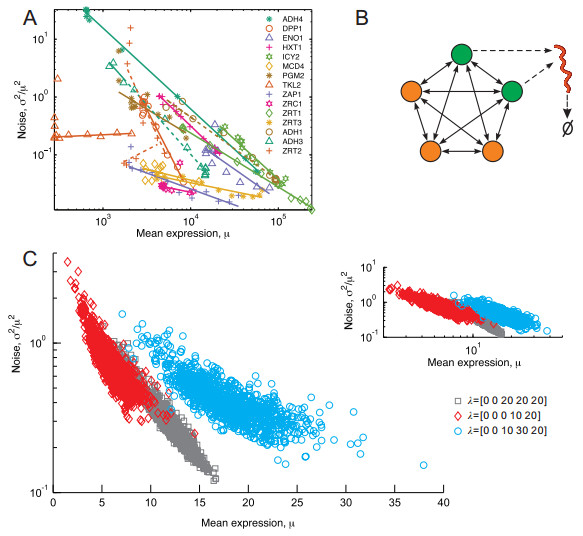
Gene transcription in single cells is inherently a probabilistic process. The relationship between variance (σ2) and mean expression (μ) is of paramount importance for investigations into the evolutionary origins and consequences of noise in gene expression. It is often formulated as log(σ2/μ2)=βlogμ+logα, where β is a key parameter since its sign determines the qualitative dependence of noise on mean. We reveal that the sign of β is controlled completely by external regulation, but independent of promoter structure. Specifically, it is negative if regulators as stochastic variables are independent but positive if they are correlated. The essential mechanism revealed here can well interpret diverse experimental phenomena underlying expression noise. Our results imply that external regulation rather than promoter sequence governs the mean-noise relationship.
Citation: Meiling Chen, Tianshou Zhou, Jiajun Zhang. Correlation between external regulators governs the mean-noise relationship in stochastic gene expression[J]. Mathematical Biosciences and Engineering, 2021, 18(4): 4713-4730. doi: 10.3934/mbe.2021239
[1] | Guilherme Giovanini, Alan U. Sabino, Luciana R. C. Barros, Alexandre F. Ramos . A comparative analysis of noise properties of stochastic binary models for a self-repressing and for an externally regulating gene. Mathematical Biosciences and Engineering, 2020, 17(5): 5477-5503. doi: 10.3934/mbe.2020295 |
[2] | Zhenquan Zhang, Junhao Liang, Zihao Wang, Jiajun Zhang, Tianshou Zhou . Modeling stochastic gene expression: From Markov to non-Markov models. Mathematical Biosciences and Engineering, 2020, 17(5): 5304-5325. doi: 10.3934/mbe.2020287 |
[3] | Qiuying Li, Lifang Huang, Jianshe Yu . Modulation of first-passage time for bursty gene expression via random signals. Mathematical Biosciences and Engineering, 2017, 14(5&6): 1261-1277. doi: 10.3934/mbe.2017065 |
[4] | Heli Tan, Tuoqi Liu, Tianshou Zhou . Exploring the role of eRNA in regulating gene expression. Mathematical Biosciences and Engineering, 2022, 19(2): 2095-2119. doi: 10.3934/mbe.2022098 |
[5] | Jiajun Zhang, Tianshou Zhou . Stationary moments, distribution conjugation and phenotypic regions in stochastic gene transcription. Mathematical Biosciences and Engineering, 2019, 16(5): 6134-6166. doi: 10.3934/mbe.2019307 |
[6] | Qi Wang, Lifang Huang, Kunwen Wen, Jianshe Yu . The mean and noise of stochastic gene transcription with cell division. Mathematical Biosciences and Engineering, 2018, 15(5): 1255-1270. doi: 10.3934/mbe.2018058 |
[7] | Pierre Degond, Maxime Herda, Sepideh Mirrahimi . A Fokker-Planck approach to the study of robustness in gene expression. Mathematical Biosciences and Engineering, 2020, 17(6): 6459-6486. doi: 10.3934/mbe.2020338 |
[8] | Xuan Zhang, Huiqin Jin, Zhuoqin Yang, Jinzhi Lei . Effects of elongation delay in transcription dynamics. Mathematical Biosciences and Engineering, 2014, 11(6): 1431-1448. doi: 10.3934/mbe.2014.11.1431 |
[9] | Gerardo Aquino, Andrea Rocco . Bimodality in gene expression without feedback: from Gaussian white noise to log-normal coloured noise. Mathematical Biosciences and Engineering, 2020, 17(6): 6993-7017. doi: 10.3934/mbe.2020361 |
[10] | Qiqi Deng, Aimin Chen, Huahai Qiu, Tianshou Zhou . Analysis of a non-Markov transcription model with nuclear RNA export and RNA nuclear retention. Mathematical Biosciences and Engineering, 2022, 19(8): 8426-8451. doi: 10.3934/mbe.2022392 |
Gene transcription in single cells is inherently a probabilistic process. The relationship between variance (σ2) and mean expression (μ) is of paramount importance for investigations into the evolutionary origins and consequences of noise in gene expression. It is often formulated as log(σ2/μ2)=βlogμ+logα, where β is a key parameter since its sign determines the qualitative dependence of noise on mean. We reveal that the sign of β is controlled completely by external regulation, but independent of promoter structure. Specifically, it is negative if regulators as stochastic variables are independent but positive if they are correlated. The essential mechanism revealed here can well interpret diverse experimental phenomena underlying expression noise. Our results imply that external regulation rather than promoter sequence governs the mean-noise relationship.
Gene expression is a complex stochastic process where transcription as a key step occurs often in a bursty manner, creating cell-to-cell variation in mRNA and further protein abundance [1,2,3], In unicellular organisms, this variation improves fitness by generating phenotypic differences within a population of genetically identical cells, thus enabling a rapid response to fluctuating environments [4,5]. In multi-cellular organisms, the variation plays an important role in development since it allows identical progenitor cells to acquire very different fates [6,7]. Owing to this functional importance of variation, an important task in the post-genome era is to identify and dissect the molecular mechanisms that generate and control variation.
Recent studies [8,9,10,11,12,13,14,15,16,17,18] have focused on the relationship between the cell-to-cell variance (σ2) of mRNAs or proteins and its mean expression level (μ). This relationship is of particular importance for probing the evolutionary origins and consequences of noise in gene expression since it allows biologists to correctly normalize mRNA or protein variations to identify which mRNAs or proteins display unexpectedly high single-cell variances around their mean levels. Experimental data have indicated that the variance is linearly related to the mean when plotted on a log-log scale, suggesting a power-law like relationship (σ2∝μk).The dissection and interpretation of this relationship have been aided by analyzing theoretical or experimental models of gene expression [19,20,21,22,23,24,25,26,27,28,29,30,31,32,33,34,35,36], but conflicting views or evidence have also appeared: (1) The existence of a mean-noise trend line, which is followed by a large number of genes, seems to be in conflict with the idea that gene-specific mechanisms of transcriptional regulation (for which there is an abundance of in vitro biochemical evidence [24]) can lead to a characteristic noise signature, quantified by the dependence of variance on mean; (2) Several experimental studies [8,9] implies that the slope of the log-log line for the relationship between noise (σ2/μ2) and mean (μ) is always negative whereas another experimental study [10] indicates that this slope may be positive or negative (referring to Figure 1A).
Given these conflicting views or evidences, two important questions then arise: what molecular mechanisms underlying expression noise govern the mean-noise relationship? To what degree is this relationship universal? In this article, we address these issues and reveal that the sign of the slope is controlled completely by the correlation coefficient between external regulators, but independent of promoter structure.
Since the size of relative fluctuations (i.e., the noise intensity) defined as the ratio of variance to mean can quantify the noise level more reasonably than variance [25], we mathematically formulate and dissect the mean-noise relationship as
(σ2/μ2)=αμβ+C, | (2.1) |
where α and β are two parameters that represent the level of internal noise, constant C represents the level of extrinsic noise such as cell cycle and DNA replication. It was noted that when the average expression level of molecular was low, the uncertainty of molecular fluctuation caused the internal noise of cell expression to be much higher than the external noise. Therefore, we ignore the extrinsic noise C and rewrite (2.1) as follow
log(σ2/μ2)=βlogμ+logα. | (2.2) |
In principle, these parameters can be easily estimated from experimental data (Figure 1A), as done in the literature [8,9,10,11,12,13,14,15,16], but molecular mechanisms influencing them are unclear. Note that in contrast to α, β is a more important parameter since its sign determines the qualitative dependence of the noise level on the mean whereas its size determines the degree of the dependence of the former on the latter. We use gene expression model to study mean-noise relationship. In fact, the degradation of mRNA is much faster than that of protein [37]. The two stage process (transcription and translation) can be integrated into one single step process with translational bursting [38]. Meanwhile, all the models used in this paper assume proteins are produced one molecule at a time (i.e., without translational bursting), although it is a common mode in eukaryotes and prokaryotes [21,22]. These conditions are sufficient to ensure that protein production can be done in one step [39], which has been applied in some theoretical models [40]. Furthermore, stationary mRNA levels also can well explain protein levels in many conditions [39]. Therefore, it is reasonable to directly regard the expression of mRNA as protein abundance in the gene expression model.
First, we want to explore whether the architecture of promoters affects the mean-noise relationship. We introduce the multi-state promoter gene expression model without regulation [24], which is an extension of the common ON-OFF model [26,27,28,29]. The advantage of this model is that it can have any number of ON and OFF states instead of the original two limited states, and the states can be switched arbitrarily between each other, which is more in line with the actual complex promoter in biology.
We assume that the promoter of a gene contains a total of N states, including L active states and K=N−L inactive states. Figure 1B shows a five-state gene expression model containing 3 inactive states and 2 active states. In order to simplify the model, we further assume that the promoter will translate only in the active state, regardless of the leakage of mRNA in the inactive state and their transition rate among states accords with the Markov process, which is a constant. Then for the degradation rate of protein, we assume it obeys the linear hypothesis. To sum up, the biochemical reaction of the promoter multi-state model can be written as
Gikij→Gj,Gjkji→Gi,Giλi→Gi+X,Xδi→∅, | (2.3) |
where Gi and Gj represent two different states of the promoter, respectively. X represents the protein. Let Pk(m;t) denote the probability distribution of the number of protein m molecules in the kth promoter state at t time, then P=(P1,⋯,PN)T is a column vector composed of N probability distributions. The transition rate between promoters of each state is described by a N×N transition matrix A=(kij), where kij represents the transition rate from state i to state j. We use two diagonal matrices Λ=diag(λ1,⋯,λN) and δ=diag(δ1,⋯,δN) to describe the rate of translation and degradation of protein, where λi is the translation rate of promoter state i (when it is in inactive state, λi=0), δi is the degradation rate of protein produced by promoter state i. Therefore, under this multi-state promoter model, the chemical master equation can be described in the following form:
∂∂tP(m;t)=AP(m;t)+Λ(E−1−I)[P(m;t)]+δ(E−I)[mP(m;t)], | (2.4) |
where E is the step operators and E−1 is its inverse, and I is the identity operator. For this chemical master equation, we can use the convergent moment approach to reconstruct the stationary distribution [42]. Denoted by b1 and b2 the first- and second-order binomial moments, the mean expression level μp and the intensity of the noise η2p in protein can be computed as follow:
μp=b1η2p=1b1+2b2−b21b21. | (2.5) |
Next, we present numerical results. We computationally find that promoter sequence influences α more remarkably than β. In particular, the sign of β is always negative and independent of promoter structure (Figure 1C). This indicates that promoter structure primarily affects the intercept rather than the slope in the log-log line described by (2.2).
The expression of a gene is inevitably regulated by intracellular or/and extracellular factors. These factors would be so complex that any existing theoretical models of gene expression are flawed. Here we particularly assume that for gene regulation, except for their own product proteins to regulate themselves, all other regulations belong to external regulation. So the transcription factor is also one of the external regulators (ERs). In order to reveal the essential molecular mechanism of how ERs regulate the relationship between mean and noise, here we introduce a simple yet representative gene model (Figure 2A), which captures main molecular events occurring in gene expression. This model assumes that the gene has one active (ON) and one inactive (OFF) states with bidirectional transitions between them. This model is a special case of (2.3) mentioned above. Thus, the gene model used is described by
GOFFkON→GON,GONkOFF→GOFF,GONλ→GON+X,Xδ→∅, | (2.6) |
where GON and GOFF represent the active and inactive states of the promoter, respectively. X represents the protein. kOFF and kON are transition rates from inactive and active states and vice versa. λ is the rate of translation when the gene is in the active state and δ is the rate of protein decay. Note that if kOFF and kON are constants, the corresponding gene expression model is just the common ON-OFF model. If any transition rate between ON and OFF is not a constant, this actually corresponds to the translational regulation in biology. Therefore, we consider two ERs regulate the gene expression by adjusting two transition rates between ON and OFF states. This setting is used to model either the effect of regulation from the other genes inside the cell or uncertainty of extracellular environments or both. In this situation, kOFF and kON are functions of concentration of ERs.
For clear demonstration, let us first consider two extreme cases: (1) Two distinct ERs independently regulate two transition rates between promoter activity states (Figure 2A) with the settings kON=f1(ER1)=1/(1+ERn11) and kOFF=f2(ER2)=1/(1+ERn22); (2) A common ER simultaneously regulates the transition rates (Figure 2B) with the settings kON=f1(ER)=1/(1+ERn1) and kOFF=f2(ER)=1/(1+ERn2). In each case, ERs take values randomly in a finite range. In Figure 2, ER1 and ER2 are generated in the interval (0.001,1000). We find that the mean-noise relationship is characterized by a negative slope in case (1) (Figure 2E), and by a negative or positive slope in case (2) (Figure 2G). These numerical results are in accord with experimental observations [8,10]. In both cases, the dependence of the proteins mean levels on the inducers concentration exhibits different characteristics (comparing Figure 2D with Figure 2F).
Then, consider a more general case: two ERs regulate two transition rates between the promoter activity states in a correlated manner with a correlation coefficient r (Figure 2H). In this case, two regulated parameters are set as kON=4+2ERn11 and kOFF=1+ERn22, where ER1 and ER2 are generated randomly and with a correlation coefficient r in the interval (0.001,1000). We can see that small r's can correspond to the model in Figure 2A whereas large r's to the model in Figure 2B. To reveal how regulation affects the mean-noise relationships, we study the dependence of the slope on the correlation coefficient. Figure 2I shows that the sign of β is controlled completely by correlation: small or moderate r's correspond to negative slopes whereas r's close to 1 correspond to positive slopes. We choose four representative points on the β vs r curve, three of which are located at the shadowed part with negative or zero slopes and the one is located at the outside of the shadowed part with a positive slope, to demonstrate different characteristics of the ER-regulated mean-noise relationship (Figure 2J). Interestingly, this relationship is randomly distributed if the slope is zero (Figure 2J).
Next, we perform theoretical analysis, focusing on the essential mechanism governing the mean-noise relationship. In general, the protein noise can be divided into two parts
η2m=η2int+η2prom, | (2.7) |
where η2int represents noise generated in the process of synthesis and degradation of protein, whereas η2prom represents the noise comes from the promoter architecture.
To reveal a general mechanism underlying the mean-noise relationship, we consider a gene model as which is different from the model shown in Figure 1B:
OFFfoff(t)→ON,ONfon(t)→OFF,ONμ→ON+X,Xδ→∅, | (2.8) |
where OFF-state and ON-state lifetimes follow general distributions with probability density functions denoted by foff(t) and fon(t) [30,35]. Assume that translation and protein degradation are described as first-order processes with rate constants μ and δ respectively [29,33,34,35,36]. We point out that the corresponding gene model can be taken as a GIX/M/∞ system known in the queueing literature (see [43] for details). To determine the protein mean and noise and further the mean-noise relationship in this model, the key is to derive analytical expressions for the first two moments of the protein number distribution.
Suppose that there are N proteins at initial time t=0. At time t, every protein with the molecule number denoted by Xi (1≤i≤N) has a survival probability P=e−δt, and the total number of protein molecules is given by S=X1+…+XN. Denote by W(z;t) the moment-generating function (MGF) of the protein distribution at time t. In particular, denote Winit(z) = W(z;0). Assume that these random variables are independent of one another, with each following a Bernoulli distribution with MGF given by 1+p(ez−1). Applying the theorem of total expectation in probability theory, we find that W(z;t) can be expressed by W(z;0) [29,30]. That is,
W(z;t)=Winit(log(1+e−δt(ez−1))), | (2.9) |
With (2.9) combined with a boundary condition for one cycle of OFF and ON states, we can further arrive at the following integral equation of Winit(z)
Winit(z)=∫∞s=0∫∞u=0Winit(log(1+e−δ(s+u)(ez−1)))eρ(u)(ez−1)foff(s)fon(u)dsdu, | (2.10) |
where ρ(u)=λ(1−e−δu)/δ represents the transient average for a birth-death process associated with creation and degradation of protein. This equation is a pivot for calculating the first two moments of the steady-state protein distribution.
Let ⟨mk⟩s and ⟨mk⟩u be the kth moment of the protein copy number distribution at time t=s during the OFF-state and at time t=τoff+u during the ON-state, respectively. Then, moments of the steady-state distribution of the total protein number are calculated according to
⟨mk⟩=1⟨τon⟩+⟨τoff⟩[∫∞s=0[⟨mk⟩s∫∞sfoff(t)dt]ds+∫∞u=0[⟨mk⟩u∫∞ufon(t)dt]du], | (2.11) |
where ⟨τoff⟩=∫∞0tfoff(t)dt and ⟨τon⟩=∫∞0tfon(t)dt are the mean times that the gene dwells at OFF and ON states respectively, whereas ∫∞sfoff(t)dt and ∫∞ufon(t)dt represent survival probabilities (which are actually the cumulative distribution functions of the distributions of off-state and on-state lifetimes, respectively). By (2.11), we find that the exact mean and noise intensity for protein can be expressed separately as (see Appendix)
⟨m⟩=λδ⟨τon⟩⟨τon⟩+⟨τoff⟩ | (2.12) |
and
η2m=1⟨m⟩⏟intrinsic noise+⟨τoff⟩⟨τon⟩−⟨τoff⟩+⟨τon⟩δ⟨τon⟩2(1−Lon(δ))(1−Loff(δ))1−Lon(δ)Loff(δ)⏟promoter noise, | (2.13) |
where Loff(s) and Lon(s) are Laplace transforms of foff(t) and fon(t), respectively. The (2.13) highlights how ON and OFF lifetime distributions contribute to the promoter noise and further the protein noise.
While (2.13) is valid for general waiting-time distributions, it is of interest to consider specific examples. Consider the common ON-OFF model of gene expression at the translation level, where transition rates between promoter activity states are assumed as two constants. In this case, foff(t)=(1/⟨τoff⟩)e−(1/⟨τoff⟩)t and fon(t)=(1/⟨τon⟩)e−(1/⟨τon⟩)t. Therefore, the protein mean is given by ⟨m⟩=(λ⟨τon⟩)/(⟨τoff⟩+⟨τon⟩), which is a known result [24], whereas the promoter noise is given by η2promoter=⟨τoff⟩2/(⟨τon⟩+⟨τoff⟩+⟨τon⟩⟨τoff⟩), which is also a known result [24].
After having derived the analytical expressions for protein mean and noise, we next determine two parameters α and β in the mean-noise relationship described by (2.2). For this, if denote x=log(⟨m⟩) and y=log(η2int+η2prom)=log(η2m), then we set
β=cov(x,y)σ2x,log(α)=⟨y⟩−β⟨x⟩, | (2.14) |
where cov(x,y) represents the covariance between x and y, and σ2x represents the variance of x (similarly, we let σ2y represent the variance of y). For a gene model with general waiting-time distributions, it is difficult to determine the sign of β. For the preceding ON-OFF model, however, it is possible. In fact, we first observe that the protein noise is necessarily correlated to the protein expression level through two random variables τon and τoff. Then, we note that stochastic variable y is inversely correlated to stochastic variable x because of y=−x+log(1+η2ext/η2int). Thus, from the expression β=σyσxcov(x,y)σxσy=rσyσx, we know β<0, where r represents the correlation coefficient between x and y. Such analysis indicates that in the unregulated case, β is always negative, in accordance with the results obtained by analysis of experimental data as well as with the above numerical results. Moreover, promoter structure has little effect on the size of β, as shown in the above numerical simulation. Note that we demonstrate theoretically that β is always negative in the unregulated case, but cannot give a theoretical result in correlation regulated case because of mathematical difficulty. Nevertheless, we have numerically show that mean-noise relationship is controlled by correlation between transcription factors.
In summary, we have revealed the essential molecular mechanism that controls two parameters α and β including the sign of β in the empirical mean-noise relationship formulated by log(η2)=βlogμ+logα. This mechanism revealed not only can well interpret diverse experimental phenomena in the existing literature but also can be used in analysis of sources of phenotypic heterogeneity in isogenic populations. The settings of α and β can provide insight into how detailed biochemical processes of gene expression impact the dependence of expression noise on mean. In addition, these settings can be used in the analysis of inverse problems, e.g., using experimental measurements of expression noise to determine parameters of the underlying kinetic models. Such efforts, in turn, can lead to further insights into cellular factors that impact gene regulation, based on experimental observations of expression noise [44,45,46,47].
However, there are still some limitations in our model. First, we use a model without translation bursts, though they are common in prokaryotic and eukaryotic cells [21,22]. Hence we ignore the translational bursting noise. Second, in our article, we do not consider the external noise. External noise plays an important role in biological functions, such as cell cycle and DNA replication [31,48,49]. The defects of these models can be explored as a clue in future work.
This study would have a number of potential applications. For example, from a pharmaceutical science and drug screening perspective, simply checking the mean-noise dependence line in the log space (i.e., so-called noise screening) presents an orthogonal axis to detect synergistic drug combinations [14,50]. Compared with random synergy screening, noise screening requires substantially fewer tests. In fact, blind synergy searches for pairwise combinations of M compounds require ∼M2 tests whereas noise screening needs only ∼M tests. In addition, noise screening might help identify compounds for manipulating other fate-switching phenotypes such as cellular reprogramming, metastasis, and bacterial persistence.
This work was supported by grants 91530320, 11775314, 11475273, and 11631005 from Natural Science Foundation of P. R. China; 2014CB964703 from Science and Technology Department, P. R. China; 2020B1212060032 from Guangdong Province Key Laboratory of Computational Science at the Sun Yat-sen University.
The authors have declared that no competing interest exists.
As is well known, transcription and translation occur often in a burst fashion, leading to variation in protein. Translational bursting kinetics can be characterized by burst frequency and burst size. Suppose the time intervals between bursts are independent of the burst size. These intervals are described by the distribution of the off-state life times. This description corresponds to a G/M/∞ queue with batch arrival in queuing theory [1].
Consider a gene model [2, 3], where the promoter is assumed to have one on-state and one off-state (i.e., the so-called on-off model) but off and on state life lengths are assumed to follow general distributions, denoted respectively by f(τoff) and g(τon). For simplicity, we consider only zero-order translation and first-order protein degradation kinetics, with constant translation and degradation rates denoted respectively by λ and δ.
Denote the moment-generating function of the protein distribution at time t as W(z;t). In addition, denote Winit(z)=W(z;0). Since each protein molecule has a survival probability of e−δt, the moment-generating function at time t can be expressed as a function of Winit(z) [3–5], i.e.,
W(z;t)=Winit(log(1+e−δt(ez−1))). | (3.1) |
In particular, at the end of an off-state, W(z;t) can be expressed as
W(z;τoff)=∫∞t=0Winit(log(1+e−δt(ez−1)))f(t)dt. | (3.2) |
Except that an on-period degradation of the protein molecules that have been present at the beginning of the burst continues as described through the function 1+e−δt(ez−1) with Wdeg(z;τoff+u)=Winit(log(1+e−δ(u+τoff)(ez−1))), proteins are also created and degraded according to a birth-death process with exponential waiting times. Moreover, the transient distribution for this birth-death process is a Poisson distribution with average λu=λ(1−e−δu)/δ and moment-generating function Weffect(z;τoff+u)=eλu(ez−1) [6]. The combination of them produces an effective burst size. During an on-state, the probability distribution of the protein number is given by the convolution of the distribution of the number of those molecules that are still present from previous bursts and the effective burst-size distribution. Thus, we have
W(z;τoff+u)=∫∞s=0Winit(log(1+e−δ(s+u)(ez−1)))e(μ/δ)(1−e−δu)(ez−1)f(s)ds. | (3.3) |
Note that complete of one cycle (off- and on-state) defines a boundary condition:
W(z;τon+τoff)=∫∞s=0∫∞t=sWinit(z;t)g(t−s)f(t)dtds. | (3.4) |
With (5.4), we obtain the following integral equation with respect to Winit(z)
W(z;τon+τoff)=W(z;0)=Winit(z)=∫∞s=0∫∞u=0Winit(log(1+e−δ(s+u)(ez−1)))e(λ/δ)(1−e−δu)(ez−1)f(s)g(u)dsdu. | (3.5) |
In general, solving (5.5) is very difficult for general waiting time distributions f(t) and g(t). However, we can derive the formal expressions for first two moments of the protein distribution at time t=0. In fact, differentiating both sides of (5.5) at z=0 yields
W′init(0)=dWinit(z)dz|z=0=Wini(0)∫∞u=0ρug(u)du1−∫∞u=0∫∞s=0e−δ(u+s)g(u)f(s)duds, | (3.6) |
where ρu=λ(1−e−δu)/δ. According to the definition of moment-generating function, we know that Winit(0)=1 but W′init(0) is equal to the protein mean at time t=0, denoted by ⟨m⟩init. Thus, we obtain
⟨m⟩init=W′init(0)=∫∞u=0ρug(u)du1−∫∞u=0∫∞s=0e−δ(u+s)g(u)f(s)duds. | (3.7) |
Similarly, we have the expression for the second-order moment at time t=0
⟨m2⟩init=W″init(0)=∫∞s=0∫∞u=0[ρu(1+ρu)+⟨m⟩init(e−δ(s+u)−e−2δ(s+u)+2ρue−δ(s+u))]f(s)g(u)dsdu1−∫∞s=0∫∞u=0e−2δ(s+u)f(s)g(u)dsdu. | (3.8) |
Finally, using these expressions for moments at the initial moment, we can calculate first two steady-state moments of protein. First, note that the moments of the full protein distribution can be obtained by averaging over the moments at times weighted according to the probability that the next burst has not yet occurred at time t, i.e., the survival probability Ps(t)=1−F(t) with F(t) being the cumulative distribution function of the distribution of off-state life times defined as F(t)=∫t0f(s)ds. Similarly, we define G(t)=∫t0g(s)ds. Then, to obtain the moments of the total protein number distribution, we need to average over all times according to their probabilities. This can be expressed as
⟨mk⟩=1⟨τon⟩+⟨τoff⟩[∫∞s=0⟨mk⟩s(1−F(s))ds+∫∞u=0⟨mk⟩u(1−G(u))du], | (3.9) |
with k=1,2, where F(s) and G(s) are the cumulative distribution functions of the duration distributions of OFF- and ON-states, respectively. In (5.9), ⟨mk⟩s is the kth moment of the protein copy number distribution at time S during the off-state, which is given by the k order derivative of (5.1) at z=0, ⟨mk⟩u is the kth moment of the protein copy number distribution at time t=⟨τoff⟩+u during the on-state, which is given by the k order derivative of (5.3) at z=0. By calculation, we find these quantities are
⟨m⟩s=ddzWinit(log[1+e−δs(ez−1)])|z=0=W′init(0)e−δs,⟨m2⟩s=d2dz2Winit(log[1+e−δs(ez−1)])|z=0=W″init(0)e−2δs+W′init(0)(e−δs−e−2δs), | (3.10) |
⟨m⟩u=ddz∫∞s=0Winit(log(1+e−δ(s+u)(ez−1)))eρu(ez−1)f(s)ds|z=0=W′init(0)e−δu∫∞0e−δsf(s)ds+ρuWinit(0),⟨m2⟩u=d2dz2∫∞s=0Winit(log(1+e−δ(s+u)(ez−1)))eρu(ez−1)f(s)ds|z=0=ρu(1+ρu)+W″init(0)∫∞s=0e−2δ(s+u)f(s)ds+W′init(0)∫∞s=0[e−δ(s+u)+2ρue−δ(s+u)−e−2δ(s+u)]f(s)ds. | (3.11) |
First, we derive the analytical expression for the protein mean. Note that
(⟨τon⟩+⟨τoff⟩)⟨m⟩=∫∞s=0⟨m⟩s(1−F(s))ds+∫∞u=0⟨m⟩u(1−G(u))du=∫∞s=0W′init(0)e−δs(1−∫∞0f(t)dt)ds+∫∞u=0[W′init(0)e−δu∫∞0e−δsf(s)ds+ρu](1−∫∞0g(t)dt)du=W′init(0)∫∞s=0e−δs∫∞sf(t)dtds+W′init(0)∫∞0e−δsf(s)ds∫∞u=0e−δu∫∞ug(t)dtdu+∫∞u=0ρu∫∞ug(t)dtdu. |
Because of
∫∞s=0e−δs∫∞sf(t)dtds=1δ−1δ∫∞s=0e−δsf(s)ds,∫∞u=0e−δu∫∞ug(t)dtds=1δ−1δ∫∞u=0e−δug(u)du,∫∞u=0ρu∫∞ug(t)dtdu=λδ∫∞u=0∫∞ug(t)dtd(u+1δe−δu)=λδ∫∞u=0(u+1δe−δu)g(u)du−λδ2=λδ⟨τon⟩−λδ2+λδ2∫∞u=0e−δug(u)du, |
we have
(⟨τon⟩+⟨τoff⟩)⟨m⟩=W′init(0)(1δ−1δ∫∞s=0e−δsf(s)ds)+W′init(0)∫∞0e−δsf(s)ds(1δ−1δ∫∞u=0e−δug(u)du)=+λδ⟨τon⟩−λδ2+λδ2∫∞u=0e−δug(u)du=λδ⟨τon⟩−λδ2+λδ2∫∞u=0e−δug(u)du+W′init(0)δ(1−∫∞s=0∫∞u=0e−δ(s+u)f(s)g(u)dsdu)=λδ⟨τon⟩−λδ2+1δ∫∞u=0(ρu+λδe−δu)g(u)du=λδ⟨τon⟩−λδ2+λδ2∫∞u=0g(u)du=λδ⟨τon⟩. |
Therefore,
⟨m⟩=λδ⟨τon⟩⟨τon⟩+⟨τoff⟩, | (3.12) |
which is the same as in the case of no regulation.
Then, we derive the analytical expression for the mRNA noise intensity. The key is to give the second-order moment ⟨m2⟩. Note that
(⟨τon⟩+⟨τoff⟩)⟨m2⟩=∫∞s=0⟨m2⟩s(1−F(s))ds+∫∞u=0⟨m2⟩u(1−G(u))du =∫∞s=0(⟨m2⟩s∫∞sf(t)dt)ds+∫∞u=0(⟨m2⟩u∫∞ug(t)dt)du =∫∞s=0[W″init(0)e−2δs+W′init(0)(e−δs−e−2δs)]∫∞sf(t)dtds +∫∞u=0{ρu(1+ρu)+W″init(0)∫∞s=0e−2δ(s+u)f(s)ds}∫∞ug(t)dtdu +∫∞u=0{W′init(0)∫∞s=0[e−δ(s+u)+2ρue−δ(s+u)−e−2δ(s+u)]f(s)ds}∫∞ug(t)dtdu =W″init(0)(∫∞s=0e−2δs∫∞sf(t)dt+∫∞u=0∫∞s=0e−2δ(s+u)f(s)ds∫∞ug(t)dtdu) +W′init(0)∫∞s=0(e−δs−e−2δs)∫∞sf(t)dtds +W′init(0)∫∞u=0∫∞s=0[e−δ(s+u)+2ρue−δ(s+u)−e−2δ(s+u)]f(s)ds∫∞ug(t)dtdu +∫∞u=0ρu(1+ρu)∫∞ug(t)dtdu. |
Therefore
(⟨τon⟩+⟨τoff⟩)⟨m2⟩=∫∞u=0ρu(1+ρu)∫∞ug(t)dtdu+12δW″init(0)[1−∫∞s=0∫∞u=0e−2(s+u)f(s)g(u)duds]+W′init(0)∫∞s=0(e−δs−e−2δs)∫∞sf(t)dtds+W′init(0)∫∞u=0∫∞s=0[e−δ(s+u)+2ρue−δ(s+u)−e−2δ(s+u)]f(s)ds∫∞ug(t)dtdu. |
Using (5.8), we obtain
(⟨τon⟩+⟨τoff⟩)⟨m2⟩=∫∞u=0ρu(1+ρu)(∫∞ug(t)dt+12δg(u))du+W′init(0)∫∞s=0(e−δs−e−2δs)∫∞sf(t)dtds+W′init(0)∫∞u=0∫∞s=0[e−δ(s+u)+2ρue−δ(s+u)−e−2δ(s+u)]f(s)ds(∫∞ug(t)dt+12δg(u))du. | (3.13) |
By calculation, we know
∫∞s=0(e−δs−e−2δs)∫∞sf(t)dtds=12δ−1δ∫∞0e−δsf(s)ds+12δ∫∞0e−2δsf(s)ds, |
and
∫∞u=0∫∞s=0[e−δ(s+u)+2ρue−δ(s+u)−e−2δ(s+u)]f(s)ds∫∞ug(t)dtdu=(1δ+λδ2)∫∞s=0e−δsf(s)ds−1δ(1+2λδ)∫∞s=0∫∞u=0e−δ(s+u)f(s)g(u)dsdu+λδ2∫∞s=0∫∞u=0e−δ(s+2u)f(s)g(u)dsdu−12δ∫∞s=0e−2δsf(s)ds+12δ∫∞s=0∫∞u=0e−2δ(s+u)f(s)g(u)dsdu. |
Substituting them into (5.13) yields
(⟨τon⟩+⟨τoff⟩)⟨m2⟩=−W′init(0)1δ∫∞s=0ρsf(s)ds+∫∞u=0ρu(1+ρu)∫∞ug(t)dtdu+∫∞u=0[ρu(1+ρu)2δ+1δ(12+λδ)ρu]g(u)du. | (3.14) |
Note that
∫∞u=0ρu(1+ρu)∫∞ug(t)dtdu=λδ(1+μδ)⟨τon⟩−λδ2(1+3μ2δ)+λδ2(1+2λδ)∫∞0e−δug(u)du−λ22δ3∫∞0e−2δug(u)du, |
and
∫∞u=0[ρu(1+ρu)2δ+1δ(12+λδ)ρu]g(u)du=λδ2(1+3λ2δ)−λδ2(1+2λδ)∫∞u=0e−δug(u)du+λ22δ2∫∞u=0e−2δug(u)du. |
Thus, we have
⟨m2⟩=(1+μδ)⟨m⟩−W′init(0)⟨τon⟩+⟨τoff⟩1δ∫∞s=0ρsf(s)ds. | (3.15) |
Using (5.7), we thus obtain the analytical expression for the protein noise intensity
η2m=1⟨m⟩+⟨τoff⟩⟨τon⟩−δ(⟨τoff⟩+⟨τon⟩)λ2⟨τon⟩2∫∞s=0∫∞u=0ρsρuf(s)g(u)dsdu1−∫∞s=0∫∞u=0e−δ(s+u)f(s)g(u)dsdu. | (3.16) |
If f(s) and g(u) follow exponential distribution, i.e., f(s)=(1/⟨τoff⟩)e−s/⟨τoff⟩ and g(u)=(1/⟨τon⟩)e−s/⟨τon⟩, then we have
∫∞s=0∫∞u=0ρsρuf(s)g(u)dsdu=λ2⟨τoff⟩⟨τon⟩(δ⟨τoff⟩+1)(δ⟨τon⟩+1), |
and
1−∫∞s=0∫∞u=0e−δ(s+u)f(s)g(u)dsdu=δ2⟨τoff⟩⟨τon⟩+δ(⟨τoff⟩+⟨τon⟩)(δ⟨τoff⟩+1)(δ⟨τon⟩+1). |
Therefore, we finally obtain
η2m=1⟨m⟩+⟨τoff⟩⟨τon⟩−δ(⟨τoff⟩+⟨τon⟩)λ2⟨τon⟩2∫∞s=0∫∞u=0ρsρuf(s)g(u)dsdu1−∫∞s=0∫∞u=0e−δ(s+u)f(s)g(u)dsdu, | (3.17) |
which is a know result [7].
References
1. L. Liu, B. R. K. Kashyap, J. G. C. Templeton, On the GIX/G/∞ system, J. Appl. Probab., 27 (1990), 671–683.
2. T. Jia, R. V. Kulkarni, Intrinsic noise in stochastic models of gene expression with molecular memory and bursting, Phys. Rev. Lett., 106 (2011), 058102.
3. J. J. Zhang, T. S. Zhou, Stationary moments, distribution conjugation and phenotypic regions in stochastic gene transcription, Math. Biosci. Eng., 16 (2019), 6134–6166.
4. E. Cinlar, Introduction to stochastic processes, in Prentice Hall: Englewood Cliffs, (1975).
5. A. Schwabe, K. N. Rybakova, F. J. Bruggeman, Transcription stochasticity of complex gene regulation models, Biophys. J., 103 (2012), 1152–1161.
6. M. Hemberg, H. Barahona, Perfect sampling of the master equation for gene regulatory networks, Biophys. J., 93 (2007), 401–410.
7. J. J. Zhang, L. N. Chen, T. S. Zhou, Analytical distribution and tunability of noise in a model of promoter progress, Biophys. J., 102 (2012), 1247–1257.
[1] |
A. Sanchez, S. Choubey, J. Kondev, Regulation of noise in gene expression, Annu. Rev. Biophys., 42 (2013), 469–491. doi: 10.1146/annurev-biophys-083012-130401
![]() |
[2] |
M. Kaern, T. C. Elston, W. J. Blake, J. J. Collins, Stochasticity in gene expression: from theories to phenotypes, Nat. Rev. Genet., 6 (2005), 451–464. doi: 10.1038/nrg1615
![]() |
[3] |
J. M. Raser, E. K. O'Shea, Control of Stochasticity in eukaryotic gene expression, Science, 304 (2004), 1811–1814. doi: 10.1126/science.1098641
![]() |
[4] |
W. J. Blake, G. Balazsi, M. A. Kohanski, F. J. Isaacs, K. F. Murphy, Y. Kuang, et al., Phenotypic consequences of promoter-mediated transcriptional noise, Mol. Cell, 24 (2006), 853–865. doi: 10.1016/j.molcel.2006.11.003
![]() |
[5] |
N. Q. Balaban, J. Merrin, R. Chait, L. Kowalik, S. Leibler, Bacterial persistence as a phenotypic switch, Science, 305 (2004), 1622–1625. doi: 10.1126/science.1099390
![]() |
[6] |
H. H. Chang, M. Hemberg, M. Barahona, D. E. Ingber, S. Huang, Transcriptome-wide noise controls lineage choice in mammalian progenitor cells, Nature, 453 (2008), 544–547. doi: 10.1038/nature06965
![]() |
[7] |
A. Raj, S. A. Rifkin, E. Andersen, A Van Oudenaarden, Variability in gene expression underlies incomplete penetrance, Nature, 463 (2010), 913–918. doi: 10.1038/nature08781
![]() |
[8] |
G. Hornung, R. Bar-Ziv, D. Rosin, N. Tokuriki, D. S. Tawfik, M. Oren, et al., Noise-mean relationship in mutated promoters, Genome Res., 22 (2012), 2409–2417. doi: 10.1101/gr.139378.112
![]() |
[9] |
A. Sanchez, I. Golding, Genetic determinants and cellular constraints in noisy gene expression, Science, 342 (2013), 1188–1193. doi: 10.1126/science.1242975
![]() |
[10] |
L. B. Carey, D. Van Dijk, P. M. Sloot, J. A. Kaandorp, E. Segal, Promoter sequence determines the relationship between expression level and noise, PLoS Biol., 11 (2013), e1001528. doi: 10.1371/journal.pbio.1001528
![]() |
[11] |
F. L. M. Vallania, M. Sherman, Z. Goodwin, I. Mogno, B. A. Cohen, R. D. Mitra, Origin and consequences of the relationship between protein mean and variance, PLoS ONE, 9 (2014), e102202. doi: 10.1371/journal.pone.0102202
![]() |
[12] |
S. S. Dey, J. E. Foley, P. Limsirichai, D. V. Schaffer, A. P. Arkin, Orthogonal control of expression mean and variance by epigenetic features at different genomic loci, Mol. Syst. Biol., 11 (2015), 806. doi: 10.15252/msb.20145704
![]() |
[13] |
R. D. Dar, S. M. Shaffer, A. Singh, B. S. Razooky, M. L. Simpson, A. Raj, et al., Transcriptional bursting explains the noise-versus-mean relationship in mRNA and protein levels, PLoS ONE, 11 (2016), e0158298. doi: 10.1371/journal.pone.0158298
![]() |
[14] |
R. D. Dar, N. N. Hosmane, M. R. Arkin, R. F. Siliciano, L. S. Weinberger, Screening for noise in gene expression identifies drug synergies, Science, 344 (2014), 1392–1396. doi: 10.1126/science.1250220
![]() |
[15] |
R. D. Dar, B. S. Razooky, A. Singh, T. V. Trimeloni, J. M. McCollum, C. D. Cox, et al., Transcriptional burst frequency and burst size are equally modulated across the human genome, Proc. Natl. Acad. Sci. USA, 109 (2012), 17454–17459. doi: 10.1073/pnas.1213530109
![]() |
[16] |
A. Singh, B. Razooky, C. D. Cox, M. L. Simpson, L. S.Weinberger, Transcriptional bursting from the HIV-1 promoter is a significant source of stochastic noise in HIV-1 gene expression, Biophys. J., 98 (2010), L32–L34. doi: 10.1016/j.bpj.2010.03.001
![]() |
[17] | K. Franz, A. Singh, L. S. Weinberger, Lentiviral vectors to study stochastic noise in gene expression, Methods Enzymol., 497 (2011), 603622. |
[18] |
R. Skupsky, J. C. Burnett, J. E. Foley, D. V. Schaffer, A. P. Arkin, HIV promoter integration site primarily modulates transcriptional burst size rather than frequency, PLoS Comput. Biol., 6 (2010), e1000952. doi: 10.1371/journal.pcbi.1000952
![]() |
[19] |
A. Bar-Even, J. Paulsson, N. Maheshri, M. Carmi, E. O'Shea, Y. Pilpel, et al., Noise in protein expression scales with natural protein abundance, Nat. Genet., 38 (2006), 636–643. doi: 10.1038/ng1807
![]() |
[20] |
L. H. So, A. Ghosh, C. Zong, L. A. Sepúlveda, R. Segev, I. Golding, General properties of transcriptional time series in Escherichia coli, Nat. Genet., 43 (2011), 554–560. doi: 10.1038/ng.821
![]() |
[21] |
Y. Taniguchi, P. J. Choi, G. W. Li, H. Chen, M. Babu, J. Hearn, et al., Quantifying E. coli proteome and transcriptome with single-molecule sensitivity in single cells, Science, 329 (2010), 533–538. doi: 10.1126/science.1188308
![]() |
[22] |
B. Zoller, S. C. Little, T. Gregor, Diverse spatial expression patterns emerge from unified kinetics of transcriptional bursting, Cell, 175 (2018), 835–847. doi: 10.1016/j.cell.2018.09.056
![]() |
[23] |
J. Rodriguez, G. Ren, C. R. Day, K. Zhao, C. C. Chow, D. R. Larson, Intrinsic dynamics of a human gene reveal the basis of expression heterogeneity, Cell, 176 (2019), 213–226. doi: 10.1016/j.cell.2018.11.026
![]() |
[24] |
J. J. Zhang, T. S. Zhou, Promoter-mediated transcriptional dynamics, Biophys. J., 106 (2014), 479–488. doi: 10.1016/j.bpj.2013.12.011
![]() |
[25] |
J. Paulsson, Summing up the noise in gene networks, Nature, 427 (2004), 415–418. doi: 10.1038/nature02257
![]() |
[26] |
T. B. Kepler, T. C. Elston, Stochasticity in transcriptional regulation: Origins, consequences, and mathematical representations, Biophys. J., 81 (2001), 3116–3136. doi: 10.1016/S0006-3495(01)75949-8
![]() |
[27] |
J. Peccoud, B. Ycart, Markovian modeling of gene-product synthesis, Theor. Popul. Biol., 48 (1995), 222–234. doi: 10.1006/tpbi.1995.1027
![]() |
[28] |
A. Raj, C. S. Peskin, D. Tranchina, D. Y. Vargas, S. Tyagi, Stochastic mRNA synthesis in mammalian cells, PLoS Biol., 4 (2006), e309. doi: 10.1371/journal.pbio.0040309
![]() |
[29] |
A. Schwabe, K. N. Rybakova, F. J. Bruggeman, Transcription stochasticity of complex gene regulation models, Biophys. J., 103 (2012), 1152–1161. doi: 10.1016/j.bpj.2012.07.011
![]() |
[30] |
J. J. Zhang, T. S. Zhou, Stationary moments, distribution conjugation and phenotypic regions in stochastic gene transcription, Math. Biosci. Eng., 16 (2019), 6134–6166. doi: 10.3934/mbe.2019307
![]() |
[31] | P. S. Swain, M. B. Elowitz, E. D. Siggia, Intrinsic and extrinsic contributions to stochasticity in gene expression, Proc. Natl. Acad. Sci. USA, 999 (2002), 12795–12800. |
[32] |
T. Q. Liu, J. J. Zhang, T. S. Zhou, Effect of interaction between chromatin loops on cell-to-cell variability in gene expression, PLoS Comput. Biol., 12 (2016), e1004917. doi: 10.1371/journal.pcbi.1004917
![]() |
[33] |
J. M. Pedraza, J. Paulsson, Effects of molecular memory and bursting on fluctuations in gene expression, Science, 319 (2008), 339–343. doi: 10.1126/science.1144331
![]() |
[34] |
T. Jia, R. V. Kulkarni, Intrinsic noise in stochastic models of gene expression with molecular memory and bursting, Phys. Rev. Lett., 106 (2011), 058102. doi: 10.1103/PhysRevLett.106.058102
![]() |
[35] |
J. J. Zhang, T. S. Zhou, Markovian approaches to modeling intracellular reaction processes with molecular memory, Proc. Natl. Acad. Sci. USA, 116 (2019), 23542–23550. doi: 10.1073/pnas.1913926116
![]() |
[36] |
N. Kumar, A. Singh, R. V. Kulkarni, Transcriptional bursting in gene expression analytical results for general stochastic models, PLoS. Comput. Biol., 11 (2015), e1004292. doi: 10.1371/journal.pcbi.1004292
![]() |
[37] | R. Milo, R. Phillips, emphCell biology by the numbers, Garland Science, (2015). |
[38] |
J. Holehouse, Z. Cao, R. Grima, Stochastic modeling of autoregulatory genetic feedback loops: A review and comparative study, Biophys. J., 118 (2020), 1517–1525. doi: 10.1016/j.bpj.2020.02.016
![]() |
[39] |
V. Shahrezaei, P. S. Swain, Analytical distributions for stochastic gene expression, Proc. Natl. Acad. Sci. USA, 105 (2008), 17256–17261. doi: 10.1073/pnas.0803850105
![]() |
[40] |
N. Friedman, L. Cai, X. S. Xie, Linking stochastic dynamics to population distribution: an analytical framework of gene expression, Phys. Rev. Lett., 97 (2006), 168302. doi: 10.1103/PhysRevLett.97.168302
![]() |
[41] |
Y. Liu, A. Beyer, R. Aebersold, On the dependency of cellular protein levels on mRNA abundance, Cell, 165 (2016), 535–550. doi: 10.1016/j.cell.2016.03.014
![]() |
[42] |
J. J. Zhang, Q. Nie, T. S. Zhou, A moment-convergence method for stochastic analysis of biochemical reaction networks, J. Chem. Phys., 144 (2016), 194109. doi: 10.1063/1.4950767
![]() |
[43] |
L. Liu, B. R. K. Kashyap, J. G. C. Templeton, On the GIX/G/∞ system, J. Appl. Probab., 27 (1990), 671–683. doi: 10.2307/3214550
![]() |
[44] |
D. Nicolas, B. Zoller, D. M. Suter, F. Naef, Modulation of transcriptional burst frequency by histone acetylation, Proc. Natl. Acad. Sci. USA, 115 (2018), 7153–7158. doi: 10.1073/pnas.1722330115
![]() |
[45] |
S. Wu, K. Li, Y. Li, T. Zhao, T. Li, Y. F. Yang, et al., Independent regulation of gene expression level and noise by histone modifications, PLoS Comput. Biol., 13 (2017), e1005585. doi: 10.1371/journal.pcbi.1005585
![]() |
[46] |
X. Chen, J. Zhang, The genomic landscape of position effects on protein expression level and noise in yeast, Cell Syst., 2 (2016), 347–35 doi: 10.1016/j.cels.2016.03.009
![]() |
[47] |
J. M. Schmiedel, L. B. Carey, B. Lehner, Empirical mean-noise fitness landscapes reveal the fitness impact of gene expression noise, Nat. Commun., 10 (2019), 3180. doi: 10.1038/s41467-019-11116-w
![]() |
[48] |
J. Paulsson, Models of stochastic gene expression, Phys. Life. Rev., 2 (2005), 157–175. doi: 10.1016/j.plrev.2005.03.003
![]() |
[49] |
C. H. L. Beentjes, R. Perez-Carrasco, R. Grima, Exact solution of stochastic gene expression models with bursting, cell cycle and replication dynamics, Phys. Rev. E, 101 (2020), 032403. doi: 10.1103/PhysRevE.101.032403
![]() |
[50] |
B. H. Qiu, T. S. Zhou, J. J. Zhang, Stochastic fluctuations in apoptotic threshold of tumour cells can enhance apoptosis and combat fractional killing, R. Soc. Open. Sci., 7 (2020), 190462. doi: 10.1098/rsos.190462
![]() |
1. | Kayden KM Low, Maurice HT Ling, 2024, 9780128096338, 10.1016/B978-0-323-95502-7.00105-6 |