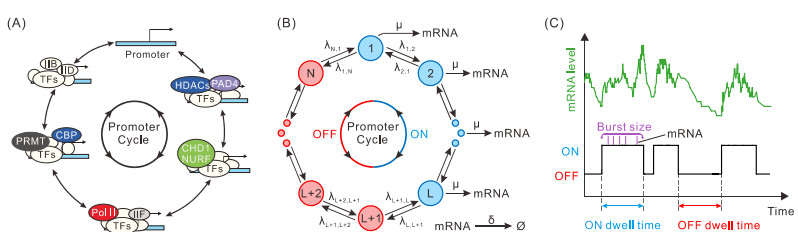
While transcription often occurs in a bursty manner, various possible regulations can lead to complex promoter patterns such as promoter cycles, giving rise to an important question: How do promoter kinetics shape transcriptional bursting kinetics? Here we introduce and analyze a general model of the promoter cycle consisting of multi-OFF states and multi-ON states, focusing on the effects of multi-ON mechanisms on transcriptional bursting kinetics. The derived analytical results indicate that burst size follows a mixed geometric distribution rather than a single geometric distribution assumed in previous studies, and ON and OFF times obey their own mixed exponential distributions. In addition, we find that the multi-ON mechanism can lead to bimodal burst-size distribution, antagonistic timing of ON and OFF, and diverse burst frequencies, each further contributing to cell-to-cell variability in the mRNA expression level. These results not only reveal essential features of transcriptional bursting kinetics patterns shaped by multi-state mechanisms but also can be used to the inferences of transcriptional bursting kinetics and promoter structure based on experimental data.
Citation: Xiyan Yang, Zihao Wang, Yahao Wu, Tianshou Zhou, Jiajun Zhang. Kinetic characteristics of transcriptional bursting in a complex gene model with cyclic promoter structure[J]. Mathematical Biosciences and Engineering, 2022, 19(4): 3313-3336. doi: 10.3934/mbe.2022153
[1] | Jiajun Zhang, Tianshou Zhou . Stationary moments, distribution conjugation and phenotypic regions in stochastic gene transcription. Mathematical Biosciences and Engineering, 2019, 16(5): 6134-6166. doi: 10.3934/mbe.2019307 |
[2] | Qiuying Li, Lifang Huang, Jianshe Yu . Modulation of first-passage time for bursty gene expression via random signals. Mathematical Biosciences and Engineering, 2017, 14(5&6): 1261-1277. doi: 10.3934/mbe.2017065 |
[3] | Meiling Chen, Tianshou Zhou, Jiajun Zhang . Correlation between external regulators governs the mean-noise relationship in stochastic gene expression. Mathematical Biosciences and Engineering, 2021, 18(4): 4713-4730. doi: 10.3934/mbe.2021239 |
[4] | Qi Wang, Lifang Huang, Kunwen Wen, Jianshe Yu . The mean and noise of stochastic gene transcription with cell division. Mathematical Biosciences and Engineering, 2018, 15(5): 1255-1270. doi: 10.3934/mbe.2018058 |
[5] | Orit Lavi, Doron Ginsberg, Yoram Louzoun . Regulation of modular Cyclin and CDK feedback loops by an E2F transcription oscillator in the mammalian cell cycle. Mathematical Biosciences and Engineering, 2011, 8(2): 445-461. doi: 10.3934/mbe.2011.8.445 |
[6] | Xuan Zhang, Huiqin Jin, Zhuoqin Yang, Jinzhi Lei . Effects of elongation delay in transcription dynamics. Mathematical Biosciences and Engineering, 2014, 11(6): 1431-1448. doi: 10.3934/mbe.2014.11.1431 |
[7] | Heli Tan, Tuoqi Liu, Tianshou Zhou . Exploring the role of eRNA in regulating gene expression. Mathematical Biosciences and Engineering, 2022, 19(2): 2095-2119. doi: 10.3934/mbe.2022098 |
[8] | Shengming Zhou, Jia Zheng, Cangzhi Jia . SPREAD: An ensemble predictor based on DNA autoencoder framework for discriminating promoters in Pseudomonas aeruginosa. Mathematical Biosciences and Engineering, 2022, 19(12): 13294-13305. doi: 10.3934/mbe.2022622 |
[9] | Qiqi Deng, Aimin Chen, Huahai Qiu, Tianshou Zhou . Analysis of a non-Markov transcription model with nuclear RNA export and RNA nuclear retention. Mathematical Biosciences and Engineering, 2022, 19(8): 8426-8451. doi: 10.3934/mbe.2022392 |
[10] | LanJiang Luo, Haihong Liu, Fang Yan . Dynamic behavior of P53-Mdm2-Wip1 gene regulatory network under the influence of time delay and noise. Mathematical Biosciences and Engineering, 2023, 20(2): 2321-2347. doi: 10.3934/mbe.2023109 |
While transcription often occurs in a bursty manner, various possible regulations can lead to complex promoter patterns such as promoter cycles, giving rise to an important question: How do promoter kinetics shape transcriptional bursting kinetics? Here we introduce and analyze a general model of the promoter cycle consisting of multi-OFF states and multi-ON states, focusing on the effects of multi-ON mechanisms on transcriptional bursting kinetics. The derived analytical results indicate that burst size follows a mixed geometric distribution rather than a single geometric distribution assumed in previous studies, and ON and OFF times obey their own mixed exponential distributions. In addition, we find that the multi-ON mechanism can lead to bimodal burst-size distribution, antagonistic timing of ON and OFF, and diverse burst frequencies, each further contributing to cell-to-cell variability in the mRNA expression level. These results not only reveal essential features of transcriptional bursting kinetics patterns shaped by multi-state mechanisms but also can be used to the inferences of transcriptional bursting kinetics and promoter structure based on experimental data.
Transcription is a core step of gene-expression processes. For most genes in eukaryotic cells, transcription often occurs in a bursty fashion characterized by mRNA synthesis in short periods of activity followed by typically longer periods of inactivity. Single-cell experimental observations have provided evidence for such transcription bursting [1,2,3,4]. This variability, which potentially gives rise to cell-to-cell variability in gene expression levels [5,6,7], can propagate to downstream proteins or target genes through a network. It is believed that the expression noise can not only contribute to alternative cellular fates [8] but also maintain cellular functioning [9,10,11]. Revealing the mechanism of transcriptional bursting using mathematical models is an important step toward understanding fundamental cellular processes.
Transcriptional bursting kinetics is often characterized by two parameters: burst size and burst timing (or burst frequency). The former is defined as the number of transcripts produced during an active period, whereas the latter involves waiting times between transcription initiation events. For rate-limiting transcription or for transcription with exponential waiting times, mRNA burst sizes follow geometric distributions, which were also observed in early experiments [1,3,10,12]. However, transcription is a complex process involving many regulatory proteins and molecular factors, such as transcription factors, RNA polymerase, and chromatin remodeling complexes [13,14,15,16]. Recent experiments have shown that these factors or processes can result in non-exponential waiting-time distribution for genes' active (ON) and inactive (OFF) durations [17,18,19,20]. In addition, some experimental studies have also suggested complex promoter structures [21,22,23], which often involve nucleosomes competing with or being removed by transcription factors [24], and epigenetic regulation via histone modifications [25,26,27]. For example, Dunham et al. [28] found that the transcriptional deactivation occurs with graded, stepwise decreases in transcription rate, implying that the gene promoter has variable transcription states. Sepúlveda et al. [29] demonstrated that the lysogeny maintenance promoter of phage lambda switches between multiple promoter states. Neuert et al. [30] showed that the transcription is a multistate process for the osmotic stress response pathway in Saccharomyces cerevisiae. In a word, transcription is finished not in a simple single-step manner but often in a complex multistep fashion [31,32,33,34].
It has been verified that mathematical models are a powerful tool for estimating the impacts of molecular mechanisms on transcriptional bursting. In past decades, many theoretical models have been developed to characterize transcriptional bursting kinetics including burst size, burst frequency, and variability in the mRNA abundance [35,36]. Of these models, the most widely used model is the two-state model [17,37,38,39,40], where the promoter is assumed to switch between transcriptionally active (ON) and inactive (OFF) states with constant switching rates. Queuing models have also been proposed to analyze the impact of transcription regulation on bursting kinetics [31,41,42,43], where waiting-time distributions are general (either exponential or non-exponential). For example, Schwabe et al. used Erlang-distributed times to model ON-phase and transcription ignition, and found that burst sizes peaked distribution [44]. Kumar et al. derived steady-state moments of mRNA distribution and estimated burst parameters for non-geometric burst distribution [45]. Multistate gene models such as multi-OFF models [46,47], and multi-ON models [48,49], have been proposed to analyze transcription dynamics. Recently, Zoller et al. [50] combined mathematical modeling with experimental measurement to estimate burst parameters in a multi-OFF promoter cycle. In contrast, Rybakova et al. [51] used stochastic and ordinary differential equation models to estimate burst parameters in a multi-ON promoter cycle. In addition, Daigle et al. [52] inferred the number and configuration of promoter topologies from single-cell gene expression data. In spite of these efforts, how promoter kinetics affect transcription bursting kinetics remains poorly understood. Neither gene models with multi-ON states or with cyclic promoter structure, whose prototypes can be found in natural and synthetic systems [53], have been systematically studied, nor analytical results on burst size and timing have been reported.
In this paper, we introduce and analyze a model of stochastic transcription with cyclic promoter structure (i.e., ON and OFF states constitute a cycle), focusing on analysis of the effect of multi-ON mechanism on transcriptional bursting kinetics. Interesting results are obtained, e.g., burst size follows a mixed geometric distribution rather than a single geometric distribution that was assumed often in previous studies; each of ON and OFF times obeys a mixed exponential distribution; and the multi-ON mechanism can lead to bimodal burst-size distribution, antagonistic timing of ON and OFF. While our results reveal essential characteristics of transcriptional bursting kinetics, our analysis provides a framework for studying more complex transcription processes.
Here we introduce a biologically reasonable model for transcription bursting (Figure 1(A)), which considers the effects of many processes (or factors) such as recruitment of various polymerases and transcription factors, assembly of the pre-initiation complexes, chromatin remodeling, and histone modifications [13,14,15]. Then, we map this model into a theoretical model of multistate promoter cycle (Figure 1(B)) where the promoter proceeds sequentially through several reversible active (ON) states and inactive (OFF) states and returns to the active (ON) state, forming a cycle. We assume that transcription occurs in a bursting manner at each active state but is prohibited at the inactive states (Figure 1(C)). Specifically, we assume that the gene promoter has N states in total, which consists of L active states and K inactive states. We call L and K as active and inactive indexes, respectively. Denoted by A1,⋅⋅⋅,AL ON states and by I1,⋅⋅⋅,IK OFF states. The corresponding biochemical reactions are listed in Table 1.
Note that both L=1 and N>2 correspond to the multi-OFF model (i.e., the promoter has more than one OFF state but only one ON state), and both K=1 and N>2 correspond to the multi-ON model (i.e., the promoter has more than one ON state but only one OFF state). Therefore, the model introduced here includes transcription models that were previously studied [46,47,48,49], and can be used to model general transcription dynamics generated by gene promoters with multiple states. We point out that for the multi-OFF and multi-ON models, some analytical results on steady-state mRNA distribution and noise have been obtained [46,48], but for multistate promoter models, the analytical expressions on transcription bursting kinetics, such as distributions of burst size and timing, have not been derived so far.
Reactions | Description |
Aiλi,i+1⟷λi+1,iAi+1i=1,2,⋅⋅⋅,L−1 | Transitions between active states |
IjλL+j,L+j+1⟷λL+j+1,L+jIj+1,j=1,2,⋅⋅⋅,K−1 | Transitions between inactive states |
A1λ1,N⟷λN,1IK,ALλL,L+1⟷λL+1,LI1 | Transitions between active and inactive states |
Aiμ→Ai+mRNA, i=1,2,⋅⋅⋅,L | Transcription |
mRNAδ→∅ | mRNA degradation |
Let U represent the promoter's active state, which is a time-dependent random variable, i.e., U(t)=u∈{A1,⋯,AL}, B(t) be burst size, i.e., B(t)=n, n=0,1,2,⋅⋅⋅, and T represent the exit time (i.e., burst termination time) from an active state. We assume that at time t=0, the promoter begins to switch to active state A1 from inactive state IK, or to active state AL from inactive state I1. Let Sn,u(t) represents the survival probability that n mRNAs are produced per burst in state u at time t. Then, the transcriptional system's state at time t can be described as Sn,u(t)=Prob{T>t|B(t)=n,U(t)=u}, where we stipulate Sn,u(t)≡0(n<0). Note that the probability that burst termination time T falls within an infinitesimal interval (t,t+Δt) can be expressed as
Prob{T>t|B(t)=n,U(t)=u}=Sn,A1(t)λ1,NΔt+Sn,AL(t)λL,L+1Δt. | (1) |
Therefore, the joint probability density function Pburst(n,t) for burst size B(t) (a discrete random variable) and burst termination time T (a continuous random variable) includes all incoming fluxes driving the system from active state A1 to inactive state IK, or from active state AL to inactive state I1, that is, Pburst(n,t)=λ1,NSn,A1(t)+λL,L+1Sn,AL(t). Then, the steady-state probability distribution for burst size B is calculated according to
Pburst(n)=∫∞0Pburst(n,t)dt=λ1,N∫∞0Sn,A1(t)dt+λL,L+1∫∞0Sn,AL(t)dt. | (2) |
The key question is how Sn,A1(t) and Sn,AL(t) are obtained. However, if we rewrite Sn,Al(t) as Sn,l(t) for convenience, the master equation for survival probability Sn,l(t) can be described as
dSn,l(t)dt=μSn−1,l(t)−[μ+δl,1λl,N+(1−δl,1)λl,l−1+λl,l+1]Sn,l(t)+(1−δl,1)λl−1,lSn,l−1(t)+(1−δk,L)λl+1,lSn,l+1(t), | (3) |
where δi,j is a Kronecker delta. Note that this equation can be solved by introducing probability-generating functions. Specifically, defining Gl(z,t)=∞∑n=0Sn,l(t)zn (1⩽l⩽L) and denoting G(z,t)=(G1(z,t),⋯,GL(z,t))T, we can derive a linear partial differential equation group of the form: ∂G(z,t)/∂G(z,t)∂t∂t=H(z)G(z,t) [54,55], where the coefficient matrix H(z) is a tridiagonal matrix with the diagonal elements taking the form hl(z)=μ(z−1)−δl,1λl,N−(1−δl,1)λl,l−1−λl,l+1 (1⩽l⩽L) (seeing Appendix A for details).
Note that the distribution of the time that the promoter dwells on active (or inactive) state, denoted by fON(t) (or fOFF(t)), is equal to the sum of the times dwelling on all ON (or OFF) states. In order to calculate fON(t) and fOFF(t), we introduce two column vectors SON(t)=(SON1(t),⋯,SONL(t))T and SOFF(t)=(SOFF1(t),⋯,SOFFK(t))T, where SONl(t) and SOFFk(t) are the survival probabilities that the promoter is still at lth ON state and kth OFF state, respectively.
Now, we establish the master equation for SONl(t) and SOFFk(t). Denote by TON (L×L matrix) and TOFF (K×K matrix) the transition matrices of promoter among the active states and among the inactive states, respectively. Then, the master equations of the survival probability for ON and OFF states can take the following unified form
dSϑ(t)dt=TϑSϑ(t), | (4) |
where ϑ=ON or OFF.
Equation (4) is a linear equation group and is easily solved. In order to derive the analytical expression of Sϑ(t), we first introduce two matrices ΛON=diag(−λON1,⋯,−λONL) and ΛOFF=diag(−λOFF1,⋯,−λOFFK), where −λONl and −λOFFk are eigenvalues of matrices TON and TOFF, respectively. Then, we introduce two diagonal matrices RON=diag(γON1,⋅⋅⋅,γONL), where γON1=1 and γONl=l∏i=2√λi,i−1/λi,i−1λi−1,iλi−1,i (2⩽l⩽L), and ROFF=diag(γOFF1,⋅⋅⋅,γOFFK), where γOFF1 = 1 and γOFFk=k∏i=2√λL+i,L+i−1/λL+i,L+i−1λL+i−1,L+iλL+i−1,L+i (2⩽k⩽K). In addition, we define ˜Sϑ(t)=RϑSϑ(t) and ˜Tϑ=RϑTϑ(Rϑ)−1, where ϑ=ON or OFF. Note that there is an orthogonal matrix ˜Vϑ such that (˜Vϑ)T˜Tϑ˜Vϑ=Λϑ. Then, vector Sϑ(t) for the survival probabilities can be analytically expressed as
Sϑ(t)=(Rϑ)−1˜Vϑexp(Λϑt)(˜Vϑ)TRϑSϑ(0), | (5) |
where Sϑ(0) is the vector of initial survival probabilities determined by the steady-state distribution of the promoter states. To that end, the dwell-time distribution at the active and inactive states can be calculated according to
fϑ(t)=−∑jdSϑj(t)dt, | (6) |
where ϑ=ON or OFF.
After having obtained two dwell-time distributions at ON and OFF states, we can separately calculate the mean ON and OFF times, denoted by ⟨τON⟩ and ⟨τOFF⟩. In addition, the ON dwell-time noise (denoted by η2ON) and OFF dwell-time noise (denoted by η2OFF) can also be calculated according to Eq (6). Furthermore, the mean burst frequency (BF) can be formally expressed as
BF=1⟨τOFF⟩+⟨τON⟩. | (7) |
Note that the master equation for mRNA is different from that for burst size. Here we first establish the master equation for the former and then calculate the mean and noise of mRNA.
Let Ps(m;t) (1⩽s⩽N) represent the probability that the number of mRNA molecules is m at state A1,⋅⋅⋅,AL,I1,⋅⋅⋅,IK at time t, respectively. Set P(m;t)=[P1(m;t),⋅⋅⋅,PN(m;t)]T, and denote by A=(λk,l) the N×N transition matrix of a promoter. Note that λk,l=0 means that there is no transition occurrence from state k to state l. Let Ω=diag(μ,μ,⋅⋅⋅,μ,0,0,⋅⋅⋅,0) represent the transcription matrix. Then, the corresponding chemical master equation describing the mRNA dynamics takes the following form:
dP(m;t)dt=AP(m;t)+Ω(E−1−I)[P(m;t)]+δ(E−I)[mP(m;t)], | (8) |
where E,E−1 are shift operators and I is the identity operator.
Equation (8) can be solved using the binomial moment (BM) method that we previously developed [56]. The framework of this method is stated below. If the factorial BM for the probability Ps(m;t) is defined as a(s)i(t)=∑m⩾i(mi)Ps(m;t) (1⩽s⩽N), then the total BM for the total probability defined as P(m;t)=∑Ns=1Ps(m;t)(t) is calculated by bi(t)=∑Ns=1a(s)i(t). Note that b0(t)=1 due to conservative condition: ∑∞m=0P(m;t)=1. Furthermore, if we introduce column vector ai(t)=(a(1)i(t),⋅⋅⋅,a(N)i(t))T, it follows from Eq (8) that
ddtai(t)=−(iδI−A)ai(t)+Ωai−1(t), | (9) |
which is a linear ordinary differential equation group and is easily solved. Once all a(s)i(t) and further the total BMs bi(t) are obtained, the mRNA probability distribution can be reconstructed according to
P(m;t)=∑i⩾m(−1)i−m(im)bi(t). | (10) |
In addition, if the mRNA noise is quantified by the ratio of the variance over the squared mean (called noise intensity and denoted by η2mRNA), then η2mRNA is calculated according to
η2mRNA=2b2+b1−b21b21. | (11) |
Here we present analytical results, obtained according to the above analysis framework, to help understand the mechanism of how the cyclic promoter structure affects transcriptional bursting kinetics.
First, we present analytical results for burst-size distribution. Let P(0)s represent the steady-state probability that the promoter is in state s, where s=1,2,⋅⋅⋅,N, then these probabilities can be analytically derived (see Appendix B for details). Interestingly, we find that initial conditions Sn,l(0) are directly related to the steady-state distributions at the OFF states. Specifically, S0,1(0)=P(0)NλN,1/P(0)NλN,1(P(0)NλN,1+P(0)L+1λL+1,L)(P(0)NλN,1+P(0)L+1λL+1,L), S0,L(0)=P(0)L+1λL+1,L/P(0)L+1λL+1,L(P(0)NλN,1+P(0)L+1λL+1,L)(P(0)NλN,1+P(0)L+1λL+1,L), and Sn,l(0)=0 for other subscripts. Owing to the special structure of matrix H(z), generating function Gl(z,t) can be analytically expressed as
Gl(z,t)=L∑j=11γONl(λN,1P(0)N˜Vh1jγON1λN,1P(0)N+λL+1,LP(0)L+1+λL+1,LP(0)L+1˜VhLjγONLλN,1P(0)N+λL+1,LP(0)L+1)˜Vhlje−λhjt, | (12) |
where −λhl(1⩽l⩽L) are eigenvalues of matrix H(z) and thus are functions of z, and ˜Vhlj(1⩽l,j⩽L) are the elements of orthogonal eigenvector ˜Vhl = (˜Vh1l,˜Vh2l,⋯,˜VhLl)T associated with the eigenvalues −λhl. Furthermore, generating function Gburst(z) for burst-size distribution Pburst(n) can be expressed as
Gburst(z)=L∑j=1(λN,1P(0)N˜Vh1jγON1λN,1P(0)N+λL+1,LP(0)L+1+λL+1,LP(0)L+1˜VhLjγONLλN,1P(0)N+λL+1,LP(0)L+1)(λ1,N˜Vh1jγON1λhj+λL,L+1˜VhLjγONLλhj). | (13) |
Thus, burst-size distribution Pburst(n) can be given via Pburst(n)=(1/1n!n!)dnGburst(z)/dnGburst(z)dzndzn|z=0.
Second, we present analytical results for dwell-time distributions. Note that SONl(t) and SOFFk(t) for survival probabilities at the lth active state and at the kth inactive state can be respectively expressed as
SONl(t)=L∑j=11γONl(λN,1P(0)N˜VON1jγON1λN,1P(0)N+λL+1,LP(0)L+1+λL+1,LP(0)L+1˜VONLjγONLλN,1P(0)N+λL+1,LP(0)L+1)˜VONlje−λONjt, | (14a) |
SOFFk(t)=K∑j=11γOFFk(λ1,NP(0)1˜VOFF1jγOFF1λ1,NP(0)1+λL,L+1P(0)L+λL,L+1P(0)L˜VOFFKjγOFFKλ1,NP(0)1+λL,L+1P(0)L)˜VOFFkje−λOFFjt, | (14b) |
where ˜VONlj(1⩽l,j⩽L) and ˜VOFFkj(1⩽k,j⩽K) are the elements of matrices ˜VON=(˜VONlj) and ˜VOFF=(˜VOFFkj), respectively.
Using Eqs (14a) and (14b), the dwell-time distributions are thus expressed as
fϑ(t)=∑jcϑjλϑje−λϑjt, | (15) |
where if ϑ=ON, then
cONj=L∑k=11γONk(λN,1P(0)N˜VON1jγON1λN,1P(0)N+λL+1,LP(0)L+1+λL+1,LP(0)L+1˜VONLjγONLλN,1P(0)N+λL+1,LP(0)L+1)˜VONkj,1⩽j⩽L, |
and if ϑ=OFF, then
cOFFj=K∑k=11γOFFk(λ1,NP(0)1˜VOFF1jγOFF1λ1,NP(0)1+λL,L+1P(0)L+λL,L+1P(0)L˜VOFFKjγOFFKλ1,NP(0)1+λL,L+1P(0)L)˜VOFFkj,1⩽j⩽K. |
Equation (15) indicates that the dwell-time distribution at ON or OFF states is in general the sum of exponential distributions.
Third, we present analytical results for BMs. We find that the steady-state total BMs take the following form
bi=uN∏1l=i[(lδI−A)∗Ω] a0∏il=1det(lδI−A), | (16) |
where uN=(1,1,⋅⋅⋅,1) is an N− dimensional row vector, (iδI−A)∗ and det(iδI−A) are respectively the adjacency matrix and the determinant of matrix (iδI−A), and the initial vector a0 is the steady-state distribution of promoter states (see Appendix B).
In particular, the first-order binominal moment, i.e., the mRNA mean is given by (see Appendix C)
⟨mRNA⟩=b1=μδL∑s=1P(0)s, | (17) |
and the second-order binomial moment takes the form
b2=μ2∑Ll=1∑Lk=1W(1)klP(0)l2δN+1∏N−1k=1(1+α(1)k), | (18) |
where W(1)kl represents a determinant of an (N−1)×(N−1) matrix that is the left part of matrix (δI−A) by crossing out its kth row and lth column elements, and −α(1)1, −α(1)2, ⋅⋅⋅,−α(1)N−1 are the nonzero eigenvalues of matrix A/Aδδ. Then, we can show that the Fano factor for mRNA, defined as the ratio of the variance over the mean, is in general not equal to one, implying that the mRNA distribution is in general not Poissonian but may be super-Poissonian or sub-Poissonian.
We point out that all the above analytical results are formal and tell us the finite information on the effect of the cyclic promoter structure on transcriptional bursting kinetics. In order to clearly see how the cyclic promoter structure affects transcriptional bursting kinetics, we will consider special cases.
Here we consider two representative cases: (1) multi-OFF mechanism [46], i.e., L=1 and K=N−1; (2) mixture mechanism where all the forward (i.e., clockwise) transition rates of active states are identical (denoted by λf) and all the backward (i.e., counterclockwise) transitions of active states are also identical (denoted by λb); and all the forward transition rates of inactive states are the same (denoted by ρfλf with ρf being a common forward ratio) whereas all the backward transition rates of inactive states are also the same (denoted by ρbλb with ρb being a common backward ratio).
For the multi-OFF mechanism, according to general formula Eq (13), it is not difficult to show that the generating function for burst size is given by
Gburst(z)=λ1,N+λ1,2μ+λ1,N+λ1,2−z. | (19) |
Thus, the burst-size distribution takes the form
Pburst(n)=λ1,N+λ1,2μ+λ1,N+λ1,2(1−λ1,N+λ1,2μ+λ1,N+λ1,2)n,n=0,1,2,⋯ | (20) |
which is a geometric distribution. These results are in agreement with previous assumptions [44].
For the mixture mechanism, we denote a=λfλb and b=ρfλfρbλb. Using general formula Eq (15) and noting the fact: A∑l=1sin2lπA+1=A+12(A=L,K), we can show that
cONj=2bP(0)NsinjπL+1+P(0)L+1(√a)1−LsinLjπL+1(L+1)(bP(0)N+P(0)L+1)(L∑l=1(√a)l−1sinljπL+1),λONj=λb(1+a−2√acosjπL+1), | (21a) |
where j=1,2,⋯,L, and
cOFFj=2aP(0)LsinjπK+1+P(0)1(√b)1−KsinKjπK+1(K+1)(P(0)1+aP(0)L)(K∑k=1(√b)k−1sinkjπK+1),λOFFj=ρbλb(1+b−2√bcosjπK+1), | (21b) |
where j=1,2,⋯,K. Correspondingly, generating function Gburst(z) for burst size is given by (see Appendix D for derivation)
Gburst(z)=L∑j=1qj/qjλbλbμ(1−z)/μ(1−z)λbλb+1+a−2√acos(jπ/jπ(L+1)(L+1)), | (22) |
where
qj=2L+1(sinjπL+1+a(√a)L−1sinLjπL+1)(bP(0)NsinjπL+1bP(0)N+P(0)L+1+P(0)L+1(√a)1−LsinLjπL+1bP(0)N+P(0)L+1). |
Thus, the burst-size distribution takes the form
Pburst(n)=L∑j=1wjθj(1−θj)n, | (23) |
where n=0,1,2,⋯, and
θj=1+a−2√acos(jπ/jπ(L+1)(L+1))μ/μλbλb+1+a−2√acos(jπ/jπ(L+1)(L+1)), wj = qj/qjλbλb1+a−2√acos(jπ/jπ(L+1)(L+1)) with L∑j=1wj=1.
Equation (23) indicates that burst-size distribution is a mixture of geometric distributions. At the same time, the mean burst size is given by
BS=∂Gburst(z)∂z|z=1=L∑j=1μqj/μqjλ2bλ2b(1+a−2√acos(jπ/jπ(L+1)(L+1)))2. | (24) |
In addition, we can also calculate the burst-size noise, which is given by
η2burst=1BS+(2L∑j=1qjr3j)(L∑j=1qjr2j)−2−1, | (25) |
where rj=λb(1+a−2√acos(jπ/jπ(L+1)(L+1))). The corresponding noise will contribute to the mRNA noise.
In contrast to previous studies that focused on the effect of multi-OFF mechanisms on gene expression, here we numerically demonstrate influences of multi-ON mechanisms on transcriptional bursting kinetics.
Bursting kinetics is commonly characterized by burst size (BS) and burst frequency (BF). Here, we analyze how the multi-ON mechanism affects burst size and its distribution. Previous studies of the two-state gene model assumed that burst size follows a geometrical distribution [1,12], but whether or not this assumption is reasonable is unclear. In particular, it is not clear whether the burst size still obeys a geometric distribution in the case of multi-ON mechanisms. By performing numerical calculations, we find that multi-ON mechanism can induce bimodal distribution of burst sizes, referring to Figure 2(A)–(C). Figure 2(A), (B) show two representative unimodal and bimodal burst-size distributions, respectively, and Figure 2(C) shows how the number of the most probable mRNA molecules obtained by a statistical method depends on active index L. In Figure 2(A), (B), histograms show stochastic simulations obtained using the Gillespie stochastic simulation algorithm (SSA) [57], and solid lines are theoretical predictions. Figure 2(C) shows that the bimodal mRNA distribution exists for a large range of L. Specifically, the burst-size distribution is unimodal at the origin with L=1 (i.e., multi-OFF model), a geometric distribution as predicted by Eq (20). Then, the burst-size distribution becomes a single peak away from the origin if we increase L, e.g., L=2,3. Finally, the burst-size distribution becomes bimodal with one peak at the origin and another peak away from the origin for larger L with L⩾4. These results imply that burst-size distribution in the case of the multi-ON mechanism is non-geometric but a mixture of geometric distributions as predicted by Eq (23).
Next, we analyze the influences of the multi-ON mechanism on mean burst size and burst-size noise, referring to Figure 2(D)–(F), where three representative promoter loops are shown. In all loops, we observe that mean burst size is always a monotonically increasing function of the active index, implying that more active states can lead to a larger burst size. In addition, we observe that burst-size noise is a monotonically decreasing function of the active index in the loops where the forward ratio is equal to the backward ratio, and the forward ratio is smaller than the backward ratio. However, there is a non-monotonic relationship between the active index and burst-size noise if the forward ratio is larger than the backward ratio. These results indicate that burst-size noise in the case of the multi-ON mechanism can exhibit different characteristics.
Previous studies showed that genes with a multistep mechanism could have peaked burst-size distribution and reduce noise in burst size [44]. Here, we point out that the multi-ON mechanism can induce more complex behaviors of burst size, such as bimodal burst-size distribution and non-monotonic burst-size noise.
Here we focus on analyzing how the multi-ON mechanism influences burst timing between transcription initial events by performing numerical calculations, referring to Figure 3. The first and second rows of Figure 3 show two representative OFF dwell-time distributions (Figure 3(A), (B)) and ON dwell-time distributions (Figure 3(D), (E)), as well as how the times for peak appearance in OFF dwell-time distribution (Figure 3(C)) and ON dwell-time distribution (Figure 3(F)) depend on active index L, respectively. First, we observe that OFF dwell-time distribution changes from unimodality to bimodality, whereas ON dwell-time distribution varies from bimodality to unimodality with the increase of active index L (Comparing Figure 3(A)–(C) with Figure 3(D)–(F)). For example, the OFF dwell-time distribution is bimodal with one peak at the origin and another peak away from the origin for a broad range of L with 1⩽L⩽8, and then this distribution becomes unimodal with a single peak away from the origin if L=9 and further becomes unimodal with a peak at the origin if L=10 (see Figure 3(C)). However, for the ON dwell time, the distribution is unimodal with a peak at the origin if L=1, and is an exponential distribution corresponding to a multi-OFF model. The distribution changes from unimodality with one single peak away from the origin if L=2 to bimodality with one peak at the origin and another peak away from the origin for a broader range of L : 3⩽L⩽10 (see Figure 3(F)).
The bottom row of Figure 3 shows the mean and noise of OFF dwell time (Figure 3(G)) and ON dwell-time (Figure 3(H)), respectively, as well as mean burst frequency for three representative loops (Figure 3(I)). We observe that with the rise of the active index, the mean OFF time decreases whereas mean ON time increases, but the OFF time noise increases and ON time noise decreases. In addition, we find that with increasing the active index, the mean burst frequency can exhibit different characteristics, such as no change with ρf=ρb=1, decrease with ρf=0.5,ρb=2, and increase with ρf=2,ρb=0.5.
The above analysis implies that the multi-ON mechanism can give rise to antagonistic timing of ON and OFF, and induce different characteristics of burst frequency, hinting that the multi-ON mechanism has a considerable influence on transcriptional bursting timing.
Single-molecule experiments have verified that mRNAs are produced often in a bursting manner [1,2]. It is of interest to understand how bursting kinetics affect the variations in mRNA levels. Previous studies focused on the influence of burst parameters such as mean burst size and burst frequency on gene expression levels and noise by analyzing queuing models [32,33,44,45]. However, how the burst size and timing affect gene expression dynamics in a multistate promoter remains elusive (see Figure 1(C)). Here, we explore the influences of burst-size distribution and dwell-time distribution on mRNA distribution, and the numerical results are shown in Figure 4(A)–(H).
From Figure 4(A), (B), we first observe that the burst-size distribution, OFF dwell-time distribution, and ON dwell-time distribution are bimodal, and the mRNA distribution is also bimodal. From Figure 4(C), (D), we find that the burst-size distribution, OFF dwell-time distribution, and ON dwell-time distribution are unimodal, but the mRNA distribution is bimodal. These results indicate that both unimodal and bimodal distributions of burst size and dwell time can lead to bimodal mRNA distribution. On the other hand, we find that the bimodal or unimodal distributions of burst size, OFF dwell time, and ON dwell time can lead to unimodal mRNA distribution, referring to Figure 4(E)–(H). A previous study showed that unimodal or bimodal gene expression has important biological implications, i.e., a cause of phenotypic diversity in a population of genetically identical cells [58]. These results indicate that the variation in the distributions of burst size and dwell-time can give rise to variability in the distribution of gene expression production and further phenotypic variation.
Next, we analyze the influence of burst-size noise, OFF dwell-time noise, and ON dwell-time noise on mRNA noise, with numerical results shown in Figure 5. We observe that the changes in burst-size noise, OFF dwell-time noise, and ON dwell-time noise have different characteristics such as increasing, decreasing, and non-monotonic with the increase of active index L, referring to Figure 5(A), (C), and (E). However, the mRNA noise is always a monotonically decreasing function of active index L (see the last column of Figure 5). These results indicate that the integration of the burst-size noise and dwell-time noise may reduce gene expression noise. Our previous study also showed this by analyzing multi-OFF models [46]. Together, the results imply that a multistep process is a universal mechanism of reducing gene expression noise.
The above analysis shows that the multi-ON mechanism can induce complex transcription kinetics, e.g., unimodal or bimodal distribution of mRNA counts, different burst sizes and timing, and different characteristics of noise. The obtained results imply that the propagation of bursting kinetics to gene expression is complex, and would be important for understanding cellular processes and variation in cell populations.
Transcription is an inherently stochastic process and often occurs in a bursting fashion. Because of involving recruitment of TFs and DNA polymerases, chromatin remodeling, and sequence of transitions between activity states of the promoter, the kinetics of transcriptional bursting is poorly understood. Previous studies showed that mRNA burst-size distribution is geometric with exponential waiting time for two-state promoter models [38,40], but how transcription burst size and timing in multistate promoter models remains unclear.
In the present work, we have analyzed a gene model that corporate the complexity of promoter structure, focusing on the effects of multi-ON mechanisms on transcriptional bursting kinetics. By establishing the survival probability master equations for mRNA bursting production and promoter dwelling at ON/OFF states, we have successfully derived analytical expressions for burst-size distribution and ON/OFF waiting time distributions, and have found that the burst size is a mixed geometric distribution and the ON/OFF dwell time is a mixed exponential distribution in the case of the mixture mechanism. These obtained distributions can be used to analyze transcriptional burst size and timing for arbitrarily complex promoter structure shown in Figure 1.
While previous studies on multi-OFF models [47] and multi-ON models [48] focused on analysis of mRNA distribution and transcriptional noise, in the present work we have explored the effects of the multi-ON mechanism on transcriptional bursting kinetics. We have shown that this mechanism can lead to bimodal burst-size distribution and different patterns of burst-size noise, which is different from previous results that the multistep mechanism can give rise to a peaked burst-size distribution and a reduction in the noise of burst size [44]. In addition, we found that the multi-ON mechanism can lead to antagonistic timing of ON and OFF. For example, the OFF waiting time distribution changes from bimodality to unimodality, whereas the ON waiting time distribution changes from unimodality to bimodality, as the active index increases. Finally, by exploring the distributions and noise for burst size, OFF/ON dwell time, and mRNA copy-number, we found that the propagation of bursting kinetics to mRNA variability is complex. In contrast to previous studies on two-state gene models, which showed that the lower expression noise could result from higher burst frequency, or smaller burst size [35,40,59], here we showed that the qualitative conclusions no longer hold in multistate promoter models. More specifically, we find that mRNA noise always reduces, but the burst-size noise, OFF/ON dwell-time noise, and burst frequency can exhibit complex behaviors in the case of the multi-ON mechanism.
Recently, many studies aimed at the inference of kinetic parameters from experimental data on single cells [50,52]. In a previous study, we showed that different ON/OFF waiting time distributions can exhibit identical steady-state mRNA distributions [48], implying that when one infers a gene system based on transcription dynamics obtained by measurements of fluctuations of mRNA levels, caution must be kept. However, our results obtained here would be used to infer the promoter structure and investigate the variability of the transcription output based on the experimental data. It should be pointed out that the intermediate processes of gene expression such as the partitioning of mRNA at cell division [60], the alternative splicing [61], and the feedback loop [62], would additionally influence the obtained results, but they are neglected in our study. How details of these factors affect transcriptional bursting kinetics is worth further investigation.
This work was supported by the National Nature Science Foundation of P. R. China (Nos. 11601094, 12171494, 11631005, 11775314, 11931019), Guangdong Key Research and Development Project (2019B0233002), and Guangdong Province Key Laboratory of Computational Science at the Sun Yat-sen University (2020B1212060032).
The authors have declared that no competing interest exists.
According to the definition of generating function and Eq (3) in the main text, we can show the expression for the coefficient matrix
H(z)=(h1(z)λ2,1λ1,2h2(z)λ3,2λ2,3h3(z)λ4,3⋱⋱⋱λL−2,L−1hL−1(z)λL,L−1λL−1,LhL(z)), | (A1) |
where hl(z)=μ(z−1)−δl1λl,N−(1−δl1)λl,l−1−λl,l+1, l=1,2,⋅⋅⋅,L.
According to Table 1 in the main text, we can establish the following master equation for promoter kinetics
dP(t)dt=AP(t), | (B1) |
where P(t)=(P1(t),P2(t),⋯,PN(t))T with Pk(t) being the time-dependent probability of promoter at state k, and A is the transcription matrix of promoter state. Our interest is in deriving the steadystate probability P(0)=(P(0)1,P(0)2,⋯,P(0)N) satisfying the condition: AP(0)=0.
First, note that the transition matrix A is an M-matrix (i.e., the sum of every column elements is equal to zero). So we can assume the eigenvalues of the matrix A as 0,−α1,−α2⋅⋅⋅,−αN−1 with αs≠0, and the eigenvalues of the matrix Ak (i.e., crossing out the kth row and kth column entry of matrix A) as −β(k)1,−β(k)2,⋯,−β(k)N−1 with β(k)s≠0. This assumption implies that the characteristic polynomial of A and Ak takes the following form, respectively
gA(x)=det(xI−A)=xN−1∏s=1(x+αs),gAk(x)=det(xI−Ak)=N−1∏s=1(x+β(k)s). | (B2) |
Note that Laplace's formula for the M-matrix A gives
A[det(A1),det(A2),det(A3),⋅⋅⋅,det(AN)]T=0. | (B3) |
Since the null space of A is one-dimensional, we have P(0)=ρ[det(A1),det(A2),⋅⋅⋅,det(AN)]T. From the conservative condition uNP(0)=1 with uN=(1,1,⋅⋅⋅,1) being a row vector, we can obtain
ρ=[N∑k=1det(Ak)]−1=(−1)3[N∑k=1N−1∏s=1β(k)s]−1. | (B4) |
From Eq (B2), we have dgA(x)dx|x=0=N−1∏s=1αs. On the other hand, Jacobi's formula gives
dgA(x)dx|x=0=tr((−A)∗)=(−1)N−1N∑k=1det(Ak)=N∑k=1N−1∏s=1β(k)s, | (B5) |
where "tr" represents the trace of a matrix. The combination of both yields the equality N∑k=1N−1∏s=1β(k)s=N−1∏s=1αs. Finally, we obtain the explicit expression of steady-state probability for promoter at every state
P(0)k=ρdet(Ak)=(−1)N−1(N∑k=1N−1∏s=1β(k)s)−1(−1)N−1N−1∏s=1β(k)s=N−1∏s=1β(k)sαs, 1⩽k⩽N | (B6) |
First, we compute the binomial moments (BMs). Let W(i)kl represent a determinant of a (N−1)×(N−1) matrix, which is from matrix (iδI−A) by crossing out its kth row and lth column elements. Denoting by W(i)k=(W(i)k1,⋯,W(i)kN)T a vector, then the adjacency matrix of (iδI−A) can be written as
[(iδ)I−A]∗=[W(i)1,⋯,W(i)L,W(i)L+1⋯,W(i)N], | (C1) |
Therefore, it is easy to get the following expression
[(iδ)I−A]∗Ω=μ(W(i)1,⋯,W(i)L,0,0,⋯0)T, | (C2) |
In addition, the determinant is
det(iδI−A) = (iδ)NN−1∏s=1(1+α(i)s), | (C3) |
where −α(i)1,⋅⋅⋅,−α(i)N−1 are the nonzero eigenvalues of M-matrix ˜A(i)=A/iδ. Substituting Eqs (C2) and (C3) into expression ai=(iδI−A)∗Ωdet(iδI−A)ai−1, which can be derived from Eq (9) in the main text, we can obtain the expressions of the factorial BMs
ai=(a(1)i⋮a(N)i) = μ(L∑k=1W(i)k1a(k)i−1,⋯,L∑k=1W(i)kNa(k)i−1)T(iδ)NN−1∏s=1(1+α(i)s). | (C4) |
Finally, we can get the expression of the total BMs
bi=N∑s=1a(s)i=μiδ(L∑s=1a(s)i−1). | (C5) |
Equations (C4) and (C5) imply that the vectors of BMs ai and total BMs bi can be analytically given based on a0, i.e., on the steady-state probabilities of promoter states.
For the mixture loop model, matrix H(z) becomes
H(z)=(h(z)λbλfh(z)λbλfh(z)λb⋱⋱⋱λfh(z)λbλfh(z)), | (D1) |
where h(z)=μ(z−1)−λf−λb. Note that matrix H(z) has eigenvalues −λh1,−λh2,⋯,−λhL with
λhj=−[h(z)+2√λbλfcosjπL+1],j=1,2,⋯L. | (D2) |
In addition, the eigenvectors associated with the eigenvalues −λhj are given by Vhj=(Vh1j,⋯,VhLj)T with Vhlj=√2L+1(λfλb)(l−1)/(l−1)22sinljπL+1. If denoting by Vh=(Vh1,⋯,VhL), then we have (Vh)−1H(z)Vh=Λh=diag(−λh1,⋯,−λhL)T. Making the transform ˜Vh = RONVh, and we obtain the orthogonal matrix ˜Vh=(˜Vhlj) with ˜Vhlj=√2L+1sinljπL+1. Substituting this expression and Eq (D2) into Eq (13) in the main text, we obtain the explicit expression of generating function in the case of the mixture mechanism.
[1] |
I. Golding, J. Paulsson, S. M. Zawilski, E. C. Cox, Real-time kinetics of gene activity in individual bacteria, Cell, 123 (2005), 1025–1036. https://doi.org/10.1016/j.cell.2005.09.031 doi: 10.1016/j.cell.2005.09.031
![]() |
[2] |
A. Raj, C. S. Peskin, D. Tranchina, D. Y. Vargas, S. Tyagi, Stochastic mRNA synthesis in mammalian cells, PLoS Biol., 4 (2006), e309. https://doi.org/10.1371/journal.pbio.0040309 doi: 10.1371/journal.pbio.0040309
![]() |
[3] |
J. R. Chubb, T. Trcek, S. M. Shenoy, R. H. Singer, Transcriptional pulsing of a developmental gene, Curr. Biol., 16 (2006), 1018–1025. https://doi.org/10.1016/j.cub.2006.03.092 doi: 10.1016/j.cub.2006.03.092
![]() |
[4] |
L. So, A. Ghosh, C. Zong, L. A. Sepúlveda, R. Segev, I. Golding, General properties of transcriptional time series in Escherichia coli., Nat. Genet., 43 (2011), 554–560. https://doi.org/10.1038/ng.821 doi: 10.1038/ng.821
![]() |
[5] |
M. B. Elowitz, A. J. Levine, E. D. Siggia, P. S. Swain, Stochastic gene expression in a single cell, Science, 297 (2002), 1183–1186. https://doi.org/10.1126/science.1070919 doi: 10.1126/science.1070919
![]() |
[6] |
J. M. Raser, E. K. O'Shea, Control of stochasticity in eukaryotic gene expression, Science, 304 (2004), 1811–1184. https://doi.org/10.1126/science.1098641 doi: 10.1126/science.1098641
![]() |
[7] |
A. Becskei, B. B. Kaufmann, A. van Oudenaarden, Contributions of low molecule number and chromosomal positioning to stochastic gene expression, Nat. Genet., 37 (2005), 937–944. https://doi.org/10.1038/ng1616 doi: 10.1038/ng1616
![]() |
[8] |
G. Balázsi, A. van Oudenaarden, J. J. Collins, Cellular decision making and biological noise: from microbes to mammals, Cell, 144 (2011), 910–925. https://doi.org/10.1016/j.cell.2011.01.030 doi: 10.1016/j.cell.2011.01.030
![]() |
[9] |
A. N. Boettiger, M. Levine, Synchronous and stochastic patterns of gene activation in the Drosophila embryo, Science, 325 (2009), 471–473. https://doi.org/10.1126/science.1173976 doi: 10.1126/science.1173976
![]() |
[10] |
A. Raj, S. A. Rifkin, E. Andersen, A. van Oudenaarden, Variability in gene expression underlies incomplete penetrance, Nature, 463 (2010), 913–918. https://doi.org/10.1038/nature08781 doi: 10.1038/nature08781
![]() |
[11] |
A. Eldar, M. B. Elowitz, Functional roles for noise in genetic circuits, Nature, 467 (2010), 167–173. https://doi.org/10.1038/nature09326 doi: 10.1038/nature09326
![]() |
[12] |
P. J. Choi, L. Cai, K. Frieda, X. S. Xie, A stochastic single-molecule event triggers phenotype switching of a bacterial cell, Science, 322 (2008), 442–446. https://doi.org/10.1126/science.1161427 doi: 10.1126/science.1161427
![]() |
[13] |
M. Ptashne, A. Gann, Transcriptional activation by recruitment, Nature, 386 (1997), 569–577. https://doi.org/10.1038/386569a0 doi: 10.1038/386569a0
![]() |
[14] |
G. L. Hager, C. Elbi, T. A. Johnson, T. Voss, A. K. Nagaich, R. L. Schiltz, et al., Chromatin dynamics and the evolution of alternate promoter states, Chromosome Res., 14 (2006), 107–116. https://doi.org/10.1007/s10577-006-1030-0 doi: 10.1007/s10577-006-1030-0
![]() |
[15] |
C. R. Clapier, B. R. Cairns, The biology of chromatin remodeling complexes, Annu. Rev. Biochem., 78 (2009), 273–304. https://doi.org/10.1146/annurev.biochem.77.062706.153223 doi: 10.1146/annurev.biochem.77.062706.153223
![]() |
[16] |
T. J. Stasevich, J. G. McNally, Assembly of the transcription machinery: ordered and stable, random and dynamic, or both?, Chromosoma, 120 (2011), 533–545. https://doi.org/10.1007/s00412-011-0340-y doi: 10.1007/s00412-011-0340-y
![]() |
[17] |
D. R. Larson, What do expression dynamics tell us about the mechanism of transcription?, Curr. Opin. Genet. Dev., 21 (2011), 591–599. https://doi.org/10.1016/j.gde.2011.07.010 doi: 10.1016/j.gde.2011.07.010
![]() |
[18] |
D. M. Suter, N. Molina, D. Gatfield, K. Schneider, U. Schibler, F. Naef, Mammalian genes are transcribed with widely different bursting kinetics, Science, 332 (2011), 472–474. https://doi.org/10.1126/science.1198817 doi: 10.1126/science.1198817
![]() |
[19] |
C. V. Harper, B. Finkenstädt, D. J. Woodcock, S. Friedrichsen, S. Semprini, L. Ashall, et al., Dynamic analysis of stochastic transcription cycles, PLoS Biol., 9 (2011), e1000607. https://doi.org/10.1371/journal.pbio.1000607 doi: 10.1371/journal.pbio.1000607
![]() |
[20] |
M. Kandhavelu, A. Hӓkkinen, O. Yli-Harja, A. S. Ribeiro, Single-molecule dynamics of transcription of the lar promoter, Phys. Biol., 9 (2012), 026004. https://doi.org/10.1088/1478-3975/9/2/026004 doi: 10.1088/1478-3975/9/2/026004
![]() |
[21] |
W. J. Blake, G. Balázsi, M. A. Kohanski, F. J. Isaacs, K. F. Murphy, Y. Kuang, et al., Phenotypic consequences of promoter-mediated transcriptional noise, Mol. Cell., 24 (2006), 853–865. https://doi.org/10.1016/j.molcel.2006.11.003 doi: 10.1016/j.molcel.2006.11.003
![]() |
[22] |
S. L. Berger, The complex language of chromatin regulation during transcription, Nature, 447 (2007), 407–412. https://doi.org/10.1038/nature05915 doi: 10.1038/nature05915
![]() |
[23] |
N. J. Fuda, M. B. Ardehali, J. T. Lis, Defining mechanisms that regulate RNA polymerase II transcription in vivo, Nature, 461 (2009), 186–192. https://doi.org/10.1038/nature08449 doi: 10.1038/nature08449
![]() |
[24] |
G. Hornung, R. Bar-Ziv, D. Rosin, N. Tokuriki, D. S. Tawfik, M. Oren, et al., Noise-mean relationship in mutated promoters, Genome Res., 22 (2012), 2409–2417. https://doi.org/10.1101/gr.139378.112 doi: 10.1101/gr.139378.112
![]() |
[25] |
A. Halme, S. Bumgarner, C. Styles, G. R. Fink, Genetic and epigenetic regulation of the FLO gene family generates cell-surface variation in yeast, Cell, 116 (2004), 405–415. https://doi.org/10.1016/S0092-8674(04)00118-7 doi: 10.1016/S0092-8674(04)00118-7
![]() |
[26] |
L. M. Octavio, K. Gedeon, N. Maheshri, Epigenetic and conventional regulation is distributed among activators of FLO11 allowing tuning of population-level heterogeneity in its expression, PLoS. Genet., 5 (2009), e1000673. https://doi.org/10.1371/journal.pgen.1000673 doi: 10.1371/journal.pgen.1000673
![]() |
[27] |
G. Reid, R. Gallais, R. Métivier, Marking time: the dynamic role of chromatin and covalent modification in transcription, Int. J. Biochem. Cell Biol., 41 (2009), 155–163. https://doi.org/10.1016/j.biocel.2008.08.028 doi: 10.1016/j.biocel.2008.08.028
![]() |
[28] |
L. S. S. Dunham, H. Momiji, C. V. Harper, P. J. Downton, K. Hey, A. McNamara, et al., Asymmetry between activation and deactivation during a transcriptional pulse, Cell Syst., 5 (2017), 646–653. https://doi.org/10.1016/j.cels.2017.10.013 doi: 10.1016/j.cels.2017.10.013
![]() |
[29] |
L. A. Sepúlveda, H. Xu, J. Zhang, M. Wang, I. Golding, Measurement of gene regulation in individual cells reveals rapid switching between promoter states, Science, 351 (2016), 1218–1222. https://doi.org/10.1126/science.aad0635 doi: 10.1126/science.aad0635
![]() |
[30] |
G. Neuert, B. Munsky, R. Z. Tan, L. Teytelman, M. Khammash, A. van Oudenaarden, Systematic identification of signal-activated stochastic gene regulation, Science, 339 (2013), 584–587. https://doi.org/10.1126/science.1231456 doi: 10.1126/science.1231456
![]() |
[31] |
J. M. Pedraza, J. Paulsson, Effects of molecular memory and bursting on fluctuations in gene expression, Science, 319 (2008), 339–343. https://doi.org/10.1126/science.1144331 doi: 10.1126/science.1144331
![]() |
[32] |
T. Jia, R. V. Kulkarni, Intrinsic noise in stochastic models of gene expression with molecular memory and bursting, Phys. Rev. Lett., 106 (2011), 058102. https://doi.org/10.1103/PhysRevLett.106.058102 doi: 10.1103/PhysRevLett.106.058102
![]() |
[33] |
J. Zhang, T. Zhou, Markovian approaches to modeling intracellular reaction processes with molecular memory, Proc. Natl. Acad. Sci. USA, 116 (2019), 23542–23550. https://doi.org/10.1073/pnas.1913926116 doi: 10.1073/pnas.1913926116
![]() |
[34] |
X. Yang, Y. Chen, T. Zhou, J. Zhang, Exploring dissipative sources of non-Markovian biochemical reaction systems, Phys. Rev. E, 103 (2021), 052411. https://doi.org/10.1103/PhysRevE.103.052411 doi: 10.1103/PhysRevE.103.052411
![]() |
[35] |
L. B. Carey, D. van Dijk, P. M. A. Sloot, J. A. Kaandorp, E. Segal, Promoter sequence determines the relationship between expression level and noise, PLoS Biol., 11 (2013), e1001528. https://doi.org/10.1371/journal.pbio.1001528 doi: 10.1371/journal.pbio.1001528
![]() |
[36] |
Y. Wang, T. Ni, W. Wang, F. Liu, Gene transcription in bursting: a unified mode for realizing accuracy and stochasticity, Biol. Rev., 94 (2019), 248–258. https://doi.org/10.1111/brv.12452 doi: 10.1111/brv.12452
![]() |
[37] |
J. Peccoud, B. Ycart, Markovian modeling of gene-product synthesis, Theor. Pop. Biol., 48 (1995), 222–234. https://doi.org/10.1006/tpbi.1995.1027 doi: 10.1006/tpbi.1995.1027
![]() |
[38] |
V. Shahrezaei, P. S. Swain, Analytical distributions for stochastic gene expression, Proc. Nat. Acad. Sci. USA, 105 (2008), 17256–17261. https://doi.org/10.1073/pnas.0803850105 doi: 10.1073/pnas.0803850105
![]() |
[39] |
M. Dobrzyński, F. J. Bruggeman, Elongation dynamics shape bursty transcription and translation, Proc. Natl. Acad. Sci. USA, 106 (2009), 2583–2588. https://doi.org/10.1073/pnas.0803507106 doi: 10.1073/pnas.0803507106
![]() |
[40] |
R. D. Dar, B. S. Razooky, A. Singh, T. V. Trimeloni, J. M. McCollum, et al., Transcriptional burst frequency and burst size are equally modulated across the human genome, Proc. Nat. Acad. Sci. USA, 109 (2012), 17454–17459. https://doi.org/10.1073/pnas.1213530109 doi: 10.1073/pnas.1213530109
![]() |
[41] |
J. Zhang, T. Zhou, Stationary moments, distribution conjugation and phenotypic regions in stochastic gene transcription, Math. Biosci. Eng. 16 (2019), 6134–6166. https://doi.org/10.3934/mbe.2019307 doi: 10.3934/mbe.2019307
![]() |
[42] |
C. Shi, Y. Jia, T. Zhou, Queuing models of gene expression: analytical distributions and beyond, Biophys. J., 119 (2020), 1606–1616. https://doi.org/10.1016/j.bpj.2020.09.001 doi: 10.1016/j.bpj.2020.09.001
![]() |
[43] |
Z. Zhang, Q. Deng, Z. Wang, Y. Chen, T. Zhou, Exact results for queuing models of stochastic transcription with memory and crosstalk, Phys. Rev. E, 103 (2021), 062414. https://doi.org/10.1103/PhysRevE.103.062414 doi: 10.1103/PhysRevE.103.062414
![]() |
[44] |
A. Schwabe, K. N. Rybakova, F. J. Bruggeman, Transcription stochasticity of complex gene regulation models, Biophys. J., 103 (2012), 1152–1161. https://doi.org/10.1016/j.bpj.2012.07.011 doi: 10.1016/j.bpj.2012.07.011
![]() |
[45] |
N. Kumar, A. Singh, R. V. Kulkarni, Transcriptional bursting in gene expression: analytical results for general stochastic models, PLoS Comput. Biol., 11 (2015), e1004292. https://doi.org/10.1371/journal.pcbi.1004292 doi: 10.1371/journal.pcbi.1004292
![]() |
[46] |
J. Zhang, L. Chen, T. Zhou, Analytical distribution and tunability of noise in a model of promoter progress, Biophys. J., 102 (2012), 1247–1257. https://doi.org/10.1016/j.bpj.2012.02.001 doi: 10.1016/j.bpj.2012.02.001
![]() |
[47] |
T. Zhou, J. Zhang, Analytical results for a multistate gene model, SIAM J. Appl. Math. 72 (2012), 789-818. https://doi.org/10.1137/110852887 doi: 10.1137/110852887
![]() |
[48] |
J. Zhang, T. Zhou, Promoter-mediated transcription dynamics, Biophys. J., 106 (2014), 479–488. https://doi.org/10.1016/j.bpj.2013.12.011 doi: 10.1016/j.bpj.2013.12.011
![]() |
[49] |
S. Choubey, J. Kondev, A. Sanchez, Distribution of initiation times reveals mechanisms of transcriptional regulation in single cells, Biophys. J., 114 (2018), 2072–2082. https://doi.org/10.1016/j.bpj.2018.03.031 doi: 10.1016/j.bpj.2018.03.031
![]() |
[50] |
B. Zoller, D. Nicolas, N. Molina, F. Naef, Structure of silent transcription intervals and noise characteristics of mammalian genes, Mol. Syst. Biol., 11 (2015), 823. https://doi.org/10.15252/msb.20156257 doi: 10.15252/msb.20156257
![]() |
[51] |
K. N. Rybakova, F. J. Bruggeman, A. Tomaszewska, M. J. Moné, C. Carlberg, H. V. Westerhoff, Multiplex eukaryotic transcription (in) activation: timing, bursting and cycling of a ratchet clock mechanism, PLoS Comput. Biol., 11(2015), e1004236. https://doi.org/10.1371/journal.pcbi.1004236 doi: 10.1371/journal.pcbi.1004236
![]() |
[52] |
[52] B. J. Daigle, J. M. Soltani, L. R. Petzold, A. Singh, Inferring single-cell gene expression mechanisms using stochastic simulation, Bioinformatics, 31 (2015), 1428–1435. https://doi.org/10.1093/bioinformatics/btv007 doi: 10.1093/bioinformatics/btv007
![]() |
[53] |
T. L. To, N. Maheshri, Noise can induce bimodality in positive transcriptional feedback loops without bistability, Science, 327(2010), 1142. https://doi.org/10.1126/science.1178962 doi: 10.1126/science.1178962
![]() |
[54] |
P. Bokes, A. Borri, P. Palumbo, A. Singh, Mixture distributions in a stochastic gene expression model with delayed feedback: a WKB approximation approach, J. Math. Biol., 81 (2020) 343–367. https://doi.org/10.1007/s00285-020-01512-y doi: 10.1007/s00285-020-01512-y
![]() |
[55] |
P. Bokes, Postponing production exponentially enhances the molecular memory of a stochastic switch, Eur. J. Appl. Math., 2020 (2020), 1–18. https://doi.org/10.1017/S0956792520000479 doi: 10.1017/S0956792520000479
![]() |
[56] |
J. Zhang, Q. Nie, T. Zhou, A moment-convergence method for stochastic analysis of biochemical reaction networks, J. Chem. Phys., 144 (2016), 194109. https://doi.org/10.1063/1.4950767 doi: 10.1063/1.4950767
![]() |
[57] |
D. Gillespie, Exact stochastic simulation of coupled chemical reactions, J. Phys. Chem., 81 (1977), 2340–2361. https://doi.org/10.1021/j100540a008 doi: 10.1021/j100540a008
![]() |
[58] |
M. Kærn, T. C. Elston, W. J. Blake, J. J. Collins, Stochasticity in gene expression: from theories to phenotypes, Nat. Rev. Genet., 6 (2005), 451–464. https://doi.org/10.1038/nrg1615 doi: 10.1038/nrg1615
![]() |
[59] |
A. S. Hansen, E. K. O'Shea, Promoter decoding of transcription factor dynamics involves a trade-off between noise and control of gene expression, Mol. Syst. Biol., 9 (2013), 704. https://doi.org/10.1038/msb.2013.56 doi: 10.1038/msb.2013.56
![]() |
[60] |
Z. Cao, R. Grima, Analytical distributions for detailed models of stochastic gene expression in eukaryotic cells, Proc. Natl. Acad. Sci. USA, 117 (2020), 4682–4692. https://doi.org/10.1073/pnas.1910888117 doi: 10.1073/pnas.1910888117
![]() |
[61] |
Q. Wang, T. Zhou, Alternative-splicing-mediated gene expression, Phys. Rev. E, 89 (2014), 012713. https://doi.org/10.1103/PhysRevE.89.012713 doi: 10.1103/PhysRevE.89.012713
![]() |
[62] |
L. Fang, Z. Yuan, P. Liu, T. Zhou, Feedback-induced counterintuitive correlations of gene expression noise with bursting kinetics, Phys. Rev. E, 90 (2014), 052702. https://doi.org/10.1103/PhysRevE.90.052702 doi: 10.1103/PhysRevE.90.052702
![]() |
1. | Xiyan Yang, Songhao Luo, Zhenquan Zhang, Zihao Wang, Tianshou Zhou, Jiajun Zhang, Silent transcription intervals and translational bursting lead to diverse phenotypic switching, 2022, 24, 1463-9076, 26600, 10.1039/D2CP03703C | |
2. | Changhong Shi, Xiyan Yang, Tianshou Zhou, Jiajun Zhang, Nascent RNA kinetics with complex promoter architecture: Analytic results and parameter inference, 2024, 110, 2470-0045, 10.1103/PhysRevE.110.034413 | |
3. | Nissrin Alachkar, Dale Norton, Zsofia Wolkensdorfer, Mark Muldoon, Pawel Paszek, Variability of the innate immune response is globally constrained by transcriptional bursting, 2023, 10, 2296-889X, 10.3389/fmolb.2023.1176107 | |
4. | Xiaojun Bai, Sizhe Wang, Xin Zhang, Haohua Wang, Molecular-Memory-Induced Counter-Intuitive Noise Attenuator in Protein Polymerization, 2024, 16, 2073-8994, 315, 10.3390/sym16030315 | |
5. | Xinxin Chen, Ying Sheng, Liang Chen, Moxun Tang, Feng Jiao, Quantifying cell fate change under different stochastic gene activation frameworks, 2025, 13, 2095-4689, 10.1002/qub2.82 |
Reactions | Description |
Aiλi,i+1⟷λi+1,iAi+1i=1,2,⋅⋅⋅,L−1 | Transitions between active states |
IjλL+j,L+j+1⟷λL+j+1,L+jIj+1,j=1,2,⋅⋅⋅,K−1 | Transitions between inactive states |
A1λ1,N⟷λN,1IK,ALλL,L+1⟷λL+1,LI1 | Transitions between active and inactive states |
Aiμ→Ai+mRNA, i=1,2,⋅⋅⋅,L | Transcription |
mRNAδ→∅ | mRNA degradation |
Reactions | Description |
Aiλi,i+1⟷λi+1,iAi+1i=1,2,⋅⋅⋅,L−1 | Transitions between active states |
IjλL+j,L+j+1⟷λL+j+1,L+jIj+1,j=1,2,⋅⋅⋅,K−1 | Transitions between inactive states |
A1λ1,N⟷λN,1IK,ALλL,L+1⟷λL+1,LI1 | Transitions between active and inactive states |
Aiμ→Ai+mRNA, i=1,2,⋅⋅⋅,L | Transcription |
mRNAδ→∅ | mRNA degradation |