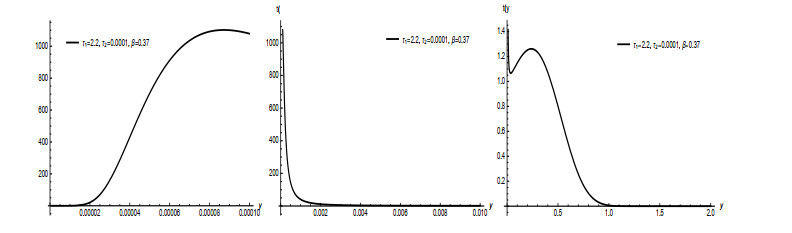
Statistical modeling and forecasting of time-to-events data are crucial in every applied sector. For the modeling and forecasting of such data sets, several statistical methods have been introduced and implemented. This paper has two aims, i.e., (i) statistical modeling and (ii) forecasting. For modeling time-to-events data, we introduce a new statistical model by combining the flexible Weibull model with the Z-family approach. The new model is called the Z flexible Weibull extension (Z-FWE) model, where the characterizations of the Z-FWE model are obtained. The maximum likelihood estimators of the Z-FWE distribution are obtained. The evaluation of the estimators of the Z-FWE model is assessed in a simulation study. The Z-FWE distribution is applied to analyze the mortality rate of COVID-19 patients. Finally, for forecasting the COVID-19 data set, we use machine learning (ML) techniques i.e., artificial neural network (ANN) and group method of data handling (GMDH) with the autoregressive integrated moving average model (ARIMA). Based on our findings, it is observed that ML techniques are more robust in terms of forecasting than the ARIMA model.
Citation: Rashad A. R. Bantan, Zubair Ahmad, Faridoon Khan, Mohammed Elgarhy, Zahra Almaspoor, G. G. Hamedani, Mahmoud El-Morshedy, Ahmed M. Gemeay. Predictive modeling of the COVID-19 data using a new version of the flexible Weibull model and machine learning techniques[J]. Mathematical Biosciences and Engineering, 2023, 20(2): 2847-2873. doi: 10.3934/mbe.2023134
[1] | Yinghui Zhou, Zubair Ahmad, Zahra Almaspoor, Faridoon Khan, Elsayed tag-Eldin, Zahoor Iqbal, Mahmoud El-Morshedy . On the implementation of a new version of the Weibull distribution and machine learning approach to model the COVID-19 data. Mathematical Biosciences and Engineering, 2023, 20(1): 337-364. doi: 10.3934/mbe.2023016 |
[2] | Saleh I. Alzahrani, Wael M. S. Yafooz, Ibrahim A. Aljamaan, Ali Alwaleedi, Mohammed Al-Hariri, Gameel Saleh . AI-driven health analysis for emerging respiratory diseases: A case study of Yemen patients using COVID-19 data. Mathematical Biosciences and Engineering, 2025, 22(3): 554-584. doi: 10.3934/mbe.2025021 |
[3] | Suzan Farhang-Sardroodi, Mohammad Sajjad Ghaemi, Morgan Craig, Hsu Kiang Ooi, Jane M Heffernan . A machine learning approach to differentiate between COVID-19 and influenza infection using synthetic infection and immune response data. Mathematical Biosciences and Engineering, 2022, 19(6): 5813-5831. doi: 10.3934/mbe.2022272 |
[4] | Vitaliy Yakovyna, Natalya Shakhovska . Modelling and predicting the spread of COVID-19 cases depending on restriction policy based on mined recommendation rules. Mathematical Biosciences and Engineering, 2021, 18(3): 2789-2812. doi: 10.3934/mbe.2021142 |
[5] | Haiyan Wang, Nao Yamamoto . Using a partial differential equation with Google Mobility data to predict COVID-19 in Arizona. Mathematical Biosciences and Engineering, 2020, 17(5): 4891-4904. doi: 10.3934/mbe.2020266 |
[6] | Michael James Horry, Subrata Chakraborty, Biswajeet Pradhan, Maryam Fallahpoor, Hossein Chegeni, Manoranjan Paul . Factors determining generalization in deep learning models for scoring COVID-CT images. Mathematical Biosciences and Engineering, 2021, 18(6): 9264-9293. doi: 10.3934/mbe.2021456 |
[7] | Shubashini Velu . An efficient, lightweight MobileNetV2-based fine-tuned model for COVID-19 detection using chest X-ray images. Mathematical Biosciences and Engineering, 2023, 20(5): 8400-8427. doi: 10.3934/mbe.2023368 |
[8] | Walid Emam, Ghadah Alomani . Predictive modeling of reliability engineering data using a new version of the flexible Weibull model. Mathematical Biosciences and Engineering, 2023, 20(6): 9948-9964. doi: 10.3934/mbe.2023436 |
[9] | Hamdy M. Youssef, Najat A. Alghamdi, Magdy A. Ezzat, Alaa A. El-Bary, Ahmed M. Shawky . A new dynamical modeling SEIR with global analysis applied to the real data of spreading COVID-19 in Saudi Arabia. Mathematical Biosciences and Engineering, 2020, 17(6): 7018-7044. doi: 10.3934/mbe.2020362 |
[10] | Qian Shen . Research of mortality risk prediction based on hospital admission data for COVID-19 patients. Mathematical Biosciences and Engineering, 2023, 20(3): 5333-5351. doi: 10.3934/mbe.2023247 |
Statistical modeling and forecasting of time-to-events data are crucial in every applied sector. For the modeling and forecasting of such data sets, several statistical methods have been introduced and implemented. This paper has two aims, i.e., (i) statistical modeling and (ii) forecasting. For modeling time-to-events data, we introduce a new statistical model by combining the flexible Weibull model with the Z-family approach. The new model is called the Z flexible Weibull extension (Z-FWE) model, where the characterizations of the Z-FWE model are obtained. The maximum likelihood estimators of the Z-FWE distribution are obtained. The evaluation of the estimators of the Z-FWE model is assessed in a simulation study. The Z-FWE distribution is applied to analyze the mortality rate of COVID-19 patients. Finally, for forecasting the COVID-19 data set, we use machine learning (ML) techniques i.e., artificial neural network (ANN) and group method of data handling (GMDH) with the autoregressive integrated moving average model (ARIMA). Based on our findings, it is observed that ML techniques are more robust in terms of forecasting than the ARIMA model.
The first infected case of the COVID-19 pandemic appeared in China during the last week of December 2019. Since that, the deadly pandemic has caused a dramatic loss of human life around the globe and presented a serious challenge to all sectors of life. For example, (i) to read about the COVID-19's effect on the economy, we refer to [1], (ii) education [2], (iii) healthcare [3], (iv) Business sectors [4], (v) sports sectors [5], and (vi) tourism sector [6].
Among the mentioned sectors affected by the COVID-19 epidemic, the healthcare sector is the most affected area. Based on the latest updates up to November 18, 2021, 10:36 GMT, around 255.9 million cases were registered worldwide, 5.1422 million people have died, and the total number of recovered cases has reached 231.26 million. The top five countries with the most registered COVID-19 cases are (i) United States of America (USA) with 48.29 million, (ii) India with 34.48 million, (iii) Brazil with 21.978 million, (iv) United Kingdom with 9.675 million, (v) Russia with 9.219 mission. The top five countries with the most death cases due to COVID-19 are (i) USA with 0.787 million, (ii) Brazil with 0.611 million, (iii) India 0.464 million, (iv) Mexico with 0.291 million, and (v) Russia with 0.260 million, https://www.worldometers.info/coronavirus.
The implementation of statistical models for dealing with lifetime data in healthcare sectors is very crucial and an important research area. In the available statistical distributions, the flexible weibull extension (FWE) model has attracted researchers [7]. The CDF (cumulative distribution function) M(y;κ) of the FWE model is
M(y;κ)=1−e−eτ1y−τ2y,y≥0, | (1.1) |
where κ=(τ1,τ2) with τ1>0 and τ2>0.
Corresponding to the CDF in Eq (1.1), the PDF (probability density function) m(y;κ) of the FWE distribution is
m(y;κ)=(τ1+τ2y2)eτ1y−τ2ye−eτ1y−τ2y,y>0. |
El-Gohary et al. [8] developed the inverse version of the FWE distribution with CDF, given by
M(y;κ)=e−eτ1y−τ2y,y≥0. |
El-Gohary et al. [9] further generalized the WFE model by using the exponentiation strategy with CDF, given by
M(y;θ1,κ)=(1−e−eτ1y−τ2y)θ1,θ1>0,y≥0. |
El-Damcese et al. [10] investigated another generalization of the FWE model by adopting the Kumaraswamy approach with CDF, given by
M(y;θ1,θ2,κ)=1−(1−(1−e−eτ1y−τ2y)θ1)θ2,θ1>0,θ2>0,y≥0. |
This paper introduces a further extension of the FWE model to improve the fitting power of the FWE model. To perform this activity, we combine M(y;κ)=1−e−eτ1y−τ2y with the Z-family approach [11]. The CDF T(y;β,κ) of the Z-family is
T(y;β,κ)=1−ˉM(y;κ)βM(y;κ),y∈R,β>0, | (1.2) |
with SF (survival function) S(y;β,κ)=1−T(y;β,κ), given by
S(y;β,κ)=ˉM(y;κ)βM(y;κ),y∈R, | (1.3) |
where ˉM(y;κ)=1−M(y;κ).
Corresponding to T(y;β,κ), the PDF t(y;β,κ)=ddyT(y;β,κ) and HF (hazard function) h(y;β,κ)=t(y;β,κ)1−T(y;β,κ) are given by
t(y;β,κ)=m(y;κ)βM(y;κ)[1+(logβ)ˉM(y;κ)],y∈R, |
and
h(y;β,κ)=m(y;κ)ˉM(y;κ)[1+(logβ)ˉM(y;κ)],y∈R, |
respectively.
By incorporating M(y;κ)=1−e−eτ1y−τ2y in Eq (1.2), we obtain a new model, namely, the Z-flexible Weibull extension (Z-FWE) distribution. The Z-FWE is a more flexible version of the FWE model. This fact is illustrated by applying the Z-FWE distribution to a data set in the health sector.
If Y has the Z-FWE model with parameters β>0,τ1>0, and τ2>0, its CDF is
T(y;β,κ)=1−e−eτ1y−τ2yβ(1−e−eτ1y−τ2y),y≥0, | (2.1) |
with PDF
t(y;β,κ)=(τ1+τ2y2)eτ1y−τ2ye−eτ1y−τ2yβ(1−e−eτ1y−τ2y)[1+(logβ)e−eτ1y−τ2y],y>0, | (2.2) |
respectively.
Different behaviors for the PDF of the Z-FWE distribution are shown visually in Figures 1 and 2.
Proposition 1. The PDF of the proposed model has a Unimodal (U) shape of bimodal (B) shape.
Proof. The first derivative of the PDF of the Z-FWE distribution model is determined as follows
ddyt(y;β,κ)=eτ1y−3eτ1y−τ2y−2τ2yβe−eτ1y−τ2y−1y4eτ2y+eτ1y−τ2y[τ22+τ21y4+2τ2y(τ1y−1)]×(log(β)+eeτ1y−τ2y)−eτ1y−3eτ1y−τ2y−2τ2yβe−eτ1y−τ2y−1y4×eτ1y(τ2+τ1y2)2(log2(β)+3log(β)eeτ1y−τ2y+e2eτ1y−τ2y), |
ddyt(y;β,κ)=eτ1y−3eτ1y−τ2y−2τ2yβe−eτ1y−τ2y−1y4L(y;β,κ), |
where
L(y;β,κ)=Z(y;β,κ)[τ22+τ21y4+2τ2y(τ1y−1)]−R(y;β,κ)(log2(β)+3log(β)eeτ1y−τ2y+e2eτ1y−τ2y). |
The terms Z(y;β,κ) and R(y;β,κ) are given by
Z(y;β,κ)=eτ2y+eτ1y−τ2y(log(β)+eeτ1y−τ2y), |
and
R(y;β,κ)=eτ1y(τ2+τ1y2)2, |
respectively.
We can see that ddyt(y;β,κ) and L(y;β,κ) have the same signs. Also, Z(y;β,κ) and R(y;β,κ)>0 ∀y,τ1>0,τ2>0, and β≥0.37. When the sign of L(y;β,κ) changes from + to −, then there is a unique critical point which maximize the PDF of the Z-FWE distribution and give a unimodal shape. When the sign of L(y;β,κ) changes from + to − twice, then there are two critical points which maximize the PDF of the Z-FWE distribution and give a bimodal shape.
The unimodal band biomodal behaviors of the PDF of the Z-FWE distribution are also proved numerically. The numerical results in Table 1 confirm the unimodal and bimodal behaviors of the PDF of the Z-FWE distribution.
Parameters values | Measures | Measures values | Results | |||||||||
τ1=0.0001 | τ2=0.00001 | β=0.37 | y ⟶ | 10−8 | 10−6 | 0.5 | 1.5 | 15 | 50 | 10000 | 100000 | B PDF |
L ⟶ | 1.13007×10422 | 1.03080×10−8 | -0.000046 | -0.00014 | 0.00083 | 0.2729 | -2.8949×1010 | -1.9409×1019148 | ||||
τ1=0.001 | τ2=0.01 | β=0.75 | y ⟶ | 10−8 | 10−6 | 0.5 | 1.5 | 15 | 50 | 10000 | 20000 | B PDF |
L ⟶ | 2.1606×10434290 | 6.27199×104338 | -0.0645362 | -0.197144 | -1.96613 | 0.622783 | -1.8573×1019146 | -3.47908×10421408943 | ||||
τ1=0.1 | τ2=0.25 | β=1.5 | y ⟶ | 10−8 | 10−6 | 0.5 | 1.5 | 15 | 50 | 80 | 100 | U PDF |
L ⟶ | 9.8012×1010857360 | 3.6658×10108572 | -1.7303 | -7.8697 | -1.23457×107 | -1.7075×10135 | -1.71954×102590 | -3.2969×1019094 | ||||
τ1=2.2 | τ2=0.0001 | β=0.37 | y ⟶ | 10−8 | 10−6 | 10−4 | 0.01 | 0.1 | 0.2 | 5 | 10 | B PDF |
L ⟶ | 5.0609×104332 | 1.51416×1033 | -5.34296×10−9 | -9.302293×10−6 | 0.00239836 | 0.0251161 | -1.72409×1052013 | -3.94217×103113784610 | ||||
τ1=2.4 | τ2=5.5 | β=1.1 | y ⟶ | 10−8 | 10−6 | 10−4 | 0.01 | 0.1 | 0.2 | 5 | 10 | U PDF |
L ⟶ | 3.6902×10238861966 | 1.48159×102388621 | 5.2089×1023887 | 2.40261×10240 | 2.47911×1025 | 2.79741×1013 | -6.8501×1047065 | -8.05508×1013274553069 | ||||
τ1=2.4 | τ2=2.5 | β=1.1 | y ⟶ | 10−8 | 10−6 | 10−4 | 0.01 | 0.1 | 0.2 | 5 | 10 | U PDF |
L ⟶ | 2.04752×10108573621 | 1.0969×101085737 | 1.5755×1010858 | 2.54468×10109 | 4.62999×1011 | 1.68683×106 | -1.7082×1085752 | -5.22575×1017918772372 | ||||
τ1=1.5 | τ2=1.5 | β=1.0 | y ⟶ | 10−8 | 10−6 | 10−4 | 0.01 | 0.1 | 3 | 10 | 15 | U PDF |
L ⟶ | 4.341804×1065144172 | 1.1886×10651442 | 5.8796×106514 | 3.09466×1065 | 6.52243×106 | -5.27417×1051 | -7.1416×101169 | -1.78388×104645267231 |
Remark 1. The plots in Figure 1 are actually one plot, but we divided them into three plots to show that the PDF of the Z-FWE distribution can be bimodal. If we combine them in one plot, then, the bimodal shape of the Z-FWE distribution doesn't appear due to the range of the function. The actual range of the plot is from 0.00001 to 2. We have divided this range into three pieces, such as (i) 0.00001 to 0.00010 (ii) 0.00010 to 0.010, and (iii) 0.010 to 2.
This section offers the heavy-tailed behavior and regular variational results of the Z-FWE distribution. Probability distributions that are right-skewed and possess heavy-tailed behavior are very useful in providing the best description of the biomedical data sets. A probability model is called a heavy-tailed distribution, if it satisfies
limy⟶∞epy[1−T(y;β,κ)]=∞,∀p>0. |
An important property of the heavy-tailed probability distributions is called the regular variational property (RVP). A probability distribution is called regularly varying, if it satisfies
1−T(py;β,κ)1−T(y;β,κ)=pa,∀p>0,a>0, |
where a represents an index of regular variation.
Here, we derive the RVP of the Z-FWE distribution. According to Karamata's theorem (Seneta [12]), in terms of SF S(y;β,κ), we have
Theorem: If S(y;κ)=1−M(y;κ) is the SF of the regular varying model, then S(y;β,κ) is also a regular varying distribution.
Proof: Suppose limy⟶∞S(py;β,κ)S(y;β,κ)=f(p) is finite but non-zero ∀p>0. Then, using Eq (1.3), we have
S(py;β,κ)S(y;β,κ)=S(py;κ)eM(y;κ)S(y;κ)eM(py;κ). | (3.1) |
Applying limy⟶∞ on both sides of Eq (3.1), we get
limy⟶∞S(py;β,κ)S(y;β,κ)=limy⟶∞S(py;κ)S(y;κ)×eM(y;κ)eM(py;κ). | (3.2) |
Using Eq (1.1) in Eq (3.2), we get
limy⟶∞S(py;β,κ ) S(y;β,κ ) =limy⟶∞S(py;κ ) S(y;κ ) ×e(1−e−eτ1y−τ2y ) e(1−e−eτ1(py)−τ2(py) ) , |
limy⟶∞S(py;β,κ ) S(y;β,κ ) =limy⟶∞S(py;κ ) S(y;κ ) ×e(1−e−eτ1∞−τ2∞ ) e(1−e−eτ1(p∞)−τ2(p∞) ) , |
limy⟶∞S(py;β,κ ) S(y;β,κ ) =limy⟶∞S(py;κ ) S(y;κ ) ×e(1−e−e∞−0 ) e(1−e−eτ1(∞)−τ2(∞) ) , |
limy⟶∞S(py;β,κ ) S(y;β,κ ) =limy⟶∞S(py;κ ) S(y;κ ) ×e(1−e−e∞ ) e(1−e−e∞−0 ) , |
limy⟶∞S(py;β,κ ) S(y;β,κ ) =limy⟶∞S(py;κ ) S(y;κ ) ×e(1−e−∞ ) e(1−e−∞ ) , |
limy⟶∞S(py;β,κ ) S(y;β,κ ) =limy⟶∞S(py;κ ) S(y;κ ) ×e(1−0 ) e(1−0 ) , |
limy⟶∞S(py;β,κ ) S(y;β,κ ) =limy⟶∞S(py;κ ) S(y;κ ) ×1, |
limy⟶∞S(py;β,κ)S(y;β,κ)=f(p), | (3.3) |
where f(p) has the form pa. So, the expression in Eq (3.3) is finite and non-zero ∀p>0. thus, S(py;β,κ) is a regular varying distribution.
This section offers the characterizations of the Z-FWE distribution via implementing three different approaches. These characterizations are obtained using (i) a simple relationship between two TMs (truncated moments), (ii) the HF, and (iii) the CE (conditional expectation) of a function of the RV (random variable).
The result of the first characterization is obtained due to the theorem in Glänzel [13], see the below Theorem 1. It is important to note that the result of the first characterization also holds true when the interval expressed by W is not closed.
Furthermore, it could also be implemented when the CDF F is not in closed form. As Glänzel [14] showed that this characterization is stable even under weak convergence.
Theorem 1. Consider a given PS (probability space) (Ω,F,P) and let W=[θ1,θ2] represents an interval for some θ1<θ2(θ1=−∞,θ2=∞ might as well be allowed). Now, let Y:Ω→W be a continuous RV with the CDF T and let g(.) and h(.) be two real functions defined on the interval W such that
E[g(Y) | Y≥y]=E[h(Y) | Y≥y]ϑ(y),y∈W, |
is defined with some real function ϑ. Let suppose that g, h∈K1(W), ϑ∈K2(W) and T is twice CD (continuously differentiable) and strictly MF (monotone function) on the set W. Also, let assume that the equation ϑh=g has no real solution in the interior of W. Then T is uniquely determined by the functions g, h and ϑ, particularly
T(y)=∫yaK|ϑ′(v)ϑ(v)h(v)−g(v)|exp(−s(v))dv, |
where s is a solution of the DE (differential equation) given by s′=ϑ′ hϑ h −g and the quantity K represents a normalization constant, such that ∫WdT=1.
Proposition 2. Let Y:Ω→(0,∞) be a continuous RV and let h(y)=[1+log(β)e−eτ1y−τ2y]−1 and g(y)=h(y)e−eτ1y−τ2y for y>0. The RV Y has PDF in Eq (2.2) if and only if the function ϑ defined in Theorem 1 has the form
ϑ(y)=12e−eτ1y−τ2y, y>0. |
Proof. Let Y be a RV with PDF in Eq (2.2), then
(1−T(y))E[h(Y) | Y≥y]=e−eτ1y−τ2y, y>0, |
and
(1−T(y))E[h(Y) | Y≥y]=12e−2eτ1y−τ2y, y>0, |
and finally
ϑ(y)h(y)−g(y)=−12h(y)e−eτ1y−τ2y<0 for y>0. |
Conversely, if the function κ is given as mentioned above, then
s′(y)=ϑ′(y)h(y)ϑ(y)h(y)−g(y)=(τ1+τ2y2)eτ1y−τ2y, |
and hence
s(y)=eτ1y−τ2y, y>0. |
Now, in view of the result in Theorem 1, Y has PDF in Eq (2.2).
Corollary 1. Consider a RV Y:Ω→(0,∞) and let the function h(y) be as defined in Proposition 2. The PDF of Y is Eq (2.2), if and only if the functions g and ϑ defined in Theorem 1 satisfying the below DE
ϑ′(x)h(x)ϑ(x)h(x)−g(x)=(τ1+τ2y2)eτ1y−τ2y, y>0. |
Corollary 2. The general solution of the DE in Corollary 1 is
ϑ(y)=eeτ1y−τ2y[−∫(τ1+τ2y2)eτ1y−τ2ye−eτ1y−τ2y(h(y))−1g(y)+C], |
where the term C is a constant quantity. It should be noted that a set of functions obeying the DE provided above is given in Proposition 2 with C=0.
It is obvious that the HF, hT, of a twice differentiable DF, T with PDF t, obeys the first order DE
t ′(y)t (y)=h′T(y)hT(y)−hT(y). |
In terms of the HF, this is the only characterization available for many univariate CMs (continuous models). In terms of the HF, the following characterization establishes a characterization of Z-FWE distribution, which is not of the trivial form proivded above.
Proposition 3. Consider Y:Ω→(0,∞) be a continuous RV. Then Y has the PDF in Eq (2.2), if and only if its HF hT(y) obyes the DE
h′T(y)−(τ1+τ2y2)hT(y)=−eτ1y−τ2y−eτ1y−τ2y{2τ2y3+(τ1+τ2y2)2}, y>0, |
with the initial condition given by limy→0hT(y)=0.
Proof. Is straightforward and hence omitted.
The CE is a well-known approach to characterize statistical distributions; see Hamedani [15]. Here, we use this approach to characterize the Z-FWE distribution.
Proposition 4. Let Y:Ω→ (λ1,λ2) be a continuous RV with CDF T. Let φ(y) be a differentiable function on (λ1,λ2) with limy→0+φ(y)=1. Then for η≠1,
E[φ(Y) | Y≥y]=ηφ(y),y∈(λ1,λ2), |
if and only if
φ(y)=(1−F(y))1η−1,y∈(λ1,λ2). |
Remark 2. For (λ1,λ1)=(0,∞) , φ(y)=e−12eτ1y−τ2yβ12(1−e−eτ1y−τ2y) and η=23, Proposition 2 provides a characterization of Z-FWE distribution.
This section is composed of two subsections. The very first subsection offers the derivation of the estimators (^τ1,^τ2,ˆβ) of the parameters (τ1,τ2,β) of the Z-FWE distribution. Whereas, the second subsection, offers the evaluation of ^τ1,^τ2 and ˆβ through a simulation study.
Here, we obtain the estimators ^τ1,^τ2 and ˆβ of the parameters of the Z-FWE model τ1,τ2 and β, respectively. The estimators ^τ1,^τ2 and ˆβ are obtained by using five different approaches. These methods are the (i) maximum likelihood estimation, (ii) ordinary least-square estimation, (iii) weighted least-square estimation, (iv) maximum product of spacing estimation, and (v) Anderson-Darling estimation.
Consider a RS (random sample) say y1,y2,...,yn taken from t(y;β,κ). Then in link to t(y;β,κ), the LF (likelihood function) λ(y;β,κ) is
λ(y;β,κ)=n∏k=1t(yk;β,κ). | (5.1) |
Using Eq (2.2) in Eq (5.1), we have
λ(y;β,κ)=n∏k=1(τ1+τ2y2k)eτ1yk−τ2yke−eτ1yk−τ2ykβ(1−e−eτ1yk−τ2yk)[1+(logβ)e−eτ1yk−τ2yk]. | (5.2) |
Corresponding to Eq (5.2), the log LF δ(y;β,κ) is given by
δ(y;β,κ)=n∑k=1log(τ1+τ2y2k)+n∑k=1(τ1yk−τ2yk)−n∑k=1(1−e−eτ1yk−τ2yk)logβ−n∑k=1eτ1yk−τ2yk+n∑k=1log[1+(logβ)e−eτ1yk−τ2yk]. |
With respect to τ1,τ2 and β, the partial derivatives of δ(y;β,κ) are given by
∂∂τ1δ(y;β,κ)=n∑k=11(τ1+τ2y2k)+n∑k=1yk−n∑k=1(logβ)ykeτ1yk−τ2yke−eτ1yk−τ2yk−n∑k=1ykeτ1yk−τ2yk−n∑k=1(logβ)ykeτ1yk−τ2yke−eτ1yk−τ2yk[1+(logβ)e−eτ1yk−τ2yk], |
∂∂τ2δ(y;β,κ)=n∑k=11y2k(τ1+τ2y2k)−n∑k=11y2k+n∑k=1(logβ)y2keτ1yk−τ2yke−eτ1yk−τ2yk+n∑k=11y2keτ1yk−τ2yk+n∑k=1(logβ)y2keτ1yk−τ2yke−eτ1yk−τ2yk[1+(logβ)e−eτ1yk−τ2yk], |
and
∂∂βδ(y;β,κ)=−n∑k=1(1−e−eτ1yk−τ2yk)β+n∑k=11βe−eτ1yk−τ2yk[1+(logβ)e−eτ1yk−τ2yk], |
respectively.
In solving ∂∂τ1δ(y;β,κ)=0, ∂∂τ2δ(y;β,κ)=0, and ∂∂βδ(y;β,κ)=0, we get the maximum likelihood estimators (MLEs) ^τ1,^τ2 and ˆβ, respectively.
Let y(1),y(2),⋯,y(n) be the order statistics of a sample of size n from T(y;β,κ) in Eq (1.2). The ordinary least-squares estimators (OLSEs) ˆβOLSE and ˆκOLSE can be obtained by minimizing
V(β,κ)=n∑k=1[T(y(k)|β,κ)−in+1]2, |
with respect to β and κ. Or equivalently, the OLSEs follow by solving the non-linear equations
n∑k=1[T(y(k)|β,κ)−in+1]Δm(y(k)|β,κ)=0,m=1,2, |
where
Δ1(y(k)|β,κ)=∂∂βT(y(k)|β,κ), |
and
Δ2(y(k)|β,κ)=∂∂κT(y(k)|β,κ). |
Note that the solution of Δm for m=1,2 can be obtained numerically.
The weighted least-squares estimators (WLSEs) ˆβWLSE and ˆκWLSE can be obtained by minimizing
W(β,κ)=n∑k=1(n+1)2(n+2)k(n−k+1)[T(y(k)|β,κ)−kn+1]2. |
Moreover, the WLSEs can also be obtained by solving the non-linear equations
n∑k=1(n+1)2(n+2)k(n−k+1)[T(y(k)|β,κ)−kn+1]2Δm(y(k)|β,κ)=0,m=1,2. |
Let Dk(β,κ)=T(y(k)|β,κ)−T(y(k−1)|β,κ), for k=1,2,…,n+1, be the uniform spacing of a random sample from the Z-FWE distribution, where T(y(0)|β,κ)=0, T(y(n+1)|β,κ)=1, and ∑n+1k=1Dk(β,κ)=1. Then, the maximum product of spacing estimators (MPSEs) ˆβMPSE and ˆκMPSE can be obtained by maximizing the geometric mean of the spacing
T(β,κ)=[n+1∏k=1Dk(β,κ)]1n+1, |
with respect to β and κ, or, equivalently, by maximizing the logarithm of the geometric mean of sample spacing
H(β,κ)=1n+1n+1∑k=1logDk(β,κ). |
The MPSEs of the Z-FWE parameters can be obtained by solving the nonlinear equations defined by
1n+1n+1∑k=11Dk(β,κ)[Δm(y(k)|β,κ)−Δm(y(k−1)|β,κ)]=0,m=1,2. |
The Anderson-Darling estimators (ADEs) of the parameters of the Z-FWE distribution are obtained by minimizing
A(β,κ)=−n−1nn∑k=1(2k−1)[logT(y(k)|β,κ)+logS(y(k)|β,κ)], |
with respect to β and κ. These ADEs can also be obtained by solving the non-linear equations
n∑k=1(2k−1)[Δm(y(k)|β,κ)T(y(k)|β,κ)−Δj(y(n+1−k)|β,κ)S(y(n+1−k)|β,κ)]=0,m=1,2. |
Furthermore, we investigate the performances of ^τ1,^τ2, and ˆβ via a simulation study. To carry out the evaluation of ^τ1,^τ2, and ˆβ, random samples, say, n=20,50,150,300 are generated from t(y;β,κ) using the inverse DF approach.
The evaluation of ^τ1,^τ2, and ˆβ has been done for three sets of parameters values such as (a) ^τ1=0.6,^τ2=1.3,ˆβ=0.9 (b) ^τ1=0.12,^τ2=0.8,ˆβ=1.2, and (c) ^τ1=1.3,^τ2=1.5,ˆβ=0.7.
Furthermore, certain statistical methodologies such as (i) MSEs and (ii) absolute biases were selected to check the performances of ^τ1,^τ2, and ˆβ. The values of the selected measures were respectively obtained using the expressions
MSE(ˆϵ)=1600n∑k=1(ˆϵ−ϵ)2, |
and
|Bias(ˆϵ)|=|1600n∑k=1(ˆϵ−ϵ)|, |
where ˆϵ=(β,κ).
Corresponding to (a) ^τ1=0.6,^τ2=1.3,ˆβ=0.9 (b) ^τ1=0.12,^τ2=0.8,ˆβ=1.2, and (c) ^τ1=1.3,^τ2=1.5,ˆβ=0.7. the simulation results of the Z-FWE model are provided in Table 2.
p | Criteria | MLE | LSE | WLSE | MPSE | ADE |
τ1=0.8,τ2=1.3,β=0.9 | ||||||
20 | |Bias| | 0.12745895 | 0.12984254 | 0.13145896 | 0.16369694 | 0.14369857 |
MSE | 0.01369548 | 0.01699527 | 0.01936955 | 0.03265855 | 0.02968856 | |
50 | |Bias| | 0.07023698 | 0.08593632 | 0.09745269 | 0.11236985 | 0.07452996 |
MSE | 0.00696585 | 0.00742696 | 0.00892024 | 0.01496657 | 0.00639957 | |
150 | |Bias| | 0.04025955 | 0.04128963 | 0.04593582 | 0.05985352 | 0.04236958 |
MSE | 0.00230288 | 0.00285295 | 0.00369021 | 0.00597102 | 0.00283015 | |
300 | |Bias| | 0.02336951 | 0.02478037 | 0.03352865 | 0.03259560 | 0.03252014 |
MSE | 0.00112023 | 0.00141260 | 0.00123695 | 0.00289635 | 0.00147848 | |
τ1=0.12,τ2=0.8,β=1.2 | ||||||
20 | |Bias| | 0.14789622 | 0.15236974 | 0.14890395 | 0.18930325 | 0.17485630 |
MSE | 0.02023985 | 0.03636920 | 0.03630215 | 0.05014790 | 0.04012856 | |
50 | |Bias| | 0.09639519 | 0.10303255 | 0.10920203 | 0.12306954 | 0.11425036 |
MSE | 0.01021589 | 0.01636985 | 0.01547496 | 0.02036991 | 0.01636982 | |
150 | |Bias| | 0.04852036 | 0.06696350 | 0.06745622 | 0.07412696 | 0.07012650 |
MSE | 0.00523694 | 0.00530236 | 0.00595621 | 0.00669521 | 0.00610236 | |
300 | |Bias| | 0.03203695 | 0.03365541 | 0.04012556 | 0.05120356 | 0.03746222 |
MSE | 0.00210236 | 0.00242369 | 0.00345890 | 0.00463697 | 0.00323405 | |
τ1=1.3,τ2=1.5,β=0.7 | ||||||
20 | |Bias| | 0.66256985 | 0.67458852 | 0.68520136 | 0.73265996 | 0.69203214 |
MSE | 0.25369414 | 0.25896894 | 0.28957748 | 0.29652120 | 0.26358544 | |
50 | |Bias| | 0.31258967 | 0.32369841 | 0.33695252 | 0.38569520 | 0.33737712 |
MSE | 0.11482069 | 0.12987039 | 0.13698549 | 0.14529602 | 0.12369501 | |
150 | |Bias| | 0.18595452 | 0.19395225 | 0.20369521 | 0.24473699 | 0.21032369 |
MSE | 0.03636654 | 0.03852178 | 0.03715985 | 0.04236985 | 0.03920369 | |
300 | |Bias| | 0.11532684 | 0.12399596 | 0.10158697 | 0.15639023 | 0.12699529 |
MSE | 0.00936998 | 0.00985632 | 0.01058554 | 0.01239857 | 0.01158859 |
This section is devoted to data analysis to illustrate the crucial role of the Z-FWE in data modeling. To carry out the illustration of the Z-FWE model, a data set from the medical sector is considered. The data consists of one hundred and six (106) observations and represents the mortality rate of patients during the COVID-19 pandemic in Mexico. This was recorded during the period between March 4, 2020, to July 20, 2020; see Almongy et al. [16]. For the simplicity of analysis, each observation is divided by five and is given by: 1.7652, 1.2210, 1.8782, 2.9924, 2.0766, 1.4534, 2.6440, 3.2996, 2.3330, 1.2030, 2.1710, 1.2244, 1.3312, 0.6880, 1.1708, 2.1370, 2.0070, 1.0484, 0.8688, 1.0286, 1.5260, 2.9208, 1.5806, 1.2740, 0.7074, 1.2654, 0.9460, 0.6430, 1.8568, 2.5756, 1.7626, 2.0086, 1.4520, 1.1970, 1.2824, 0.6790, 0.8848, 1.9870, 1.5680, 1.9100, 0.6998, 0.7502, 1.3936, 0.6572, 2.0316, 1.6216, 1.3394, 1.4302, 1.3120, 0.4154, 0.7556, 0.5976, 0.6672, 1.3628, 1.6650, 1.5708, 1.7102, 0.6456, 1.4972, 1.3250, 1.2280, 0.9818, 0.9322, 1.0784, 2.4084, 1.7392, 0.3630, 0.6654, 1.0812, 1.2364, 0.2082, 0.3600, 0.9898, 0.8178, 0.6718, 0.4140, 0.6596, 1.0634, 1.0884, 0.9114, 0.8584, 0.5000, 1.3070, 0.9296, 0.9394, 1.0918, 0.8240, 0.7844, 0.6438, 0.2804, 0.4876, 0.6514, 0.7264, 0.6466, 0.6054, 0.4704, 0.2410, 0.6436, 0.5852, 0.5202, 0.4130, 0.6058, 0.4116, 0.4652, 0.5012, 0.3846.
The summary measures of this data are: smallest observation (minimum) = 0.2082, 1st Quartile = 0.6578, Median = 1.0559, Mean = 1.1645, 3rd Quartile = 1.5188, the largest value (maximum) = 3.2996, variance = 0.4225, and standard deviation = 0.6500. Corresponding to the mortality rate data, the total time test (TTT) plot is obtained in Figure 3. In addition to the TTT plot, the histogram of the mortality rate data is also provided within the same figure.
Using the given mortality rate data, we show the closer-fitting capability of the Z-WFE distribution. To perform the numerical illustration of the Z-FWE distribution, its comparison is done with some well-known competing models. These models include the (i) baseline FWE model, (ii) the exponentiated version of the FWE distribution, namely, exponentiated FWE (E-FWE), (iii) Weibull model, (iv) a generalization of the Weibull, namely, exponentiated Weibull (E-Weibull) distribution, (v) another famous extension of the Weibull is called, the Kumaraswamy Weibull (K-Weibull) distribution, (v) another generalized form of the Weibull model, namely, a modified new flexible Weibull extension (MNFWE) distribution, and (vi) a new modified flexible Weibull extension (NMFWE) distribution.
The above-mentioned competing models are very popular for modeling and describing real-phenomena of nature. The SFs of the selected competing models are
● FWE
M(y;κ)=e−eτ1y−τ2y,y≥0, |
where τ1>0 and τ2>0.
● E-FWE
M(y;θ1,κ)=(1−e−eτ1y−τ2y)θ1,y≥0, |
where τ1>0, τ2>0, and θ1>0.
● Weibull
M(y;κ)=1−e−τ2yτ1,y≥0, |
where τ1>0 and τ2>0.
● E-Weibull
M(y;θ1,κ)=(1−e−τ2yτ1)θ1,y≥0, |
where τ1>0, τ2>0, and θ1>0.
● K-Weibull
M(y;θ1,θ2,κ)=1−[1−(1−e−τ2yτ1)θ1]θ2,y≥0, |
where τ1>0, τ2>0, θ1>0, and θ2>0.
● MNFWE
M(y;θ,λ,κ)=1−e−λ{e(τ2yτ1+θy) },y≥0, |
where τ1>0, τ2>0, θ>0, and λ>0.
● NMFWE
M(y;λ,κ)=λ−λe−eτ1y−τ2yλ−e−eτ1y−τ2y,y≥0. |
After selecting the competing models, the very next step is to choose statistical tools to evaluate the performance of the Z-FWE and other models. For the evaluation of these distributions, certain statistical tools and tests were chosen. These tools are given by
● Akaike information criterion
The Akaike information criterion (AIC) is a mathematical approach for testing and evaluating how close/well a probability model fits the given data. In the literature, the AIC is calculated to compare different selected competing models and determine which one better fits the data set under consideration. In this paper, we consider the AIC to compare the fitting results of the Z-FWE distribution with other considered competing distributions. It is calculated as
AIC=2k−2ℓ. |
● Bayesian information criterion
Another criterion that we considered as a comparative tool is called the Bayesian information criterion (BIC). It is also called the Schwarz information criterion. For a given data set, the BIC also determine the best competing model among a finite set of models. It is calculated as
BIC=klog(n)−2ℓ. |
● Corrected Akaike information criterion
The Corrected Akaike information criterion (CAIC) is another useful statistical tool for checking the quality of the fitting of the possible competing distributions. For a given data set, a probability model with the lowest value of CAIC is considered the best competing distribution. The CAIC is calculated as
CAIC=2nkn−k−1−2ℓ. |
● Hannan–Quinn information criterion
The Hannan–Quinn information criterion (HQIC) is another statistical criterion for model selection among a set of possible competing statistical distributions. It can be used as an alternative to AIC and BIC for checking the fitting power of the competing distributions. It is given as
HQIC=2klog(log(n))−2ℓ. |
● Anderson Darling test
The Anderson Darling (AD) test is a well-known statistical procedure to check whether a sample of the underlined data is drawn from a given statistical distribution. The AD test is most often implemented in contexts where a family of statistical distributions is being tested. The AD test statistic is calculated as
AD=−n−1nn∑l=1(2l−1)[logT(ul)+log{1−T(un−l+1)}]. |
● Cramér-von Mises test
The Cramér-von Mises (CM) test is another useful statistical approach used for judging the goodness of fit of the given probability distributions. The CM test statistic is calculated as
CM=112n+n∑l=1[2l−12n−T(ul)]2. |
● Kolmogorov-Smirnov test
The Kolmogorov–Smirnov (KS) test is a prominent statistical approach that is used to quantify the distance between the empirical CDF of the sample and the CDF of the reference probability distribution. A lower distance between the empirical CDF and the CDF of the probability indicates the best fitting of the corresponding probability distribution. The KS test statistic is calculated as
KS=supu[Tn(u)−T(u)]. |
Furthermore, another statistical quantity called p-value is also considered a tool for the illustration of the fitted model. A higher p-value associated with any model indicates the best competitor among the competing models.
After performing the analysis, the numerical values of ^τ1,^τ2,ˆβ,^θ1,^θ2,ˆθ, and ˆλ are presented in Table 3. Whereas, the values of the comparative tools are presented in Tables 4 and 5.
Model | ^τ1 | ^τ2 | ˆβ | ^θ1 | ^θ2 | ˆθ | ˆλ |
Z-FWE | 0.60578 (0.06790) |
1.30376 (0.20545) |
1.52213 (0.68089) |
- | - | - | - |
FWE | 0.64201 (0.05039) |
1.11759 (0.10961) |
- | - | - | - | - |
E-FWE | 0.65089 (0.11588) |
1.35112 (2.14650) |
- | 0.82677 (1.39368) |
- | - | - |
Weibull | 1.92159 (0.14090) |
0.58694 (0.07121) |
- | - | - | - | - |
E-Weibull | 1.00398 (0.32020) |
1.78865 (0.75560) |
- | 4.02508 (3.10070) |
- | - | - |
K-Weibull | 1.44294 (0.14370) |
3.76192 (NaN) |
- | 3.13605 (1.63131) |
0.24665 (NaN) |
- | - |
NMFWE | 0.61568 (0.06012) |
1.33469 (0.20807) |
- | - | - | - | 2.86140 (1.76820) |
MNFWE | 1.45714 (0.17835) |
1.52803 (0.90054) |
- | - | - | 4.11707 (1.05217) |
0.038181 (0.00983) |
Model | AIC | CAIC | BIC | HQIC |
Z-FWE | 186.07860 | 186.31390 | 194.06900 | 189.31720 |
FWE | 189.04580 | 189.16230 | 196.37270 | 191.20480 |
E-FWE | 187.01970 | 187.25500 | 195.01000 | 190.25820 |
Weibull | 191.38590 | 191.50240 | 196.71280 | 193.54490 |
E-Weibull | 188.2469 0 | 188.48220 | 196.23720 | 191.48540 |
K-Weibull | 189.18680 | 189.58290 | 199.84060 | 193.50490 |
NMFWE | 186.12600 | 186.36130 | 194.11630 | 189.36450 |
MNFWE | 214.87380 | 215.26980 | 225.52750 | 219.19180 |
Model | CM | AD | KS | p-value |
Z-FWE | 0.03228 | 0.20038 | 0.04683 | 0.97420 |
FWE | 0.03963 | 0.26343 | 0.05313 | 0.92580 |
E-FWE | 0.03866 | 0.25671 | 0.05589 | 0.89500 |
Weibull | 0.10233 | 0.65790 | 0.06967 | 0.68220 |
E-Weibull | 0.05380 | 0.29853 | 0.06758 | 0.71820 |
NMFWE | 0.03276 | 0.20485 | 0.05085 | 0.94680 |
MNFWE | 0.26322 | 1.70311 | 0.09473 | 0.29740 |
From the numerical illustrations in Tables 4 and 5, we observe that the Z-FWE distribution is the best competitor for modeling the mortality rate data. For the Z-FWE distributions, the numerical values of the selected measures are AIC = 186.07860, CAIC = 186.31390, BIC = 194.06900, HQIC = 189.31720, CM = 0.03228, AD = 0.20038, KS = 0.04683, with p-value = 0.97420. Whereas, the second best model is the NMFWE distribution. For the NMFWE distribution, the values of the analytical measures are AIC = 186.12600, CAIC = 186.36130, BIC = 194.11630, HQIC = 189.36450, CM = 0.03276, AD = 0.20485, KS = 0.05085, and p-value = 0.94680.
From the above discussion, as well as the results in Tables 4 and 5, it is now obvious that the Z-FWE is the best choice for modeling the mortality rate data. Visual support for the numerical illustrations is presented in Figure 4. For the visual illustration of the Z-FWE distribution, the plots of the CDF, SF, PP, and QQ functions were obtained. These plots visually confirm the best fitting of the Z-FWE distribution.
In the previous section, we compared several distributions with the Z-FWE model using the mortality rate of patients due to the COVID-19 pandemic in Mexico. In this section, the study compares time series and machine learning techniques through forecasting on the same data set. Autoregressive Integrated Moving Average is a time series model, whereas Artificial Neural Network and group methods of data handling. For comparison purposes, we divide the data set into two parts: a training set and a testing set. The training set consists of 80 percent data and the testing set consists of 20 percent data, followed by [17]. The models are estimated on training data and then their performance is assessed on testing data. Below, the following methods are elaborated.
Numerous linear models have been devised in the literature on time series forecasting. Among them, the ARIMA model is the most well-known and frequently used over the previous few decades that has enjoyed fruitful applications in engineering, economics, and finance [18]. Basically, the ARIMA model has included the components, the autoregressive (AR) and moving average (MA). If the underlying time series is non-stationary, then it is transformed by using differencing approach [19].
ARIMA model specification is needed in several steps. In the initial step, we apply the autocorrelation function (ACF) and partial autocorrelation (PACF) to determine the order of AR and MA terms included in the model. In the second step, the estimated model is passed through diagnostic tests. If the estimated model is not suitable, a new tentative model should be diagnosed and will be followed by the same steps i.e., parameters estimation and model verification. This whole process is repeated until an adequate model is finally selected. In the last step, the final model can be utilized for forecasting aims [20]. Mathematically, the ARIMA model can be expressed as:
ϑ(U)∇d(Mt−π)=φ(U)βt, |
where βt and Mt represent the error term and observed value at time t, respectively. ϑ(U)=1−∑pu=1ϑuUu and φ(U)=1−∑qv=1φvUv are polynomials in U of degree p and q, ϑu(1,2,...,p) and φv(1,2,...,q) are the unknown parameters of the model. ∇=(1−U), where U is the backward shift operator, d denotes the length of difference, p and q indicate the length of AR and MA terms, respectively.
Qurban et al. [21] declared that nonlinear problems are commonly overcome by machine learning (ML) techniques. The most widely used ML techniques are Artificial neural networks (ANNs) and the group method of data handling (GMDH).
The ANN models can model a wide range of non-linear problems. In comparison with other nonlinear models, ANN can approximate a huge group of nonlinear functions with good accuracy. This accuracy is achieved due to the parallel processing of the information from the data. The construction of the ANN model does not require any prior assumption regarding model form. Rather, the model is substantially ascertained by the characteristics of the data [22]. There are many types of ANN, but multilayer perceptron (MLP), is one of the most popular forms of ANN for forecasting in time series setup. The nexus between input and output layers has the following mathematical representation
Mt=φ0+q∑v=1φvρ(φ0u+p∑u=1φuvMt−i+βt), |
where φuv(u=1,2,...,p,v=1,2,...,q) and φv(v=0,1,2,...,q) represent connection weights, p denotes input layers and q denotes the number of hidden layers. There is no hard and fast rule to select the input layers and hidden layers. The sole possible way is the error and trial approach to select the optimum number of p and q; see Khashei and Hajirahimi [23].
The GMDH-type neural network can be illustrated as a collection of neurons where numerous pairs are related throughout every layer utilizing quadratic polynomial, which in turn induces new neurons all over the next layers. Such type of information can be utilized while connecting the input layers with the output layer. If the primary structure is tentative then it leads to a slight improvement in parameters estimation of a model. Primarily, the GMDH tries to achieve the hierarchic solution, by seeking a large set of simple models, keeping the best, and developing them iteratively to get a composition of functions like a statistical model [24]. The polynomial nodes or building blocks have often the quadratic form
M=φ0+φ1u1+φ2u2++φ3u21++φ4u22++φ5u1u2, |
M is the output of the model, ui(i=1,2) shows the input variables and the weight vector φ.
The predictive power of the statistical models is assessed by using metrics computed from a holdout set (test data). From a statistical point of view, the forecast error is a more plausible criterion to judge the forecasting ability and choose the best method. The commonly used criteria such as root-mean-square error (RMSE), and mean absolute error (MAE) (Diebold and Mariano [25]) test are employed in this study.
The RMSE measures the magnitude of error in forecast comparison, and it is a quadratic scaled measure. The RMSE gives relatively more weight to large errors. A low value of RMSE for the model is preferred for a good forecast. It is given as
RMSE=√1HH∑t=1(Yt−^Yt)2. |
The mean absolute error (MAE) is another widely implemented measure of forecasting accuracy. It is defined as
MAE=|1HH∑t=1(Yt−^Yt)2|. |
In the RMSE and MAPE formulas, Yt and ^Yt indicate the actual and forecast values respectively, and H shows the forecast horizon.
When using different models for the prediction of a single variable, then it is better to check which model outperforms in terms of prediction. For this purpose, Diebold and Mariano (DM) test can be used. The DM statistic is a squared error difference mean of two competing predictive models, whose covariance matrix is obtained by accounting for the autocorrelation produced in the multistep forecasts. The DM test statistic tests the null hypotheses that two models have the same forecasting ability, i.e., to test forecast errors of two sets, say e1i and e2i having equal mean. A loss function is defined to evaluate the forecast error, mainly squared forecast error and absolute forecast error are used. The corresponding test statistics are used for hypothesis testing, that the two models have equal predictive performance, which is given by
DM=ˉD√Var(ˉD), |
where ˉD=1p∑pk=1Dk, the ˉD has an asymptotic variance is expressed as
Var(ˉD)=1p[ω0+2p−1∑k=1ωk], |
where ω0 is the variance of Dk and ωk is the kth autocovariance of Dk and p is the number of forecast steps, which is estimated by
^ωl=1pp∑k=i+1(Dk−ˉD)(Dk−i−ˉD). |
In this section, we elaborate on the findings of our prediction experiments along with a graphical representation. Figure 5 indicates the non-stationarity pattern in the series. In other words, the statistical properties such as mean, variance, and covariance of the original series are not constant over time. It represents a trend in the data, but we require a plain series having no trend. To achieve stationary series, we performed a differenced transformation. The ACF and PACF of the original and differenced series are given in Figure 6. It can be observed that the ACF associated with the original series is gradually dropping, but after transformation, the ACF declines at a swift pace. This shows that the COVID-19 series is the first difference stationary.
The output of the Box-Ljung test and Jarqua-Bera test are reported in Table 6. The corresponding p-values are greater than 5 percent, thus we cannot reject the null hypothesis, under which it is assumed that the residuals of the estimated model are random and normally distributed. In other words, it is stated that the residuals of the estimated model are normally distributed and uncorrelated. Thus, the model can be used for forecasting.
Test | χ2 | p-value |
Box-Ljung test | 23.697 | 0.10 |
JB test | 1.932 | 0.38 |
Another way to know the randomness and normality of the residuals of the fitted model is by considering the graphs of ACF, PACF, and QQ-plot of the residuals; see Figure 7. They reveal that the residuals are random and normally distributed.
The RMSE and MAE using the COVID-19 dataset are given in Table 7. We can observe that RMSE and MAE associated with machine learning (ML) algorithms such as ANN and GMDH are considerably lower than the time series model. Thus, it can be inferred that machine learning algorithms are superior to traditional time series models in terms of forecasting. Moreover, considering ML algorithms, the GMDH has produced a more accurate forecast than ANN. The forecast comparison is also portrayed in Figure 8. The plots in Figure 8 reveal that the ML algorithms, particularly GMDH, have captured the COVID-19 trend very well. Basically, these error metrics are loss functions, and in the field of statistics and econometrics, we are often interested in statistical differences. Therefore, apply the DM test for this purpose.
Criteria | ARIMA | ANN | GMDH |
RMSE | 0.359 | 0.274 | 0.139 |
MAE | 0.320 | 0.214 | 0.130 |
The values in Table 8 indicate the p-values for the DM test. Under the null hypothesis, it is assumed that models in row and column have the same forecasting accuracy. Under the rival hypothesis, the model in the column is more accurate than the row model. We found another piece of evidence in the form of a statistical test. From Table 8, it is quite clear that p-values are sufficiently small, which clearly demonstrates that the ML algorithms have high predictive power than ARIMA model.
Models | ARIMA | ANN | GMDH |
ARIMA | - | 0.001 | 0.001 |
ANN | - | - | 0.001 |
GMDH | - | - | - |
The COVID-19 pandemic has dramatically affected the economy, education, and health sectors, etc. Among them, the health sector is the most affected one. To have the best description and knowledge of the COVID-19 pandemic, numerous statistical studies have appeared. This paper offers a new statistical model for analyzing the mortality rate of the COVID-19 pandemic in Mexico. Some characterizations along with estimators of the new model were obtained. The new model was named the Z-FWE distribution and applied to the COVID-19 data in comparison with other statistical models. Based on the findings of this research, it is shown that the Z-FWE model was the best competitor for dealing with mortality rate data. Later, we compared the predictive power of ML techniques, i.e., ANN and GMDH, with the ARIMA. For comparison, we used RMSE, MAE, and DM tests. Based on all these criteria, it is inferred that ML techniques are superior in terms of forecasting than the ARIMA model. Furthermore, GMDH outperformed the ANN while getting small forecast errors. The study recommends that policymakers can utilize ML techniques, particularly GMDH, for forecasting the mortality rate of COVID-19 patients.
This research work was funded by Institutional Fund Projects under grant no. (IFPIP: 549-150-1443). The authors gratefully acknowledge technical and financial support provided by the Ministry of Education and King Abdulaziz University, DSR, Jeddah, Saudi Arabia.
All authors declare no conflicts of interest in this paper.
[1] | M. Gupta, A. Abdelmaksoud, M. Jafferany, T. Lotti, R. Sadoughifar, M. Goldust, COVID-19 and economy, Dermatologic Ther., 33 (2020), e13329. https://doi.org/10.1111/dth.13329 |
[2] |
S. Rashid, S. S. Yadav, Impact of COVID-19 pandemic on higher education and research, Indian J. Hum. Dev., 14 (2020), 340–343. https://doi.org/10.1177/0973703020946700 doi: 10.1177/0973703020946700
![]() |
[3] |
K. S. Khan, M. A. Mamun, M. D. Griffiths, I. Ullah, The mental health impact of the COVID-19 pandemic across different cohorts, Int. J. Mental Health Addict., 20 (2022), 380–386. https://doi.org/10.1007/s11469-020-00367-0 doi: 10.1007/s11469-020-00367-0
![]() |
[4] |
P. Seetharaman, Business models shifts: Impact of COVID-19, Int. J. Inf. Manage., 54 (2020), 102173. https://doi.org/10.1016/j.ijinfomgt.2020.102173 doi: 10.1016/j.ijinfomgt.2020.102173
![]() |
[5] |
H. Wardle, C. Donnachie, N. Critchlow, A. Brown, C. Bunn, F. Dobbie, et al., The impact of the initial COVID-19 lockdown upon regular sports bettors in Britain: Findings from a cross-sectional online study, Addict. Behav., 118 (2021), 106876. https://doi.org/10.1016/j.addbeh.2021.106876 doi: 10.1016/j.addbeh.2021.106876
![]() |
[6] |
S. Jaipuria, R. Parida, P. Ray, The impact of COVID-19 on tourism sector in India, Tourism Recreation Res., 46 (2021), 245–260. https://doi.org/10.1080/02508281.2020.1846971 doi: 10.1080/02508281.2020.1846971
![]() |
[7] | M. Bebbington, C. D. Lai, R. Zitikis, A flexible Weibull extension, iReliab. Eng. Syst. Saf., 92 (2007), 719–726. https://doi.org/10.1016/j.ress.2006.03.004 |
[8] | A. El-Gohary, A. H. El-Bassiouny, M. El-Morshedy, Exponentiated flexible Weibull extension distribution, Int. J. Math. Appl., 3 (2015), 1–12. Available from: http://ijmaa.in/index.php/ijmaa/article/view/440. |
[9] | A. El-Gohary, A. H. El-Bassiouny, M. El-Morshedy, Inverse flexible Weibull extension distribution. Int. J. Comput. Appl., 115 (2015), 46–51. https://doi.org/10.5120/20127-2211 |
[10] | M. A. El-Damcese, A. Mustafa, B. S. El-Desouky, M. E. Mustafa, The Kumaraswamy flexible Weibull extension, Int. J. Math. Appl., 4 (2016), 1–14. Available from: http://ijmaa.in/index.php/ijmaa/article/view/540. |
[11] | Z. Ahmad, E. Mahmoudi, O. Kharazmi, On modeling the earthquake insurance data via a new member of the TX family, Comput. Intell. Neurosci., 2020 (2020). https://doi.org/10.1155/2020/7631495 |
[12] |
E. Seneta, Karamata's characterization theorem, feller and regular variation in probability theory, Publ. Inst. Math., 71 (2002), 79–89. https://doi.org/10.2298/PIM0271079S doi: 10.2298/PIM0271079S
![]() |
[13] | W. Glänzel, A characterization theorem based on truncated moments and its application to some distribution families, in Mathematical Statistics and Probability Theory, (1987), 75–84. https://doi.org/10.1007/978-94-009-3965-3_8 |
[14] |
W. Glänzel, Some consequences of a characterization theorem based on truncated moments, Statistics, 21 (1990), 613–618. https://doi.org/10.1080/02331889008802273 doi: 10.1080/02331889008802273
![]() |
[15] | G. G. Hamedani, On certain generalized gamma convolution distributions II, Tech. Rep., (2013), 484. |
[16] |
H. M. Almongy, E. M. Almetwally, H. M. Aljohani, A. S. Alghamdi, E. H. Hafez, A new extended Rayleigh distribution with applications of COVID-19 data, Results Phys., 23 (2021), 104012. https://doi.org/10.1016/j.rinp.2021.104012 doi: 10.1016/j.rinp.2021.104012
![]() |
[17] |
M. Qi, G. P. Zhang, An investigation of model selection criteria for neural network time series forecasting, Eur. J. Oper. Res., 132 (2001), 666–680. https://doi.org/10.1016/S0377-2217(00)00171-5 doi: 10.1016/S0377-2217(00)00171-5
![]() |
[18] |
M. Khashei, M. Bijari, An artificial neural network (p, d, q) model for timeseries forecasting, Expert Syst. Appl., 37 (2010), 479–489. https://doi.org/10.1016/j.eswa.2009.05.044 doi: 10.1016/j.eswa.2009.05.044
![]() |
[19] |
V. Ş. Ediger, S. Akar, ARIMA forecasting of primary energy demand by fuel in Turkey, Energy Policy, 35 (2007), 1701–1708. https://doi.org/10.1016/j.enpol.2006.05.009 doi: 10.1016/j.enpol.2006.05.009
![]() |
[20] | M. Khashei, M. Bijari, Which methodology is better for combining linear and non-linear models for time series forecasting? Int. J. Ind. Syst. Eng., 4 (2011), 265–285. Available from: file:///C:/Users/97380/Downloads/111420120405-1.pdf. |
[21] | M. Qurban, X. Zhang, H. M. Nazir, I. Hussain, M. Faisal, E. E. Elashkar, et al., Development of hybrid methods for prediction of principal mineral resources, Math. Probl. Eng., 2021 (2021). https://doi.org/10.1155/2021/6362660 |
[22] |
G. P. Zhang, Time series forecasting using a hybrid ARIMA and neural network model, Neurocomputing, 50 (2003), 159–175. https://doi.org/10.1016/S0925-2312(01)00702-0 doi: 10.1016/S0925-2312(01)00702-0
![]() |
[23] |
M. Khashei, Z. Hajirahimi, A comparative study of series arima/mlp hybrid models for stock price forecasting, Commun. Stat.- Simul. Comput., 48 (2019), 2625–2640. https://doi.org/10.1080/03610918.2018.1458138 doi: 10.1080/03610918.2018.1458138
![]() |
[24] |
P. Ravisankar, V. Ravi, Financial distress prediction in banks using Group Method of Data Handling neural network, counter propagation neural network and fuzzy ARTMAP, Knowledge Based Syst., 23 (2010), 823–831. https://doi.org/10.1016/j.knosys.2010.05.007 doi: 10.1016/j.knosys.2010.05.007
![]() |
[25] |
F. X. Diebold, R. S. Mariano, Comparing predictive accuracy, J. Bus. Econ. Stat., 13 (1995), 253–263. https://doi.org/10.1080/07350015.1995.10524599 doi: 10.1080/07350015.1995.10524599
![]() |
1. | Chaoyu Li, Bin Lin, Zhijie Zhou, Lingming Meng, Jian Yu, A new probabilistic model: Its implementations to the reliability products and art tools, 2024, 109, 11100168, 347, 10.1016/j.aej.2024.08.099 | |
2. | Hasnain Iftikhar, Murad Khan, Mohammed Saad Khan, Mehak Khan, Short-Term Forecasting of Monkeypox Cases Using a Novel Filtering and Combining Technique, 2023, 13, 2075-4418, 1923, 10.3390/diagnostics13111923 | |
3. | Sanaa Al-Marzouki, Afaf Alrashidi, Christophe Chesneau, Mohammed Elgarhy, Rana H. Khashab, Suleman Nasiru, On improved fitting using a new probability distribution and artificial neural network: Application, 2023, 13, 2158-3226, 10.1063/5.0176715 | |
4. | Li Jiang, Jin-Taek Seong, Marwan H. Alhelali, Basim S.O. Alsaedi, Fatimah M. Alghamdi, Ramy Aldallal, A new cosine-based approach for modelling the time-to-event phenomena in sports and engineering sectors, 2024, 98, 11100168, 19, 10.1016/j.aej.2024.04.037 | |
5. | Ahmed M. Gemeay, Kadir Karakaya, M. E. Bakr, Oluwafemi Samson Balogun, Mintodê Nicodème Atchadé, Eslam Hussam, Power Lambert uniform distribution: Statistical properties, actuarial measures, regression analysis, and applications, 2023, 13, 2158-3226, 10.1063/5.0170964 | |
6. | Mohamed F. Hussein, Sarah A. Ibrahim, Suzan Abdel-Rahman, Abdelhamid Elshabrawy, Haqema A. A. Nasr, Saja Yazbek, Abdul Jabbar, Cinaria T. Albadri, Mariam Alsanafi, Narjiss Aji, Naglaa Youssef, Hammad M. Hammad, Fatimah S. A. Abdullah, Ehab Elrewany, Mohamed M. Tahoun, Mahmoud Tolba, Mohamed K. Abo Salama, Ramy M. Ghazy, Psychological antecedents of vaccine inequity: keys to improve the rates of vaccination, 2024, 99, 2090-262X, 10.1186/s42506-024-00175-7 | |
7. | Tianqing Lin, Liqun Shen, Najla M. Aloraini, Alia A. Alkhathami, Huda M. Alshanbari, Hamiden Abd El-Wahed Khalifa, A new asymmetrical probability model: Its empirical exploration using the price quotations of the ceramic products, 2025, 117, 11100168, 66, 10.1016/j.aej.2025.01.003 | |
8. | Dongmei Wang, Jie Wang, A new statistical framework: Exploring its identifiability characteristics and applications within music therapy and engineering, 2025, 117, 11100168, 53, 10.1016/j.aej.2025.01.001 | |
9. | Xianqing Hu, Amit Yadav, Asif Khan, Abhishek Pratap Sah, Sami Azam, 2025, Construction of PCOS Prediction Model Based on BP Neural Network, 979-8-3315-2266-7, 885, 10.1109/ICMCSI64620.2025.10883524 | |
10. | Cui Tianmeng, Xintao Ma, Dongmei Wang, Omalsad Hamood Odhah, Mohammed A. Alshahrani, On the implications of a new statistical model and machine learning algorithms in music engineering, 2025, 122, 11100168, 496, 10.1016/j.aej.2025.03.008 | |
11. | Hatem Semary, Ahmad Abubakar Suleiman, Aliyu Ismail Ishaq, Jamilu Yunusa Falgore, Umar Kabir Abdullahi, Hanita Daud, Mohamed A. Abd Elgawad, Mohammad Elgarhy, A new modified Sine-Weibull distribution for modeling medical data with dynamic structures, 2025, 18, 16878507, 101427, 10.1016/j.jrras.2025.101427 | |
12. | Zubir Shah, Ehab M. Almetwally, Dost Muhammad Khan, Farrukh Jamal, A novel odd Type-X family of distributions: Model, theory, and applications to medical, insurance, and engineering data sets, 2025, 18, 16878507, 101451, 10.1016/j.jrras.2025.101451 | |
13. | Muteb Faraj Alharthi, Samirah Alzubaidi, A novel discrete statistical model with applications on medical and health real data, 2025, 125, 11100168, 42, 10.1016/j.aej.2025.04.026 |
Parameters values | Measures | Measures values | Results | |||||||||
τ1=0.0001 | τ2=0.00001 | β=0.37 | y ⟶ | 10−8 | 10−6 | 0.5 | 1.5 | 15 | 50 | 10000 | 100000 | B PDF |
L ⟶ | 1.13007×10422 | 1.03080×10−8 | -0.000046 | -0.00014 | 0.00083 | 0.2729 | -2.8949×1010 | -1.9409×1019148 | ||||
τ1=0.001 | τ2=0.01 | β=0.75 | y ⟶ | 10−8 | 10−6 | 0.5 | 1.5 | 15 | 50 | 10000 | 20000 | B PDF |
L ⟶ | 2.1606×10434290 | 6.27199×104338 | -0.0645362 | -0.197144 | -1.96613 | 0.622783 | -1.8573×1019146 | -3.47908×10421408943 | ||||
τ1=0.1 | τ2=0.25 | β=1.5 | y ⟶ | 10−8 | 10−6 | 0.5 | 1.5 | 15 | 50 | 80 | 100 | U PDF |
L ⟶ | 9.8012×1010857360 | 3.6658×10108572 | -1.7303 | -7.8697 | -1.23457×107 | -1.7075×10135 | -1.71954×102590 | -3.2969×1019094 | ||||
τ1=2.2 | τ2=0.0001 | β=0.37 | y ⟶ | 10−8 | 10−6 | 10−4 | 0.01 | 0.1 | 0.2 | 5 | 10 | B PDF |
L ⟶ | 5.0609×104332 | 1.51416×1033 | -5.34296×10−9 | -9.302293×10−6 | 0.00239836 | 0.0251161 | -1.72409×1052013 | -3.94217×103113784610 | ||||
τ1=2.4 | τ2=5.5 | β=1.1 | y ⟶ | 10−8 | 10−6 | 10−4 | 0.01 | 0.1 | 0.2 | 5 | 10 | U PDF |
L ⟶ | 3.6902×10238861966 | 1.48159×102388621 | 5.2089×1023887 | 2.40261×10240 | 2.47911×1025 | 2.79741×1013 | -6.8501×1047065 | -8.05508×1013274553069 | ||||
τ1=2.4 | τ2=2.5 | β=1.1 | y ⟶ | 10−8 | 10−6 | 10−4 | 0.01 | 0.1 | 0.2 | 5 | 10 | U PDF |
L ⟶ | 2.04752×10108573621 | 1.0969×101085737 | 1.5755×1010858 | 2.54468×10109 | 4.62999×1011 | 1.68683×106 | -1.7082×1085752 | -5.22575×1017918772372 | ||||
τ1=1.5 | τ2=1.5 | β=1.0 | y ⟶ | 10−8 | 10−6 | 10−4 | 0.01 | 0.1 | 3 | 10 | 15 | U PDF |
L ⟶ | 4.341804×1065144172 | 1.1886×10651442 | 5.8796×106514 | 3.09466×1065 | 6.52243×106 | -5.27417×1051 | -7.1416×101169 | -1.78388×104645267231 |
p | Criteria | MLE | LSE | WLSE | MPSE | ADE |
τ1=0.8,τ2=1.3,β=0.9 | ||||||
20 | |Bias| | 0.12745895 | 0.12984254 | 0.13145896 | 0.16369694 | 0.14369857 |
MSE | 0.01369548 | 0.01699527 | 0.01936955 | 0.03265855 | 0.02968856 | |
50 | |Bias| | 0.07023698 | 0.08593632 | 0.09745269 | 0.11236985 | 0.07452996 |
MSE | 0.00696585 | 0.00742696 | 0.00892024 | 0.01496657 | 0.00639957 | |
150 | |Bias| | 0.04025955 | 0.04128963 | 0.04593582 | 0.05985352 | 0.04236958 |
MSE | 0.00230288 | 0.00285295 | 0.00369021 | 0.00597102 | 0.00283015 | |
300 | |Bias| | 0.02336951 | 0.02478037 | 0.03352865 | 0.03259560 | 0.03252014 |
MSE | 0.00112023 | 0.00141260 | 0.00123695 | 0.00289635 | 0.00147848 | |
τ1=0.12,τ2=0.8,β=1.2 | ||||||
20 | |Bias| | 0.14789622 | 0.15236974 | 0.14890395 | 0.18930325 | 0.17485630 |
MSE | 0.02023985 | 0.03636920 | 0.03630215 | 0.05014790 | 0.04012856 | |
50 | |Bias| | 0.09639519 | 0.10303255 | 0.10920203 | 0.12306954 | 0.11425036 |
MSE | 0.01021589 | 0.01636985 | 0.01547496 | 0.02036991 | 0.01636982 | |
150 | |Bias| | 0.04852036 | 0.06696350 | 0.06745622 | 0.07412696 | 0.07012650 |
MSE | 0.00523694 | 0.00530236 | 0.00595621 | 0.00669521 | 0.00610236 | |
300 | |Bias| | 0.03203695 | 0.03365541 | 0.04012556 | 0.05120356 | 0.03746222 |
MSE | 0.00210236 | 0.00242369 | 0.00345890 | 0.00463697 | 0.00323405 | |
τ1=1.3,τ2=1.5,β=0.7 | ||||||
20 | |Bias| | 0.66256985 | 0.67458852 | 0.68520136 | 0.73265996 | 0.69203214 |
MSE | 0.25369414 | 0.25896894 | 0.28957748 | 0.29652120 | 0.26358544 | |
50 | |Bias| | 0.31258967 | 0.32369841 | 0.33695252 | 0.38569520 | 0.33737712 |
MSE | 0.11482069 | 0.12987039 | 0.13698549 | 0.14529602 | 0.12369501 | |
150 | |Bias| | 0.18595452 | 0.19395225 | 0.20369521 | 0.24473699 | 0.21032369 |
MSE | 0.03636654 | 0.03852178 | 0.03715985 | 0.04236985 | 0.03920369 | |
300 | |Bias| | 0.11532684 | 0.12399596 | 0.10158697 | 0.15639023 | 0.12699529 |
MSE | 0.00936998 | 0.00985632 | 0.01058554 | 0.01239857 | 0.01158859 |
Model | ^τ1 | ^τ2 | ˆβ | ^θ1 | ^θ2 | ˆθ | ˆλ |
Z-FWE | 0.60578 (0.06790) |
1.30376 (0.20545) |
1.52213 (0.68089) |
- | - | - | - |
FWE | 0.64201 (0.05039) |
1.11759 (0.10961) |
- | - | - | - | - |
E-FWE | 0.65089 (0.11588) |
1.35112 (2.14650) |
- | 0.82677 (1.39368) |
- | - | - |
Weibull | 1.92159 (0.14090) |
0.58694 (0.07121) |
- | - | - | - | - |
E-Weibull | 1.00398 (0.32020) |
1.78865 (0.75560) |
- | 4.02508 (3.10070) |
- | - | - |
K-Weibull | 1.44294 (0.14370) |
3.76192 (NaN) |
- | 3.13605 (1.63131) |
0.24665 (NaN) |
- | - |
NMFWE | 0.61568 (0.06012) |
1.33469 (0.20807) |
- | - | - | - | 2.86140 (1.76820) |
MNFWE | 1.45714 (0.17835) |
1.52803 (0.90054) |
- | - | - | 4.11707 (1.05217) |
0.038181 (0.00983) |
Model | AIC | CAIC | BIC | HQIC |
Z-FWE | 186.07860 | 186.31390 | 194.06900 | 189.31720 |
FWE | 189.04580 | 189.16230 | 196.37270 | 191.20480 |
E-FWE | 187.01970 | 187.25500 | 195.01000 | 190.25820 |
Weibull | 191.38590 | 191.50240 | 196.71280 | 193.54490 |
E-Weibull | 188.2469 0 | 188.48220 | 196.23720 | 191.48540 |
K-Weibull | 189.18680 | 189.58290 | 199.84060 | 193.50490 |
NMFWE | 186.12600 | 186.36130 | 194.11630 | 189.36450 |
MNFWE | 214.87380 | 215.26980 | 225.52750 | 219.19180 |
Model | CM | AD | KS | p-value |
Z-FWE | 0.03228 | 0.20038 | 0.04683 | 0.97420 |
FWE | 0.03963 | 0.26343 | 0.05313 | 0.92580 |
E-FWE | 0.03866 | 0.25671 | 0.05589 | 0.89500 |
Weibull | 0.10233 | 0.65790 | 0.06967 | 0.68220 |
E-Weibull | 0.05380 | 0.29853 | 0.06758 | 0.71820 |
NMFWE | 0.03276 | 0.20485 | 0.05085 | 0.94680 |
MNFWE | 0.26322 | 1.70311 | 0.09473 | 0.29740 |
Test | χ2 | p-value |
Box-Ljung test | 23.697 | 0.10 |
JB test | 1.932 | 0.38 |
Criteria | ARIMA | ANN | GMDH |
RMSE | 0.359 | 0.274 | 0.139 |
MAE | 0.320 | 0.214 | 0.130 |
Models | ARIMA | ANN | GMDH |
ARIMA | - | 0.001 | 0.001 |
ANN | - | - | 0.001 |
GMDH | - | - | - |
Parameters values | Measures | Measures values | Results | |||||||||
τ1=0.0001 | τ2=0.00001 | β=0.37 | y ⟶ | 10−8 | 10−6 | 0.5 | 1.5 | 15 | 50 | 10000 | 100000 | B PDF |
L ⟶ | 1.13007×10422 | 1.03080×10−8 | -0.000046 | -0.00014 | 0.00083 | 0.2729 | -2.8949×1010 | -1.9409×1019148 | ||||
τ1=0.001 | τ2=0.01 | β=0.75 | y ⟶ | 10−8 | 10−6 | 0.5 | 1.5 | 15 | 50 | 10000 | 20000 | B PDF |
L ⟶ | 2.1606×10434290 | 6.27199×104338 | -0.0645362 | -0.197144 | -1.96613 | 0.622783 | -1.8573×1019146 | -3.47908×10421408943 | ||||
τ1=0.1 | τ2=0.25 | β=1.5 | y ⟶ | 10−8 | 10−6 | 0.5 | 1.5 | 15 | 50 | 80 | 100 | U PDF |
L ⟶ | 9.8012×1010857360 | 3.6658×10108572 | -1.7303 | -7.8697 | -1.23457×107 | -1.7075×10135 | -1.71954×102590 | -3.2969×1019094 | ||||
τ1=2.2 | τ2=0.0001 | β=0.37 | y ⟶ | 10−8 | 10−6 | 10−4 | 0.01 | 0.1 | 0.2 | 5 | 10 | B PDF |
L ⟶ | 5.0609×104332 | 1.51416×1033 | -5.34296×10−9 | -9.302293×10−6 | 0.00239836 | 0.0251161 | -1.72409×1052013 | -3.94217×103113784610 | ||||
τ1=2.4 | τ2=5.5 | β=1.1 | y ⟶ | 10−8 | 10−6 | 10−4 | 0.01 | 0.1 | 0.2 | 5 | 10 | U PDF |
L ⟶ | 3.6902×10238861966 | 1.48159×102388621 | 5.2089×1023887 | 2.40261×10240 | 2.47911×1025 | 2.79741×1013 | -6.8501×1047065 | -8.05508×1013274553069 | ||||
τ1=2.4 | τ2=2.5 | β=1.1 | y ⟶ | 10−8 | 10−6 | 10−4 | 0.01 | 0.1 | 0.2 | 5 | 10 | U PDF |
L ⟶ | 2.04752×10108573621 | 1.0969×101085737 | 1.5755×1010858 | 2.54468×10109 | 4.62999×1011 | 1.68683×106 | -1.7082×1085752 | -5.22575×1017918772372 | ||||
τ1=1.5 | τ2=1.5 | β=1.0 | y ⟶ | 10−8 | 10−6 | 10−4 | 0.01 | 0.1 | 3 | 10 | 15 | U PDF |
L ⟶ | 4.341804×1065144172 | 1.1886×10651442 | 5.8796×106514 | 3.09466×1065 | 6.52243×106 | -5.27417×1051 | -7.1416×101169 | -1.78388×104645267231 |
p | Criteria | MLE | LSE | WLSE | MPSE | ADE |
τ1=0.8,τ2=1.3,β=0.9 | ||||||
20 | |Bias| | 0.12745895 | 0.12984254 | 0.13145896 | 0.16369694 | 0.14369857 |
MSE | 0.01369548 | 0.01699527 | 0.01936955 | 0.03265855 | 0.02968856 | |
50 | |Bias| | 0.07023698 | 0.08593632 | 0.09745269 | 0.11236985 | 0.07452996 |
MSE | 0.00696585 | 0.00742696 | 0.00892024 | 0.01496657 | 0.00639957 | |
150 | |Bias| | 0.04025955 | 0.04128963 | 0.04593582 | 0.05985352 | 0.04236958 |
MSE | 0.00230288 | 0.00285295 | 0.00369021 | 0.00597102 | 0.00283015 | |
300 | |Bias| | 0.02336951 | 0.02478037 | 0.03352865 | 0.03259560 | 0.03252014 |
MSE | 0.00112023 | 0.00141260 | 0.00123695 | 0.00289635 | 0.00147848 | |
τ1=0.12,τ2=0.8,β=1.2 | ||||||
20 | |Bias| | 0.14789622 | 0.15236974 | 0.14890395 | 0.18930325 | 0.17485630 |
MSE | 0.02023985 | 0.03636920 | 0.03630215 | 0.05014790 | 0.04012856 | |
50 | |Bias| | 0.09639519 | 0.10303255 | 0.10920203 | 0.12306954 | 0.11425036 |
MSE | 0.01021589 | 0.01636985 | 0.01547496 | 0.02036991 | 0.01636982 | |
150 | |Bias| | 0.04852036 | 0.06696350 | 0.06745622 | 0.07412696 | 0.07012650 |
MSE | 0.00523694 | 0.00530236 | 0.00595621 | 0.00669521 | 0.00610236 | |
300 | |Bias| | 0.03203695 | 0.03365541 | 0.04012556 | 0.05120356 | 0.03746222 |
MSE | 0.00210236 | 0.00242369 | 0.00345890 | 0.00463697 | 0.00323405 | |
τ1=1.3,τ2=1.5,β=0.7 | ||||||
20 | |Bias| | 0.66256985 | 0.67458852 | 0.68520136 | 0.73265996 | 0.69203214 |
MSE | 0.25369414 | 0.25896894 | 0.28957748 | 0.29652120 | 0.26358544 | |
50 | |Bias| | 0.31258967 | 0.32369841 | 0.33695252 | 0.38569520 | 0.33737712 |
MSE | 0.11482069 | 0.12987039 | 0.13698549 | 0.14529602 | 0.12369501 | |
150 | |Bias| | 0.18595452 | 0.19395225 | 0.20369521 | 0.24473699 | 0.21032369 |
MSE | 0.03636654 | 0.03852178 | 0.03715985 | 0.04236985 | 0.03920369 | |
300 | |Bias| | 0.11532684 | 0.12399596 | 0.10158697 | 0.15639023 | 0.12699529 |
MSE | 0.00936998 | 0.00985632 | 0.01058554 | 0.01239857 | 0.01158859 |
Model | ^τ1 | ^τ2 | ˆβ | ^θ1 | ^θ2 | ˆθ | ˆλ |
Z-FWE | 0.60578 (0.06790) |
1.30376 (0.20545) |
1.52213 (0.68089) |
- | - | - | - |
FWE | 0.64201 (0.05039) |
1.11759 (0.10961) |
- | - | - | - | - |
E-FWE | 0.65089 (0.11588) |
1.35112 (2.14650) |
- | 0.82677 (1.39368) |
- | - | - |
Weibull | 1.92159 (0.14090) |
0.58694 (0.07121) |
- | - | - | - | - |
E-Weibull | 1.00398 (0.32020) |
1.78865 (0.75560) |
- | 4.02508 (3.10070) |
- | - | - |
K-Weibull | 1.44294 (0.14370) |
3.76192 (NaN) |
- | 3.13605 (1.63131) |
0.24665 (NaN) |
- | - |
NMFWE | 0.61568 (0.06012) |
1.33469 (0.20807) |
- | - | - | - | 2.86140 (1.76820) |
MNFWE | 1.45714 (0.17835) |
1.52803 (0.90054) |
- | - | - | 4.11707 (1.05217) |
0.038181 (0.00983) |
Model | AIC | CAIC | BIC | HQIC |
Z-FWE | 186.07860 | 186.31390 | 194.06900 | 189.31720 |
FWE | 189.04580 | 189.16230 | 196.37270 | 191.20480 |
E-FWE | 187.01970 | 187.25500 | 195.01000 | 190.25820 |
Weibull | 191.38590 | 191.50240 | 196.71280 | 193.54490 |
E-Weibull | 188.2469 0 | 188.48220 | 196.23720 | 191.48540 |
K-Weibull | 189.18680 | 189.58290 | 199.84060 | 193.50490 |
NMFWE | 186.12600 | 186.36130 | 194.11630 | 189.36450 |
MNFWE | 214.87380 | 215.26980 | 225.52750 | 219.19180 |
Model | CM | AD | KS | p-value |
Z-FWE | 0.03228 | 0.20038 | 0.04683 | 0.97420 |
FWE | 0.03963 | 0.26343 | 0.05313 | 0.92580 |
E-FWE | 0.03866 | 0.25671 | 0.05589 | 0.89500 |
Weibull | 0.10233 | 0.65790 | 0.06967 | 0.68220 |
E-Weibull | 0.05380 | 0.29853 | 0.06758 | 0.71820 |
NMFWE | 0.03276 | 0.20485 | 0.05085 | 0.94680 |
MNFWE | 0.26322 | 1.70311 | 0.09473 | 0.29740 |
Test | χ2 | p-value |
Box-Ljung test | 23.697 | 0.10 |
JB test | 1.932 | 0.38 |
Criteria | ARIMA | ANN | GMDH |
RMSE | 0.359 | 0.274 | 0.139 |
MAE | 0.320 | 0.214 | 0.130 |
Models | ARIMA | ANN | GMDH |
ARIMA | - | 0.001 | 0.001 |
ANN | - | - | 0.001 |
GMDH | - | - | - |