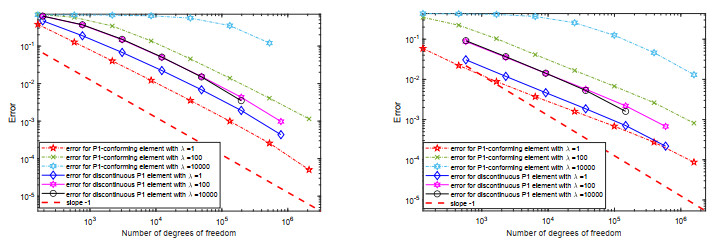
Since the COVID-19 epidemic, mathematical and simulation models have been extensively utilized to forecast the virus's progress. In order to more accurately describe the actual circumstance surrounding the asymptomatic transmission of COVID-19 in urban areas, this research proposes a model called Susceptible-Exposure-Infected-Asymptomatic-Recovered-Quarantine in a small-world network. In addition, we coupled the epidemic model with the Logistic growth model to simplify the process of setting model parameters. The model was assessed through experiments and comparisons. Simulation results were analyzed to explore the main factors affecting the spread of the epidemic, and statistical analysis that was applied to assess the model's accuracy. The results are consistent well with epidemic data from Shanghai, China in 2022. The model can not only replicate the real virus transmission data, but also anticipate the development trend of the epidemic based on available data, so that health policy-makers can better understand the spread of the epidemic.
Citation: Qinghua Liu, Siyu Yuan, Xinsheng Wang. A SEIARQ model combine with Logistic to predict COVID-19 within small-world networks[J]. Mathematical Biosciences and Engineering, 2023, 20(2): 4006-4017. doi: 10.3934/mbe.2023187
[1] | Justin W. L. Wan . Multigrid method for pricing European options under the CGMY process. AIMS Mathematics, 2019, 4(6): 1745-1767. doi: 10.3934/math.2019.6.1745 |
[2] | Shixian Ren, Yu Zhang, Ziqiang Wang . An efficient spectral-Galerkin method for a new Steklov eigenvalue problem in inverse scattering. AIMS Mathematics, 2022, 7(5): 7528-7551. doi: 10.3934/math.2022423 |
[3] | Song Lunji . A High-Order Symmetric Interior Penalty Discontinuous Galerkin Scheme to Simulate Vortex Dominated Incompressible Fluid Flow. AIMS Mathematics, 2016, 1(1): 43-63. doi: 10.3934/Math.2016.1.43 |
[4] | Yones Esmaeelzade Aghdam, Hamid Mesgarani, Zeinab Asadi, Van Thinh Nguyen . Investigation and analysis of the numerical approach to solve the multi-term time-fractional advection-diffusion model. AIMS Mathematics, 2023, 8(12): 29474-29489. doi: 10.3934/math.20231509 |
[5] | Shuangbing Guo, Xiliang Lu, Zhiyue Zhang . Finite element method for an eigenvalue optimization problem of the Schrödinger operator. AIMS Mathematics, 2022, 7(4): 5049-5071. doi: 10.3934/math.2022281 |
[6] | Lingling Sun, Hai Bi, Yidu Yang . A posteriori error estimates of mixed discontinuous Galerkin method for a class of Stokes eigenvalue problems. AIMS Mathematics, 2023, 8(9): 21270-21297. doi: 10.3934/math.20231084 |
[7] | Cagnur Corekli . The SIPG method of Dirichlet boundary optimal control problems with weakly imposed boundary conditions. AIMS Mathematics, 2022, 7(4): 6711-6742. doi: 10.3934/math.2022375 |
[8] | Yanhua Gu . High-order numerical method for the fractional Korteweg-de Vries equation using the discontinuous Galerkin method. AIMS Mathematics, 2025, 10(1): 1367-1383. doi: 10.3934/math.2025063 |
[9] | Zuliang Lu, Xiankui Wu, Fei Huang, Fei Cai, Chunjuan Hou, Yin Yang . Convergence and quasi-optimality based on an adaptive finite element method for the bilinear optimal control problem. AIMS Mathematics, 2021, 6(9): 9510-9535. doi: 10.3934/math.2021553 |
[10] | Lanyin Sun, Kunkun Pang . Numerical solution of unsteady elastic equations with C-Bézier basis functions. AIMS Mathematics, 2024, 9(1): 702-722. doi: 10.3934/math.2024036 |
Since the COVID-19 epidemic, mathematical and simulation models have been extensively utilized to forecast the virus's progress. In order to more accurately describe the actual circumstance surrounding the asymptomatic transmission of COVID-19 in urban areas, this research proposes a model called Susceptible-Exposure-Infected-Asymptomatic-Recovered-Quarantine in a small-world network. In addition, we coupled the epidemic model with the Logistic growth model to simplify the process of setting model parameters. The model was assessed through experiments and comparisons. Simulation results were analyzed to explore the main factors affecting the spread of the epidemic, and statistical analysis that was applied to assess the model's accuracy. The results are consistent well with epidemic data from Shanghai, China in 2022. The model can not only replicate the real virus transmission data, but also anticipate the development trend of the epidemic based on available data, so that health policy-makers can better understand the spread of the epidemic.
The Steklov spectrum coincides with that of the Dirichlet-to-Neumann map for the Laplacian (see, e.g., [1]), and the Steklov eigenvalue problem for the Laplace operator has been well-studied in the mathematical community. In linear elasticity, the study of the Dirichlet-to-Neumann map is important in elastostatic problems, and has attracted the attention of scholars (see, e.g., [2,3,4]). In 2021, Domˊinguez [5] first introduced the Steklov-Lamé eigenvalue problem in which the spectral parameter appears on a Robin boundary condition. [5] investigated the existence of the countable spectrum of this problem and studied the conforming finite element methods for the Steklov Lamé problem. Later, Li and Bi [6] proposed a discontinuous finite element method for this problem and gave the a priori error estimates.
As we know, for numerical solutions of the problems in linear planar elasticity, standard conforming finite elements may suffer a deterioration in performance as the Lamˊe constant λ→∞, that is locking phenomenon (see [7,8]). To overcome the locking phenomenon, several numerical approaches have been developed including the p-version method [9], the PEERS method [10], the mixed method [11], the Galerkin least squares method [12], the nonconforming triangular elements [13,14] and the discontinuous finite element method [15,16,17], and so on.
On the other hand, based on standard finite element methods, people design many efficient discretization schemes/algorithms to get approximations with high accuracy or to reduce the computation costs. The finite element multigrid discretizations is one of such design approaches. This method benefits from the two-grid discretization scheme which was first proposed by Xu and Zhou [18,19]. The basic idea of the two-grid discretizations is to transform solving an eigenvalue problem on a fine grid into solving the eigenvalue problem on a coarse grid and solving a series of algebraic equations on the fine grid. This kind of method can save calculation time while keeping the accuracy of approximations, or improving the accuracy under the same degrees of freedom. So far, two-grid and multigrid finite element discretization schemes have been successfully applied to solving eigenvalue problems, such as elliptic eigenvalue problem [20], Steklov eigenvalue problem [21,22,23,24], biharmonic eigenvalue problem [25], semilinear elliptic eigenvalue problem [26], quantum eigenvalue problem [27], Stokes eigenvalue problem [28,29], Maxwell eigenvalue problem [30], 2m-order elliptic eigenvalue problem [31], etc.
At present, there is not much numerical research report on the Steklov-Lamé eigenproblem. In view of the characteristics of discontinuous finite element method (DGFEM) and multigrid discretizations and based on the work in [6,32], for the Steklov-Lamé eigenvalue problem we will design and analyze a multigrid discretization scheme of DGFEM based on the shifted-inverse iteration. The rest of this paper is organized as follows. In Section 2, the discontinuous finite element approximation of the Steklov-Lamé eigenvalue problem and its a prior error estimates are given. In Section 3, a multigrid discretization scheme of DGFEM based on the shifted-inverse iteration is established, and the error estimates of the proposed scheme is presented. Finally, in Section 4, an adaptive multigrid algorithm is provided coupled with some numerical experiment results. The numerical results show that our method is efficient and locking-free.
Before the discussion, let us specify some notations. Scalars are denoted by general letters, vectors are denoted by bold letters and tensors in bold Greek letters. For tensors σ,τ∈Rn×n, the double dot product notation σ:τ=tr(τTσ) where tr(⋅) denotes the trace of a tensor (sum of the main diagonal). This inner product induces the Frobenius norm for tensors which is denoted as ‖. Let H^s(\Omega) and H^s(\partial\Omega) be the usual Sobolev space with order s of scalar fields on \Omega and \partial\Omega , respectively, whereas for tensor fields we use the symbols {\boldsymbol H}^s(\Omega) and {\boldsymbol H}^s(\partial\Omega) and each element in {\boldsymbol H}^s belongs to H^s . The norm in H^s(\Omega) and {H}^s(\partial\Omega) are denoted by \|\cdot\|_{s} and \|\cdot\|_{s, \partial\Omega} , respectively, and the same symbols are also used for the norms in {\boldsymbol {H^s}}(\Omega) and {\boldsymbol H}^s(\partial\Omega) when there is no ambiguity. H^0(\partial\Omega) = L^2(\partial\Omega) . Throughout this paper, we use the letter C , with or without subscript, to denote a generic positive constant independent of the mesh size h and the Lamé parameters, which may not be the same at each occurrence. We use the symbol a\lesssim b to mean that a\leq C b .
Suppose that an isotropic and linearly elastic material occupies the region \Omega in \mathbb{R}^n (n = 2\; or\; 3) where \Omega is a bounded convex polygonal with Lipschitz continuous boundary \partial \Omega . Consider the following Steklov-Lamé eigenvalue problem: Find non-zero displacement vector {\boldsymbol u} and the frequencies \omega\in \mathbb{R} satisfying
\begin{align} \begin{cases} -div {\boldsymbol {\sigma}} ({\boldsymbol {u}}) = {\bf 0} \quad in \ \Omega, \\ {\boldsymbol {\sigma}} ({\boldsymbol {u}}){\boldsymbol {n}} = \omega p {\boldsymbol {u}} \quad on \ \partial \Omega, \end{cases} \end{align} | (2.1) |
where {\boldsymbol {n}} is the unit outward normal to \partial\Omega , {\boldsymbol {\sigma}} ({\boldsymbol {u}}) is the Cauchy stress tensor defined as
{\boldsymbol {\sigma}} ({\boldsymbol {u}}) = 2 \mu {\boldsymbol {\epsilon}} ({\boldsymbol {u}}) + \lambda tr({\boldsymbol {\epsilon}} ({\boldsymbol {u}})) {\boldsymbol {I}} , |
where \boldsymbol{I} \in \mathbb{R} ^{n \times n} is the identity matrix, {\boldsymbol {\epsilon (u)}} is the strain tensor given by
{\boldsymbol {\epsilon}} ({\boldsymbol {u}}) = \frac{1}{2} \left(\nabla {\boldsymbol {u}} + (\nabla {\boldsymbol {u}})^T \right), |
\nabla {\boldsymbol {u}} is the displacement gradient tensor, and \lambda \in \mathbb{R} and \mu > 0 are the Lamé parameters satisfying 0 < \mu_1 < \mu < \mu_2 and 0 < \lambda < \infty .
Suppose that the density of material p \in L^\infty (\partial \Omega) has positive lower bound on \partial \Omega .
Denote
{\bf{RM}}(\Omega): = \{{\boldsymbol {v}}\in {\boldsymbol {H}}^1(\Omega)| {\boldsymbol {v(x)}} = {\boldsymbol {a}}+{\boldsymbol {Bx}}, {\boldsymbol {a}}\in \mathbb{R}^n, {\boldsymbol {B}}\in \mathbb{R}^{n\times n}, {\boldsymbol {B}}^{T} = -{\boldsymbol {B}}, \boldsymbol{x}\in \Omega \}. |
It is obvious that 0 is an eigenvalue of (2.1) with the associated eigenfunction {\boldsymbol {u}}\in {\bf{RM}}(\Omega) (see [5]). To find non-zero eigenvalues of (2.1) , we adopt the following weak formulation: Seek (\kappa, {\boldsymbol {u}}) \in \mathbb{R} \times {\boldsymbol H}^1 (\Omega) such that
\begin{eqnarray} a({\boldsymbol {u}},{\boldsymbol {v}}) = \kappa b({\boldsymbol {u}},{\boldsymbol {v}}),\ \forall {\boldsymbol {v}} \in {\boldsymbol H}^1 (\Omega), \end{eqnarray} | (2.2) |
where \kappa = \omega + 1 ,
\begin{eqnarray} a({\boldsymbol {u}},{\boldsymbol {v}})&: = & \int _\Omega {\boldsymbol {\sigma}} ({\boldsymbol {u}}) : {\boldsymbol {\epsilon}} ({\boldsymbol {v}}) dx + \int_{\partial \Omega} p {\boldsymbol {u}} \cdot {\boldsymbol {v}} ds\\ & = & 2\mu \int _\Omega {\boldsymbol {\epsilon}} ({\boldsymbol {u}}) : {\boldsymbol {\epsilon}} ({\boldsymbol {v}}) dx + \lambda \int _\Omega (div {\boldsymbol {u}})(div {\boldsymbol {v}})dx + \int _{\partial \Omega} p {\boldsymbol {u}} \cdot {\boldsymbol {v}} ds,\ \forall {\boldsymbol {u}},{\boldsymbol {v}} \in {\boldsymbol H}^1 (\Omega), \qquad \\ b({\boldsymbol {u}},{\boldsymbol {v}})&: = & \int _{\partial \Omega} p {\boldsymbol {u}} \cdot {\boldsymbol {v}} ds,\ \forall {\boldsymbol {u}},{\boldsymbol {v}} \in {\boldsymbol H}^1 (\Omega). \end{eqnarray} |
Reference[5] proved that a(\cdot, \cdot) is a continuous and \boldsymbol H^1 -coercive bilinear form in \boldsymbol H^1(\Omega) , b(\cdot, \cdot) is bounded.
Without losing generality, we assume that p\equiv 1 in the rest of this paper. Denote \| {\boldsymbol {v}} \|_b = b({\boldsymbol {v}}, {\boldsymbol {v}})^{\frac{1}{2}} , then it is clear that \| \cdot \|_b = \| \cdot \|_{0, \partial\Omega} .
Let \mathcal{T}_h = \{ K \} be a shape-regular partition of \Omega , and h = \max \{ h_K: K \in \mathcal{T}_h\} is the diameter of \mathcal{T}_h where h_K is the diameter of element K . When n = 2 , K is a triangle and a tetrahedron when n = 3 . Let e\in \partial K be an edge/face of element K with diameter h_e , and let \Gamma_h = \Gamma_h ^i \cup \Gamma _h ^b where \Gamma_h ^i denotes the interior edges/faces set and \Gamma _h ^b denotes the set of edges/faces lying on the boundary \partial\Omega . In the following, when there is no confusion we always use {\boldsymbol {n}} to represent the unit outward normal on the boundary of \Omega or element K .
Define the broken Sobolev space:
{\boldsymbol {H}}^s(\mathcal{T}_h) = \{\boldsymbol{v}\in [L_2(\Omega)]^n:{\boldsymbol {v}}\mid_K \in [H ^s (K)]^n,\forall K \in \mathcal{T}_h \}. |
For any {\boldsymbol {v}}\in {\boldsymbol {H}}^s(\mathcal{T}_h) , define the jump [\![ {\boldsymbol {v}} ]\!] and the average \{{\boldsymbol {v}}\} on e as follows:
\begin{eqnarray} [\![ {\boldsymbol {v}} ]\!] = \begin{cases} {\boldsymbol {v}}^+-{\boldsymbol {v}}^- ,\quad e \in \Gamma _h ^i,\\ {\boldsymbol {v}}^+ \qquad \ ,\quad e \in \Gamma _h ^b, \end{cases} \{{\boldsymbol {v}}\} = \begin{cases} \frac{{\boldsymbol {v}}^+ + {\boldsymbol {v}}^-}{2} , \quad e \in \Gamma _h ^i,\\ {\boldsymbol {v}}^+ \quad \ ,\quad \ e \in \Gamma _h ^b, \end{cases} \end{eqnarray} |
where {\boldsymbol {v}}^+ = {\boldsymbol {v}} |_ {K^+}, {\boldsymbol {v}}^- = {\boldsymbol {v}} | _ {K^-} , e\in \partial K^+ \cap \partial K^- .
Define the DGFEM space:
\boldsymbol{S}^h = \{{\boldsymbol {v}}\in [L_2(\Omega)]^n:{\boldsymbol {v}} \mid _K \in [\mathcal{P} ^k (K)]^n,\forall K \in \mathcal{T}_h \}, |
where \mathcal{P} ^k (K) is the space of polynomials defined on K with degree less than or equal to k\geq 1 .
The DGFEM discretization for the problem (2.2) is to find (\kappa _h, {\boldsymbol u}_h) \in \mathbb{R} \times {\boldsymbol S}^h, {\boldsymbol u}_h \neq 0, \kappa _h = \omega _h + 1 , such that
\begin{eqnarray} a_h({\boldsymbol u}_h,{\boldsymbol v}_h) = \kappa _h b_h({\boldsymbol u}_h,{\boldsymbol v}_h),\ \forall {\boldsymbol v}_h \in {\boldsymbol S}^h , \end{eqnarray} | (2.3) |
where
\begin{eqnarray} a_h({\boldsymbol u}_h,{\boldsymbol v}_h) & = & 2\mu \left( \sum _{K \in \mathcal{T}_h} \int _K {\boldsymbol {\epsilon}} ({\boldsymbol u}_h): {\boldsymbol {\epsilon}} ({\boldsymbol v}_h) dx -\sum _{e \in \Gamma _h ^i} \int _e \{ {\boldsymbol {\epsilon}} ({\boldsymbol u}_h) {\boldsymbol {n}} \} \cdot [\![ {\boldsymbol v}_h ]\!] ds \right. \\ &&- \left. \sum _{e \in \Gamma _h ^i} \int _e \{ {\boldsymbol {\epsilon}} ({\boldsymbol v}_h) {\boldsymbol {n}} \} \cdot [\![ {\boldsymbol u}_h ]\!] ds + \sum _{e \in \Gamma _h ^i} \frac{\gamma _\mu}{h_e} \int _e [\![ {\boldsymbol u}_h ]\!] \cdot [\![ {\boldsymbol v}_h ]\!] ds \right) \\ &&+ \lambda \left( \sum _{K \in \mathcal{T}_h} \int _K (div {\boldsymbol u}_h)(div {\boldsymbol v}_h)dx - \sum _{e \in \Gamma _h ^i} \int _e \{ div {\boldsymbol u}_h \} [\![ {\boldsymbol v}_h \cdot {\boldsymbol {n}} ]\!] ds \right. \\ &&- \left. \sum _{e \in \Gamma _h ^i} \int _e \{ div {\boldsymbol v}_h \} [\![ {\boldsymbol u_h} \cdot {\boldsymbol {n}} ]\!] ds + \sum _{e \in \Gamma _h ^i} \frac{\gamma _\lambda}{h_e} \int _e [\![ {\boldsymbol u}_h \cdot {\boldsymbol {n}} ]\!] [\![ {\boldsymbol v}_h \cdot {\boldsymbol {n}} ]\!] ds \right) \\ &&+ \sum _{e \in \Gamma _h ^b} \int _e {\boldsymbol u}_h \cdot {\boldsymbol v}_h ds, \\ b_h ({\boldsymbol u}_h,{\boldsymbol v}_h) & = & \sum _{e \in \Gamma _h ^b} \int _e {\boldsymbol u}_h \cdot {\boldsymbol v}_h ds, \end{eqnarray} |
and the penalty constants \gamma_\mu, \; \gamma_\lambda are independent of the shape of K and h . The determination of \gamma_\mu and \gamma_\lambda is to ensure that (2.4) is valid. It is easy to see that the discretization (2.3) is symmetric which is called symmetric internal penalty method (SIPG) in DGFEM.
Define the DG norm:
\begin{eqnarray} \| {\boldsymbol u}_h \| _G ^2 & = & 2 \mu \sum _{K \in \mathcal{T}_h} \| {\boldsymbol {\epsilon}} ({\boldsymbol u}_h) \| _{0,K} ^2 + 2 \mu \sum _{e \in \Gamma _h ^i} \gamma _\mu h_e ^{-1} \| [\![ {\boldsymbol u}_h ]\!] \| _{0,e} ^2 + \lambda \sum _{K \in \mathcal{T}_h} \| div {\boldsymbol u}_h \| _{0,K} ^2 \\ &+& \lambda \sum _{e \in \Gamma _h ^i} \gamma _\lambda h_e ^{-1} \| [\![ {\boldsymbol u}_h \cdot {\boldsymbol {n}} ]\!] \| _{0,e} ^2 + \sum _{e \in \Gamma _h ^b} \| {\boldsymbol u}_h \| _{0,e} ^2, \end{eqnarray} |
and the energy-like norm:
\begin{eqnarray} \| {\boldsymbol u}_h \| _h ^2 & = & \| {\boldsymbol u}_h \| _G ^2 +2 \mu \sum _{e \in \Gamma _h ^i} h_e \| \{ {\boldsymbol {\epsilon}} ({\boldsymbol u}_h) {\boldsymbol {n}} \} \| _{0,e} ^2 + \lambda \sum _{e \in \Gamma _h ^i} h_e \| \{ div {\boldsymbol u}_h \} \| _{0,e} ^2. \end{eqnarray} |
From Lemma 4 in [33] we know that there exist constants C_{\mu} and C_{\lambda} , independent of h, h_{e}, \mu and \lambda , such that
\begin{eqnarray*} \label{eq6} \|h_{e}^{1 / 2} {\boldsymbol {\epsilon}}(\boldsymbol{v}) \boldsymbol{n}\|_{0,e}^{2} \leq C_{\mu}\|{\boldsymbol {\epsilon}}(\boldsymbol{v})\|_{0,K}^{2},\\ \label{eq7} \|h_{e}^{1/2} div \boldsymbol{v}\|_{0,e}^{2} \leq C_{\lambda}\|div \boldsymbol{v}\|_{0,K}^{2} . \end{eqnarray*} |
Then, for 0 < \beta < 1 , when \gamma _{\mu}\geq C_{\mu}/(1-\beta)^2 , \gamma _{\lambda}\geq C_{\lambda}/(1-\beta)^2 , the bilinear form a_h(\cdot, \cdot) is coercive on {\boldsymbol S}^h (see Lemma 2.2 in [6]):
\begin{eqnarray} \beta \| {\boldsymbol v}_h \| _G ^2 \leq a_h({\boldsymbol v}_h,{\boldsymbol v}_h), \; \; \; \forall {\boldsymbol v}_h \in {\boldsymbol S}^h. \end{eqnarray} | (2.4) |
Using Cauchy-Schwartz inequality, it is easy to prove that the bilinear form a_h(\cdot, \cdot) is continuous:
\begin{eqnarray} |a_h ({\boldsymbol {u}},{\boldsymbol {v}}) | \leq M \| {\boldsymbol {u}} \|_h \| {\boldsymbol {v}} \|_h , \quad \forall {\boldsymbol {u}},{\boldsymbol {v}} \in {\boldsymbol H}^{1+s}(\mathcal{T}_h),s > \frac{1}{2}. \label{a2.5} \end{eqnarray} |
In order to derive the convergence and the error estimates of DG approximations by using Babu \breve{s} ka-Osborn spectral approximation theory, we consider the following source problem associated with the eigenvalue problem (2.2): find {\boldsymbol {w}} \in {\boldsymbol H}^1 (\Omega) such that
\begin{array}{l} a({\boldsymbol {w}},{\boldsymbol {v}}) = b({\boldsymbol {f}},{\boldsymbol {v}}),\ \forall {\boldsymbol {v}} \in {\boldsymbol H}^1 (\Omega). \end{array} | (2.5) |
The DG approximation of (2.5) is to find {\boldsymbol w}_h \in {\boldsymbol S}^h such that
\begin{array}{l} a_h({\boldsymbol w}_h,{\boldsymbol v}_h) = b_h({\boldsymbol {f}},{\boldsymbol v}_h),\ \forall {\boldsymbol v}_h \in {\boldsymbol S}^h. \end{array} | (2.6) |
Since a(\cdot, \cdot) and a_h(\cdot, \cdot) are continuous and coercive on {\boldsymbol H}^1 (\Omega) and {\boldsymbol S}^h , respectively, b(\cdot, \cdot) and b_h(\cdot, \cdot) are bounded, from Lax-Milgram Theorem we know that (2.5) and (2.6) admit the unique solution {\boldsymbol {w}} and {\boldsymbol {w}}_h , respectively.
The following regularity estimates of the solution of (2.5) has been discussed in Lemma 3.1 of [6].
(1) Let {\boldsymbol {w}} be the solution of (2.5) . If {\boldsymbol {f}} \in {\boldsymbol {H}}^{r-\frac{1}{2}} (\partial \Omega) , then {\boldsymbol {w}} \in {\boldsymbol {H}}^{r+1}(\Omega) and
\begin{eqnarray} \| {\boldsymbol {w}} \| _{r+1} + \lambda \| div {\boldsymbol {w}} \| _{r} \leq C_R \| {\boldsymbol {f}} \| _{r-\frac{1}{2},\partial \Omega}, \label{a2.8} \end{eqnarray} |
where r = 1 when \Omega is a convex polygonal, and r can be large enough when \partial \Omega is sufficiently smooth;
(2) If {\boldsymbol {f}} \in {\boldsymbol {H}}^{-\frac{1}{2}} (\partial \Omega) , then {\boldsymbol {w}} \in {\boldsymbol {H}}^1 (\Omega) and
\begin{eqnarray} \| {\boldsymbol {w}} \| _{1} + \lambda \| div {\boldsymbol {w}} \| _{0} \leq C_R \| {\boldsymbol {f}} \| _{-\frac{1}{2},\partial \Omega}; \label{a2.9} \end{eqnarray} |
(3) If {\boldsymbol {f}} \in {\boldsymbol {L}}^{2} (\partial \Omega) , then {\boldsymbol {w}} \in {\boldsymbol {H}}^{1+\frac{1}{2}} (\Omega) and
\begin{eqnarray} \| {\boldsymbol {w}} \| _{1+\frac{1}{2}} + \lambda \| div {\boldsymbol {w}} \| _{\frac{1}{2}} \leq C_R \| {\boldsymbol {f}} \| _{0,\partial \Omega}, \end{eqnarray} | (2.7) |
where the constant C_R is independent of \mu and \lambda .
For any given {\boldsymbol {f}} \in {\boldsymbol L}^{2} (\partial \Omega) , from (2.7) we have {\boldsymbol {w}} \in {\boldsymbol {H}}^{1+r} (\Omega), r < \frac{1}{2} and r can be arbitrarily close to \frac{1}{2} , and
\begin{eqnarray} \| {\boldsymbol {w}} \| _{1+r} + \lambda \| div {\boldsymbol {w}} \| _{r} \leq C_R \| {\boldsymbol {f}} \| _{0,\partial \Omega} . \end{eqnarray} | (2.8) |
Let {\boldsymbol {w}} and {\boldsymbol w}_h be the solution of (2.5) and (2.6), respectively, then the SIPG approximation (2.6) of (2.5) is consistent (see Lemma 3.3 in [6]):
\begin{array}{l} a_h({\boldsymbol {w}}-{\boldsymbol w}_h,{\boldsymbol v}_h) = 0,\ \forall {\boldsymbol v}_h \in {\boldsymbol S}^h. \end{array} | (2.9) |
For the source problem (2.5), let {\boldsymbol {f}} \in {\boldsymbol L}^{2} (\partial \Omega) , define the solution operator \ A : {\boldsymbol L}^{2} (\partial \Omega) \rightarrow {\boldsymbol H}^{1} (\Omega) by
a(A {\boldsymbol {f}} ,{\boldsymbol {v}}) = b( {\boldsymbol {f}} ,{\boldsymbol {v}}) ,\ \forall {\boldsymbol {v}} \in {\boldsymbol H}^{1} (\Omega), |
and define the operator \ T : {\boldsymbol L}^{2} (\partial \Omega) \rightarrow {\boldsymbol L}^{2} (\partial \Omega) :
T{\boldsymbol {f}} = (A {\boldsymbol {f}} )^\prime , |
where \ ^\prime denotes the restriction on \ \partial\Omega . Then, (2.2) has the following equivalent operator form:
A {\boldsymbol {u}} = \frac{1}{\kappa}{\boldsymbol{u}}. |
Similarly, from (2.6) we can define the discrete solution operator \ A_h : {\boldsymbol L}^{2} (\partial \Omega) \rightarrow {\boldsymbol S}^{h} by
a_h(A_h {\boldsymbol {f}} ,{\boldsymbol {v}}) = b_h( {\boldsymbol {f}} ,{\boldsymbol {v}}) ,\ \forall {{\boldsymbol {v}}} \in {\boldsymbol {S}}^{h}, |
and the operator \ T_h : {\boldsymbol L}^{2} (\partial \Omega) \rightarrow \delta {\boldsymbol {S}}^{h}\subset {\boldsymbol L}^{2} (\partial \Omega) satisfying
T_h{\boldsymbol {f}} = (A_h {\boldsymbol {f}} )^\prime , |
where \ \delta {\boldsymbol {S}}^{h} is the restriction of {\boldsymbol {S}}^{h} on \partial\Omega . Then (2.3) has the following equivalent operator form:
A_h {\boldsymbol u}_{h} = \frac{1}{\kappa _h}{{\boldsymbol u}_{h}}. |
Denote \rho = \frac{1}{\kappa}, \rho_h = \frac{1}{\kappa_h} . In this paper, \kappa , \kappa_h and \rho , \rho_h are all called eigenvalues.
From the definition of A_h and (2.4) , noticing that \| \cdot \|_h and \| \cdot \|_G are equivalent on {\boldsymbol S}^{h} , we can deduce that
\begin{eqnarray} \| A_h{\boldsymbol {f}} \| _{h} ^2 \lesssim a_h(A_h{\boldsymbol {f}},A_h{\boldsymbol {f}}) = b_h({\boldsymbol {f}},A_h{\boldsymbol {f}})\lesssim \| {\boldsymbol {f}} \| _{0,\partial \Omega}\| A_h{\boldsymbol {f}} \| _{0,\partial \Omega} \lesssim \| {\boldsymbol {f}} \| _{0,\partial \Omega}\| A_h{\boldsymbol {f}} \| _{h}, \end{eqnarray} |
thus,
\begin{eqnarray} \| A_h{\boldsymbol {f}} \| _{h} \leqslant C \| {\boldsymbol {f}} \| _{0,\partial \Omega }\leq C \| {\boldsymbol {f}} \| _{h}. \end{eqnarray} | (2.10) |
Reference [6] gave the a priori error estimates of DG approximation of (2.5).
Theorem 2.1. For any given {\boldsymbol {f}} \in {\boldsymbol {L}}^{2}(\partial \Omega) , let {\boldsymbol {w}}\in {\boldsymbol {H}}^{1+r}(\Omega)(0 < r < \frac{1}{2}) be the solution of (2.5) , and let {\boldsymbol w}_h be the solution of (2.6) . Assume that the regularity estimate (2.8) is valid, then there hold
\begin{eqnarray*} \label{a2.14} \| {\boldsymbol w} - {\boldsymbol w}_h \|_G \lesssim h^r \| {\boldsymbol {f}} \|_{0,\partial\Omega} ,\\ \label{a2.15} \| {\boldsymbol w} - {\boldsymbol w}_h \|_{0,\partial \Omega} \lesssim h^{2r} \| {\boldsymbol {f}} \|_{0,\partial\Omega}; \end{eqnarray*} |
Further, when \ {\boldsymbol {w}} \in {\boldsymbol {H}}^{1+s}(\Omega)(\frac{1}{2} < s \leq k) , there hold
\begin{eqnarray*} \label{a2.17} \| {\boldsymbol w} - {\boldsymbol w}_h \|_h \lesssim h^r \left( \sqrt{2\mu} \|{\boldsymbol {w}}\|_{1+r} + \sqrt{\lambda} \| div{\boldsymbol {w}}\|_{r} \right) ,\\ \label{a2.16} \| {\boldsymbol w} - {\boldsymbol w}_h \|_{0,\partial \Omega} \lesssim h^{r+s} \left( \sqrt{2\mu} \|{\boldsymbol {w}}\|_{1+s} + \sqrt{\lambda} \| div{\boldsymbol {w}}\|_{s} \right) . \end{eqnarray*} |
Proof. See Theorems 3.6–3.8 in [6].
Suppose that \kappa is the j th eigenvalue of (2.2) with algebraic multiplicity q , i.e., \kappa = \kappa_j = \kappa_{j+1} = \cdot \cdot \cdot = \kappa_{j+q-1} . [5] proved that \|T - T_h \|_{0, \partial \Omega} \rightarrow 0 when h \rightarrow 0 , therefore, q eigenvalues \kappa_{j, h}, \kappa_{j+1, h}, \cdots, \kappa_{j+q-1, h} of (2.3) will converge to \kappa . Let M(\kappa) be the space of eigenfunctions of (2.2) associated with eigenvalue \kappa , and M_h(\kappa) be the direct sum of the generalized eigenspace of (2.3) associated with \kappa_h that converge to \kappa , M(\rho) = M(\kappa) and M_h(\rho) = M_h(\kappa) . From [34] we have the following error estimates.
Theorem 2.2. Assume that the regularity estimate (2.8) is valid, and let M(\kappa) \subset {\boldsymbol {H}}^{1+s}(\Omega)(\frac{1}{2} < s), t = \min\{k, s\} , then there holds
\begin{eqnarray} | \kappa - \kappa_h | \lesssim h^{2t}; \end{eqnarray} | (2.11) |
Let {\boldsymbol u}_h \in M_h(\kappa) be an eigenfunction of (2.3) , then there exists {\boldsymbol {u}} \in M(\kappa) such that
\begin{eqnarray} \| {\boldsymbol {u}} - {\boldsymbol u}_h \|_{0,\partial \Omega} & \lesssim & h^{r+t}, \end{eqnarray} | (2.12) |
\begin{eqnarray} \| {\boldsymbol {u}} - {\boldsymbol u}_h \|_h & \lesssim & h^{t}; \end{eqnarray} | (2.13) |
Let {\boldsymbol {u}} \in {M}(\kappa) be an eigenfunction of (2.2) , then there exists {\boldsymbol u}_h \in M_h(\kappa) such that
\begin{eqnarray} \| {\boldsymbol {u}} - {\boldsymbol u}_h \|_h & \lesssim & h^{t}. \end{eqnarray} | (2.14) |
Proof. See Theorem 3.10 in [6] for the proofs of (2.11)–(2.13). By similar arguments we can get (2.14).
Let \{ \mathcal{T}_{h_i} \}^l_0 be a family of regular meshes of \Omega , h_{i-1}\gg h_i , and let {\boldsymbol {S}}^{h_i} be the DG space defined on \mathcal{T}_{h_i} . Denote \mathcal{T}_H = \mathcal{T}_{h_{0}}, {\boldsymbol {S}}^{H} = {\boldsymbol {S}}^{h_{0}} . Now, for the eigenvalue problem (2.3) we give the following multigrid discretization scheme of DGFEM based on the shifted inverse iteration.
Scheme 3.1. Given the iteration times \ l\ .
Step 1: Solve (2.3) on {\boldsymbol {S}}^{H} : Find (\kappa_{H}, {{\boldsymbol {u}}_{H}})\in \mathbb{R} \times {\boldsymbol {S}}^H, such that \| {{\boldsymbol {u}}_{H}} \|_{0, \partial\Omega} = 1 and
\begin{eqnarray*} a_H({{\boldsymbol {u}}_{H}},{{\boldsymbol {v}}}) = \kappa_H b_H({{\boldsymbol {u}}_{H}},{\boldsymbol {v}}),\ \forall {{\boldsymbol {v}}} \in {\boldsymbol {S}}^{H} . \label{a3.10} \end{eqnarray*} |
Step 2: {{\boldsymbol {u}}^{h_0}} \Leftarrow {{\boldsymbol {u}}_{H}}, \kappa^{h_0} \Leftarrow \kappa_{H} , i \Leftarrow 1 .
Step 3: Solve a linear system on {{\boldsymbol {S}}^{h_i}} : Find {{\boldsymbol {u}}^\prime} \in {{\boldsymbol {S}}^{h_i}} such that
\begin{eqnarray*} a_{h_i}({{\boldsymbol {u}}^\prime},{{\boldsymbol {v}}})- \kappa^{h_{i-1}} b_{h_i}({{\boldsymbol {u}}^\prime},{{\boldsymbol {v}}}) = b_{h_i}({{\boldsymbol {u}}^{h_{i-1}}},{{\boldsymbol {v}}}) ,\ \forall {{\boldsymbol {v}}} \in {{\boldsymbol {S}}^{h_i}}. \label{a3.11} \end{eqnarray*} |
Set {{\boldsymbol {u}}^{h_i}} = \frac{{{\boldsymbol {u}}^\prime}}{\|{{\boldsymbol {u}}^\prime}\|_{0, \partial\Omega} }.
Step 4: Compute the Rayleigh quotient
\begin{eqnarray} \kappa^{h_{i}} = \frac{a_{h_i}({{\boldsymbol {u}}^{h_i}},{{\boldsymbol {u}}^{h_i}})}{b_{h_i}({{\boldsymbol {u}}^{h_i}},{{\boldsymbol {u}}^{h_i}})}. \end{eqnarray} |
Step 5: If i = l , then output (\kappa^{h_{l}}, {{\boldsymbol {u}}^{h_l}}) , stop; else, i \Leftarrow i+1 and return to Step 3.
Next we will conduct the error analysis on Scheme 3.1.
From (2.9) we define the projection operator P_h: {\boldsymbol {H}}^{1}(\Omega)+{\boldsymbol S}^{h} \rightarrow {\boldsymbol S}^{h} \subset {\boldsymbol L}^{2} (\partial \Omega) satifying
\begin{eqnarray} a_{h}({\boldsymbol {u}} - P_h{\boldsymbol {u}},{\boldsymbol v}_h) = 0,\quad\quad\forall {\boldsymbol v}_h \in {\boldsymbol S}^h. \end{eqnarray} | (3.1) |
Then, from (2.9) and (3.1) together with A {\boldsymbol {f}} = {\boldsymbol {w}}, A_h {\boldsymbol {f}} = {\boldsymbol w}_h , we can prove easily that A_h = P_h A .
We first give the following lemmas to prepare for the error analysis.
Lemma 3.1. Let (\kappa, {\boldsymbol {u}}) be an eigenpair of (2.2) , then for any {\boldsymbol {v}} \in {\boldsymbol {S}}^h and \| {\boldsymbol {v}} \|_b \neq 0 , the Rayleigh quotient R({\boldsymbol {v}}) = \frac{a_{h}({\boldsymbol {v}}, {\boldsymbol {v}})}{\| {\boldsymbol {v}} \|_b^2} satisfies
\begin{eqnarray} R({\boldsymbol {v}}) - \kappa = \frac{ a_{h}({\boldsymbol {v}}-{\boldsymbol {u}},{\boldsymbol {v}}-{\boldsymbol {u}})}{\| {\boldsymbol {v}} \|_b^2}- \kappa \frac{ \| {\boldsymbol {v}}-{\boldsymbol {u}}\|_b^2 }{\| {\boldsymbol {v}} \|_b^2}. \end{eqnarray} | (3.2) |
Proof. From (2.9) we have
\begin{eqnarray*} a_h({\boldsymbol {u}},{\boldsymbol {v}}) = b( \kappa {\boldsymbol {u}},{\boldsymbol {v}}) = b_h( \kappa {\boldsymbol {u}},{\boldsymbol {v}}),\; \; \; \forall {\boldsymbol {v}} \in {\boldsymbol {S}}^h, \end{eqnarray*} |
thus,
\begin{eqnarray} && a_h({\boldsymbol {v}} - {\boldsymbol {u}},{\boldsymbol {v}} - {\boldsymbol {u}}) - \kappa b( {\boldsymbol {v}} - {\boldsymbol {u}},{\boldsymbol {v}} - {\boldsymbol {u}}) \\ & = & a_h({\boldsymbol {v}},{\boldsymbol {v}}) - 2 a_h({\boldsymbol {u}},{\boldsymbol {v}}) + a_h({\boldsymbol {u}},{\boldsymbol {u}}) - \kappa b( {\boldsymbol {v}},{\boldsymbol {v}}) + 2 \kappa b( {\boldsymbol {u}},{\boldsymbol {v}}) - \kappa b( {\boldsymbol {u}},{\boldsymbol {u}}) \\ & = & a_h({\boldsymbol {v}},{\boldsymbol {v}}) - 2 b(\kappa {\boldsymbol {u}},{\boldsymbol {v}}) + a({\boldsymbol {u}},{\boldsymbol {u}}) - \kappa b( {\boldsymbol {v}},{\boldsymbol {v}}) + 2 \kappa b( {\boldsymbol {u}},{\boldsymbol {v}}) - \kappa b( {\boldsymbol {u}},{\boldsymbol {u}}) \\ & = & a_h({\boldsymbol {v}},{\boldsymbol {v}}) - \kappa b( {\boldsymbol {v}},{\boldsymbol {v}}), \end{eqnarray} |
dividing both sides by \| {\boldsymbol {v}} \|_b^2 we obtain (3.2).
Lemma 3.2. For any non-zero elements u, v in any normed linear space (V, \|\cdot\|) , it is valid that
\begin{eqnarray*} \|\frac{u}{\|u\|}-\frac{v}{\|v\|}\| \leq 2 \frac{\|u-v\|}{\|u\|} \ , \ \nonumber \|\frac{u}{\|u\|}-\frac{v}{\|v\|}\| \leq 2 \frac{\|u-v\|}{\|v\|} \ . \nonumber \end{eqnarray*} |
Proof. See Lemma 3.1 in [20].
Denote d = dim \boldsymbol{S}^h , dist(\boldsymbol{u}, {\boldsymbol {S}}^h) = \inf\limits_{\boldsymbol{v} \in \boldsymbol{S}^h} \|\boldsymbol{u}- \boldsymbol{v}\|_h. Referring to Lemma 4.1 in [20] we prove the following result which plays an important role in our analysis.
Lemma 3.3. Let (\rho_0, {{\boldsymbol {w}}_0}) be an approximation of the j th eigenpair (\rho, {{\boldsymbol {u}}}) of (2.2) where \rho_0 is not an eigenvalue of A_h , {{\boldsymbol {w}}_0} \in {{\boldsymbol {S}}^h}, \| {{\boldsymbol {w}}_0} \|_{0, \partial\Omega} = 1. And let {\boldsymbol {u}}_0 = \frac{A_{h} {\boldsymbol {w}}_0}{\|A_{h}{\boldsymbol {w}}_0\|_{0, \partial \Omega}} . Suppose that
(C1) \inf \limits_{{\boldsymbol {v}} \in M_{h}(\rho)} \|{\boldsymbol {w}}_0-{\boldsymbol {v}} \|_{0, \partial \Omega} \leq \frac{1}{2};
(C2)\; |\rho_0 -\rho| \leq \frac{\vartheta}{4}, |\rho_{m, h} -\rho_m| \leq \frac{\vartheta}{4}, m = j-1, j, j+q(m\neq 0), where \vartheta = \min\limits_{m \neq j} |\rho_m -\rho| is the separate constant of the eigenvalue \rho ;
(C3)\; {{\boldsymbol {u}}}^\prime \in {{\boldsymbol {S}}^h} and {{\boldsymbol {u}}^{h}} \in {{\boldsymbol {S}}}^h satisfy
\begin{eqnarray} (\rho_0 - A_h){\boldsymbol u}^\prime = {\boldsymbol u}_0, \quad {\boldsymbol u}^{h} = \frac{{\boldsymbol u}^\prime}{\|{\boldsymbol u}^\prime \|_{0,\partial\Omega}}. \end{eqnarray} | (3.3) |
Then
\begin{eqnarray*} dist({\boldsymbol u}^{h}, M_h(\rho)) \leq \frac{C}{\vartheta} \max\limits_{j \leq m \leq j+q-1} | \rho_0 - \rho_{m,h} | dist({\boldsymbol w}_0, M_h(\rho)). \label{a3.13} \end{eqnarray*} |
Proof. Let \{ {\boldsymbol u}_{m, h}\}^d_{m = 1} be eigenfunctions of A_h satisfying b({\boldsymbol u}_{m, h}, {\boldsymbol u}_{i, h}) = \delta_{m, i} . Then
\begin{eqnarray*} {\boldsymbol u}_{0} = \sum\limits_{m = 1}^{d}b({\boldsymbol u}_{0}, {\boldsymbol u}_{m, h}){\boldsymbol u}_{m, h}. \label{q3.6} \end{eqnarray*} |
Since \rho_0 is not an eigenvalue of A_h , from (3.3) we can get
\begin{eqnarray} \left(\rho_{0}-\rho_{j, h}\right) {\boldsymbol u}^{\prime} = \left(\rho_{0}-\rho_{j, h}\right)\left(\rho_{0}-A_{h}\right)^{-1} {\boldsymbol u}_{0} = \sum\limits_{m = 1}^{d} \frac{\rho_{0}-\rho_{j, h}}{\rho_{0}-\rho_{m, h}}b({\boldsymbol u}_{0}, {\boldsymbol u}_{m, h}) {\boldsymbol u}_{m, h} . \end{eqnarray} | (3.4) |
Using triangle inequality and the condition (C2) we derive
\begin{eqnarray*} \left|\rho_{0}-\rho_{j, h}\right| & \leq & \left|\rho_{0}-\rho\right|+\left|\rho-\rho_{j, h}\right| \leq \frac{\vartheta}{4}+\frac{\vartheta}{4} = \frac{\vartheta}{2} , \\ \left|\rho_{0}-\rho_{m, h}\right| & \geq & \left|\rho-\rho_{m}\right|-\left|\rho_{0}-\rho\right|-\left|\rho_{m}-\rho_{m, h}\right| \geq \vartheta-\frac{\vartheta}{4}-\frac{\vartheta}{4} = \frac{\vartheta}{2}, \end{eqnarray*} |
where m = j-1, j+q (m \neq 0) . Hence, we have
\begin{eqnarray} \left|\rho_{0}-\rho_{m, h}\right| \geq \frac{\vartheta}{2} \quad m \neq j, j+1, \cdots, j+q-1 . \end{eqnarray} | (3.5) |
Because the operator T_h is selfadjoint with respect to b(\cdot, \cdot) , in fact, for \forall {\boldsymbol {f}} \in {\boldsymbol L}^{2} (\partial \Omega) , from the symmetry of a_h(\cdot, \cdot) and b(\cdot, \cdot) and b(\cdot, \cdot) = b_h(\cdot, \cdot) we have b(T_h {\boldsymbol {f}}, {\boldsymbol {v_h}}) = b({\boldsymbol {v_h}}, T_h {\boldsymbol {f}}) = b_h({\boldsymbol {v_h}}, T_h {\boldsymbol {f}}) = a_h(T_h {\boldsymbol {v_h}}, T_h {\boldsymbol {f}}) = a_h(T_h {\boldsymbol {f}}, T_h {\boldsymbol {v_h}}) = b_h({\boldsymbol {f}}, T_h {\boldsymbol {v_h}}) = b({\boldsymbol {f}}, T_h {\boldsymbol {v_h}}) and A_{h} {\boldsymbol u}_{h} = \rho_{h} {\boldsymbol u}_{h} , then, for m = 1, 2, \cdots, d , there holds
\begin{eqnarray} b( T_{h} {\boldsymbol w}_{0}, {\boldsymbol u}_{m, h}) {\boldsymbol u}_{m, h} = b( {\boldsymbol w}_{0}, T_{h} {\boldsymbol u}_{m, h}) {\boldsymbol u}_{m, h} = b( {\boldsymbol w}_{0}, \rho_{m, h} {\boldsymbol u}_{m, h}) {\boldsymbol u}_{m, h} \\ = b({\boldsymbol w}_{0}, {\boldsymbol u}_{m, h}) \rho_{m, h} {\boldsymbol u}_{m, h} = b({\boldsymbol w}_{0}, {\boldsymbol u}_{m, h}) A_{h} {\boldsymbol u}_{m, h}. \end{eqnarray} | (3.6) |
Noticing that \{ {\boldsymbol u}_{m, h} \}^{j+q-1}_{m = j} is a standard orthogonal basis of M_h(\rho) with respect to the {\boldsymbol L}^2(\partial\Omega) inner product b(\cdot, \cdot) , from {\boldsymbol u}_{0} = \frac{A_{h} {\boldsymbol w}_{0}}{\|A_{h} {\boldsymbol w}_{0}\|_{0, \partial \Omega}} , (3.4), (3.6), (2.10) and (3.5) we deduce
\begin{eqnarray} && \|(\rho_{0}-\rho_{j, h} ) {\boldsymbol u}^{\prime}-\sum\limits_{m = j}^{j+q-1} \frac{\rho_{0}-\rho_{j, h}}{\rho_{0}-\rho_{m, h}} b({\boldsymbol u}_{0}, {\boldsymbol u}_{m, h}) {\boldsymbol u}_{m, h} \|_{h} \\ & = & \| \sum\limits_{m \neq j, j+1, \cdots, j+q-1} \frac{\rho_{0}-\rho_{j, h}}{\rho_{0}-\rho_{m, h}}b({\boldsymbol u}_{0}, {\boldsymbol u}_{m, h}) {\boldsymbol u}_{m, h} \|_{h} \\ & = & \frac{1}{\left\|A_{h} {\boldsymbol w}_{0}\right\|_{0, \partial \Omega}}\left\|\sum\limits_{m \neq j, j+1, \cdots, j+q-1} \frac{\rho_{0}-\rho_{j, h}}{\rho_{0}-\rho_{m, h}}b( A_{h}{\boldsymbol w}_{0}, {\boldsymbol u}_{m, h}) {\boldsymbol u}_{m, h}\right\|_{h} \\ & = & \frac{1}{\left\|A_{h} {\boldsymbol w}_{0}\right\|_{0, \partial \Omega}}\left\|\sum\limits_{m \neq j, j+1, \cdots, j+q-1} \frac{\rho_{0}-\rho_{j, h}}{\rho_{0}-\rho_{m, h}}b( T_{h} {\boldsymbol w}_{0}, {\boldsymbol u}_{m, h}) {\boldsymbol u}_{m, h}\right\|_{h} \\ & = & \frac{1}{\left\|A_{h} {\boldsymbol w}_{0}\right\|_{0, \partial \Omega}}\left\|\sum\limits_{m \neq j, j+1, \cdots, j+q-1} \frac{\rho_{0}-\rho_{j, h}}{\rho_{0}-\rho_{m, h}}b( {\boldsymbol w}_{0}, {\boldsymbol u}_{m, h}) A_{h} {\boldsymbol u}_{m, h}\right\|_{h} \\ & = & \frac{1}{\left\|A_{h} {\boldsymbol w}_{0}\right\|_{0, \partial \Omega}}\left\|A_{h}\left(\sum\limits_{m \neq j, j+1, \cdots, j+q-1} \frac{\rho_{0}-\rho_{j, h}}{\rho_{0}-\rho_{m, h}}b({\boldsymbol w}_{0}, {\boldsymbol u}_{m, h}) {\boldsymbol u}_{m, h}\right)\right\|_{h} \\ & \leq & \frac{C}{\left\|A_{h} {\boldsymbol w}_{0}\right\|_{0, \partial \Omega}}\left\|\sum\limits_{m \neq j, j+1, \cdots, j+q-1} \frac{\rho_{0}-\rho_{j, h}}{\rho_{0}-\rho_{m, h}}b({\boldsymbol w}_{0}, {\boldsymbol u}_{m, h}){\boldsymbol u}_{m, h}\right\|_{0, \partial \Omega} \\ & \leq & \frac{2 C}{\vartheta\left\|A_{h} {\boldsymbol w}_{0}\right\|_{0, \partial \Omega}}\left|\rho_{0}-\rho_{j, h}\right|\left(\sum\limits_{m \neq j, j+1, \cdots, j+q-1} b^2({\boldsymbol w}_{0}, {\boldsymbol u}_{m, h})\right)^{\frac{1}{2}} \\ & \leq & \frac{2 C}{\vartheta\left\|A_{h} {\boldsymbol w}_{0}\right\|_{0, \partial \Omega}}\left|\rho_{0}-\rho_{j, h}\right|\left\|{\boldsymbol w}_{0}-\sum\limits_{m = j}^{j+q-1}b( {\boldsymbol w}_{0}, {\boldsymbol u}_{m, h}) {\boldsymbol u}_{m, h}\right\|_{0, \partial \Omega} \\ & = & \frac{2 C}{\vartheta\left\|A_{h} {\boldsymbol w}_{0}\right\|_{0, \partial \Omega}}\left|\rho_{0}-\rho_{j, h}\right|\inf\limits_{{\boldsymbol v} \in M_{h}(\rho)}\left\|{\boldsymbol w}_{0}-{\boldsymbol v}\right\|_{0, \partial \Omega} \\ & \leq & \frac{2 C}{\vartheta\left\|A_{h} {\boldsymbol w}_{0}\right\|_{0, \partial \Omega}}\left|\rho_{0}-\rho_{j, h}\right| {\rm{dist}}\left({\boldsymbol w}_{0}, M_{h}(\rho)\right) \text {. } \end{eqnarray} | (3.7) |
Taking the norm on both sides of (3.4), and noting that {\boldsymbol u}_{0} = \frac{A_{h} {\boldsymbol w}_{0}}{\|A_{h} {\boldsymbol w}_{0}\|_{0, \partial \Omega}} , the condition (C1) and (3.6), we get
\begin{eqnarray} && \left\|\left(\rho_{0}-\rho_{j, h}\right) {\boldsymbol u}^{\prime}\right\|_{0, \partial \Omega} = \frac{1}{\left\|A_{h} {\boldsymbol w}_{0}\right\|_{0, \partial \Omega}}\left\|\sum\limits_{m = 1}^{d} \frac{\rho_{0}-\rho_{j, h}}{\rho_{0}-\rho_{m, h}}b( A_{h} {\boldsymbol w}_{0}, {\boldsymbol u}_{m, h}){\boldsymbol u}_{m, h}\right\|_{0, \partial \Omega} \\ && = \frac{1}{\left\|A_{h} {\boldsymbol w}_{0}\right\|_{0, \partial \Omega}}\left\|\sum\limits_{m = 1}^{d} \frac{\rho_{0}-\rho_{j, h}}{\rho_{0}-\rho_{m, h}}b( T_{h} {\boldsymbol w}_{0}, {\boldsymbol u}_{m, h}) {\boldsymbol u}_{m, h}\right\|_{0, \partial \Omega} \\ && = \frac{1}{\left\|A_{h} {\boldsymbol w}_{0}\right\|_{0, \partial \Omega}}\left(\sum\limits_{m = 1}^{d}\left(\frac{\rho_{0}-\rho_{j, h}}{\rho_{0}-\rho_{m, h}}b^{2}( {\boldsymbol w}_{0}, \rho_{m, h} {\boldsymbol u}_{m, h})\right)\right)^{\frac{1}{2}} \\ && \geq \frac{C}{\left\|A_{h} {\boldsymbol w}_{0}\right\|_{0, \partial \Omega}} \min _{j \leq m \leq j+q-1}\left|\frac{\rho_{0}-\rho_{j, h}}{\rho_{0}-\rho_{m, h}}\right|\left(\sum\limits_{m = j}^{j+q-1}b^{2}( {\boldsymbol w}_{0}, {\boldsymbol u}_{m, h})\right)^{\frac{1}{2}} \\ && = \frac{C}{\left\|A_{h} {\boldsymbol w}_{0}\right\|_{0, \partial \Omega}} \min _{j \leq m \leq j+q-1}\left|\frac{\rho_{0}-\rho_{j, h}}{\rho_{0}-\rho_{m, h}}\right|\left\|{\boldsymbol w}_{0}-\left({\boldsymbol w}_{0}-\sum\limits_{m = j}^{j+q-1}b({\boldsymbol w}_{0}, {\boldsymbol u}_{m, h}) {\boldsymbol u}_{m, h}\right)\right\|_{0, \partial \Omega} \\ && \geq \frac{C}{2\left\|A_{h} {\boldsymbol w}_{0}\right\|_{0, \partial \Omega}} \min _{j \leq m \leq j+q-1}\left|\frac{\rho_{0}-\rho_{j, h}}{\rho_{0}-\rho_{m, h}}\right| . \end{eqnarray} | (3.8) |
From (3.7) and (3.8) we derive
\begin{eqnarray*} && {\rm{dist}}\left({\boldsymbol u}^{h}, M_{h}(\rho)\right) = {\rm{dist}}\left({\rm{sign}}\left(\rho_{0}-\rho_{j, h}\right) {\boldsymbol u}^{h}, M_{h}(\rho)\right) \\ & \leq& \left\|{\rm{sign}}\left(\rho_{0}-\rho_{j, h}\right) {\boldsymbol u}^{h}-\frac{1}{\left\|\left(\rho_{0}-\rho_{j, h}\right) {\boldsymbol u}^{\prime}\right\|_{0, \partial \Omega}} \sum\limits_{m = j}^{j+q-1} \frac{\rho_{0}-\rho_{j, h}}{\rho_{0}-\rho_{m, h}}b({\boldsymbol u}_{0}, {\boldsymbol u}_{m, h}){\boldsymbol u}_{m, h}\right\|_{h} \\ & = & \left\|\frac{\left(\rho_{0}-\rho_{j, h}\right) {\boldsymbol u}^{\prime}}{\left\|\left(\rho_{0}-\rho_{j, h}\right) {\boldsymbol u}^{\prime}\right\|_{0, \partial \Omega}}-\frac{1}{\left\|\left(\rho_{0}-\rho_{j, h}\right) {\boldsymbol u}^{\prime}\right\|_{0, \partial \Omega}} \sum\limits_{m = j}^{j+q-1} \frac{\rho_{0}-\rho_{j, h}}{\rho_{0}-\rho_{m, h}}b({\boldsymbol u}_{0}, {\boldsymbol u}_{m, h}) {\boldsymbol u}_{m, h}\right\|_{h} \\ & \leq& \frac{2}{C}\left\|A_{h} {\boldsymbol w}_{0}\right\|_{0, \partial \Omega} \max _{j \leq m \leq j+q-1}\left|\frac{\rho_{0}-\rho_{m, h}}{\rho_{0}-\rho_{j, h}}\right|\left\|\left(\rho_{0}-\rho_{j, h}\right) {\boldsymbol u}^{\prime}-\sum\limits_{m = j}^{j+q-1} \frac{\rho_{0}-\rho_{j, h}}{\rho_{0}-\rho_{m, h}}b( {\boldsymbol u}_{0}, {\boldsymbol u}_{m, h}){\boldsymbol u}_{m, h}\right\|_{h} \\ & \leq& \frac{C}{\vartheta} \max _{j \leq m \leq j+q-1}\left|\rho_{0}-\rho_{m, h}\right| {\rm{dist}}\left({\boldsymbol w}_{0}, M_{h}(\rho)\right) . \end{eqnarray*} |
The proof is completed.
Now we can analyze the error of multigrid discretization scheme 3.1 by using Theorem 2.2 and Lemma 3.3. We first consider the case of l = 1 . Denote H = h_0, h = h_1 .
Theorem 3.1. Suppose that M(\kappa_j)\subset {\boldsymbol H}^{1+s}(\Omega)(s\geq r) , and t = \min\{k, s\} . Let (\kappa^{h}_j, {\boldsymbol u}^{h}_j) be an approximate eigenpair obtained by Scheme 3.1 ( l = 1 ) and H is sufficiently small, then there exists {\boldsymbol u}_j \in M(\kappa _j) such that
\begin{eqnarray} \left \|{\boldsymbol u}_{j}^{h} - {\boldsymbol u}_{j} \right \|_{h} &\leq & C ({H}^{3t}+{h}^{t}), \end{eqnarray} | (3.9) |
\begin{eqnarray} \left\|{\boldsymbol u}_{j}^{h}-{\boldsymbol u}_{j}\right\|_{0,\partial \Omega} &\leq & C ({H}^{3t}+{h}^{r+t}), \end{eqnarray} | (3.10) |
\begin{eqnarray} |\kappa^h_j - \kappa_j| &\leq& C \left({H}^{3t}+{h}^{t}\right)^{2}. \end{eqnarray} | (3.11) |
Proof. We will use Lemma 3.3 to complete the proof. Take \rho _0 = \frac{1}{\kappa _H}, {\boldsymbol w}_0 = {\boldsymbol u}_H and {\boldsymbol u}_0 = \frac{A_{h} {\boldsymbol u}_H}{\|A_{h} {\boldsymbol u}_H\|_{0, \partial\Omega}} . From (2.13) we know that there exists \bar{{\boldsymbol u}}\in M(\kappa_j) such that
\begin{eqnarray*} \left\|{\boldsymbol u}_{H}-\bar{{\boldsymbol u}}\right\|_{h} \leq C {H}^{t}. \end{eqnarray*} |
From the triangle inequality and (2.14) we have
\begin{eqnarray} dist({\boldsymbol u}_H, M_h(\kappa_j))&\leq&\left\|{\boldsymbol u}_{H}-\bar{{\boldsymbol u}}\right\|_{H}+ dist(\bar{{\boldsymbol u}},M_h(\kappa_j)) \\ &\leq& C ({H}^{t}+{h}^{t})\leq C {H}^{t}, \end{eqnarray} | (3.12) |
thus,
\begin{eqnarray*} \inf _{{\boldsymbol v} \in M_{h}(\kappa_j)}\left\|{\boldsymbol u}_{H}-{\boldsymbol v}\right\|_{0, \partial\Omega} \leq C {H}^{t}, \end{eqnarray*} |
when H is small enough, the condition (C1) in Lemma 3.3 is valid.
From (2.11) we get
\begin{eqnarray*} \left|\rho_{0}-\rho_{j}\right|& = &\frac{\left|\kappa_{H}-\kappa_{j}\right|}{|\kappa_{H} \kappa_{j}|} \leq C {H}^{2t} \leq \frac{\vartheta}{4};\\ \left|\rho_{m}-\rho_{m, h}\right|& = &\frac{\left|\kappa_{m, h}-\kappa_{m}\right|}{|\kappa_{m, h} \kappa_{m}|} \leq C {h}^{2t} \leq \frac{\vartheta}{4}, \quad m = j-1, j, \ldots, j+q, m \neq 0 , \end{eqnarray*} |
i.e., the condition (C2) in Lemma 3.3 holds.
From the definition of A_h we know that Step 3 in Scheme 3.1 is equivalent to the following:
\begin{equation*} a_{h}({\boldsymbol u}^{\prime}, {\boldsymbol v})-\kappa_{H} a_{h}(A_{h} {\boldsymbol u}^{\prime}, {\boldsymbol v}) = a_{h}(A_{h} {\boldsymbol u}_{H}, {\boldsymbol v}) \quad \forall {\boldsymbol v} \in {\boldsymbol S}^{h}, \end{equation*} |
and {\boldsymbol u}_{j}^{h} = \frac{{\boldsymbol u}^{\prime}}{\left\|{\boldsymbol u}^{\prime}\right\|_{0, \partial\Omega}} , i.e.,
\begin{equation*} \left(\kappa_{H}^{-1}-A_{h}\right) {\boldsymbol u}^{\prime} = \kappa_{H}^{-1} A_{h} {\boldsymbol u}_{H}, \quad {\boldsymbol u}_{j}^{h} = \frac{{\boldsymbol u}^{\prime}}{\left\|{\boldsymbol u}^{\prime}\right\|_{0,\partial\Omega}}. \end{equation*} |
Note that \kappa_{H}^{-1} A_{h} {\boldsymbol u}_{H} and {\boldsymbol u}_0 differ by only one constant, thus, Step 3 in Scheme 3.1 is equivalent to
\begin{equation*} \left(\kappa_{H}^{-1}-A_{h}\right) {\boldsymbol u}^{\prime} = {\boldsymbol u}_{0}, \quad {\boldsymbol u}_{j}^{h} = \frac{{\boldsymbol u}^{\prime}}{\left\|{\boldsymbol u}^{\prime}\right\|_{0,\partial\Omega}}. \end{equation*} |
So far, all conditions of Lemma 3.3 are valid.
Since M_{h}(\kappa_j) is a q -dimensional space, there must exist {\boldsymbol u}^{*}\in M_{h}(\kappa_j) such that
\begin{equation*} \left\|{\boldsymbol u}_{j}^{h}-{\boldsymbol u}^{*}\right\|_{h} = {\rm{dist}}\left({\boldsymbol u}_{j}^{h}, M_{h}(\kappa_{j})\right). \end{equation*} |
For m = j, j+1, \ldots, j+q-1 , according to (2.11) we have
\begin{eqnarray} \mid \rho_{0} -\rho_{m, h} \mid & = & | \frac{1}{\kappa_{H}}-\frac{1}{\kappa_{m, h}} | \leq \frac{\left|\kappa_{H}-\kappa_{m, h}\right|}{\left|\kappa_{H} \kappa_{m,h}\right|} \\ & \leq & C (|\kappa_{H}-\kappa_{j}|+|\kappa_{j}-\kappa_{m, h}|)\leq C {H}^{2t}. \end{eqnarray} | (3.13) |
Therefore, from Lemma 3.3, (3.12) and (3.13) we get
\begin{eqnarray} &&\|{\boldsymbol u}_{j}^{h}-{\boldsymbol u}^{*}\|_{h} = {\rm{dist}}\left({\boldsymbol u}_{j}^{h}, M_{h}(\kappa_{j})\right)\\ &\leq& \frac{C}{\vartheta} \max\limits_{j \leq m \leq j+q-1}\left|\rho_{0}-\rho_{m, h}\right| {\rm{dist}}\left({\boldsymbol u}_{H}, M_{h}(\kappa_{j})\right)\leq C {H}^{3t}. \end{eqnarray} | (3.14) |
From (2.13) we know that there exists {\boldsymbol u}_j\in M(\kappa_j) , such that \|{\boldsymbol u}^{*}-{\boldsymbol u}_j\|_{h} = {\rm{dist}}\left({\boldsymbol u}^{*}, M(\kappa_{j})\right) , and
\begin{equation*} \|{\boldsymbol u}^{*}-{\boldsymbol u}_j\|_{h}\leq C {h}^{t}, \end{equation*} |
then
\begin{equation*} \|{\boldsymbol u}_{j}^{h}-{\boldsymbol u}_j\|_{h}\leq \|{\boldsymbol u}_{j}^{h}-{\boldsymbol u}^{*}\|_{h}+\|{\boldsymbol u}^{*}-{\boldsymbol u}_j\|_{h}\leq C ({H}^{3t}+{h}^{t}), \end{equation*} |
that is (3.9).
Next, we will prove (3.10). From (2.12) we have
\begin{equation*} \|{\boldsymbol u}^{*}-{\boldsymbol u}_j\|_{0,\partial\Omega}\leq C {h}^{r+t}, \end{equation*} |
which together with (3.14) yields
\begin{equation*} \|{\boldsymbol u}_{j}^{h}-{\boldsymbol u}_j\|_{0,\partial\Omega}\leq \|{\boldsymbol u}_{j}^{h}-{\boldsymbol u}^{*}\|_{0,\partial\Omega}+\|{\boldsymbol u}^{*}-{\boldsymbol u}_j\|_{0,\partial\Omega}\leq C({H}^{3t} + {h}^{r+t}). \end{equation*} |
Finally, we use Lemma 3.1 to derive (3.11). From Step 4 of Scheme 3.1, Lemma 3.1, (3.9) and (3.10) we deduce that
\begin{eqnarray*} |\kappa^{h}_j-\kappa_j| & = & \left| \frac{a_h({\boldsymbol u}^{h}_{j}-{\boldsymbol u}_{j}, {\boldsymbol u}^{h}_{j}-{\boldsymbol u}_{j})}{\|{\boldsymbol u}_{j}^{h}\|^2_{b}}-\kappa_j\frac{b( {\boldsymbol u}^{h}_{j}-{\boldsymbol u}_{j}, {\boldsymbol u}^{h}_{j}-{\boldsymbol u}_{j})}{\|{\boldsymbol u}_{j}^{h}\|^2_{b}}\right| \\ &\leq& C \left(\|{\boldsymbol u}^{h}_{j}-{\boldsymbol u}_{j}\|^2_{h}+|\kappa_j|\|{\boldsymbol u}^{h}_{j}-{\boldsymbol u}_{j}\|^2_{0,\partial\Omega}\right)\\ &\leq& C\left( {H}^{3t} + {h}^{t} \right)^{2}. \end{eqnarray*} |
The proof is completed.
Remark 3.1. Using Theorem 3.1 and referring to Theorem 4.2 in [32], we can give the error estimates of Scheme 3.1. To ensure that the error is independent of the number of iterations in the multigrid refinement, we also need the following conditions.
Condition 3.1. For any given \varepsilon \in (0, 1) , there exists t_i\in (1, 2-\varepsilon] (i = 1, 2, \cdots) , such that h_{i} = O(h_{i-1}^{t_{i}}) , and h_i\rightarrow 0\; (i\rightarrow \infty).
Condition 3.1 is easy to be satisfied. For instance, for smooth eigenfunctions, using uniform meshes and linear elements and taking \varepsilon = 0.1, h_0 = \frac{\sqrt{2}}{8}, h_1 = \frac{\sqrt{2}}{32}, h_2 = \frac{\sqrt{2}}{128}, \cdots , then t_i = \frac{log(h_i)}{log(h_{i-1})} = \frac{log(h_{i-1}-log(4)}{log(h_{i-1})} , thus, t_1 \approx 1.80, t_2 \approx 1.44, t_3 \approx 1.31, \cdots , and t_{i}\searrow 1 when i\rightarrow \infty .
Theorem 3.2. Suppose that Condition 3.1 holds and M(\kappa_j)\subset H^{1+s}(\Omega)\; (s\geq r) , and t = \min\{k, s\} . Let (\kappa_j^{h_l}, {\boldsymbol u}_j^{h_l}) be an approximate eigenpair obtained by Scheme 3.1, then, when h_0 = H is small enough, there exists {\boldsymbol u}_j \in M(\kappa_j) such that
\begin{eqnarray} \left\|{\boldsymbol u}_{j}^{h_{l}}-{\boldsymbol u}_{j}\right\|_{h} &\leq& C {h_{l}^{t}} , \end{eqnarray} | (3.15) |
\begin{eqnarray} \left\|{\boldsymbol u}_{j}^{h_{l}}-{\boldsymbol u}_{j}\right\|_{0,\partial \Omega} &\leq& C {h_{l}^{r+t}}, \end{eqnarray} | (3.16) |
\begin{eqnarray} \left|\kappa_{j}^{h_{l}}-\kappa_{j}\right| &\leq& C {h_{l}^{2t}}, \quad l \geq 1 . \end{eqnarray} | (3.17) |
In this section, we will report some numerical experiments to show the efficiency of the DG-multigrid method (Scheme 3.1) for solving the Steklov-Lamé eigenproblem. We conduct the numerical experiments on the MATLAB 2022a on a ThinkBook 14p Gen 2 PC with 16G memory, and our program makes use of the package of iFEM [35]. The test domains are set to be the unit square \Omega_S = (0, 1)^2 and the L-shaped domain \Omega_L = (-1, 1)^2\backslash [0, 1)^2 .
Example 4.1. We use Scheme 3.1 to compute the approximation for the 1st eigenvalue \kappa_1 of the problem (2.2). We adopt piecewise polynomial of degree 1 ( P1 element) to compute on uniform isosceles right triangulations. We produce the initial coarse grid \mathcal{T}_{H} = \mathcal{T}_{h_0} and refine the coarse grid in a uniform way (each triangle is divided into four congruent triangles) repeatedly to obtain fine grids \mathcal{T}_{h_i}, i = 1, 2, ..., l . By using the basis functions of {\boldsymbol {S}}^{H} , the eigenvalue problem on the initial coarse grid in Step 1 of Scheme 3.1 can be rewritten as a generalized matrix eigenvalue problem
\begin{eqnarray} K^{H}\bar{\boldsymbol u}_{1,H} = \kappa_{1,H}M^{H}\bar{\boldsymbol u}_{1,H}, \end{eqnarray} | (4.1) |
where the elements of array \bar{\boldsymbol u}_{1, H} are the coordinates of \boldsymbol u_{1, H} under the basis functions in {\boldsymbol {S}}^{H} . Similarly, by using the basis functions of {\boldsymbol {S}}^{h_{i}} , the algebraic system in Step 3 of Scheme 3.1 can be rewritten as
\begin{eqnarray} (K^{h_{i}}- \kappa_{1}^{h_{i-1}}M^{h_{i}})\hat{\boldsymbol u} = M^{h_{i}}\hat{\boldsymbol u}_{1}^{h_{i-1}} \end{eqnarray} | (4.2) |
and \bar{\boldsymbol u}_{1}^{h_{i}} = \frac{\hat{\boldsymbol u}}{\sqrt{\hat{\boldsymbol u}^T M^{h_{i}}\hat{\boldsymbol u}}} where \hat{\boldsymbol u}_{1}^{h_{i-1}} is actually the projection of the solution \bar{\boldsymbol u}_{1}^{h_{i-1}} obtained on the previous grid \mathcal{T}_{h_{i-1}} in \mathcal{T}_{h_{i}} . For example, if \mathcal{T}_{h_{0}} contains NT = 2 elements with the associated solution \bar{{\boldsymbol u}}_1^{h_{0}} = \bar{\boldsymbol u}_{1, H} , denote \bar{{\boldsymbol u}}_{1}^{h_{0}} = [u_{1}^{h_{0}}, u_{2}^{h_{0}}]^T with u_{1}^{h_{0}} = [a_{11}, a_{21}, a_{12}, a_{22}, a_{13}, a_{23}] , u_{2}^{h_{0}} = [b_{11}, b_{21}, b_{12}, b_{22}, b_{13}, b_{23}] and a_{\iota j}, b_{\iota j}(j = 1, 2, 3) being the coordinates of the basis function \{1, x, y\} on the element \iota in \mathcal{T}_{h_{0}} , and encrypt \mathcal{T}_{h_{0}} once (each triangle is divided into four congruent triangles) to get \mathcal{T}_{h_{1}} which contains 4NT = 8 elements, then the projection of \bar{{\boldsymbol u}}_1^{h_{0}} in \mathcal{T}_{h_{1}} is as follows:
\hat{{\boldsymbol u}}_1^{h_{0}} = Q_1\bar{{\boldsymbol u}}_1^{h_{0}}, |
where Q_1 is the projection (restriction) operator:
Q_1 = \left [ {\begin{array}{cc} Q_{11} & 0 \\ 0 & Q_{12} \\ \end{array} } \right ] ,\; Q_{11} = Q_{12} = \left [ {\begin{array}{ccc} I_{NT}^4 & 0 & 0 \\ 0 &I_{NT}^4 & 0 \\ 0 &0 & I_{NT}^4 \\ \end{array} } \right ], \; I_{NT}^4 = \left [ {\begin{array}{c} I_{NT} \\ I_{NT} \\ I_{NT} \\ I_{NT} \\ \end{array} } \right ],\; I_{NT} = I_{2} = \left [ {\begin{array}{cc} 1 & 0 \\ 0 & 1 \\ \end{array} } \right ]. |
If bisecting encryption is used, that is, each triangle is divided into two triangles, then just replace I_{NT}^4 with I_{NT}^2 = \left [ {\begin{array}{c} I_{NT} \\ I_{NT} \\ \end{array} } \right ] . We use the command " {\mathtt{eigs}} " of MATLAB to solve the discrete algebraic eigenvalue problem (4.1), and use the command " \setminus " in MATLAB to solve the linear system (4.2). Further, there has no difficulty with solving the system (4.2) (see Lecture 27.4 in [36]).
For comparison, we also use the multigrid method of conforming finite elements by adopting P1 element to compute. The error curves are depicted in Figure 1 where the reference value are taken as the most accurate approximations that we can compute. From Figure 1, we can see that as the Lamé parameter \lambda increases, the DG-multigrid method is robust compared with the multigrid method of conforming finite elements, which is a major advantage of using DG method to solve elastic problems.
Example 4.2. Adaptive computation.
Adaptive algorithm based on the a posterior error estimation is an efficient and important numerical approach for solving partial differential equations. Referring to [37], we combine the multigrid scheme 3.1 and the a posteriori error indicator to establish the adaptive multigrid algorithm. Referring to the a posterior error indicator (25) for the linear elastic source problem in [37], we formally give the following local error indicator for the underlying eigenvalue problem:
\begin{eqnarray*} \zeta_{K}^2 (\kappa_{h},{{\boldsymbol {u}}}_h)& = &h_{K}^2\| div {\boldsymbol {\sigma}} ({\boldsymbol u}_{h}) \|_{L^2(K)}^2 + h_{K} \left( \| \Sigma_{{\boldsymbol {n}}}({{\boldsymbol {u}}}_h) - {{\boldsymbol {n}}} \cdot {\boldsymbol {\sigma}}({{\boldsymbol {u}}}_h) \|_{L^2({\partial K \setminus \partial\Omega})}^2 \right. \\ && +\left. \| \Sigma_{{\boldsymbol {n}}}({{\boldsymbol {u}}}_h) - {{\boldsymbol {n}}} \cdot {\bf \boldsymbol \sigma}({{\boldsymbol {u}}}_h) \|_{L^2({\partial K \cap \partial\Omega})}^2 \right)+ h_K^{-1} \| [\![ {{\boldsymbol {u}}}_h ]\!] \|_{L^2({\partial K \backslash \partial\Omega})}^2 , \end{eqnarray*} |
where
\begin{eqnarray*} \Sigma_{{\boldsymbol {n}}}({{\boldsymbol {u}}}_h)&: = & \left \{ \begin{array}{ll}{{\boldsymbol {n}}} \cdot \{ {\bf \boldsymbol \sigma}({{\boldsymbol {u}}}_h) \} - \gamma_{\mu}h_{e}^{-1} [\![ {{\boldsymbol {u}}}_h ]\!] - h_{e}^{-1} \gamma_{\lambda} {{\boldsymbol {n}}}({{\boldsymbol {n}}} \cdot [\![ {{\boldsymbol {u}}}_h ]\!]),\quad\quad\; on\; \partial K \setminus \partial\Omega,\\ (\kappa_{h}-1){{\boldsymbol {u}}}_h,\quad\quad\; \; on\; \partial K \cap \partial\Omega. \end{array} \right. \end{eqnarray*} |
Define the global error indicator:
\zeta_{\Omega} = (\sum\limits_{K\in \mathcal{T}_{h}}{\zeta}_{K}^2(\kappa_{h},{{\boldsymbol {u}}}_h))^{\frac{1}{2}}. |
Based on the above error indicators and Scheme 3.1, we design the following adaptive multigrid algorithm bases on the shifted inverse iteration.
Algorithm 4.1. Choose parameter 0 < \alpha < 1 .
Step 1: Pick any initial mesh \mathcal{T}_{h_0} .
Step 2: Solve (2.3) on \mathcal{T}_{h_0} for discrete solution (\kappa_{j, h_0}, {{\boldsymbol {u}}}_{j, h_0}) .
Step 3: Let l = 1 . {{\boldsymbol {u}}}_{j}^{h_1} \Leftarrow {{\boldsymbol {u}}}_{j, h_0}, \kappa_{j}^{h_1} \Leftarrow \kappa_{j, h_0} .
Step 4: Compute the local indicator \zeta_{K}(\kappa_{j}^{h_l}, {{\boldsymbol {u}}}_{j}^{h_l}) .
Step 5: Construct \widehat{\mathcal{T}}_{h_l} \subset \ \mathcal{T}_{h_l} by Mark Strategy and parameter \alpha .
Step 6: Refine \mathcal{T}_{h_l} to get a new mesh \mathcal{T}_{h_{l+1}} by procedure REFINE.
Step 7: Find {\boldsymbol {\widetilde{u}}} \in {{\boldsymbol {S}}}^{h_{l+1}} such that
\begin{eqnarray} a_{h_{l+1}}({\boldsymbol {\widetilde{u}}},{{\boldsymbol {\psi}}})- \kappa_{j}^{h_l} b_{h_{l+1}}({\boldsymbol {\widetilde{u}}},{\bf \boldsymbol \psi}) = b_{h_{l+1}}({{\boldsymbol {u}}}_{j}^{h_l},{{\boldsymbol {\psi}}}) ,\ \forall {{\boldsymbol {\psi}}} \in {\boldsymbol S}^{h_{l+1}}. \end{eqnarray} |
Denote {{\boldsymbol {u}}}_{j}^{h_{l+1}} = \frac{{\boldsymbol {\widetilde{u}}}}{\| {\boldsymbol {\widetilde{u}}} \|_{0, \partial\Omega}} and compute the Rayleigh quotient
\begin{eqnarray} \kappa_{j}^{h_{l+1}} = \frac{a_{h_{l+1}}({{\boldsymbol {u}}}_{j}^{h_{l+1}},{{\boldsymbol {u}}}_{j}^{h_{l+1}})}{b_{h_{l+1}}({{\boldsymbol {u}}}_{j}^{h_{l+1}},{{\boldsymbol {u}}}_{j}^{h_{l+1}})}. \end{eqnarray} |
Step 8: Let l = l+1 and go to Step 4.
Mark Strategy
Given parameter 0 < \alpha < 1 .
Step 1: Construct a minimal subset \widehat{\mathcal{T}}_{h_{l}} of \mathcal{T}_{h_{l}} by selecting some elements in \mathcal{T}_{h_{l}} such that
\begin{eqnarray*} \sum\limits_{K\in \widehat{\mathcal{T}}_{h_{l}}}{\zeta}_{K}^2(\kappa_{j}^{h_{l}}, {{\boldsymbol {u}}}_{j}^{h_{l}}) \geq \alpha\sum\limits_{K\in {\mathcal{T}}_{h_{l}}}{\zeta}_{K}^2(\kappa_{j}^{h_{l}}, {{\boldsymbol {u}}}_{j}^{h_{l}}). \end{eqnarray*} |
Step 2: Mark all elements in \widehat{\mathcal{T}}_{h_{l}} .
Mark Strategy was first proposed in [38], and the procedure REFINE is some iterative or recursive bisection (see, e.g., [39,40]) of elements with the minimal refinement condition that marked elements are bisected at least once.
In addition, to investigate the efficiency of Algorithm 4.1, referring to the standard popular adaptive algorithm [41] we give the following Algorithm 4.2 for comparison.
Algorithm 4.2. Choose parameter 0 < \alpha < 1 .
Step 1: Pick any initial mesh \mathcal{T}_{h_0} .
Step 2: Solve (2.3) on \mathcal{T}_{h_0} for discrete solution (\kappa_{j, h_1}, {{\boldsymbol {u}}}_{j, h_1}) .
Step 3: Let l = 1 .
Step 4: Compute the local indicators \zeta_{K}(\kappa_{j, h_l}, {{\boldsymbol {u}}}_{j, h_l}) .
Step 5: Construct \widehat{\mathcal{T}}_{h_l} \subset \ \mathcal{T}_{h_l} by Mark Strategy and parameter \alpha .
Step 6: Refine \mathcal{T}_{h_l} to get a new mesh \mathcal{T}_{h_{l+1}} by procedure REFINE.
Step 7: Solve (2.3) on \mathcal{T}_{h_{l+1}} for discrete solution (\kappa_{j, h_{l+1}}, {{\boldsymbol {u}}}_{j, h_{l+1}}) .
Step 8: Let l = l+1 and go to Step 4.
We use the adaptive DG-multigrid method (Algorithm 4.1) with polynomials of degree 1 ( P1 element) and degree 2 ( P2 element) to compute, and take \alpha = 0.5 . For convenience of reading, we specify the following notations in our tables and figures.
- N_{j, l} : the degrees of freedom at the l th iteration;
- \kappa_{j}^{h_l} : the j th eigenvalue obtained by Algorithm 4.1 at the \ l \ th iteration;
- \kappa_{j, h_l} : the j th eigenvalue obtained by Algorithm 4.2 at the \ l \ th iteration;
- CPU_{j, l}(s) : the CPU time(s) from the first iteration beginning to the calculate results of the l th iteration appearing by using Algorithm 4.1/4.2;
- e_j : the error of the j th approximate eigenvalue by Algorithm 4.1;
- \zeta_j : the error indicator of the j th approximate eigenvalue by Algorithm 4.1.
We first give a numerical experiment comparison between using DGFEM to solve directly on fine meshes and using the adaptive DG-multigrid method (Algorithm 4.1) for the 1st nonzero eigenvalue of (2.3). The error curves are shown in Figures 2 and 3. An observation of the left and right subgraphs in Figures 2 and 3 tells us that the regularity of the eigenfunction in \Omega_L is lower than that in \Omega_S , which is consistent with the general conclusion of the regularity of solutions to PDEs. From Figure 2 we can see that the error curves of adaptive DG-multigrid method are all parallel to the line with slope -1 but the error curves of directly computing by DGFEM do not parallel, which indicates that the approximate eigenvalues obtained by the adaptive DG-multigrid method achieve the optimal convergence order. The same conclusion can be seen from Figure 3.
Now we use Algorithms 4.1 and 4.2 with P1 and P2 elements to compute the first 7 non-zero eigenvalues of (2.3) in \Omega_S and \Omega_L , respectively. When using P1 element, the parameters \mu = 1, \lambda = 1, \gamma_\mu = \gamma_\lambda = 10 and the diameter of initial mesh is taken as \frac{\sqrt{2}}{16} . Limited to space, we list the 1st, the 3rd, the 4th and the 6th approximate eigenvalue in Tables 1 and 2. We also depict the error curves of approximate eigenvalues by Algorithm 4.1 and the curve of error indicators in Figure 4, where the reference values are taken as the most accurate approximations that we can compute. In addition, for the 1st non-zero eigenvalue of (2.3), we investigate the influence of Lamé parameter by taking \lambda = 1, 10,100, 1000, 10000 , and the corresponding error curves are shown in Figure 5. When using P2 element, the parameters \mu = \lambda = 1, \gamma_\mu = \gamma_\lambda = 40 and the diameter of initial mesh is taken as \frac{\sqrt{2}}{8} . In Tables 3 and 4 we list the 1st, the 3rd, the 4th and the 6th approximate eigenvalue. We also plot the error curves of approximate eigenvalues by Algorithm 4.1 and the curve of error indicators in Figure 6. For the 1st non-zero eigenvalue of (2.3), we investigate the influence of Lamé parameter by taking \lambda = 1, 10,100, 1000, 10000 , and the corresponding error curves are shown in Figure 7.
j | l | N_{j, l} | \kappa_{j}^{h_l} | CPU_{j, l}(s) | l | N_{j, l} | \kappa_{j, h_l} | CPU_{j, l}(s) |
1 | 1 | 3600 | 2.5834151705 | 0.05433 | 1 | 3600 | 2.5834151539 | 0.06679 |
1 | 8 | 26328 | 2.5365029347 | 1.62136 | 8 | 26328 | 2.5365029348 | 2.52953 |
1 | 21 | 1208232 | 2.5310939833 | 249.69061 | 21 | 1208232 | 2.5310939827 | 391.04103 |
1 | 22 | 1570164 | 2.5310583228 | 485.28739 | 22 | 1570164 | 2.5310583228 | 651.02095 |
1 | 23 | 2050932 | 2.5310346038 | 817.8231911 | 23 | 2050932 | 2.531034604 | 1167.502765 |
3 | 1 | 3324 | 2.7404121518 | 0.02745 | 1 | 3324 | 2.7414582353 | 0.07244 |
3 | 11 | 30156 | 2.6778807957 | 1.75582 | 16 | 43908 | 2.6778901580 | 7.73674 |
3 | 20 | 428676 | 2.6740736381 | 57.92155 | 31 | 605400 | 2.6740696701 | 244.04938 |
3 | 23 | 1026540 | 2.6739097335 | 212.85588 | 34 | 1025280 | 2.6739660124 | 488.60793 |
3 | 24 | 1349388 | 2.6738786576 | 395.09095 | 35 | 1219860 | 2.6739372698 | 624.67912 |
4 | 1 | 3888 | 3.7164345114 | 0.05795 | 1 | 3888 | 3.7164345114 | 0.10192 |
4 | 9 | 44460 | 3.7115741607 | 3.84681 | 9 | 44460 | 3.7115741607 | 5.61523 |
4 | 22 | 1762944 | 3.7111432638 | 194.69801 | 22 | 1762944 | 3.7111432638 | 341.67732 |
4 | 23 | 2344044 | 3.7111398968 | 269.71127 | 23 | 2344044 | 3.7111398969 | 470.55583 |
4 | 24 | 3028620 | 3.7111378848 | 385.01813 | 24 | 3028620 | 3.7111378848 | 654.91996 |
6 | 1 | 3792 | 5.2873876626 | 0.06042 | 1 | 3792 | 5.2873876273 | 0.09334 |
6 | 5 | 11784 | 5.2632333578 | 0.70311 | 5 | 11784 | 5.2632333578 | 1.11777 |
6 | 20 | 942528 | 5.2537917257 | 198.46971 | 20 | 942528 | 5.2537917253 | 306.96848 |
6 | 21 | 1259328 | 5.2537597122 | 334.17056 | 21 | 1259328 | 5.2537597120 | 452.24058 |
6 | 22 | 1636512 | 5.2537348070 | 605.55218 | 22 | 1636512 | 5.2537348072 | 796.76924 |
j | l | N_{j, l} | \kappa_{j}^{h_l} | CPU_{j, l}(s) | l | N_{j, l} | \kappa_{j, h_l} | CPU_{j, l}(s) |
1 | 1 | 9252 | 1.1578406889 | 0.08684 | 1 | 9252 | 1.1578406889 | 0.21686 |
1 | 4 | 9960 | 1.1568717901 | 0.51428 | 4 | 9960 | 1.1568717901 | 1.05144 |
1 | 22 | 854196 | 1.1551704849 | 83.61915 | 22 | 854520 | 1.1551704801 | 143.97265 |
1 | 23 | 1125306 | 1.1551665423 | 116.92568 | 23 | 1125690 | 1.1551665389 | 193.87293 |
1 | 24 | 1469730 | 1.1551634294 | 330.33730 | 24 | 1470228 | 1.1551634258 | 431.50241 |
3 | 1 | 9852 | 2.0253499592 | 0.10770 | 1 | 9852 | 2.0253499590 | 0.24006 |
3 | 3 | 13236 | 2.0200276681 | 0.62807 | 3 | 13236 | 2.0200276680 | 1.21075 |
3 | 18 | 1072320 | 2.0137654513 | 221.81309 | 18 | 1072320 | 2.0137654513 | 344.53795 |
3 | 19 | 1431672 | 2.0137487824 | 351.63554 | 19 | 1431672 | 2.0137487822 | 590.05031 |
3 | 20 | 1924536 | 2.0137351315 | 711.94221 | 20 | 1924536 | 2.0137351315 | 1029.63061 |
4 | 1 | 9540 | 2.1396949065 | 0.10559 | 1 | 9540 | 2.1396949061 | 0.23500 |
4 | 4 | 13446 | 2.1322409848 | 0.70466 | 4 | 13446 | 2.1322409847 | 1.33492 |
4 | 19 | 823536 | 2.1258417612 | 147.60184 | 19 | 823536 | 2.1258417612 | 260.40436 |
4 | 20 | 1085094 | 2.1258166076 | 225.83332 | 20 | 1085094 | 2.1258166075 | 386.16427 |
4 | 21 | 1437324 | 2.1257991175 | 324.24898 | 21 | 1437324 | 2.1257991172 | 566.48046 |
6 | 1 | 9828 | 2.7939491213 | 0.15553 | 1 | 9828 | 2.7939490858 | 0.31958 |
6 | 3 | 12894 | 2.7668920157 | 0.78224 | 3 | 12894 | 2.7668920151 | 1.00058 |
6 | 18 | 965010 | 2.7367899041 | 163.71460 | 18 | 965010 | 2.7367899035 | 335.07813 |
6 | 19 | 1292184 | 2.7367099740 | 271.08934 | 19 | 1292184 | 2.7367099727 | 503.74878 |
6 | 20 | 1729368 | 2.7366439994 | 494.77192 | 20 | 1729368 | 2.7366439984 | 797.84910 |
j | l | N_{j, l} | \kappa_{j}^{h_l} | CPU_{j, l}(s) | l | N_{j, l} | \kappa_{j, h_l} | CPU_{j, l}(s) |
1 | 1 | 1632 | 2.5399573563 | 0.20754 | 1 | 1632 | 2.5399573531 | 0.33385 |
1 | 5 | 3600 | 2.5321126514 | 0.80285 | 5 | 3600 | 2.5321126514 | 1.16189 |
1 | 24 | 760152 | 2.5309641786 | 211.46084 | 24 | 760152 | 2.5309641786 | 239.26807 |
1 | 25 | 1003368 | 2.5309641677 | 278.22507 | 25 | 1003368 | 2.5309641677 | 320.01464 |
1 | 26 | 1339404 | 2.5309641607 | 378.58015 | 26 | 1339404 | 2.5309641607 | 439.05795 |
3 | 1 | 1584 | 2.6862908794 | 0.12511 | 1 | 1584 | 2.6862921826 | 0.40313 |
3 | 18 | 43920 | 2.6737990072 | 8.60574 | 24 | 46896 | 2.6737995054 | 17.94984 |
3 | 30 | 813360 | 2.6737894142 | 210.84097 | 42 | 823224 | 2.6737894180 | 409.26749 |
3 | 31 | 1021272 | 2.6737894039 | 275.45868 | 44 | 1129272 | 2.6737894027 | 587.31383 |
3 | 32 | 1276776 | 2.6737893962 | 363.84821 | 45 | 1328904 | 2.6737893981 | 708.17810 |
4 | 1 | 1824 | 3.7114311740 | 0.13369 | 1 | 1824 | 3.7114311740 | 0.21961 |
4 | 5 | 6360 | 3.7111583273 | 0.89324 | 5 | 6360 | 3.7111583273 | 1.89695 |
4 | 22 | 754512 | 3.7111313470 | 178.28979 | 22 | 754512 | 3.7111313471 | 250.31766 |
4 | 23 | 939408 | 3.7111313465 | 244.08245 | 23 | 939408 | 3.7111313466 | 341.16368 |
4 | 24 | 1218768 | 3.7111313461 | 339.61412 | 24 | 1218768 | 3.7111313462 | 460.76539 |
6 | 2 | 1920 | 5.2557235447 | 0.49529 | 2 | 1920 | 5.2557235444 | 0.59788 |
6 | 8 | 8904 | 5.2537750836 | 1.87161 | 8 | 8904 | 5.2537750836 | 2.28091 |
6 | 24 | 838920 | 5.2536682063 | 197.38184 | 24 | 838920 | 5.2536682064 | 267.40500 |
6 | 25 | 1119096 | 5.2536682008 | 270.08041 | 25 | 1119096 | 5.2536682009 | 376.06184 |
6 | 26 | 1456968 | 5.2536681969 | 380.87500 | 26 | 1456968 | 5.2536681971 | 520.85769 |
j | l | N_{j, l} | \kappa_{j}^{h_l} | CPU_{j, l}(s) | l | N_{j, l} | \kappa_{j, h_l} | CPU_{j, l}(s) |
1 | 1 | 4656 | 1.1562458918 | 0.38986 | 1 | 4656 | 1.1562458918 | 0.55317 |
1 | 25 | 66996 | 1.1551541513 | 18.31403 | 25 | 66996 | 1.1551541513 | 22.07079 |
1 | 38 | 774480 | 1.1551532609 | 260.06329 | 38 | 774480 | 1.1551532609 | 325.52552 |
1 | 39 | 923052 | 1.1551532590 | 320.61556 | 39 | 923052 | 1.1551532591 | 400.40419 |
1 | 40 | 1099044 | 1.1551532576 | 398.76151 | 40 | 1099044 | 1.1551532576 | 496.73607 |
3 | 1 | 4680 | 2.0159225406 | 0.46936 | 1 | 4680 | 2.0159225405 | 0.57221 |
3 | 9 | 19224 | 2.0137381688 | 4.00481 | 9 | 19224 | 2.0137381688 | 5.20982 |
3 | 23 | 976428 | 2.0136927626 | 233.96942 | 23 | 976428 | 2.0136927626 | 313.17266 |
3 | 24 | 1280052 | 2.0136927550 | 324.92148 | 24 | 1280052 | 2.0136927550 | 421.48383 |
3 | 25 | 1679592 | 2.0136927505 | 458.31975 | 25 | 1679592 | 2.0136927505 | 578.37647 |
4 | 10 | 11316 | 2.1259084959 | 3.98294 | 10 | 11316 | 2.1259084959 | 4.01302 |
4 | 21 | 105648 | 2.1257416544 | 27.89323 | 21 | 105648 | 2.1257416544 | 37.43586 |
4 | 32 | 822120 | 2.1257391878 | 297.07841 | 32 | 822120 | 2.1257391878 | 368.95873 |
4 | 33 | 980688 | 2.1257391755 | 362.49125 | 33 | 980688 | 2.1257391755 | 456.94719 |
4 | 34 | 1181328 | 2.1257391658 | 446.73985 | 34 | 1181328 | 2.1257391658 | 572.04947 |
6 | 1 | 4680 | 2.7468959498 | 0.40303 | 1 | 4680 | 2.7468959503 | 0.61874 |
6 | 3 | 5232 | 2.7405213916 | 0.92105 | 3 | 5232 | 2.7405213907 | 1.21365 |
6 | 23 | 1000704 | 2.7364519542 | 234.72412 | 23 | 1000704 | 2.7364519542 | 334.60146 |
6 | 24 | 1312716 | 2.7364519227 | 327.39089 | 24 | 1312716 | 2.7364519227 | 448.35877 |
6 | 25 | 1726080 | 2.7364519037 | 468.01995 | 25 | 1726080 | 2.7364519038 | 608.05296 |
It can be seen from Tables 1–4 that to get the same accurate approximate eigenvalues, Algorithm 4.1 uses less time or less degrees of freedom than Algorithm 4.2. In Figure 4, the error curves e_1, e_3, e_4 and e_6 are all parallel to the line with slope -1 , and in Figure 6 the error curves e_1, e_3, e_4 and e_6 are parallel to the line with slope -2 , which indicate that the approximate eigenvalues obtained by Algorithm 4.1 achieve the optimal convergence order. Meanwhile, in Figure 5, the error curves e_1, e_3, e_4 and e_6 are almost parallel to the curve of \zeta_1, \zeta_3, \zeta_4 and \zeta_6 respectively, and in Figure 7, the curves of e_1, e_3, e_4 and e_6 are parallel to \zeta_1, \zeta_3, \zeta_4 and \zeta_6 , which indicate that the error indicators are reliable and efficient. Figures 5 and 7 then show that Algorithm 4.1 is robust in both \Omega_S and \Omega_L .
In this paper, we discussed a multigrid discretization scheme of DGFEM based on the shifted-inverse iteration. Theoretical analysis and numerical results all showed that this method can efficiently solve the Steklov-Lamé eigenproblem as we expected. Generally, the time of solving a linear algebraic system is much less than that of solving an eigenvalue problem. Further, we observe from Tables 1–4 that although the CPU time of the adaptive DG-multigrid method is less than that of the standard adaptive DGFEM, the advantage is not obvious. We think that this may be because we use " \setminus " to solve linear algebraic systems. We notice that in recent research, the multigrid method has been combined with other methods to form many efficient algorithms and applied to many problems, as combined with the DG method in this paper. For example, the multigrid-homotopy method to diffusion equation [42], the multigrid method for the semilinear interface problem based on the modified two-grid method [43], the multigrid method for nonlinear eigenvalue problems based on Newton iteration [44], etc. It is of interest for us to explore more applications of multigrid methods and more efficient solvers for solving linear algebraic equations in multigrid methods.
This work was supported by the National Natural Science Foundation of China (No. 12261024) and Science and Technology Planning Project of Guizhou Province (Guizhou Kehe fundamental research-ZK[2022] No.324).
This work does not have any conflicts of interest.
[1] |
M. EI-Doma, Analysis of an age-dependent SI epidemic model with disease-induced mortality and proportionate mixing assumption: The case of vertically transmitted diseases, J. Appl. Math., 2004 (2004), 235–254. https://doi.org/10.1155/S1110757X0430118X doi: 10.1155/S1110757X0430118X
![]() |
[2] |
W. O. Kermack, A. G. McKendrick, A contribution to the mathematical theory of epidemics, Proc R. Soc. Lond. B Biol. Sci., 115 (1927), 700–721. https://doi.org/10.1098/rspa.1927.0118E. doi: 10.1098/rspa.1927.0118E
![]() |
[3] |
I. Mimmo, M. Y. Kim, J. Park, Asymptotic behavior for an SIS epidemic model and its approximation, Nonlinear Anal. Theory Methods Appl., 35 (1999), 797–814. https://doi.org/10.1016/S0362-546X(97)00597-X doi: 10.1016/S0362-546X(97)00597-X
![]() |
[4] |
I. B. Schwartz, H. L. Smith, Infinite subharmonic bifurcation in an SEIR epidemic model, J. Math. Biol., 18 (1983), 233–253. https://doi.org/10.1007/BF00276090 doi: 10.1007/BF00276090
![]() |
[5] |
R. A. Brown, A simple model for control of COVID-19 infections on an urban campus, Proc. Natl. Acad. Sci. U S A, 118 (2021), e2105292118. https://doi.org/10.1073/pnas.2105292118 doi: 10.1073/pnas.2105292118
![]() |
[6] |
X. X. Liu, S. J. Fong, N. Dey, R. G. Crespo, E. H. Viedma, A new SEAIRD pandemic prediction model with clinical and epidemiological data analysis on COVID-19 outbreak, Appl. Intell., 51 (2021), 4162–4198. https://doi.org/10.1007/s10489-020-01938-3 doi: 10.1007/s10489-020-01938-3
![]() |
[7] |
D. J. Watts, S. H. Strogatz, Collective dynamics of small-world9 networks, Nature, 393 (1998), 440–442. https://doi.org/10.1038/30918 doi: 10.1038/30918
![]() |
[8] |
L. A. Amaral, A. Scala, M. Barthelemy, H. E. Stanley, Classes of small-world networks, Proc. Natl. Acad. Sci. U S A, 97 (2000), 11149–11152. https://doi.org/10.1073/pnas.200327197 doi: 10.1073/pnas.200327197
![]() |
[9] |
M. Liu, Y. Xiao, Modeling and analysis of epidemic diffusion within small-world network, J. Appl. Math., 2012 (2012), 841531. https://doi.org/10.1155/2012/841531 doi: 10.1155/2012/841531
![]() |
[10] |
F. Z. Younsi, A. Bounnekar, D. Hamdadou, O. Boussaid, SEIR-SW, simulation model of influenza spread based on the Small World network, Tsinghua Sci. Technol., 20 (2015), 460–473. https://doi.org/10.1109/TST.2015.7297745 doi: 10.1109/TST.2015.7297745
![]() |
[11] |
J. Saramäki, K. Kaski, Modelling development of epidemics with dynamic small-world networks, J. Theor. Biol., 234 (2005), 413–421. https://doi.org/10.1016/j.jtbi.2004.12.003 doi: 10.1016/j.jtbi.2004.12.003
![]() |
[12] | S. Ren, W. Wang, R. Gao, A. Zhou, Omicron variant (B.1.1.529) of SARS-COV-2: Mutation, infectivity, transmission, and vaccine resistance, World J. Clin. Cases, 10 (2022), 1–11. https://doi.org/10.12998/wjcc.v10.i1.1 |
[13] |
H. W. Hethcote, The mathematics of infectious diseases, SIAM Rev., 42 (2000), 599–653, https://doi.org/10.1137/S0036144500371907. doi: 10.1137/S0036144500371907
![]() |
[14] |
P. Driessche, J. Watmough, Reproduction numbers and sub-threshold endemic equilibria for compartmental models of disease transmission, Math. Biosci., 180 (2002), 29–48. https://doi.org/10.1016/S0025-5564(02)00108-6 doi: 10.1016/S0025-5564(02)00108-6
![]() |
[15] |
O. Diekmann, J. A. P. Heesterbeek, J. A. J. Metz, On the definition and the computation of the basic reproduction ratio R0 in models for infectious diseases in heterogeneous populations, J. Math. Biol., 28 (1990), 365–382. https://doi.org/10.1007/BF00178324 doi: 10.1007/BF00178324
![]() |
[16] |
E. Y. Boateng, D. A. Abaye, A review of the Logistic Regression model with emphasis on medical research, J. Data Anal. Inform. Proc., 7 (2019), 190–207. https://doi.org/10.4236/jdaip.2019.74012 doi: 10.4236/jdaip.2019.74012
![]() |
[17] |
S. A. Morsi, M. E. Alzahrani, Advanced computing approach for modeling and prediction COVID-19 pandemic, Appl. Bionics and Biomech., 2022 (2022), 6056574. https://doi.org/10.1155/2022/6056574 doi: 10.1155/2022/6056574
![]() |
[18] | Shanghai Municipal Health Commission, Shanghai Municipal Bureau Statistics: From epidemic notification. Available from: https://wsjkw.sh.gov.cn. |
[19] |
Y. Ma, S. Xu, Q. An, M. Qin, S. Li, K. Lu, et al., Coronavirus disease 2019 epidemic prediction in Shanghai under the dynamic zero-COVID policy using time-dependent SEAIQR model, J. Biosaf. Biosecur., 4 (2022), 105–113. https://doi.org/10.1016/j.jobb.2022.06.002 doi: 10.1016/j.jobb.2022.06.002
![]() |
[20] | World Health Organization: WHO Coronavirus (COVID-19) Dashboard with Vaccination Data, 2022. Available from: https://covid19.who.int. |
1. | Noura Alhouiti, Fatemah Mofarreh, Fatemah Abdullah Alghamdi, Akram Ali, Piscoran-Ioan Laurian, Geometric topology of CR-warped products in six-dimensional sphere, 2024, 9, 2473-6988, 25114, 10.3934/math.20241224 |
j | l | N_{j, l} | \kappa_{j}^{h_l} | CPU_{j, l}(s) | l | N_{j, l} | \kappa_{j, h_l} | CPU_{j, l}(s) |
1 | 1 | 3600 | 2.5834151705 | 0.05433 | 1 | 3600 | 2.5834151539 | 0.06679 |
1 | 8 | 26328 | 2.5365029347 | 1.62136 | 8 | 26328 | 2.5365029348 | 2.52953 |
1 | 21 | 1208232 | 2.5310939833 | 249.69061 | 21 | 1208232 | 2.5310939827 | 391.04103 |
1 | 22 | 1570164 | 2.5310583228 | 485.28739 | 22 | 1570164 | 2.5310583228 | 651.02095 |
1 | 23 | 2050932 | 2.5310346038 | 817.8231911 | 23 | 2050932 | 2.531034604 | 1167.502765 |
3 | 1 | 3324 | 2.7404121518 | 0.02745 | 1 | 3324 | 2.7414582353 | 0.07244 |
3 | 11 | 30156 | 2.6778807957 | 1.75582 | 16 | 43908 | 2.6778901580 | 7.73674 |
3 | 20 | 428676 | 2.6740736381 | 57.92155 | 31 | 605400 | 2.6740696701 | 244.04938 |
3 | 23 | 1026540 | 2.6739097335 | 212.85588 | 34 | 1025280 | 2.6739660124 | 488.60793 |
3 | 24 | 1349388 | 2.6738786576 | 395.09095 | 35 | 1219860 | 2.6739372698 | 624.67912 |
4 | 1 | 3888 | 3.7164345114 | 0.05795 | 1 | 3888 | 3.7164345114 | 0.10192 |
4 | 9 | 44460 | 3.7115741607 | 3.84681 | 9 | 44460 | 3.7115741607 | 5.61523 |
4 | 22 | 1762944 | 3.7111432638 | 194.69801 | 22 | 1762944 | 3.7111432638 | 341.67732 |
4 | 23 | 2344044 | 3.7111398968 | 269.71127 | 23 | 2344044 | 3.7111398969 | 470.55583 |
4 | 24 | 3028620 | 3.7111378848 | 385.01813 | 24 | 3028620 | 3.7111378848 | 654.91996 |
6 | 1 | 3792 | 5.2873876626 | 0.06042 | 1 | 3792 | 5.2873876273 | 0.09334 |
6 | 5 | 11784 | 5.2632333578 | 0.70311 | 5 | 11784 | 5.2632333578 | 1.11777 |
6 | 20 | 942528 | 5.2537917257 | 198.46971 | 20 | 942528 | 5.2537917253 | 306.96848 |
6 | 21 | 1259328 | 5.2537597122 | 334.17056 | 21 | 1259328 | 5.2537597120 | 452.24058 |
6 | 22 | 1636512 | 5.2537348070 | 605.55218 | 22 | 1636512 | 5.2537348072 | 796.76924 |
j | l | N_{j, l} | \kappa_{j}^{h_l} | CPU_{j, l}(s) | l | N_{j, l} | \kappa_{j, h_l} | CPU_{j, l}(s) |
1 | 1 | 9252 | 1.1578406889 | 0.08684 | 1 | 9252 | 1.1578406889 | 0.21686 |
1 | 4 | 9960 | 1.1568717901 | 0.51428 | 4 | 9960 | 1.1568717901 | 1.05144 |
1 | 22 | 854196 | 1.1551704849 | 83.61915 | 22 | 854520 | 1.1551704801 | 143.97265 |
1 | 23 | 1125306 | 1.1551665423 | 116.92568 | 23 | 1125690 | 1.1551665389 | 193.87293 |
1 | 24 | 1469730 | 1.1551634294 | 330.33730 | 24 | 1470228 | 1.1551634258 | 431.50241 |
3 | 1 | 9852 | 2.0253499592 | 0.10770 | 1 | 9852 | 2.0253499590 | 0.24006 |
3 | 3 | 13236 | 2.0200276681 | 0.62807 | 3 | 13236 | 2.0200276680 | 1.21075 |
3 | 18 | 1072320 | 2.0137654513 | 221.81309 | 18 | 1072320 | 2.0137654513 | 344.53795 |
3 | 19 | 1431672 | 2.0137487824 | 351.63554 | 19 | 1431672 | 2.0137487822 | 590.05031 |
3 | 20 | 1924536 | 2.0137351315 | 711.94221 | 20 | 1924536 | 2.0137351315 | 1029.63061 |
4 | 1 | 9540 | 2.1396949065 | 0.10559 | 1 | 9540 | 2.1396949061 | 0.23500 |
4 | 4 | 13446 | 2.1322409848 | 0.70466 | 4 | 13446 | 2.1322409847 | 1.33492 |
4 | 19 | 823536 | 2.1258417612 | 147.60184 | 19 | 823536 | 2.1258417612 | 260.40436 |
4 | 20 | 1085094 | 2.1258166076 | 225.83332 | 20 | 1085094 | 2.1258166075 | 386.16427 |
4 | 21 | 1437324 | 2.1257991175 | 324.24898 | 21 | 1437324 | 2.1257991172 | 566.48046 |
6 | 1 | 9828 | 2.7939491213 | 0.15553 | 1 | 9828 | 2.7939490858 | 0.31958 |
6 | 3 | 12894 | 2.7668920157 | 0.78224 | 3 | 12894 | 2.7668920151 | 1.00058 |
6 | 18 | 965010 | 2.7367899041 | 163.71460 | 18 | 965010 | 2.7367899035 | 335.07813 |
6 | 19 | 1292184 | 2.7367099740 | 271.08934 | 19 | 1292184 | 2.7367099727 | 503.74878 |
6 | 20 | 1729368 | 2.7366439994 | 494.77192 | 20 | 1729368 | 2.7366439984 | 797.84910 |
j | l | N_{j, l} | \kappa_{j}^{h_l} | CPU_{j, l}(s) | l | N_{j, l} | \kappa_{j, h_l} | CPU_{j, l}(s) |
1 | 1 | 1632 | 2.5399573563 | 0.20754 | 1 | 1632 | 2.5399573531 | 0.33385 |
1 | 5 | 3600 | 2.5321126514 | 0.80285 | 5 | 3600 | 2.5321126514 | 1.16189 |
1 | 24 | 760152 | 2.5309641786 | 211.46084 | 24 | 760152 | 2.5309641786 | 239.26807 |
1 | 25 | 1003368 | 2.5309641677 | 278.22507 | 25 | 1003368 | 2.5309641677 | 320.01464 |
1 | 26 | 1339404 | 2.5309641607 | 378.58015 | 26 | 1339404 | 2.5309641607 | 439.05795 |
3 | 1 | 1584 | 2.6862908794 | 0.12511 | 1 | 1584 | 2.6862921826 | 0.40313 |
3 | 18 | 43920 | 2.6737990072 | 8.60574 | 24 | 46896 | 2.6737995054 | 17.94984 |
3 | 30 | 813360 | 2.6737894142 | 210.84097 | 42 | 823224 | 2.6737894180 | 409.26749 |
3 | 31 | 1021272 | 2.6737894039 | 275.45868 | 44 | 1129272 | 2.6737894027 | 587.31383 |
3 | 32 | 1276776 | 2.6737893962 | 363.84821 | 45 | 1328904 | 2.6737893981 | 708.17810 |
4 | 1 | 1824 | 3.7114311740 | 0.13369 | 1 | 1824 | 3.7114311740 | 0.21961 |
4 | 5 | 6360 | 3.7111583273 | 0.89324 | 5 | 6360 | 3.7111583273 | 1.89695 |
4 | 22 | 754512 | 3.7111313470 | 178.28979 | 22 | 754512 | 3.7111313471 | 250.31766 |
4 | 23 | 939408 | 3.7111313465 | 244.08245 | 23 | 939408 | 3.7111313466 | 341.16368 |
4 | 24 | 1218768 | 3.7111313461 | 339.61412 | 24 | 1218768 | 3.7111313462 | 460.76539 |
6 | 2 | 1920 | 5.2557235447 | 0.49529 | 2 | 1920 | 5.2557235444 | 0.59788 |
6 | 8 | 8904 | 5.2537750836 | 1.87161 | 8 | 8904 | 5.2537750836 | 2.28091 |
6 | 24 | 838920 | 5.2536682063 | 197.38184 | 24 | 838920 | 5.2536682064 | 267.40500 |
6 | 25 | 1119096 | 5.2536682008 | 270.08041 | 25 | 1119096 | 5.2536682009 | 376.06184 |
6 | 26 | 1456968 | 5.2536681969 | 380.87500 | 26 | 1456968 | 5.2536681971 | 520.85769 |
j | l | N_{j, l} | \kappa_{j}^{h_l} | CPU_{j, l}(s) | l | N_{j, l} | \kappa_{j, h_l} | CPU_{j, l}(s) |
1 | 1 | 4656 | 1.1562458918 | 0.38986 | 1 | 4656 | 1.1562458918 | 0.55317 |
1 | 25 | 66996 | 1.1551541513 | 18.31403 | 25 | 66996 | 1.1551541513 | 22.07079 |
1 | 38 | 774480 | 1.1551532609 | 260.06329 | 38 | 774480 | 1.1551532609 | 325.52552 |
1 | 39 | 923052 | 1.1551532590 | 320.61556 | 39 | 923052 | 1.1551532591 | 400.40419 |
1 | 40 | 1099044 | 1.1551532576 | 398.76151 | 40 | 1099044 | 1.1551532576 | 496.73607 |
3 | 1 | 4680 | 2.0159225406 | 0.46936 | 1 | 4680 | 2.0159225405 | 0.57221 |
3 | 9 | 19224 | 2.0137381688 | 4.00481 | 9 | 19224 | 2.0137381688 | 5.20982 |
3 | 23 | 976428 | 2.0136927626 | 233.96942 | 23 | 976428 | 2.0136927626 | 313.17266 |
3 | 24 | 1280052 | 2.0136927550 | 324.92148 | 24 | 1280052 | 2.0136927550 | 421.48383 |
3 | 25 | 1679592 | 2.0136927505 | 458.31975 | 25 | 1679592 | 2.0136927505 | 578.37647 |
4 | 10 | 11316 | 2.1259084959 | 3.98294 | 10 | 11316 | 2.1259084959 | 4.01302 |
4 | 21 | 105648 | 2.1257416544 | 27.89323 | 21 | 105648 | 2.1257416544 | 37.43586 |
4 | 32 | 822120 | 2.1257391878 | 297.07841 | 32 | 822120 | 2.1257391878 | 368.95873 |
4 | 33 | 980688 | 2.1257391755 | 362.49125 | 33 | 980688 | 2.1257391755 | 456.94719 |
4 | 34 | 1181328 | 2.1257391658 | 446.73985 | 34 | 1181328 | 2.1257391658 | 572.04947 |
6 | 1 | 4680 | 2.7468959498 | 0.40303 | 1 | 4680 | 2.7468959503 | 0.61874 |
6 | 3 | 5232 | 2.7405213916 | 0.92105 | 3 | 5232 | 2.7405213907 | 1.21365 |
6 | 23 | 1000704 | 2.7364519542 | 234.72412 | 23 | 1000704 | 2.7364519542 | 334.60146 |
6 | 24 | 1312716 | 2.7364519227 | 327.39089 | 24 | 1312716 | 2.7364519227 | 448.35877 |
6 | 25 | 1726080 | 2.7364519037 | 468.01995 | 25 | 1726080 | 2.7364519038 | 608.05296 |
j | l | N_{j, l} | \kappa_{j}^{h_l} | CPU_{j, l}(s) | l | N_{j, l} | \kappa_{j, h_l} | CPU_{j, l}(s) |
1 | 1 | 3600 | 2.5834151705 | 0.05433 | 1 | 3600 | 2.5834151539 | 0.06679 |
1 | 8 | 26328 | 2.5365029347 | 1.62136 | 8 | 26328 | 2.5365029348 | 2.52953 |
1 | 21 | 1208232 | 2.5310939833 | 249.69061 | 21 | 1208232 | 2.5310939827 | 391.04103 |
1 | 22 | 1570164 | 2.5310583228 | 485.28739 | 22 | 1570164 | 2.5310583228 | 651.02095 |
1 | 23 | 2050932 | 2.5310346038 | 817.8231911 | 23 | 2050932 | 2.531034604 | 1167.502765 |
3 | 1 | 3324 | 2.7404121518 | 0.02745 | 1 | 3324 | 2.7414582353 | 0.07244 |
3 | 11 | 30156 | 2.6778807957 | 1.75582 | 16 | 43908 | 2.6778901580 | 7.73674 |
3 | 20 | 428676 | 2.6740736381 | 57.92155 | 31 | 605400 | 2.6740696701 | 244.04938 |
3 | 23 | 1026540 | 2.6739097335 | 212.85588 | 34 | 1025280 | 2.6739660124 | 488.60793 |
3 | 24 | 1349388 | 2.6738786576 | 395.09095 | 35 | 1219860 | 2.6739372698 | 624.67912 |
4 | 1 | 3888 | 3.7164345114 | 0.05795 | 1 | 3888 | 3.7164345114 | 0.10192 |
4 | 9 | 44460 | 3.7115741607 | 3.84681 | 9 | 44460 | 3.7115741607 | 5.61523 |
4 | 22 | 1762944 | 3.7111432638 | 194.69801 | 22 | 1762944 | 3.7111432638 | 341.67732 |
4 | 23 | 2344044 | 3.7111398968 | 269.71127 | 23 | 2344044 | 3.7111398969 | 470.55583 |
4 | 24 | 3028620 | 3.7111378848 | 385.01813 | 24 | 3028620 | 3.7111378848 | 654.91996 |
6 | 1 | 3792 | 5.2873876626 | 0.06042 | 1 | 3792 | 5.2873876273 | 0.09334 |
6 | 5 | 11784 | 5.2632333578 | 0.70311 | 5 | 11784 | 5.2632333578 | 1.11777 |
6 | 20 | 942528 | 5.2537917257 | 198.46971 | 20 | 942528 | 5.2537917253 | 306.96848 |
6 | 21 | 1259328 | 5.2537597122 | 334.17056 | 21 | 1259328 | 5.2537597120 | 452.24058 |
6 | 22 | 1636512 | 5.2537348070 | 605.55218 | 22 | 1636512 | 5.2537348072 | 796.76924 |
j | l | N_{j, l} | \kappa_{j}^{h_l} | CPU_{j, l}(s) | l | N_{j, l} | \kappa_{j, h_l} | CPU_{j, l}(s) |
1 | 1 | 9252 | 1.1578406889 | 0.08684 | 1 | 9252 | 1.1578406889 | 0.21686 |
1 | 4 | 9960 | 1.1568717901 | 0.51428 | 4 | 9960 | 1.1568717901 | 1.05144 |
1 | 22 | 854196 | 1.1551704849 | 83.61915 | 22 | 854520 | 1.1551704801 | 143.97265 |
1 | 23 | 1125306 | 1.1551665423 | 116.92568 | 23 | 1125690 | 1.1551665389 | 193.87293 |
1 | 24 | 1469730 | 1.1551634294 | 330.33730 | 24 | 1470228 | 1.1551634258 | 431.50241 |
3 | 1 | 9852 | 2.0253499592 | 0.10770 | 1 | 9852 | 2.0253499590 | 0.24006 |
3 | 3 | 13236 | 2.0200276681 | 0.62807 | 3 | 13236 | 2.0200276680 | 1.21075 |
3 | 18 | 1072320 | 2.0137654513 | 221.81309 | 18 | 1072320 | 2.0137654513 | 344.53795 |
3 | 19 | 1431672 | 2.0137487824 | 351.63554 | 19 | 1431672 | 2.0137487822 | 590.05031 |
3 | 20 | 1924536 | 2.0137351315 | 711.94221 | 20 | 1924536 | 2.0137351315 | 1029.63061 |
4 | 1 | 9540 | 2.1396949065 | 0.10559 | 1 | 9540 | 2.1396949061 | 0.23500 |
4 | 4 | 13446 | 2.1322409848 | 0.70466 | 4 | 13446 | 2.1322409847 | 1.33492 |
4 | 19 | 823536 | 2.1258417612 | 147.60184 | 19 | 823536 | 2.1258417612 | 260.40436 |
4 | 20 | 1085094 | 2.1258166076 | 225.83332 | 20 | 1085094 | 2.1258166075 | 386.16427 |
4 | 21 | 1437324 | 2.1257991175 | 324.24898 | 21 | 1437324 | 2.1257991172 | 566.48046 |
6 | 1 | 9828 | 2.7939491213 | 0.15553 | 1 | 9828 | 2.7939490858 | 0.31958 |
6 | 3 | 12894 | 2.7668920157 | 0.78224 | 3 | 12894 | 2.7668920151 | 1.00058 |
6 | 18 | 965010 | 2.7367899041 | 163.71460 | 18 | 965010 | 2.7367899035 | 335.07813 |
6 | 19 | 1292184 | 2.7367099740 | 271.08934 | 19 | 1292184 | 2.7367099727 | 503.74878 |
6 | 20 | 1729368 | 2.7366439994 | 494.77192 | 20 | 1729368 | 2.7366439984 | 797.84910 |
j | l | N_{j, l} | \kappa_{j}^{h_l} | CPU_{j, l}(s) | l | N_{j, l} | \kappa_{j, h_l} | CPU_{j, l}(s) |
1 | 1 | 1632 | 2.5399573563 | 0.20754 | 1 | 1632 | 2.5399573531 | 0.33385 |
1 | 5 | 3600 | 2.5321126514 | 0.80285 | 5 | 3600 | 2.5321126514 | 1.16189 |
1 | 24 | 760152 | 2.5309641786 | 211.46084 | 24 | 760152 | 2.5309641786 | 239.26807 |
1 | 25 | 1003368 | 2.5309641677 | 278.22507 | 25 | 1003368 | 2.5309641677 | 320.01464 |
1 | 26 | 1339404 | 2.5309641607 | 378.58015 | 26 | 1339404 | 2.5309641607 | 439.05795 |
3 | 1 | 1584 | 2.6862908794 | 0.12511 | 1 | 1584 | 2.6862921826 | 0.40313 |
3 | 18 | 43920 | 2.6737990072 | 8.60574 | 24 | 46896 | 2.6737995054 | 17.94984 |
3 | 30 | 813360 | 2.6737894142 | 210.84097 | 42 | 823224 | 2.6737894180 | 409.26749 |
3 | 31 | 1021272 | 2.6737894039 | 275.45868 | 44 | 1129272 | 2.6737894027 | 587.31383 |
3 | 32 | 1276776 | 2.6737893962 | 363.84821 | 45 | 1328904 | 2.6737893981 | 708.17810 |
4 | 1 | 1824 | 3.7114311740 | 0.13369 | 1 | 1824 | 3.7114311740 | 0.21961 |
4 | 5 | 6360 | 3.7111583273 | 0.89324 | 5 | 6360 | 3.7111583273 | 1.89695 |
4 | 22 | 754512 | 3.7111313470 | 178.28979 | 22 | 754512 | 3.7111313471 | 250.31766 |
4 | 23 | 939408 | 3.7111313465 | 244.08245 | 23 | 939408 | 3.7111313466 | 341.16368 |
4 | 24 | 1218768 | 3.7111313461 | 339.61412 | 24 | 1218768 | 3.7111313462 | 460.76539 |
6 | 2 | 1920 | 5.2557235447 | 0.49529 | 2 | 1920 | 5.2557235444 | 0.59788 |
6 | 8 | 8904 | 5.2537750836 | 1.87161 | 8 | 8904 | 5.2537750836 | 2.28091 |
6 | 24 | 838920 | 5.2536682063 | 197.38184 | 24 | 838920 | 5.2536682064 | 267.40500 |
6 | 25 | 1119096 | 5.2536682008 | 270.08041 | 25 | 1119096 | 5.2536682009 | 376.06184 |
6 | 26 | 1456968 | 5.2536681969 | 380.87500 | 26 | 1456968 | 5.2536681971 | 520.85769 |
j | l | N_{j, l} | \kappa_{j}^{h_l} | CPU_{j, l}(s) | l | N_{j, l} | \kappa_{j, h_l} | CPU_{j, l}(s) |
1 | 1 | 4656 | 1.1562458918 | 0.38986 | 1 | 4656 | 1.1562458918 | 0.55317 |
1 | 25 | 66996 | 1.1551541513 | 18.31403 | 25 | 66996 | 1.1551541513 | 22.07079 |
1 | 38 | 774480 | 1.1551532609 | 260.06329 | 38 | 774480 | 1.1551532609 | 325.52552 |
1 | 39 | 923052 | 1.1551532590 | 320.61556 | 39 | 923052 | 1.1551532591 | 400.40419 |
1 | 40 | 1099044 | 1.1551532576 | 398.76151 | 40 | 1099044 | 1.1551532576 | 496.73607 |
3 | 1 | 4680 | 2.0159225406 | 0.46936 | 1 | 4680 | 2.0159225405 | 0.57221 |
3 | 9 | 19224 | 2.0137381688 | 4.00481 | 9 | 19224 | 2.0137381688 | 5.20982 |
3 | 23 | 976428 | 2.0136927626 | 233.96942 | 23 | 976428 | 2.0136927626 | 313.17266 |
3 | 24 | 1280052 | 2.0136927550 | 324.92148 | 24 | 1280052 | 2.0136927550 | 421.48383 |
3 | 25 | 1679592 | 2.0136927505 | 458.31975 | 25 | 1679592 | 2.0136927505 | 578.37647 |
4 | 10 | 11316 | 2.1259084959 | 3.98294 | 10 | 11316 | 2.1259084959 | 4.01302 |
4 | 21 | 105648 | 2.1257416544 | 27.89323 | 21 | 105648 | 2.1257416544 | 37.43586 |
4 | 32 | 822120 | 2.1257391878 | 297.07841 | 32 | 822120 | 2.1257391878 | 368.95873 |
4 | 33 | 980688 | 2.1257391755 | 362.49125 | 33 | 980688 | 2.1257391755 | 456.94719 |
4 | 34 | 1181328 | 2.1257391658 | 446.73985 | 34 | 1181328 | 2.1257391658 | 572.04947 |
6 | 1 | 4680 | 2.7468959498 | 0.40303 | 1 | 4680 | 2.7468959503 | 0.61874 |
6 | 3 | 5232 | 2.7405213916 | 0.92105 | 3 | 5232 | 2.7405213907 | 1.21365 |
6 | 23 | 1000704 | 2.7364519542 | 234.72412 | 23 | 1000704 | 2.7364519542 | 334.60146 |
6 | 24 | 1312716 | 2.7364519227 | 327.39089 | 24 | 1312716 | 2.7364519227 | 448.35877 |
6 | 25 | 1726080 | 2.7364519037 | 468.01995 | 25 | 1726080 | 2.7364519038 | 608.05296 |