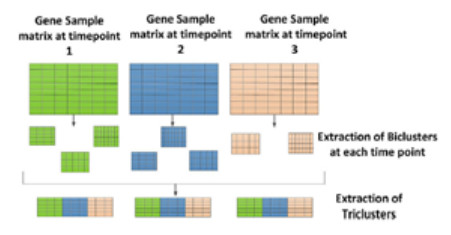
HIV-1 is a virus that destroys CD4 + cells in the body's immune system, causing a drastic decline in immune system performance. Analysis of HIV-1 gene expression data is urgently needed. Microarray technology is used to analyze gene expression data by measuring the expression of thousands of genes in various conditions. The gene expression series data, which are formed in three dimensions, are analyzed using triclustering. Triclustering is an analysis technique for 3D data that aims to group data simultaneously into rows and columns across different times/conditions. The result of this technique is called a tricluster. A tricluster is a subspace in the form of a subset of rows, columns, and time/conditions. In this study, we used the δ-Trimax, THD Tricluster, and MOEA methods by applying different measures, namely, transposed virtual error, the New Residue Score, and the Multi Slope Measure. The gene expression data consisted of 22,283 probe gene IDs, 40 observations, and four conditions: normal, acute, chronic, and non-progressor. Tricluster evaluation was carried out based on intertemporal homogeneity. An analysis of the probe ID gene that affects AIDS was carried out through this triclustering process. Based on this analysis, a gene symbol which is biomarkers associated with AIDS due to HIV-1, HLA-C, was found in every condition for normal, acute, chronic, and non-progressive HIV-1 patients.
Citation: Titin Siswantining, Alhadi Bustamam, Devvi Sarwinda, Saskya Mary Soemartojo, Moh. Abdul Latief, Elke Annisa Octaria, Anggrainy Togi Marito Siregar, Oon Septa, Herley Shaori Al-Ash, Noval Saputra. Triclustering method for finding biomarkers in human immunodeficiency virus-1 gene expression data[J]. Mathematical Biosciences and Engineering, 2022, 19(7): 6743-6763. doi: 10.3934/mbe.2022318
[1] | Titin Siswantining, Alhadi Bustamam, Devvi Sarwinda, Saskya Mary Soemartojo, Moh. Abdul Latief, Elke Annisa Octaria, Anggrainy Togi Marito Siregar, Oon Septa, Herley Shaori Al-Ash, Noval Saputra . Correction: Triclustering method for finding biomarkers in human immunodeficiency virus-1 gene expression data. Mathematical Biosciences and Engineering, 2023, 20(4): 7298-7301. doi: 10.3934/mbe.2023316 |
[2] | Saskya Mary Soemartojo, Titin Siswantining, Yoel Fernando, Devvi Sarwinda, Herley Shaori Al-Ash, Sarah Syarofina, Noval Saputra . Iterative bicluster-based Bayesian principal component analysis and least squares for missing-value imputation in microarray and RNA-sequencing data. Mathematical Biosciences and Engineering, 2022, 19(9): 8741-8759. doi: 10.3934/mbe.2022405 |
[3] | Wei Kong, Feifan Xu, Shuaiqun Wang, Kai Wei, Gen Wen, Yaling Yu . Application of orthogonal sparse joint non-negative matrix factorization based on connectivity in Alzheimer's disease research. Mathematical Biosciences and Engineering, 2023, 20(6): 9923-9947. doi: 10.3934/mbe.2023435 |
[4] | Ahmed Hammad, Mohamed Elshaer, Xiuwen Tang . Identification of potential biomarkers with colorectal cancer based on bioinformatics analysis and machine learning. Mathematical Biosciences and Engineering, 2021, 18(6): 8997-9015. doi: 10.3934/mbe.2021443 |
[5] | Fang Niu, Zongwei Liu, Peidong Liu, Hongrui Pan, Jiaxue Bi, Peng Li, Guangze Luo, Yonghui Chen, Xiaoxing Zhang, Xiangchen Dai . Identification of novel genetic biomarkers and treatment targets for arteriosclerosis-related abdominal aortic aneurysm using bioinformatic tools. Mathematical Biosciences and Engineering, 2021, 18(6): 9761-9774. doi: 10.3934/mbe.2021478 |
[6] | Sijie Lu, Juan Xie, Yang Li, Bin Yu, Qin Ma, Bingqiang Liu . Identification of lncRNAs-gene interactions in transcription regulation based on co-expression analysis of RNA-seq data. Mathematical Biosciences and Engineering, 2019, 16(6): 7112-7125. doi: 10.3934/mbe.2019357 |
[7] | Yu Jin, Zhe Ren, Wenjie Wang, Yulei Zhang, Liang Zhou, Xufeng Yao, Tao Wu . Classification of Alzheimer's disease using robust TabNet neural networks on genetic data. Mathematical Biosciences and Engineering, 2023, 20(5): 8358-8374. doi: 10.3934/mbe.2023366 |
[8] | Youlong Lv, Jie Zhang . A genetic regulatory network based method for multi-objective sequencing problem in mixed-model assembly lines. Mathematical Biosciences and Engineering, 2019, 16(3): 1228-1243. doi: 10.3934/mbe.2019059 |
[9] | Ming-Xi Zhu, Tian-Yang Zhao, Yan Li . Insight into the mechanism of DNA methylation and miRNA-mRNA regulatory network in ischemic stroke. Mathematical Biosciences and Engineering, 2023, 20(6): 10264-10283. doi: 10.3934/mbe.2023450 |
[10] | Shuaiqun Wang, Tianshun Zhang, Wei Kong, Gen Wen, Yaling Yu . An improved MOPSO approach with adaptive strategy for identifying biomarkers from gene expression dataset. Mathematical Biosciences and Engineering, 2023, 20(2): 1580-1598. doi: 10.3934/mbe.2023072 |
HIV-1 is a virus that destroys CD4 + cells in the body's immune system, causing a drastic decline in immune system performance. Analysis of HIV-1 gene expression data is urgently needed. Microarray technology is used to analyze gene expression data by measuring the expression of thousands of genes in various conditions. The gene expression series data, which are formed in three dimensions, are analyzed using triclustering. Triclustering is an analysis technique for 3D data that aims to group data simultaneously into rows and columns across different times/conditions. The result of this technique is called a tricluster. A tricluster is a subspace in the form of a subset of rows, columns, and time/conditions. In this study, we used the δ-Trimax, THD Tricluster, and MOEA methods by applying different measures, namely, transposed virtual error, the New Residue Score, and the Multi Slope Measure. The gene expression data consisted of 22,283 probe gene IDs, 40 observations, and four conditions: normal, acute, chronic, and non-progressor. Tricluster evaluation was carried out based on intertemporal homogeneity. An analysis of the probe ID gene that affects AIDS was carried out through this triclustering process. Based on this analysis, a gene symbol which is biomarkers associated with AIDS due to HIV-1, HLA-C, was found in every condition for normal, acute, chronic, and non-progressive HIV-1 patients.
Currently, many studies are being conducted on gene expression. Several technologies can aid in the process of measuring gene expression [1,2,3]. The most commonly used technology is microarray technology. Microarray technology is used to measure the expression of thousands of genes simultaneously, resulting in a very large data source called a gene expression matrix, which is formed in a structured manner. In recent years, microarray technology has been used to measure the expression values of thousands of genes under a wide variety of experimental conditions at different points in time. Such a dataset can be referred to as gene-sample-time (GST) expression data. In addition to the GST expression data, other types of three-dimensional (3D) data are used in the biomedical and social fields. These include individual-feature-time and node-node-time data, which is commonly referred to as attribute-data-context.
One of the techniques often used for gene expression data analysis is clustering. The analyzed gene expression data are presented in the form of a matrix, with rows representing the probe gene ID and columns representing observations [4,5,6]. Clustering is a grouping technique that works on two-dimensional (2D) data, which aims to group rows or columns in a matrix to obtain a sub-matrix from one of them. This technique was developed into biclustering, with the aim of grouping 2D data in the form of rows and columns in a matrix simultaneously to obtain a sub-matrix from both of them [7,8,9]. As the data grew larger and more complex and in response to constraints on classifying gene expressions with a certain time/condition, this technique was further developed into triclustering. Triclustering aims to group 3D data in rows and columns across different times/conditions. The result of this technique is called a tricluster, which is a subspace in the form of a subset of rows, columns, and time/conditions [10,11,12].
In 2018, Kakati researched triclustering using a method called THD-Tricluster. This method consists of two steps: generating biclusters and generating triclusters. This method analyzes 3D gene expression data and can identify various patterns, such as absolute shifting and scaling as well as shifting and scaling using the Shifting and Scaling Similarity (SSSim) measure[13]. The SSSim measure has been used to overcome the limitations of several previous studies, which identified only one pattern and not shifting and scaling patterns. This research is new because it can mine data in various patterns, especially shifting and scaling. In addition, the algorithm was used by Kakati to build a tricluster using MATLAB software. Apart from the SSSim measure, there are also new residue scores and transposed virtual error, which is a measure that handles various patterns, especially shifting and scaling. This measure works by looking for correlations between rows and columns of a matrix, known as Pearson correlation [14,15,16]. In this study, researchers were inspired to implement the THD-Tricluster method by using a new residue score on the 3D gene expression data for HIV-1 disease. HIV-1 is a deadly disease that requires analysis to inhibit disease progression because late diagnosis causes many HIV-1 sufferers to die [17]. In 2006, Sushmita Mitra and Haider Banka introducing bicluster search phase uses the Multi-Objective Evolutionary Algorithm with Mean Square Residue (MSR) measurement. Then [18] build a tricluster with the Trigen algorithm.
In this study, we use the THD tricluster method with a new residue score, Transposed Virtual Error, and we use MOEA for optimization in searching for biclusters as well as the Trigen algorithm with multi-slope measurement. Then, the results are compared with those using the δ-Trimax method, which uses the mean square residual measure. The obtained triclusters are evaluated based on inter-temporal homogeneity, and the tricluster results are analyzed to find biomarkers against HIV-1. Open source R-based and python programming language is used to implement the program used to build a tricluster with a multi-objective and δ-Trimax approach.
This research uses developmental data for HIV-1 referred to in [19] and obtained from the National Center for Biotechnology Information (NCBI) website with the website address http://www.ncbi.nlm.nih.gov/ with ID GSE6740. The NCBI is a site that provides access to biomedical and genome information. The data used by [19] 22,283 gene ID probes, 10 observations and four conditions. These conditions include HIV Acute sufferers, HIV Chronic sufferers, HIV non-progressors, and uninfected individuals. The four data have different gene expression values. Before using the triclustering method, the gene ID probe selection process was carried out, and 2557 gene ID probes were produced along with 10 observations for each condition.
THD-Tricluster is a triclustering method capable of mining 3D data with absolute patterns, shifts, scaling, shifting, and scaling, with high biological significance. The THD-Tricluster method consists of two stages: generate biclusters and generating triclusters. The THD-Tricluster workflow with three-time points can be seen in Figure 1.
The CC algorithm is the first algorithm used by [20] to identify biclustering in gene expression data using the Mean Square Residue (MSR) as a measure. MSR measured is used to solve overlapping clusters with a high similarity, but this is not capable for scalling patterns. In this research, the CC algorithm is modified using the Transposed Virtual Error VEt measure, which can group data based on shifting patterns and scaling. The CC algorithm used consists of multiple node deletion, single node deletion, and single node addition phases.
Multiple Node Deletion Phase The input in the multiple node deletion phases is a matrix of gene expression data. In the CC's algorithm, we need the alpha (γ) parameter, which represents the limits of the selected data. This phase involves deleting rows and columns simultaneously if they do not meet the chosen parameters.
Single Node Deletion Phase The input of this phase is the submatrix obtained from the multiple node deletion phase. The parameter used is the delta (δ). If in the multiple node deletion stage rows and columns can be deleted simultaneously, then in this phase the columns and rows are only deleted one by one. The deletion of rows and columns occurs by comparing the transposed virtual error value with the delta (δ). Selected rows and columns are deleted, which requires several steps, including calculating the average row value of the matrix element minus the virtual condition (mr) and the average column value of the matrix element minus the virtual condition (mc) and then finding the max value (mri,mcj). Columns or rows that have a greater value are deleted. This process takes place until it the selected delta parameter values are met.
Single Node Addition Phase In this phase, the parameter used is the delta (δ), and the input is a matrix obtained from the single node addition phase. The matrix is added again with rows or columns that have been deleted in the multiple node deletion phase. This is done to produce a more optimal bicluster by adding a column or row so that the transposed virtual error value obtained is close to the limit of the selected parameter value.
Lift Algorithm The lift algorithm consists of two phases: single node deletion and single node addition [21]. Single node deletion algorithm aims to remove nodes in the form of rows or columns on the matrix that have values exceeding the threshold. In this study, the value in question is the result of the calculation of the new residue score (S). Iterations of this step continue until S≤δ [16]. The submatrix obtained by single node deletion may not be maximal, so we check on the deleted nodes again in the single node deletion step using the single node addition step. The checking is done by recalculating the value of S in rows or columns that have been deleted on the condition that it can maintain an S value that is less than node so that nodes that produce a small S value will be added. This iteration will end when no more nodes can be added [16].
The generate triclusters stage aims to produce a set of triclusters. Triclusters are found by finding the slices of all bicluster combinations for each condition.
New Residue Score The purpose of the new residue score is to find the correlation between rows and columns of a matrix known as the Pearson correlation. This correlation indicates the degree of the linear relation between two vectors and will produce a perfect bicluster correlation.
cor(vi,wi)=∑ni=1(vi−ˉv)(wi−ˉw)√∑ni=1(vi−ˉv)2×∑ni=1(wi−ˉw)3 | (2.1) |
The result of this correlation is a number between 1 and -1, which means that there is a perfect positive (or negative) linear relationship between the indexed submatrices [16]. The correlation value in the indexed submatrix column (I, J) is defined as:
Scol(I,J)=minj1∈j(SIj1) | (2.2) |
which
(SIj1)=1−1|J|−1∑j2≠j1,j2∈J|cor(xIj1,xIj2)| | (2.3) |
The values of Scol and Srow indicate the degree of correlation of the respective columns and rows of a submatrix. The correlation values of the indexed submatrix (I,J) are defined as follows:
Given a submatrix indexed (I,J), the correlation value (residue) of the submatrix is:
S(I,J)=min(Srow(I,J),Scol(I,J)) | (2.4) |
The lower the correlation value, the better the column and row correlation will be. According to [16], the determination of the bicluster residual value is based on the minimum value of row and column correlation [16].
Transpose Virtual Error The purpose behind Virtual Error(VE) is to measure the genes that have a general tendency to bicluster. To determine the general trend of this gene across the conditions contained in the bicluster, new virtual lines are counted from the bicluster gene, which is called a virtual pattern or virtual ρ gene. Each element ρj of ρ is calculated as the average of the jth column or experimental conditions, as in the following equation:
ρj=1|J||J|∑j=1bij | (2.5) |
In the VEt calculation, a standardization process is carried out on each data element and the virtual conditions. The process of standardizing the elements of each submatrix involves calculating the average value for each row and calculating the standard deviation value for each row, which can be expressed as follows:
ˆbij=ˆbij−μciσci | (2.6) |
Meanwhile, the virtual standardization process can be calculated by calculating the average of each column and the standard:
ˆρj=ρj−μρjσρj | (2.7) |
The VEt value for the submatrix B is obtained using the standardization of the virtual condition value ˆρ together with the standardized submatrix elements ˆbij. The formula for calculating the VEt value is as follows:
VEt(B)=1|I|⋅|J|i=|I|∑i=1j=|J|∑j=1|ˆbij−ˆρi| | (2.8) |
VEt has been shown to be zero for biclusters with perfect shifting, scaling, or compound patterns. Therefore, it is efficient to recognize shifting and scaling patterns in biclusters either simultaneously or independently [22].
Multi Slope Measure (MSL) Understanding MSL requires a graphic representation of tricluster TRIxyz, where x, y, and z are one of gene G, condition C, and time or depth T so that element x on TRIxyz will be on the X-axis, and element y on TRIxyz will outline the panels that are elements z on TRIxyz as shown in Figure 2 [23]. MSL calculates the difference among the angles formed by every series traced on each of three representations, taking into account TRIgct, TRIgtc, and TRItgc on Figure 3. MSL accounts for the effect of adjacent points in time.
MSL measures the average difference between the angles formed by the probe ID gene in all rows and columns for each individual or candidate tricluster. To calculate the MSL measure of a tricluster, a multiangular ratio calculation is first performed. Define the FGmulti tricluster TRItgc as the average difference Δ from an angle vector avyz∈angset from all of the outlines y, for each panel p(Vmc), and the same for the rest of panels (Hmc), where Nmc is the number of differences that are formed. All the angular vectors of an outline y on panel z are defined as a set of angles formed by outline y, taking into account each data point on the X-axis. Each outline will have many (axis mark X–1) angles. The difference Δ between two vector angles avA and avB is defined as the average of MAX−MIN (MAX is the maximum, and MIN is the minimum of the two angles avA(i) and avB(i)) of any component or angle i from avA and avB.
FGmulti(TRIxyz)=Vmc+HmcNmc | (2.9) |
where:
angset={avy1z1,avy2z1,avy3z1,...,avy1z2,y1z3,...,avyUT,zAN}Vmc=∑angsetΔ(avyz,avnext(y)z)Hmc=∑angsetΔ(avyz,avynext(z))Nmc=|y|×|z|×(|y|+|z|−2)2avy={axi} where i=1,2,...,AX−1Δ(avA,avB)=∑i∈avA,avBMAX(avA(i),avB(i))−MIN(avA(i),avB(i))|avA,B| |
FGmulti is based on multiple operations with the avyz angle vector. These elements are obtained based on the concept of a series, so the series Syz from the outline y for each panel z is the set of value pairs from the X-axis (xi) and expression level (elj), which forms the outline. For each set Syz, the angle of alpha axi is the spin arctangent of the slope of the line formed by the points (xi,eli) and (xnext(i),elnext(i)). The spin operation from an angle is the positive equivalent of the angle if it is negative.
Syz={(x0,el0),...,(xAX,elL)} | (2.10) |
axi=spin(arctan(xnext(i)−xielnext(i)−eli)) | (2.11) |
spin(axi)=axi, if axi≥0spin(axi=axi+(2+π) if axi<0 |
To conclude, the MSL measure of a TRI tricluster as shown in Eq (2.12) is the mean of the angular comparisons of three graphical representations of a tricluster.
MSL(TRI)=13[FGmulti(TRIgct)+FGmulti(TRIgtc)+FGmulti(TRItgc)] | (2.12) |
Multi-objective optimization is a development of a genetic algorithm. The genetic algorithm is used to solve problems in the search for optimization values. Multi-objective evolution is based on genetic processes in living things, including the process of developing generations in a population, which is gradually based on natural selection. This algorithm is used to search for optimal biclusters and triclusters.
Initialization of the population is the first step in multi-objective optimization. Population is the number of individuals who represent the desired solution. In this study, individuals are the number of submatrices in the first method (MOEA and THD tricluster) and subspaces in the second method (Triclustering Genetic Based) obtained from the gene expression matrix. The following are the population initialization stages:
1) Determine the number of sub-matrixes expressed as individuals. For example, m individuals where m represents the number of rows in the population matrix.
2) The column for the population matrix is the number of probe id genes and observations on gene expression data expressed as n.
3) Form a population matrix of size m×n randomly with the encoding method. This study uses binary coding, namely 1 and 0. The number 1 means that the probe id gene and observation are included in the sub-matrix, while the number 0 means that the probe id gene and observations are not included in the sub-matrix.
4) Change the population matrix by decoding, so that sub-matrixes are obtained which will be evaluated through transpose virtual error.
The initial population is generated randomly so that an initial solution is obtained. Initialization is done by coding. Coding is an important aspect of multi-objective optimization. Encoding is a technique for expressing the initial population as a potential solution to a problem onto an individual chromosome. In this study, the researchers used binary coding, which is used to encode (encode) all chromosomes. Each possible bicluster or tricluster is represented by the total bits of the binary string |G|+|S|+|T| used. Bits from binary string |G| are used to encode genes, bits of the binary string |S| are used to encode observations, and bits of the binary string |T| are used to encode conditions. When coding, 1 in the binary string means that the gene, observation, or condition is grouped into a bicluster/tricluster, while 0 means that the gene, observation, or condition is not grouped in a bicluster/tricluster.
The local search consists of multiple node deletion, single node deletion and single node addition. Local search is used in the MOEA process. The steps of the multiple node deletion algorithm on the matrix M(I,J) are as follow:
1) When VEt≥δ, then go to step 2, otherwise the process is discontinued and M(I,J) is given as the final result of this algorithm.
2) Delete all the probe ID genes i∈I if they satisfy : 1|J|∑i∈I|^bij−^ρi|>α×VEt
3) Recalculate the value of VEt after deletion.
4) Delete all of the observations j∈J if they satisfy : 1|I|∑j∈J|^bij−^ρi|>α×VEt
5) Recalculate the value of VEt after deletion.
6)These results then serve as input to the single node deletion algorithm.
The steps of the single node deletion algorithm are as follows:
1) Detect the probe ID gene and observation that has the highest VEt score in the following ways:
2) Row score for ID gene to-i, ∀i∈I, μi=1|J|∑i∈I|^bij−^ρi|
3)Column score for observation to-j, ∀j∈J, μj=1|I|∑j∈J|^bij−^ρi|
4) Delete the probe ID gene or observation with the highest score.
5) Recalculate the value of VEt.
6) Repeat steps a to c. If the value of VEt≤δ, then stop the iteration
.
The final result from the node addition algorithm is data subspace M(I′,J′), where I′⊆I and J′⊆J. Subspace data produced from this algorithm are in the form of a bicluster with VEt≤δ of the maximum size.
In each generation, the chromosomes will go through an evaluation process using a measuring instrument called fitness. The fitness value of a chromosome describes the quality of the chromosomes in the population. This process will evaluate each population by calculating the fitness value of each chromosome and evaluating it until the stop criteria are met. Some of the criteria for stopping that are often used include stopping at a certain generation, stopping after several successive generations when the highest fitness value has not changed, and stopping in n generations if a higher fitness value is not achieved.
In this study, for the first method, namely, the search for biclusters with MOEA, the researcher wanted the size of the bicluster to meet the large homogeneity criteria and the error value of the minimum bicluster results. Based on the two criteria above, a formula is created for the value of the fitness functions f1 and f2.
f1=|I|×|J||G|×|S| | (2.13) |
f2={VEtδif VEt≤δ0ifVEt>δ | (2.14) |
where |I| is the number of probe ID genes, |J| is the number of observations in the submatrix or bicluster, and |G| and |S| are the number of probe ID genes and the number of observations in the preliminary data, respectively. VEt is the Transpose Virtual Error of the bicluster, while δ is a given error tolerance limit.
An individual can be said to dominate other individuals if it meets the following:
i. Individual A is no worse off than individul B on all objectives (A≥B).
ii. Individual A has at least one better objective than individul B.
Based on these rules, each individual is compared with other individuals in a population. The optimization problem in this case is to find the minimum value of two objective functions so that the domination condition is that an individual is not worse than another individual and has at least one objective whose value is greater than that of the other individuals. This individual can be at the first front. Then, the next front is filled in based on individuals who were dominated in the previous front.
The crowding distance is calculated for each individual to measure how close the individual is to its neighbor. The average crowding distance will result in better diversity in the population. The parents are selected from the population using a binary selection tournament based on the crowding distance. Individuals are selected in this rank if they are lower than others or if the crowding distance is greater than others. The population that produces the offspring of the crossover and mutation operators is selected, which will be discussed in detail in the next section. Populations with current populations and current descendants are sorted again based on non-dominated sorting, and only the best N individuals are selected, where N is the population size.
Crowding distance gives the highest value for the solution limit and the average distance of two solutions, that is, the solution to (i+1) and the solution to (i−1) and i for each purpose. The crowding distance calculation step for the i−th solution is as follows:
1) Sort all solutions i with Pareto-Front in ascending order from fm and calculate
CDim=fm(xi+1)−fm(xi−1)fm(xmax)−fm(xmin),i=2,...,(l−1) |
2) Repeat step 1 for each objective function and find the crowding distance for the i−th solution
CDi=M∑m=1CDim |
3) Two solutions i and j, solution i is better than solution j if Ri<Rj or Ri=Rj and CDi>CDj
There are several methods for selecting individuals that are often used, including roulette wheel selection, rank selection, and crowded tournament selection. In this study, the crowded tournament selection was utilized. The crowded tournament selection operator is defined as follows: Solution i wins the tournament with another solution that is solution j if one of the following is fulfilled:
1) Solution i has a better ranking, ri<rj
2) if both solutions are on the same front, namely, ri=rj, then the crowding distance of the i solution is greater than the crowding distance of the j solution (CDi>CDj).
The genetic operators including selection, crossover, and mutase are described below:
a) Selection: Three groups of individuals are randomly selected in order from lowest to highest according to the fitness function, and then a random selection of the three groups is made. The cell parameter shows how many of these individuals will pass to the next generation.
b) Crossover: To complete the next generation, a new individual was created with the following operators: two individuals (parent, A and B) are combined to create two new individuals (offspring, child1 and child2). Parents are randomly selected.
i. Calculate the average of row for each condition
ii. Calculate the average of row for all conditions
λt=∑t∈T′corr(mr(t),mmr)|T′| |
mr(t) is the row average condition of each tricluster, mmr is the row average of all conditions from each tricluster and |T′| is the number of conditions or the depth of the tricluster.
Correlation is the relationship between two vectors. A widely used correlation measure is Pearson's correlation. Pearson's correlation formula based on [16] is for vector mr(t) and mmr is Mutation: an individual can mutate according to the possibility of mutation. The mutation probability is verified for each individual. First, it is necessary to generate a random number from 0-1 equal to the total number of genes, i.e., the individual multiplied by the number of n-bits, and if the random number generated is less than the specified probability of mutation, then a mutation will be carried out in the gene. If the value generated is more than the mutation probability, the mutation process is carried out in the gene. This action is to change the value of the gene if 0 becomes 1 and if the initial value 1 is mutated to 0.
The main steps in the MOEA algorithm are as follows: 1) Generate a random size population matrix P, 2) Delete or add rows or columns using local search, 3) Calculate the fitness function, 4) Rank the population using dominant criteria, 5) Calculate the crowding distance value, 6) Show the selection results using the crowding tournament selection, 7) Perform crossover and mutation to produce offspring populations, 8) Combine the parent and offspring population.
Intertemporal homogeneity measures gene homogeneity in various fields of gene observation. For triclusters, intertemporal homogeneity is calculated as follows:
corr(mr(t),mmr)=∑(mr(t)−¯(mr(t)))(mmr−(¯mmr))√∑(mr(t)−(¯mr(t)))2×√∑(mmr−¯mmr)2 | (2.15) |
Pearson's correlation has a value of −1≤corr(mr(t),mmr)≤1. A correlation of +1 means that both are positively linear, while a correlation of −1 means that both are negatively linear. Perfect correlation is a correlation that has a value of 0.
Trigen algorithm is an algorithm based on the theory of evolution, genetic algorithm [18]. Genetic algorithm is an algorithm that aims to maximize a problem that will produce the best solution. Because in the Trigen algorithm we want to produce N-set triclusters, we need to do a genetic algorithm that is N-times.
For the Trigen algorithm, MSL results are added to the genetic algorithm fitness function FF(TRI) along with the individul size and overlap control [23]. MSL combined with six other factors to be a weighted average. The first three factors is 1−|TRIG||DG|, 1−|TRIC||DC|, and 1−|TRIT||DT| measure the number of genes, conditions, and time of TRI(TRIG,C,T) compared to the size of the dataset (|DG,C,T|). Because MSL minimizes the fitness function, therefore on these three factors are made 1- each proportion to produce a TRI with a larger size when the parameter wg, wc or wt is increased. The next three factors RG(TRI,SOL)|TRIG|×|SOLL|, RC(TRI,SOL)|TRIC|×|SOLL|, and RT(TRI,SOL)|TRIT|×|SOLL| measure the number of genes, conditions, and time or depth elements TRI on the set of solutions that have been found previously SOL(|TRIG,C,T|×|SOL|) to produce TRI with a small overlap as the wag, wac, or wat value increases. Finally, the main function MSL(TRI)2π measures the MSL(TRI) proportional value close to its maximum value of 2π to produce TRI with a small MSL value when the wf value is increased. The default total configuration value for wf, wg, wc, wt, wag, wac, wat is 1, with fix value for wf is 0.8 and the total others variables are 0.2.
FF(TRI)=(wf×MSL(TRI)2π+wg(1−|TRIG|DG)+wc(1−|TRIC|DC)+wt(1−|TRIT|DT)+wag×RG(TRI,SOL)|TRIG|×|SOL|+wac×Rc(TRI,SOL)|TRIC|×|SOL|+wat×RT(TRI,SOL)|TRIT|×|SOL|) | (2.16) |
The δ-Trimax method is a development of the CC algorithm. This method was developed by Anirban Bhar, who generated triclusters in the form of sub-spaces from 3D data. This method aims to find triclusters that have a small mean square residual from δ, where the δ is the threshold determined by the researcher [10].
Suppose 3D data Z(A,B,C), M⊆Z, so that M(I,J,K) is subspace with I⊆A, J⊆B and K⊆C. The mean square residual(MSR) can be obtained using Eq (2.17)
S=1|I||J||K|∑i∈I,j∈J,k∈Kr2ijk=1|I||J||K|∑i∈I,j∈J,k∈K(mijk−miJK−mIjK−mIJk+2mIJK)2 | (2.17) |
where i∈I, j∈J, k∈K.
The δ-Trimax method consists of several algorithms, including single node deletion, multiple node deletion, node addition, and masking. In the single node deletion and multiple node deletion stages, the nodes will be deleted until the MSR is smaller than the specified delta. After deletion is done, node addition continues to maximize the obtained tricluster volume while still maintaining a small MSR of the delta. Then, masking is done to find other triclusters. The flowchart Trimax delta algorithm can be seen in the Figure 4.
In this research, we do preliminary research to find biomarkers of HIV-1 disease using 3 Tricluster Methods: THD Tricluster, TRIGen, and δ-Trimax with three dimension Microarray data namely observations, probe id genes, and conditions (acute, chronic, non-progressor, uninfected) (Figure 5).
In the generate biclusters stage, a bicluster search is carried out for the new residue score using a lift algorithm that has two stages, namely, single-node deletion and single-node addition, by setting a threshold value of δ=0.08. Biclusters are obtained in different amounts from each condition. The normal condition results in three biclusters, the acute condition results in 100 biclusters, the chronic condition results in 100 biclusters, and the non-progressor condition results in 13 biclusters. In the generate triclusters stage, the tricluster search is carried out in stages, which include looking for the probe ID slices and observations of the bicluster. In the tricluster search process, based on the results of the bicluster with a new residue score, the minimum probe ID minp=5 is determined, and the minimum observation mino=2 for the tricluster is obtained. This determination results in 32 triclusters. The results of all triclusters after validation through the GPL96 platform downloaded on NCBI showed that three genes were known to be associated with HIV-1: JUN, ELF-1, and HLA-C.
Biclustering with a modified CC algorithm using transposed virtual error size and parameter δ=0.4 and λ=2.5 results in less than 50 biclusters. Biclustering results in 38 biclusters in normal conditions, 31 in acute conditions, 49 in chronic conditions, and 37 in non-progressor conditions. The bicluster results of this bicluster are then sliced under each condition. In the tricluster search process, based on the biclustering results with transposed virtual error, the minimum probe ID minp=15 is determined along with the minimum observation mino=3 for the tricluster. From this determination, four triclusters are obtained.
The use of transposed virtual errors in the THD triclustering method successfully solves the triclustering problem in 3D gene expression data by producing four triclusters at a depth of four (normal, acute, chronic, and non-progressor conditions), with an inter-temporal value of 0.9 for each tricluster. This algorithm requires several parameters to work: alpha and delta symbolized by α and δ in the biclustering process and the minimum parameters of observation and minimum probe gene symbolized by mo and mp in the triclustering process. The parameters selected in this study use the same value for each condition. The parameters α=2.5 and δ=0.4 are selected for the biclustering search, and the minimum probability of the selected gene is 15 with a minimum of four observations in the triclustering search process. From the whole research process, four tricluster are generated at a depth of four (normal, acute, chronic, and non-progressor conditions).
In this study, the number of individuals used per iteration was 100, the number of multi-objective functions was two, and the threshold (δ) selection was based on the preliminary data transpose virtual error (VEt) value. The researcher used 10 generations with a crossover probability of 0.8 and a mutation probability of 0.1. Two trials were conducted to select the best bicluster for the threshold of 0.5. The results of the MOEA cluster are shown in Tables 1 and 2.
Condition | Measure |
Uninfected | 1264 probe × 4 observation |
Acute | 1266 probe × 3 observation |
Chronic | 1311 probe × 6 observation |
Nonprogressor | 1208 probe × 3 observation |
Condition | Measure |
Uninfected | 1273 probe × 5 observation |
Acute | 1265 probe × 5 observation |
Chronic | 1292 probe × 5 observation |
Nonprogressor | 1183 probe × 5 observation |
Figure 6 is an example of a bicluster gene expression graph for non-progressor conditions. If we strike a line through the dots, we can see the slope of the lines between probe ID genes are almost the same, so it can be concluded that the shift and scaling patterns of the graph are fulfilled by transpose virtual error detection.
From the bicluster results, each condition was examined for a slice between the biclusters to obtain a tricluster with a depth of two. The results of triclusters with a depth of two from trials one and two are shown in Table 3.
Condition | Measure |
Uninfected and acute (1) | 621 probe × 2 observation |
Uninfected and chronic (1) | 655 probe × 4 observation |
Acute and chronic (1) | 657 probe × 2 observation |
Uninfected and acute (2) | 626 probe × 4 observation |
Uninfected and chronic (2) | 644 probe × 4 observation |
Acute and chronic (2) | 647 probe × 4 observation |
Acute and nonprogressor (2) | 657 probe × 2 observation |
*Note: (1) = Experiment 1, (2) = Experiment 2 |
The three-state tricluster is the result of a two-state tricluster slice. In this study, the first step was extracting a tricluster with a depth of two, which was extracted based on the results of the tricluster uninfected with acute, uninfected with chronic, and acute with chronic. The two-depth tricluster was obtained by slicing the probe ID gene and the samples in the two-depth tricluster. The results of the three-depth tricluster are shown in Table 4.
Condition | Measure |
Uninfected, acute and chronic (1) | 325 probe × 2 observation |
Uninfected, acute and chronic (2) | 326 probe × 3 observation |
Uninfected, acute and nonprogressor (2) | 310 probe × 1 observation |
*Note: (1)=Experiment 1, (2)=Experiment 2 |
From the results of Experiment 1, it was obtained tricluster with three conditions, namely uninfected, acute and chronic conditions with 325 probe id genes in observation one and observation two. Whereas in Experiment 2, the tricluster obtained in the Uninfected, Acute and Chronic conditions with the probe id genes was 326 in conditions two, three and four. In experiment two with a depth of three, a slice between Acute, Chronic and Nonprogressor was obtained, but only one observation. In this study, there was no tricluster with a depth of 4.
Intertemporal homogeneity was used to measure the tricluster results. This involved an evaluation of each tricluster. Based on the intertemporal homogeneity, the tricluster results for the two-condition tricluster and the three-condition tricluster were very good because the correlation values for all triclusers were close to one. The following shows the results of the tricluster correlation for Experiments 1 and 2.
Based on Experiments 1 and 2 above, it was found that triclusters were only obtained at depths two and three, while none were found at depth four. Experiment 1 showed that there was no relationship between non-progressors and the other three conditions (i.e., uninfected, acute, and chronic conditions) from the probe ID genes selected through the relative deviation and absolute deviation probe ID genes. Meanwhile, for the tricluster with a depth of three, the probe ID gene 208812_x_at contained HLA-C and probe ID gene 209602_s_at contained GATA-3. According to Kakati, et al., HLA-C and GATA-3 are genes related to HIV-1. In the second experiment, the tricluster with a depth of three obtained the same results, that is, the probe ID gene 208812_x_at contained HLA-C, while another probe ID gene, 201465_s_at, contained a gene with the name JUN. In the THD-tricluster paper, JUN showed a relation with HIV-1.
Correlation Value | Uninfected | Acute | Chronic |
(Un, Ac, Ch) | 0.989 | 0.983 | 0.976 |
Un, Ac | 0.996 | 0.994 | |
Un, Ch | 0.993 | 0.993 | |
Ac, Ch | 0.978 | 0.987 |
Correlation Value | Un | Ac | Ch | Np |
(Un, Ac, Ch) | 0.986 | 0.987 | 0.984 | |
Un, Ac | 0.994 | 0.99 | ||
Un, Ch | 0.991 | 0.994 | ||
Ac, Ch | 0.986 | 0.993 | ||
Ac, Np | 0.985 | 0.986 |
Thus, in this study, the probe ID gene with ID 208812_x_at was obtained with the gene name HLA-C, the probe ID gene 209602_s_at with the gene name GATA-3, and the probe ID gene 201465_s_at with the name JUN. All three of these probe ID genes are associated with HIV-1. In addition to the analysis of gene ID probes obtained by [19], the researchers collected gene ID probe data from the NCBI website https://www.ncbi.nlm.nih.gov/geo/query/acc.cgi?acc=GPL96.
For the Trigen algorithm with a multi-slope measure size, the 10 tricluster results obtained are of the size shown in Table 7.
Measure | Probe | Observation | Depth |
TRI1 | 1260 | 2(O2,O5) | 4 condition |
TRI2 | 1344 | 5(O4,O5,O7,O9,O10) | 4 condition |
TRI3 | 1256 | 3(O1,O7,O9) | 4 condition |
TRI4 | 1259 | 7(O1,O2,O4,O6,O8,O9,O10) | 4 condition |
TRI5 | 1264 | 5(O2,O4,O5,O6,O7) | 4 condition |
TRI6 | 1245 | 3(O2,O4,O8) | 4 condition |
TRI7 | 1300 | 3(O1,O7,O9) | 4 condition |
TRI8 | 1276 | 7(O1,O2,O3,O4,O6,O7,O10) | 4 condition |
TRI9 | 1291 | 3(O2,O4,O10) | 4 condition |
TRI10 | 1297 | 4(O4,O5,O7,O9) | 4 condition |
First, it could be seen based on Table 7 that the resulting tricluster weight size is maximum. Moreover, the number of probe ID genes, which exceeded 50% of the total probe ID genes in the initial dataset, was 2577. Second, it can be seen the tricluster solutions did not have a large overlap. Only one or two samples in this study had the same observations, and there were also tricluster results that did not have the same observation coordinates. This indicated that the overlap parameters worked properly. The following is a graphical example of the representation of the results of tricluster 1 in Figure 7. It can be seen that the variations in the probe ID gene expressions of the two samples or observations have almost the same shape for each condition. This showed that the results of the grouping had the same pattern in all conditions.
Hence, based on the aim of the Trigen algorithm, which maximizes the fitness function in which the Trigen fitness algorithm function in this study has been added, the multi-slope measure measure worked well for grouping data that have the same pattern. From the analysis of the 10 tricluster results obtained, six genes related to HIV were obtained based on the gene bank table [19], namely HLA-C, JUN, CCR5, ELF1, CX3CR1, and GATA-3.
A tricluster search of HIV data with δ-Trimax was carried out by [10]. By using δ=0.0046 and λ=1.25, we get 202 triclusters. Next, the tricluster with the smallest TQI is selected. From this tricluster, genes related to HIV-1 were obtained, namely AGFG1, EGR1, and HLA-C.
A comparison of the results of each method can be seen in the Table 8. The results of each tricluster contains several genes associated with HIV disease, these HIV genes refer to research that has been carried out by Kakati [19].
Method | Measurement | Parameter | Total of tricluster | Gene HIV-1 |
THD-Tricluster | New Residue Score | minp=5;mino=2;δ=0.08;δin=0.8 | 32 | HLA-C; ELF-1; JUN |
Transposed Virtual Error | minp=5;mino=2;α=2.5;δ=0.4;δin=0.9 | 4 | ELF-1; HLA-C | |
MOEA with Transposed Virtual Error | α=1.5;δ=0.5; Iteration 10 | 1 | HLA-C; GATA-3; JUN | |
Trigen | Trigen with MSL | Iteration 5 | 10 | HLA-C; JUN; CCR5; ELF1; CX3CR1; GATA-3 |
δ-Trimax | Mean Square Residual | δ=0.0046 and λ=1.25 | 202 | AGFG1; EGR1; HLA-C |
Based on the THD-Tricluster method, the tricluster results obtained by using a new residue score resulted in 32 triclusters with genes associated with HIV, including ELF-1, HLA-C, and JUN. The tricluster results obtained using the transposed virtual error size included triclusters with two genes associated with HIV: ELF-1 and HLA-C. When using δ-trimax, 202 triclusters were obtained with three genes associated with HIV: AGFG1, EGR1, and HLA-C.
The bicluster results were used to generate triclusters. Tricluster results were obtained for depth two and depth three. The tricluster results regarding HIV-1 gene expression data showed genes associated with HIV-1, namely, HLA-C, GATA-3, and JUN. Based on the simulation results of the Trigen algorithm program with multi-slope measure evaluation, the target of 10 triclusters containing HIV-1 biomarkers (HLA-C, JUN, CCR5, ELF1, CX3CR1, and GATA-3) was successfully achieved in all conditions (i.e., uninfected, acute, chronic, and non-progressors). Therefore, based on the five methods utilized in this study, an HIV biomarker was obtained: HLA-C.
NKB-035/UN2.F3/HKP.05.00/2021 is the number of the research grant provided by FMIPA Universitas Indonesia.
The authors declare there is no conflict of interest.
[1] | G. Ardaneswari, A. Bustamam, T. Siswantining, Implementation of parallel k-means algorithm for two-phase method biclustering in carcinoma tumor gene expression data, in AIP Conference Proceedings, 1825 (2017). https://doi.org/10.1063/1.4978973 |
[2] |
T. Siswantining, N. P. Purwandani, M. Susilowati, A. Wibowo, Geoinformatics of tuberculosis (TB) disease in Jakarta city Indonesia, GEOMATE J., 19 (2020), 35–42. https://doi.org/10.21660/2020.72.5599 doi: 10.21660/2020.72.5599
![]() |
[3] | M. A. Latief, A. Bustamam, T. Siswantining, Performance evaluation xgboost in handling missing value on classification of hepatocellular carcinoma gene expression data, in 2020 4th International Conference on Informatics and Computational Sciences (ICICoS), (2020), 1–6. |
[4] | T. Siswantining, T. Anwar, D. Sarwinda, H. Al-Ash, A novel centroid initialization in missing value imputation towards mixed datasets, Commun. Math. Biol. Neurosci., 2021 (2021). https://doi.org/10.28919/cmbn/5344 |
[5] | M. A. Latief, T. Siswantining, A. Bustamam, D. Sarwinda, A comparative performance evaluation of random forest feature selection on classification of hepatocellular carcinoma gene expression data, in 2019 3rd International Conference on Informatics and Computational Sciences (ICICoS), (2019), 1–6. |
[6] | D. A. Apriana, T. Siswantining, D. Sarwinda, S. M. Soemartojo, Triclustering analysis using extended dimension iterative signature algorithm (edisa) on lung disease gene expression data, in 2020 3rd International Conference on Biomedical Engineering (IBIOMED), IEEE, (2020), 7–12. |
[7] | I. M. Sari, S. M. Soemartojo, T. Siswantining, D. Sarwinda, Mining biological information from 3d medulloblastoma cancerous gene expression data using timesvector triclustering method, in 2020 4th International Conference on Informatics and Computational Sciences (ICICoS), (2020), 1–6. |
[8] |
A. Bustamam, T. Siswantining, T. P. Kaloka, O. Swasti, Application of BiMax, POLS, and LCM-MBC to find bicluster on interactions protein between HIV-1 and human, Austrian J. Stat., 49 (2020), 1–18. https://doi.org/10.17713/ajs.v49i3.1011 doi: 10.17713/ajs.v49i3.1011
![]() |
[9] |
O. Alter, G. H. Golub, Singular value decomposition of genome-scale mrna lengths distribution reveals asymmetry in rna gel electrophoresis band broadening, Proc. Natl. Acad. Sci., 103 (2006), 11828–11833. https://doi.org/10.1073/pnas.0604756103 doi: 10.1073/pnas.0604756103
![]() |
[10] |
T. Siswantining, N. Saputra, D. Sarwinda, H. S. Al-Ash, Triclustering discovery using the δ-trimax method on microarray gene expression data, Symmetry, 13 (2021), 437. https://doi.org/10.3390/sym13030437 doi: 10.3390/sym13030437
![]() |
[11] | H. Ahmed, P. Mahanta, D. Bhattacharyya, J. Kalita, A. Ghosh, Intersected coexpressed subcube miner: An effective triclustering algorithm, in 2011 World Congress on Information and Communication Technologies, IEEE, (2011), 846–851. |
[12] | P. S. Mahiskar, A. Bhade, P. Chatur, The data mining triclustering algorithm for mining real valued datasets-a review, Int. J. Comput. Sci. Eng. Technol., 2 (2012). |
[13] | A. Rachma, S. Soemartojo, T. Siswantining, Thd-tricluster method on gene expression data of multiple sclerosis patients receiving interferon-beta therapy, in AIP Conference Proceedings, 2374 (2021), 030002. https://doi.org/10.1063/5.0058711 |
[14] | E. A. Octaria, T. Siswantining, A. Bustamam, D. Sarwinda, Kernel PCA and SVM-RFE based feature selection for classification of dengue microarray dataset, in AIP Conference Proceedings, 2264 (2020), 03004. https://doi.org/10.1063/5.0023930 |
[15] | A. T. M. Siregar, T. Siswantining, A. Bustamam, D. Sarwinda, Comparison of supervised models in hepatocellular carcinoma tumor classification based on expression data using principal component analysis (PCA), in AIP Conference Proceedings, 2264 (2020), 030002. https://doi.org/10.1063/5.0023931 |
[16] | W. H. Yang, D. Q. Dai, H. Yan, Finding correlated biclusters from gene expression data, IEEE Trans. Knowl. Data Eng., 23 (2011), 568–584. |
[17] |
A. Trkola, Hiv–host interactions: vital to the virus and key to its inhibition, Curr. Opin. Microbiol., 7 (2004), 407–411. https://doi.org/10.1016/j.mib.2004.06.002 doi: 10.1016/j.mib.2004.06.002
![]() |
[18] |
D. Gutiérrez-Avilés, C. Rubio-Escudero, F. Martínez-Álvarez, J. C. Riquelme, TriGen: A genetic algorithm to mine triclusters in temporal gene expression data, Neurocomputing, 132 (2014), 42–53. https://doi.org/10.1016/j.neucom.2013.03.061 doi: 10.1016/j.neucom.2013.03.061
![]() |
[19] |
T. Kakati, H. A. Ahmed, D. K. Bhattacharyya, J. K. Kalita, Thd-tricluster: A robust triclustering technique and its application in condition specific change analysis in hiv-1 progression data, Comput. Biol. Chem., 75 (2018), 154–167. https://doi.org/10.1016/j.compbiolchem.2018.05.007 doi: 10.1016/j.compbiolchem.2018.05.007
![]() |
[20] | Y. Cheng, G. M. Church, Biclustering of expression data, in Ismb, 8 (2000), 93–103. |
[21] |
A. Bustamam, S. Formalidin, T. Siswantining, Z. Rustam, Finding correlated biclusters from microarray data using the modified lift algorithm based on new residue score, Int. J. Data Min. Bioinf., 24 (2020), 326. https://doi.org/10.1504/ijdmb.2020.113691 doi: 10.1504/ijdmb.2020.113691
![]() |
[22] |
B. Pontes, R. Girldez, J. S. Aguilar-Ruiz, Quality measures for gene expression biclusters, PloS One, 10 (2015), e0115497. https://doi.org/10.1371/journal.pone.0115497 doi: 10.1371/journal.pone.0115497
![]() |
[23] | D. Gutiérrez-Avilés, C. Rubio-Escudero, MSL: a measure to evaluate three-dimensional patterns in gene expression data, Evol. Bioinf., 11 (2015), EBO-S25822. https://journals.sagepub.com/doi/full/10.4137/EBO.S25822 |
1. | Wilhelmina A.N. Daeng, Titin Siswantining, Alhadi Bustamam, Prasnurzaki Anki, 2022, $\delta$-TRIMAX Method with Silhouette Coefficient on Microarray Gene Expression Data for Early Detection of Heart Failure, 978-1-6654-5140-6, 412, 10.1109/ICOIACT55506.2022.9971865 | |
2. | David Gutierrez-Aviles, Jose F. Torres, Francisco Martinez-Alvarez, Jairo Cugliari, 2024, An evolutionary triclustering approach to discover electricity consumption patterns in France, 9798400702433, 386, 10.1145/3605098.3636034 | |
3. | Marcin Michalak, Searching for Continuous n-Clusters with Boolean Reasoning, 2024, 16, 2073-8994, 1286, 10.3390/sym16101286 | |
4. | Marcin Michalak, Triclustering based on Boolean reasoning — a proof–of–concept, 2024, 246, 18770509, 2351, 10.1016/j.procs.2024.09.538 |
Condition | Measure |
Uninfected | 1264 probe × 4 observation |
Acute | 1266 probe × 3 observation |
Chronic | 1311 probe × 6 observation |
Nonprogressor | 1208 probe × 3 observation |
Condition | Measure |
Uninfected | 1273 probe × 5 observation |
Acute | 1265 probe × 5 observation |
Chronic | 1292 probe × 5 observation |
Nonprogressor | 1183 probe × 5 observation |
Condition | Measure |
Uninfected and acute (1) | 621 probe × 2 observation |
Uninfected and chronic (1) | 655 probe × 4 observation |
Acute and chronic (1) | 657 probe × 2 observation |
Uninfected and acute (2) | 626 probe × 4 observation |
Uninfected and chronic (2) | 644 probe × 4 observation |
Acute and chronic (2) | 647 probe × 4 observation |
Acute and nonprogressor (2) | 657 probe × 2 observation |
*Note: (1) = Experiment 1, (2) = Experiment 2 |
Condition | Measure |
Uninfected, acute and chronic (1) | 325 probe × 2 observation |
Uninfected, acute and chronic (2) | 326 probe × 3 observation |
Uninfected, acute and nonprogressor (2) | 310 probe × 1 observation |
*Note: (1)=Experiment 1, (2)=Experiment 2 |
Correlation Value | Uninfected | Acute | Chronic |
(Un, Ac, Ch) | 0.989 | 0.983 | 0.976 |
Un, Ac | 0.996 | 0.994 | |
Un, Ch | 0.993 | 0.993 | |
Ac, Ch | 0.978 | 0.987 |
Correlation Value | Un | Ac | Ch | Np |
(Un, Ac, Ch) | 0.986 | 0.987 | 0.984 | |
Un, Ac | 0.994 | 0.99 | ||
Un, Ch | 0.991 | 0.994 | ||
Ac, Ch | 0.986 | 0.993 | ||
Ac, Np | 0.985 | 0.986 |
Measure | Probe | Observation | Depth |
TRI1 | 1260 | 2(O2,O5) | 4 condition |
TRI2 | 1344 | 5(O4,O5,O7,O9,O10) | 4 condition |
TRI3 | 1256 | 3(O1,O7,O9) | 4 condition |
TRI4 | 1259 | 7(O1,O2,O4,O6,O8,O9,O10) | 4 condition |
TRI5 | 1264 | 5(O2,O4,O5,O6,O7) | 4 condition |
TRI6 | 1245 | 3(O2,O4,O8) | 4 condition |
TRI7 | 1300 | 3(O1,O7,O9) | 4 condition |
TRI8 | 1276 | 7(O1,O2,O3,O4,O6,O7,O10) | 4 condition |
TRI9 | 1291 | 3(O2,O4,O10) | 4 condition |
TRI10 | 1297 | 4(O4,O5,O7,O9) | 4 condition |
Method | Measurement | Parameter | Total of tricluster | Gene HIV-1 |
THD-Tricluster | New Residue Score | minp=5;mino=2;δ=0.08;δin=0.8 | 32 | HLA-C; ELF-1; JUN |
Transposed Virtual Error | minp=5;mino=2;α=2.5;δ=0.4;δin=0.9 | 4 | ELF-1; HLA-C | |
MOEA with Transposed Virtual Error | α=1.5;δ=0.5; Iteration 10 | 1 | HLA-C; GATA-3; JUN | |
Trigen | Trigen with MSL | Iteration 5 | 10 | HLA-C; JUN; CCR5; ELF1; CX3CR1; GATA-3 |
δ-Trimax | Mean Square Residual | δ=0.0046 and λ=1.25 | 202 | AGFG1; EGR1; HLA-C |
Condition | Measure |
Uninfected | 1264 probe × 4 observation |
Acute | 1266 probe × 3 observation |
Chronic | 1311 probe × 6 observation |
Nonprogressor | 1208 probe × 3 observation |
Condition | Measure |
Uninfected | 1273 probe × 5 observation |
Acute | 1265 probe × 5 observation |
Chronic | 1292 probe × 5 observation |
Nonprogressor | 1183 probe × 5 observation |
Condition | Measure |
Uninfected and acute (1) | 621 probe × 2 observation |
Uninfected and chronic (1) | 655 probe × 4 observation |
Acute and chronic (1) | 657 probe × 2 observation |
Uninfected and acute (2) | 626 probe × 4 observation |
Uninfected and chronic (2) | 644 probe × 4 observation |
Acute and chronic (2) | 647 probe × 4 observation |
Acute and nonprogressor (2) | 657 probe × 2 observation |
*Note: (1) = Experiment 1, (2) = Experiment 2 |
Condition | Measure |
Uninfected, acute and chronic (1) | 325 probe × 2 observation |
Uninfected, acute and chronic (2) | 326 probe × 3 observation |
Uninfected, acute and nonprogressor (2) | 310 probe × 1 observation |
*Note: (1)=Experiment 1, (2)=Experiment 2 |
Correlation Value | Uninfected | Acute | Chronic |
(Un, Ac, Ch) | 0.989 | 0.983 | 0.976 |
Un, Ac | 0.996 | 0.994 | |
Un, Ch | 0.993 | 0.993 | |
Ac, Ch | 0.978 | 0.987 |
Correlation Value | Un | Ac | Ch | Np |
(Un, Ac, Ch) | 0.986 | 0.987 | 0.984 | |
Un, Ac | 0.994 | 0.99 | ||
Un, Ch | 0.991 | 0.994 | ||
Ac, Ch | 0.986 | 0.993 | ||
Ac, Np | 0.985 | 0.986 |
Measure | Probe | Observation | Depth |
TRI1 | 1260 | 2(O2,O5) | 4 condition |
TRI2 | 1344 | 5(O4,O5,O7,O9,O10) | 4 condition |
TRI3 | 1256 | 3(O1,O7,O9) | 4 condition |
TRI4 | 1259 | 7(O1,O2,O4,O6,O8,O9,O10) | 4 condition |
TRI5 | 1264 | 5(O2,O4,O5,O6,O7) | 4 condition |
TRI6 | 1245 | 3(O2,O4,O8) | 4 condition |
TRI7 | 1300 | 3(O1,O7,O9) | 4 condition |
TRI8 | 1276 | 7(O1,O2,O3,O4,O6,O7,O10) | 4 condition |
TRI9 | 1291 | 3(O2,O4,O10) | 4 condition |
TRI10 | 1297 | 4(O4,O5,O7,O9) | 4 condition |
Method | Measurement | Parameter | Total of tricluster | Gene HIV-1 |
THD-Tricluster | New Residue Score | minp=5;mino=2;δ=0.08;δin=0.8 | 32 | HLA-C; ELF-1; JUN |
Transposed Virtual Error | minp=5;mino=2;α=2.5;δ=0.4;δin=0.9 | 4 | ELF-1; HLA-C | |
MOEA with Transposed Virtual Error | α=1.5;δ=0.5; Iteration 10 | 1 | HLA-C; GATA-3; JUN | |
Trigen | Trigen with MSL | Iteration 5 | 10 | HLA-C; JUN; CCR5; ELF1; CX3CR1; GATA-3 |
δ-Trimax | Mean Square Residual | δ=0.0046 and λ=1.25 | 202 | AGFG1; EGR1; HLA-C |