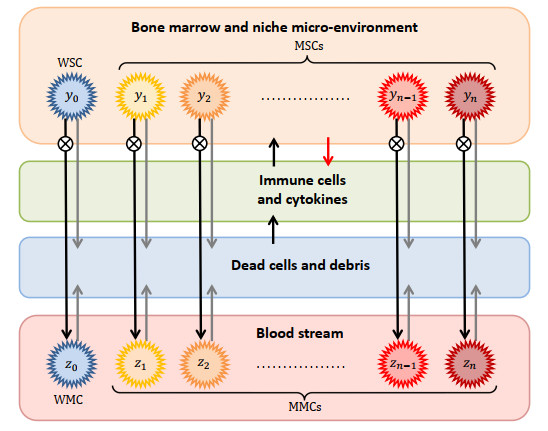
Facial makeup transfer is a special form of image style transfer. For the reference makeup image with large-pose, improving the quality of the image generated after makeup transfer is still a challenging problem worthy of discussion. In this paper, a large-pose makeup transfer algorithm based on generative adversarial network (GAN) is proposed. First, a face alignment module (FAM) is introduced to locate the key points, such as the eyes, mouth and skin. Secondly, a face parsing module (FPM) and face parsing losses are designed to analyze the source image and extract the face features. Then, the makeup style code is extracted from the reference image and the makeup transfer is completed through integrating facial features and makeup style code. Finally, a large-pose makeup transfer (LPMT) dataset is collected and constructed. Experiments are carried out on the traditional makeup transfer (MT) dataset and the new LPMT dataset. The results show that the image quality generated by the proposed method is better than that of the latest method for large-pose makeup transfer.
Citation: Qiming Li, Tongyue Tu. Large-pose facial makeup transfer based on generative adversarial network combined face alignment and face parsing[J]. Mathematical Biosciences and Engineering, 2023, 20(1): 737-757. doi: 10.3934/mbe.2023034
[1] | Zizi Wang, Zhiming Guo, Hal Smith . A mathematical model of oncolytic virotherapy with time delay. Mathematical Biosciences and Engineering, 2019, 16(4): 1836-1860. doi: 10.3934/mbe.2019089 |
[2] | Elzbieta Ratajczyk, Urszula Ledzewicz, Maciej Leszczynski, Avner Friedman . The role of TNF-α inhibitor in glioma virotherapy: A mathematical model. Mathematical Biosciences and Engineering, 2017, 14(1): 305-319. doi: 10.3934/mbe.2017020 |
[3] | Jianjun Paul Tian . The replicability of oncolytic virus: Defining conditions in tumor virotherapy. Mathematical Biosciences and Engineering, 2011, 8(3): 841-860. doi: 10.3934/mbe.2011.8.841 |
[4] | Peter S. Kim, Joseph J. Crivelli, Il-Kyu Choi, Chae-Ok Yun, Joanna R. Wares . Quantitative impact of immunomodulation versus oncolysis with cytokine-expressing virus therapeutics. Mathematical Biosciences and Engineering, 2015, 12(4): 841-858. doi: 10.3934/mbe.2015.12.841 |
[5] | Donggu Lee, Aurelio A. de los Reyes V, Yangjin Kim . Optimal strategies of oncolytic virus-bortezomib therapy via the apoptotic, necroptotic, and oncolysis signaling network. Mathematical Biosciences and Engineering, 2024, 21(3): 3876-3909. doi: 10.3934/mbe.2024173 |
[6] | Taeyong Lee, Adrianne L. Jenner, Peter S. Kim, Jeehyun Lee . Application of control theory in a delayed-infection and immune-evading oncolytic virotherapy. Mathematical Biosciences and Engineering, 2020, 17(3): 2361-2383. doi: 10.3934/mbe.2020126 |
[7] | Nada Almuallem, Dumitru Trucu, Raluca Eftimie . Oncolytic viral therapies and the delicate balance between virus-macrophage-tumour interactions: A mathematical approach. Mathematical Biosciences and Engineering, 2021, 18(1): 764-799. doi: 10.3934/mbe.2021041 |
[8] | Abdulhamed Alsisi, Raluca Eftimie, Dumitru Trucu . Nonlocal multiscale modelling of tumour-oncolytic viruses interactions within a heterogeneous fibrous/non-fibrous extracellular matrix. Mathematical Biosciences and Engineering, 2022, 19(6): 6157-6185. doi: 10.3934/mbe.2022288 |
[9] | Tuan Anh Phan, Jianjun Paul Tian . Basic stochastic model for tumor virotherapy. Mathematical Biosciences and Engineering, 2020, 17(4): 4271-4294. doi: 10.3934/mbe.2020236 |
[10] | Khaphetsi Joseph Mahasa, Rachid Ouifki, Amina Eladdadi, Lisette de Pillis . A combination therapy of oncolytic viruses and chimeric antigen receptor T cells: a mathematical model proof-of-concept. Mathematical Biosciences and Engineering, 2022, 19(5): 4429-4457. doi: 10.3934/mbe.2022205 |
Facial makeup transfer is a special form of image style transfer. For the reference makeup image with large-pose, improving the quality of the image generated after makeup transfer is still a challenging problem worthy of discussion. In this paper, a large-pose makeup transfer algorithm based on generative adversarial network (GAN) is proposed. First, a face alignment module (FAM) is introduced to locate the key points, such as the eyes, mouth and skin. Secondly, a face parsing module (FPM) and face parsing losses are designed to analyze the source image and extract the face features. Then, the makeup style code is extracted from the reference image and the makeup transfer is completed through integrating facial features and makeup style code. Finally, a large-pose makeup transfer (LPMT) dataset is collected and constructed. Experiments are carried out on the traditional makeup transfer (MT) dataset and the new LPMT dataset. The results show that the image quality generated by the proposed method is better than that of the latest method for large-pose makeup transfer.
Hematopoietic stem cells reside in the bone marrow and are responsible for continuously replenishing the blood cells; red blood cells, white blood cells, or platelets. The hematopoietic stem cells possess the abilities of self-renewal and extensive proliferation which are essential for the maintenance of the formation of blood cells, hematopoiesis [1].
The proliferation rate for each of the 104−105 normal hematopoietic stem cells is about once per every 25th to 50th week [2,3]. Cell numbers are amplified through a sequence of maturation stages, with estimated numbers of mitotic events being 31 [4]. As a result approximately one million new mature blood cells are generated per second corresponding to 1011 mature blood cells daily which is comparable to the number of stars in the Milky Way [5]. Evidently, a tight regulation of hematopoiesis is crucial and disturbances to the regulation of the stem cell cycle may have severe consequences [6]. Several hematopoietic diseases arise from mutations in the hematopoietic stem cells causing the regulation of hematopoiesis to be disturbed. Such distortion may cause disturbances or uncontrolled growth of dysfunctional blood cells resulting in diseases, e.g., blood cancer.
Approximately 175, 000 Americans are each year diagnosed with myeloid malignancies, e.g., acute myeloid leukemia (AML), myelodysplastic syndromes (MDS), chronic myeloid leukemia (CML) and the Philadelphia-negative myeloprofilerative neoplasms (MPNs). MPNs develop slowly on a time scale of 15-25 years whereas other leukemias, like AML and CML develop on a time scale of 1 year or even on a shorter time scale [7].
MPNs associated with the Philadelphia-negative mutations are driven by the JAK2V617F (hencefort JAK2), MPL, CALR or TET2 mutations [8]. MPNs mutations relate to the diseases; essential thrombocythemia (ET), polycythemia vera (PV) and primary myelofibrosis (PMF) [9]. The chronic MPNs are characterized by excessive myeloproliferation, which cause disturbance of hematopoiesis [10]. As a consequence, patients with MPNs have a high risk of thrombosis with cardiovascular complications and increased propensity to develop autoimmune and chronic inflammatory diseases [11,12,13]. Likewise, patients with chronic inflammatory disease have an elevated risk of an MPN disease and other cancers [14,15]. Moreover, MPNs may ultimately develop into AML which is a more rapidly progressing disease with a poor prognosis [16].
We present a proof of concept model referred to as the Multiple Clone Cancitis (MCC) model allowing for few as well as multiple clones in a flexible mathematical framework. The MCC model describes; the interaction of several types of competing stem cells in the bone marrow, whereof some may be malignant, their mature offspring in the blood circulation, the amount of dead cells and the activity of the immune system degrading the debris from the dead cells as well as the cytotoxic T-cell response of the adaptive immune system (see Figure 1 for a conceptual diagram of the MCC model).
The model is a generalization of the model presented by Andersen et al. in 2017 [17], analyzed in [18] and investigated using a reduction approach in [19], expanded to include the adaptive T-cell response explicitly by Ottesen et al. in 2019 [20], and used for prediction of a randomized trial in Ottesen 2020 [21]. In contrast to its predecessors, the presented MCC model allows for multiple competing clones and thereby enables reproduction of complex dynamics which its predecessors cannot account for.
We use the properties of the micro-environment, i.e., the cell to cell inhibition and immunoediting, to explain very different clinical observations in a diversity of hematopoietic disorders using the MCC model. In particular, we focus on the MCC models ability to reproduce the clinical observations;
● Observed fast oscillations in cell count of time scale 1 month for MPN patients.
● Consequences of CHIP leading to good, fair, or poor responders to treatment.
● Observed cell count during hydroxyurea treatment of MPNs.
● Elimination, equilibrium, and escape phases related to immunoediting.
● Developed resistance to treatment.
● Observed slow oscillations in cell count of time scale 2-3 months for CML patients.
Mathematical modeling of the above mentioned bullets have, to our knowledge, not been investigated before in a unified framework. By additionally including changes in the self-renewal rates the model also explains the evolution of clones over a life span of a human. The structure of the MCC model is chosen deliberately to ensure that the MCC model inherits the previous findings of the Cancitis model, e.g., observed non-oscillatory cell count progression and observed levels of cancer specific related cytokines, c-reactive proteins, and lactic dehydrogenase. Hence, the MCC model provides an agile model framework with the ability to unify all phenomena described.
As early as in 1970 Kennedy reported cyclic oscillations (period 35-40 days) in leukocyte counts in CML during hydroxyurea therapy [22]. Chikkappa et al. confirmed the finding in 1976 (period 53-69 days) and in addition similar oscillations were also observed in platelets, neutrophils, eosinophils, basophils and reticulocytes which continued for a period after off anti-leukemic therapy [23]. In a similar investigation from 1999 Fortin and Mackey estimated the period to 37-83 days [24]. Later Hirrayama et al. found oscillations in platelets and white blood cells in a patient diagnosed with CML (period 8 weeks corresponding to 244 days) [25]. More recently, Clapp et al. in 2015 and 2018 suggested such oscillations to be related to an inhibitory immune response by the amount of mature cells in a modeling study [26,27]. In 2019 Knauer et al. [28] used a mathematical model to achieve oscillations with a 20 day period fitting data from a patient with cyclic neutropenia. Considering patients with JAK2 positive MPNs (JAK2+ MPNs) faster oscillations (period 27 days on average) have also been observed clinically by several studies [29,30,31].
Competition or selection of clones as a driver for cancer evolution has been of long term interest. In this study we consider a clone as a group of cells having identical genome, i.e., DNA. Different clones may arise from mutations altering the genome and hereby constituting another clone. Such mutated cells may or may not be malignant and generally multiple sequential mutations are believed to take place before malignant cells arise. The frequency of somatic mutations in the hematopoietic stem cells increases many-folds with age; from an insignificant level for age less than 20 years to a frequency around 10% at age above 70 [32]. Based on a meta study, Heuser et al. 2016 concluded that clonal hematopoiesis of indeterminate potential (CHIP) is common and the frequency increases with age to around 10% at age 70-80 [33]. Park et al. investigated the role of CHIP and its role for stem cell transplantation [34]. Similar cytogenetic clonal evolution in relation to CML was detected by Cortes et al. in 2004 over a span of different disease burdens [35].
Mutations may accumulate during growth, resulting in heterogeneous malignant cells referred to as cancer heterogeneity [36]. Walter et al. reported that blood samples for AML patients may contain persistent antecedent founding of a clone containing 182 to 660 somatic mutations. Subclones harboring up to hundreds of mutations may emerge or outgrow the founding clone [37]. Ding et al. attributed relapse in AML treated patients to the existence of various clones which may lie latently, possible as a small amount of cells [38]. Cancer heterogeneity affect the response to therapy and can present a great challenge for cancer treatment [39]. Stiehl et al. investigated the role of heterogeneity of AML clones and resistance to therapy using a mathematical model [40].
Clinical experiences show that therapy may work well on a subetaoup having MPNs but not on others for unknown reasons. These subetaoups are denoted good and poor responders, respectively. A third subetaoup responds partially on the treatment, giving rise to coexistent equilibrium (or possibly an coexisting oscillatory) states where malignant clones can coexist with healthy clones for long time.
The three Es of cancer immunoediting describe elimination, equilibrium, and escape phases in cancer development [41]. In the presented work the three Es of immunoediting are related to CHIP as well as to the coexisting steady or oscillate states becoming resistant or gaining additional mutations which are less immuno-suppressible.
To understand complex systems it is preferable first to understand simple related systems which can be done either by in vitro or in silico experiments. This approach holds for investigating cancers too, where a single isolated or two competing cell types are often studied.
Inspired by clinical observations, the MCC model has been formulated in attempt to gain an understanding of the more complex dynamics such as resistance and oscillations.
The mutated stem cells (MSCs) include malignant as well as non-malignant stem cells. The malignant stem cells share the characteristics of the wild type stem cells (WSCs) but may have acquired the ability of 'indefinite' self-renewal [42,43] and may ultimately suppress the production of healthy blood cells [6]. The corresponding mature blood cells are shortly referred to as hematopoietic mature cells consisting of both wild type mature cells and mutated mature cells whereof some may be cancerous mature cells. Distortion in the number of mature blood cells may affect many vital functions such as fighting off infections, preventing serious bleeding or causing thrombosis.
A conceptual diagram of the MCC model is depicted in Figure 1. The WSCs can self renew, differentiate, die or mutate to MSCs with altered properties such as the malignant stem cells, which behave similar to the WSCs. The hematopoietic stem cell niches provide negative feedback, restoring stem cell numbers upon perturbations. Mature blood cells are the outcome of multiple mitosis events. For brevity we omit intermediate maturation stages, normally represented by approximately 30 generations of proliferative progenitor cells, and let the differentiation output of hematopoietic stem cells enter the production of mature cells with a multiplication factor corresponding to the amplification of intermediate cell divisions. The apoptotic cells have to be cleared by immune cells which provide feedback to the hematopoietic stem cell production. Prior to a malignant mutation, the model is calibrated such that typical clinical values are reproduced, e.g., stem cell numbers, mature cell numbers, time for restoration upon perturbation and well established life time of blood cells. Inflammation - a hallmark of cancer - is known to provide further growth advantage to mutated cells, thus the stem cell niche and the inflammatory level are included to investigate this effect [44].
The MSCs may share the characteristics of malignant stem cells and thereby cause cancer heterogeneity. Cancer heterogeneity are often believed to arise through a sequential mutation process, since an agammaessive malignant cell type is likely to arise only from such a sequence of mutations. A conceptual diagram of sequential mutations is illustrated in Figure S.2 where the WSCs mutate into an MSC line which then sequentially mutates further n times. Likewise, a conceptual model of parallel mutations is illustrated in Figure S.1, where WSCs mutate multiple times in parallel into distinct MSCs. Each cell after multiple mutations may be the result of the mutations arriving in any order, thus resulting in cells with identical DNA. However, the phenotype of the cells may differ causing the cells to function differently, i.e., having different RNA activities.
Note that in Figure 1, each hematopoietic cell line can either be the result of a sequential or parallel mutation. Thus a very large class of mutational landscapes is possible due to these phenomena. In this paper, we will mainly consider the two limits, either the WSCs mutate into different MSCs (parallel mutations), or the WSCs mutates into a single MSC line which initiate a sequence of mutations (sequential mutations). The last mutation scenario is considered to happen more frequently than the first, but in reality, it is expected that an intermediate scenario is the most realistic one.
In simulations we may randomly choose an existing cell to mutate and randomly choose the type of mutation in each sufficiently small time intervals. Doing a Monte Carlo simulation of such random process gives information about mean and variation but less mechanistic insight. However, exhausting simulation studies reveal that the points outlined in the present paper are robust with respect to which type of mutation we choose. Thus we mainly discuss the deterministic sequential MCC model to ease the explanatory insight gained.
The model equations arise from formulating the biological mechanisms in subsection 2.1 directly into nonlinear, ordinary, differential equations. A differential equation is formulated for each of the cell types; WSC (y0), MSC (y1, y2, ..., yn) and their mature blood cell counterparts (z0,z1,…,zn), dead cells (a) and the immune cells (s). The default parameters are listed in Table A1. Furthermore, a useful scaling of each of the variables is introduced to bring the model into dimensionless form which is a common approach for a mathematical analysis of such equations. The chosen scaling constants are listed in Table A2.
In line with the biological knowledge, the mature blood cells, immune cells and dead cells quickly equilibrate with the slower stem cell dynamics. This implies that the mature cells, dead cells and immune cells can be explicitly expressed in terms of WSC and the n MSC types, thus the model is reduced significantly from 2(n+1)+2 to n+1 equations. Note that n can can be chosen as any positive integer and the model can therefore be used for any number of mutations. Let the dimensionless cell types and time scale be denoted by Y0,Y1,…,Yn and T, respectively. The resulting dimensionless equations are (see Supplementary for derivation).
dY0dT=Φ0SY0−D0Y0+Ξ0 | (2.1a) |
dYidT=RiΦiSYi−(Di+KiYi)Yi+Ξi,i=1,...,n, | (2.1b) |
with the immune cells, S, expressed as
S=J+√J2+n∑j=02BjYj. | (2.2) |
J is a parameter describing the inflammatory stimuli not caused by the mutated cells, e.g., exogenous inflammatory stimuli. Increasing this value leads to an increased production of immune cells. Di describes the death rate of cell type i and Ki is a death rate of the second order elimination of mutant i due to activated immune clearance of the mutants by cytotoxic T-cells. Bi describes the balance between loss of cells from the stem cell pools and elimination from the dead cell pool due to immune cell clearance.
To maintain function, stem cells must interact with the stem cell niche. The stem cell niche uses negative feedback mechanisms to stabilize wild type stem cells under normal circumstances, e.g., in absence of cancer mutants. When several stem cell types are present, there is a competition for space and resources through the stem cell niche feedback (the Φ-function),
Φi=11+∑nj=0Ci,jYj, | (2.3) |
where the weighting coefficient Ci,j describes the inhibiting strength of cell type j onto cell type i for i,j∈{0,1,2,…,n}.
A way to include the fact that cancer cells are less constrained by environmental signals than hematopoietic stem cells is to enforce Φi>Φ0 for i=1,…,n, i.e., large weighting coefficients imply high niche feedback inhibition strengths. In the current work we are exploring the C-dependence on the overall dynamics of cell populations. Finally, Ri denote the self renewal rates of the mutated cells relative to the wild type.
Stochastic or continuous mutation processes between stem cell types are incorporated through Ξi. Hence, the first mutant is initiated by a wild type mutation, while secondary mutations may happen sequentially (the mutant mutates further), in parallel (a new mutation from the wild type cell pool), or as a mixture of these, where any of the existing stem cells wild type or mutated ones may mutate. The stochastic process is a scaled Poisson process but in the deterministic limit Ξi=∑nj=0(Mi,jYj−Mj,iYi) where Mi,j describes the rate that cell type j mutates into cell type i. For the parallel case the expression for Ξi reduces to Ξ0=∑nj=1−Mj,0Y0 and Ξi=Mi,0Y0 for i=1,2,…,n, while for the sequential case the expressions are given by Ξ0=−M1,0Y0 and Ξi=Mi,i−1Yi−1−Mi+1,iYi for i=1,2,…,n where Mn,n+1=0, i.e., the last mutation cannot initiate further differentiation.
By presenting the equations in dimensionless form as in (2.1), the death rates as well as the self renewal rates for WSCs become normalized to 1, i.e., D0=1 and R0=1, respectively. Likewise, the self niche feedback becomes 1, i.e., Ci,i=1. The advantage in putting the equations in dimensionless form is not only to ease the analysis, but also to make reliable simulations and promote insights. However, the results of the in silico investigations are presented using the dimensional variables (y and z) instead of the corresponding dimensionless ones (Y and Z), while the parameters will be listed in dimensionless form.
For the simulations we will investigate how clones with distinct micro-environment can give rise to complex dynamics observed in clinical studies such as resistance and oscillations. The properties of the micro-environment are modeled by the cell to cell inhibition (C) and immunoediting (K) and these are the parameters of main interest in the simulations while keeping the remaining parameters fixed at their default values listed in Table A3. In sections 3.7 and 3.1 we will also consider the effect of the self renewal rate R.
The default parameter values for the models listed in Table A3 are for the JAK2+ MPNs [20] and for each experiment, only the parameter values deviating from the default value will be reported.
At this point we emphasize that the structure of the model is independent of the specific blood cancer that a given mutation may cause. However, the parameter values depend on the specific mutations causing the cancer to develop. Thus, the model structure is general but the parameter values are mutation-specific taking all involved clones into account.
Before turning our attention to the results of the MCC model, we briefly outline the results for the scenario with one or two clones which is described by the MCC models predecessors.
For the simple case of a single clone, the dynamical behavior of the model is simple - either the cell number approaches the carrying capacity or the cell type goes extinct. The case of two competing cell types, i.e., the wild type and one mutant, has been extensively studied in [18,20] illuminating cancer elimination, escape or equilibrium depending on the parameter values. No oscillatory dynamics has been observed in the studies, only trajectories approaching steady state values. For JAK2+ MPNs the parameter values were estimated to reproduce observed cell counts, see [17,20]. Remarkably, the immune response reproduces observed average values for cohorts.
Now we will present the simulations for the MCC model which allow for the number of clones to exceed two and thereby enabling more complex dynamics than its predecessors can describe.
The first investigation is for the case with two distinct MSCs present in the bone marrow in addition to the WSC. The aim is to simulate a scenario where a less agammaessive MSC is reduced by treatment and thereby enabling a more agammaessive MSC to initiate a fatal growth, i.e. wild type cells get extinct.
The two MSCs differ in their susceptibility to the immune response and by their self-renewal rates. The second malignant cell line y2 is more agammaessive as it has a lower susceptibility to the immune response and a higher self-renewal rate. However, y2 is suppressed by the presence of y1, corresponding to a scenario where y1 could be an earlier mutation with advantageous growth conditions due to the lack of presence of additional mutations in the initial growth phase.
Figure 2a depicts the scenario where the MSCs reach a co-existing steady state with the WSCs. The malignant cells, y2 cannot initialize a fatal growth as it is sufficiently suppressed by y1. Note there is a non-fatal reduction in the WSCs which could lead to the onset of targeted treatment of y1, i.e., treatment that does only affect y1. This is exactly the scenario explored in Figure 2b, where the non-fatal reduction in y0 triggers a treatment of the virtual patient which boosts the immune response to y1 (increasing K1) at year 40. The treatment results in a reduction of the amount of y1, which initially gives an improved prognosis as the amount of WSCs (y0) increases. However, the inhibiting effect for the more agammaessive malignant cells y2 wears off as the amount of y1 reduces, and a fatal malignant growth of y2 begins.
The second simulation scenario explores the concept of resistance. At time t=0 there is a WSC line y0 and single MSC line y1. The two cell lines enter a co-existing steady state as the immune surveillance keeps the malignant cell type at a non-fatal level. Thus, a fatal growth can initiate if y1 acquires a mutation making the mutated cells y2 less susceptible to the immune response.
In Figure 3a the MSC line y1 does not gain resistance and the patient may live with a coexisting steady state for the healthy and malignant cells. However, in Figure 3b the non-fatal malignant cell type y1 gives rise to a resistant cell line y2 which initializes a fatal growth. This behavior resemble that described by Shlush et al. in 2017 [45] and discussed by others in [46,47,48] where the basic idea is that some malignant stem cells may survive treatment either due to previously developed resistance or for other reasons thus causing a fatal relapse for some AML patients. A sustained remission is seen in less than 35% of the young adults and 15% of older patients [48].
The stem cell pool can be highly heterogeneous [3] and we will investigate such a scenario where circular inhibiting effects between the MSCs give rise to oscillations and possible complications of such oscillations. At least three clones additional to the WSCs are needed to account for various observed dynamic complexities. Oscillating cell numbers could possibly be explained by having three or more malignant stem cells that inhibit each other.
For the case n=3 we could have that y1 is highly inhibited by y2 but only slightly inhibited by y3. In addition y2 is not highly affected by the presence of y1 but is highly affected by the presence of y3 and lastly y3 is highly affected by y1 but not much by y2. This specific form of inhibiting effect is illustrated in Figure 4 and the corresponding trajectories are seen in Figure 5.
Figure 6a explores a similar scenario but where the WSCs are affected differently by the three types of malignant cells, i.e., the WSCs are most inhibited by the presence of y3 and least inhibited by y1. Hence, if the virtual patient receives targeted treatment then the outcome depends on the targeted clone.
The outcome of applying treatment in Figure 6a depends severely on which of the malignant cells the intervention addresses. Targeted treatment of y1, y2 and y3 is depicted in Figure 6b-6d, respectively.
Figure 6b shows treatment targeting y1 which inhibits the WSCs the least of the existing MSCs. The suppression of y1 may unintentionally improve the growth conditions for the more agammaessive y3 and initiate a fatal growth. Figure 6c shows that treatment targeting y2 may increase the amount of WSCs as the treatment of y2 improves the growth conditions for the less agammaessive y1 which inhibits the WSCs the least. Figure 6d shows that treatment targeting y3 results in a somewhat neutral outcome.
The aim of the simulated study presented in Figure 7 is to model a scenario observed for patients treated with hydroxyurea, namely a response having a fast drop followed by a rebound with oscillations as seen in the clinic.
Initially a fatal growth of a malignant cell type y1 starts increasing the total amount of blood cells rapidly. This increase in cell counts triggers symptoms such as thrombosis and a targeted treatment for y1 is initiated. The targeted treatment successfully reduces the amount of y1 and after the treatment, y1 enters a cyclic state with the two less agammaessive malignant types y2 and y3. The treatment initiates a drop in the total amount of cells. After some time the total amount of cells increases and stabilizes with oscillations at a non-fatal level.
As mentioned, the MCC model is not mutation specific, such as JAK2, and can model the faster developing CML by changing the parameter values governing the micro-environment as an example. Observed oscillations in white blood cells for CML and platelets for a JAK2+ MPN, are depicted in Figure 8, after treatment onset with hydroxyurea. The data are from [22,23,24,25,31], respectively. The oscillations differ in period for the patients and the period of the oscillations in Figure 8 are reported to be 35-40 days, 53-69 days, 37-83 days, and 244 days for CML, respectively, while it is 27 days for MPNs.
To compare model output with data the outputs have been normalized to achieve the means and the amplitudes of the data. The linear drift seen in Figure 8b is artificial imposed on the model output to make the periods comparable. However, the deterministic model struggles to reproduce the irregularities of the measured oscillations, e.g., those in Figure 8a. For the data fits we have chosen Mi,j=0 corresponding to ignoring additional mutations during the relatively short time spans considered.
A common understanding is that an agammaessive malignant cell type arises from a sequence of mutations where the fitness of the malignant type increases with the number of mutations. We will explore both a deterministic and stochastic scenario depicted in Figures 9a and 9b, respectively. In the deterministic scenario the fitness of the malignant daughter clone increases in each mutation by an increase in the self-renewal rate and an inhibiting effect on the mother cell line. The result is a step-wise reduction in the amount of WSCs as depicted in Figure 9a.
In the stochastic scenario we have two cell lines present y0 and y1 at time t=0. During the simulation they are both allowed to mutate. Each time a mutation happens, the daughter cells will have the parameters of the mother cell but inhibiting effect (C), self-renewal rate (R) and T-cell specific death rate (K) are sampled from log-normal distributions with the parameter values of the mother cells as mean values. Hence, the daughter cells have the possibility of becoming either more agammaessive or less fit than the mother cells. The new clone will have the possibility to mutate itself and thereby enabling the simulation to have a mixture of the sequential and the parallel aspects. The outcomes of the deterministic and the stochastic approach are analogues despite the variability in the stochastic approach and they illustrate how the healthy cells are step-wise out-competed due to the clonal evolution.
A sensitivity analysis of the parameters used for Figure 5 is provided in Supplementary section S.2. The sensitivity was performed for the non-default parameter values K and C with a 10% change. Perturbing the parameter values by 10% did not change the qualitative behavior of the system, i.e., oscillations were still observed for the perturbed parameter values. However, the amplitude and frequency of the oscillations did change as a consequence of the perturbation of the parameters as illustrated in Figures S.3 and S.4 and quantified in Table 1.
parameters | C,K | C,K+10% | C,K−10% | C+10%,K | C−10%,K |
mean | 0.164 | −9% | 11% | 3% | −1% |
amplitude | 0.188 | −40% | 36% | 26% | −46% |
frequency | 0.033 Hz | 6% | −22% | −22% | 6% |
Moreover, the sensitivity analysis revealed an inverse correlation between K and C such that increasing K by 10% and lowering C by 10% has almost identical simulation outcome. These observations align with the model understanding as a decrease in K and an increase in the cells inhibitory effect will promote growth, thus giving larger oscillations whereas an increase in K and a decrease in the cells inhibitory effects will decrease the growth potential thus making the amplitude smaller.
The presented work builds on previous models by allowing for the emergence of multiple populations of wild-type stem cells to mutated stem cells, which interact with each other as well as indirectly via the stem cell niche and the innate and adaptive immune response. A simulation study is described, exploring model dynamics under a minimal number of different parameter combinations focusing on the niche feedback inhibitions and the strength of the adaptive immune response. The different in silico scenarios encompass competition between clones of haematopoietic stem cells, wild-type ones and malignant ones, including during treatment with hydroxyurea, in a heterogeneous mixture of multiple clones. Findings are related back to various clinical and experimental observations. The model predictions show agreement between model predictions and observations of white blood cell and platelet counts, JAK2 allele burden, and cytokine counts in patients with haematologic malignancies. This is in favor of the validity of the model.
The most common telling is that if a driver mutation happens then the malignant cell type increases sigmoidally while the healthy cell type decreases in concert as illustrated in Figure 2a. The healthy cell type does not need to go extinct as the two cell types may coexist and they may even coexist at a non-fatal level for the virtual patient, see Figure 2a. The healthy cell type may also coexist with a heterogeneous cancer, i.e., multiple malignant clones, as illustrated in Figure 5.
If the healthy cell line has entered such a coexisting state a treatment of one malignant cell type may leave room for the most fit of the remaining clones to increase vitally. We consider three clones, a healthy cell type and two malignant cell types, the second more agammaessive than the first and examine the result of treatment which leads to an increase in the T-cell related death rate of the malignant clone. In case of no treatment the first malignant clone may raise to a level causing complications, e.g., risk of thrombosis, but without eradicating the normal cell type while the second malignant clone persists at an insignificant level (Figure 2a). If instead the first malignant clone is suppressed by treatment, the second and more agammaessive malignant clone may bloom if it is insensitive to the treatment, thereby suppressing the healthy cells (Figure 2b). Hence, the treatment initiates a fatal development for the patient, who may had preferred to live with the risk of thrombosis.
In earlier studies, it is found that coexistence of clones being possible only for equal parameter values for the self-renewal rate of stem cells (R) [28]. We disprove this since we have shown that co-existence can occur for different values of R, as illustrated in Figure 2a. Moreover, the simulation shows that the clone with highest self-renewal rate (R) does not need to become the dominating clone if an earlier arrived clone has a sufficient suppressing niche feedback effect on the first one (high C value).
A similar outcome results if the clone becomes resistant or less sensitive to the immune surveillance over time, illustrated by Figure 3. Notice, the developed resistance or decreased sensitivity to the immune response will cause the agammaegated cell count to increase toward a fatal level, as illustrated by the Brachiosaurus shaped development shown in Figure 3. Thus, the model explains the three Es of cancer immunoediting, elimination, equilibrium, and escape phases. First cancer grows exponentially but then the immune system becomes activated and initiates a defense, leading to stagnation of the cancer burden at a plateau. After a while either some of the malignant cells become resistant to the immune response or an additional mutation occurs resulting in a less immune-sensitive clone emerge. In both cases the immuno-insensitive clone starts to grow exponentially which is interpreted as the cancer escaping the immune response [41].
Medical action to changes in levels of clones may also be subject to caution. Consider three clones in addition to the normal clone as is the common idea of CHIPs where the number of cells of each clone may oscillate as illustrated in Figure 5. Assume that the three malignant clones have the same parameter values (but a little higher self-renewal than the normal clone to suppress it slightly) except for their mutually inhibitory effects; malignant clone 2 possesses a raised inhibition of malignant clone 1, malignant clone 3 has a raised inhibition of malignant clone 2, and malignant clone 1 imposes a raised inhibition of malignant clone 3 compared to the rest of the inhibitions (circular inhibition, see conceptual diagram in Figure 4). The number of cells of each malignant clone may oscillate, as illustrated in Figure 5. If in addition, some of the malignant clones have increased inhibition onto the normal clone, then oscillations in the normal cell counts will be more pronounced.
Figure 5 shows such an example, where the third malignant clone has a slightly increased inhibition of the normal clone. Depending on the values of the parameters, the oscillations and their mean may be more or less pronounced as illustrated in Figure 6. The period of the normal clone oscillating and its amplitude comes from the dominating malignant clone period and amplitude. If the patients blood is screened for specific mutations using NGS some of the clones may have common mutations. Thus one, two, or three of the malignant clones in said example may be registered as the same mutation, e.g., the different clones may all have the JAK2 mutation and thus only differ due to apparently 'insignificant' differences. In Figure 6a the sum of the clones is shown as an example and we may imagine that all three mutations may be classified as the JAK2 mutation. In a clinical setting such measurement may be taken rarely, e.g., each third month, every sixth month, yearly or not at all. To reliably observe periodic oscillations at least 3-5 measurements during such period are necessary otherwise measurements will be considered as 'random' fluctuations.
For the three species Lotka-Volterra equation (generalized logistic growth) global stable steady states of one, two or three (co-existing) species may occur. However, periodic solutions [49,50,51] or even heteroclinic orbits may exist [52,53] and for five species chaotic solutions exist [54]. Thus, oscillation dynamic of the current model is not surprising. However, another possible explanation for the observed oscillations could be that it is due to time delay.
For simplicity consider a single-type malignant stem cell pool. From Murray [55] we have that the steady state of the delay logistic equation with delay T is locally asymptotic stable if 0≤ryT<π2 and unstable if ryT>π2. Murray proved that for ryT=2.1 (>π2) a periodic solution with period P≈4.54T exist. If the hydroxyurea induced oscillation with period P≈100 days is observed, corresponding to a time delay T≈25 days, then ry<π50≈0.064 per day holds in the case of MPNs, CML and in AML. Hence, such time delay would hardly cause oscillatory behavior.
Continuing with the complex scenario of four clones of which three are malignant and one of the malignant clones possess a slightly elevated inhibitory effect on the normal clone, we return to the treatment discussion. We consider a hypothetical treatment which reduces the self-renewal rate for one clone only, yielding enormous differences in treatment outcome, depending on which clone the treatment targets. In Figures 6b, c, and 6d the self-renewal of clone 1, 2, and 3 is reduced at year 30. If malignant clone 1 is affected by the treatment, the malignant clone 3 expands resulting in a fatal eradication of the normal clone. If the malignant clone 2 is suppressed by treatment then the malignant clone 1 increases slightly but the normal clone almost recover fully resulting in a bearable coexisting steady state. If malignant clone 3 is reduced by the treatment, the outcome is neutral as the oscillations stops but the allele burden is unchanged for the patient. This coexisting steady state does have less functional cells and relatively many malignant cells. By changing parameter values the level of cells in the steady state may be adjusted. The relatively high number of malignant cells increase the probability of getting more agammaessive mutations. For a given probability (it is a Poisson process) the numbers of such are proportional to the number of mother cells. If the number of malignant cells, for some reasons is elevated by a factor k then the number of more agammaessive additionally mutation per time increases by the same factor [56]. A reduction in the number of normal cells may cause reduced transport of oxygen and carbon dioxide by red blood cells throughout the body.
We emphasize that the model accounts for the apparently paradoxical effect of treatment, namely, how eradication of a clone may stimulate the growth of another, more fatal, one.
In clinical practice roughly half of all MPN-patients receiving treatment are characterized as good responders and the rest as poor responders when ignoring those taken off the treatment due to adverse events. [56] This phenomenon of good versus poor responders is not well understood but the multiple clone approach gives a plausible hypothesis. The multiple discoveries of visible oscillations in cell numbers over time support the hypothesis: Cyclic oscillations with period 35-40 days in leukocyte counts in CML during hydroxyurea therapy have been reported [22], similar oscillations with a period of 53-69 days were observed in platelets, neutrophils, eosinophils, basophils and reticulocytes too and continuing for a period after anti-leukemic therapy in [23]. In [24] oscillations with a period of 37-83 days were estimated, whereas [25] found oscillations with period of 244 days in platelets and white blood cells in a patient diagnosed with CML. All of these periods can be explained by the model, noticing that the time-scale results from the physiological parameters, which differ from mutation to mutation and thus from diagnosis to diagnosis, and in [29,30,31] faster oscillations with period 27 days have been observed clinically for JAK2+ MPNs. The MCC model accurately reproduces all these clinical observations as illustrated in Figure 8. Additional support for such oscillations comes from the fact that CHIPs have been observed in a number of studies [32,33,37,38].
In the clinic we observe strong fluctuations in JAK2 allele burden data and leukocyte data from MPN-patients treated with hydroxyurea. These fluctuations are typically not pronounced until after one year after treatment onset. The typical transient is a steep decay in allele burden (the fraction of malignant mature cell count) and total cell count followed by relapse toward the levels before treatment. After such transient the JAK2 allele burden may partly decay, stagnate, or even increase but in a fluctuating way. The MCC model can reproduce such observations as illustrated in Figures 6 and 7. The model illustrates the current clinical experience and knowledge that treating a blood cancer may introduce oscillatory cell counts.
The MCC model may also explain the paradigm of a mutational evolution landscape as a sequential cascade of mutations. First one not very harmful mutation (possible as a result of a sequence of mutations) capable of surviving occurs. Next, an additional mutation (or sequence of such) occurs resulting in a more competitive clone, which more or less out-competes the first clone. These mutated stem cells may mutate further resulting in more and more harmful clones. In each step the preceding clone is inhibited and the normal cell type become more and more inhibited by the resulting clone after each additional mutation as illustrated in Figure 9a, where for simplicity only the self-renewal is increased in each mutational step. In Figure 9b, a stochastic simulation of a scenario reveals the same overall outcome, namely that the WSCs are stepwise outcompeted by increasingly agammaessive mutations.
In conclusion, the MCC model contributes to a better understanding and explanation of several observations in patients with myeloid cancers, including the previously observed (before the interferon-era and treatment with tyrosine kinase inhibitors) slow oscillations in cell counts of time scale 2-3 months for some CML patients. Similarly, the MCC model may explain the increased risk of thrombosis in MPNs despite cytoreductive therapy by demonstrating fast oscillations in cell counts of time scale 1 month for some MPN patients. Importantly, in the context of "Tumor Immune Surveillance" the different phases of immunoediting, elimination, equilibrium and escape, are explained in the model as well.
The model also suggests an evolution of CHIP towards the development of overt MPNs and the development of MPNs in the biological continuum from the early cancer stages towards the advanced myelofibrosis stage and ultimately leukemic transformation. Indeed, all these scenarios, being likely driven by chronic inflammation and inflammatory cytokines with increasing genomic instability and formation of subclones over time are explained in the model. Thus, most observations are a consequence of the model independent of whether one assumes sequential, parallel, or stochastic mutations. Finally, the large class of phenomena explained by the model and its ability to reproduce clinical data serve as a strong support for the validity of the model.
As a perspective for future studies, targets for therapeutic control by combination of drugs might be added to the model and an optimal control strategy might then be proposed to qualitatively study the behavior of the population of clones, with an objective of eradication or containment.
Parameter | Value | Unit | Parameter | Value | Unit |
r0 | 8.7⋅10−4 | day−1 | dzi | 0.01 | day−1 |
ri | 1.3⋅10−3 | day−1 | c00 | 5.6⋅10−5 | − |
a0 | 1.1⋅10−5 | day−1 | c0i | 5.4⋅10−5 | − |
ai | 1.1⋅10−5 | day−1 | ci0 | 5.2⋅10−5 | − |
A0 | 3.6⋅109 | − | cii | 5.0⋅10−5 | − |
Ai | 3.6⋅109 | − | es | 2 | day−1 |
dy0 | 2⋅10−3 | day−1 | ea | 1.6⋅105 | day−1 |
dyi | 2⋅10−3 | day−1 | rs | 3⋅10−4 | day−1 |
dz0 | 0.01 | day−1 | I | 7 | day−1 |
Scaling constants | ||||
ˉs=dy0+a0r0 | ˉa=esrsˉs | ˉt=1dy0+a0 | ˉyi=1cii | ˉzi=aiAiciidzi |
Parameters | |||
R0 | 1 | Rk | 1.49 |
Di | 1.00 | Ci,i | 1 |
C0,k | 1.08 | Ck,i | 0.93 |
K0 | 0 | Kk | 0.10 |
J | 0.76 | Mi,j | 0 |
B0 | 0.06 | Bk | 0.07 |
Parameters | Initial conditions | ||
K1 | 2.00 | Y0 | 0.56 |
C12,C21 | 5.00 | Y1 | 0.50⋅10−4 |
R1 | 1.40 | Y2 | 0.50⋅10−6 |
Parameters | Initial conditions | ||
K1 | 2.00 | Y0 | 0.56 |
M21 | 1.00⋅10−4 | Y1 | 0.50⋅10−4 |
Y2 | 0.00 |
Parameters | Initial conditions | ||
C1,2,C2,3,C3,1 | 6.80 | Y0 | 0.56 |
K1, K2, K3 | 1.4 | Y1 | 2.00⋅10−4 |
Y2 | 1.00⋅10−4 | ||
Y3 | 0.50⋅10−4 |
Parameters | Initial conditions | ||
K1, K2, K3 | 1.00 | Y0 | 0.56 |
C1,2,C2,3,C3,1 | 4.80 | Y1 | 2.00⋅10−4 |
C0,1 | 0.50 | Y2 | 1.00⋅10−4 |
C0,3 | 1.50 | Y3 | 0.50⋅10−4 |
Parameters | Initial conditions | ||
K2, K3 | 1.1 | Y0 | 0.56 |
C0,1,C0,2,C0,3 | 0.53 | Y1 | 5.00⋅10−4 |
C1,2,C2,3,C3,1 | 5.8 | Y2 | 5.00⋅10−4 |
Y3 | 5.00⋅10−3 |
Parameters | Initial conditions | ||
n | 5 | Y0 | 0.56 |
Ri | 2.2 | Y1 | 7.50⋅10−3 |
Ki | 2.4 | Y2 | 1.50⋅10−3 |
C1,2,C2,3,C3,4,C4,5,C5,1 | 9.31 | Y3 | 5.00⋅10−4 |
C1,3,C2,4,C3,5,C4,1,C5,2 | 8.32 | Y4 | 5.00⋅10−4 |
C1,4,C2,5,C3,1,C4,2,C5,3 | 4.65 | Y5 | 5.00⋅10−4 |
Parameters | Initial conditions | ||
n | 4 | Y0 | 0.56 |
Ri | 2 | Y1 | 2.50⋅10−3 |
Ki | 3 | Y2 | 5.00⋅10−4 |
Ci,j | 0.73 | Y3 | 5.00⋅10−4 |
C1,2,C2,3,C3,4,C4,1 | 10.24 | ||
C1,3,C2,4,C3,1,C4,2 | 11.26 |
Parameters | Initial conditions | ||
n | 4 | Y0 | 0.56 |
Ri | 2 | Y1 | 2.50⋅10−3 |
Ki | 3.5 | Y2 | 5.00⋅10−4 |
Ci,j | 0.73 | Y3 | 5.00⋅10−4 |
C1,2,C2,3,C3,4,C4,1 | 9.31 | ||
C1,3,C2,4,C3,1,C4,2 | 9.31 |
Parameters | Initial conditions | ||
n | 3 | Y0 | 0.56 |
Ri | 1.26 | Y1 | 2.00⋅10−3 |
Ki | 1.26 | Y2 | 1.00⋅10−3 |
C0.1 | 0.29 | Y3 | 5.00⋅10−4 |
C1,2,C2,3,C3,1 | 6.31 | ||
D0 | 0.95 | ||
Ci,j | 0.73 |
Parameters | Initial conditions | ||
n | 3 | Y0 | 0.56 |
Ri | 1.26 | Y1 | 2.00⋅10−3 |
Ki | 1.26 | Y2 | 1.00⋅10−3 |
C0.1 | 0.29 | Y3 | 5.00⋅10−4 |
C1,2,C2,3,C3,1 | 5.31 | ||
D0 | 0.95 | ||
Ci,j | 0.73 |
Parameters | Initial conditions | ||||||
R1 | 1.1000 | R5 | 1.3900 | Ki | 1 | Y0 | 0.56 |
R2 | 1.1700 | R6 | 1.4600 | Ci,j for j>i | 3.2 | Yi for i>0 | 0.56 |
R3 | 1.2400 | R7 | 1.5300 | Cj,i for j>i | 1.1 | ||
R4 | 1.3100 | R8 | 1.6000 | Mi | 10−8 |
[1] | W. S. Tong, C. K. Tang, M. S. Brown, Y. Q. Xu, Example-based cosmetic transfer, in 15th Pacific Conference on Computer Graphics and Applications (PG'07), (2007), 211-218. https://doi.org/10.1109/PG.2007.31 |
[2] | S. Liu, X. Ou, R. Qian, W. Wang, X. Cao, Makeup like a superstar: deep localized makeup transfer network, preprint, arXiv: 1604.07102. |
[3] | D. Guo, T. Sim, Digital face makeup by example, in 2009 IEEE Conference on Computer Vision and Pattern Recognition, (2009), 73-79. https://doi.org/10.1109/CVPR.2009.5206833 |
[4] | L. Xu, Y. Du, Y. Zhang, An automatic framework for example-based virtual makeup, in 2013 IEEE International Conference on Image Processing, (2013), 3206-3210. https://doi.org/10.1109/ICIP.2013.6738660 |
[5] | C. Li, K. Zhou, S. Lin, Simulating makeup through physics-based manipulation of intrinsic image layers, in 2015 IEEE Conference on Computer Vision and Pattern Recognition (CVPR), (2015), 4621-4629. https://doi.org/10.1109/CVPR.2015.7299093 |
[6] | W. Xu, C. Long, R. Wang, G. Wang, DRB-GAN: A dynamic resblock generative adversarial network for artistic style transfer, in 2021 IEEE/CVF International Conference on Computer Vision (ICCV), (2021), 6383-6392. https://doi.org/10.1109/ICCV48922.2021.00632 |
[7] | H. Chen, L. Zhao, H. Zhang, Z. Wang, Z. Zuo, A. Li, et al., Diverse image style transfer via invertible cross-space mapping, in 2021 IEEE/CVF International Conference on Computer Vision (ICCV), (2021), 14860-14869. https://doi.org/10.1109/ICCV48922.2021.01461 |
[8] | Y. Hou, L. Zheng, Visualizing adapted knowledge in domain transfer, in 2021 IEEE/CVF Conference on Computer Vision and Pattern Recognition (CVPR), (2021), 13824-13833. https://doi.org/10.1109/CVPR46437.2021.01361 |
[9] | X. Zhang, Z. Cheng, X. Zhang, H. Liu, Posterior promoted GAN with distribution discrimi-nator for unsupervised image synthesis, in 2021 IEEE/CVF Conference on Computer Vision and Pattern Recognition (CVPR), (2021), 6519-6528. https://doi.org/10.1109/CVPR46437.2021.00645 |
[10] | P. Wang, Y. Li, N. Vasconcelos, Rethinking and improving the robustness of image style transfer, in 2021 IEEE/CVF Conference on Computer Vision and Pattern Recognition (CVPR), (2021), 124-133. https://doi.org/10.1109/CVPR46437.2021.00019 |
[11] | J. Y. Zhu, T. Park, P. Isola, A. A. Efros, Unpaired image-to-image translation using cycle-consistent adversarial networks, in 2017 IEEE International Conference on Computer Vision (ICCV), (2017), 2223-2232. https://doi.org/10.1109/ICCV.2017.244 |
[12] | H. Chang, J. Lu, F. Yu, A. Finkelstein, Pairedcyclegan: Asymmetric style transfer for applying and removing makeup, in 2018 IEEE/CVF Conference on Computer Vision and Pattern Recognition, (2018), 40-48. https://doi.org/10.1109/CVPR.2018.00012 |
[13] | H. J. Chen, K. M. Hui, S. Y. Wang, L. W. Tsao, H. H. Shuai, W. H. Cheng, Beautyglow: On-demand makeup transfer framework with reversible generative network, in 2019 IEEE/CVF Conference on Computer Vision and Pattern Recognition (CVPR), (2019), 10042-10050. https://doi.org/10.1109/CVPR.2019.01028 |
[14] | T. Li, R. Qian, C. Dong, S. Liu, Q. Yan, W. Zhu, et al., Beautygan: Instance-level facial makeup transfer with deep generative adversarial network, in Proceedings of the 26th ACM international conference on Multimedia, (2018), 645-653. https://doi.org/10.1145/3240508.3240618 |
[15] | T. Nguyen, A. T. Tran, M. Hoai, Lipstick ain't enough: beyond color matching for in-the-wild makeup transfer, in 2021 IEEE/CVF Conference on Computer Vision and Pattern Recognition (CVPR), (2021), 13305-13314. https://doi.org/10.1109/cvpr46437.2021.01310 |
[16] | Z. Sun, Y. Chen, S. Xiong, SSAT: A symmetric semantic-aware transformer network for makeup transfer and removal, in Proceedings of the AAAI Conference on Artificial Intelligence, 36 (2022), 2325-2334. https://doi.org/10.1609/aaai.v36i2.20131 |
[17] | Z. Huang, Z. Zheng, C. Yan, H. Xie, Y. Sun, J. Wang, et al., Real-world automatic makeup via identity preservation makeup net, in International Joint Conferences on Artificial Intelligence Organization, (2020), 652-658. https://doi.org/10.24963/ijcai.2020/91 |
[18] | Z. Wan, H. Chen, J. An, W. Jiang, C. Yao, J. Luo, et al., Facial attribute transformers for precise and robust makeup transfer, in 2022 IEEE/CVF Winter Conference on Applications of Computer Vision (WACV), (2022), 1717-1726. https://doi.org/10.1109/wacv51458.2022.00317 |
[19] | J. Lee, E. Kim, Y. Lee, D. Kim, J. Chang, J. Choo, Reference-based sketch image colorization using augmented-self reference and dense semantic correspondence, in 2020 IEEE/CVF Conference on Computer Vision and Pattern Recognition (CVPR), (2020), 5800–5809. https://doi.org/10.1109/cvpr42600.2020.00584 |
[20] | W. Jiang, S. Liu, C. Gao, J. Cao, R. He, J. Feng, et al., Psgan: Pose and expression robust spatial-aware gan for customizable makeup transfer, in 2020 IEEE/CVF Conference on Computer Vision and Pattern Recognition (CVPR), (2020), 5194–5202. https://doi.org/10.1109/CVPR42600.2020.00524 |
[21] | A. Vaswani, N. Shazeer, N. Parmar, J. Uszkoreit, L. Jones, A. N. Gomez, et al., Attention is all you need, in Advances in Neural Information Processing Systems, 30 (2017), 5998-6008. |
[22] | H. Deng, C. Han, H. Cai, G. Han, S. He, Spatially-invariant style-codes controlled makeup transfer, in 2021 IEEE/CVF Conference on Computer Vision and Pattern Recognition (CVPR), (2021), 6549-6557. https://doi.org/10.1109/CVPR46437.2021.00648 |
[23] |
K. Zhang, Z. Zhang, Z. Li, Y. Qiao, Joint face detection and alignment using multitask cascaded convolutional networks, IEEE Signal Process Lett., 23 (2016), 1499-1503. https://doi.org/10.1109/LSP.2016.2603342 doi: 10.1109/LSP.2016.2603342
![]() |
[24] | Y. Wang, J. M. Solomon, Prnet: Self-supervised learning for partial-to-partial registration, in 33rd Conference on Neural Information Processing Systems (NeurIPS 2019), 32 (2019), 8812-8824. Available from: https://proceedings.neurips.cc/paper/2019/file/ebad33b3c9fa1d10327bb55f9e79e2f3-Paper.pdf. |
[25] | K. Simonyan, A. Zisserman, Very deep convolutional networks for large-scale image recognition, preprint, arXiv: 1409.1556. |
[26] | D. P. Kingma, P. Dhariwal, Glow: Generative flow with invertible 1x1 convolutions, in 32nd Conference on Neural Information Processing Systems (NeurIPS 2018), 31 (2018), 10236-10245. Available from: https://proceedings.neurips.cc/paper/2018/file/d139db6a236200b21cc7f752979132d0-Paper.pdf. |
[27] | K. He, X. Zhang, S. Ren, J. Sun, Deep residual learning for image recognition, in 2016 IEEE Conference on Computer Vision and Pattern Recognition (CVPR), (2016), 770-778. https://doi.org/10.1109/CVPR.2016.90 |
[28] | C. Yu, J. Wang, C. Peng, C. Gao, G. Yu, N. Sang, Bisenet: Bilateral segmentation network for real-time semantic segmentation, in Computer Vision – ECCV 2018, 11217 (2018), 334-349. https://doi.org/10.1007/978-3-030-01261-8_20 |
[29] | T. Karras, S. Laine, T. Aila, A style-based generator architecture for generative adversarial networks, in 2019 IEEE/CVF Conference on Computer Vision and Pattern Recognition (CVPR), (2019), 4401-4410. https://doi.org/10.1109/CVPR.2019.00453 |
[30] | X. Huang, S. Belongie, Arbitrary style transfer in real-time with adaptive instance normalization, in 2017 IEEE International Conference on Computer Vision (ICCV), (2017), 1501-1510. https://doi.org/10.1109/ICCV.2017.167 |
[31] | I. Goodfellow, J. Pouget-Abadie, M. Mirza, B. Xu, D. Warde-Farley, S. Ozair, et al., Generative adversarial nets, in Proceedings of the 27th International Conference on Neural Information Processing Systems, 2 (2014), 2672–2680. https://dl.acm.org/doi/10.5555/2969033.2969125 |
[32] | J. Johnson, A. Alahi, L. Fei-Fei, Perceptual losses for real-time style transfer and super-resolution, in Computer Vision – ECCV 2016, 9906 (2016), 694-711. https://doi.org/10.1007/978-3-319-46475-6_43 |
[33] | D. P. Kingma, J. Ba, Adam: a method for stochastic optimization, preprint, arXiv: 1412.6980. |
[34] | Y. Lyu, J. Dong, B. Peng, W. Wang, T. Tan, SOGAN: 3D-aware shadow and occlusion robust GAN for makeup transfer, in Proceedings of the 29th ACM International Conference on Multimedia, (2021), 3601-3609. https://doi.org/10.1145/3474085.3475531 |
[35] | J. Liao, Y. Yao, L. Yuan, G. Hua, S. B. Kang, Visual attribute transfer through deep image analogy, preprint, arXiv: 1705.01088. |
[36] | Q. Gu, G. Wang, M. T. Chiu, Y. W. Tai, C. K. Tang, Ladn: Local adversarial disentangling network for facial makeup and de-makeup, in 2019 IEEE/CVF International Conference on Computer Vision (ICCV), (2019), 10481-10490. https://doi.org/10.1109/ICCV.2019.01058 |
[37] | B. Yan, Q. Lin, W. Tan, S. Zhou, Assessing eye aesthetics for automatic multi-reference eye in-painting, in 2020 IEEE/CVF Conference on Computer Vision and Pattern Recognition (CVPR), (2020), 13509-13517. https://doi.org/10.1109/CVPR42600.2020.01352 |
1. | E Safitri, T Tarmizi, M Ramli, S Wahyuni, M Ikhwan, Model of virus therapy and chemotherapy for cancer, 2019, 364, 1755-1315, 012029, 10.1088/1755-1315/364/1/012029 | |
2. | Lili He, Anran Yu, Li Deng, Hongwei Zhang, Eradicating the Roots: Advanced Therapeutic Approaches Targeting Breast Cancer Stem Cells, 2020, 26, 13816128, 2009, 10.2174/1381612826666200317132949 | |
3. | A.M. Elaiw, A.D. Hobiny, A.D. Al Agha, Global dynamics of reaction-diffusion oncolytic M1 virotherapy with immune response, 2020, 367, 00963003, 124758, 10.1016/j.amc.2019.124758 | |
4. | A. M. Elaiw, A. D. Al Agha, A reaction–diffusion model for oncolytic M1 virotherapy with distributed delays, 2020, 135, 2190-5444, 10.1140/epjp/s13360-020-00188-z | |
5. | Regina Padmanabhan, Nader Meskin, Ala-Eddin Al Moustafa, 2021, Chapter 10, 978-981-15-8639-2, 215, 10.1007/978-981-15-8640-8_10 | |
6. | Joseph Malinzi, Kevin Bosire Basita, Sara Padidar, Henry Ademola Adeola, Prospect for application of mathematical models in combination cancer treatments, 2021, 23, 23529148, 100534, 10.1016/j.imu.2021.100534 | |
7. | Angelica P. Aspirin, Aurelio A. de los Reyes V, Yangjin Kim, Polytherapeutic strategies with oncolytic virus–bortezomib and adjuvant NK cells in cancer treatment, 2021, 18, 1742-5689, 20200669, 10.1098/rsif.2020.0669 | |
8. | Joseph Malinzi, Mathematical Analysis of a Mathematical Model of Chemovirotherapy: Effect of Drug Infusion Method, 2019, 2019, 1748-670X, 1, 10.1155/2019/7576591 | |
9. | A.M. Elaiw, A.D. Al Agha, Analysis of a delayed and diffusive oncolytic M1 virotherapy model with immune response, 2020, 55, 14681218, 103116, 10.1016/j.nonrwa.2020.103116 | |
10. | Cristiana J. Silva, Carla Cruz, Delfim F. M. Torres, Alberto P. Muñuzuri, Alejandro Carballosa, Iván Area, Juan J. Nieto, Rui Fonseca-Pinto, Rui Passadouro, Estevão Soares dos Santos, Wilson Abreu, Jorge Mira, Optimal control of the COVID-19 pandemic: controlled sanitary deconfinement in Portugal, 2021, 11, 2045-2322, 10.1038/s41598-021-83075-6 | |
11. | Carlos Campos, Cristiana J. Silva, Delfim F. M. Torres, Numerical Optimal Control of HIV Transmission in Octave/MATLAB, 2019, 25, 2297-8747, 1, 10.3390/mca25010001 | |
12. | Hanwen Wang, Oleg Milberg, Imke H. Bartelink, Paolo Vicini, Bing Wang, Rajesh Narwal, Lorin Roskos, Cesar A. Santa-Maria, Aleksander S. Popel, In silico simulation of a clinical trial with anti-CTLA-4 and anti-PD-L1 immunotherapies in metastatic breast cancer using a systems pharmacology model , 2019, 6, 2054-5703, 190366, 10.1098/rsos.190366 | |
13. | F. Adi-Kusumo, L. Aryati, S. Risdayati, S. Norhidayah, Hopf Bifurcation on a Cancer Therapy Model by Oncolytic Virus Involving the Malignancy Effect and Therapeutic Efficacy, 2020, 2020, 0161-1712, 1, 10.1155/2020/4730715 | |
14. | Johannes P. W. Heidbuechel, Daniel Abate-Daga, Christine E. Engeland, Heiko Enderling, 2020, Chapter 21, 978-1-4939-9793-0, 307, 10.1007/978-1-4939-9794-7_21 | |
15. | Jing Li, Yifu Wang, Boundedness in a haptotactic cross-diffusion system modeling oncolytic virotherapy, 2021, 270, 00220396, 94, 10.1016/j.jde.2020.07.032 | |
16. | Said Seif Salim, Eunice Mureithi, Nyimvua Shaban, Joseph Malinzi, Mathematical modelling of the dynamics of prostate cancer with a curative vaccine, 2021, 11, 24682276, e00715, 10.1016/j.sciaf.2021.e00715 | |
17. | Mobina Mobaraki, Hamed Moradi, Design of robust control strategy in drug and virus scheduling in nonlinear process of chemovirotherapy, 2021, 150, 00981354, 107318, 10.1016/j.compchemeng.2021.107318 | |
18. | Majda El Younoussi, Zakaria Hajhouji, Khalid Hattaf, Noura Yousfi, Dynamics of a reaction-diffusion fractional-order model for M1 oncolytic virotherapy with CTL immune response, 2022, 157, 09600779, 111957, 10.1016/j.chaos.2022.111957 | |
19. | H. Lefraich, 2022, Chapter 16, 978-3-031-12514-0, 287, 10.1007/978-3-031-12515-7_16 | |
20. | Ana Costa, Nuno Vale, Strategies for the treatment of breast cancer: from classical drugs to mathematical models, 2021, 18, 1551-0018, 6328, 10.3934/mbe.2021316 | |
21. | Engida Endriyas Endashaw, Dawit Melese Gebru, Haileyesus Tessema Alemneh, Khalid Hattaf, Coinfection Dynamics of HBV-HIV/AIDS with Mother-to-Child Transmission and Medical Interventions, 2022, 2022, 1748-6718, 1, 10.1155/2022/4563577 | |
22. | Zizi Wang, Qian Zhang, Yong Luo, A general non-local delay model on oncolytic virus therapy, 2022, 102, 0307904X, 423, 10.1016/j.apm.2021.09.045 | |
23. | Aurelio A. de los Reyes, Yangjin Kim, Optimal regulation of tumour-associated neutrophils in cancer progression, 2022, 9, 2054-5703, 10.1098/rsos.210705 | |
24. | Jinqiong Jiang, Weida Wang, Weineng Xiang, Lin Jiang, Qian Zhou, The phosphoinositide 3-kinase inhibitor ZSTK474 increases the susceptibility of osteosarcoma cells to oncolytic vesicular stomatitis virus VSVΔ51 via aggravating endoplasmic reticulum stress, 2021, 12, 2165-5979, 11847, 10.1080/21655979.2021.1999372 | |
25. | Khaphetsi Joseph Mahasa, Rachid Ouifki, Amina Eladdadi, Lisette de Pillis, A combination therapy of oncolytic viruses and chimeric antigen receptor T cells: a mathematical model proof-of-concept, 2022, 19, 1551-0018, 4429, 10.3934/mbe.2022205 | |
26. | Anusmita Das, Hemanta Kr. Sarmah, Debashish Bhattacharya, Kaushik Dehingia, Kamyar Hosseini, Combination of virotherapy and chemotherapy with optimal control for combating cancer, 2022, 194, 03784754, 460, 10.1016/j.matcom.2021.12.004 | |
27. | Qian Zhao, Bin Liu, Dampening effects on global boundedness in a quartic haptotactic model with fusogenic oncolytic virus and syncytia, 2022, 73, 0044-2275, 10.1007/s00033-022-01882-x | |
28. | Anusmita Das, Kaushik Dehingia, Hemanta Kr Sarmah, Kamyar Hosseini, An optimally controlled chemotherapy treatment for cancer eradication, 2022, 0228-6203, 1, 10.1080/02286203.2022.2155601 | |
29. | Anusmita Das, Kaushik Dehingia, Hemanta Kumar Sharmah, Choonkil Park, Jung Rye Lee, Khadijeh Sadri, Kamyar Hosseini, Soheil Salahshour, Optimal control of effector-tumor-normal cells dynamics in presence of adoptive immunotherapy, 2021, 6, 2473-6988, 9813, 10.3934/math.2021570 | |
30. | Engida Endriyas Endashaw, Temesgen Tibebu Mekonnen, Frank Werner, Modeling the Effect of Vaccination and Treatment on the Transmission Dynamics of Hepatitis B Virus and HIV/AIDS Coinfection, 2022, 2022, 1687-0042, 1, 10.1155/2022/5246762 | |
31. | A. Mhlanga, T. V. Mupedza, A patchy theoretical model for the transmission dynamics of SARS-Cov-2 with optimal control, 2022, 12, 2045-2322, 10.1038/s41598-022-21553-1 | |
32. | Qian Li, Yanni Xiao, Modeling the virus-induced tumor-specific immune response with delay in tumor virotherapy, 2022, 108, 10075704, 106196, 10.1016/j.cnsns.2021.106196 | |
33. | Fazal Subhan, Muhammad Adnan Aziz, Inam Ullah Khan, Muhammad Fayaz, Marcin Wozniak, Jana Shafi, Muhammad Fazal Ijaz, Cancerous Tumor Controlled Treatment Using Search Heuristic (GA)-Based Sliding Mode and Synergetic Controller, 2022, 14, 2072-6694, 4191, 10.3390/cancers14174191 | |
34. | Adedapo Chris Loyinmi, Timilehin Kingsley Akinfe, Abiodun Abisoye Ojo, Qualitative analysis and dynamical behavior of a Lassa haemorrhagic fever model with exposed rodents and saturated incidence rate, 2021, 14, 24682276, e01028, 10.1016/j.sciaf.2021.e01028 | |
35. | G. V. R. K. Vithanage, Sophia R-J Jang, Optimal Immunotherapy of Oncolytic Viruses and Adopted Cell Transfer in Cancer Treatment, 2022, 19, 2224-2902, 140, 10.37394/23208.2022.19.15 | |
36. | Raqqasyi Rahmatullah Musafir, Nur Shofianah, 2022, 2501, 0094-243X, 020019, 10.1063/5.0082986 | |
37. | Javaria Iqbal, Salman Ahmad, Muhammad Marwan, Ayesha Rafiq, Control analysis of virotherapy chaotic system, 2022, 16, 1751-3758, 585, 10.1080/17513758.2022.2104391 | |
38. | M. Moksud Alam, S.M.E.K. Chowdhury, J.T. Chowdhury, Mohammad Mahmud Hasan, M.A. Ullah, Shams Forruque Ahmed, Tumor treatment with chemo-virotherapy and MEK inhibitor: A mathematical model of Caputo fractional differential operator, 2023, 71, 11100168, 173, 10.1016/j.aej.2023.03.010 | |
39. | Said Seif Salim, Joseph Malinzi, Eunice Mureithi, Nyimvua Shaban, Mathematical modelling of chemovirotherapy cancer treatment, 2023, 0228-6203, 1, 10.1080/02286203.2023.2204355 | |
40. | Joseph Malinzi, Victor Ogesa Juma, Chinwendu Emilian Madubueze, John Mwaonanji, Godwin Nwachukwu Nkem, Elias Mwakilama, Tinashe Victor Mupedza, Vincent Nandwa Chiteri, Emmanuel Afolabi Bakare, Isabel Linda-Zulu Moyo, Eduard Campillo-Funollet, Farai Nyabadza, Anotida Madzvamuse, COVID-19 transmission dynamics and the impact of vaccination: modelling, analysis and simulations, 2023, 10, 2054-5703, 10.1098/rsos.221656 | |
41. | Tedi Ramaj, Xingfu Zou, On the treatment of melanoma: A mathematical model of oncolytic virotherapy, 2023, 365, 00255564, 109073, 10.1016/j.mbs.2023.109073 | |
42. | Donggu Lee, Aurelio A. de los Reyes V, Yangjin Kim, Optimal strategies of oncolytic virus-bortezomib therapy via the apoptotic, necroptotic, and oncolysis signaling network, 2024, 21, 1551-0018, 3876, 10.3934/mbe.2024173 | |
43. | Mohammad Amini, Maryam Niroomandfard, Pariya Khalili, Ramin Vatankhah, 2023, The Improvement of Chemovirotherapy Effectiveness Utilizing Hybrid GA-PSO, 979-8-3503-5973-2, 205, 10.1109/ICBME61513.2023.10488649 | |
44. | Salaheldin Omer, Hermane Mambili-Mamboundou, Assessing the impact of immunotherapy on oncolytic virotherapy in the treatment of cancer, 2024, 1598-5865, 10.1007/s12190-024-02139-8 | |
45. | Sabrina Glaschke, Hana M. Dobrovolny, Spatiotemporal spread of oncolytic virus in a heterogeneous cell population, 2024, 183, 00104825, 109235, 10.1016/j.compbiomed.2024.109235 | |
46. | Taeyong Lee, Hee-Dae Kwon, Jeehyun Lee, Constrained optimal control problem of oncolytic viruses in cancer treatment, 2024, 03784754, 10.1016/j.matcom.2024.10.019 | |
47. | Zizi Wang, Modeling oncolytic virus therapy with distributed delay and nonlocal diffusion, 2024, 2024, 2731-4235, 10.1186/s13662-024-03859-8 | |
48. | Sunil S. Kumbhar, Sarita Thakar, Galerkin finite element method for oncolytic M1 cancer virotherapy reaction–diffusion model, 2025, 18, 1793-5245, 10.1142/S1793524523500870 | |
49. | G. V. R. K. Vithanage, Sophia R-J Jang, Optimal Immunotherapy Interactions in Oncolytic Viral Therapy and Adopted Cell Transfer for Cancer Treatment with Saturation Effects, 2025, 22, 2224-2902, 223, 10.37394/23208.2025.22.23 |
parameters | C,K | C,K+10% | C,K−10% | C+10%,K | C−10%,K |
mean | 0.164 | −9% | 11% | 3% | −1% |
amplitude | 0.188 | −40% | 36% | 26% | −46% |
frequency | 0.033 Hz | 6% | −22% | −22% | 6% |
Parameter | Value | Unit | Parameter | Value | Unit |
r0 | 8.7⋅10−4 | day−1 | dzi | 0.01 | day−1 |
ri | 1.3⋅10−3 | day−1 | c00 | 5.6⋅10−5 | − |
a0 | 1.1⋅10−5 | day−1 | c0i | 5.4⋅10−5 | − |
ai | 1.1⋅10−5 | day−1 | ci0 | 5.2⋅10−5 | − |
A0 | 3.6⋅109 | − | cii | 5.0⋅10−5 | − |
Ai | 3.6⋅109 | − | es | 2 | day−1 |
dy0 | 2⋅10−3 | day−1 | ea | 1.6⋅105 | day−1 |
dyi | 2⋅10−3 | day−1 | rs | 3⋅10−4 | day−1 |
dz0 | 0.01 | day−1 | I | 7 | day−1 |
Scaling constants | ||||
ˉs=dy0+a0r0 | ˉa=esrsˉs | ˉt=1dy0+a0 | ˉyi=1cii | ˉzi=aiAiciidzi |
Parameters | |||
R0 | 1 | Rk | 1.49 |
Di | 1.00 | Ci,i | 1 |
C0,k | 1.08 | Ck,i | 0.93 |
K0 | 0 | Kk | 0.10 |
J | 0.76 | Mi,j | 0 |
B0 | 0.06 | Bk | 0.07 |
Parameters | Initial conditions | ||
K1 | 2.00 | Y0 | 0.56 |
C12,C21 | 5.00 | Y1 | 0.50⋅10−4 |
R1 | 1.40 | Y2 | 0.50⋅10−6 |
Parameters | Initial conditions | ||
K1 | 2.00 | Y0 | 0.56 |
M21 | 1.00⋅10−4 | Y1 | 0.50⋅10−4 |
Y2 | 0.00 |
Parameters | Initial conditions | ||
C1,2,C2,3,C3,1 | 6.80 | Y0 | 0.56 |
K1, K2, K3 | 1.4 | Y1 | 2.00⋅10−4 |
Y2 | 1.00⋅10−4 | ||
Y3 | 0.50⋅10−4 |
Parameters | Initial conditions | ||
K1, K2, K3 | 1.00 | Y0 | 0.56 |
C1,2,C2,3,C3,1 | 4.80 | Y1 | 2.00⋅10−4 |
C0,1 | 0.50 | Y2 | 1.00⋅10−4 |
C0,3 | 1.50 | Y3 | 0.50⋅10−4 |
Parameters | Initial conditions | ||
K2, K3 | 1.1 | Y0 | 0.56 |
C0,1,C0,2,C0,3 | 0.53 | Y1 | 5.00⋅10−4 |
C1,2,C2,3,C3,1 | 5.8 | Y2 | 5.00⋅10−4 |
Y3 | 5.00⋅10−3 |
Parameters | Initial conditions | ||
n | 5 | Y0 | 0.56 |
Ri | 2.2 | Y1 | 7.50⋅10−3 |
Ki | 2.4 | Y2 | 1.50⋅10−3 |
C1,2,C2,3,C3,4,C4,5,C5,1 | 9.31 | Y3 | 5.00⋅10−4 |
C1,3,C2,4,C3,5,C4,1,C5,2 | 8.32 | Y4 | 5.00⋅10−4 |
C1,4,C2,5,C3,1,C4,2,C5,3 | 4.65 | Y5 | 5.00⋅10−4 |
Parameters | Initial conditions | ||
n | 4 | Y0 | 0.56 |
Ri | 2 | Y1 | 2.50⋅10−3 |
Ki | 3 | Y2 | 5.00⋅10−4 |
Ci,j | 0.73 | Y3 | 5.00⋅10−4 |
C1,2,C2,3,C3,4,C4,1 | 10.24 | ||
C1,3,C2,4,C3,1,C4,2 | 11.26 |
Parameters | Initial conditions | ||
n | 4 | Y0 | 0.56 |
Ri | 2 | Y1 | 2.50⋅10−3 |
Ki | 3.5 | Y2 | 5.00⋅10−4 |
Ci,j | 0.73 | Y3 | 5.00⋅10−4 |
C1,2,C2,3,C3,4,C4,1 | 9.31 | ||
C1,3,C2,4,C3,1,C4,2 | 9.31 |
Parameters | Initial conditions | ||
n | 3 | Y0 | 0.56 |
Ri | 1.26 | Y1 | 2.00⋅10−3 |
Ki | 1.26 | Y2 | 1.00⋅10−3 |
C0.1 | 0.29 | Y3 | 5.00⋅10−4 |
C1,2,C2,3,C3,1 | 6.31 | ||
D0 | 0.95 | ||
Ci,j | 0.73 |
Parameters | Initial conditions | ||
n | 3 | Y0 | 0.56 |
Ri | 1.26 | Y1 | 2.00⋅10−3 |
Ki | 1.26 | Y2 | 1.00⋅10−3 |
C0.1 | 0.29 | Y3 | 5.00⋅10−4 |
C1,2,C2,3,C3,1 | 5.31 | ||
D0 | 0.95 | ||
Ci,j | 0.73 |
Parameters | Initial conditions | ||||||
R1 | 1.1000 | R5 | 1.3900 | Ki | 1 | Y0 | 0.56 |
R2 | 1.1700 | R6 | 1.4600 | Ci,j for j>i | 3.2 | Yi for i>0 | 0.56 |
R3 | 1.2400 | R7 | 1.5300 | Cj,i for j>i | 1.1 | ||
R4 | 1.3100 | R8 | 1.6000 | Mi | 10−8 |
parameters | C,K | C,K+10% | C,K−10% | C+10%,K | C−10%,K |
mean | 0.164 | −9% | 11% | 3% | −1% |
amplitude | 0.188 | −40% | 36% | 26% | −46% |
frequency | 0.033 Hz | 6% | −22% | −22% | 6% |
Parameter | Value | Unit | Parameter | Value | Unit |
r0 | 8.7⋅10−4 | day−1 | dzi | 0.01 | day−1 |
ri | 1.3⋅10−3 | day−1 | c00 | 5.6⋅10−5 | − |
a0 | 1.1⋅10−5 | day−1 | c0i | 5.4⋅10−5 | − |
ai | 1.1⋅10−5 | day−1 | ci0 | 5.2⋅10−5 | − |
A0 | 3.6⋅109 | − | cii | 5.0⋅10−5 | − |
Ai | 3.6⋅109 | − | es | 2 | day−1 |
dy0 | 2⋅10−3 | day−1 | ea | 1.6⋅105 | day−1 |
dyi | 2⋅10−3 | day−1 | rs | 3⋅10−4 | day−1 |
dz0 | 0.01 | day−1 | I | 7 | day−1 |
Scaling constants | ||||
ˉs=dy0+a0r0 | ˉa=esrsˉs | ˉt=1dy0+a0 | ˉyi=1cii | ˉzi=aiAiciidzi |
Parameters | |||
R0 | 1 | Rk | 1.49 |
Di | 1.00 | Ci,i | 1 |
C0,k | 1.08 | Ck,i | 0.93 |
K0 | 0 | Kk | 0.10 |
J | 0.76 | Mi,j | 0 |
B0 | 0.06 | Bk | 0.07 |
Parameters | Initial conditions | ||
K1 | 2.00 | Y0 | 0.56 |
C12,C21 | 5.00 | Y1 | 0.50⋅10−4 |
R1 | 1.40 | Y2 | 0.50⋅10−6 |
Parameters | Initial conditions | ||
K1 | 2.00 | Y0 | 0.56 |
M21 | 1.00⋅10−4 | Y1 | 0.50⋅10−4 |
Y2 | 0.00 |
Parameters | Initial conditions | ||
C1,2,C2,3,C3,1 | 6.80 | Y0 | 0.56 |
K1, K2, K3 | 1.4 | Y1 | 2.00⋅10−4 |
Y2 | 1.00⋅10−4 | ||
Y3 | 0.50⋅10−4 |
Parameters | Initial conditions | ||
K1, K2, K3 | 1.00 | Y0 | 0.56 |
C1,2,C2,3,C3,1 | 4.80 | Y1 | 2.00⋅10−4 |
C0,1 | 0.50 | Y2 | 1.00⋅10−4 |
C0,3 | 1.50 | Y3 | 0.50⋅10−4 |
Parameters | Initial conditions | ||
K2, K3 | 1.1 | Y0 | 0.56 |
C0,1,C0,2,C0,3 | 0.53 | Y1 | 5.00⋅10−4 |
C1,2,C2,3,C3,1 | 5.8 | Y2 | 5.00⋅10−4 |
Y3 | 5.00⋅10−3 |
Parameters | Initial conditions | ||
n | 5 | Y0 | 0.56 |
Ri | 2.2 | Y1 | 7.50⋅10−3 |
Ki | 2.4 | Y2 | 1.50⋅10−3 |
C1,2,C2,3,C3,4,C4,5,C5,1 | 9.31 | Y3 | 5.00⋅10−4 |
C1,3,C2,4,C3,5,C4,1,C5,2 | 8.32 | Y4 | 5.00⋅10−4 |
C1,4,C2,5,C3,1,C4,2,C5,3 | 4.65 | Y5 | 5.00⋅10−4 |
Parameters | Initial conditions | ||
n | 4 | Y0 | 0.56 |
Ri | 2 | Y1 | 2.50⋅10−3 |
Ki | 3 | Y2 | 5.00⋅10−4 |
Ci,j | 0.73 | Y3 | 5.00⋅10−4 |
C1,2,C2,3,C3,4,C4,1 | 10.24 | ||
C1,3,C2,4,C3,1,C4,2 | 11.26 |
Parameters | Initial conditions | ||
n | 4 | Y0 | 0.56 |
Ri | 2 | Y1 | 2.50⋅10−3 |
Ki | 3.5 | Y2 | 5.00⋅10−4 |
Ci,j | 0.73 | Y3 | 5.00⋅10−4 |
C1,2,C2,3,C3,4,C4,1 | 9.31 | ||
C1,3,C2,4,C3,1,C4,2 | 9.31 |
Parameters | Initial conditions | ||
n | 3 | Y0 | 0.56 |
Ri | 1.26 | Y1 | 2.00⋅10−3 |
Ki | 1.26 | Y2 | 1.00⋅10−3 |
C0.1 | 0.29 | Y3 | 5.00⋅10−4 |
C1,2,C2,3,C3,1 | 6.31 | ||
D0 | 0.95 | ||
Ci,j | 0.73 |
Parameters | Initial conditions | ||
n | 3 | Y0 | 0.56 |
Ri | 1.26 | Y1 | 2.00⋅10−3 |
Ki | 1.26 | Y2 | 1.00⋅10−3 |
C0.1 | 0.29 | Y3 | 5.00⋅10−4 |
C1,2,C2,3,C3,1 | 5.31 | ||
D0 | 0.95 | ||
Ci,j | 0.73 |
Parameters | Initial conditions | ||||||
R1 | 1.1000 | R5 | 1.3900 | Ki | 1 | Y0 | 0.56 |
R2 | 1.1700 | R6 | 1.4600 | Ci,j for j>i | 3.2 | Yi for i>0 | 0.56 |
R3 | 1.2400 | R7 | 1.5300 | Cj,i for j>i | 1.1 | ||
R4 | 1.3100 | R8 | 1.6000 | Mi | 10−8 |