
C. Zhang, G. Ma, Y. Sun, C. Li, Prescribed performance adaptive attitude tracking control for flexible spacecraft with active vibration suppression, Nonlinear Dyn., 96 (2019), 1909–1926. https://doi.org/10.1007/s11071-019-04894-x doi: 10.1007/s11071-019-04894-x
![]() |
[25] |
L. Zhang, S. Xu, X. Ju, N. Cui, Flexible satellite control via fixed-time prescribed performance control and fully adaptive component synthesis vibration suppression, Nonlinear Dyn., 100 (2020), 3413–3432. https://doi.org/10.1007/s11071-020-05662-y doi: 10.1007/s11071-020-05662-y
![]() |
[26] |
S. Gao, X. Liu, Y. Jing, G. M. Dimirovski, A novel finite-time prescribed performance control scheme for spacecraft attitude tracking, Aerosp. Sci. Technol., 118 (2021), 107044. https://doi.org/10.1016/j.ast.2021.107044 doi: 10.1016/j.ast.2021.107044
![]() |
[27] |
R. Chen, Z. Wang, W. Che, Adaptive sliding mode attitude-tracking control of spacecraft with prescribed time performance, Mathematics, 10 (2022), 401. https://doi.org/10.3390/math10030401 doi: 10.3390/math10030401
![]() |
[28] |
X. Bu, B. Jiang, H. Lei, Performance guaranteed finite-time non-affine control of waverider vehicles without function-approximation, IEEE Trans. Intell. Transp. Syst., 2022 (2022), 1–11. https://doi.org/10.1109/TITS.2022.3224424 doi: 10.1109/TITS.2022.3224424
![]() |
[29] |
X. Bu, B. Jiang, H. Lei, Nonfragile quantitative prescribed performance control of waverider vehicles with actuator saturation, IEEE Trans. Aerosp. Electron. Syst., 58 (2022), 3538–3548. https://doi.org/10.1109/TAES.2022.3153429 doi: 10.1109/TAES.2022.3153429
![]() |
[30] |
X. Bu, Q. Qi, B. Jiang, A simplified finite-time fuzzy neural controller with prescribed performance applied to waverider aircraft, IEEE Trans. Fuzzy Syst., 30 (2022), 2529–2537. https://doi.org/10.1109/TFUZZ.2021.3089031 doi: 10.1109/TFUZZ.2021.3089031
![]() |
[31] |
C. Qian, W. Lin, Non-lipschitz continuous stabilizers for nonlinear systems with uncontrollable unstable linearization, Syst. Control Lett., 42 (2001), 185–200. https://doi.org/10.1016/S0167-6911(00)00089-X doi: 10.1016/S0167-6911(00)00089-X
![]() |
[32] |
Y. Sun, L. Zhang, Fixed-time adaptive fuzzy control for uncertain strict feedback switched systems, Inf. Sci., 546 (2021), 742–752. https://doi.org/10.1016/j.ins.2020.08.059 doi: 10.1016/j.ins.2020.08.059
![]() |
[33] | G. H. Hardy, J. E. Littlewood, G. Pólya, Inequalities, Cambridge university press, 1952. |
[34] |
B. Xiao, S. Yin, Velocity-free fault-tolerant and uncertainty attenuation control for a class of nonlinear systems, IEEE Trans. Ind. Electron., 63 (2016), 4400–4411. https://doi.org/10.1109/TIE.2016.2532284 doi: 10.1109/TIE.2016.2532284
![]() |
[35] |
M. Golestani, S. M. Esmaeilzadeh, S. Mobayen, Fixed-time control for high-precision attitude stabilization of flexible spacecraft, Eur. J. Control., 57 (2021), 222–231. https://doi.org/10.1016/j.ejcon.2020.05.006 doi: 10.1016/j.ejcon.2020.05.006
![]() |
[36] |
Q. Hu, B. Chi, M. R. Akella, Anti-unwinding attitude control of spacecraft with forbidden pointing constraints, J. Guid. Control Dyn., 42 (2019), 822–835. https://doi.org/10.2514/1.G003606 doi: 10.2514/1.G003606
![]() |
[37] |
H. Sai, Z. Xu, C. Xia, X. Sun, Approximate continuous fixed-time terminal sliding mode control with prescribed performance for uncertain robotic manipulators, Nonlinear Dyn., 110 (2022), 431–448. https://doi.org/10.1007/s11071-022-07650-w doi: 10.1007/s11071-022-07650-w
![]() |
[38] |
M. Golestani, S. M. Esmaeilzadeh, B. Xiao, Fault-tolerant attitude control for flexible spacecraft subject to input and state constraint, Trans. Inst. Meas. Control, 42 (2020), 2660–2674. https://doi.org/10.1177/0142331220923780 doi: 10.1177/0142331220923780
![]() |
[39] |
P. Yang, Y. Su, Proximate fixed-time prescribed performance tracking control of uncertain robot manipulators, IEEE/ASME Trans. Mechatron., 27 (2022), 3275–3285. https://doi.org/10.1109/TMECH.2021.3107150 doi: 10.1109/TMECH.2021.3107150
![]() |
1. | Yuhan Su, Shaoping Shen, Adaptive global prescribed performance control for rigid spacecraft subject to angular velocity constraints and input saturation, 2023, 111, 0924-090X, 21691, 10.1007/s11071-023-08979-6 | |
2. | Zikun Hu, Shaopin Shen, Yuhan Su, Zhibin Li, 2024, Dc Motor Control: A Global Prescribed Performance Control Strategy Based on Extended State Observer, 979-8-3503-8778-0, 3490, 10.1109/CCDC62350.2024.10587568 | |
3. | Jing Wang, Wei Zhao, Jinde Cao, Ju H. Park, Hao Shen, Reinforcement Learning-Based Predefined-Time Tracking Control for Nonlinear Systems Under Identifier-Critic–Actor Structure, 2024, 54, 2168-2267, 6345, 10.1109/TCYB.2024.3431670 | |
4. | Yue Sun, Youmin Gong, Jie Mei, Yanning Guo, Guangfu Ma, Weiren Wu, Collision-Free Approximate Optimal Control of Spacecraft Formation With Predefined Performance, 2025, 61, 0018-9251, 4192, 10.1109/TAES.2024.3501235 |