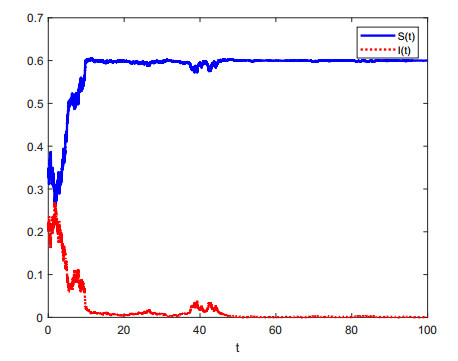
In this paper, a stochastic epidemic model with logistic growth is discussed. Based on stochastic differential equation theory, stochastic control method, etc., the properties of the solution of the model nearby the epidemic equilibrium of the original deterministic system are investigated, the sufficient conditions to ensure the stability of the disease-free equilibrium of the model are established, and two event-triggered controllers to drive the disease from endemic to extinction are constructed. The related results show that the disease becomes endemic when the transmission coefficient exceeds a certain threshold. Furthermore, when the disease is endemic, we can drive the disease from endemic to extinction by choosing suitable event-triggering gains and control gains. Finally, the effectiveness of the results is illustrated by a numerical example.
Citation: Tingting Cai, Yuqian Wang, Liang Wang, Zongying Tang, Jun Zhou. Analysis and event-triggered control for a stochastic epidemic model with logistic growth[J]. Mathematical Biosciences and Engineering, 2023, 20(2): 2243-2260. doi: 10.3934/mbe.2023105
[1] | Dong Xu, Xinling Li, Weipeng Tai, Jianping Zhou . Event-triggered stabilization for networked control systems under random occurring deception attacks. Mathematical Biosciences and Engineering, 2023, 20(1): 859-878. doi: 10.3934/mbe.2023039 |
[2] | Duoduo Zhao, Fang Gao, Jinde Cao, Xiaoxin Li, Xiaoqin Ma . Mean-square consensus of a semi-Markov jump multi-agent system based on event-triggered stochastic sampling. Mathematical Biosciences and Engineering, 2023, 20(8): 14241-14259. doi: 10.3934/mbe.2023637 |
[3] | Meici Sun, Qiming Liu . An SIS epidemic model with time delay and stochastic perturbation on heterogeneous networks. Mathematical Biosciences and Engineering, 2021, 18(5): 6790-6805. doi: 10.3934/mbe.2021337 |
[4] | Hui Wang . Prescribed-time control of stochastic high-order nonlinear systems. Mathematical Biosciences and Engineering, 2022, 19(11): 11399-11408. doi: 10.3934/mbe.2022531 |
[5] | Wenjing Wang, Jingjing Dong, Dong Xu, Zhilian Yan, Jianping Zhou . Synchronization control of time-delay neural networks via event-triggered non-fragile cost-guaranteed control. Mathematical Biosciences and Engineering, 2023, 20(1): 52-75. doi: 10.3934/mbe.2023004 |
[6] | Mohsen Dlala, Sharifah Obaid Alrashidi . Rapid exponential stabilization of Lotka-McKendrick's equation via event-triggered impulsive control. Mathematical Biosciences and Engineering, 2021, 18(6): 9121-9131. doi: 10.3934/mbe.2021449 |
[7] | Jinhui Zhang, Jingli Ren, Xinan Zhang . Dynamics of an SLIR model with nonmonotone incidence rate and stochastic perturbation. Mathematical Biosciences and Engineering, 2019, 16(5): 5504-5530. doi: 10.3934/mbe.2019274 |
[8] | Leonid Shaikhet . Stability of a positive equilibrium state for a stochastically perturbed mathematical model ofglassy-winged sharpshooter population. Mathematical Biosciences and Engineering, 2014, 11(5): 1167-1174. doi: 10.3934/mbe.2014.11.1167 |
[9] | Guodong Zhao, Haitao Li, Ting Hou . Survey of semi-tensor product method in robustness analysis on finite systems. Mathematical Biosciences and Engineering, 2023, 20(6): 11464-11481. doi: 10.3934/mbe.2023508 |
[10] | Jiabao Gu, Hui Wang, Wuquan Li . Output-Feedback stabilization for stochastic nonlinear systems with Markovian switching and time-varying powers. Mathematical Biosciences and Engineering, 2022, 19(11): 11071-11085. doi: 10.3934/mbe.2022516 |
In this paper, a stochastic epidemic model with logistic growth is discussed. Based on stochastic differential equation theory, stochastic control method, etc., the properties of the solution of the model nearby the epidemic equilibrium of the original deterministic system are investigated, the sufficient conditions to ensure the stability of the disease-free equilibrium of the model are established, and two event-triggered controllers to drive the disease from endemic to extinction are constructed. The related results show that the disease becomes endemic when the transmission coefficient exceeds a certain threshold. Furthermore, when the disease is endemic, we can drive the disease from endemic to extinction by choosing suitable event-triggering gains and control gains. Finally, the effectiveness of the results is illustrated by a numerical example.
As we know, due to the limitation of medical knowledge, the pathogenesis of many infectious diseases such as influenza A, AIDS, etc. still are not fully understood, which makes it difficult to cure them in a short time. In order to control these diseases, some mathematical models which can reflect the transmission modes of diseases have been established to assist in the formulation of public policies during infectious disease outbreaks [1,2,3,4]. In early research, some ordinary differential equation models were used to characterize the dynamic transmission characteristics of infectious diseases [5,6,7,8].
Owing to the non-negligible effect of noise [9,10], many researchers introduced stochastic perturbations into deterministic models to transform the deterministic problem into a corresponding stochastic problem [11,12,13,14]. Recently, the stochastic epidemic model with logistic growth has attracted extensive attention due to the assumption that logical growth input of the susceptible individuals and infected individuals is more reasonable for a disease with a high death rate [15,16,17,18,19,20]. Although [15,16,17] investigated the stochastic epidemic model with logistic growth, they introduced Gaussian white noise into the deterministic model by perturbing the recruitment rate coefficient and the death rate coefficient of the epidemic model. In fact, for an epidemic model, it is more worth discussing that its transmission coefficient is perturbed [18], since the infection rate of infectious diseases often plays a key role in the persistence or extinction of the disease and more easily fluctuates around some average value due to the continuous environmental stochastic perturbations. The current situation of COVID-19 shows that the rate of infections fluctuates with the temperature. When the temperature goes up, it becomes higher. Although [19,20] introduced Gaussian white noise into the deterministic model by perturbing the transmission coefficient, they have not discussed the properties of the solution of the stochastic epidemic model near the equilibrium of the original deterministic system.
On the other hand, many researchers recently began to pay attention to the systems and control theory toward the epidemic models, which can explore how to drive diseases from epidemic to extinction. Until now, a wide variety of control approaches have been used to mitigate the epidemic spreading processes. For example, sliding mode control [21,22,23], state feedback control [24,25], impulse control [26,27] and optimal control [28,29]. Event-triggered control updates the control inputs only when certain trigger conditions are met, rather than updating the inputs at every time instant, and it is a novel feedback control strategy [30,31,32]. Compared with the traditional control method, the event-triggered control may be more practical, since the latter can reduce the number of control actuation updates. For example, when the number of medical resources or the level of traffic regulations is regarded as one of the control inputs for controlling the epidemic spreading processes, the traditional control strategies force us to update continuously or even per unit of time the control inputs (the number of medical resources or the level of traffic regulations), which is neither economical nor practical because even a small adjustment should require tremendous efforts. A more suitable approach is that the control input (the number of medical resources or the level of traffic regulations) is updated only when some indicators related to the number of infected individuals meet a limitation condition, which is called the event-triggered condition in the event-triggered control [33]. Although the event-triggered control for the epidemic processes is reasonable and useful in practice, relevant results that treat the epidemic as a system and design the event-triggered controller for the epidemic model are rare in the existing literature. References [33] first investigated the event-triggered control problem for a susceptible-infected-susceptible (SIS) model, where the discussed object is a deterministic system. To the best of our knowledge, the event-triggered control for a stochastic epidemic model has not been investigated.
Motivated by the aforementioned discussions, this paper aims to discuss the properties of the solution of a stochastic epidemic model with logistic growth and design two event-triggered controllers to drive the disease from endemic to extinction. By analyzing the linear form of the stochastic epidemic model, the threshold of being endemic or extinction of the infectious disease has been obtained. Based on the Lyapunov stability theory, the mean square stability of the disease-free equilibrium of the stochastic system under event-triggered control has been analyzed. By applying the comparison principle, stochastic analysis method, etc., the almost sure exponential stability for the stochastic epidemic model under event-triggered control has been achieved. Finally, the effectiveness of the theoretical results has been demonstrated in a numerical example.
The remainder of the paper is organized as follows: In Section 2, a classical plant infectious disease model is introduced. In Section 3, the existence of a global positive solution of the system is discussed, the sufficient conditions for being endemic or extinction of the infectious disease are given, and when the disease becomes endemic, two event-triggered controllers are designed to achieve the mean square stability and almost sure exponential stability of the disease-free equilibrium for the stochastic epidemic system. In Section 4, an illustrative example is used to demonstrate. Finally, conclusions and discussion are presented.
Notation: Let R+ be the set of non-negative real numbers, and Rn+ be n dimensional Euclidean space. Rn×n stands for the set of all n×n real matrices, and ||⋅|| denotes the Euclidean norm in Rn. The superscript T denotes matrix transposition. a∨b represents the maximum of a and b, and a∧b denotes the minimum of a and b. (Ω,F,P) stands for a complete probability space with a filtration {Ft}t≥0, and C2,1(R2+×R+;R+) denotes the set of functions from R2+×R+ to R+ which are continuously twice differentiable in ξ(0)∈R2+ and once differentiable in t∈R+. <M(t),M(t)>t≥0 is called the quadratic variation of M(t).
Next, a model which is typical for a large class of plant infectious disease dynamics and may be applicable to other ecological realms is given, as follows:
{dS(t)=S(t)(α1−βI(t)−δ1S(t))dtdI(t)=I(t)(−α2+βS(t)−δ2I(t))dt, | (2.1) |
where S(t) is the number of healthy individuals who are susceptible to infection, and I(t) is the number of infected individuals who can transmit the disease to the healthy ones. α1>0 and α2>0 denote the recruitment rate of susceptible individuals and the death rate of infected individuals, respectively. β>0 is the transmission coefficient of susceptible individuals when they contact the infectious. δ1>0 and δ2>0 denote respectively the intraspecific competition coefficients of susceptible individuals and infected individuals, which are caused by the limitation of natural resources and environmental conditions.
By simple computation, it is not difficult to obtain the threshold value γ0=α1βδ1α2 of the system (2.1), i.e., when α2β<α1δ1, system (2.1) has a locally stable equilibrium (S∗,I∗), where S∗=α1δ2+βα2β2+δ1δ2 and I∗=βα1−δ1α2β2+δ1δ2. When α2β>α1δ1, system (2.1) exists a stable disease-free equilibrium (S0,0), where S0=α1δ1.
In fact, in the real environment, some parameters of the system will inevitably be disturbed by noise [9,10]. Recall that the parameter β represents the transmission coefficient of susceptible individuals when they contact the infectious. In practice, when considering stochastic environmental disturbance, we usually estimate it by an average value plus an error term. By the central limit theorem, the error term often follows a normal distribution and can be described by Gaussian white noise. Therefore, we may model the case by perturbing the transmission coefficient β of the system (2.1) by β+ρdω(t) to obtain the following stochastic differential equations:
{dS(t)=S(t)(α1−βI(t)−δ1S(t))dt−ρS(t)I(t)dω(t)dI(t)=I(t)(−α2+βS(t)−δ2I(t))dt+ρS(t)I(t)dω(t), | (2.2) |
where ω(t) is a Brownian motion defined on the complete probability space (Ω,F,P) with a natural filtration {Ft}t≥0. ρ is the Gaussian white noise intensity coefficient.
Remark 1. The general method of introducing noise to the deterministic epidemic model is to perturb some important parameters in a deterministic model. In this paper, the transmission coefficient β of the system (2.1) is perturbed, which is different from many studies [15,16,17], where the parameters α1 and α2 are perturbed.
Remark 2. In this paper, the transmission coefficient β has been estimated as an average value β0 plus an error term, and the error term follows a normal distribution with a mean of zero. Another approach for introducing environmental stochasticity to the disease transmission coefficient is to model the transmission coefficient as a time-dependent stochastic process β(t), where β(t) may be a Wiener process and approximately normally distributed with mean β0 [34]. Although both methods are biologically reasonable, the first method is more suitable for autonomous systems.
To prove the main results, the following Lemma is needed.
Lemma 1. For the systems [35]
dX(t)=f(t,X(t))dt+g(t,X(t))dω(t), | (2.3) |
and the linear form of Eq (2.3)
dX(t)=F(t)X(t)dt+G(t)X(t)dω(t), | (2.4) |
If the linear system (2.4) with constant coefficients is asymptotically stable almost surely (or in probability), and the coefficients of systems (2.3) and (2.4) satisfy inequality
|f(t,X)−F(t)⋅X|+|g(t,X)−G(t)⋅X|≤γ|X|, for all (t,X)∈[t0,∞)×Rd, | (2.5) |
in a sufficiently small neighbourhood of the point x=0 and with a sufficiently small constant γ, then the trivial solution X(t)=0 of the system (2.3) is globally asymptotically stable.
In this section, we will discuss the existence of a global positive solution of the system (2.2), the asymptotic stability of the disease-free equilibrium (S0,0) of the system (2.2), the properties of the solution of the system (2.2) near the epidemic equilibrium (S∗,I∗) of the original system (2.1), the mean square stability of the disease-free equilibrium of the system (2.2) via event-triggered control, and the almost sure exponential stability of the disease-free equilibrium of the system (2.2) via event-triggered control.
Next, we will prove the existence of a global positive solution of the system (2.2) by a similar method as in [36] and [37].
Theorem 1. If the Gaussian white noise intensity ρ satisfies 12ρ2<δ1 and 12ρ2<δ2, then the system (2.2) has a unique global positive solution (S(t),I(t)) with probability one on t≥0 for any initial value (S(0),I(0))∈R2+.
Proof. The coefficients of the system (2.2) satisfy the local Lipschitz condition. When the initial value (S(0),I(0)) of the system (2.2) is positive, it follows from [37] there exists a unique local positive solution for system (2.2) on t∈[0,τ∞), where τ∞ is the explosion time. Next, we need to prove τ∞=∞ a.s. If this statement is not true, then we can find a pair of positive constants ξ and T such that
P(τ∞≤T)>ξ. | (3.1) |
Choose a sufficiently large integer k0≥0 so that S(0)∈(1k0,k0) and I(0)∈(1k0,k0). For each integer k>k0, define the stopping time
τk=inf{t∈[0,τ∞):min{S(t),I(t)}≤1k or max{S(t),I(t)}≥k}. | (3.2) |
For τk→τ∞ almost surely, we have
P(τk≤T)>ξ. | (3.3) |
Define a function V∈C2,1(R+×R+;R+) by
V(S,I)=S(t)−1−ln(S(t))+I(t)−1−ln(I(t)). | (3.4) |
For any t0≤t≤T, by the Dynkin Foumula [37], we have
EV(S(t∧τk),I(t∧τk))=V(S(t0),I(t0))+E∫t∧τkt0LV(S(s),I(s))ds, | (3.5) |
where LV(S,I) is defined by
LV(S,I)=(S(t)−1)(α1−βI(t)−δ1S(t))+12ρ2I2(t)+(I(t)−1)(−α2+βS(t)−δ2I(t))+12ρ2S2(t)=(12ρ2−δ1)S2(t)+(δ1−β+α1)S(t)−α1+(12ρ2−δ2)I2(t)+(δ2+β−α2)I(t)+α2. | (3.6) |
Based on conditions 12ρ2<δ1 and 12ρ2<δ2, we get 12ρ2−δ1<0 and 12ρ2−δ2<0. So, there must exist a positive constant H satisfying LV(S,I)≤H. Substituting this into (3.5), we have
EV(S(T∧τk),I(T∧τk))≤V(S(t0),I(t0))+HT. | (3.7) |
Set Ak={τk≤T}, and by (3.3), we have
P(Ak)>ξ. | (3.8) |
For every ω∈Ak, S(ω,τk) and I(ω,τk) equal to either k or 1k, by (3.7), we obtain
V(S(t0),I(t0))+HT≥E[1Ak(ω)V(S(ω,τk),I(ω,τk))]≥ξ[(k−1−lnk)∧(1k−1−ln1k)]. | (3.9) |
Letting k→∞ leads to the contradiction
∞>V(S(t0),I(t0))+HT=∞, | (3.10) |
so we must have τ∞=∞ a.s. The proof is complete.
Let ˜S(t)=S(t)−S0 and ˜I(t)=I(t), and then the system (2.2) can be written as follows:
{d˜S(t)=(˜S(t)+S0)(−β˜I(t)−δ1˜S(t))dt−ρ(˜S(t)+S0)˜I(t)dω(t)d˜I(t)=˜I(t)(−α2+β(˜S(t)+S0)−δ2˜I(t))dt+ρ(˜S(t)+S0)˜I(t)dω(t). | (3.11) |
Letting (S(t),I(t))=(0,0) is the trivial solution of system (3.11). By using the above variables substitution, the stochastic asymptotic stability of the disease-free equilibrium (S0,0) of the system (2.2) is equivalent to the stochastic asymptotic stability of the trivial solution (0,0) of the system (3.11).
In order to achieve the stochastic asymptotic stability of trivial solution of the system (3.11), we first consider the linear form of the system (3.11),
{d˜S(t)=(−βS0˜I(t)−δ1S0˜S(t))dt−ρS0˜I(t)dω(t)d˜I(t)=(βS0−α2)˜I(t)dt+ρS0˜I(t)dω(t). | (3.12) |
Set R∗=α2S0+12ρ2S0 and prove the following result:
Lemma 2. If β<R∗, then the trivial solution of the system (3.12) is globally asymptotically stable.
Proof. The second equation of (3.12) has the explicit solution
˜I(t)=˜I(t0)exp{∫tt0(βS0−α2−12ρ2S20)ds+∫tt0ρS0dω(s)}. | (3.13) |
Taking logarithms of both sides of (3.13),
log˜I(t)=log˜I(t0)+(t−t0)(βS0−α2−12ρ2S20)+M(t), | (3.14) |
where M(t)=∫tt0ρS0dω(s) is a local martingale, and the quadratic variation ⟨M(t),M(t)⟩ of M(t) is bounded. Based on the Strong law of large numbers for local martingales [37], we have
limt→∞M(t)t=0. | (3.15) |
Taking limits on both sides of (3.14) and from (3.15), we obtain
limt→∞1tlog˜I(t)=βS0−α2−12ρ2S20. | (3.16) |
Based on the condition β<R∗, we have
limt→∞˜I(t)=0. a.s. | (3.17) |
Next, consider the first equation of (3.12), and based on Itô's formula,
log˜S(t)=log˜S(t0)+∫tt0(−δ1S0−βS0˜I(s)˜S(s)−12ρ2S20(˜I(s)˜S(s))2)ds−∫tt0ρS0˜I(s)˜S(s)dω(s). | (3.18) |
Taking limits on both sides of (3.18),
limt→∞1tlog˜S(t)≤limt→∞[−1t∫tt0δ1S0ds−1t∫tt0βS0˜I(s)˜S(s)ds−1t∫tt012ρ2S20(˜I(s)˜S(s))2ds]≤0. | (3.19) |
So, we have
limt→∞˜S(t)=0. a.s. | (3.20) |
Theorem 2. If β<R∗, then the disease-free equilibrium (S0,0) of the system (2.2) is globally asymptotically stable.
Proof. Consider the left-hand side of the system (3.11) and its linear form (3.12)
√((˜S+S0)(−β˜I−δ1˜S)+(βS0˜I+δ1S0˜S))2+(˜I(−α2+β(˜S+S0)−δ2˜I)−(βS0−α2)˜I)2+√(−ρ(˜S+S0)˜I+ρS0˜I)2+(ρ(˜S+S0)˜I−ρS0˜I)2=√(β˜S˜I+δ1˜S2)2+(β˜S˜I−δ2˜I2)2+√2ρ2˜S2˜I2≤√2ρ˜S˜I+√2β˜S˜I+δ1˜S2+δ2˜I2<η||Λ(t)||, | (3.21) |
where Λ(t)=[˜ST(t),˜IT(t)]T, ˜S(t)∈(−ε,ε), ˜I(t)∈(−ε,ε), and η=4(√2ρε∨√2βε∨δ1ε∨δ2ε).
Base on Lemmas 1 and 2, the trivial solution of the system (3.11) is globally asymptotically stable. Accordingly, if condition β<R∗ holds, the disease-free equilibrium (S0,0) of the system (2.2) is globally asymptotically stable, i.e., the disease fades out.
To obtain the threshold of being endemic or extinction of the infectious disease, we will investigate the properties of the solution of the model (2.2) near the epidemic equilibrium of the original deterministic system (2.1).
Theorem 3. If β>R∗ and δ2>12ρ2S∗, then the solution of the system (2.2) satisfies
lim supt→∞1tE∫tt0(S(s)−2δ1S∗2δ1−ρ2I∗)2+(I(s)−2δ2I∗2δ2−ρ2S∗)2ds≤hh3, | (3.22) |
where h=ρ2δ1I∗(S∗)22δ1−ρ2I∗+ρ2δ2S∗(I∗)22δ2−ρ2S∗, h1=δ1−12ρ2I∗, h2=δ2−12ρ2S∗, h3=h1∧h2.
Proof. For the system (2.2), construct the following Lyapunov function V∈C2,1(R+×R+;R+):
V(S,I)=S(t)−S∗−S∗ln(S(t)/S∗)+I(t)−I∗−I∗ln(I(t)/I∗). | (3.23) |
Computing LV along the trajectory of the system (2.2), we get
LV(S,I)=(S(t)−S∗)(−β(I(t)−I∗)−δ1(S(t)−S∗))+12ρ2S∗I2(t)+(I(t)−I∗)(β(S(t)−S∗)−δ2(I(t)−I∗))+12ρ2I∗S2(t)=−δ1(S(t)−S∗)2−δ2(I(t)−I∗)2+12ρ2S∗I2(t)+12ρ2I∗S2(t)=−(δ1−12ρ2I∗)(S(t)−2δ1S∗2δ1−ρ2I∗)2+ρ2δ1I∗(S∗)22δ1−ρ2I∗−(δ2−12ρ2S∗)(I(t)−2δ2I∗2δ2−ρ2S∗)2+ρ2δ2S∗(I∗)22δ2−ρ2S∗. | (3.24) |
Based on the Dynkin formula [37], we have
EV(S(t),I(t))=EV(S(t0),I(t0))+E∫tt0LV(S(s),I(s))ds≥0. | (3.25) |
Set h1=δ1−12ρ2I∗, h2=δ2−12ρ2S∗, h=ρ2δ1I∗(S∗)22δ1−ρ2I∗+ρ2δ2S∗(I∗)22δ2−ρ2S∗. When conditions β>R∗ and δ2>12ρ2S∗ hold, h1>0 and h2>0. So, we obtain
lim supt→∞1tE∫tt0(S(s)−2δ1S∗2δ1−ρ2I∗)2+(I(s)−2δ2I∗2δ2−ρ2S∗)2ds≤hh3, | (3.26) |
where h3=h1∧h2.
Remark 3. By Theorem 3, we obtain that when conditions β>R∗ and δ2>12ρ2S∗ hold, the solution of the system (2.2) finally fluctuates around the point Q(2δ1S∗2δ1−ρ2I∗,2δ2I∗2δ2−ρ2S∗), which is related to the epidemic equilibrium (S∗,I∗) of the original deterministic system (2.1). Especially, when the noise intensity coefficient ρ=0, the point Q(2δ1S∗2δ1−ρ2I∗,2δ2I∗2δ2−ρ2S∗) becomes the epidemic equilibrium (S∗,I∗).
Remark 4. By Theorems 2 and 3, we notice that when the condition β<R∗ holds, the disease fades out; and when the conditions β>R∗ and δ2>12ρ2S∗ hold, the disease becomes endemic. The threshold value R∗ is related to the noise intensity coefficient. It is obvious that reducing the transmission rate β is helpful to control the disease.
How to mitigate the epidemic-spreading processes when the transmission rate β is very large and the disease has become epidemic is the next question.
By Theorem 3, when β>R∗ and δ2>12ρ2S∗, the disease becomes endemic. In order to drive the disease from epidemic to extinction and maintain stability at the disease-free equilibrium point (S0,0), a feedback controller U(t) should be required, as follows:
{dS(t)=S(t)(α1−βI(t)−δ1S(t))dt−ρS(t)I(t)dω(t)dI(t)=[I(t)(−α2+βS(t)−δ2I(t))+U(t)]dt+ρS(t)I(t)dω(t), | (3.27) |
where U(t) is a control input.
As previously described in the Introduction, traditional control strategies for epidemic models assume that the control inputs (the number of medical resources and the level of traffic regulations) can be updated continuously or even per unit of time. However, such frequent control updates are neither economical nor practical because even a small change to control inputs should require tremendous effort. In order to reduce the number of control actuation updates, the event-triggered control is considered in the controlled system (3.27). In the system with an event trigger mechanism, the event-triggered control is updated only when certain trigger conditions are met. Suppose t0,t1,...,tk,... is the event-triggering time sequence, which will be determined by the next proposed event-triggered condition. By introducing the event-triggered protocol, the system (3.27) will be changed into
{dS(t)=S(t)(α1−βI(t)−δ1S(t))dt−ρS(t)I(t)dω(t)dI(t)=[I(t)(−α2+βS(t)−δ2I(t))+U(tk)]dt+ρS(t)I(t)dω(t).∀t∈[tk,tk+1),k=0,1,... | (3.28) |
Remark 5. From the system (3.28), It is easy to find that the control input U(t) of the event-triggered control holds a constant U(tk) in the time interval [tk,tk+1), which is often referred to as sample-and-hold in the literature and different from feedback control where control inputs are updated continuously (for the continuous-time case) or per unit of time (for the discrete-time case).
In order to achieve the mean square stability of the disease-free equilibrium for the system (2.2), the control input U(t) of the system is designed in the following form:
U(t)=−γI(t), | (3.29) |
where γ denotes the isolation or cure rate of infected individuals and is often affected by the number of medical resources and the level of traffic regulations. In the time interval [tk,tk+1), the control input U(t)=U(tk) is only related to the control gain γ and the number of infected individuals I(tk) at the time tk.
Consider the following event-triggered condition:
|ε(t)|≤λ1I2(t), | (3.30) |
where ε(t)=I(t)−I(tk) is the state measurement error of the current state and the state at the last event-triggering instant for the system, λ1 is called the event-triggering gain. When the state measurement error of these states meets the trigger condition (3.30), the controller will update to drive the disease from endemic to extinction.
For the convenience of calculation, the system (3.28) can be rewritten:
{dS(t)=S(t)(α1−βI(t)−δ1S(t))dt−ρS(t)I(t)dω(t)dI(t)=[I(t)(−α2+βS(t)−δ2I(t))−γ(I(t)−ε(t))]dt+ρS(t)I(t)dω(t).∀t∈[tk,tk+1) | (3.31) |
Theorem 4. Assume β>R∗ and δ2>12ρ2S∗. If there exist the control gain γ>0 of the control input (3.29) and the event-triggering gain λ1>0 of the event-triggered condition (3.30) satisfying
γ=βS0−α2, | (3.32) |
λ1≤2δ2−S0ρ22βS0−2α2, | (3.33) |
then the disease-free equilibrium (S0,0) is globally asymptotically stable in the mean square under the controller U(t), i.e., the disease fades out.
Proof. For the system (3.31), set the initial value ξ(0)∈R2+ and construct the following Lyapunov function V∈C2,1(R+×R+;R+):
V(S,I)=S(t)−S0−S0ln(S(t)/S0)+I(t). ∀t∈[tk,tk+1). | (3.34) |
Then, based on Itô's formula and from (3.32), we have
dV(S,I)=LV(S,I)dt+ρS0I(t)dω(t), | (3.35) |
where
LV(S,I)=(S(t)−S0)(−βI(t)−δ1(S(t)−S0))+12S0ρ2I2(t)+I(t)(−α2+βS(t)−δ2I(t))−(βS0−α2)(I(t)−ε(t))=−δ1(S(t)−S0)2−(δ2−12S0ρ2)I2(t)+(βS0−α2)ε(t). | (3.36) |
For β>R∗, we have
LV(S,I)≤−δ1(S(t)−S0)2−(δ2−12S0ρ2)I2(t)+(βS0−α2)|ε(t)|. | (3.37) |
Making use of the event-triggered condition (3.30), we obtain
LV(S,I)≤−δ1(S(t)−S0)2−(δ2−12S0ρ2−λ1(βS0−α2))I2(t). | (3.38) |
Based on the condition (3.33), we have
LV(S,I)≤0. | (3.39) |
Taking the mathematical expectation operator of both sides of (3.35) and from (3.39), we have
dEV(S,I)=ELV(S,I)dt≤0. | (3.40) |
Finally, we get
dEV(S,I)dt≤0. | (3.41) |
By the Lyapunov stability theory, the system (2.2) is asymptotically stable in the mean square under the controller U(t). The proof is complete.
Remark 6. For the system (2.2), when β>R∗ and δ2>12ρ2S∗, the disease is epidemic. However, by choosing suitable control gain (3.32) and event-triggering gain (3.33), we can achieve mean square stability of the disease-free equilibrium (see Fig. 3), i.e., the disease fades out.
Remark 7. In Theorem 4, the triggering conditions are designed to guarantee that the time derivative of the similar Lyapunov function V(S,I) along the trajectory of the system (2.2) is negative, so as to achieve mean square stability of the disease-free equilibrium.
For a stochastic sequence, it is certain that the almost sure convergence cannot be obtained from the mean-square convergence. Consequently, it is necessary to design the other event-triggered controller to achieve the almost sure asymptotical stability of the disease-free equilibrium for the stochastic system (2.2). In order to obtain the almost sure asymptotical stability of the disease-free equilibrium for the system, consider the following event-triggered condition:
|ε(t)|≤λ2I(t), | (3.42) |
where λ2∈(0,1) is the event-triggering gain.
Remark 8. By Theorem 1 in [30], when a control system satisfies Lipschitz continuity, for the event-triggering condition (3.42) with λ2∈(0,1), there exists a minimal inter-event time τ∈R+ such that tk+1−tk≥τ for every k∈N. In other words, the inter-event times are always positive. The event-triggering gain λ2 is required as λ2∈(0,1) in the above event-triggered condition (3.42), which can avoid the Zeno behavior.
Let ˜S(t)=S(t)−S0 and ˜I(t)=I(t), then the system (3.31) can be written as follows
{d˜S(t)=(˜S(t)+S0)(−β˜I(t)−δ1˜S(t))dt−ρ(˜S(t)+S0)˜I(t)dω(t)d˜I(t)=[˜I(t)(−α2+β(˜S(t)+S0)−δ2˜I(t))−γ(˜I(t)−ε(t))]dt+ρ(˜S(t)+S0)˜I(t)dω(t).∀t∈[tk,tk+1) | (3.43) |
Next, consider the linear form of the system (3.43),
{d˜S(t)=(−βS0˜I(t)−δ1S0˜S(t))dt−ρS0˜I(t)dω(t)d˜I(t)=[(βS0−α2−γ)˜I(t)+γε(t)]dt+ρS0˜I(t)dω(t).∀t∈[tk,tk+1) | (3.44) |
Theorem 5. Assume β>R∗ and δ2>12ρ2S∗. If there exist the control gain γ>0 of the control input (3.29) and the event-triggering gain λ2∈(0,1) of the event-triggering condition (3.42) satisfying
(1−λ2)γ≥βS0−α2−12ρ2S20, | (3.45) |
then the disease-free equilibrium (S0,0) is almost surely exponentially stable under the controller U(t), i.e., the disease fades out.
Proof. Consider the following equation:
dφ(t)=(βS0−α2−(1−λ2)γ)φ(t)dt+ρS0φ(t)dω(t). ∀t∈[tk,tk+1), | (3.46) |
Eq (3.46) has the explicit solution
φ(t)=φ(tk)exp{∫ttk(βS0−α2−(1−λ2)γ−12ρ2S20)ds+∫ttkρS0dω(s)}. | (3.47) |
Taking logarithms and limits on both sides of (3.47),
limt→∞1tlogφ(t)=βS0−α2−(1−λ2)γ−12ρ2S20. | (3.48) |
From (3.45) and β>R∗, we obtain
limt→∞φ(t)=0. a.s. | (3.49) |
For the second equation of the linear system (3.44), making use of the condition (3.42) and based on the comparison principle, we have
limt→∞˜I(t)=0. a.s. | (3.50) |
For the system (3.43), similar to the proof to Theorem 2, we obtain
limt→∞1tlog˜S(t)≤0 and limt→∞1tlog˜I(t)≤0, | (3.51) |
and then the disease-free equilibrium (S0,0) of the system (3.43) is almost surely exponentially stable under the controller U(t), i.e., the disease fades out.
Remark 9. From the inequality (3.45) in Theorem 5, we notice that when we improve the control gain γ or decrease the event-triggering gain λ2, i.e., improve the isolation or cure rate of infected individuals or decrease the event-triggering threshold so as to enhance the frequency of event-triggered control, the disease becomes extinct.
In this section, an example of the system (2.2) will considered. Let α1=0.6,α2=0.25,δ1=1,δ2=1,ρ=0.8. When β=0.6, R∗=0.608, and the condition β<R∗ holds. By Theorem 2, the disease-free equilibrium (S0,0) of the system (2.2) is globally asymptotically stable. To illustrate this, we depict the evolution figure of the stability for the system (2.2) (see Figure 1), which shows that the system is finally stable at (S0,0).
When β=1, we derive R∗=0.608 and 12ρ2S∗=0.136. The conditions β>R∗ and δ2>12ρ2S∗ hold. By Theorem 3, the solution of the system (2.2) fluctuates around point Q. To illustrate this, we depict the evolution figure of the system (2.2) (see Figure 2), which shows that the disease becomes endemic.
When the conditions β>R∗ and δ2>12ρ2S∗ hold, the disease becomes endemic. Assume the event-triggering gain λ1=0.9 of the event-triggered control, and the condition λ1≤(2δ2−S0ρ22βS0−2α2=2.822) holds. By Theorem 4, the system (2.2) is stable in the mean square at the disease-free point (S0,0) under the controller U(t). To illustrate this, we depict the evolution figure of the stability for the system (see Figure 3), which shows that the system is finally stable at (S0,0). Figure 4 shows the event-triggered control input U(t).
When the disease becomes endemic, assume the event-triggering gain λ2=0.8 and control gain γ=2 of the event-triggered control, and then the condition (1−λ2)γ≥(βS0−α2−12ρ2S20=0.3428) holds. By Theorem 5, the system (2.2) is almost surely exponentially stable at the disease-free point (S0,0) under the controller U(t). To illustrate this, we depict the evolution figure of the stability for the system (see Figure 5), which shows that the system is finally stable at (S0,0). Figure 6 shows the event-triggered control input U(t).
In this paper, we have discussed the stability at the disease-free equilibrium (S0,0) of the system (2.2), the properties of the solution of the system (2.2) near the epidemic equilibrium (S∗,I∗) of the original system (2.1), and the event-triggered control for the stochastic epidemic model. By Theorems 2 and 3, we achieve that when β<R∗, the disease fades out. When β>R∗ and δ2>12ρ2S∗, the solution of the system (2.2) fluctuates around a point, i.e., the disease becomes endemic. By Theorems 4 and 5, we obtain that when the disease is endemic, if the event-triggering gain and control gain are correctly chosen, the disease can be driven from endemic to extinction under the event-triggered controller.
This work was supported by China Rongtong Agricultural Development Group Corporation Limited, National Natural Science Foundation of China (61573095) and Yunnan Fundamental Research Projects (202001AT070112).
The authors declared that there is no conflict of interest in this work.
[1] |
J. S. Weitz, J. Dushoff, Modeling post-death transmission of Ebola: Challenges for inference and opportunities for control, Sci. Rep., 5 (2015), 1–7. https://doi.org/10.1038/srep08751 doi: 10.1038/srep08751
![]() |
[2] |
R. E. Morrison, Jr. A. Cunha, Embedded model discrepancy: A case study of Zika modeling, Chaos, 30 (2020), 051103. https://doi.org/10.1063/5.0005204 doi: 10.1063/5.0005204
![]() |
[3] |
K. A. Hattaf, New generalized definition of fractional derivative with non-singular kernel, Computation, 8 (2020), 49. https://doi.org/10.3390/computation8020049 doi: 10.3390/computation8020049
![]() |
[4] |
K. A. Hattaf, On the stability and numerical scheme of fractional differential equations with application to biology, Computation, 10 (2022), 97. https://doi.org/10.3390/computation10060097 doi: 10.3390/computation10060097
![]() |
[5] |
M. Lipsitch, T. Cohen, B. Cooper, J. M. Robins, S. Ma, L. James, et al., Transmission dynamics and control of severe acute respiratory syndrome, Science, 300 (2003), 1966–1970. https://doi.org/10.1126/science.1086616 doi: 10.1126/science.1086616
![]() |
[6] |
C. Nowzari, V. M. Preciado, G. J. Pappas, Analysis and control of epidemics: A survey of spreading processes on complex networks, IEEE Control Syst. Mag., 36 (2016), 26–46. https://doi.org/10.1109/MCS.2015.2495000 doi: 10.1109/MCS.2015.2495000
![]() |
[7] |
X. Li, S. Song, J. Wu, Exponential stability of nonlinear systems with delayed impulses and applications, IEEE Trans. Autom. Control, 64 (2019), 4024–4034. https://doi.org/10.1109/TAC.2019.2905271 doi: 10.1109/TAC.2019.2905271
![]() |
[8] |
I. Ahn, S. Heo, S. Ji, K. H. Kim, T. Kim, E. J. Lee, et al., Investigation of nonlinear epidemiological models for analyzing and controlling the MERS outbreak in Korea, J. Theor. Biol., 437 (2018), 17–28. https://doi.org/10.1016/j.jtbi.2017.10.004 doi: 10.1016/j.jtbi.2017.10.004
![]() |
[9] | R. M. May, Stability and Complexity in Model Ecosystems, Princeton: Princeton University Press, 2001. https://doi.org/10.1515/9780691206912 |
[10] |
D. Vasseur, P. Yodzis, The color of environmental noise, Ecology, 85 (2004), 1146–1152. https://doi.org/10.1890/02-3122 doi: 10.1890/02-3122
![]() |
[11] |
Q. Yang, X. Mao, Stochastic dynamics of SIRS epidemic models with random perturbation, Math. Biosci. Eng., 11 (2014), 1003–1025. https://doi.org/10.3934/mbe.2014.11.1003 doi: 10.3934/mbe.2014.11.1003
![]() |
[12] |
X. Xiao, S. Joshi, J. Cecil, Critical assessment of shape retrieval tools (SRTs), Int. J. Adv. Manuf. Technol., 116 (2021), 3431–3446. https://doi.org/10.1007/s00170-021-07681-4 doi: 10.1007/s00170-021-07681-4
![]() |
[13] |
Q. Liu, Q. Chen, Analysis of the deterministic and stochastic SIRS epidemic models with nonlinear incidence, Phys. A, 428 (2015), 140–153. https://doi.org/10.1016/j.physa.2015.01.075 doi: 10.1016/j.physa.2015.01.075
![]() |
[14] |
X. Xiao, C. Waddell, C. Hamilton, H. Xiao, Quality prediction and control in wire arc additive manufacturing via novel machine learning framework, Micromachines, 13 (2022), 137. https://doi.org/10.3390/mi13010137 doi: 10.3390/mi13010137
![]() |
[15] |
W. Zhao, J. Li, T. Zhang, X. Meng, T. Zhang, Persistence and ergodicity of plant disease model with markov conversion and impulsive toxicant input, Commun. Nonlinear Sci. Numer. Simul., 48 (2017), 70–84. https://doi.org/10.1016/j.cnsns.2016.12.020 doi: 10.1016/j.cnsns.2016.12.020
![]() |
[16] |
Q. Liu, D. Jiang, T. Hayat, A. Alsaedi, B. Ahmad, A stochastic SIRS epidemic model with logistic growth and general nonlinear incidence rate, Phys. A, 551 (2020), 124152. https://doi.org/10.1016/j.physa.2020.124152 doi: 10.1016/j.physa.2020.124152
![]() |
[17] |
X. Meng, X. Wang, Stochastic predator-prey system subject to Lévy jumps, Discrete Dyn. Nat. Soc., (2016), 5749892. https://doi.org/10.1155/2016/5749892 doi: 10.1155/2016/5749892
![]() |
[18] |
A. J. Gray, D. Greenhalgh, L. Hu, X. Mao, J. Pan, A stochastic differential equation SIS epidemic model, Soc. Ind. Appl. Math., 71 (2011), 876–902. https://doi.org/10.1137/10081856X doi: 10.1137/10081856X
![]() |
[19] |
Q. Liu, D. Jiang, N. Shi, T. Hayat, A. Alsaedi, Periodic solution for a stochastic nonautonomous SIR epidemic model with logistic growth, Phys. A, 462 (2016), 816–826. https://doi.org/10.1016/j.physa.2016.06.052 doi: 10.1016/j.physa.2016.06.052
![]() |
[20] |
S. Rajasekar, M. Pitchaimani, Q. Zhu, Progressive dynamics of a stochastic epidemic model with logistic growth and saturated treatment, Phys. A, (2019), 122649. https://doi.org/10.1016/j.physa.2019.122649 doi: 10.1016/j.physa.2019.122649
![]() |
[21] | A. R. Khalili, A. Heydari, M. R. Zarrabi, Analysis and control of SEIR epidemic model via sliding mode control, Adv. Model. Optim., 18 (2016), 153–162. |
[22] |
M. Sharifi, H. Moradi, Nonlinear robust adaptive sliding mode control of influenza epidemic in the presence of uncertainty, J. Process Control, 56 (2017), 48–57. https://doi.org/10.1016/j.jprocont.2017.05.010 doi: 10.1016/j.jprocont.2017.05.010
![]() |
[23] |
G. Rohith, K. B. Devika, Dynamics and control of COVID-19 pandemic with nonlinear incidence rates, Nonlinear Dyn., 101 (2020), 2013–2026. https://doi.org/10.1007/s11071-020-05774-5 doi: 10.1007/s11071-020-05774-5
![]() |
[24] |
L. J. Chen, J. T. Sun, Global stability of an SI epidemic model with feedback controls, Appl. Math. Lett., 28 (2014), 53–55. https://doi.org/10.1016/j.aml.2013.09.009 doi: 10.1016/j.aml.2013.09.009
![]() |
[25] |
X. Xiao, B. Roh, C. Hamilton, Porosity management and control in powder bed fusion process through process-quality interactions, CIRP J. Manuf. Sci. Technol., 38 (2022), 120–128. https://doi.org/10.1016/j.cirpj.2022.04.005 doi: 10.1016/j.cirpj.2022.04.005
![]() |
[26] |
S. Gao, D. Zhong, Z. Yan, Analysis of novel stochastic switched SILI epidemic models with continuous and impulsive control, Phys. A, 495 (2018), 162–171. https://doi.org/10.1016/j.physa.2017.12.050 doi: 10.1016/j.physa.2017.12.050
![]() |
[27] |
X. Li, D. Peng, J. Cao, Lyapunov stability for impulsive systems via event-triggered impulsive control, IEEE Trans. Autom. Control, 65 (2020), 4908–4913. https://doi.org/10.1109/TAC.2020.2964558 doi: 10.1109/TAC.2020.2964558
![]() |
[28] | M. Ogura, V. M. Preciado, Optimal containment of epidemics in temporal and adaptive networks, in Temporal Network Epidemiology, Springer, (2017), 241–266. https://doi.org/10.1007/978-981-10-5287-3 https://doi.org/10.1007/978-981-10-5287-3_11 |
[29] |
C. Nowzari, V. M. Preciado, G. J. Pappas, Optimal resource allocation for control of networked epidemic models, IEEE Trans. Control Network Syst., 4 (2017), 159–169. https://doi.org/10.1109/TCNS.2015.2482221 doi: 10.1109/TCNS.2015.2482221
![]() |
[30] |
P. Tabuada, Event-triggered real-time scheduling of stabilizing control tasks, IEEE Trans. Autom. Control, 52 (2007), 1680–1685. https://doi.org/10.1109/TAC.2007.904277 doi: 10.1109/TAC.2007.904277
![]() |
[31] | W. Heemels, K. H. Johansson, P. Tabuada, An introduction to event-triggered and self-triggered control, in Proceedings of the 51st IEEE Conference on Decision and Control, (2012), 3270–3285. https://doi.org/10.1109/CDC.2012.6425820 |
[32] |
X. Li, X. Yang, J. Cao, Event-triggered impulsive control for nonlinear delay systems, Automatica, 117 (2020), 108981. https://doi.org/10.1016/j.automatica.2020.108981 doi: 10.1016/j.automatica.2020.108981
![]() |
[33] |
K. Hashimoto, Y. Onoue, M. Ogura, T. Ushio, Event-triggered control for mitigating SIS spreading processes, Ann. Rev. Control, 52 (2021), 479–494. https://doi.org/10.1016/j.arcontrol.2021.08.001 doi: 10.1016/j.arcontrol.2021.08.001
![]() |
[34] | E. Allen, Modeling with Ito Stochastic Differential Equations, Springer-Verlag, Dordrecht, The Netherlands, 2007. |
[35] | R. Z. Hasminskij, Stochastic Stability of Differential Equations, Sijthoof and Noordhoof, Alphen aan den Rijn, The Netherlands, 1980. |
[36] |
K. Hattaf, M. Mahrouf, J. Adnani, N. Yousfi, Qualitative analysis of a stochastic epidemic model with specific functional response and temporary immunity, Phys. A, 490 (2018), 591–600. https://doi.org/10.1016/j.physa.2017.08.043 doi: 10.1016/j.physa.2017.08.043
![]() |
[37] | X. Mao, C. Yuan, Stochastic Differential Equations with Markovian Switching, Imperial College Press: London, 2006. |