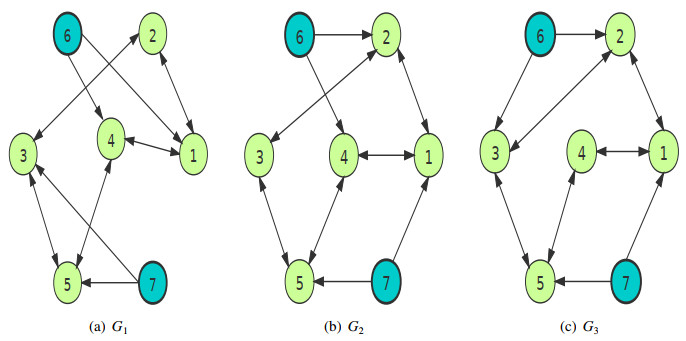
The multi-leader-follower group consensus issue of a class of stochastic time-delay multi-agent systems subject to Markov switching topology is investigated. The purpose is to determine a distributed control protocol to make sure that the followers' states converge in mean square to a convex hull generated by the leaders' states. Through a model transformation, the problem is transformed into a mean-square stability issue of a new system. Then, an easy-to-check sufficient condition for the solvability of the multi-leader-follower group consensus issue is proposed by utilizing the Lyapunov stability theory, graph theory, as well as several inequality techniques. It is shown that the required feedback gain can be acquired once the condition is satisfied. Finally, an example is used to illustrate the effectiveness of the control protocol.
Citation: Tong Guo, Jing Han, Cancan Zhou, Jianping Zhou. Multi-leader-follower group consensus of stochastic time-delay multi-agent systems subject to Markov switching topology[J]. Mathematical Biosciences and Engineering, 2022, 19(8): 7504-7520. doi: 10.3934/mbe.2022353
[1] | Jiangtao Dai, Ge Guo . A leader-following consensus of multi-agent systems with actuator saturation and semi-Markov switching topologies. Mathematical Biosciences and Engineering, 2024, 21(4): 4908-4926. doi: 10.3934/mbe.2024217 |
[2] | Duoduo Zhao, Fang Gao, Jinde Cao, Xiaoxin Li, Xiaoqin Ma . Mean-square consensus of a semi-Markov jump multi-agent system based on event-triggered stochastic sampling. Mathematical Biosciences and Engineering, 2023, 20(8): 14241-14259. doi: 10.3934/mbe.2023637 |
[3] | Na Zhang, Jianwei Xia, Tianjiao Liu, Chengyuan Yan, Xiao Wang . Dynamic event-triggered adaptive finite-time consensus control for multi-agent systems with time-varying actuator faults. Mathematical Biosciences and Engineering, 2023, 20(5): 7761-7783. doi: 10.3934/mbe.2023335 |
[4] | Xiaofeng Chen . Double-integrator consensus for a switching network without dwell time. Mathematical Biosciences and Engineering, 2023, 20(7): 11627-11643. doi: 10.3934/mbe.2023516 |
[5] | Wangming Lu, Zhiyong Yu, Zhanheng Chen, Haijun Jiang . Prescribed-time cluster practical consensus for nonlinear multi-agent systems based on event-triggered mechanism. Mathematical Biosciences and Engineering, 2024, 21(3): 4440-4462. doi: 10.3934/mbe.2024196 |
[6] | Qiushi Wang, Hongwei Ren, Zhiping Peng, Junlin Huang . Dynamic event-triggered consensus control for nonlinear multi-agent systems under DoS attacks. Mathematical Biosciences and Engineering, 2024, 21(2): 3304-3318. doi: 10.3934/mbe.2024146 |
[7] | Yan-Xiao Liu , Ching-Nung Yang, Qin-Dong Sun . Enhance embedding capacity for switch map based multi-group EMD data hiding. Mathematical Biosciences and Engineering, 2019, 16(5): 3382-3392. doi: 10.3934/mbe.2019169 |
[8] | Yuting Zhu, Wenyu Zhang, Junjie Hou, Hainan Wang, Tingting Wang, Haining Wang . The large-scale group consensus multi-attribute decision-making method based on probabilistic dual hesitant fuzzy sets. Mathematical Biosciences and Engineering, 2024, 21(3): 3944-3966. doi: 10.3934/mbe.2024175 |
[9] | Mingxia Gu, Zhiyong Yu, Haijun Jiang, Da Huang . Distributed consensus of discrete time-varying linear multi-agent systems with event-triggered intermittent control. Mathematical Biosciences and Engineering, 2024, 21(1): 415-443. doi: 10.3934/mbe.2024019 |
[10] | Meiqiao Wang, Wuquan Li . Distributed adaptive control for nonlinear multi-agent systems with nonlinear parametric uncertainties. Mathematical Biosciences and Engineering, 2023, 20(7): 12908-12922. doi: 10.3934/mbe.2023576 |
The multi-leader-follower group consensus issue of a class of stochastic time-delay multi-agent systems subject to Markov switching topology is investigated. The purpose is to determine a distributed control protocol to make sure that the followers' states converge in mean square to a convex hull generated by the leaders' states. Through a model transformation, the problem is transformed into a mean-square stability issue of a new system. Then, an easy-to-check sufficient condition for the solvability of the multi-leader-follower group consensus issue is proposed by utilizing the Lyapunov stability theory, graph theory, as well as several inequality techniques. It is shown that the required feedback gain can be acquired once the condition is satisfied. Finally, an example is used to illustrate the effectiveness of the control protocol.
In practical applications of a multi-agent system (MAS), there will be some agents that are the tracking targets of other agents. These agents are commonly called leaders and the other agents in the MAS are called followers accordingly. Leader-following consensus has attracted much interest due to its wide application in formation flight, sensor networks, and other engineering areas [1,2,3]. In the case of a single leader, the leader-following issue can be summarized as follows: how to construct an appropriate control protocol (or algorithm) that is able to make the followers' states in the system track the state of the leader. Tang et al. [4] designed a distributed coordination protocol on the basis of a sampled-data control scheme to solve the leader-following consensus problem. Wang and Ji [5] considered directed communication topology and gave leader-follower consensus conditions for general linear MASs. In Reference [6], Jiang et al. proposed a non-fragile sampled-data control protocol, under which the H∞ leader-following consensus is reached in the presence of the Lipschitz nonlinearity. To address the leader-following consensus under cyber attacks, Liu et al. [7] proposed an event-triggered control consensus protocol.
As for the multiple leaders' case, the purpose of consensus control is often to make followers' states go into the region generated by the states of these leaders. This type of consensus is called multi-leader-follower (MLF) group consensus (or containment consensus). In the case when there are multiple leaders, Ji et al. [8] proposed hybrid stop-go schemes to ensure the MLF group consensus. In Reference [9], Ma et al. checked the MLF group consensus of MASs modeled by discrete-time equations and presented several distributed control protocol designs based on either state feedback or output feedback. In Reference [10], Wang et al. considered a class of high-order MASs and proposed a novel distributed observer-based MLF group consensus control protocol. For recent work on MLF group consensus, one can refer to References [11,12,13,14,15,16,17] and papers cited therein.
Although significant progress has been made in the area of MLF group consensus of MASs, there are two issues that need to be investigated further. On the one hand, the agents under consideration in most of the existing literature are assumed to have deterministic delay-free dynamic behaviors. As noted in References [18,19,20], however, sometimes it is necessary to use stochastic or functional differential equations to model the dynamics of agents in engineering applications. On the other hand, most studies are based on a fixed communication topology. In practice, however, the communication topology between agents may change greatly with time, and the next topology often only depends on the current topology (see e.g., References [21,22,23]). In view of this, compared with the fixed topology, the Markov switching topology seems to be a better candidate.
Motivated by the above observations, this paper focuses on the MLF group consensus issue for stochastic time-delay MASs subject to Markov switching topology. Unlike the existing literature on this issue, the system considered here is assumed to be perturbed by Brownian motion and the communication topology between agents is assumed to be dynamically changing but obey a continuous-time finite-state Markov chain. The purpose is to determine a distributed control protocol to make sure that the followers' states converge in mean square to a convex hull generated by the leaders' states. The major contributions of this work are two-fold: 1) By introducing a model transformation, the MLF group consensus is transformed into a mean-square stability issue of a new system; 2) By utilizing the Lyapunov stability theory, graph theory, as well as several inequality techniques, an easy-to-check sufficient condition is proposed, under which the required feedback gain can be easily acquired.
Notations: In this paper, Rq and Rp×q denote the q-dimensional Euclidean space as well as the family of all p×q real matrices, respectively. The symbol ‖⋅‖ stands for the Euclidean norm and ⊗ represents the Kronecker product. For a matrix M∈Rp×p, let λM(M) and λm(M) denote the maximum and minimum eigenvalues of matrix M respectively, and S(M) be the sum of M itself and its transpose [24]. Set T⊂Rq is called convex if (1−σ)γ+σρ ∈ T for any γ,ρ∈T and σ∈[0,1]. For a set O={o1,o2,⋯,or}, oi∈Rq, i=1,2,⋯,r, denote its convex hull by co{O}={Σri=1pioi|pi≥0,Σri=1pi=1}. For a vector v=[v1,⋯,vm]T (respectively, a matrix U=(uij)m×n), denote its 1 norm by ‖v‖1=∑ni=1|vi| (respectively, ‖U‖1=max1≤j≤n∑ni=1|uij|) and ∞ norm by ‖v‖∞=max1≤i≤n|vi| (respectively, ‖U‖∞=‖UT‖1=max1≤i≤n∑nj=1|uij|). For column vectors u1,⋯,um, denote [uT1,⋯,uTm]T as col{u1,⋯,um}.
Moreover, let G=(V,E,A) be a directed graph representing the communication topology of the MAS, V={v1,v2,⋯,vn+m} be the node set, E={eji:eji=(vj,vi)}⊆V×V be the edge set, and A=[aij] be the adjacency matrix with aij=1 for eji∈ E and aij=0 for eji∉ E. For eji∈E, vj is viewed as a neighbor of vi. A node is called a leader if it has no neighbors, and a follower if it has at least one neighbor. Define L=[lij]∈R(n+m)×(n+m) as the Laplacian matrix of G, where lij=− aij for i≠j and lii=Σnj=1,j≠i aij. Denote by s the number of possible interaction topologies, and st:[0,∞]→S={1,⋯,s} a switching signal that governs the switches between the variable topologies. Then, the directed graph G at time t is defined as Gst=(Vst,Est,Ast). Simultaneously, the adjacency matrix and the Laplacian matrix can be re-defined as Ast=[astij] and Lst=[lstij], respectively. Assume that there are m leaders and n followers. Then Lst can be partitioned as:
Lst=[Lst1Lst20m×n0m×m], |
where
Lst1=[lst11lst12⋯lst1nlst21lst22⋯lst2n⋮⋯⋯⋮lstn1lstn2⋯lstnn],Lst2=[lst1,n+1lst1,n+2⋯lst1,n+mlst2,n+1lst2,n+2⋯lst2,n+m⋮⋯⋯⋮lstn,n+1lstn,n+2⋯lstn,n+m]. | (2.1) |
Consider a class of MAS driven by Brownian motion. The dynamic process of the i-th follower affected by random disturbance is described as follows:
˙xi(t)=Axi(t)+Aτxi(t−τ)+Bui(t)+Cxi(t)η(t), i∈N1, | (2.2) |
in which xi(t)∈Rm1 is the state of the i-th follower, ui(t)∈Rm2 is the control input, η(t)∈R is the one-dimensional standard white noise, A∈Rm1×m1, Aτ∈Rm1×m1, B∈Rm1×m2, and C∈Rm1×m1 are constant matrices, τ stands for a constant time delay [25,26], and N1={1,2,⋯,n} denotes the set of indices for the followers. One can rewrite system (2.2) as the following Itô stochastic differential equation:
dxi(t)=(Axi(t)+Aτxi(t−τ)+Bui(t))dt+Cxi(t)dω(t), i∈N1, | (2.3) |
in which ω(t) denotes a standard Brownian motion defined on a complete probability space (Ω,Ft,P) [27]. The i-th leader's dynamic equation is as follows:
˙xi(t)=Axi(t)+Aτxi(t−τ)+Bfi(xi(t),t)+Cxi(t)η(t), i∈N2, | (2.4) |
where N2={n+1,⋯,n+m} is the set of indices for the leaders, xi(t)∈Rm1 denotes the leader's state, and fi(xi(t),t)∈Rm2 stands for a pre-designed control input. It is assumed that the following conditions are met:
||fi(xi(t),t)||∞≤ε, i∈N2, | (2.5) |
where ε >0. System (2.4) can also be rewritten in the standard Itô differential form as:
dxi(t)=(Axi(t)+Aτxi(t−τ)+Bfi(xi(t),t))dt+Cxi(t)dω(t), i∈N2. | (2.6) |
It should be noted that the form of random disturbance considered in systems (2.3) and (2.6) is state-dependent, which means that the disturbance is not entirely normal exterior noise.
Suppose that {st,t≥0} is a continuous-time finite-time Markov chain that chooses values in S={1,...,s} according to transition probability
P{st+ζ=j|st=i}={πijζ+o(ζ), i≠j1+πijζ+o(ζ), i=j |
where ζ>0, limζ→0(o(ζ)/ζ)=0, and πij≥0 (for i≠j) is the transition rate from mode i at time t to mode j at time t+ζ and πii=−Σsj=1,j≠iπij [28,29,30].
For the analysis and synthesis of stochastic MASs which are given in Eqs (2.3) and (2.6), we make the following assumptions for deriving our main results.
Assumption 1. For every follower i∈N1, there is at least one leader j∈N2 that has a directed path from j to i.
Assumption 2. At any time t, the communication topology among followers is an undirected connected graph.
In the present work we proposed a distributed control protocol as follows:
ui(t)=αKstξi(t)+βsgn(Kstξi(t)), i∈N1, | (2.7) |
where α and β are positive scalars, and Kst∈Rm2×m1 is the feedback gain. The specific form of ξi(t) is as follows:
ξi(t)=Σj∈N1∪N2astij(xi(t)−xj(t)), i∈N1. | (2.8) |
Remark 1 Assumptions 1 and 2 have been broadly adopted in the existing literature on the MLF group consensus issue (see, e.g., [12,13,15,31]). It follows from Assumption 1 that each follower is reachable from its leader. Assumption 2 further implies that the followers of a leader can communicate with each other.
Remark 2. During the last two decades, a number of results on the MLF group consensus of MASs have been reported (see, e.g., [11,12,13,14,15,16,17]). Unlike the existing literature, which always assumes that all agents have deterministic and delay-free dynamic behaviors, both the leaders and the followers considered in this paper are modeled by stochastic functional differential equations. It is also worth mentioning that most studies on this topic are based on a fixed communication topology, while in this paper, the communication topology is allowed to be changeable over time according to a Markov chain. Thus, the research object of this paper is more practical and general regardless of the dynamics of agents or topology structure.
At this point, the MLF group consensus issue discussed can be summarized as: designing a control protocol in the form of (2.7) so that the followers' states described by system (2.3) converge to a convex hull generated by the leaders' states of system (2.6) in mean square.
To solve the MLF group consensus issue, the following three Lemmas are useful:
Lemma 1. [32] With Assumptions 1 and 2, one has that Lst1>0, each entry of −(Lst1)−1Lst2 is nonnegative, and the sum of each row of −(Lst1)−1Lst2 equals to one, where Lst1 and Lst2 are defined in (2.1).
Lemma 2. [33] For any matrices Z1∈Rm×n,Z2∈Rp×q,Z3∈Rn×r, and Z4∈Rq×s, one has(Z1⊗Z2)(Z3⊗Z4)=(Z1Z3)⊗(Z2Z4).
Lemma 3. [34] Given a symmetric matrix Z=[Z11Z12Z21Z22], Z<0 is equivalent to Z22<0and Z11−Z12Z−122ZT12<0.
For convenience, we define xϕ(t)=col{x1(t),⋯,xn(t)}, uϕ(t)=col{u1(t),⋯,un(t)}, xψ(t)=col{xn+1(t),⋯,xn+m(t)}, and ξ(t)=col{ξ1(t),⋯,ξn(t)}. Then (2.8) is able to be written as
ξ(t)=(Lst1⊗Im1)xϕ(t)+(Lst2⊗Im1)xψ(t). | (3.1) |
Define
θ(t)=((Lst1)−1⊗Im1)ξ(t). | (3.2) |
Then, by Lemmas 1 and 2, we obtain
θ(t)=xϕ(t)+(((Lst1)−1Lst2)⊗Im1)xψ(t). | (3.3) |
It follows from (2.7) and (3.3) that
uϕ(t)=α(Lst1⊗Kst)θ(t)+βsgn((Lst1⊗Kst)θ(t)). | (3.4) |
Substituting (3.4) into (2.3) results in
dxϕ(t)=(In⊗A)xϕ(t)dt+(In⊗Aτ)xϕ(t−τ)dt+α(Lst1⊗(BKst))θ(t)dt+β(In⊗B)sgn((Lst1⊗Kst)θ(t))dt+(In⊗C)xϕ(t)dω(t),dxψ(t)=(Im⊗A)xψ(t)dt+(Im⊗Aτ)xψ(t−τ)dt+(Im⊗B)f(t)dt+(Im⊗C)xψ(t)dω(t), | (3.5) |
where f(t)=col{fn+1(xn+1(t),t),⋯,fn+m(xn+m(t),t)}. From (3.3) and (3.5), we have
dθ(t)=dxϕ(t)+(((Lst1)−1Lst2)⊗Im1)dxψ(t)=(In⊗A)xϕ(t)dt+(In⊗Aτ)xϕ(t−τ)dt+α(Lst1⊗(BKst))θ(t)dt+β(In⊗B)sgn((Lst1⊗Kst)θ(t))dt+(In⊗C)xϕ(t)dω(t)+(((Lst1)−1Lst2)⊗Im1)((Im⊗A)xψ(t)dt+(Im⊗Aτ)xψ(t−τ)dt+(Im⊗B)f(t)dt+(Im⊗C)xψ(t)dω(t)). | (3.6) |
Then, using (3.3), (3.6), and Lemma 2, gives
dθ(t)=((In⊗A)+α(Lst1⊗(BKst)))θ(t)dt+(In⊗Aτ)θ(t−τ)dt+β(In⊗B)sgn((Lst1⊗Kst)θ(t))dt+(In⊗B)(((Lst1)−1Lst2)⊗Im2)f(t)dt+(In⊗C)θ(t)dω(t). | (3.7) |
The following theorem gives the criterion for the MLF group consensus issue.
Theorem 1. If system (3.7) is stable inmean square, then the followers' states in (2.3) converge toa convex hull generated bythe leaders' states in (2.6) in mean-square. More specifically,
limt→∞E{xϕ(t)−gψ(t)}=0, |
where
gψ(t)≜((−(Lst1)−1Lst2)⊗Im1)xψ(t). |
Proof. In view of (3.3) and (3.7), the proof of this theorem can be easily completed and is thus omitted here.
Remark 3. Theorem 3.1 shows that, through the model transformation in (3.2), the MLF group consensus issue for system (3.1) can be transformed into a mean-square stability issue for system (3.7), which will be handled later by using the stochastic Lyapunov stability theory, graph theory, as well as several inequality techniques.
It is time to present our design method for the needed control protocol.
Theorem 2. Suppose that there exist two scalars α>0, β>ε, and matrices Pl>0 and Q>0\ such that
[Λ1Λ2Λ3PlˉP∗−Q000∗∗Λ400∗∗∗−Q0∗∗∗∗ˉΣ]<0, | (3.8) |
for any i∈{1,⋯,n} and l∈{1,⋯,s}, where
Λ1=S(λliAPl−α(λli)2BBT)+λliPlπll,Λ2=λliAτQ,Λ3=PlCT,Λ4=−1λliPl,ˉP=[Pl⋯Pl⋯Pl],ˉΣ=diag{−1λliπl1P1,⋯,−1λliπljPj,⋯,−1λliπlsPs} |
with λli being the i-th eigenvalue of Ll1, j≠l, and πlj≠0.Then, the MLF group consensus issue is solvable, and the required control protocol is given by (2.7) with α, β, and
Kl=−BTP−1l(l=1,⋯,s). | (3.9) |
Proof: Define a Lyapunov functional as
V(θ(t),st)=θT(t)(Lst1⊗P−1st)θ(t)+∫tt−τθT(s)(In⊗Q−1)θ(s)ds. | (3.10) |
Then, we can get
V(θ(t),st)≤λM(Lst1⊗P−1st)θT(t)θ(t)+∫tt−τλM(In⊗Q−1)θT(s)θ(s)ds. | (3.11) |
Utilizing the generalized Itô formula [35] to ektV(t)(k>0) yields
E{ektV(θ(t),st}=E{V(θ(0),s0}+E{t∫0eku[kV(θ(u),su)+LV(θ(u),su)]du}, | (3.12) |
where L is used to represent the weak infinitesimal operator. For st=l, we can write
LV(θ(t),l)=2θT(t)(Ll1⊗P−1l)((In⊗A)+α(Ll1⊗(BKl)))θ(t)+2θT(t)(Ll1⊗P−1l)(In⊗Aτ)θ(t−τ)+2θT(t)(Ll1⊗P−1l)×β(In⊗B)sgn((Ll1⊗Kl)θ(t))+2θT(t)(Ll1⊗P−1l)((In⊗B)(((Ll1)−1Ll2)⊗Im2)f(t))+θT(t)(In⊗C)T(Ll1⊗P−1l)(In⊗C)θ(t)+N∑j=1πljθT(t)(Lj1⊗P−1j)θ(t)+θT(t)(In⊗Q−1)θ(t)−θT(t−τ)(In⊗Q−1)θ(t−τ). | (3.13) |
Substituting (3.9) into (3.13) yields
LV(θ(t),l)=2θT(t)(Ll1⊗P−1l)((In⊗A)−α(Ll1⊗(BBTP−1l)))θ(t)+2θT(t)(Ll1⊗P−1l)(In⊗Aτ)θ(t−τ)+2θT(t)(Ll1⊗P−1l)β(In⊗B)sgn((Ll1⊗(−BTP−1l))θ(t))+2θT(t)(Ll1⊗P−1l)((In⊗B)(((Ll1)−1Ll2)⊗Im2))f(t)+θT(t)(In⊗C)T(Ll1⊗P−1l)(In⊗C)θ(t)+N∑j=1πljθT(t)(Lj1⊗P−1j)θ(t)+θT(t)(In⊗Q−1)θ(t)−θT(t−τ)(In⊗Q−1)θ(t−τ)≤2θT(t)(Ll1⊗P−1l)((In⊗A)−α(Ll1⊗(BBTP−1l)))θ(t)+2θT(t)(Ll1⊗P−1l)(In⊗Aτ)θ(t−τ)+2θT(t)(Ll1⊗P−1l)×β(In⊗B)sgn((Ll1⊗(−BTP−1l))θ(t))+2‖θT(t)(Ll1⊗P−1l)((In⊗B)(((Ll1)−1Ll2)⊗Im2))f(t)‖∞+θT(t)(In⊗C)T(Ll1⊗P−1l)(In⊗C)θ(t)+N∑j=1πljθT(t)(Lj1⊗P−1j)θ(t)+θT(t)(In⊗Q−1)θ(t)−θT(t−τ)(In⊗Q−1)θ(t−τ). | (3.14) |
According to the properties of ∞ norm and 1-norm for real vectors, we can get
‖θT(t)(Ll1⊗P−1l)((In⊗B)(((Ll1)−1Ll2)⊗Im2))f(t)‖∞≤‖θT(t)(Ll1⊗P−1l)(In⊗B)‖∞×‖((Ll1)−1Ll2)⊗Im2‖∞‖f(t)‖∞. | (3.15) |
θT(t)(Ll1⊗P−1l)(In⊗B)sgn((Ll1⊗(−BTP−1l))θ(t))=−‖(Ll1⊗(BTP−1l))θ(t)‖1. | (3.16) |
Using (2.5) and Lemma 1, it follows from (3.15) that
‖θT(t)(Ll1⊗P−1l)((In⊗B)(((Ll1)−1Ll2)⊗Im2))f(t)‖∞≤ε‖θT(t)(Ll1⊗P−1l)(In⊗B)‖∞. | (3.17) |
Using (3.14), (3.16), (3.17) and noting ‖Ψ‖∞≤‖Ψ‖1, we have
LV(θ(t),l)≤θT(t)[2(Ll1⊗P−1l)(In⊗A)−2α((Ll1)2⊗P−1lBBTP−1l)+(In⊗C)T(Ll1⊗P−1l)(In⊗C)+N∑j=1πlj(Lj1⊗P−1j)+In⊗Q−1]θ(t)+2θT(t)(Ll1⊗P−1l)(In⊗Aτ)θ(t−τ)−θT(t−τ)(In⊗Q−1)θ(t−τ)−2β‖(Ll1⊗(BTP−1l))θ(t)‖1+2ε‖θT(t)(Ll1⊗P−1l)(In⊗B)‖1. | (3.18) |
Because Ll1 is symmetrical, there exists a unitary matrix U∈Rn×n such that
UTLl1U=diag{λl1,⋯,λln}. |
Define
˜θ(t)=(UT⊗Im1)θ(t). |
Then (3.18) reduces to
LV(θ(t),l)≤˜θTi(t)[2(λliP−1lA−α(λli)2P−1lBBTP−1l)+λliCTP−1lC+Q−1+λlis∑j=1πljP−1j]˜θi(t)+2λli˜θTi(t)(P−1lAτ)˜θi(t−τ)−˜θTi(t−τ)Q−1˜θ(t−τ), |
which can be rewritten as follows:
LV(θ(t),l)≤ΘT∇Θ, | (3.19) |
where
Θ=[˜θi(t)˜θ(t−τ)],∇=[Ξ1λliP−1lAτ∗−Q−1],Ξ1=S(λliP−1lA−α(λli)2P−1lBBTP−1l)+λliCTP−1lC+Q−1+λlis∑j=1πljP−1j. |
Using a congruent transformation to ∇ by pre and post multiplying it with [Pl00Q] and its transpose gives
[Pl00Q]∇[Pl00Q]T=[Ξ2λliAτQ∗−Q], | (3.20) |
where
Ξ2=S(λliAPl−α(λli)2BBT)+λliPlCTP−1lCPl+PlQ−1Pl+λliPls∑j=1πljP−1jPl. |
By Lemma 3, (3.8) guarantees that [Ξ2λliAτQ∗−Q]<0, which together with (3.20) implies ∇<0. Thus, we have from (3.19) that
LV(θ(t),l)≤λM(∇)˜θTi(t)˜θi(t)≤λM(∇)λM(UUT⊗Im1)θT(t)θ(t). |
For convenience, we set ˜λl=λM(∇)λM(UUT⊗Im1) and write the above inequality as
LV(θ(t),l)≤˜λlθT(t)θ(t). | (3.21) |
Now, by (3.10)–(3.12), and (3.21), we have
minlλm(Ll1⊗P−1l)ektE{||θ(t))||2}≤E{ektV(θ(t),l)}≤E{λM(Ll1⊗P−1l)θT(0)θ(0)+∫0−τ(λM(In⊗Q−1))θT(s)θ(s)ds}+E{t∫0ekzk[λM(Ll1⊗P−1l)θT(z)θ(z)+∫zz−τλM(In⊗Q−1)θT(s)θ(s)ds]dz}+E{t∫0ekz˜λθT(z)θ(z)dz}≤D0lE{t∫0ekz||θ(z)||2dz}+D1lE{||θ||2τ}, | (3.22) |
where
D0l=kλM(Ll1⊗P−1l)+(ekτ−1)(In⊗Q−1)+˜λl,D1l=λM(Ll1⊗P−1l)+τλM(In⊗Q−1)+1k(ekτ−1)(1−e−kτ)(In⊗Q−1),||θ||τ=sup−τ≤z≤0||θ(z)||. |
Select a constant k>0 small enough such that D0l<0 and define
ˉλ=maxlD1lE{||θ||2τ}/minlλm(Ll1⊗P−1l). |
Then, with the aid of (3.22), it is not hard to obtain
E{||θ(t))||2}≤ˉλe−kt, |
which means that system (3.7) is exponential stable in mean square and, thus, in view of Theorem 1, the MLF group consensus is reached. This completes the proof.
When there is no time delay, system (3.7) reduces to
dθ(t)=((In⊗A)+α(Lst1⊗(BKst)))θ(t)dt+β(In⊗B)sgn((Lst1⊗Kst)θ(t))dt+(In⊗B)(((Lst1)−1Lst2)⊗Im2)f(t)dt+(In⊗C)θ(t)dω(t). |
In the case, the following corollary can be derived directly from Theorem 2. The proof is omitted for the sake of brevity.
Corollary 1. Given two positive constants β and ε, with β>ε, suppose that there are matrices Pl>0\and Q>0\ such that
[ˆΛ1PlCTPlˉP∗−1λliPl00∗∗−Q0∗∗∗ˉΣ]<0 |
for any i∈{1,⋯,n} and l∈{1,⋯,s}, where
ˆΛ1=S(λliAPl−α(λli)2BBT)+λliPlπll,ˉP=[Pl⋯Pl⋯Pl],ˉΣ=diag{−1λliπl1P1,⋯,−1λliπljPj,⋯,−1λliπlsPs}, |
with λli being the i-th eigenvalue of Ll1, j≠l, and πlj≠0. Then, the MLF group consensus issue is solvable. And the required control protocol is given by (2.7) with α, β, and Kl=−BTP−1l(l=1,⋯,s).
Next, consider the situation that the MAS is subjected to a fixed topology. Then, (3.7) becomes
dθ(t)=((In⊗A)+α(L1⊗(BK)))θ(t)dt+(In⊗Aτ)θ(t−τ)dt+β(In⊗B)sgn((L1⊗K)θ(t))dt+(In⊗B)(((L1)−1L2)⊗Im2)f(t)dt+(In⊗C)θ(t)dω(t), |
and one can write the following result:
Corollary 2. Given two positive scalars β and ε with β>ε, suppose that there are matrices P >0 and Q>0, such that
[ˇΛ1λAτQPCTP∗−Q00∗∗−1λP0∗∗∗−Q]<0 |
holds, where
ˇΛ1=S(λAP−αλ2BBT),λ=λmin(L1). |
Then, the MLF group consensus issue is solvable. And the required control protocol is given by (2.7) with α, β, and K=−BTP−1.
Consider a MAS consisting of seven dynamic multi-agents, where each agent corresponds to a mass-spring-damper system [36]. Let the 6-th and 7-th agents are leaders and the rest are followers. The parameter settings are as follows:
A=[01.5−1.5−3],Aτ=[01−1−2],B=[01],C=[0.15−0.020.080.25],x1(s)=[0.50],x2(s)=[1.3−0.2],x3(s)=[0.80],x4(s)=[−1.20],x5(s)=[2.5−0.5],x6(s)=[10],x7(s)=[−10],s∈[−1,0],α=2,f6(x6(t),t)=−[−0.251]x6(t)+4sin(2t),f7(x7(t),t)=−[−0.251]x7(t)+sin(t). |
Note that, for f6(x6(t),t) and f7(x7(t),t), the parameter ε can be chosen as ε=13.
Figure 1 shows three possible communication topologies, where nodes 1–7 correspond to agents 1–7, respectively. Set β=15 and the transition rate matrix between G1, G2, and G3 as
πlj=[−9363−6327−9]. |
Then, with the aid of Theorem 2, the feedback gain matrices can be gained as
K1=[−0.0608−0.0609],K2=[−0.0595−0.0596],K3=[−0.0603−0.0604]. |
Figure 2 depicts the Markov switching signal, and the three modes correspond to the three communication topologies in Figure 1. Under the designed controller, Figures 3 and 4 show the evolutions of the system states trajectories. It can be observed that, after a period of time, the states of agents 1–5 (the followers) enter the region generated by the state trajectories of agents 6 and 7 (the leaders). Therefore, the simulation results confirm that the designed control protocol is effective.
In this paper, the MLF group consensus issue of a class of stochastic time-delay agent systems with Markov switching topology has been studied. By using a model transformation, the group consensus problem has been transformed into the stability control problem of the system in (3.7). Then, it has been shown that the MLF group consensus can be guaranteed if the system in (3.7) is stable in mean-square (see Theorem 3.1). With the aid of Lyapunov stability theory, graph theory, as well as several inequality techniques, a sufficient condition for the solvability of the MLF group consensus issue has been proposed (see Theorem 3.2). It has been proved that the control protocol can ensure the followers' states converge to a convex hull generated by the leaders' states in mean square. Lastly, a numerical example with simulation has been provided to verify the effectiveness of the given MLF group control protocol.
This work was supported by the Natural Science Foundation of the Anhui Higher Education Institutions (Grant no. KJ2020A0248).
The authors declare there is no conflict of interest.
[1] | W. Ren, R. W. Beard, Deep space spacecraft formation flying, in Distributed Consensus in Multi-Vehicle Cooperative Control, London, U.K.: Springer-Verlag, (2008), 225–246. https://doi.org/10.1007/978-1-84800-015-5 |
[2] |
S. Safavi, U. A. Khan, Leader-follower consensus in mobile sensor networks, IEEE Signal Process. Lett., 22 (2015), 2249–2253. https://doi.org/10.1109/LSP.2015.2474134 doi: 10.1109/LSP.2015.2474134
![]() |
[3] |
C. Ma, W. Wu, Distributed leader-follower consensus of nonlinear multi-agent systems with unconsensusable switching topologies and its application to flexible-joint manipulators, Syst. Sci. Control Eng., 6 (2018), 200–207. https://doi.org/10.1080/21642583.2018.1547991 doi: 10.1080/21642583.2018.1547991
![]() |
[4] |
Z. Tang, T. Huang, J. Shao, J. Hu, Leader-following consensus for multi-agent systems via sampled-data control, IET Control Theory Appl., 5 (2011), 1658–1665. https://doi.org/10.1049/iet-cta.2010.0653 doi: 10.1049/iet-cta.2010.0653
![]() |
[5] |
C. Wang, H. Ji, Leader-following consensus of multi-agent systems under directed communication topology via distributed adaptive nonlinear protocol, Syst. Control Lett., 70 (2014), 23–29. https://doi.org/10.1016/j.sysconle.2014.05.010 doi: 10.1016/j.sysconle.2014.05.010
![]() |
[6] |
X. Jiang, G. Xia, Z. Feng, T. Li, Non-fragile H∞ consensus tracking of nonlinear multi-agent systems with switching topologies and transmission delay via sampled-data control, Inf. Sci., 509 (2020), 210–226. https://doi.org/10.1016/j.ins.2019.08.078 doi: 10.1016/j.ins.2019.08.078
![]() |
[7] |
J. Liu, T. Yin, D. Yue, H. R. Karimi, J. Cao, Event-based secure leader-following consensus control for multiagent systems with multiple cyber attacks, IEEE Trans. Cybern., 51 (2021), 162–173. https://doi.org/10.1109/TCYB.2020.2970556 doi: 10.1109/TCYB.2020.2970556
![]() |
[8] |
M. Ji, G. Ferrari-Trecate, M. Egerstedt, A. Buffa, Containment control in mobile networks, IEEE Trans. Autom. Control, 53 (2008), 1972–1975. https://doi.org/10.1109/TAC.2008.930098 doi: 10.1109/TAC.2008.930098
![]() |
[9] |
Q. Ma, F. L. Lewis, S. Xu, Cooperative containment of discrete-time linear multi-agent systems, Int. J. Robust Nonlin. Control, 25 (2015), 1007–1018. https://doi.org/10.1002/rnc.3124 doi: 10.1002/rnc.3124
![]() |
[10] |
D. Wang, N. Zhang, J. Wang, W. Wang, Cooperative containment control of multiagent systems based on follower observers with time delay, IEEE Trans. Syst., Man, Cybern., Syst., 47 (2016), 13–23. https://doi.org/10.1109/TSMC.2016.2577578 doi: 10.1109/TSMC.2016.2577578
![]() |
[11] |
G. Albi, L. Pareschi, M. Zanella, Boltzmann games in heterogeneous consensus dynamics, J. Stat. Phys., 175 (2019), 97–125. https://doi.org/10.1007/s10955-019-02246-y doi: 10.1007/s10955-019-02246-y
![]() |
[12] |
L. Zhao, J. Yu, H. Yu, C. Lin, Neuroadaptive containment control of nonlinear multiagent systems with input saturations, Int. J. Robust Nonlin. Control, 29 (2019), 2742–2756. https://doi.org/10.1002/rnc.4520 doi: 10.1002/rnc.4520
![]() |
[13] |
Q. Zhou, W. Wang, H. Liang, M. V. Basin, B. Wang, Observer-based event-triggered fuzzy adaptive bipartite containment control of multi-agent systems with input quantization, IEEE Trans. Fuzzy Syst., 29 (2021), 372–384. https://doi.org/10.1109/TFUZZ.2019.2953573 doi: 10.1109/TFUZZ.2019.2953573
![]() |
[14] |
L. Martinović, Ž. Zečević, B. Krstajić, Cooperative tracking control of single-integrator multi-agent systems with multiple leaders, Eur. J. Control, 63 (2022), 232–239. https://doi.org/10.1016/j.ejcon.2021.11.003 doi: 10.1016/j.ejcon.2021.11.003
![]() |
[15] |
H. Liang, Y. Zhou, H. Zhang, Containment control for singular multi-agent systems with an internal model compensator, Sci. China Inf. Sci., 63 (2020), 229202. https://doi.org/10.1007/s11432-018-9780-3 doi: 10.1007/s11432-018-9780-3
![]() |
[16] |
T. Hu, L. Li, Y. Wu, W. Sun, Consensus dynamics in noisy trees with given parameters, Mod. Phys. Lett. B, 36 (2022), 2150608. https://doi.org/10.1142/S0217984921506089 doi: 10.1142/S0217984921506089
![]() |
[17] |
T. Liu, J. Qi, Z. Jiang, Distributed containment control of multi-agent systems with velocity and acceleration saturations, Automatica, 117 (2020), 108992. https://doi.org/10.1016/j.automatica.2020.108992 doi: 10.1016/j.automatica.2020.108992
![]() |
[18] |
L. Ma, Z. Wang, Q. Han, Y. Liu, Consensus control of stochastic multi-agent systems: a survey, Sci. China Inf. Sci., 60 (2017), 120201. https://doi.org/10.1007/s11432-017-9169-4 doi: 10.1007/s11432-017-9169-4
![]() |
[19] |
J. Zhou, Y. Wang, X. Zheng, Z. Wang, H. Shen, Weighted H∞ consensus design for stochastic multi-agent systems subject to external disturbances and ADT switching topologies, Nonlinear Dyn., 96 (2019), 853–868. https://doi.org/10.1007/s11071-019-04826-9 doi: 10.1007/s11071-019-04826-9
![]() |
[20] |
X. Wang, J. H. Park, H. Yang, An improved protocol to consensus of delayed MASs with UNMS and aperiodic DoS cyber-attacks, IEEE Trans. Netw. Sci. Eng., 8 (2021), 2506–2516. https://doi.org/10.1109/TNSE.2021.3098258 doi: 10.1109/TNSE.2021.3098258
![]() |
[21] |
Z. Xu, H. Ni, H. R. Karimi, D. Zhang, A Markovian jump system approach to consensus of heterogeneous multiagent systems with partially unknown and uncertain attack strategies, Int. J. Robust Nonlin. Control, 30 (2020), 3039–3053. https://doi.org/10.1002/rnc.4923 doi: 10.1002/rnc.4923
![]() |
[22] |
M. Fang, C. Zhou, X. Huang, X. Li, J. Zhou, H∞ couple-group consensus of stochastic multi-agent systems with fixed and Markovian switching communication topologies, Chin. Phys. B, 28 (2019), 010703. https://doi.org/10.1088/1674-1056/28/1/010703 doi: 10.1088/1674-1056/28/1/010703
![]() |
[23] |
S. Huo, L. Zhang, S. Chen, Y. Zhang, H∞ consensus control of multi-agent systems under attacks with partially unknown Markovian probabilities, J. Frankl. Inst., 358 (2021), 4917–4928. https://doi.org/10.1016/j.jfranklin.2021.04.013 doi: 10.1016/j.jfranklin.2021.04.013
![]() |
[24] |
Z. Yan, X. Huang, J. Cao, Variable-sampling-period dependent global stabilization of delayed memristive neural networks based on refined switching event-triggered control, Sci. China Inf. Sci., 63 (2020), 212201. https://doi.org/10.1007/s11432-019-2664-7 doi: 10.1007/s11432-019-2664-7
![]() |
[25] |
X. Huang, Y. Liu, Y. Wang, J. Zhou, M. Fang, Z. Wang, L2−L∞ consensus of stochastic delayed multi-agent systems with ADT switching interaction topologies, Appl. Math. Comput., 368 (2020), 124800. https://doi.org/10.1016/j.amc.2019.124800 doi: 10.1016/j.amc.2019.124800
![]() |
[26] |
Z. Zhang, X. Huang, Y. Chen, J. Zhou, Input-to-state H∞ learning of recurrent neural networks with delay and disturbance, Int. J. Adapt. Control Signal Process., 35 (2021), 1438–1453. https://doi.org/10.1002/acs.3251 doi: 10.1002/acs.3251
![]() |
[27] |
J. Li, X. Liu, C. Wei, The impact of fear factor and self-defence on the dynamics of predator-prey model with digestion delay, Math. Biosci. Eng., 18 (2021), 5478–5504. https://doi.org/10.3934/mbe.2021277 doi: 10.3934/mbe.2021277
![]() |
[28] |
R. Sakthivel, M. Rathika, S. Santra, M. Muslim, Observer-based dissipative control for Markovian jump systems via delta operators, Int. J. Syst. Sci., 48 (2017), 247–256. https://doi.org/10.1080/00207721.2016.1177131 doi: 10.1080/00207721.2016.1177131
![]() |
[29] |
Y. Xia, W. Zhou, Z. Yang, Global analysis and optimal harvesting for a hybrid stochastic phytoplankton-zooplankton-fish model with distributed delays, Math. Biosci. Eng., 17 (2020), 6149–6180. https://doi.org/10.3934/mbe.2020326 doi: 10.3934/mbe.2020326
![]() |
[30] |
M. S. Ali, M. Usha, O. M. Kwon, N. Gunasekaran, K. G. Thakur, H∞/passive non-fragile synchronisation of Markovian jump stochastic complex dynamical networks with time-varying delays, Int. J. Syst. Sci., 52 (2021), 1270–1283. https://doi.org/10.1080/00207721.2020.1856445 doi: 10.1080/00207721.2020.1856445
![]() |
[31] |
Y. Cao, W. Ren, M. Egerstedt, Distributed containment control with multiple stationary or dynamic leaders in fixed and switching directed networks, Automatica, 48 (2012), 1586–1597. https://doi.org/10.1016/j.automatica.2012.05.071 doi: 10.1016/j.automatica.2012.05.071
![]() |
[32] |
G. Wen, Z. Duan, Y. Zhao, W. Yu, J. Cao, Robust containment tracking of uncertain linear multi-agent systems: a non-smooth control approach, Int. J. Control, 87 (2014), 2522–2534. https://doi.org/10.1080/00207179.2014.930930 doi: 10.1080/00207179.2014.930930
![]() |
[33] | H. Lütkepohl, Rules for matrix operations, in Handbook of Matrices, New York: Wiley, (1996), 15–22. https://doi.org/10.1017/S0266466698143086 |
[34] | S. Boyd, L. E. Ghaoui, E. Feron, V. Balakrishnan, Some standard problems involving LMIs, in Linear Matrix Inequalities in System and Control Theory, Philadelphia: SIAM, (1994), 7–36. https://doi.org/10.1137/1.9781611970777.ch2 |
[35] |
X. Mao, Razumikhin-type theorems on exponential stability of stochastic functional differential equations, Stoch. Process. Appl., 65 (1996), 233–250. https://doi.org/10.1016/S0304-4149(96)00109-3 doi: 10.1016/S0304-4149(96)00109-3
![]() |
[36] |
K. Khandani, M. Parsa, Distributed containment control of a class of multi-agent systems driven by Brownian motion, Int. J. Dyn. Control, 7 (2019), 1035–1045. https://doi.org/10.1007/s40435-019-00561-6 doi: 10.1007/s40435-019-00561-6
![]() |
1. | Sai Zhang, Li Tang, Yan-Jun Liu, Formation deployment control of multi-agent systems modeled with PDE, 2022, 19, 1551-0018, 13541, 10.3934/mbe.2022632 | |
2. | Jinxin Du, Lei Liu, Adaptive fuzzy fixed time formation control of state constrained nonlinear multi-agent systems against FDI attacks, 2024, 21, 1551-0018, 4724, 10.3934/mbe.2024207 | |
3. | Xiaofeng Chen, Double-integrator consensus for a switching network without dwell time, 2023, 20, 1551-0018, 11627, 10.3934/mbe.2023516 | |
4. | Yuhang Yao, Jiaxin Yuan, Tao Chen, Xiaole Yang, Hui Yang, Distributed convex optimization of bipartite containment control for high-order nonlinear uncertain multi-agent systems with state constraints, 2023, 20, 1551-0018, 17296, 10.3934/mbe.2023770 | |
5. | Xinling Li, Xiaofeng Ma, Weipeng Tai, Jianping Zhou, Designing an event-triggered H∞ filter with possibly inconsistent modes for Markov jump systems, 2023, 139, 10512004, 104092, 10.1016/j.dsp.2023.104092 |