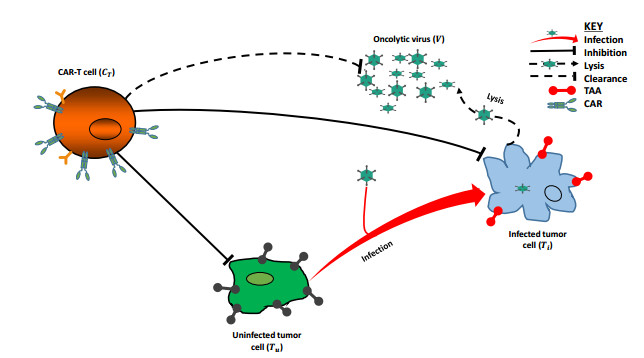
Combining chimeric antigen receptor T (CAR-T) cells with oncolytic viruses (OVs) has recently emerged as a promising treatment approach in preclinical studies that aim to alleviate some of the barriers faced by CAR-T cell therapy. In this study, we address by means of mathematical modeling the main question of whether a single dose or multiple sequential doses of CAR-T cells during the OVs therapy can have a synergetic effect on tumor reduction. To that end, we propose an ordinary differential equations-based model with virus-induced synergism to investigate potential effects of different regimes that could result in efficacious combination therapy against tumor cell populations. Model simulations show that, while the treatment with a single dose of CAR-T cells is inadequate to eliminate all tumor cells, combining the same dose with a single dose of OVs can successfully eliminate the tumor in the absence of virus-induced synergism. However, in the presence of virus-induced synergism, the same combination therapy fails to eliminate the tumor. Furthermore, it is shown that if the intensity of virus-induced synergy and/or virus oncolytic potency is high, then the induced CAR-T cell response can inhibit virus oncolysis. Additionally, the simulations show a more robust synergistic effect on tumor cell reduction when OVs and CAR-T cells are administered simultaneously compared to the combination treatment where CAR-T cells are administered first or after OV injection. Our findings suggest that the combination therapy of CAR-T cells and OVs seems unlikely to be effective if the virus-induced synergistic effects are included when genetically engineering oncolytic viral vectors.
Citation: Khaphetsi Joseph Mahasa, Rachid Ouifki, Amina Eladdadi, Lisette de Pillis. A combination therapy of oncolytic viruses and chimeric antigen receptor T cells: a mathematical model proof-of-concept[J]. Mathematical Biosciences and Engineering, 2022, 19(5): 4429-4457. doi: 10.3934/mbe.2022205
[1] | Prathibha Ambegoda, Hsiu-Chuan Wei, Sophia R-J Jang . The role of immune cells in resistance to oncolytic viral therapy. Mathematical Biosciences and Engineering, 2024, 21(5): 5900-5946. doi: 10.3934/mbe.2024261 |
[2] | Peter S. Kim, Joseph J. Crivelli, Il-Kyu Choi, Chae-Ok Yun, Joanna R. Wares . Quantitative impact of immunomodulation versus oncolysis with cytokine-expressing virus therapeutics. Mathematical Biosciences and Engineering, 2015, 12(4): 841-858. doi: 10.3934/mbe.2015.12.841 |
[3] | Donggu Lee, Aurelio A. de los Reyes V, Yangjin Kim . Optimal strategies of oncolytic virus-bortezomib therapy via the apoptotic, necroptotic, and oncolysis signaling network. Mathematical Biosciences and Engineering, 2024, 21(3): 3876-3909. doi: 10.3934/mbe.2024173 |
[4] | Jianjun Paul Tian . The replicability of oncolytic virus: Defining conditions in tumor virotherapy. Mathematical Biosciences and Engineering, 2011, 8(3): 841-860. doi: 10.3934/mbe.2011.8.841 |
[5] | Lu Gao, Yuanshun Tan, Jin Yang, Changcheng Xiang . Dynamic analysis of an age structure model for oncolytic virus therapy. Mathematical Biosciences and Engineering, 2023, 20(2): 3301-3323. doi: 10.3934/mbe.2023155 |
[6] | Joseph Malinzi, Rachid Ouifki, Amina Eladdadi, Delfim F. M. Torres, K. A. Jane White . Enhancement of chemotherapy using oncolytic virotherapy: Mathematical and optimal control analysis. Mathematical Biosciences and Engineering, 2018, 15(6): 1435-1463. doi: 10.3934/mbe.2018066 |
[7] | Elzbieta Ratajczyk, Urszula Ledzewicz, Maciej Leszczynski, Avner Friedman . The role of TNF-α inhibitor in glioma virotherapy: A mathematical model. Mathematical Biosciences and Engineering, 2017, 14(1): 305-319. doi: 10.3934/mbe.2017020 |
[8] | Nada Almuallem, Dumitru Trucu, Raluca Eftimie . Oncolytic viral therapies and the delicate balance between virus-macrophage-tumour interactions: A mathematical approach. Mathematical Biosciences and Engineering, 2021, 18(1): 764-799. doi: 10.3934/mbe.2021041 |
[9] | Zizi Wang, Zhiming Guo, Hal Smith . A mathematical model of oncolytic virotherapy with time delay. Mathematical Biosciences and Engineering, 2019, 16(4): 1836-1860. doi: 10.3934/mbe.2019089 |
[10] | Taeyong Lee, Adrianne L. Jenner, Peter S. Kim, Jeehyun Lee . Application of control theory in a delayed-infection and immune-evading oncolytic virotherapy. Mathematical Biosciences and Engineering, 2020, 17(3): 2361-2383. doi: 10.3934/mbe.2020126 |
Combining chimeric antigen receptor T (CAR-T) cells with oncolytic viruses (OVs) has recently emerged as a promising treatment approach in preclinical studies that aim to alleviate some of the barriers faced by CAR-T cell therapy. In this study, we address by means of mathematical modeling the main question of whether a single dose or multiple sequential doses of CAR-T cells during the OVs therapy can have a synergetic effect on tumor reduction. To that end, we propose an ordinary differential equations-based model with virus-induced synergism to investigate potential effects of different regimes that could result in efficacious combination therapy against tumor cell populations. Model simulations show that, while the treatment with a single dose of CAR-T cells is inadequate to eliminate all tumor cells, combining the same dose with a single dose of OVs can successfully eliminate the tumor in the absence of virus-induced synergism. However, in the presence of virus-induced synergism, the same combination therapy fails to eliminate the tumor. Furthermore, it is shown that if the intensity of virus-induced synergy and/or virus oncolytic potency is high, then the induced CAR-T cell response can inhibit virus oncolysis. Additionally, the simulations show a more robust synergistic effect on tumor cell reduction when OVs and CAR-T cells are administered simultaneously compared to the combination treatment where CAR-T cells are administered first or after OV injection. Our findings suggest that the combination therapy of CAR-T cells and OVs seems unlikely to be effective if the virus-induced synergistic effects are included when genetically engineering oncolytic viral vectors.
Abbreviations: CAR-T cells: Chimeric antigen receptor T cells; OVs: Oncolytic viruses; TME: Tumor microenvironment; ODE: Ordinary differential equations; eFAST: Extended fourier amplitude sensitivity test
An emerging and promising cancer treatment strategy involves the use of chimeric antigen receptor (CAR)-T cells. In this therapy, T cells are extracted from a patient, genetically engineered to express chimeric antigen receptors (CARs), which aid T cells to recognize tumor-derived antigens or neo-antigens and eliminate tumor cells [1,2,3], and are infused back to the patient. CAR-T cell therapy has received the Food and Drug Administration (FDA) approval for treating B-cell malignancies [4]. Despite the remarkable therapeutic success of CAR-T cell therapy for blood cancers [5] and the promising results in treating hematological cancers and CD19+ B cell malignancies [6,7,8], there are still several challenges that hinder a broader clinical application [6]. Some major limitations include reduced identification of tumor-spefic antigens [9], immunosuppressive tumor microenvironment (TME) [10], and antigen loss in solid tumors [11]. Moreover, in some cases, CAR-T cells express certain T cell receptors (TCRs) which are found on normal T cell surfaces [12]. This often leads to graft versus host reactions that results in destruction of normal cells. To overcome this challenge, the patient's own T cells are used to generate CAR-T cells. The extraction of T cells from the patient is, however, very costly and sometimes unsafe [12,13]. Downregulation or complete loss of target antigens on tumor cell surfaces (also known as antigen escape) is another hurdle, which often leads to tumor escape from T-cell mediated immunity [11,13,14], faced by CAR-T cells [15,16]. Hence, there is a need for more research to explore the potential of combination therapy that can synergize with CAR-T cell therapy to surmount some of these challenges experienced by CAR-T cells.
Therapy with oncolytic viruses (OVs), which are naturally occurring or genetically engineered viruses that selectively replicate within tumor cells without damage to the normal tissues [17,18,19], recently emerged as a promising novel cancer modality that may be further combined with existing therapeutics to enhance their effects. In modern gene-based therapies, OVs have emerged as promising antitumor therapeutic modalities in scientific research and clinical practice [20]. In addition to their direct tumor-selective oncolysis, OVs posses many attractive properties that enable them to be used in combination with other treatment modalities [12,21,22]. OV can be genetically engineered to specifically lyse tumor cells, while sparing normal healthy cells, by either deleting or inserting new therapeutic transgenes [23]. Moreover, OVs can directly or indirectly (or can be genetically modified to) stimulate the immune system [24], which can further provide additional antitumor activity. Despite their varying efficacies in treating several cancers, accumulating evidence indicates that OVs been shown to provide a robust antitumoral effect in numerous preclinical studies [20,25]. However, these promising results, when used as a standalone treatment, the therapeutic benefits of OVs have so far been limited. In particular, systemic delivery of oncolytic virus has been restricted by antiviral immune response, neutralizing antibodies, and complement proteins [26], to mention a few. Even though tumors develop numerous defective or immune escape mechanisms, these mechanisms render them more susceptible to viral infections [20]. Furthermore, and most remarkably, OVs are known to heat up "cold" tumors into "hot" tumors, which are easily recognized by adaptive immune cells, especially T cells. Hence, OVs, by their ability to revert the immunosuppressive tumors, to specifically infect and replicate in tumor cells, as well as their capability to activate and recruit immune cells into the TME, are attractive agents for cancer immunotherapy.
Clinical evidence suggests that using OVs offers a great potential in overcoming challenges, such as tumor-mediated immunosuppression, which are faced by T cells or CAR-T cell therapy [27]. Additionally, OVs can foster infected tumor cells to express target tumor antigens that can be recognised by CAR-T [12]. Furthermore, some OVs have the ability to stimulate CAR-T cells's recruitment into the TME and enhance their ability to kill tumor cells [28]. Several other studies established that a combination therapy of OV and CAR-T cells, is a promising treatment modality for treating solid and metastatic tumors [1,2,3,9,11,22,29,30,31]. However, these studies also highlighted that even though it is quite beneficial to use OVs and CAR-T cells in combination, it is extremely important to find an appropriate time between OVs and CAR-T cells injections. An experimental study indicated that there was a modest tumor reduction when OVs and CAR-T cells were administered at the same time compared to when administered sequentially [32]. Most importantly, compelling evidence indicates that timing of OVs and CAR-T cells dosage is an important factor that can impact the treatment outcome [33,34,35], even though the strength and timing of OV-induced CAR-T cell recruitment varies depending on virus biology [36,37]. In particular, an effective combination therapy, requires that OVs are injected first to lyse tumor cells or modulate immunosuppressive tumor TME for CAR-T cells function [11,33,38,39]. It is therefore natural to ask whether a single dose or multiple sequential doses of OVs during treatment with CAR-T cells can have any synergistic effect on tumor cell reduction. Of course, this depends on (a) the virus oncolytic potency (viral clearance, infection rate and burst size) and (b) the intensity of the virus-induced synergy (virus-induced increased levels in the migration of CAR-T cells to the TME, and in their killing ability of tumor cells). Thus, the range of OV infection, replication and lytic OV potencies during the combination treatment with CAR-T cells also require further investigation [33].
Mathematical models are useful tools to explore these important aspects, they have provided valuable insights in various tumor immunotherapy under preclinical, translational and clinical settings (e.g., see the reviews [40,41,42]) and in CAR-T cell therapy (e.g., see the review [43]). In recent years, several mathematical models that explore different aspects of tumor growth and progression under CAR-T cell therapy have been developed [44,45,46,47,48,49]. In particular, a recent work by Leˊon-Triana et al. [45] investigated the effects of CAR-T cells targeting two antigens (CD19 and tumour-associated antigens) against glioblastoma. Of note, with model simulations, it was shown that injecting large numbers of activated CAR-T cells can overcome the challenge of immunosuppressive TME. The complex dynamic interactions between tumor cells, various immune cells, and OVs have been extensively studied in numerous models [50,51,52,53,54,55]. To the best of our knowledge, there is only one mathematical model that considered the dynamic effects of a combination therapy of CAR-T cells and OVs. In particular, Walker et al. [56] developed two different mathematical models that describe tumor response to cytotoxic activities of CAR-T cells and OVs. With model simulations, the authors illustrated that there are some synergistic effects between CAR-T and OVs with respect to total tumor reduction [56]. The model further predicted that the combination therapy of OVs and CAR-T cells resulted in a significant reduction of tumor cells. Moreover, model simulations showed that when co-injected, OVs and CAR-T cells could not completely eliminate tumor cells [56]. However, these models ignored the OVs-induced synergistic effect on CAR-T cells' recruitment and their ability to kill that is reported in [28]. We note that this synergy can cause CAR-T cells to significantly reduce the infected tumor cell population, which may hinder the replication of OVs. It is therefore of paramount importance to investigate the effect of the reported synergy between OVs and CAR-T cells on the outcome of the combined CAR-T/OV therapy.
For this purpose, we develop a simple mathematical model that describes the local interactions between effector CAR-T cells, tumor cells, and OVs. For the sake of keeping our model simple, our model specifically mimics and considers brief encounter of tumor cells with OVs and CAR-T cells under the treatment scenario of immunodeficient "mouse" model. In particular, we explore whether there is any potential synergism in administrating OVs prior to CAR-T cell therapy. While each treatment modality can still cytoreduce tumor growth by its own, we hypothesize that the efficacy of CAR-T cell therapy can be greatly enhanced by prior administration of OVs. Hence, we shall investigate various dose regimens that may provide robust virus-induced synergistic effects on CAR-T cell cytotoxicity against targeted tumor cells.
As a first step in understanding the complex dynamic effects of CAR-T cells in oncolytic virotherapy, we devise an ordinary differential equations (ODE) model that focuses mainly on the factors regulating tumor response to a combination therapy of OVs and CAR-T cells. While there exists a wide variety of mathematical models in oncolytic virotherapy, based on ODEs [50,57,58,59], partial differential equations (PDEs) [60], stochastic differential equations (SDE) [61], as well as models based on hybrid computational methods such as multi-scale [62] and agent-based modeling approaches [63], in the present study, we considered a simple ODE modeling framework with 4 variables to understand the basic interaction dynamics between tumor cells, CAR-T cells and OVs within the TME. We adopted this simplistic modeling framework because ODE models are, generally speaking, easy to analyze and the resulting solutions are easily tractable [64,65,66,67]. Most importantly, some significant insights gained from such simple ODE-based models are easily validated with experimental data [18,58,68,69], even with clinical data [70], and could also provide vital information pertaining to clinical implementation of OV-based treatment protocols [71,72]. The model consists of three cell populations: the uninfected tumor cells (Tu), tumor cells infected with OVs (Ti) and effector CAR-T cells (CT), and an OV population (V). A schematic representation of the local interactions considered in the model is depicted in Figure 1, and the biological description of the model parameters and their values is provided in Table 1.
Parameter | Description | Baseline value | Units | References |
r | Growth rate of uninfected tumor cells | 0.206 | day−1 | [81] |
K | Tumor carrying capacity | 1.404×108(107−109) | cells | [51,66,79] |
β | Infection rate of uninfected tumor cells by free viruses | 4.7×10−4(0.0008−0.008) | (PFU−1)(day−1) | [79], Assumed |
δT | Killing rate of tumor cells by CAR-T cells | 10−7 | (cells⋅day)−1 | Assumed |
ϵ | Virus-induced synergistic rate | 10−2 | PFU−1 | Assumed |
ξV | Virus-induced CAR-T cell recruitment rate | 10−8 | (PFU−1)(day−1) | Assumed |
lv | Lysis rate of infected tumor cells | 0.4(0.0034−11) | day−1 | [82] |
b | Viruses released by lysed infected tumor cell | 3×103(10−1,350) | (PFU)(cells⋅day)−1 | [78] |
ω | Viral clearance rate | 0.6 | day−1 | [78] |
γT | Viral clearance rate by CAR-T cells | 2 | day−1 | Assumed |
αT | Inactivation rate of CAR-T cells by tumor cells | 0.266(0.01−0.99) | (cells⋅day)−1 | [45] |
ρT | Proliferation rate of CAR-T cells | 0.265(0.2−0.9) | (cells⋅day)−1 | [44,45] |
λV | Initial virus inoculum | 2×106(103−1010) | (PFU)(cell⋅day)−1 | [83] |
λCT | Adaptive CAR-T cell therapy | 2×106 | cells⋅day−1 | [44,77] |
μT | Natural death of CAR-T cells | 0.350 | day−1 | [44] |
A system of equations describing the cellular and OV dynamics of the populations considered in this study is given by
dTudt=rTu(1−Tu+TiK)−βTuVTu+Ti−δT(1+ϵV)CTTu | (2.1a) |
dTidt=βTuVTu+Ti−δT(1+ϵV)CTTi−lvTi | (2.1b) |
dVdt=λV(t)+bTi−γTCTV−ωV | (2.1c) |
dCTdt=λCT(t)+ξVCTV+ρT(Tu+Ti)CT−αT(Tu+Ti)CT−μTCT | (2.1d) |
In Eq (2.1a), the first term accounts for the logistic growth of tumor cells in the absence of therapy. The second term denotes the infection of tumor cells by OV at the rate β. It is important to note that in our modeling framework, we have assumed that there are no multiple infections. That is, once a virus has successfully infected a certain tumor cell, then that cell cannot be infected by another virus. Hence, the probability of a virus infecting a tumor solely depends on the number of available uninfected tumor cells. Mathematically, this dependency is modeled through the frequency-dependent kinetics, βTuV/(Tu+Ti), as done in previous models [73,74,75]. Moreover, the choice of this frequency-dependent infection for our model is justified by our assumption that we are modeling interactions between tumor cells, OV and CAR-T cells within the TME. Thus, the OV infection rate is governed mainly by the virus concentration within the TME and the frequency of available uninfected tumor cells. The third term accounts for tumor cell killing by the effector CAR-T cells. The presence of the term (1+ϵV) is to reflect the OV-induced increase in CAR-T's killing of tumor cells. OV infection causes a cascade of subcellular inflammatory events that often result in recruitment of CAR-T cells to the tumor, and enable tumor to be easily infiltrated by effector CAR-T cells [33,36].
The increment of infected tumor cell population is represented by the first term in Eq (2.1b). The second term denotes the killing rate of tumor cells by the effector CAR-T cells. The third rate represents the lysis of infected tumor cells with the rate lv.
In Eq (2.1c), the first term, λV(t) (discussed in detail later), denotes the injection of OVs. The second term accounts for an increase in OV concentration in the TME due to the lysis of infected tumor cells with the burst size of b. The killing of OVs by effector CAR-T cells is denoted by the third term. The last term represent the viral clearance.
In Eq (2.1d), an administration of adaptive CAR-T cell therapy is represented by the first term, λCT(t) (discussed in detail later). The second term represents an OV-induced recruitment of CAR-T cells as in [28]. The third term denotes that effector CAR-T cells are stimulated by tumor cells during their interactions, which result in proliferation of effector CAR-T cells with constant rate ρT. The fourth term, which includes the natural death, describes inactivation of effector CAR-T cells by tumor cells following their interactions at the rate αT.
One disadvantage of many preclinical models is a frequent dosage of OV injections in order to achieve a statistically significant treatment outcome [76], as mathematically highlighted in [50]. In the present study, we propose a single dosing schedule for each of OV and CAR-T cell based therapies. We propose to use the following hyperbolic tangent type function
ζ(A,B,τ,t)=A+B−A1+e−k(t−τ). |
It is clear that this function is smooth enough to ensure the well posedness of our model. More importantly, this function has two interesting properties: (a) it tends to A (respectively B) for small (respectively large) values of t, and (b) it moves from A to B around the time t=τ, with a gradient that is controlled by k.
Using this function, we are now able to construct the injection rates λV(t) (in units: plaque−formingunits(PFU)⋅cell−1⋅day−1), in Eq (2.1c), and λCT(t) (in units: cells⋅day−1), in Eq (2.1d), as follows:
λX(t)=ζ(k1X,λ0X,λmX,τ1X,t)+ζ(k2X,0,λ0X−λmX,τ2X,t),with X=V and X=CT,respectively. |
The functions λV(t), λCT(t), start and stay at the value λ0X until time t is close to τ1X where they move to λ0X and remains at this value until around t=τ2X where they move to 0.
To have a profile that a is typical of an OV or CAR-T single dose injections, we chose λ0V=0 PFU⋅cell−1⋅day−1, λ0CT=0 cells⋅day−1, λmV=106 PFU⋅cell−1⋅day−1, k1X=k2X=1.5 unitless, λmCT=2×106 cells⋅day−1 and τ1V=40 day, τ2V=42 day and τ2CT=(τ1CT+2) day, where τ1CT takes various values that accommodate our simulation scenarios. The resulting profile of a single dose injection of OV is given in Figure 2.
For the sake of our simulations, we assume that at time t=0 the tumor is at its maximum carrying capacity, with no infected cells, viruses or CAR-T cells. This ensures that the tumor size is always the same regardless of the times of the initial OV and CAR-T doses are. Note also that the maximum tumor carrying capacity, K=1.404×108 cells, considered in this study, is below the carrying capacity of K=3×109, which is equivalent to a tumor size of 3000 mm3 that is lethal to mice [73]. Accordingly, the initial conditions of the system (Eq (2.1a)–(2.1d)) are
Tu(0)=K cells, Ti(0)=0 cells, V(0)=0 PFU⋅cell−1⋅day−1, CT(0)=0 cells. | (2.2) |
We note that when ϵ=ξV=0, model (2.1) describes the dynamics of tumor cells under the combined CAR-T/OV therapy without synergy. Compared to the ODE model in [56], our model without virus-induced synergy, includes the OV terms and excludes CAR-T cells circulating in the blood, and the overall inflammatory response. We note that there are other plausible possible formulations to mathematically characterize virus-induced synergy between OV and CAR-T cells, but we choose this simple formulation as there is no appropriate experimental data to validate our model about the kinetics of the parameter ϵ.
Since our modeling framework is based on immunodeficient mouse models, for CAR-T cell therapy, the baseline parameter values in Table 1 were taken directly from CAR-T cell immunotherapy models in [44,45,77]. For oncolytic virotherapy, the baseline parameter values were taken from various mathematical models [51,66,71,78,79,80] because not all parameter values were available in one study. Indeed, various oncolytic viruses result in different treatment outcomes, partly because of their intrinsic or genetically engineered characteristics. For example, considering a vesicular stomatitis virus (VSV), infected tumor lysis rate (lv) is 11 per day in [71], 1 per day in [79], while 0.475 per day is reported in [80].
Thus, there is a diverse, yet very scarce information regarding lysis rate of infected tumor cells by OV. Similarly, it is very difficult to find an appropriate value for viral particles than can trigger infection within the tumor. Thus, our model has a few "free" parameters which we vary within a plausible range to assess their impart on model predictions. These free parameters are infection rate β, lysis rate of infected tumor cells lv, rate of CAR-T cell recruitment by OVs ξV and viral burst size b.
The aim of this work is to investigate how tumor cells respond to a combination therapy of CAR-T cells and OVs, and provide a quantitative description of local effects of CAR-T cells during oncolytic virotherapy. Especially, we explore the effect of the virus oncolytic potency and the intensity of the virus-induced synergy on the output of the combined therapy. In particular, we investigate the effects of (ⅰ) infection rate, (ⅱ) burst size, (ⅲ) viral clearance, (ⅳ) virus-induced increased levels in the migration of CAR-T cells to the TME, and (ⅳ) virus-induced increased levels in CART-T cells' killing ability of tumor cells.
We first hypothesize that timing of OV injections is a major determinant of a combination therapy with CAR-T cells. A major reason for timely injections of OVs and CAR-T cells is to prevent premature OV clearance by effector CAR-T cells. For effective combination therapy, it is also equally crucial to minimize tumor escape by injecting CAR-T cells at an appropriate time to provide complementary anti-tumor effect. Thus, the results in this study are categorized into two major components: Model simulations for combination therapy without virus-induced synergistic effects and Model simulations for combination therapy with virus-induced synergistic effects. The numerical simulations were performed in Matlab (R2017b) using ode23s solver. We now discuss these results in detail.
We performed a global sensitivity analysis using the extended Fourier Amplitude Sensitivity Test (eFAST) [84], a variance-based sensitivity method, to understand how the uncertainty in parameter values impacts the model predictions with respect to the total tumor cell population. The parameter values were varied from half to twice the baseline values in Table 1 to account for a possible range of uncertainty in the model parameter values. eFAST provides two sensitivity indices, namely the first-order indices, Si, and the total-order indices, Sti. The first-order indices (Si) measure the influence of a particular parameter on the variance of the total tumor cell population (the model output) without the local interactions with other model parameters, while the total-order indices (Si) measure the influence of a particular parameter on the total tumor cell population with possible interactions with other model parameters. Given these two indices, it is easy to quantify whether the total tumor reduction occurs due to a direct influence of the given parameter or through the influence of other model parameters. To compute the sensitivity indices with the eFAST method, we used a sample size of 4000 from the normal distribution of each parameter to examine the uncertainty and sensitivity of our model predictions to variations in model parameter values. We implemented the eFAST method using the generic MATLAB code developed by Kirschner et al. [110], and the results are given in Figure 3. We find that the reductions in the total tumor cell population are also heavily affected by the virus infection rate (β) and the rate at which infected tumor cells are lyzed by the OVs (lv) at day 40. The tumor growth rate (r) also significantly affects the model predictions (Figure 3(A)), at day 10 and 40. Intuitively, this means that tumor aggressiveness (governed by the proliferation rate r) plays an important role in determining the likelihood of the therapy success or failure [50,68,85,86]. On the other hand, the virus-induced synergistic rate (ϵ) and the virus-induced CAR-T cell recruitment rate (ξV), parameters which we have assumed their values in our model, have very little influence on the model predictions, since they have lower Si and Sti values than the dummy variable (last column bar) in Figure 3(A). Therefore, the uncertainty of these two parameters does not significantly affect the robustness of the predictions. Since the carrying capacity, K, was the most influential parameter in the model, as evidenced by both the first-order indices and total-order indices, in the numerical simulations, we set the tumor size to its maximum carrying capacity at time t=0, with no infected cells, viruses or CAR-T cells. This assumption ensures that the total tumor size is the same regardless of the initial CAR-T and OV doses, and hence would allow us to quantify the effects of most sensitive parameters and the order of dosages of the two therapies. Collectively, the sensitivity analysis illustrates that, in addition to the tumor carrying capacity, the model is mostly affected by few virus related parameters. Thus, we explicitly study the influence of such parameters on total tumor cell numbers by individually varying these parameters to predict their effects on the combination therapy.
It is argued that CAR T-cells are less likely to synergistically benefit from OV therapy if OV infection induces an apoptotic tumor cell death, rather than immunogenic cell death [31]. We investigated this proposition by investigating the behavior of model, Eq (2.1a)–(2.1d), without virus-induced synergy, i.e., ϵ=ξV=0. This therapeutic scenario resembles the case where OVs induce apoptotic cell death (i.e., tumor cell death does not induce any T cell responses). Here, in order to mimic a more plausible dosing schedule, we propose a dosing schedule that allows for sufficient virus propagation in infected cells, depicted in Figure 4.
Note that in Figure 4, the dosing day 42 for CAR-T cells is the same time used in the experiments in [77], while day 17 is an adhoc time for OV injection. Indeed, for the combination therapy with adoptive T cells, OVs can be injected as early as day 7 post tumor implantation in mice [87].
As suggested in the literature that OVs should be administered first to change the TME to a state that favors CAR-T cell function and permissiveness, and directly induce oncolytic effect on tumor cells [33], we explored the effects of varying OV infection strength (described by parameter β in Eq (2.1a)) on tumor growth. We believe that the strength of OV infection can determine whether adding an adjuvant therapy of CAR-T cells can lead to improved or deleterious effects on tumor growth. Indeed, OV infection rate plays a vital role in oncolytic virotherapy [71,88]. The therapeutic effects of varying OV infection on tumor cell killing are shown in Figure 5.
As can be seen in Figure 5(A) when infection rate is low (i.e., β=9.0×10−4), OVs alone can ultimately lead to tumor elimination as expected, but it takes unrealistically long time to reach a tumor-free state for given duration of in silico experiment, indicating the need for an adjuvant therapy. It should, however, be noted that if OVs infect tumor cells at a little higher rate (i.e., β=6.6×10−4), then there is a notable and observable difference in the number of infected tumor cells (Figure 5(D)) compared to those receiving slower infecting OVs (in Figure 5(C)), indicating that change in the infection rate seems to bring significant effect on tumor growth. In all cases, we note that CAR-T cells leads to rapid elimination of tumor cells.
Similarly, it is equally important to examine the effects of varying infection rates on tumor cell growth. If OVs have a lower infection rate, then many free OVs would remain vulnerable to CAR-T cell clearance, as depicted in Figure 6. Of note, in Figure 6(A), (B), there is a relatively slow increasing virus accumulation in the TME, which is swiftly interrupted by injection of effector CAR-T cells. While injection of CAR-T cells on day 42 (see Figure 6(C), (D)) seems not to provide a notable clearance of free OVs within the TME, we note that their cytotoxic effect is more prudent on tumor cells (see Figure 5(A), (B)).
More importantly, consistent with experimental studies [77], we note that the effector CAR-T cells rapidly decay to zero in the absence of OVs and tumor cells. We should emphasize that decaying to zero means that either the CAR-T cells are eliminated from the TME due to natural death or they are becoming memory CAR-T cells, and would be readily available upon tumor re-challenge, as shown in [44,89].
As a first step, we investigated where a single dose of 2×106 cells is sufficient to drive the total tumor cell population to extinction. Figure 7 indicates that a single dose of 2×106 CAR-T cells sufficed to reduce the total tumor load to a smaller size in a few days; however, the tumor rapidly regrew to its maximum carrying capacity. This implies that a single dose of CAR-T cells can lead only to a small transient tumor reduction, and thus the therapy cannot control tumor growth in this in silico experiment (see Figure 7). We should emphasize that this observation could be related to the particular choice of parameter values and initial conditions used in our model, and would significantly change under different parameter values and initial conditions.
Thus, an initial dose of CAR-T cells results in small reduction in the total tumor cell population (Figure 7), necessitating the need for additional CAR-T cell doses to control tumor growth in silico. It imporatnt to note that this observation is related to the particular choice of parameter values used in this study, and the outcomes are likely change under different conditions.
Adding a single dose of OV, we observe in Figure 8 that using a single dose of CAR-T cells given at any of the times given in the legend, the total tumor size converges to zero.
Interestingly, in Figure 8, we observe that using a single dose of OV and single dose of CAR-T cells given at any of the times given in the legend, the total tumor size reaches to zero.
In summary, using our proposed model, without virus-induced synergism, we explored diverse scenarios which can lead to eradication of tumor cell populations. In particular, it was shown that variations in OV infection rates may determine if CAR-T cells can augment or inhibit virus oncolysis. Depending on the dosage and timing of OV administration, if the virus has lower infection rate, then its propagation within the tumor cell or TME is likely to be halted by effector CAR-T cells. On the other hand, if the virus has higher infection rates, then it is likely to eradicate all tumor cells prior to injection of CAR-T cells. Collectively, these results illustrate that combating tumor burden with a combination therapy of OVs and CAR-T cells does not always provide superior therapeutic outcome as CAR-T cells can lead to either augmentative or deleterious effects. Furthermore, we showed that a single dose of CAR-T cells is not enough to eradicate tumor cells.
We now use our model, Eq (2.1a)–(2.1d), to investigate where OVs can co-operate with CAR-T cells by inducing immunogenic tumor cell death, rather than apoptotic tumor cell death considered previously. In particular, we investigate whether there is any synergism between OV and CAR-T cells in killing tumor cells and how the order of OV and/ or CAR-T cell administration impact the overall combination therapeutic outcome.
To determine whether OVs can synergistically enhance CAR-T cell lytic activity against tumor cells, we simulated the treatment scenario where tumor is treated with a fixed dose of each therapy. In particular, we propose a treatment scenario where tumor is treated with one dose of 106) PFU on day 40 and same fixed doses of 2×106 CAR-T cells at various time points (i.e., before, concurrent with, and after OV administration). This treatment setting is motivated by, but sightly different from, the in vivo experiment done by Krabbe et al. [87] (where the antigen-specific OTI T cells are injected once and oncolytic vesicular stomatitis virus (VSV), VSV-NDV, is injected prior and posterior to OTI T cell injection). Figure 9 illustrates examples of the model's (including the synergy parameter, (2.1a)–(2.1d)) simulations where a potential synergistic effect between OVs and CAR-T cell therapies is considered with respect to dosage and timing of each therapy.
As can be seen in Figure 9(A), CAR-T cell monotherapy is unable to control tumor growth, applied in day 10 and 20, but when applied in combination with OVs, on day 40, there is a significant increase in cytotoxicity of tumor cells, consistent with recent experimental results in [87]. It is also important to note that because OVs are also susceptible to CAR-T cell clearance, there would be a rapid decline in free virus concentration within the TME (Figure 9(B)). Since the tumor is not completely eliminated, we observe that the same fixed subsequent dosage of CAR-T cells is still unable to reduce tumor progression, and tumor escapes to some lower stable steady state (Figure 9(A)), though below a lethal threshold of 1×1010 cells considered in [44] or 3×109 cells for mice [73]. In agreement with the findings in [90], depending on the initial tumor size, it is plausible that the total tumor size can be reduced to a smaller size than the carrying. This result further suggests that complete tumor elimination can be observed via increase in CAR-T cell dosages, in agreement with numerical simulations in [44]. Of particular note, in Figure 9(A), the maximum tumor reduction is observed when virus concentration is high, indicating synergistic therapeutic effects as a result of combination therapy (i.e., co-administration of OVs and CAR-T cells). While this result seems counter-intuitive because one may expect that OV infection would rapidly be forestalled by CAR-T cells [31], the observation in Figure 9 is consistent with many in vitro and in vivo studies that consider a combination treatment of OVs and CAR-T cells [2,3,11,87]. One possible explanation for this increased antitumor activity is that OV infection results in immunogenic cell death of tumor cells, which often results in recruitment of T cells to the TME [29,31,91,92,93]. In other words, this means that since not all OVs will be cleared by CAR-T cells, during simultaneous administration, then the resulting death of infected tumor cells, may further recruit CAR-T cells towards to tumor cells. It is also important to note that there exists a non-monotinic regrowth of the total tumor (Figure 9(A)) with CAR-T doses at day 100 and 130 (purple, light blue) due to the small increases in the virus populations depicted in Figure 9(B). This observation could be attributed to low viral replication rate (which is below a certain threshold [94,95]), as a result of limited virus infection propagation by CAR-T cells.
Notably, we see in Figure 9 that the combined therapy did not succeed in eliminating all tumor cell populations. This could be explained by the fact that the synergistic effect of Ovs on CAR-T cells, enhances CAR-T cell proliferation and their antitumor activity, which may result in killing of infected tumor cells and hence hinder OV oncolysis. This gives a clear guidance why there is always a need for sequencial administration of multiple doses of CAR-T cells and OVs. The dosage and timing of the OVs and CAR-T cells governs the potential synergism that can result from the combination therapy. In particular, the dosing regime where simultaneous administration of OV and CAR-T cells is applied has the greatest overall tumor reduction compared to the dosing regimen where CAR-T cells are administered first or after simultaneous dosage of OVs and CAR-T cells.
Taken together, these results demonstrate not only the potential of simultaneously administrating CAR-T cells and OVs to obtain an improved therapeutic effect, but also highlight the importance of designing new dosing strategies to simultaneously administer CAR-T cells and OVs at different times. Moreover, it seems plausible that during the co-administration of CAR-T cells and OVs, there is some potential virus-induced synergism that enhances CAR-T cell antitumor activity. While this might be a favorable treatment outcome, it should be noted that if the CAR-T cell induction is too strong, then CAR-T cells can potentially inhibit OV infection propagation by eliminating both infected tumor cells and free OVs within the TME, as was shown with the natural killer (NK) cells by Senekal et al.[66]. Furthermore, these results highlight the importance of mathematical modeling as a tool that can be used to explore plausible therapeutic outcomes prior to in vitro or in vivo studies.
In this section, we explore the effect of the virus oncolytic potency and the intensity of the virus-induced synergy on the output of the combined therapy. In particular, we investigate the effects of (ⅰ) infection rate, (ⅱ) viral clearance, (ⅲ) burst size, (ⅳ) virus-induced recruitment of CAR-T cells to the TME, and (ⅳ) virus-induced increased levels in CAR-T cells' killing ability of tumor cells.
As suggested in the literature that OVs should be administered first to change the TME to a state that favors CAR-T cell function and permissiveness, and directly induce oncolytic effect on tumor cells [33], we first studied the effects of OV infection strength (i.e., as described by the parameter β in Eq (2.1a) on tumor growth. We believe that the strength of OV infection can determine whether adding an adjuvant therapy of CAR-T cells can lead to improved or deleterious effects on tumor growth. Indeed, OV infection rate plays a vital role in oncolytic virotherapy [71,88]. We examine the effects of strength virus infection rate by varying the infectivity rate β and examined the therapeutic impact on tumor cell killing in Figure 10.
In agreement with other recent theoretical models [66,96], if OVs infect tumor cells at a little higher rate (i.e., β=3.7×10−4), then there is a more observable difference in tumor cell reduction (Figure 10(A)) compared to those receiving slower infecting OVs (in Figure 10(B)), indicating that small changes in infection rates of OVs can have significant effects on tumor growth dynamics.
Another significant parameter that often defines the success of the therapy with OVs is the burst size, which is the number of infectious viruses produced by lyzed infected tumor cell. Figure 11 illustrates the effects of varying the burst sizes on total tumor cell population.
As demonstrated in Figure 11(A), and consistant with other biological [97] and mathematical findings [50,51,78,96], OVs with higher burst sizes have a greater antitumor impact. The rationale for this is that when OV burst size is high, then there will be more free OVs within the TME, and which, consequently, are likely to infect more tumor cells. It is worth mentioning that one vital attribute of OVs is that they can easily be genetically modified to produce higher viral titers [98], and our simulation results further highlight the essence of manipulating the virus burst size within the biologically feasible ranges.
Decay rate of OV, ω, with respect to therapy outcomes, is another important parameter worth considering. As demonstrate in Figure 12(A), if the OV has a very low virus death rate ω=0.9, there is a greater antitumor impact on tumor, compare to higher virus death rate ω=1.2, which results in minimal reduction of tumor load from equilibrium (Figure 12(B)). The longer the lifespan of an OV, the higher the lytic effect on tumor cell population.
As can be seen in Figure 12(A), even though there is an immediate tumor reduction, upon sequential injections of effector CAR-T cells at day 10 and 20, effective tumor control by CAR-T cells is observed after a long time period (around days 150 (blue curve) and 245 (yellow curve) in Figure 12(A)). One possible explanation for this occurrence is that upon the joint administration of CAR-T cells and OVs at day 40, antiviral activity of CAR-T cells was more robust than antitumor activity; thus, taking a longer time to effectively impact the total tumor load at equilibrium. In a nutshell, our study suggests that a significant reduction of the total tumor load (though it occurred on a much longer time scale) is also achievable with early time points administration of CAR-T cell monotherapy.
Oncolytic virus-induced potency is one of the key determinants of successful oncolytic virotherapy [50,99,100], thus, in Figure 13, we investigated whether an increase in the rate OV kills tumor cells, lV, and the rate of virus-induced CAR-T cell antitumor activity, ϵ, can improve or inhibit the combined antitumor effect of OV and CAR-T cells.
Of particular note, less lytic OVs do result in more tumor reduction (Figure 13(A)), as compared to OVs with high lytic ability that are able to destroy a large portion of tumor cells (Figure 13(B)). On the same hand, and most interestingly, we also observe that when there is high virus-induced synergistic killing of tumor cells by CAR-T cells, there is a greater total tumor cell reduction (Figure 13(C)), compared to low virus-induced synergism (Figure 13(D)). It is important to note that while this effect (of virus-induced synergism) is therapeutically exciting, it is by far less significant compared to the therapy without virus-induced synergistic effect (Figure 8). Taken together, these results suggest that high virus-induced tumor killing by CAR-T cells may not lead to successful combination therapy immunogenic OVs and effector CAR-T cells. We should emphasize that higher virus-induced synergy can lead to premature and rapid antiviral CAR-T cell responses, if the OV immunogenic strength is too strong as shown in [66], which in turn would inhibit OV oncolysis. This explains why the combination therapy without virus-induced synergy led to tumor elimination (Figure 8), while the one with virus-induced synergism did not Figure 13(C), (D).
The effect of recruiting effector CAR-R cells to the TME, is investigated in Figure 14.
Consistent with experimental studies [98,101,102] and mathematical models [66], in Figure 14(B), we note that OVs that immunogenetically recruit immune cells at higher rates do not offer optimal therapeutic benefits, Nonetheless, the effective recruitment of CAR T-cells into the TME dependents mainly on the cascade of events leading to diverse chemokine signalling pathways [32].
We end this section by stating the inherent limitation of the present study: Current limitations of the present study include the exclusion of tumor antigen heterogeneity when OVs and CAR-T cells are administered and fixed dosage regimes. Expression of diverse tumor antigens is one of the major hurdles of CAR-T cell based therapy against solid tumors [103,104]. While the mathematical model proposed in this study could be utilized to design and generate in silico trails to explore optimal therapy combinations, it did not consider the possibility of fractionated dosing regimens and timings of the CAR-T cells, which might reduce the possibility of CAR-T cell toxicities. Indeed, fractionated dosing regime for CAR-T cells was shown to reduce observable toxicities while still resulting in more effective CAR-T cell treatment [105].
While CAR-T cell therapy has recently demonstrated promising results against many hematological malignancies, its clinical application against solid tumor still faces many challenges, including antigen loss or heterogeneity, immunosuppressive TME and suboptimal CAR-T cell trafficking. As an attempt to improve the efficacy of CAR-T therapy, combination therapy of OVs and CAR-T cells has recently proven to be one of the promising solutions for tackling some barriers of CAR-T cell therapy. Although it has recently been shown that OVs can aid CAR-T cells in various ways, many studies are still needed to demonstrate whether an apoptotic or immunogenic cell death induced by OVs can synergize with CAR-T cells to potently kill tumor cells. In this article, we developed a simple compartmental model, with virus-induced synergism, to describe the local interactions between OVs, tumor and effector CAR-T cells with the TME.
First, using the model without synergy parameter, we explored the potential effects of administering OVs first, then followed by CAR-T cells, we noted that the level at which CAR-T cells can augment virus oncolysis depends on the strength of virus virulence (i.e., whether OVs are strongly or weakly lytic) and the dose order (i.e., whether OVs are administered before CAR-T cells administration). Second, by including the synergy parameter in our model, we further investigated whether the is any potential virus-induced synergism by considering a dosing regime where CAR-T cells are administered prior to, concurrent with OVs and posterior to simultaneous OV and CAR-T cells administration. Our sensitivity analysis (based on eFAST) results revealed that the tumor carrying capacity is a most vital parameter (indicated by high sensitivities in both first-order and total-order indices) that can significantly impact the therapy outcomes. From model simulations, it was shown that simultaneous administration of OVs and CAR-T cells considerably decreased tumor burden compared to CAR-T cell monotherapy, applied prior to or posterior to simultaneous injections of OVs and CAR-T cells. Considering how the ordering of the dosages and timings of OVs and CAR-T cells resulted in different tumor growth dynamics, it is advisable to administer OVs first and/or concurrent with CAR-T cells, to prime or sensitize CAR-T cells towards tumor. This sensitization can result in greater antitumoral effect—due to a cascade of intra-cellular signaling events mediated by OV oncolysis which would ultimately recruit CAR-T cells to TME. Moreover, and notably, our simulations illustrate that tumor response to the combination therapy of OVs and CAR-T cells is directly dependent on the intensity of virus-induced synergistic tumor killing by effector CAR-T cells, which, in turn, would decrease the efficacy of the combination treatment. Taken together, our results suggest that virus-induced synergistic effects of CAR-T cell on tumor cell killing during the treatment with OVs can potentially impede treatment success.
Although the results attained in this study are informative on how synergistically OVs can induce the direct effect of CAR T-cell function on tumor growth, there are several limitations and simplifications that suggest follow up studies. The fact that the total tumor cell killing by CAR-T cells is minimal in the present model, while CAR-T cells are known to have much antitumor effect in the literature [5,15,16,30], naturally limits the model applicability and suggests further refinement of the model's assumptions and complexity. Limited CAR-T cell effect is related to the killing rate of tumor cells by CAR-T cells, assumed here to have a fixed value of 10−7 cells per day across all tumor cells, which is in the range obtained experimentally in [44]. While this simplification was made in the spirit of keeping our model simple, in patient or mice populations, there could be some level of variation in the killing rates of CAR-T cell due to diverse antigen expression by both uninfected and infected tumor cells [106]. Implicitly, in the current model, we assumed that the CAR-T cells are able to target one antigen which is present to both uninfected and infected tumor cells. Recent advances in CAR-T cell designs focus on making CAR-T cells to target multiple antigens [33,45,107]. In future work, it would be interesting to examine how variations in the CAR-T cell killing rates affect treatment success. Another important simplification that limits the present study is that our modeling framework does not account for tumor cell heterogeneity and spatial variations in CAR T-cells, or OVs concentration. Due to the diverse nature of heterogeneity of tumor cells, intrinsic or induced by OV infections, spatial distributions and heterogeneity of tumor cells may be important considerations for future work. Furthermore, it would be interesting to explicitly investigate not only the non-localized interactions between tumor cells, CAR-T cells, and OVs, which are regulated by specific chemokine signaling pathways, but also the effects of temporal dependencies of CAR-T cell antitumor activity on tumor growth. Finally, a future direction of the present work would examine a wide range of CAR-T/OVs mechanisms (e.g., the optimal dosage that leads to maximum tumor reduction as done in [108,109]) that can lead to optimal virus-induced synergy necessary to mount potent CAR-T cell antitumor response against solid tumors.
We greatly thank the anonymous reviewers whose insights, valuable comments and suggestions have improved our manuscript. R. Ouifki acknowledges the support from the DST/NRF SARChI Chair in Mathematical Models and Methods in Biosciences and Bioengineering at the University of Pretoria. L. de Pillis acknowledges the support of the Norman F. Sprague, Jr., Chair of Life Sciences and the Department of Mathematics of Harvey Mudd College.
The authors declare there is no conflict of interest.
[1] |
N. Nishio, I. Diaconu, H. Liu, V. Cerullo, I. Caruana, V. Hoyos, et al., Armed oncolytic virus enhances immune functions of chimeric antigen receptormodified T cells in solid tumors, Cancer Res., 74 (2014), 5195–5205. https://doi.org/10.1158/0008-5472.CAN-14-0697 doi: 10.1158/0008-5472.CAN-14-0697
![]() |
[2] |
K. Tanoue, A. R. Shaw, N. Watanabe, C. Porter, B. Rana, S. Gottschalk, et al., Armed oncolytic adenovirus-expressing PD-L1 mini-body enhances antitumor effects of chimeric antigen receptor T cells in solid tumors, Cancer Res., 77 (2017), 2040–2051. https://doi.org/10.1158/0008-5472.CAN-16-1577 doi: 10.1158/0008-5472.CAN-16-1577
![]() |
[3] |
A. R. Shaw, C. E. Porter, N. Watanabe, K. Tanoue, A. Sikora, S. Gottschalk, et al., Adenovirotherapy delivering cytokine and checkpoint inhibitor augments CAR T cells against metastatic head and neck cancer, Mol. Ther., 25 (2017), 2440–2451. https://doi.org/10.1016/j.ymthe.2017.09.010 doi: 10.1016/j.ymthe.2017.09.010
![]() |
[4] |
S. J. Schuster, M. R. Bishop, C. S. Tam, E. K. Waller, P. Borchmann, J. P. McGuirk, et al., Tisagenlecleucel in adult relapsed or refractory diffuse large B-cell lymphoma., N. Engl. J. Med., 380 (2019), 45–56. https://doi.org/10.1007/s00134-018-05509-6 doi: 10.1007/s00134-018-05509-6
![]() |
[5] |
R. Huang, X. Li, Y. He, W. Zhu, L. Gao, Y. Liu, et al., Recent advances in CAR-T cell engineering, J. Hematol. Oncol., 13 (2020), 1–9. https://doi.org/10.1186/s13045-019-0838-y doi: 10.1186/s13045-019-0838-y
![]() |
[6] |
E. Jacoby, Relapse and resistance to CAR-T cells and blinatumomab in hematologic malignancies, Clin. Hematol. Int., 1 (2019), 79–84. https://doi.org/10.2991/chi.d.190219.001 doi: 10.2991/chi.d.190219.001
![]() |
[7] |
N. Albinger, J. Hartmann, E. Ullrich, Current status and perspective of CAR-T and CAR-NK cell therapy trials in Germany, Gene Ther., (2021), 1–15. https://doi.org/10.1038/s41434-021-00246-w doi: 10.1038/s41434-021-00246-w
![]() |
[8] |
Y. Guo, K. Feng, Y. Wang, W. Han, Targeting cancer stem cells by using chimeric antigen receptor-modified T cells: a potential and curable approach for cancer treatment, Protein cell, 9 (2018), 516–526. https://doi.org/10.1007/s13238-017-0394-6 doi: 10.1007/s13238-017-0394-6
![]() |
[9] |
A. K. Park, Y. Fong, S. I. Kim, J. Yang, J. P. Murad, J. Lu, et al., Effective combination immunotherapy using oncolytic viruses to deliver CAR targets to solid tumors, Sci. Transl. Med., 12 (2020), eaaz1863. https://doi.org/10.1126/scitranslmed.aaz1863 doi: 10.1126/scitranslmed.aaz1863
![]() |
[10] |
D. N. Khalil, E. L. Smith, R. J. Brentjens, J. D. Wolchok, The future of cancer treatment: immunomodulation, CARs and combination immunotherapy, Nat. Rev. Clin. Oncol., 13 (2016), 273–290. https://doi.org/10.1038/nrclinonc.2016.25 doi: 10.1038/nrclinonc.2016.25
![]() |
[11] |
A. Wing, C. A. Fajardo, A. D. J. Posey, C. Shaw, T. Da, R. M. Young, et al., Improving CART-cell therapy of solid tumors with oncolytic virus-driven production of a bispecific T-cell engager, Cancer Immunol. Res., 6 (2018), 605–616. https://doi.org/10.1158/2326-6066.CIR-17-0314 doi: 10.1158/2326-6066.CIR-17-0314
![]() |
[12] |
G. V. Kochneva, G. F. Sivolobova, A. V. Tkacheva, A. A. Gorchakov, S. V. Kulemzin, Combination of oncolytic virotherapy and CAR T/NK cell therapy for the treatment of cancer, Mol. Biol., 54 (2020), 1–12. https://doi.org/10.1134/S0026893320010100 doi: 10.1134/S0026893320010100
![]() |
[13] |
L. Zhao, Y. J. Cao, Engineered T cell therapy for cancer in the clinic, Front. Immunol., 10 (2019), 2250. https://doi.org/10.3389/fimmu.2019.02250 doi: 10.3389/fimmu.2019.02250
![]() |
[14] |
K. J. Mahasa, R. Ouifki, A. Eladdadi, L. de Pillis, Mathematical model of tumor-immune surveillance, J. Theor. Biol., 404 (2016), 312–330. https://doi.org/10.1016/j.jtbi.2016.06.012 doi: 10.1016/j.jtbi.2016.06.012
![]() |
[15] |
R. G. Majzner, C. L. Mackall, Tumor antigen escape from CAR T-cell therapy, Cancer Discovery, 8 (2018), 1219–1226. https://doi.org/10.1158/2159-8290.CD-18-0442 doi: 10.1158/2159-8290.CD-18-0442
![]() |
[16] |
R. C. Sterner, R. M. Sterner, CAR-T cell therapy: current limitations and potential strategies, Blood Cancer J., 11 (2021), 1–11. https://doi.org/10.1038/s41408-021-00459-7 doi: 10.1038/s41408-021-00459-7
![]() |
[17] |
A. R. Yoon, J. Hong, Y. Li, H. C. Shin, H. Lee, H. S. Kim, et al., Mesenchymal stem cell-mediated delivery of an oncolytic adenovirus enhances antitumor efficacy in hepatocellular carcinoma, Cancer Res., 79 (2019), 4503–4514. https://doi.org/10.1158/0008-5472.CAN-18-3900 doi: 10.1158/0008-5472.CAN-18-3900
![]() |
[18] |
K. J. Mahasa, L. de Pillis, R. Ouifki, A. Eladdadi, P. Maini, A. R. Yoon, et al., Mesenchymal stem cells used as carrier cells of oncolytic adenovirus results in enhanced oncolytic virotherapy, Sci. Rep., 10 (2020), 1–13. https://doi.org/10.1038/s41598-019-56847-4 doi: 10.1038/s41598-019-56847-4
![]() |
[19] |
J. Gao, W. Zhang, A. Ehrhardt, Expanding the spectrum of adenoviral vectors for cancer therapy, Cancers, 12 (2020), 1139. https://doi.org/10.3390/cancers12051139 doi: 10.3390/cancers12051139
![]() |
[20] |
L. Russell, K. W. Peng, S. J. Russell, R. M. Diaz, Oncolytic viruses: priming time for cancer immunotherapy, BioDrugs, 33 (2019), 485–501. https://doi.org/10.1007/s40259-019-00367-0 doi: 10.1007/s40259-019-00367-0
![]() |
[21] |
H. L. Kaufman, F. J. Kohlhapp, A. Zloza, Oncolytic viruses: a new class of immunotherapy drugs, Nat. Rev. Drug Discovery, 14 (2015), 642–662. https://doi.org/10.1038/nrd4663 doi: 10.1038/nrd4663
![]() |
[22] | B. Zhang, P. Cheng, Improving antitumor efficacy via combinatorial regimens of oncolytic virotherapy, Mol. Cancer, 19 (2020), 158. |
[23] |
Q. Zhang, F. Liu, Advances and potential pitfalls of oncolytic viruses expressing immunomodulatory transgene therapy for malignant gliomas, Cell Death Dis., 11 (2020), 1–11. https://doi.org/10.1038/s41419-020-2696-5 doi: 10.1038/s41419-020-2696-5
![]() |
[24] |
S. E. Lawler, M. C. Speranza, C. F. Cho, E. A. Chiocca, Oncolytic viruses in cancer treatment: a review, JAMA oncol., 3 (2017), 841–849. https://doi.org/10.1001/jamaoncol.2016.2064 doi: 10.1001/jamaoncol.2016.2064
![]() |
[25] |
A. H. Choi, M. P. O'Leary, Y. Fong, N. G. Chen, From benchtop to bedside: a review of oncolytic virotherapy, Biomed., 4 (2016), 18. https://doi.org/10.3390/biomedicines4030018 doi: 10.3390/biomedicines4030018
![]() |
[26] |
L. Aurelian, Oncolytic viruses as immunotherapy: progress and remaining challenges, OncoTargets Ther., 9 (2016), 2627–2637. https://doi.org/10.2147/OTT.S63049 doi: 10.2147/OTT.S63049
![]() |
[27] |
S. Zuo, M. Wei, B. He, A. Chen, S. Wang, L. Kong, et al., Enhanced antitumor efficacy of a novel oncolytic vaccinia virus encoding a fully monoclonal antibody against T-cell immunoglobulin and ITIM domain (TIGIT), EBioMedicine, 64 (2021), 103240. https://doi.org/10.1016/j.ebiom.2021.103240 doi: 10.1016/j.ebiom.2021.103240
![]() |
[28] |
N. Nishio, G. Dotti, Oncolytic virus expressing RANTES and IL-15 enhances function of CAR-modified T cells in solid tumors, Oncoimmunology, 4 (2015), e988098. https://doi.org/10.4161/21505594.2014.988098 doi: 10.4161/21505594.2014.988098
![]() |
[29] |
E. K. Moon, L. C. S. Wang, K. Bekdache, R. C. Lynn, A. Lo, S. H. Thorne, et al., Intratumoral delivery of CXCL11 via a vaccinia virus, but not by modified T cells, enhances the efficacy of adoptive T cell therapy and vaccines, Oncoimmunology, 7 (2018), e1395997. https://doi.org/10.1080/2162402X.2017.1395997 doi: 10.1080/2162402X.2017.1395997
![]() |
[30] |
N. Nishio, I. Diaconu, H. Liu, V. Cerullo, I. Caruana, V. Hoyos, et al., Armed oncolytic virus enhances immune functions of chimeric antigen receptor–modified T cells in solid tumors, Cancer Res., 74 (2014), 5195–5205. https://doi.org/10.1158/0008-5472.CAN-14-0697 doi: 10.1158/0008-5472.CAN-14-0697
![]() |
[31] | A. Ajina, J. Maher, Prospects for combined use of oncolytic viruses and CAR T cells, J. Immunother. Cancer, 5 (2017), 1–12. |
[32] |
L. Evgin, A. L. Huff, P. Wongthida, J. Thompson, T. Kottke, J. Tonne, et al., Oncolytic virus-derived type I interferon restricts CAR T cell therapy, Nat. Commun., 11 (2020), 1–15. https://doi.org/10.1038/s41467-020-17011-z doi: 10.1038/s41467-020-17011-z
![]() |
[33] | S. Guedan, R. Alemany, CAR-T cells and oncolytic viruses: joining forces to overcome the solid tumor challenge, Front. Immunol., 9 (2018), 2460. |
[34] |
L. Evgin, R. G. Vile, Parking CAR T cells in tumours: oncolytic viruses as valets or vandals, Cancers, 13 (2021), 1106. https://doi.org/10.3390/cancers13051106 doi: 10.3390/cancers13051106
![]() |
[35] |
A. Aalipour, F. L. Boeuf, M. Tang, S. Murty, F. Simonetta, A. X. Lozano, et al., Viral delivery of CAR targets to solid tumors enables effective cell therapy, Mol. Ther. Oncolytics, 17 (2020), 232–240. https://doi.org/10.1016/j.omto.2020.03.018 doi: 10.1016/j.omto.2020.03.018
![]() |
[36] |
L. Evgin, R. G. Vile, Parking CAR T cells in tumours: oncolytic viruses as valets or vandals, Cancers, 13 (2021), 1106. https://doi.org/10.3390/cancers13051106 doi: 10.3390/cancers13051106
![]() |
[37] |
N. Watanabe, M. K. McKenna, A. R. Shaw, M. Suzuki, Clinical CAR-T cell and oncolytic virotherapy for cancer treatment, Mol. The., 29 (2021), 505–520. https://doi.org/10.1016/j.ymthe.2020.10.023 doi: 10.1016/j.ymthe.2020.10.023
![]() |
[38] |
T. Shi, X. Song, Y. Wang, F. Liu, J. Wei, Combining oncolytic viruses with cancer immunotherapy: establishing a New Generation of Cancer Treatment, Front. Immunol., 11 (2020), 683. https://doi.org/10.3389/fimmu.2020.00683 doi: 10.3389/fimmu.2020.00683
![]() |
[39] |
X. Y. Tang, Y. S. Ding, T. Zhou, X. Wang, Y. Yang, Tumor-tagging by oncolytic viruses: A novel strategy for CAR-T therapy against solid tumors, Cancer Lett., 503 (2021), 69–74. https://doi.org/10.1016/j.canlet.2021.01.014 doi: 10.1016/j.canlet.2021.01.014
![]() |
[40] |
L. G. de Pillis, A. Eladdadi, A. E. Radunskaya, Modeling cancer-immune responses to therapy, J. Pharmacokinet. Pharmacodyn., 41 (2014), 461–478. https://doi.org/10.1007/s10928-014-9386-9 doi: 10.1007/s10928-014-9386-9
![]() |
[41] |
R. Walker, H. Enderling, From concept to clinic: mathematically informed immunotherapy, Curr. Probl. Cancer, 40 (2016), 68–83. https://doi.org/10.1016/j.currproblcancer.2015.10.004 doi: 10.1016/j.currproblcancer.2015.10.004
![]() |
[42] |
A. Konstorum, A. T. Vella, A. J. Adler, R. C. Laubenbacher, Addressing current challenges in cancer immunotherapy with mathematical and computational modelling, J. R. Soc. Interface, 14 (2017), 20170150. https://doi.org/10.1098/rsif.2017.0150 doi: 10.1098/rsif.2017.0150
![]() |
[43] | U. Nukala, M. R. Messan, O. N. Yogurtcu, X. Wang, H. Yang, A systematic review of the efforts and hindrances of modeling and simulation of CAR T-cell therapy, AAPS J., 23 (2021), 1–20. |
[44] |
L. R. C. Barros, E. A. Paixão, A. M. P. Valli, G. T. Naozuka, A. C. Fassoni, R. C. Almeida, CARTmath-a mathematical model of CAR-T tmmunotherapy in preclinical studies of hematological cancers, Cancers, 13 (2021), 2941. https://doi.org/10.3390/cancers13122941 doi: 10.3390/cancers13122941
![]() |
[45] |
O. León-Triana, A. Pérez-Martínez, M. Ramírez-Orellana, V. P. érez-García, Dual-target CAR-Ts with on-and off-tumour activity may override immune suppression in solid cancers: a mathematical proof of concept, Cancers, 13 (2021), 703. https://doi.org/10.3390/cancers13040703 doi: 10.3390/cancers13040703
![]() |
[46] | A. Chaudhury, X. Zhu, L. Chu, A. Goliaei, C. H. June, J. D. Kearns, et al., Chimeric antigen receptor T cell therapies: a review of cellular kinetic-pharmacodynamic modeling approaches, J. Clin. Pharmacol., 60 (2020), S147–S159. |
[47] |
P. Sahoo, X. Yang, D. Abler, D. Maestrini, V. Adhikarla, D. Frankhouser, et al., Mathematical deconvolution of CAR T-cell proliferation and exhaustion from real-time killing assay data, J. R. Soc. Interface, 17 (2020), 20190734. https://doi.org/10.1098/rsif.2019.0734 doi: 10.1098/rsif.2019.0734
![]() |
[48] | K. Owens, I. Bozic, Modeling CAR T-cell therapy with patient preconditioning, Bull. Math. Biol., 83 (2021), 1–36. |
[49] | Á. Martínez-Rubio, S. Chulián, C. B. Goñi, M. R. Orellana, A. P. Martínez, A. Navarro-Zapata, et al., A mathematical description of the bone marrow dynamics during CAR T-cell therapy in B-cell childhood acute lymphoblastic leukemia, Int. J. Mol. Sci., 22 (2021), 6371. |
[50] |
K. J. Mahasa, A. Eladdadi, L. de Pillis, R. Ouifki, Oncolytic potency and reduced virus tumor-specificity in oncolytic virotherapy. A mathematical modelling approach, PLoS One, 12 (2017), e0184347. https://doi.org/10.1371/journal.pone.0184347 doi: 10.1371/journal.pone.0184347
![]() |
[51] |
K. M. Storey, S. E. Lawler, T. L. Jackson, Modeling oncolytic viral therapy, immune checkpoint inhibition, and the complex dynamics of innate and adaptive immunity in glioblastoma treatment, Front. Phys., 11 (2020), 151. https://doi.org/10.3389/fphys.2020.00151 doi: 10.3389/fphys.2020.00151
![]() |
[52] |
G. V. R. K. Vithanage, H. C. Wei, S. R. J. Jang, Bistability in a model of tumor-immune system interactions with an oncolytic viral therapy, Math. Biosci. Eng., 19 (2021), 1559–1587. https://doi.org/10.3934/mbe.2022072 doi: 10.3934/mbe.2022072
![]() |
[53] |
J. Malinzi, R. Ouifki, A. Eladdadi, D. F. M. Torres, K. A. J. White, Enhancement of chemotherapy using oncolytic virotherapy: Mathematical and optimal control analysis, Math. Biosci. Eng., 15 (2018), 1435–1463. https://doi.org/10.3934/mbe.2018066 doi: 10.3934/mbe.2018066
![]() |
[54] |
A. Diouf, H. Mokrani, E. Afenya, B. I. Camara, Computation of the conditions for anti-angiogenesis and gene therapy synergistic effects: Sensitivity analysis and robustness of target solutions, J. Theor. Biol., 528 (2021), 110850, https://doi.org/10.1016/j.jtbi.2021.110850 doi: 10.1016/j.jtbi.2021.110850
![]() |
[55] | S. R. J. Jang, H. C. Wei, On a mathematical model of tumor-immune system interactions with an oncolytic virus therapy, Discrete Contin. Dyn. Syst. B, 2021. |
[56] |
R. Walker, P. E. Navas, S. H. Friedman, S. Galliani, A. Karolak, F. Macfarlane, et al., Enhancing synergy of CAR T cell therapy and oncolytic virus therapy for pancreatic cancer, bioRxiv, (2016), 055988. https://doi.org/10.1101/055988 doi: 10.1101/055988
![]() |
[57] | D. Wodarz, Viruses as antitumor weapons: defining conditions for tumor remission, Cancer Res., 61 (2001), 3501–3507. |
[58] | A. L. Jenner, C. O. Yun, A. Yoon, A. C. F. Coster, P. S. Kim, Modelling combined virotherapy and immunotherapy: strengthening the antitumour immune response mediated by IL-12 and GM-CSF expression, Lett. Biomath., 5 (2018), S99–S116. |
[59] |
N. Almuallem, D. Trucu, R. Eftimie, Oncolytic viral therapies and the delicate balance between virus-macrophage-tumour interactions: A mathematical approach, Math. Biosci. Eng., 18 (2021), 764–799. https://doi.org/10.3934/mbe.2021041 doi: 10.3934/mbe.2021041
![]() |
[60] |
A. Friedman, X. Lai, Combination therapy for cancer with oncolytic virus and checkpoint inhibitor: A mathematical model, PLoS One, 13 (2018), e0192449. https://doi.org/10.1371/journal.pone.0192449 doi: 10.1371/journal.pone.0192449
![]() |
[61] |
I. A. Rodriguez-Brenes, A. Hofacre, H. Fan, D. Wodarz, Complex dynamics of virus spread from low infection multiplicities: Implications for the spread of oncolytic viruses, PLoS Comput. Biol., 13 (2017), e1005241. https://doi.org/10.1371/journal.pcbi.1005241 doi: 10.1371/journal.pcbi.1005241
![]() |
[62] | L. R. Paiva, C. Binny, S. C. Ferreira, M. L. Martins, A multiscale mathematical model for oncolytic virotherapy, Cancer Res., 39 (2009), 1205–1211. |
[63] |
D. Wodarz, A. Hofacre, J. W. Lau, Z. Sun, H. Fan, N. L. Komarova, Complex spatial dynamics of oncolytic viruses in vitro: mathematical and experimental approaches, PLoS Comput. Biol., 8 (2012), e1002547. https://doi.org/10.1371/journal.pcbi.1002547 doi: 10.1371/journal.pcbi.1002547
![]() |
[64] |
A. L. Jenner, A. C. F. Coster, P. S. Kim, F. Frascoli, Treating cancerous cells with viruses: insights from a minimal model for oncolytic virotherapy, Lett. Biomath., 5 (2018), S117–S136. https://doi.org/10.30707/LiB5.2Jenner doi: 10.30707/LiB5.2Jenner
![]() |
[65] | J. P. W. Heidbuechel, D. Abate-Daga, C. E. Engeland, H. Enderling, Mathematical modeling of oncolytic virotherapy, in Oncolytic viruses, methods in molecular biology, Springer, Humana, New York, (2020), 301–320. |
[66] |
N. S. Senekal, K. J. Mahasa, A. Eladdadi, L. de Pillis, R. Ouifki, Natural killer cells recruitment in oncolytic virotherapy: a mathematical model, Bull. Math. Biol., 83 (2021), 1–51. https://doi.org/10.1007/s11538-021-00903-6 doi: 10.1007/s11538-021-00903-6
![]() |
[67] | J. King, K. S. Eroumé, R. Truckenmüller, S. Giselbrecht, A. E. Cowan, L. Loew, Ten steps to investigate a cellular system with mathematical modeling, PLoS Comput. Biol., 17 (2021), e1008921. |
[68] |
L. G. de Pillis, A. E. Radunskaya, C. L. Wiseman, A validated mathematical model of cell-mediated immune response to tumour growth, Cancer Res., 65 (2005), 7950–7958. https://doi.org/10.1158/0008-5472.CAN-05-0564 doi: 10.1158/0008-5472.CAN-05-0564
![]() |
[69] |
D. Phan, D. Wodarz, Modeling multiple infection of cells by viruses: challenges and insights, Math. Biosci., 264 (2015), 21–28. https://doi.org/10.1016/j.mbs.2015.03.001 doi: 10.1016/j.mbs.2015.03.001
![]() |
[70] |
C. Grassberger, D. McClatchy, C. Geng, S. C. Kamran, F. Fintelmann, Y. E. Maruvka, et al., Patient-specific tumor growth trajectories determine persistent and resistant cancer cell populations during treatment with targeted therapies, Cancer Res., 79 (2019), 3776–3788. https://doi.org/10.1158/0008-5472.CAN-18-3652 doi: 10.1158/0008-5472.CAN-18-3652
![]() |
[71] |
A. L. Jenner, T. Cassidy, K. Belaid, M. C. Bourgeois-Daigneault, M. Craig, In silico trials predict that combination strategies for enhancing vesicular stomatitis oncolytic virus are determined by tumor aggressivity, J. Immunother. Cancer, 9 (2021), e001387. https://doi.org/10.1136/jitc-2020-001387 doi: 10.1136/jitc-2020-001387
![]() |
[72] | H. Enderling, O. Wolkenhauer, Are all models wrong? Comput. Syst., 1 (2020), e1008. https: //doi.org/10.1002/cso2.1008 |
[73] |
P. S. Kim, J. J. Crivelli, I. K. Choi, C. O. Yun, J. R. Wares, Quantitative impact of immunomodulation versus oncolysis with cytokine-expressing virus therapeutics, Math. Biosci. Eng., 12 (2015), 841–858. https://doi.org/10.1002/cbdv.201400024 doi: 10.1002/cbdv.201400024
![]() |
[74] |
J. J. Crivelli, J. Földes, P. S. Kim, J. R. Wares, A mathematical model for cell cycle-specific cancer virotherapy, J. Biol. Dyn., 6 (2012), 104–120. https://doi.org/10.1080/17513758.2011.613486 doi: 10.1080/17513758.2011.613486
![]() |
[75] |
A. L. Jenner, P. S. Kim, F. Frascoli, Oncolytic virotherapy for tumours following a Gompertz growth law, J. Theor. Biol., 480 (2019), 129–140. https://doi.org/10.1016/j.jtbi.2019.08.002 doi: 10.1016/j.jtbi.2019.08.002
![]() |
[76] |
H. Huang, Y. Liu, W. Liao, Y. Cao, Q. Liu, Y. Guo, et al., Oncolytic adenovirus programmed by synthetic gene circuit for cancer immunotherapy, Nat. Commun., 10 (2019), 1–15. https://doi.org/10.1038/s41467-019-12794-2 doi: 10.1038/s41467-019-12794-2
![]() |
[77] |
M. Ruella, M. Klichinsky, S. S. Kenderian, O. Shestova, A. Ziober, D. O. Kraft, et al., Overcoming the immunosuppressive tumor microenvironment of Hodgkin lymphoma using chimeric antigen receptor T cells, Cancer Discovery, 7 (2017), 1154–1167. https://doi.org/10.1158/2159-8290.CD-16-0850 doi: 10.1158/2159-8290.CD-16-0850
![]() |
[78] |
A. Friedman, J. P. Tian, G. Fulci, E. A. Chiocca, J. Wang, Glioma virotherapy: effects of innate immune suppression and increased viral replication capacity, Cancer Res., 66 (2006), 2314–2319. https://doi.org/10.1158/0008-5472.CAN-05-2661 doi: 10.1158/0008-5472.CAN-05-2661
![]() |
[79] |
R. Eftimie, C. K. Macnamara, J. Dushoff, J. L. Bramson, D. J. Earn, Bifurcations and chaotic dynamics in a tumour-immune-virus system, Math. Modell. Nat. Phenom., 11 (2016), 65–85. https://doi.org/10.1051/mmnp/201611505 doi: 10.1051/mmnp/201611505
![]() |
[80] |
R. Eftimie, G. Eftimie, Investigating macrophages plasticity following tumour–immune interactions during oncolytic therapies, Acta Biotheor., 67 (2019), 321–359. https://doi.org/10.1007/s10441-019-09357-9 doi: 10.1007/s10441-019-09357-9
![]() |
[81] |
Ž Bajzer, T. Carr, K. Josić, S. J. Russell, D. Dingli, Modeling of cancer virotherapy with recombinant measles viruses, J. Theor. Biol., 252 (2008), 109–122. https://doi.org/10.1016/j.jtbi.2008.01.016 doi: 10.1016/j.jtbi.2008.01.016
![]() |
[82] |
K. Jacobsen, L. Russell, B. Kaur, A. Friedman, Effects of CCN1 and macrophage content on glioma virotherapy: a mathematical model, Bull. Math. Biol., 77 (2015), 1–29. https://doi.org/10.1007/s11538-014-0046-4 doi: 10.1007/s11538-014-0046-4
![]() |
[83] |
M. Aghi, R. L. Martuza, Oncolytic viral therapies–the clinical experience, Oncogene, 24 (2005), 7802–7816. https://doi.org/10.1038/sj.onc.1209037 doi: 10.1038/sj.onc.1209037
![]() |
[84] | A. Saltelli, M. Ratto, T. Andres, F. Campolongo, J. Cariboni, D. Gatelli, et al., Global Sensitivity Analysis: the Primer, Wiley, New York, 2008. |
[85] |
B. Niu, X. Zeng, T. A. Phan, F. Szulzewsky, S. Holte, E. C. Holland, et al., Mathematical modeling of PDGF-driven glioma reveals the dynamics of immune cells infiltrating into tumors, Neoplasia, 22 (2020), 323–332. https://doi.org/10.1016/j.neo.2020.05.005 doi: 10.1016/j.neo.2020.05.005
![]() |
[86] |
A. L. Jenner, C. O. Yun, P. S. Kim, A. C. F. Coster, Mathematical modelling of the interaction between cancer cells and an oncolytic virus: insights into the effects of treatment protocols, Bull. Math. Biol., 80 (2018), 1615–1629. https://doi.org/10.1007/s11538-018-0424-4 doi: 10.1007/s11538-018-0424-4
![]() |
[87] |
T. Krabbe, J. Marek, T. Groll, K. Steiger, R. M. Schmid, A. M. Krackhardt, et al., Adoptive T cell therapy is complemented by oncolytic virotherapy with fusogenic VSV-NDV in combination treatment of murine melanoma, Cancers, 13 (2021), 1044. https://doi.org/10.3390/cancers13051044 doi: 10.3390/cancers13051044
![]() |
[88] |
Z. P. Parra-Guillen, T. Freshwater, Y. Cao, K. Mayawala, S. Zalba, M. J. Garrido, et al., Mechanistic modeling of a novel oncolytic virus, V937, to describe viral kinetic and dynamic processes following intratumoral and intravenous administration, Front. Pharmacol., 12 (2021), 705443. https://doi.org/10.3389/fphar.2021.705443 doi: 10.3389/fphar.2021.705443
![]() |
[89] |
A. Mueller-Schoell, N. Puebla-Osorio, R. Michelet, M. R. Green, A. Künkele, W. Huisinga, et al., Early survival prediction framework in CD19-specific CAR-T cell immunotherapy using a quantitative systems pharmacology model, Cancers, 13 (2021), 2782. https://doi.org/10.3390/cancers13112782 doi: 10.3390/cancers13112782
![]() |
[90] |
G. V. R. K. Vithanage, H. C. Wei, S. R. J. Jang, Bistability in a model of tumor-immune system interactions with an oncolytic viral therapy, Math. Biosci. Eng., 19 (2021), 1559–1587. https://doi.org/10.3934/mbe.2022072 doi: 10.3934/mbe.2022072
![]() |
[91] |
A. Takasu, A. Masui, M. Hamada, T. Imai, S. Iwai, Y. Yura, Immunogenic cell death by oncolytic herpes simplex virus type 1 in squamous cell carcinoma cells, Cancer Gene Ther., 23 (2016), 107–113. https://doi.org/10.5194/npg-23-107-2016 doi: 10.5194/npg-23-107-2016
![]() |
[92] |
P. K. Bommareddy, A. Zloza, S. D. Rabkin, H. L. Kaufman, Oncolytic virus immunotherapy induces immunogenic cell death and overcomes STING deficiency in melanoma, OncoImmunology, 8 (2019), e1591875. https://doi.org/10.1080/2162402X.2019.1591875 doi: 10.1080/2162402X.2019.1591875
![]() |
[93] |
J. P. van Vloten, S. T. Workenhe, S. K. Wootton, K. L. Mossman, B. W. Bridle, Critical interactions between immunogenic cancer cell death, oncolytic viruses, and the immune system define the rational design of combination immunotherapies, J. Immunol., 200 (2018), 450–458. https://doi.org/10.4049/jimmunol.1701021 doi: 10.4049/jimmunol.1701021
![]() |
[94] |
D. Wodarz, N. Komarova, Towards predictive computational models of oncolytic virus therapy: basis for experimental validation and model selection, PLoS One, 4 (2009), e4271. https://doi.org/10.1371/journal.pone.0004271 doi: 10.1371/journal.pone.0004271
![]() |
[95] |
T. J. Paul, The replicability of oncolytic virus: defining conditions in tumor virotherapy, Math. Biosci. Eng., 8 (2011), 841–860. https://doi.org/10.3934/mbe.2011.8.841 doi: 10.3934/mbe.2011.8.841
![]() |
[96] |
P. Pooladvand, C. O. Yun, A. R. Yoon, P. S. Kim, F. Frascoli, The role of viral infectivity in oncolytic virotherapy outcomes: A mathematical study, Math. Biosci., 334 (2021), 108520. https://doi.org/10.1016/j.mbs.2020.108520 doi: 10.1016/j.mbs.2020.108520
![]() |
[97] |
S. T. Workenhe, G. Simmons, J. G. Pol, B. D. Lichty, W. P. Halford, K. L. Mossman, Immunogenic HSV-mediated oncolysis shapes the antitumor immune response and contributes to therapeutic efficacy, Mol. Ther., 22 (2014), 123–131. https://doi.org/10.1038/mt.2013.238 doi: 10.1038/mt.2013.238
![]() |
[98] |
O. J. Kwon, E. Kang, S. Kim, C. O. Yun, Viral genome DNA/lipoplexes elicit in situ oncolytic viral replication and potent antitumor efficacy via systemic delivery, J. Controlled Release, 155 (2011), 317–325. https://doi.org/10.1016/j.jconrel.2011.06.014 doi: 10.1016/j.jconrel.2011.06.014
![]() |
[99] |
A. Mohamed, R. N. Johnston, M. Shmulevitz, Potential for improving potency and specificity of reovirus oncolysis with next-generation reovirus variants, Viruses, 7 (2015), 6251–6278. https://doi.org/10.3390/v7122936 doi: 10.3390/v7122936
![]() |
[100] |
J. Altomonte, L. Wu, M. Meseck, L. Chen, O. Ebert, A. Garcia-Sastre, et al., Enhanced oncolytic potency of vesicular stomatitis virus through vector-mediated inhibition of NK and NKT cells, Cancer Gene Ther., 16 (2009), 266–278. https://doi.org/10.1038/cgt.2008.74 doi: 10.1038/cgt.2008.74
![]() |
[101] |
C. A. Alvarez-Breckenridge, J. Yu, R. Price, J. Wojton, J. Pradarelli, H. Mao, et al., NK cells impede glioblastoma virotherapy through NKp30 and NKp46 natural cytotoxicity receptors, Nat. Med., 18 (2012), 1827–1834. https://doi.org/10.1038/nm.3013 doi: 10.1038/nm.3013
![]() |
[102] |
A. L. de Matos, L. S. Franco, G. McFadden, Oncolytic viruses and the immune system: the dynamic duo, Mol. Ther. Methods Clin. Dev., 17 (2020), 349–358. https://doi.org/10.1016/j.omtm.2020.01.001 doi: 10.1016/j.omtm.2020.01.001
![]() |
[103] |
F. Marofi, R. Motavalli, V. A. Safonov, L. Thangavelu, A. V. Yumashev, M. Alexander, et al., CAR T cells in solid tumors: challenges and opportunities, Stem Cell Res. Ther., 12 (2021), 1–16. https://doi.org/10.1186/s13287-020-02006-w doi: 10.1186/s13287-020-02006-w
![]() |
[104] |
S. Kailayangiri, B. Altvater, M. Wiebel, S. Jamitzky, Overcoming heterogeneity of antigen expression for effective CAR T cell targeting of cancers, Cancers, 12 (2020), 1075. https://doi.org/10.3390/cancers12051075 doi: 10.3390/cancers12051075
![]() |
[105] | N. V. Frey, P. A. Shaw, E. O. Hexner, S. Gill, K. Marcucci, S. M. Luger, et al., Optimizing chimeric antigen receptor (CAR) T cell therapy for adult patients with relapsed or refractory (r/r) acute lymphoblastic leukemia (ALL), J. Clin. Oncol., 34 (2016), 7002. |
[106] |
J. P. W. Heidbuechel, C. E. Engeland, Oncolytic viruses encoding bispecific T cell engagers: a blueprint for emerging immunovirotherapies, J. Hematol. Oncol., 14 (2021), 1–24. https://doi.org/10.1186/s13045-021-01075-5 doi: 10.1186/s13045-021-01075-5
![]() |
[107] |
A. Wing, C. A. Fajardo, A. D. Posey, C. Shaw, T. Da, R. M. Young, et al., Improving CART-cell therapy of solid tumors with oncolytic virus–driven production of a bispecific T-cell engager, Cancer Immunol. Res., 6 (2018), 605–616. https://doi.org/10.1158/2326-6066.CIR-17-0314 doi: 10.1158/2326-6066.CIR-17-0314
![]() |
[108] |
O. P. Nave, M. Elbaz, S. Bunimovich-Mendrazitsky, Analysis of a breast cancer mathematical model by a new method to find an optimal protocol for HER2-positive cancer, Biosystems, 197 (2020), 104191. https://doi.org/10.1016/j.biosystems.2020.104191 doi: 10.1016/j.biosystems.2020.104191
![]() |
[109] |
O. P. Nave, S. Hareli, M. Elbaz, I. H. Iluz, S. Bunimovich-Mendrazitsky, BCG and IL - 2 model for bladder cancer treatment with fast and slow dynamics based on SPVF method–stability analysis, Math. Biosci. Eng., 16 (2019), 5346–5379. https://doi.org/10.3934/mbe.2019267 doi: 10.3934/mbe.2019267
![]() |
[110] |
S. Marino, I. B. Hogue, C. J. Ray, D. E. Kirschner, A methodology for performing global uncertainty and sensitivity analysis in systems biology, J. Theor. Biol., 254 (2008), 178–196. https://doi.org/10.1016/j.jtbi.2008.04.011 doi: 10.1016/j.jtbi.2008.04.011
![]() |
1. | Lu Gao, Yuanshun Tan, Jin Yang, Changcheng Xiang, Dynamic analysis of an age structure model for oncolytic virus therapy, 2022, 20, 1551-0018, 3301, 10.3934/mbe.2023155 | |
2. | G. V. R. K. Vithanage, Hsiu‐Chuan Wei, Sophia R‐J Jang, The role of tumor activation and inhibition with saturation effects in a mathematical model of tumor and immune system interactions undergoing oncolytic viral therapy, 2023, 0170-4214, 10.1002/mma.9152 | |
3. | Mohamed Ch-Chaoui, Karima Mokni, ASYMPTOTIC ANALYSIS OF AN INTEGRO-DIFFERENTIAL SYSTEM MODELING THE BLOW UP OF CANCER CELLS UNDER THE IMMUNE RESPONSE, 2022, 12, 2156-907X, 1763, 10.11948/20210296 | |
4. | Hsiu-Chuan Wei, Mathematical modeling of tumor growth and treatment: Triple negative breast cancer, 2023, 204, 03784754, 645, 10.1016/j.matcom.2022.09.005 | |
5. | Xuejin Gao, Jile Liu, Rui Sun, Jingkun Zhang, Xinping Cao, Yi Zhang, Mingfeng Zhao, Alliance between titans: combination strategies of CAR-T cell therapy and oncolytic virus for the treatment of hematological malignancies, 2024, 103, 0939-5555, 2569, 10.1007/s00277-023-05488-9 | |
6. | Jing Zhang, Jia Chen, Xiuqing Yang, Jing Han, Xiaofeng Chen, Yueping Fan, Hui Zheng, Novel risk prediction models, involving coagulation, thromboelastography, stress response, and immune function indicators, for deep vein thrombosis after radical resection of cervical cancer and ovarian cancer, 2023, 43, 0144-3615, 10.1080/01443615.2023.2204162 | |
7. |
Khaphetsi Joseph Mahasa, Rachid Ouifki, Lisette de Pillis, Amina Eladdadi,
A Role of Effector CD8+ T Cells Against Circulating Tumor Cells Cloaked with Platelets: Insights from a Mathematical Model,
2024,
86,
0092-8240,
10.1007/s11538-024-01323-y
|
|
8. | Abeer S. Alnahdi, Muhammad Idrees, Nonlinear dynamics of estrogen receptor-positive breast cancer integrating experimental data: A novel spatial modeling approach, 2023, 20, 1551-0018, 21163, 10.3934/mbe.2023936 | |
9. | Zinnia P. Parra‐Guillen, Aymara Sancho‐Araiz, Kapil Mayawala, Sara Zalba, Maria J. Garrido, Dinesh de Alwis, Iñaki F. Troconiz, Tomoko Freshwater, Assessment of Clinical Response to V937 Oncolytic Virus After Intravenous or Intratumoral Administration Using Physiologically‐Based Modeling, 2023, 114, 0009-9236, 623, 10.1002/cpt.2937 | |
10. | Soukaina Sabir, Odelaisy León-Triana, Sergio Serrano, Roberto Barrio, Victor M. Pérez-García, Mathematical Model of CAR T-Cell Therapy for a B-Cell Lymphoma Lymph Node, 2025, 87, 0092-8240, 10.1007/s11538-025-01417-1 |
Parameter | Description | Baseline value | Units | References |
r | Growth rate of uninfected tumor cells | 0.206 | day−1 | [81] |
K | Tumor carrying capacity | 1.404×108(107−109) | cells | [51,66,79] |
β | Infection rate of uninfected tumor cells by free viruses | 4.7×10−4(0.0008−0.008) | (PFU−1)(day−1) | [79], Assumed |
δT | Killing rate of tumor cells by CAR-T cells | 10−7 | (cells⋅day)−1 | Assumed |
ϵ | Virus-induced synergistic rate | 10−2 | PFU−1 | Assumed |
ξV | Virus-induced CAR-T cell recruitment rate | 10−8 | (PFU−1)(day−1) | Assumed |
lv | Lysis rate of infected tumor cells | 0.4(0.0034−11) | day−1 | [82] |
b | Viruses released by lysed infected tumor cell | 3×103(10−1,350) | (PFU)(cells⋅day)−1 | [78] |
ω | Viral clearance rate | 0.6 | day−1 | [78] |
γT | Viral clearance rate by CAR-T cells | 2 | day−1 | Assumed |
αT | Inactivation rate of CAR-T cells by tumor cells | 0.266(0.01−0.99) | (cells⋅day)−1 | [45] |
ρT | Proliferation rate of CAR-T cells | 0.265(0.2−0.9) | (cells⋅day)−1 | [44,45] |
λV | Initial virus inoculum | 2×106(103−1010) | (PFU)(cell⋅day)−1 | [83] |
λCT | Adaptive CAR-T cell therapy | 2×106 | cells⋅day−1 | [44,77] |
μT | Natural death of CAR-T cells | 0.350 | day−1 | [44] |
Parameter | Description | Baseline value | Units | References |
r | Growth rate of uninfected tumor cells | 0.206 | day−1 | [81] |
K | Tumor carrying capacity | 1.404×108(107−109) | cells | [51,66,79] |
β | Infection rate of uninfected tumor cells by free viruses | 4.7×10−4(0.0008−0.008) | (PFU−1)(day−1) | [79], Assumed |
δT | Killing rate of tumor cells by CAR-T cells | 10−7 | (cells⋅day)−1 | Assumed |
ϵ | Virus-induced synergistic rate | 10−2 | PFU−1 | Assumed |
ξV | Virus-induced CAR-T cell recruitment rate | 10−8 | (PFU−1)(day−1) | Assumed |
lv | Lysis rate of infected tumor cells | 0.4(0.0034−11) | day−1 | [82] |
b | Viruses released by lysed infected tumor cell | 3×103(10−1,350) | (PFU)(cells⋅day)−1 | [78] |
ω | Viral clearance rate | 0.6 | day−1 | [78] |
γT | Viral clearance rate by CAR-T cells | 2 | day−1 | Assumed |
αT | Inactivation rate of CAR-T cells by tumor cells | 0.266(0.01−0.99) | (cells⋅day)−1 | [45] |
ρT | Proliferation rate of CAR-T cells | 0.265(0.2−0.9) | (cells⋅day)−1 | [44,45] |
λV | Initial virus inoculum | 2×106(103−1010) | (PFU)(cell⋅day)−1 | [83] |
λCT | Adaptive CAR-T cell therapy | 2×106 | cells⋅day−1 | [44,77] |
μT | Natural death of CAR-T cells | 0.350 | day−1 | [44] |