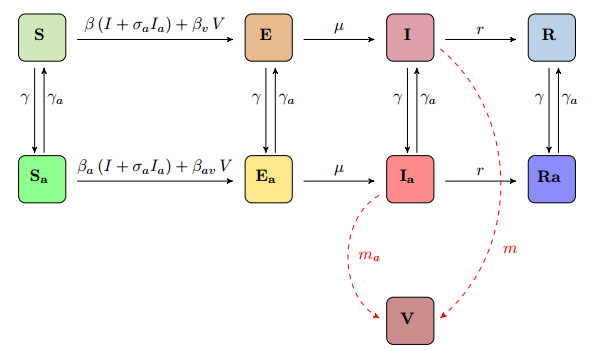
When eradication is impossible, cancer treatment aims to delay the emergence of resistance while minimizing cancer burden and treatment. Adaptive therapies may achieve these aims, with success based on three assumptions: resistance is costly, sensitive cells compete with resistant cells, and therapy reduces the population of sensitive cells. We use a range of mathematical models and treatment strategies to investigate the tradeoff between controlling cell populations and delaying the emergence of resistance. These models extend game theoretic and competition models with four additional components: 1) an Allee effect where cell populations grow more slowly at low population sizes, 2) healthy cells that compete with cancer cells, 3) immune cells that suppress cancer cells, and 4) resource competition for a growth factor like androgen. In comparing maximum tolerable dose, intermittent treatment, and adaptive therapy strategies, no therapeutic choice robustly breaks the three-way tradeoff among the three therapeutic aims. Almost all models show a tight tradeoff between time to emergence of resistant cells and cancer cell burden, with intermittent and adaptive therapies following identical curves. For most models, some adaptive therapies delay overall tumor growth more than intermittent therapies, but at the cost of higher cell populations. The Allee effect breaks these relationships, with some adaptive therapies performing poorly due to their failure to treat sufficiently to drive populations below the threshold. When eradication is impossible, no treatment can simultaneously delay emergence of resistance, limit total cancer cell numbers, and minimize treatment. Simple mathematical models can play a role in designing the next generation of therapies that balance these competing objectives.
Citation: Cassidy K. Buhler, Rebecca S. Terry, Kathryn G. Link, Frederick R. Adler. Do mechanisms matter? Comparing cancer treatment strategies across mathematical models and outcome objectives[J]. Mathematical Biosciences and Engineering, 2021, 18(5): 6305-6327. doi: 10.3934/mbe.2021315
[1] | Fulian Yin, Xinyi Tang, Tongyu Liang, Yanjing Huang, Jianhong Wu . External intervention model with direct and indirect propagation behaviors on social media platforms. Mathematical Biosciences and Engineering, 2022, 19(11): 11380-11398. doi: 10.3934/mbe.2022530 |
[2] | Roman Zúñiga Macías, Humberto Gutiérrez-Pulido, Edgar Alejandro Guerrero Arroyo, Abel Palafox González . Geographical network model for COVID-19 spread among dynamic epidemic regions. Mathematical Biosciences and Engineering, 2022, 19(4): 4237-4259. doi: 10.3934/mbe.2022196 |
[3] | Beatriz Machado, Liliana Antunes, Constantino Caetano, João F. Pereira, Baltazar Nunes, Paula Patrício, M. Luísa Morgado . The impact of vaccination on the evolution of COVID-19 in Portugal. Mathematical Biosciences and Engineering, 2022, 19(1): 936-952. doi: 10.3934/mbe.2022043 |
[4] | Pannathon Kreabkhontho, Watchara Teparos, Thitiya Theparod . Potential for eliminating COVID-19 in Thailand through third-dose vaccination: A modeling approach. Mathematical Biosciences and Engineering, 2024, 21(8): 6807-6828. doi: 10.3934/mbe.2024298 |
[5] | Elodie Yedomonhan, Chénangnon Frédéric Tovissodé, Romain Glèlè Kakaï . Modeling the effects of Prophylactic behaviors on the spread of SARS-CoV-2 in West Africa. Mathematical Biosciences and Engineering, 2023, 20(7): 12955-12989. doi: 10.3934/mbe.2023578 |
[6] | Gabriel McCarthy, Hana M. Dobrovolny . Determining the best mathematical model for implementation of non-pharmaceutical interventions. Mathematical Biosciences and Engineering, 2025, 22(3): 700-724. doi: 10.3934/mbe.2025026 |
[7] | Stefanie Fuderer, Christina Kuttler, Michael Hoelscher, Ludwig Christian Hinske, Noemi Castelletti . Data suggested hospitalization as critical indicator of the severity of the COVID-19 pandemic, even at its early stages. Mathematical Biosciences and Engineering, 2023, 20(6): 10304-10338. doi: 10.3934/mbe.2023452 |
[8] | Luyu Zhang, Zhaohua Zhang, Sen Pei, Qing Gao, Wei Chen . Quantifying the presymptomatic transmission of COVID-19 in the USA. Mathematical Biosciences and Engineering, 2024, 21(1): 861-883. doi: 10.3934/mbe.2024036 |
[9] | Hamdy M. Youssef, Najat A. Alghamdi, Magdy A. Ezzat, Alaa A. El-Bary, Ahmed M. Shawky . A new dynamical modeling SEIR with global analysis applied to the real data of spreading COVID-19 in Saudi Arabia. Mathematical Biosciences and Engineering, 2020, 17(6): 7018-7044. doi: 10.3934/mbe.2020362 |
[10] | Juan Li, Wendi Bao, Xianghong Zhang, Yongzhong Song, Zhigui Lin, Huaiping Zhu . Modelling the transmission and control of COVID-19 in Yangzhou city with the implementation of Zero-COVID policy. Mathematical Biosciences and Engineering, 2023, 20(9): 15781-15808. doi: 10.3934/mbe.2023703 |
When eradication is impossible, cancer treatment aims to delay the emergence of resistance while minimizing cancer burden and treatment. Adaptive therapies may achieve these aims, with success based on three assumptions: resistance is costly, sensitive cells compete with resistant cells, and therapy reduces the population of sensitive cells. We use a range of mathematical models and treatment strategies to investigate the tradeoff between controlling cell populations and delaying the emergence of resistance. These models extend game theoretic and competition models with four additional components: 1) an Allee effect where cell populations grow more slowly at low population sizes, 2) healthy cells that compete with cancer cells, 3) immune cells that suppress cancer cells, and 4) resource competition for a growth factor like androgen. In comparing maximum tolerable dose, intermittent treatment, and adaptive therapy strategies, no therapeutic choice robustly breaks the three-way tradeoff among the three therapeutic aims. Almost all models show a tight tradeoff between time to emergence of resistant cells and cancer cell burden, with intermittent and adaptive therapies following identical curves. For most models, some adaptive therapies delay overall tumor growth more than intermittent therapies, but at the cost of higher cell populations. The Allee effect breaks these relationships, with some adaptive therapies performing poorly due to their failure to treat sufficiently to drive populations below the threshold. When eradication is impossible, no treatment can simultaneously delay emergence of resistance, limit total cancer cell numbers, and minimize treatment. Simple mathematical models can play a role in designing the next generation of therapies that balance these competing objectives.
The severe acute respiratory syndrome coronavirus 2 (SARS-CoV-2) was first discovered in the city of Wuhan, Hubei province, China, in December 2019 [1] and has since spread all over the world with over 181.1 million reported cases and over 3.9 million deaths globally as of June 29, 2021 [2]. Following the outbreak of COVID-19, several non-pharmaceutical interventions (NPIs) such as physical distancing, isolation, hand washing, stay-at-home order, closing of schools and businesses, travel restrictions, among others, were implemented all over the world to limit the spread of the disease [3,4,5,6]. Despite implementing these NPIs, there is still a significant number of COVID-19 cases and deaths reported daily. On January 20, 2020, the World Health Organization (WHO) declared COVID-19 a public health emergency of international concern (PHEIC) [7] and a pandemic on March 11, 2020 [8]. The COVID-19 pandemic has proven to be more than just a medical emergency; But one that affects society. It has significantly affected our day-to-day activities such as socializing, entertainment, education, tourism, health, and business. The need to find an effective way of controlling the pandemic through integrated science cannot be overemphasized [9].
Since the outbreak of the SARS-CoV-2 virus, researchers have been studying its transmission dynamics. The virus is believed to be transmitted through two major pathways namely: the direct and indirect pathways [10,11,12,13,14]. The direct pathway, also known as human-to-human transmission, includes transmission through inhalation of virus-infected droplets, coughing, sneezing and having physical contact with infectious persons. Indirect transmission may occur when a susceptible individual comes in contact with a contaminated commonly shared surface or object [10,13]. These contaminations usually occur when an infectious individual touches, coughs or sneezes on the objects.
Several mathematical models have been developed to study the transmission dynamics of COVID-19 [15,16,17,18,19,20,21,22,23,24,25,26,27,28]. In [26], two differential equation models were developed to study the effect of the exposed or latency period on the dynamics of COVID-19 in China. Another model was developed in [28] to study the effect of super-spreaders on the dynamics of COVID-19 in Wuhan, China. These and many other mathematical models have provided a lot of insights into the dynamics of COVID-19. During the early stage of the COVID-19 pandemic, physical distancing, wearing of face masks, hand washing, and other non-pharmaceutical interventions (NPIs) were the primary interventions against the disease worldwide. Many mathematical models have been used to study the impact of these NPIs on the dynamics of COVID-19 [19,29]. This includes the susceptible-exposed-infected-quarantine-recovered (SEIQR) model developed in [25] to study the impact of physical distancing on the dynamics of COVID-19 in British Columbia (BC), Canada, and the model of [23] used to study the impact of different mitigation strategies on the dynamics of COVID-19 in Ontario, Canada. In [30], a {susceptible-exposed-infected-removed} (SEIR) model was designed on a temporal network, which evolves according to the activity-driven paradigm. Their model was used to study the spread of the disease as a function of the fraction of the population following public health measures. Their result shows that physical distancing and mask-wearing can effectively prevent COVID-19 outbreaks if adherence to both measures involves a substantial fraction of the susceptible population. The effect of timing of adherence to COVID-19 NPIs such as social distancing on COVID-19 in the United States was studied using an agent-based model in [31]. Their model was applied to Dane County, Wisconsin, the Milwaukee metropolitan area and New York City (NYC), and show that the timing of implementing and easing the social distancing measures has major effects on the number of COVID-19 cases. The results obtained in the works of [32,33] are also in the same line with the findings mentioned above. Many of these models focus on direct transmission of COVID-19 through in-person contacts despite the emphasis by the world health organization (WHO) on indirect transmission of the disease [13].
A few researchers have studied indirect transmission of COVID-19 [20,21,22,34,35,36,37]. In [35], a novel deterministic susceptible-exposed-infected-removed-virus-death (SEIRVD) model was developed to study the potential impact of both direct and indirect transmission of COVID-19 on the dynamics of the disease in Ontario, Canada. Their results showed significant increase in number of cases with indirect transmission. They also highlighted the importance of implementing additional preventive and control measures to minimize the spread of COVID-19 through both transmission routes. A five-compartment model for both direct and indirect transmission pathways was developed in [37], and used to study the impact of both transmission pathways on the dynamics of COVID-19. The mathematical model of [36] also used indirect transmission mechanisms to study the dynamics of COVID-19. They examined the conditions under which contaminated objects may lead to a significant spread of SARS-CoV-2 during and after lock-down using a SEIR model with the addition of a fomite term. They proposed that the addition of a fomite term will help to better understand the transmission dynamics of the virus and also in policy making. To the best of our knowledge, none of these studies have looked at the effect of adherence and non-adherence to COVID-19 NPIs on both direct and indirect transmissions of the disease.
In this study, we develop a susceptible-exposed-infectious-recovered-virus (SEIRV) compartmental model for studying the transmission dynamics of COVID-19. Our model considers both direct and indirect transmission of the disease following the approach of [35]. It has a similar structure to the model of [25] used to study the impact of physical distancing on the contact rates in British Columbia (BC), Canada, where BC population was divided into the groups practicing physical distancing and those not practicing physical distancing. Here, we stratify our population into two groups: those that adhere to all COVID-19 non-pharmaceutical interventions (NPIs) and those that do not adhere. We compute the control reproduction number and final epidemic size for our model, and study the effect of different parameters of the model on these quantities. This model is used to assess the impact of adherence to COVID-19 non-pharmaceutical interventions and indirect transmissions on the dynamics of the disease. Our study highlights the importance of adhering to the COVID-19 NPIs as instructed by the World Health Organization (WHO) in reducing infections and eventually controlling the spread of the disease.
The remaining part of this paper is organized as follows: The model is developed in Section 2. In Section 3, the control reproduction number of the disease is calculated using the next generation matrix approach. We also computed a final size relation for the epidemic in this section. In Section 4, we numerically study the effect of important model parameters on the control reproduction number calculated in Section 3. In addition, we present numerical simulations of the SEIRV model for different scenarios in this section. These simulations are used to comprehend the effect of direct and indirect transmission, with or without adherence on the dynamics of the disease. We conclude the paper in Section 5 with a brief discussion.
A compartmental SEIRV model of COVID-19 epidemic is developed and analyzed. The model considers both direct and indirect transmission routes and divides the population into two main groups: adherent and non-adherent populations. The adherent population include individuals in the population that adhere to all COVID-19 non-pharmaceutical interventions (NPIs), while the non-adherent population contains those that do not adhere to the NPIs. The compartments of the model for non-adherent population are defined as follows: susceptible (S); exposed (E); infectious (I); and recovered/removed (R). Corresponding compartments for the adherent population are given by Sa, Ea, Ia, and Ra, respectively. Note that individuals in the exposed compartments E and Ea are not infectious [26,38,39,40,41]. We assume that recovered individuals have permanent immunity from the disease (no reinfection). The compartment V accounts for the virus shed on contaminated surfaces and objects by infectious individuals.
Figure 1 shows a schematic diagram of the model, where the solid black arrows indicate the direction of the flow of individuals between the compartments at the rates indicated beside the arrows. The dashed red arrows show virus shedding or contamination of surfaces by infectious individuals. The differential equations for non-adherent population are given by
dSdt=−βSN(I+σaIa)−βvSNV+γaSa−γS,dEdt=βSN(I+σaIa)+βvSNV−μE+γaEa−γE,dIdt=μE−rI+γaIa−γI,dRdt=rI+γaRa−γR, | (2.1a) |
where β and βv are the direct and indirect transmission rates, respectively, for non-adherent population. The parameter σa, with 0≤σa≤1 is used to model the reduction in virus shedding (or contamination of surfaces), susceptibility, and onward transmission of disease due to adherence to NPIs. Parameters γa and γ are the rates of movement from adherence to non-adherence and vice versa, respectively, while μ denotes the rate of transitioning from the exposed compartment E to the infectious compartment I, and r is the recovery rate. The corresponding equations for the adherent population are given by
dSadt=−βaSaN(I+σaIa)−βavSaNV−γaSa+γS,dEadt=βaSaN(I+σaIa)+βavSaNV−μEa−γaEa+γE,dIadt=μEa−rIa−γaIa+γI,dRadt=rIa−γaRa+γR, | (2.1b) |
where Sa,Ea,Ia and Ra denote susceptible, exposed, infectious, and recovered individuals in the adherent population, respectively. The parameters βa and βav are the direct and indirect disease transmission rates, respectively, for the adherent population. We assume that individuals in both adherent and non-adherent population transition from exposed to infectious compartments, and recover from the disease at the same rates, μ and r, respectively. In addition, we assume that individuals move from adherent to non-adherent and vice versa, at the same rates in the susceptible, exposed, infectious, and recovered compartments. The disease dynamics for both non-adherent (2.1a) and adherent (2.1b) populations are coupled to the dynamics of the virus in the environment or on contaminated surfaces given by
dVdt=maIa+mI−(τ+δ)V, | (2.1c) |
where m and ma are the virus shedding (or surface contamination) rates for non-adherent and adherent populations, respectively. The parameter δ is the decay rate of SAR-CoV-2 in the environment and on surfaces, and τ is the environment cleaning/sanitization rate. We assume that our population is constant throughout the epidemic period so that N=N⋆(t)+Na(t) for all time t≥0, where N⋆(t)=S(t)+E(t)+I(t)+R(t) and Na(t)=Sa(t)+Ea(t)+Ia(t)+Ra(t). In our SEIRV model (2.1), the compartments S,E,I,R,Sa,Ea,Ia,Ra have dimension of individual, while the virus compartment V has dimension of virus particle. The dimensions of the model parameters are given as follows:
[β]=[βa]=1time,[βv]=[βav]=individualvirus particle×time,[γ]=[γa]=[μ]=[r]=[τ]=[δ]=1time,[m]=[ma]=virus particleindividual×time, | (2.2) |
where [λ] represents dimension of λ. Note that σa is a dimensionless parameter in our model.
It is important to mention that there are relationships between the parameters β,βv, and m for non-adherent population and βa,βav, and ma of adherent population, respectively. These relationships are used when computing the control reproduction number in Section 3.1 and final size relation in Section 3.2. We define the fraction of adherence in the population as f=γ/(γ+γa), on which the dynamics of the ODE system (2.1) depends. Since adherence to COVID-19 NPIs reduces transmission rate for the adherent population, we equate the direct and indirect transmission rates for the adherent population to those of non-adherent population as follow
βa=σaβandβav=σaβv, | (2.3) |
where 0≤σa≤1. When σa=0, we have βa=0, βav=0 and σaIa=0 in (2.1a) and (2.1b), which implies that adherence to NPIs is 100% effective in preventing infections in the adherent population, and that adherent individuals do not infect the non-adherent individuals. On the other hand, when σa=1, we have βa=β, βav=βv and σaIa=Ia, which implies that disease is transmitted at the same rate in both adherent and non-adherent populations. Table 1 shows the model variables and their description.
Variable | Description |
S | Non-adherent susceptible population |
E | Non-adherent exposed population |
I | Non-adherent infectious population |
R | Non-adherent recovered population |
Sa | Adherent susceptible population |
Ea | Adherent exposed population |
Ia | Adherent infectious population |
Ra | Adherent recovered population |
V | Virus compartment |
Table 2 shows the parameters of the SEIRV model (2.1) with their descriptions and values. Some of these parameters were obtained from published articles, while others were either inferred, derived or varied. References are provided for parameters that were obtained from published articles. The direct and indirect transmission rates (βa and βav), and shedding rate ma for adherent population were derived from those of non-adherent population, respectively, using the relations in (2.3).
Parameter | Description | Value | Reference |
N | Total population | 5,000,000 | Assumed |
σa | Reduction in virus shedding, susceptibility, and onward transmission of disease due to adherence to NPIs | Varied | |
β | Direct transmission rate for non-adherence population | 0.5 | [25,35,49] |
βv | Indirect transmission rate for non-adherence population | 0.15 | [35,36] |
βa | Direct transmission rate for adherence population | σaβ | Derived |
βav | Indirect transmission rate for adherence population | σaβv | Derived |
γ | Rate of movement from non-adherent to adherent | 0.3 day−1 | [25,50,51,52] |
γa | Rate of movement from adherent to non-adherent | 0.25 day−1 | [50,51,52] |
m | Virus shedding rate or the rate of contamination of surfaces by infectious individuals in non-adherent population | 0.125 ml−1 person−1 day−1 | [35,53,54] |
ma | Virus shedding rate or the rate of contamination of surfaces by infectious individuals in the adherent population | σam | Derived |
δ | Decay rate of virus in the environment | 0.4 day−1 | [55,56,57,58,59] |
τ | Environment cleaning/sanitization rate | 0.1 day−1 | Inferred |
r | Recovery rate (1/r is the infectious period) | 1/5 day−1 | [25,39,60,61,62,63] |
μ | Rate of transitioning from the exposed to infectious compartments (1/μ is the incubation period) | 1/6 day−1 | [39,40,41,63,64] |
In this section, we calculate the control reproduction number and the final size relation for the epidemic using the SEIRV model (2.1). We also consider some limiting scenarios of the epidemic and derive the control reproduction number and final size relations for these scenarios from the general control reproduction number and final size relation, respectively.
We compute the control reproduction number for our model (2.1) using the next generational matrix approach of [42,43,44,45,46,47] as used in [48]. The next generation matrix is a square matrix whose elements describes who is infecting whom in the population, and is defined as M=FV−1, where F and V are square matrices for the rates of new infections from the susceptible population and transfer between infected populations, respectively. The dominant eigenvalue of the next generation matrix is the basic (or control) reproduction number of the epidemic. See [42,43,47] for more details. To construct the F and V matrices, we consider the exposed and infectious compartments of the SEIRV model (2.1) given by,
dEdt=βSN(I+σaIa)+βvSNV−μE+γaEa−γE,dIdt=μE−rI+γaIa−γI,dEadt=βaSaN(I+σaIa)+βavSaNV−μEa−γaEa+γE,dIadt=μEa−rIa−γaIa+γI,dVdt=maIa+mI−(τ+δ)V. | (3.1) |
Upon using the relations between the transmission rates for the adherent and non-adherent populations given in (2.3), (3.1) reduce to
dEdt=βSN(I+σaIa)+βvSNV−μE+γaEa−γE,dIdt=μE−rI+γaIa−γI,dEadt=σaβSaN(I+σaIa)+σaβvSaNV−μEa−γaEa+γE,dIadt=μEa−rIa−γaIa+γI,dVdt=maIa+mI−(τ+δ)V. | (3.2) |
Recall that the adherence fraction in the population is given by f=γ/(γ+γa). Therefore, at the beginning of the epidemic, S(0)=(1−f)N=N⋆(0) and Sa(0)=fN=Na(0). Following the arrangement of the equations in (3.2) and the variables ϕ=(E,I,Ea,Ia,V), at the DFE = (N⋆(0),0,0,0,Na(0),0,0,0,0), the matrix for {the rates of new infections from the susceptible population F} is given by
F=(0β(1−f)0βσa(1−f)βv(1−f)000000σaβf0σ2aβfσaβvf0000000000). | (3.3) |
Similarly, the Jacobian matrix for the rates of transfer between infected populations at the beginning of the epidemic is given by
V=((μ+γ)0−γa00−μ(r+γ)0−γa0−γ0(μ+γa)000−γ−μ(r+γa)00−m0−ma(τ+δ)). | (3.4) |
Upon constructing the next generation matrix using (3.3) and (3.4), and computing its spectral radius using the symbolic computational software SageMath [74], we obtain that the control reproduction number satisfies
Rc=RD+RID, | (3.5a) |
where RD, which accounts for disease transmission through direct route among non-adherent and adherent individuals is given by
RD=RD⋆+σaRDa. | (3.5b) |
Here, RD⋆ and RDa are defined as
RD⋆=β(1−f)Φr(γa+γ+μ)(γa+γ+r)andRDa=βfΨr(γa+γ+μ)(γa+γ+r). | (3.5c) |
The second term in the control reproduction number (3.5a) accounts for indirect transmissions, and it is given by
RID=RID⋆+σaRIDa, | (3.5d) |
where RID⋆ and RIDa are the indirect transmissions contributed by the non-adherent and adherent populations, respectively, and are given by
RID⋆=βv(1−f)[m(χa+rμ)+maχ]r(τ+δ)(γa+γ+μ)(γa+γ+r)andRIDa=βvf[ma(χ+rμ)+mχa]r(τ+δ)(γa+γ+μ)(γa+γ+r). | (3.5e) |
The newly introduced variables χ, χa, Φ, and Ψ are defined as follows:
χ=γ2+γγa+γ(μ+r)andχa=γ2a+γγa+γa(μ+r) | (3.5f) |
Φ=χa+μr+σaχandΨ=χa+σa(χ+μr). | (3.5g) |
Suppose there is no adherent population, that is, {f=0 and γ=0 (consequently, σa=0 and γa=0), which gives Φ=μr and Ψ=0}. Therefore, (3.5a) reduces to
^Rc=1r(β+βvmδ+τ), | (3.6) |
where ˆRD=β/r accounts for infections cause through direct transmission, while ˆRID=βvm/(r(δ+τ)) accounts from those of indirect transmission. Observe that ˆRD will increase as both the transmission rate β and {infectious period} 1/r increase. This shows that an infectious individual will spread the virus/disease more if the transmission rate of the {disease increases} and if it takes longer for them to recover from the disease. For ˆRID=βvm/(r(δ+τ)), infections increases as the shedding/contamination rate of infectious individuals increase and decrease as the virus decay rate δ and the environment cleaning or sanitization rate τ increases. Assuming a fraction ˜f of the population were already adhering to COVID-19 NPIs before the disease was introduced into the population (as a precaution before the first case arrives) and there is no movement from adherent to non-adherent and vice versa, that is, γ=0 and γa=0, but σa≠0, (3.5a) reduces to
˜Rc=βr[(1−˜f)+σ2a˜f]+βvr(τ+δ)[(1−˜f)m+σama˜f], | (3.7) |
where the parameter σa with 0≤σa≤1 account for the reduction in acquiring or transmitting the disease due to adherence to COVID-19 NPIs. Similar to (3.5a) and (3.6), the control reproduction number ˜Rc for this scenario also accounts for the contribution from both direct and indirect transmissions. Here, the contribution to ˜Rc due to direct transmissions is given by
˜RD=βr[(1−˜f)+σ2a˜f], | (3.8) |
where β(1−˜f)/r accounts for infections through direct transmissions caused by those that do not adhere to the NPIs, while σ2a˜fβ/r is the contribution from those that adhere to the NPIs. As one would expect, the transmissions caused by the adherent population will always be less than the infections caused by those that do not adhere if ˜f<0.5 since 0≤σa≤1. For this scenario, the contribution from indirect transmission is given by
˜RID=βvr(τ+δ)[(1−˜f)m+σama˜f], | (3.9) |
where βv(1−˜f)m/[r(δ+τ)] is the contribution from the non-adherent population and σaβvma˜f/[r(δ+τ)] is from the adherent population. Note that ˜f is the fraction of the population that adhere to the COVID-19 NPIs at the beginning of the epidemic.
Next, we derive a relation for the final epidemic size of our SEIRV model (2.1). This final size relation gives the total number of cases of the disease in the population during the epidemic. We consider a scenario where there is no movement between adherent and non-adherent susceptible populations. In other words, we assume that susceptible individuals do not change their behaviour from adhering to the COVID-19 NPIs to non-adherence and vice versa. This way, the equation for the susceptible populations S and Sa in (2.1a) and (2.1b), respectively, reduce to
dSdt=−βSN(I+σaIa)−βvSNVanddSadt=−βaSaN(I+σaIa)−βavSaNV. | (3.10) |
We assume that a fraction f, with 0≤f≤1, of the susceptible population adhere to COVID-19 NPIs before a disease outbreak, so that S(0)=(1−f)N=N⋆(0) and Sa(0)=fN=Na(0). In addition, we assume that at the beginning of the epidemic, {there is a small number Ea(0)=Ea0 and Ia(0)=Ia0 in the adherent population, with E(0)=E0 and I(0)=I0 in the non-adherent populations. All other compartments are initially empty}. Following the approach in [48,65,66,67,68,69,70,71,72,73] and using the ODE system (2.1) together with the assumptions for the susceptible population in (3.10), we derive the final size relation for non-adherent population as
logS0S∞=♣1[1−S∞N⋆(0)]+♠1[1−Sa∞Na(0)]+♢1fIa(0)Na(0)+♡1(1−f)I(0)N⋆(0)−♣1I(0)N⋆(0)−♠1Ia(0)Na(0)+βv(1−f)V0(τ+δ)N⋆(0), | (3.11a) |
where ♣1=RD⋆+RID⋆ and ♠1=RDa+RIDa with RD⋆,RID⋆,RDa and RIDa as given in (3.5). The remaining variables ♢1 and ♡1 are defined as follows
♢1=β(σa(r+γ)+γa)r(r+γ+γa)+βv(ma(r+γ)+mγa)r(τ+δ)(r+γ+γa),♡1=β(σaγ+(r+γa))r(r+γ+γa)+βv(maγ+m(r+γa))r(τ+δ)(r+γ+γa). | (3.11b) |
Similarly, the final size relation for adherent population is given by
logSa0Sa∞=♣2[1−S∞N⋆(0)]+♠2[1−Sa∞Na(0)]+♢2fIa(0)Na(0)+♡2(1−f)I(0)N⋆(0)−♣2I(0)N⋆(0)−♠2Ia(0)Na(0)+βvfV0(τ+δ)Na(0), | (3.12) |
where ♣2=σa♣1,♠2=σa♠1,♢2=σa♢1 and ♡2=σa♡1 with ♣1,♠1,♢1 and ♡1 as given in (3.11). The final size relations in (3.11) and (3.12) for non-adherent and adherent population, imply that S∞>0 and Sa∞>0, respectively. They give the relationship between the reproduction number Rc and the final epidemic size. The total infected population over the course of the epidemic is given by Na(0)−Sa∞ and N⋆(0)−S∞ for the adherent and non-adherent population, respectively, which can be described in terms of the attack rates as [1−Sa∞Na(0)] and [1−S∞N⋆(0)] as in [67]. The final size relations in (3.11) and (3.12) can be written together in matrix form as
(logS0S∞logSa0Sa∞)=(♣1♠1σa♣1σa♠1)(1−S∞N⋆(0)1−Sa∞Na(0))−(♣1♠1σa♣1σa♠1)(I(0)N⋆(0)Ia(0)Na(0))+(♢1♡1σa♢1σa♡1)((1−f)I(0)N⋆fIa(0)Na)+(βv(1−f)V0(τ+δ)σaβvfV0(τ+δ))(1N⋆(0)1Na(0)). | (3.13) |
Also, from (3.11) and (3.12), we derive the following relation between the two final sizes
Sa∞Sa0=(S∞S0)σa | (3.14) |
Since 0≤σa≤1, we have
Sa∞Sa0≥S∞S0 | (3.15) |
When Sa∞Sa0>S∞S0, we conclude that the attack rate for the adherent population is smaller than that of the non-adherent population as a result of the reduction in the susceptibility and transmissibility of the adherent population. Observe that when σa=1, which implies that there is no protection from adhering to NPIs, we have from (3.14) that Sa∞/Sa0=S∞/S0. This implies that the {attack rate} is the same in both adherent and non-adherent populations, and as a result, we expect the epidemic size to be the same in both populations. This result agrees with our numerical simulation shown in red in the top left panel of Figure 4. On the other hand, when σa=0, which implies that adhering to NPIs is 100% effective in preventing new infections, (3.14) reduces to Sa∞=Sa0. This shows that there are no infections in the adherent population throughout the epidemic. Therefore, adherence to the NPIs during the pandemic is beneficial to reducing/containing the spread of the disease.
When the outbreak begins with no infectious individuals, that is, the outbreak begins through an indirect transmission, the final size relations in (3.11) and (3.12) reduce to
logS0S∞=♣1[1−S∞N⋆(0)]+♠1[1−Sa∞Na(0)]+βv(1−f)V0(τ+δ)N⋆(0),logSa0Sa∞=♣2[1−S∞N⋆(0)]+♠2[1−Sa∞Na(0)]+βvfV0(τ+δ)Na(0). | (3.16) |
Similarly, when the outbreak begins with only direct transmission with an infective, (3.11) and (3.12) become
logS0S∞=♣1[1−S∞N⋆(0)]+♠1[1−Sa∞Na(0)]+♢1fIa(0)Na+♡1(1−f)I(0)N⋆−♣1I(0)N⋆(0)−♠1Ia(0)Na(0),logSa0Sa∞=♣2[1−S∞N⋆(0)]+♠2[1−Sa∞Na(0)]+♢2fIa(0)Na+♡2(1−f)I(0)N⋆−♣2I(0)N⋆(0)−♠2Ia(0)Na(0). | (3.17) |
Now, consider an instance where there is no adherent population, so that f=0,σa=0,γ=0, and γa=0. For this scenario, we have ♣1=ˆRc, where ˆRc is as given in (3.6), ♠1=0, Ia(0)=0, N⋆(0)=N, ♣1=♡1. Also, since σa=0, we have ♣2=♠2=♢2=♡2=0. This way, (3.11) and (3.12) reduces to
logS0S∞=ˆRc[1−S∞N]+βvV0(τ+δ)N, | (3.18) |
where ˆRc=β/r+βvm/(r(δ+τ)) is the control reproduction number, given in (3.6), for the scenario without adherent population. As expected, when there is no adherent population, the final size relation reduces to that of a single population, and depends on the control reproduction number. When a fraction ˜f of the population adhere to COVID-19 NPIs but there is no movement between the adherent and non-adherent populations throughout the epidemic, that is, γ=γa=0 and σ≠0. The final size relation for non-adherent population given in (3.11) reduces to
logS0S∞=˜♣1[1−S∞N⋆(0)]+˜♠1[1−Sa∞Na(0)]+βv(1−f)V0(τ+δ)N⋆(0), | (3.19) |
where
˜♣1=β(1−f)r+βvm(1−f)r(τ+δ)and˜♠1=βσafr+βvfmar(τ+δ). | (3.20) |
Similarly, for the adherent population, the final size relation (3.12) reduces to
logSa0Sa∞=˜♣2[1−S∞N⋆(0)]+˜♠2[1−Sa∞Na(0)]+βvfV0(τ+δ)Na(0), | (3.21) |
where ˜♣2=σa˜♣a and ˜♠2=σa˜♠1 with ˜♣1 and ˜♠1 as defined in (3.20). Let ˜S0 and ˜S∞ be the total susceptible population at the beginning and the end of the epidemic, respectively. Then at the beginning of the epidemic, we have
S0=(1−˜f)˜S0andSa0=˜f˜S0, | (3.22) |
where S0 and Sa0 are the initial susceptibles in non-adherent and adherent populations, respectively. Since there is no movement between the adherent and non-adherent population, the following conditions are satisfied at the end of the epidemic
S∞=(1−˜f)˜S∞andSa∞=˜f˜S∞, | (3.23) |
where S∞ and Sa∞ are the susceptibles left in non-adherent and adherent populations, respectively, at the end of the epidemic. Upon adding (3.19) and (3.21), and using (3.22) and (3.23), and ma=σam, we have
log˜S0˜S∞=12(˜Rc+ˆRc)[1−˜S∞N]+βvV0(τ+δ)N, | (3.24) |
where ˆRc and ˜Rc are as given in (3.6) and (3.7), respectively.
In the next section, we present numerical computations of the control reproduction number (3.5) for different parameters of the model. We also present numerical simulations of the SEIR model (2.1), where we numerically computed final epidemic sizes for different scenarios.
In this section, we study the effect of different parameters of the model on the control reproduction number Rc, given in (3.5). These results are presented as contour plots. In addition, we present numerical simulations of the SEIRV model (2.1) and investigate the effect of different parameters of the model on the disease dynamics and final epidemic size.
In Figure 2, we present contour plots of the control reproduction number (3.5) computed as a function of different parameters of the model (2.1). The top left panel of this figure shows Rc as a function of the direct and indirect transmission rates. As one would expect, Rc increases as both transmission rates increase. We also notice that the direct transmission rate β has more effect on the control reproduction number than the indirect transmission rate βv. This may be because indirect transmissions are mitigated by environment cleaning and virus decay rate. In the top right panel of the same figure, we have Rc as a function of the adherence rate γ and the direct transmission rate β. This result shows that the adherence rate has little effect on the control reproduction number when the transmission rate is low, but has more effect on it as the transmission rate increases. When the transmission rate is low, there is a lesser chance of getting infected, as a result, adherence to the NPIs will have little effect on the spread of infection. However, when transmission rate is high, adhering to the NPIs will significantly reduce disease transmission. Similar result is shown in the middle left panel of Figure 2 for the adherence rate γ and the indirect transmission rate βv. For this scenario, Rc increases as the adherence to NPIs decreases even for a low indirect transmission rate, unlike the case of direct transmission (top right panel of Figure 2), where adherence rate does not affect Rc when β is small. The reason for this is that indirect transmission does not only depend on the transmission rate. It also depends on the environment cleaning/sanitization rate and the shedding and decay rate of the virus. If a lot of shedding is happening, or the decay rate of the virus is low, there may be a need to adhere more to the NPIs to reduce the spread of the disease.
For a fixed indirect transmission rate, Rc increases as the virus shedding rate increases, although, if the transmission rate is low, Rc remains constant irrespective of the shedding rate. Similarly, for a fixed shedding rate m for non-adherent population, Rc increases as βv increases, but remains constant when shedding rate is too low irrespective of the value of βv (see the middle right panel of Figure 2). This result shows that when infectious individuals do not shed much virus or contaminate surfaces, there will be no indirect disease transmission through these surfaces. Similar result is obtained when Rc is computed as a function of the shedding rate ma for the adherent population and the indirect transmission rate βv. In the bottom panel of Figure 2, we present Rc as a function of the environment cleaning/sanitization rate and the indirect transmission rate βv (left panel), and the environment cleaning/sanitization rate and the shedding rate of non-adherent individuals m (right panel). Both results show that Rc decreases as the environment cleaning/sanitization rate increases, and increases with increase in the transmission and shedding βv and m, respectively.
Figure 3 shows the total infectious population (I(t)+Ia(t)) over time for different rates of movement from adherent population to non-adherent and vice versa. These results are used to investigate the effect of adherence to COVID-19 NPIs on the dynamics of the disease. We consider four (4) different scenarios based on the rate of movement from adherent to non-adherent population γa and the rate of moving from non-adherent to adherent population γ for different values of the parameter σa, used to model the reduction in susceptibility, shedding of virus, and onward disease transmission for the adherent population. The first scenario shown in the top left panel of Figure 3 is for γ=γa=0, where there is no movement of individuals between non-adherence and adherence populations. For this scenario, the epidemic final size is 60.19% when σa=0.5, 80.71% when σa=0.75, and 91.43% when σa=1. Recall that the epidemic final size is the total fraction of the population that becomes infected during the course of the epidemic. As expected, the largest epidemic occurs when there is no protection derived from adhering to the NPIs, that is, when σa=1. Epidemic decreases as the protection from NPIs increases (σa decreases). Similar results are observed for the remaining scenarios in this figure. When there is movement between the adherent and non-adherent population such that the rate of going from adherence to non-adherence is the same as that of going from non-adherence to adherence, specifically, when γ=γa=0.3, the final epidemic size is 59.50% for σa=0.5, 81.20% for σa=0.75 and 91.43% for σa=1. The plots for this scenario are shown in the top right panel of Figure 3.
In the bottom panel of Figure 3, we show the results for γ=0.25 and γa=0.3 (left panel), and γ=0.3 and γa=0.25 (right panel). For the result in the left panel where the rate of movement from the adherent population to non-adherent (γa) is higher than that of going from non-adherence to adherence (γ), the epidemic final sizes are 64.86%,82.49% and 91.43% for σa=0.5,σa=0.75 and σa=1, respectively. On the other hand, for γ=0.3 and γa=0.25 (bottom right panel of Figure 3), where the rate of movement from the non-adherence to adherence is higher than that of going from adherent population to non-adherent, the epidemic final size is 53.41% for σa=0.5, 79.83% for σa=0.75, and 91.43% for σa=1. We observe from the results in this figure that the epidemic size decrease as σa decreases. This shows that fewer infections are occurring as people get more protection from adhering to the NPIs, since the benefit of adhering to NPIs increases as σa decreases. In addition, the epidemic size when σa=1 (no protection due to adherence to NPIs) is the same for any values of γ and γa. For σa=0.5 and σa=0.75, the smallest epidemics were predicted for the scenario where the rate of movement from non-adherence to adherence γ is higher than that of moving from adherence to non-adherence γa (bottom right panel of Figure 3). Whereas the largest epidemics occur when the rate of adherence γ=0.25 is lower than that of non-adherence γa=0.3. These results show that there will be smaller epidemic if more people adhere to the NPIs.
The results in Figure 4 were obtained using the same parameters and initial conditions as those in Figure 3 but plotted in terms of the adherent and non-adherent populations. Presenting the results in this way enables us to study the dynamics of the two populations as the movement rates γ and γa are varied. We notice from the results in this figure that even though the final epidemic size is the same for all four scenarios when σa=1 (as discussed earlier in Figure 3), the dynamics of the disease is different for each scenario. When γ=γa, the disease dynamics is the same for both adherent and non-adherent population when σa=1 (see the red plots in the top panel of Figure 4). This is because the population is divided into two equal halves between the adherent and non-adherent population for these scenarios, and no protection is derived from adhering to the NPIs (since σa=1). When σa≠1, for the scenario with γ=γa=0.3, the dynamics of the disease in the two populations are the same irrespective of σa (see top right panel of Figure 4). However, this is not the case for γ=γa=0, even though f=0.5 in both scenarios. For σa=0.5 and σa=0.75, there are more infections in non-adherent population than the adherent population. This is as a result of the protection derived from adhering to the COVID-19 NPIs. When γ=0.25 and γa=0.3, there are more infections in the non-adherent population than the adherent population for all values of σa. This is due to the fact that there are more people in the non-adherent population since the non-adherence rate is higher (γa>γ) and they do not get protection from the NPIs. The most interesting and counter-intuitive result is when γ=0.3 and γa=0.25 (bottom right panel of Figure 4). This result shows that there are more infections in the adherent population than non-adherent population. Recall that γ is the adherence rate and γa is the non-adherence rate. For γ=0.3 and γa=0.25, the initial fraction of the population that adhere to COVID-19 NPIs is f≈0.5455, which is larger than the population of those that do not adhere. As time goes on, more people adhere to COVID-19 NPIs than they stop adhering since γ>γa. This increasing the adherence population and consequently the adherent-susceptible and -infectious population. Therefore, causing more infections in the adherence population than in the non-adherent population. Note that even though there are more infections in the adherent population than the non-adherent population, the final epidemic size is still smaller for this scenario relative to that of the γ=0.25 and γa=0.3 scenario for σa=0.75 and σa=0.5, as discussed earlier and shown in the bottom left panel of Figure 4. The epidemic size is the same for both scenario when σa=1.
In Figure 5, we investigate the effect of the indirect transmission rate βv and the shedding rate m for non-adherent population on the dynamics of the disease. When the shedding rate is m=0.02 (left panel of Figure 5), the final epidemic size is 46.66% for βv=0.15, 48.50% for βv=0.35, and 50.25% for βv=0.55. As the shedding rate increases to m=0.125 (right panel of Figure 5), the final epidemic size for βv=0.15 is 53.44%, for βv=0.35 is 62.11%, and 68.87% for βv=0.55. This shows that the final epidemic size increases, as the transmission rate βv increases. When the shedding rate is low, there is not much difference in the epidemic size as the transmission rate βv increases. However, for a higher virus shedding rate or surface contamination rate, there is a significant difference in the final epidemic size as βv increases. This is because when infectious individuals do not shed much virus or contaminate shared surfaces, there will be no disease transmission even if the transmission rate (implicitly Rc) is high. On the other hand, when a lot of shedding or surface contamination is happening, the disease will spread more easily leading to bigger epidemics as βv increases. This results agree with the Rc computation shown in the middle left panel of Figure 2. A similar result was obtained for the shedding ma for the adherent population (result not included).
Next, we study the effect of different initial conditions on the dynamics of the diseases. We consider two main scenarios: when the epidemic starts mainly through direct transmissions and when it starts mainly through indirect transmissions. For the scenario where the epidemic starts through direct transmission, we assume that there are only twenty infected individuals in the population at the beginning of the epidemic; five in the exposed and another five in the infectious compartments of the adherent population. Similar infected population is specified for the non-adherent population, that is, E(0)=5, Ea(0)=5, Ia(0)=5 and I(0)=5, and V(0)=1, while the remaining compartments are empty. On the other hand, when the epidemic starts mainly through indirect transmissions, the initial conditions used are E(0)=1, Ea(0)=1, Ia(0)=1, I(0)=1, and V(0)=10, with the remaining compartments empty. The results for both scenarios are shown in Figure 6 for different values of the movement parameters γ and γa, where the solid curves are for the case where epidemic starts mainly through direct transmissions, and the dashed curves are for when the epidemic starts mainly through indirect transmissions. We observe from these results that for all the cases considered, the total infectious population increases more rapidly when direct transmissions dominate at the beginning of the epidemic, compared to when it starts mainly through indirect transmissions. However, the final epidemic size is the same irrespective of the initial conditions. This result agrees with the Rc computation in the top left panel of Figure 2 that shows that the control reproduction number is more sensitive to the direct transmission rate β compared to the indirect transmission rate βv.
The results in Figure 7 show the effect of the environment cleaning or sanitization rate on the disease dynamics. When βv=0.15, the final epidemic size for τ=0.1 is 53.44%, 51.26% for τ=0.3, and 50.00% when τ=0.5. As the indirect transmission rate increases to βv=0.5, the final epidemic size also increases with 67.33% for τ=0.1, 62.38% for τ=0.3, and 59.23% for τ=0.5. Overall, the final epidemic size decreases as the rate of cleaning/sanitizing the environment increases. When the indirect transmission rate βv is small, there is not much difference in the final epidemic size as τ increases, but for a higher transmission rate βv, an increase in the environment sanitizing rate significantly decreases the epidemic final size. These results are consistent with the Rc computation in the bottom left panel of Figure 2, where there is no significant difference in the control reproduction number as τ increase for a small value of βv, and a significant decrease in Rc as τ increases for a higher indirect transmission rate.
We have developed and analyzed a SEIRV model for studying the dynamics of COVID-19. Our model divides the population into two groups: adherent and non-adherent, and considers both direct and indirect transmission of the disease. Individuals in the adherent population are assumed to adhere to all COVID-19 non-pharmaceutical interventions (NPIs) such as hand washing, physical distancing, wearing face masks, and avoiding large gatherings. In contrast, those in the non-adherent population do not adhere to these NPIs. By adhering to the NPIs, those in the adherent population have reduced their susceptibility, transmissibility and infectiousness. {By direct transmission, we mean disease transmission that occurs when a susceptible individual comes directly in contact with the saliva and other respiratory droplets expelled by an infectious individual when they talk, sing, cough or sneeze}, while indirect transmission refers to the infections that occur through contaminated shared surfaces and objects such as door handles, utensils, etc. Individuals can move from adherent to non-adherent and vice versa at some rate in each human compartments.
We calculated the control reproduction number and final size relation for our model and studied the effect of the model parameters on the calculated control reproduction number. These results are presented as contour plots in Figure 2 and show that the direct transmission rate has more effect on the control reproduction number than the indirect transmission rate. We believe this is because indirect transmission depends on other factors such as shedding rate and environment cleaning rate. Similarly, when we studied Rc with respect to the adherence rate γ and the direct transmission rate β, we notice that adherence to NPIs has little effect on Rc when the transmission rate is small. As the direct transmission rate increases, our results show that adhering to the NPIs would significantly reduce Rc. On other hand, adherence to NPIs is beneficial irrespective of the values of the indirect transmission rate. We also showed that the virus shedding rate has little effect on the control reproduction number if the indirect transmission rate is too small. However, as the transmission rate increases, an increase in shedding rate increases the control reproduction number significantly.
Furthermore, we studied the effect of some model parameters on the disease dynamics. We showed that the disease dynamics is different when there is no movement between adherent and non-adherent population, compared to when the movement between the two groups happens at the same rate. When there is no movement between the groups, there are less infections in the adherent population compared to the non-adherent population as a result of protection from adhering to NPIs. On the contrary, when movement happens between the two groups at the same rate, the level of infection in the two populations is the same, simply because the entire population becomes homogeneous as a result of the movement between adherent and non-adherent population happening at the same rate. When the non-adherence rate is higher than the adherence rate, there are more infections in the non-adherent population relative to the adherent population. In a situation where the adherence rate is higher than the non-adherence rate, the infection in the adherent population is higher compared to the non-adherent population, although, one would have expected the infections in the adherent population to be low because of the protection from adhering to the NPIs. This counterintuitive observation is due to the movement parameters γ and γa, and the parameter σa used to model protection in the adherent population due to NPIs. Observe from the results in Figure 4 that we have more infections in the adherent population only when the rate γ of adhering to COVID-19 NPIs is larger than the non-adherence rate γa. For this scenario, the initial fraction of the total population adhering to the COVID-19 NPIs is larger than the non-adherence population, and as the epidemic continues, we have more people moving to the adherence population that they are becoming non-adherent. Therefore, increasing the adherent susceptible and infected populations, and consequently leading to a larger epidemic in this population compared to the non-adherent population. As the protection derived from adhering to the NPIs increases, fewer adherent individuals are getting infected as shown in the bottom left panel of Figure 4. Overall, even though there are more infections in the adherent population when the rate of movement from non-adherent to adherent is higher than that of moving vice versa, the final epidemic size is smaller for this scenario than the other scenarios. This result shows the benefits of adhering to the COVID-19 NPIs in reducing the epidemic size.
Also, we examined the effect of different initial conditions on the dynamics of the diseases. We considered when the epidemic starts mainly through direct transmissions and when it starts mainly through indirect transmissions. For the scenario where the epidemic starts through direct transmissions, we assume that only twenty infected individuals are in the population at the beginning of the epidemic with a unit of virus on contaminated surfaces. On the other hand, when the epidemic starts mainly through indirect transmissions, we assume that there are only four infected individuals in the population and ten units of viruses on contaminated surfaces at the beginning of the epidemic. These initial conditions are carefully selected to model the dominance of one transmission route over the other at the beginning of the epidemic. Our results show that the epidemic increases more rapidly when it starts mainly through direct transmissions, compared to when it starts through indirect transmissions, although the final epidemic size is the same irrespective of the initial conditions. This result agrees with the result obtained when we studied the effect of the direct and indirect transmission rates on the control reproduction number. Finally, we study the effect of environment sanitization on disease dynamics. Our results show that environmental sanitization reduces the prevalence of COVID-19 and consequently reduces the final epidemic size.
An immediate and interesting future direction in this project is to fit the model to reported cases of COVID-19 in a specific location and use region-specific parameters. By fitting the model to the cases data, important parameters that are not immediately available can be estimated from the data. The model can then be used to make forecasts/predictions and test the effect of implementing different intervention strategies. Another important future direction is to include vaccination compartments into the model. The COVID-19 vaccines became available and have been administered since early 2021. As of July 6, 2021, over 2.9 billion doses of the vaccine have been administered [2]. To properly capture the dynamics of COVID-19 in any population starting from January 2021, vaccination needs to be included in the model. It would be worthwhile to stratify the population by age so that the model can be used to answer age related questions such as those of school reopening and age-specific vaccination plans. There are currently four SARS-CoV-2 variants of concern (VOC) that are believed to be in circulation worldwide. These VOC are believed to be more contagious, leading to more severe sickness than the initial SARS-CoV-2 virus [75]. An interesting extension of our model would be to incorporate these variants of concerns.
Our model is prone to some limitations. This includes our assumptions that the population is well-mixed. We know contact rates vary from one person to another depending on their age group and activity level. Also, mixing patterns are different for individuals of different age groups. Using homogeneous mixing, we assume that everyone in the population has the same contact rate and mixing pattern. Another limitation of our model is that we have clustered all COVID-19 non-pharmaceutical interventions (NPIs) into one group, assuming that any individual that adheres to one of them will adhere to all. This may not be the case in reality. Some individuals may adhere to only a few of the NPIs. It would be nice to distinguish between the NPIs and study the effect of different NPIs on the disease dynamics. Similarly, we have assumed that the rate of movement from adherence to non-adherence and vice versa are constant over time. In a real-world scenario, these rates may change from time to time and may be affected by government policies and their implementation of the NPIs. {Further limitation to our study includes lack of stochasticity. An interesting future work may include adding uncertainties and parameter estimation into this modeling framework.} Despite these limitations, our model can capture the overall dynamics of the COVID-19 epidemic considering infections transmitted through direct and indirect routes, and with and without adherence to the COVID-19 NPIs.
This research is funded by Canada's International Development Research Centre (IDRC) and the Swedish International Development Cooperation Agency (SIDA) (Grant No. 109559-001). J.D. acknowledges that this research was conducted during her maternity leave.
The Authors declare that there is no conflict of interest.
[1] |
R. A. Gatenby, A change of strategy in the war on cancer, Nature, 459 (2009), 508–509. doi: 10.1038/459508a
![]() |
[2] |
R. A. Gatenby, J. Brown, T. Vincent, Lessons from applied ecology: Cancer control using an evolutionary double bind, Cancer Res., 69 (2009), 7499–7502. doi: 10.1158/0008-5472.CAN-09-1354
![]() |
[3] | R. A. Gatenby, A. S. Silva, R. J. Gillies, B. R. Frieden, Adaptive therapy, Cancer Res., 69 (2009), 4894–4903. |
[4] |
K. L. Pogrebniak, C. Curtis, Harnessing tumor evolution to circumvent resistance, Trends Genet., 34 (2018), 639–651. doi: 10.1016/j.tig.2018.05.007
![]() |
[5] |
J. Zhang, J. J. Cunningham, J. S. Brown, R. A. Gatenby, Integrating evolutionary dynamics into treatment of metastatic castrate-resistant prostate cancer, Nat. Commun., 8 (2017), 1816. doi: 10.1038/s41467-017-01968-5
![]() |
[6] |
S. Benzekry, E. Pasquier, D. Barbolosi, B. Lacarelle, F. Barlési, N. André, J. Ciccolini, Metronomic reloaded: Theoretical models bringing chemotherapy into the era of precision medicine, Semin. Cancer Biol., 35 (2015), 53–61. doi: 10.1016/j.semcancer.2015.09.002
![]() |
[7] |
E. Hansen, A. F. Read, Modifying adaptive therapy to enhance competitive suppression, Cancers, 12 (2020), 3556. doi: 10.3390/cancers12123556
![]() |
[8] |
K. Akakura, N. Bruchovsky, S. L. Goldenberg, P. S. Rennie, A. R. Buckley, L. D. Sullivan, Effects of intermittent androgen suppression on androgen-dependent tumors. apoptosis and serum prostate-specific antigen, Cancer, 71 (1993), 2782–2790. doi: 10.1002/1097-0142(19930501)71:9<2782::AID-CNCR2820710916>3.0.CO;2-Z
![]() |
[9] | C. Simsek, E. Esin, S. Yalcin, Metronomic chemotherapy: A systematic review of the literature and clinical experience, J. Oncol., 2019 (2019), 1–31. |
[10] |
A. Konstorum, T. Hillen, J. Lowengrub, Feedback regulation in a cancer stem cell model can cause an allee effect, Bull. Math. Biol., 78 (2016), 754–785. doi: 10.1007/s11538-016-0161-5
![]() |
[11] |
J. West, Y. Ma, P. K. Newton, Capitalizing on competition: An evolutionary model of competitive release in metastatic castration resistant prostate cancer treatment, J. Theor. Biol., 455 (2018), 249–260. doi: 10.1016/j.jtbi.2018.07.028
![]() |
[12] |
R. D. Holt, Predation, apparent competition and the structure of prey communities, Theor. Popul. Biol., 12 (1977), 197–229. doi: 10.1016/0040-5809(77)90042-9
![]() |
[13] |
E. Piretto, M. Delitala, M. Ferraro, Combination therapies and intra-tumoral competition: Insights from mathematical modeling, J. Theor. Biol., 446 (2018), 149–159. doi: 10.1016/j.jtbi.2018.03.014
![]() |
[14] |
H. Schättler, U. Ledzewicz, B. Amini, Dynamical properties of a minimally parameterized mathematical model for metronomic chemotherapy, J. Math. Biol., 72 (2016), 1255–1280. doi: 10.1007/s00285-015-0907-y
![]() |
[15] |
A. M. Ideta, G. Tanaka, T. Takeuchi, K. Aihara, A mathematical model of intermittent androgen suppression for prostate cancer, J. Nonlinear Sci., 18 (2008), 593. doi: 10.1007/s00332-008-9031-0
![]() |
[16] |
E. M. Rutter, Y. Kuang, Global dynamics of a model of joint hormone treatment with dendritic cell vaccine for prostate cancer, Discrete Contin. Dyn. Syst. B, 22 (2017), 1001–1021. doi: 10.3934/dcdsb.2017050
![]() |
[17] |
A. Zazoua, W. Wang, Analysis of mathematical model of prostate cancer with androgen deprivation therapy, Commun. Nonlinear Sci. Numer. Simul., 66 (2019), 41–60. doi: 10.1016/j.cnsns.2018.06.004
![]() |
[18] |
J. Baez, Y. Kuang, Mathematical models of androgen resistance in prostate cancer patients under intermittent androgen suppression therapy, Appl. Sci., 6 (2016), 352. doi: 10.3390/app6110352
![]() |
[19] |
H. V. Jain, S. K. Clinton, A. Bhinder, A. Friedman, Mathematical modeling of prostate cancer progression in response to androgen ablation therapy, Proc. Natl. Acad. Sci. USA, 108 (2011), 19701–19706. doi: 10.1073/pnas.1115750108
![]() |
[20] |
T. Portz, Y. Kuang, J. D. Nagy, A clinical data validated mathematical model of prostate cancer growth under intermittent androgen suppression therapy, AIP Adv., 2 (2012), 011002. doi: 10.1063/1.3697848
![]() |
[21] |
J. J. Cunningham, J. S. Brown, R. A. Gatenby, K. Staňková, Optimal control to develop therapeutic strategies for metastatic castrate resistant prostate cancer, J. Theor. Biol., 459 (2018), 67–78. doi: 10.1016/j.jtbi.2018.09.022
![]() |
[22] |
P. F. Sale, Maintenance of high diversity in coral reef fish communities, Am. Nat., 111 (1977), 337–359. doi: 10.1086/283164
![]() |
[23] |
R. A. Armstrong, R. McGehee, Competitive exclusion, Am. Nat., 115 (1980), 151–170. doi: 10.1086/283553
![]() |
[24] |
L. Schiffer, W. Arlt, K.-H. Storbeck, Intracrine androgen biosynthesis, metabolism and action revisited, Mol. Cell. Endocrinol., 465 (2018), 4–26. doi: 10.1016/j.mce.2017.08.016
![]() |
[25] |
Z. Zhu, Y.-M. Chung, O. Sergeeva, V. Kepe, M. Berk, J. Li, H.-K. Ko, Z. Li, M. Petro, F. P. DiFilippo et al., Loss of dihydrotestosterone-inactivation activity promotes prostate cancer castration resistance detectable by functional imaging, J. Biol. Chem., 293 (2018), 17829–17837. doi: 10.1074/jbc.RA118.004846
![]() |
[26] |
W. P. Harris, E. A. Mostaghel, P. S. Nelson, B. Montgomery, Androgen deprivation therapy: progress in understanding mechanisms of resistance and optimizing androgen depletion, Nat. Clin. Pract. Urol., 6 (2009), 76–85. doi: 10.1038/ncpuro1296
![]() |
[27] |
D. L. Suzman, E. S. Antonarakis, Does degree of androgen suppression matter in hormone-sensitive prostate cancer?, J. Clin. Oncol., 33 (2015), 1098–1100. doi: 10.1200/JCO.2014.60.1419
![]() |
[28] | K. E. Soetaert, T. Petzoldt, R. W. Setzer, Solving differential equations in R: package deSolve, J. Stat. Softw., 33 (2010), 1–25. |
[29] |
K. Bacevic, R. Noble, A. Soffar, O. W. Ammar, B. Boszonyik, S. Prieto, C. Vincent, M. E. Hochberg, L. Krasinska, D. Fisher, Spatial competition constrains resistance to targeted cancer therapy, Nat. Commun., 8 (2017), 1995. doi: 10.1038/s41467-017-01516-1
![]() |
[30] |
J. A. Gallaher, P. M. Enriquez-Navas, K. A. Luddy, R. A. Gatenby, A. R. Anderson, Spatial heterogeneity and evolutionary dynamics modulate time to recurrence in continuous and adaptive cancer therapies, Cancer Res., 78 (2018), 2127–2139. doi: 10.1158/0008-5472.CAN-17-2649
![]() |
[31] |
A. B. Shah, K. A. Rejniak, J. L. Gevertz, Limiting the development of anti-cancer drug resistance in a spatial model of micrometastases, Math. Biosci. Eng., 13 (2016), 1185–1206. doi: 10.3934/mbe.2016038
![]() |
[32] |
M. S. Feizabadi, Modeling multi-mutation and drug resistance: analysis of some case studies, Theor. Biol. Med. Mod., 14 (2017), 6. doi: 10.1186/s12976-017-0052-y
![]() |
[33] |
Y. Hirata, N. Bruchovsky, K. Aihara, Development of a mathematical model that predicts the outcome of hormone therapy for prostate cancer, J. Theor. Biol., 264 (2010), 517–527. doi: 10.1016/j.jtbi.2010.02.027
![]() |
[34] |
R. Salgia, P. Kulkarni, The genetic/non-genetic duality of drug 'resistance'in cancer, Trends Cancer, 4 (2018), 110–118. doi: 10.1016/j.trecan.2018.01.001
![]() |
[35] |
J. West, P. K. Newton, Cellular interactions constrain tumor growth, Proc. Natl. Acad. Sci. USA, 116 (2019), 1918–1923. doi: 10.1073/pnas.1804150116
![]() |
[36] |
A. Ballesta, J. Clairambault, Physiologically based mathematical models to optimize therapies against metastatic colorectal cancer: a mini-review, Curr. Pharm. Design, 20 (2014), 37–48. doi: 10.2174/13816128113199990553
![]() |
[37] |
G. Aguadé-Gorgorió, R. Solé, Adaptive dynamics of unstable cancer populations: The canonical equation, Evol. Appl., 11 (2018), 1283–1292. doi: 10.1111/eva.12625
![]() |
[38] |
A. Arabameri, D. Asemani, J. Hadjati, A structural methodology for modeling immune-tumor interactions including pro-and anti-tumor factors for clinical applications, Math. Biosci., 304 (2018), 48–61. doi: 10.1016/j.mbs.2018.07.006
![]() |
[39] |
M. Robertson-Tessi, A. El-Kareh, A. Goriely, A mathematical model of tumor–immune interactions, J. Theor. Biol., 294 (2012), 56–73. doi: 10.1016/j.jtbi.2011.10.027
![]() |
[40] |
A. Lorz, T. Lorenzi, M. E. Hochberg, J. Clairambault, B. Perthame, Populational adaptive evolution, chemotherapeutic resistance and multiple anti-cancer therapies, ESAIM: Math. Model. Num., 47 (2013), 377–399. doi: 10.1051/m2an/2012031
![]() |
[41] |
A. S. Silva, R. A. Gatenby, A theoretical quantitative model for evolution of cancer chemotherapy resistance, Biol. Direct, 5 (2010), 25. doi: 10.1186/1745-6150-5-25
![]() |
[42] |
J. West, L. You, J. Zhang, R. A. Gatenby, J. S. Brown, P. K. Newton, A. R. Anderson, Towards multi-drug adaptive therapy, Cancer Res., 80 (2020), 1578–1589. doi: 10.1158/0008-5472.CAN-19-2669
![]() |
[43] |
J. B. West, M. N. Dinh, J. S. Brown, J. Zhang, A. R. Anderson, R. A. Gatenby, Multidrug cancer therapy in metastatic castrate-resistant prostate cancer: An evolution-based strategy, Clin. Cancer Res., 25 (2019), 4413–4421. doi: 10.1158/1078-0432.CCR-19-0006
![]() |
[44] | J. L. Gevertz, J. R. Wares, Developing a minimally structured mathematical model of cancer treatment with oncolytic viruses and dendritic cell injections, Comput. Math. Methods Med., 2018 (2018), 8760371. |
[45] |
A. Kaznatcheev, J. Peacock, D. Basanta, A. Marusyk, J. G. Scott, Fibroblasts and Alectinib switch the evolutionary games played by non-small cell lung cancer, Nat. Ecol. Evol., 3 (2019), 450–456. doi: 10.1038/s41559-018-0768-z
![]() |
[46] | M. Gluzman, J. G. Scott, A. Vladimirsky, Optimizing adaptive cancer therapy: dynamic programming and evolutionary game theory, arXiv preprint arXiv: 1812.01805. |
[47] |
Y. Hirata, K. Morino, K. Akakura, C. S. Higano, K. Aihara, Personalizing androgen suppression for prostate cancer using mathematical modeling, Sci. Rep., 8 (2018), 2673. doi: 10.1038/s41598-018-20788-1
![]() |
[48] |
Y. Viossat, R. Noble, A theoretical analysis of tumour containment, Nat. Ecol. Evol., 5 (2021), 826–835. doi: 10.1038/s41559-021-01428-w
![]() |
[49] |
F. F. Teles, J. M. Lemos, Cancer therapy optimization based on multiple model adaptive control, Biomed. Signal Process. Control, 48 (2019), 255–264. doi: 10.1016/j.bspc.2018.09.016
![]() |
[50] |
U. Ledzewicz, S. Wang, H. Schättler, N. André, M. A. Heng, E. Pasquier, On drug resistance and metronomic chemotherapy: A mathematical modeling and optimal control approach, Math. Biosci. Eng., 14 (2017), 217–235. doi: 10.3934/mbe.2017014
![]() |
[51] |
A. Alvarez-Arenas, K. E. Starkov, G. F. Calvo, J. Belmonte-Beitia, Ultimate dynamics and optimal control of a multi-compartment model of tumor resistance to chemotherapy, Discrete Contin. Dyn. Syst. B, 24 (2019), 2017–2038. doi: 10.3934/dcdsb.2019082
![]() |
[52] | C. Cockrell, D. E. Axelrod, Optimization of dose schedules for chemotherapy of early colon cancer determined by high-performance computer simulations, Cancer Inform., 18 (2019), 1176935118822804. |
[53] | K. Normilio-Silva, A. C. de Figueiredo, A. C. Pedroso-de Lima, G. Tunes-da Silva, A. Nunes da Silva, A. Delgado Dias Levites, A. T. de Simone, P. Lopes Safra, R. Zancani, P. C. Tonini et al., Long-term survival, quality of life, and quality-adjusted survival in critically ill patients with cancer, Crit. Care Med., 44 (2016), 1327–1337. |
[54] |
T. Hatano, Y. Hirata, H. Suzuki, K. Aihara, Comparison between mathematical models of intermittent androgen suppression for prostate cancer, J. Theor. Biol., 366 (2015), 33–45. doi: 10.1016/j.jtbi.2014.10.034
![]() |
[55] | J. I. Griffiths, P. Wallet, L. T. Pflieger, D. Stenehjem, X. Liu, P. A. Cosgrove, N. A. Leggett, J. A. McQuerry, G. Shrestha, M. Rosetti, G. Sunga, P. J. Moos, F. R. Adler, J. T. Chang, S. Sharma, A. Bild, Circulating immune cell phenotype dynamics reflect the strength of tumor-immune cell interactions in patients during immunotherapy, Proc. Natl. Acad. Sci. USA, in press. |
[56] |
R. A. Beckman, G. S. Schemmann, C.-H. Yeang, Impact of genetic dynamics and single-cell heterogeneity on development of nonstandard personalized medicine strategies for cancer, Proc. Natl. Acad. Sci. USA, 109 (2012), 14586–14591. doi: 10.1073/pnas.1203559109
![]() |
[57] |
K. Staňková, J. S. Brown, W. S. Dalton, R. A. Gatenby, Optimizing cancer treatment using game theory: A review, JAMA Oncol., 5 (2019), 96–103. doi: 10.1001/jamaoncol.2018.3395
![]() |
1. | Avery Meiksin, Using the SEIR model to constrain the role of contaminated fomites in spreading an epidemic: An application to COVID-19 in the UK, 2022, 19, 1551-0018, 3564, 10.3934/mbe.2022164 | |
2. | Joel-Pascal Ntwali N'konzi, Chidozie Williams Chukwu, Farai Nyabadza, Effect of time-varying adherence to non-pharmaceutical interventions on the occurrence of multiple epidemic waves: A modeling study, 2022, 10, 2296-2565, 10.3389/fpubh.2022.1087683 | |
3. | Bin-Guo Wang, Zhi-Cheng Wang, Yan Wu, Yongping Xiong, Jiangqian Zhang, Zhuihui Ma, A mathematical model reveals the influence of NPIs and vaccination on SARS-CoV-2 Omicron Variant, 2023, 111, 0924-090X, 3937, 10.1007/s11071-022-07985-4 | |
4. | Ammar Al-Bazi, Faris Madi, Anees Abu Monshar, Yousif Eliya, Tunde Adediran, Khaled Al Khudir, Modelling the impact of non-pharmaceutical interventions on COVID-19 exposure in closed-environments using agent-based modelling, 2023, 2047-9700, 1, 10.1080/20479700.2023.2189555 | |
5. | Kolapo M Oyebola, Funmilayo C Ligali, Afolabi J Owoloye, Blessing D Erinwusi, Adesola Z Musa, Oluwagbemiga O Aina, Babatunde L Salako, Machine learning insights on the effectiveness of non-pharmaceutical interventions against COVID-19 in Nigeria, 2025, 1876-3413, 10.1093/inthealth/ihae065 | |
6. | Gabriel McCarthy, Hana M. Dobrovolny, Determining the best mathematical model for implementation of non-pharmaceutical interventions, 2025, 22, 1551-0018, 700, 10.3934/mbe.2025026 |
Variable | Description |
S | Non-adherent susceptible population |
E | Non-adherent exposed population |
I | Non-adherent infectious population |
R | Non-adherent recovered population |
Sa | Adherent susceptible population |
Ea | Adherent exposed population |
Ia | Adherent infectious population |
Ra | Adherent recovered population |
V | Virus compartment |
Parameter | Description | Value | Reference |
N | Total population | 5,000,000 | Assumed |
σa | Reduction in virus shedding, susceptibility, and onward transmission of disease due to adherence to NPIs | Varied | |
β | Direct transmission rate for non-adherence population | 0.5 | [25,35,49] |
βv | Indirect transmission rate for non-adherence population | 0.15 | [35,36] |
βa | Direct transmission rate for adherence population | σaβ | Derived |
βav | Indirect transmission rate for adherence population | σaβv | Derived |
γ | Rate of movement from non-adherent to adherent | 0.3 day−1 | [25,50,51,52] |
γa | Rate of movement from adherent to non-adherent | 0.25 day−1 | [50,51,52] |
m | Virus shedding rate or the rate of contamination of surfaces by infectious individuals in non-adherent population | 0.125 ml−1 person−1 day−1 | [35,53,54] |
ma | Virus shedding rate or the rate of contamination of surfaces by infectious individuals in the adherent population | σam | Derived |
δ | Decay rate of virus in the environment | 0.4 day−1 | [55,56,57,58,59] |
τ | Environment cleaning/sanitization rate | 0.1 day−1 | Inferred |
r | Recovery rate (1/r is the infectious period) | 1/5 day−1 | [25,39,60,61,62,63] |
μ | Rate of transitioning from the exposed to infectious compartments (1/μ is the incubation period) | 1/6 day−1 | [39,40,41,63,64] |
Variable | Description |
S | Non-adherent susceptible population |
E | Non-adherent exposed population |
I | Non-adherent infectious population |
R | Non-adherent recovered population |
Sa | Adherent susceptible population |
Ea | Adherent exposed population |
Ia | Adherent infectious population |
Ra | Adherent recovered population |
V | Virus compartment |
Parameter | Description | Value | Reference |
N | Total population | 5,000,000 | Assumed |
σa | Reduction in virus shedding, susceptibility, and onward transmission of disease due to adherence to NPIs | Varied | |
β | Direct transmission rate for non-adherence population | 0.5 | [25,35,49] |
βv | Indirect transmission rate for non-adherence population | 0.15 | [35,36] |
βa | Direct transmission rate for adherence population | σaβ | Derived |
βav | Indirect transmission rate for adherence population | σaβv | Derived |
γ | Rate of movement from non-adherent to adherent | 0.3 day−1 | [25,50,51,52] |
γa | Rate of movement from adherent to non-adherent | 0.25 day−1 | [50,51,52] |
m | Virus shedding rate or the rate of contamination of surfaces by infectious individuals in non-adherent population | 0.125 ml−1 person−1 day−1 | [35,53,54] |
ma | Virus shedding rate or the rate of contamination of surfaces by infectious individuals in the adherent population | σam | Derived |
δ | Decay rate of virus in the environment | 0.4 day−1 | [55,56,57,58,59] |
τ | Environment cleaning/sanitization rate | 0.1 day−1 | Inferred |
r | Recovery rate (1/r is the infectious period) | 1/5 day−1 | [25,39,60,61,62,63] |
μ | Rate of transitioning from the exposed to infectious compartments (1/μ is the incubation period) | 1/6 day−1 | [39,40,41,63,64] |