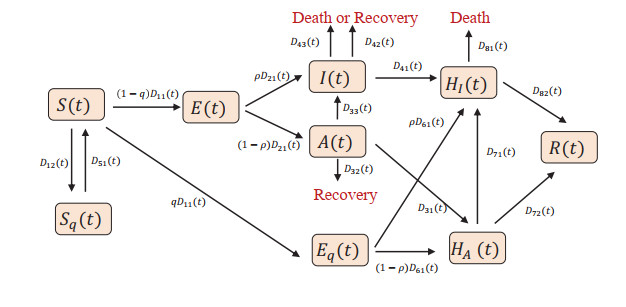
After a major outbreak of the coronavirus disease (COVID-19) starting in late December 2019, there were no new cases reported in mainland China for the first time on March 18, 2020, and no new cases reported in Hong Kong Special Administrative Region on April 20, 2020. However, these places had reported new cases and experienced a second wave since June 11, 2020. Here we develop a stochastic discrete-time epidemic model to evaluate the risk of COVID-19 resurgence by analyzing the data from the beginning of the outbreak to the second wave in these three places. In the model, we use an input parameter to represent a few potential risks that may cause a second wave, including asymptomatic infection, imported cases from other places, and virus from the environment such as frozen food packages. The effect of physical distancing restrictions imposed at different stages of the outbreak is also included in the model. Model simulations show that the magnitude of the input and the time between the initial entry and subsequent case confirmation significantly affect the probability of the second wave occurrence. Although the susceptible population size does not change the probability of resurgence, it can influence the severity of the outbreak when a second wave occurs. Therefore, to prevent the occurrence of a future wave, timely screening and detection are needed to identify infected cases in the early stage of infection. When infected cases appear, various measures such as contact tracing and quarantine should be followed to reduce the size of susceptible population in order to mitigate the COVID-19 outbreak.
Citation: Sha He, Jie Yang, Mengqi He, Dingding Yan, Sanyi Tang, Libin Rong. The risk of future waves of COVID-19: modeling and data analysis[J]. Mathematical Biosciences and Engineering, 2021, 18(5): 5409-5426. doi: 10.3934/mbe.2021274
[1] | Ryo Kinoshita, Sung-mok Jung, Tetsuro Kobayashi, Andrei R. Akhmetzhanov, Hiroshi Nishiura . Epidemiology of coronavirus disease 2019 (COVID-19) in Japan during the first and second waves. Mathematical Biosciences and Engineering, 2022, 19(6): 6088-6101. doi: 10.3934/mbe.2022284 |
[2] | Avinash Shankaranarayanan, Hsiu-Chuan Wei . Mathematical modeling of SARS-nCoV-2 virus in Tamil Nadu, South India. Mathematical Biosciences and Engineering, 2022, 19(11): 11324-11344. doi: 10.3934/mbe.2022527 |
[3] | Yukun Tan, Durward Cator III, Martial Ndeffo-Mbah, Ulisses Braga-Neto . A stochastic metapopulation state-space approach to modeling and estimating COVID-19 spread. Mathematical Biosciences and Engineering, 2021, 18(6): 7685-7710. doi: 10.3934/mbe.2021381 |
[4] | Christian Costris-Vas, Elissa J. Schwartz, Robert Smith? . Predicting COVID-19 using past pandemics as a guide: how reliable were mathematical models then, and how reliable will they be now?. Mathematical Biosciences and Engineering, 2020, 17(6): 7502-7518. doi: 10.3934/mbe.2020383 |
[5] | Qian Shen . Research of mortality risk prediction based on hospital admission data for COVID-19 patients. Mathematical Biosciences and Engineering, 2023, 20(3): 5333-5351. doi: 10.3934/mbe.2023247 |
[6] | Antonios Armaou, Bryce Katch, Lucia Russo, Constantinos Siettos . Designing social distancing policies for the COVID-19 pandemic: A probabilistic model predictive control approach. Mathematical Biosciences and Engineering, 2022, 19(9): 8804-8832. doi: 10.3934/mbe.2022409 |
[7] | Damilola Olabode, Jordan Culp, Allison Fisher, Angela Tower, Dylan Hull-Nye, Xueying Wang . Deterministic and stochastic models for the epidemic dynamics of COVID-19 in Wuhan, China. Mathematical Biosciences and Engineering, 2021, 18(1): 950-967. doi: 10.3934/mbe.2021050 |
[8] | Enahoro A. Iboi, Oluwaseun Sharomi, Calistus N. Ngonghala, Abba B. Gumel . Mathematical modeling and analysis of COVID-19 pandemic in Nigeria. Mathematical Biosciences and Engineering, 2020, 17(6): 7192-7220. doi: 10.3934/mbe.2020369 |
[9] | Xinyu Bai, Shaojuan Ma . Stochastic dynamical behavior of COVID-19 model based on secondary vaccination. Mathematical Biosciences and Engineering, 2023, 20(2): 2980-2997. doi: 10.3934/mbe.2023141 |
[10] | Hamdy M. Youssef, Najat A. Alghamdi, Magdy A. Ezzat, Alaa A. El-Bary, Ahmed M. Shawky . A new dynamical modeling SEIR with global analysis applied to the real data of spreading COVID-19 in Saudi Arabia. Mathematical Biosciences and Engineering, 2020, 17(6): 7018-7044. doi: 10.3934/mbe.2020362 |
After a major outbreak of the coronavirus disease (COVID-19) starting in late December 2019, there were no new cases reported in mainland China for the first time on March 18, 2020, and no new cases reported in Hong Kong Special Administrative Region on April 20, 2020. However, these places had reported new cases and experienced a second wave since June 11, 2020. Here we develop a stochastic discrete-time epidemic model to evaluate the risk of COVID-19 resurgence by analyzing the data from the beginning of the outbreak to the second wave in these three places. In the model, we use an input parameter to represent a few potential risks that may cause a second wave, including asymptomatic infection, imported cases from other places, and virus from the environment such as frozen food packages. The effect of physical distancing restrictions imposed at different stages of the outbreak is also included in the model. Model simulations show that the magnitude of the input and the time between the initial entry and subsequent case confirmation significantly affect the probability of the second wave occurrence. Although the susceptible population size does not change the probability of resurgence, it can influence the severity of the outbreak when a second wave occurs. Therefore, to prevent the occurrence of a future wave, timely screening and detection are needed to identify infected cases in the early stage of infection. When infected cases appear, various measures such as contact tracing and quarantine should be followed to reduce the size of susceptible population in order to mitigate the COVID-19 outbreak.
The novel coronavirus SARS-CoV-2 has caused a global pandemic of unprecedented viral pneumonia [1,2]. This infection is known as coronavirus disease 2019 (COVID-19) [3]. Because of the high human-to-human transmissibility, SARS-CoV-2 has spread rapidly around the world [4,5,6,7]. In mainland China, the outbreak started in December 2019, reached the peak in February and then the number of new confirmed cases decreased. On March 18, 2020, there were no new cases of infection for the first time and the economy and daily life gradually returned to normal [8,9,10,11]. In Hong Kong Special Administrative Region (SAR), the first case was reported on January 18, 2020. The outbreak peaked in late March 2020 with no new confirmed cases on April 23, 2020. However, spread of COVID-19 in the world is continuing and the outbreak is ongoing globally [12,13]. The infection has been confirmed in about 190 countries up to now. As of February 21, 2021, there have been over 110.6 million cases and 2.45 million deaths reported globally since the beginning of the pandemic. The European Region has the largest new cases and new deaths. The United States accounts for the greatest proportion of cumulative cases and deaths [14]. In China, although the epidemic has been under control, confirmed cases have been found occasionally in different places, which raised significant concerns on the resurgence of future waves of COVID-19.
If there are no confirmed cases in a region for a long time, then the risk of disease re-emergence might be mainly from imported cases or viruses. The major COVID-19 transmission pathway is from human to human through respiratory droplets [15,16]. In particular, asymptomatic individuals who do not have COVID-19 symptoms can still spread the virus. Transmission from asymptomatic individuals poses a significant public health challenge [17,18,19,20,21,22,23]. The cases imported from other high-risk places are another path of viral spread. To reduce the potential of imported cases, many countries have issued travel restrictions, for example, reducing the frequency of flights from abroad [24,25]. However, as the infection is still prevailing in many places, imported cases still represent a tremendous risk, which may lead to new local outbreaks [26,27,28,29]. Another possible path of SARS-CoV-2 transmission might be through the food supply chain, surfaces and environment. In China, the coronavirus was detected on frozen foods, including their packaging materials and storage environment in July 2020. There seemed to have two outbreaks related to the transmission via frozen food [30]. In view of this, interventions that reduce foodborne transmission of pathogens need to be considered [32].
Non-pharmaceutical control measures implemented so far are mainly wearing mask, hand washing, social distancing, quarantine and city/region lockdown [31]. These interventions were gradually lifted in consideration of the trade-off between economic sustainability and public health. An agent-based model was developed to evaluate the possibility of a second-wave emergence under different extents and timing of intervention relaxation [32]. More work assessed the risk of secondary waves since the control measures like lockdowns were relaxed [33,34,35,36,37,38,39,40]. The study [41] compared the data of the epidemiological pattern of COVID-19 in 53 countries or regions where the pandemic experienced two waves, and analyzed the differences between the two outbreaks. Their results suggested that there was a shift of infection to younger age groups, which may make it more difficult to control the pandemic.
In this work, we focus on the COVID-19 spread in serval places where the epidemic has been under control but new cases have been reported occasionally. To study the impact of imported cases on the dynamics of COVID-19 in China under different scenarios of prevention and control measures, Jia et al. developed an impulsive epidemic model to describe imported cases from abroad [42]. In their model, the time when the exposed cases were imported was fixed. However, the exposed cases who carry virus without symptoms are usually unknown. When an infected case is identified, the virus has probably been spreading for a period of time. In the beginning of a new wave of epidemic, the infection might be induced by a small number of infected cases. The disease transmission in this stage can be affected by many random factors. In addition, the data of new/accumulated cases were reported on every day. All of these motivate us to develop a stochastic discrete-time compartmental model that considers randomness, epidemic data, as well as the impact of input virus/cases and the initial entry time. By fitting the model to the two waves of outbreaks in two places in mainland China (Beijing and Xinjiang) and Hong Kong SAR, we evaluate the risk factors that can affect the second or future wave of COVID-19.
We develop a stochastic discrete-time model based on the classic compartmental model. Individuals who have no clinical manifestations such as fever, cough, sore throat and other symptoms that can be self-perceived or clinically recognized, but test positive in serological or blood test are referred to as asymptomatic infection. This population includes two types of individuals. One is asymptomatic infection in the incubation period. They will later develop clinical symptoms or become a confirmed case by screening test or CT (Computed Tomography) examination. The other has no symptoms until the nucleic acid test turns negative. The total population is divided into five epidemiological classes, including susceptible (S), exposed (E), asymptomatically infected (A), symptomatically infected (I), and recovered (R). Due to quarantine, the susceptible and exposed states are further divided into Sq and Eq. With hospitalization, the infected class (both asymptomatic and symptomatic) can be further divided into HA and HI. Because the infection and disease progression can be affected by random factors, we assume that the flow between any two compartments is a stochastic process [43,44,45]. For example, D11(t) is the number of susceptible individuals who become newly infected and this process obeys a binomial distribution. The diagram of the model is shown in Figure 1 and the corresponding stochastic discrete-time model is given by the following system:
St+1=St−D11(t)−D12(t)+D51(t),Et+1=Et+(1−q)D11(t)−D21(t),At+1=At+(1−ρ)D21(t)−D31(t)−D32(t)−D33(t),It+1=It+ρD21(t)−D41(t)−D42(t)−D43(t)+D33(t),Sqt+1=Sqt+D12(t)−D51(t),Eqt+1=Eqt+qD11(t)−D61(t),HAt+1=HAt+D31(t)+(1−ρ)D61(t)−D71(t)−D72(t),HIt+1=HIt+D41(t)+ρD61(t)+D71(t)−D81(t)−D82(t),Rt+1=Rt+D32(t)+D42(t)+D72(t)+D82(t), | (2.1) |
where Dij(t) obeys a binomial distribution Bin(n,p) with the parameters (n,p), and the specific form is as follows
D11(t)∼Bin(St,P11(t)), D12(t)∼Bin(St,P12(t)), D21(t)∼Bin(Et,P21),D31(t)∼Bin(At,P31(t)), D32(t)∼Bin(At,P32), D33(t)∼Bin(At,P33),D41(t)∼Bin(It,P41), D42(t)∼Bin(It,P42), D43(t)∼Bin(It,P43),D51(t)∼Bin(Sqt,P51), D61(t)∼Bin(Eqt,P61), D71(t)∼Bin(HAt,P33),D72(t)∼Bin(HAt,P32), D81(t)∼Bin(HIt,P43) D82(t)∼Bin(HIt,P42). |
P11(t)=1−exp[−βc(t)(I+θA)N], P12(t)=1−exp[−(1−β)qc(t)(I+θA)N],P21=1−exp(−σ), P31=1−exp(−δA),P32(t)=1−exp(−γA), P33=1−exp(−k),P41=1−exp(−δI), P42=1−exp(−γI),P51=1−exp(−λ), P61=1−exp(−δq). |
Here exp[−βc(t)(I+θA)Nh] is the probability of staying in the compartment S. The time period h is chosen to be one, so it is omitted in the expression. Thus, P11 is the probability of individuals leaving the susceptible compartment. The other P functions can be explained in a similar way. The meaning of each parameter in the model is summarized in Table 1.
Parameters | Definition | Values | Source | |||
Beijing | Xinjiang | Hong Kong SAR | ||||
c(t) | c0 | Contact rate at the initial time | 17.0142 | 14.0025 | 11.9175 | Estimated |
cb | Minimum contact rate under the most strict control strategies | 1.0869 | 2.0468 | 3.0460 | Estimated | |
r1 | Exponential decreasing rate of contact rate in the first period | 0.2196 | 0.2049 | 0.0703 | Estimated | |
r2 | Exponential increasing rate of contact rate in the second period | 0.0506 | 0.0200 | 0.0324 | Estimated | |
r3 | Exponential decreasing rate of contact rate in the third period | 0.2241 | 0.3096 | 0.1445 | Estimated | |
β | Probability of transmission per contact | 0.2801 | 0.2722 | 0.1504 | Estimated | |
q(t) | q0 | Quarantined rate at the initial time | – | 0.2820 | – | Estimated |
qm | Maximum quarantined rate with control strategies | – | 0.7083 | – | Estimated | |
r4 | Exponential increasing rate of quarantined rate in the first period | – | 0.2027 | – | Estimated | |
r5 | Exponential decreasing rate of quarantined rate in the second period | – | 0.1010 | – | Estimated | |
r6 | Exponential increasing rate of quarantined rate in the third period | – | 0.2028 | – | Estimated | |
q | Quarantined rate | 0.2935 | – | 0.4866 | Estimated | |
ρ | Ratio of symptomatic infection | 0.5142 | 0.5520 | 0.5529 | Estimated | |
σ | Transition rate of exposed individuals to the infected class | 1/5 | 1/5 | 1/5 | [47] | |
λ | Rate at which the quarantined uninfected contacts were released into the wider community | 1/14 | 1/14 | 1/14 | [47] | |
δI | Constant transition rate of symptomatic infected | 0.3474 | 0.2008 | 0.0999 | Estimated | |
δA | Constant transition rate of asymptomatic infected | 0.2860 | 0.2006 | 0.3027 | Estimated | |
δq | Constant transition rate of quarantined exposed | 0.3599 | 0.2816 | 0.2571 | Estimated | |
θ | Correction factor for transmission probability of asymptomatic infectious | 0.5919 | 0.5031 | 0.5041 | Estimated | |
k | Conversion rate from asymptomatic infected to symptomatic infected | 0.6124 | 0.6221 | 0.5026 | Estimated | |
γI | Recovery rate of infected individuals | 0.0701 | 0.1632 | 0.0799 | Estimated | |
γA | Recovery rate of asymptotic infected individuals | 0.0906 | 0.1629 | 0.2393 | Estimated | |
τ | The time of importation of the first case in the second wave | 17 | 16 | – | Estimated | |
pE(T2−τ) | The number of exposed cases entered at the time T2−τ in the second wave | 6 | 7.02 | – | Estimated | |
α | Disease-induced death rate | 0 | 0 | 0 | Assumed | |
– means the parameter is not included in that place. |
Due to limited pharmaceutical interventions, wearing mask and social distancing play a critical role in the control of the COVID-19 pandemic. As the epidemic is gradually controlled, people's vigilance will decrease. Strict intervention measures may have to be lifted because of economic consideration. We use a time-varying function for the contact rate to describe this change. When the pandemic began and spread rapidly, control measures such as city lockdown, wearing masks and social activity reduction greatly reduced the contact between people. We denote the time of strict control implementation by T0. When the number of infected cases gradually decrease after the peak, the control measures are relaxed and people's lives gradually return to normal. We denote this time by T1. When new cases are reported again, people's vigilance increases, and prevention and control measures are implemented again. We denote this time by T2. The following time-varying function for the contact rate c(t) is used to describe the change of human behavior and effect of control measures during the epidemic.
c(t)={c0,t<T0,(c0−cb)e−r1(t−T0)+cb,T0≤t<T1,(cb1−c0)e−r2(t−T1)+c0,T1≤t<T2,(c01−cb)e−r3(t−T2)+cb,t≥T2. | (2.2) |
Here cb1=(c0−cb)e−r1(T1−T0)+cb and c01=(cb−c0)e−r2(T2−T1)+c0.
We define the quarantine rate q(t) in a similar way. The quarantine rate increases as the epidemic gets worse and decreases as it improves. Thus, we assume that the quarantine rate is a time-dependent piecewise function, given by
q(t)={q0,t<T0,(q0−qm)e−r4(t−T0)+qm,T0≤t<T1,(qm1−q0)e−r5(t−T1)+q0,T1≤t<T2,(q01−qm)e−r6(t−T2)+qm,t≥T2, | (2.3) |
where qm1=(q0−qm)e−r4(T1−T0)+qm and q01=(qm−q0)e−r5(T2−T1)+q0. The functions c(t) and q(t) are shown in Figure 2(a–b), Figure 3(e–f) and Figure 4(a–b) for three different places.
We collected the data of Beijing and Xinjiang from the local health commissions in mainland China, and the data of Hong Kong SAR from the Centre for Health Protection. It includes the time series data of confirmed COVID-19 cases, recovered cases, and asymptomatic coronavirus carriers. On December 26, 2019, a respiratory and critical care physician in Wuhan reported the pneumonia of unknown cause for the first time. The epidemic then spread rapidly in mainland China, and the number of newly confirmed cases reached the peak on February 4, 2020. As of March 18, the number of newly confirmed cases in mainland China became 0 and the number of confirmed cases fell below 20,000. After that, the reported cases in mainland China were mainly imported cases. A few months later, infected cases began to rise again in some places. On June 11, 2020, a confirmed case was reported in Beijing, without history of traveling outside Beijing and without close contact with suspected infection in the past two weeks. This ended a 56-day streak of no local infection in Beijing. On July 15, 2020, i.e., 149 days since the previous confirmed cases, one confirmed case and three asymptomatic cases were found in Xinjiang. In Hong Kong SAR, there were sporadic confirmed cases after April 20. On July 5, a second-wave outbreak emerged. This paper will focus on the data from these three places to study the risk of the emergence of a future wave of COVID-19. The switching time T0, T1 and T2 in the piecewise function are determined by the responding time in each place.
If there are no cases for a long period of time, e.g., several months, after a wave of COVID-19 outbreak, then the new infection is likely to be caused by imported cases or exposure to the virus. The virus that caused a second wave can be summarized by the following three sources: (1) imported cases from abroad. Despite strict regulations on international travel and border inspections, there are still some reported cases imported from abroad. There is no guarantee that 100% of the infected or exposed cases entering the country will be isolated. The incubation period of the infection is not well known and may not be the same for all infected people. With fixed-duration quarantine implemented, the infected individual may become a confirmed case after the quarantine is over. This may be a risk for a second wave in mainland China. (2) Asymptomatic cases. These people carry the virus but cannot be identified if they do not have the nucleic acid test. However, they can infect other people. Therefore, asymptomatic carriers represent another risk for the occurrence of the second wave. (3) Virus from the environment. Some studies have shown that low temperature can greatly promote the persistence of coronaviruses. Frozen foods are potential carriers. Transmission occurs via touching contaminated objects that mediate the infection through mouth, nose, or eyes. This seems to be another risk of transmission that have been ignored.
The potential causes summarized above can be described by new exposed individuals added to our model at a certain time. The time point when the new confirmed case was reported is T2 but when the exposed individual was introduced remains unknown. Here we assume that the number of input exposed individuals is pE(T2−τ) where τ represents the time lag from the entry of the exposed individual to the later confirmation of infection. Thus, T2−τ is the time point when the exposed individuals entered. The equation of E(t) in model (2.1) can be replaced by the following equation
Et+1=Et+(1−q)D11(t)−D21(t)+pE(T2−τ). |
It is noted that the reported case and the imported case may not be the same person.
The increase in the susceptible population due to lifted interventions may also contribute to the second wave. The first wave of COVID-19 emerged in Wuhan in early January of 2020. The time happened to be about ten days before the Lunar New Year. This made most people stay at home and take the longest vacation, which greatly reduced the probability of contact. In addition, public transportation was terminated and schools and restaurants were all closed. This series of strict measures reduced the number of susceptible people to a very small level. In our model, we assume that the number of susceptible people in the first wave of outbreak is S01. After the first wave, social activities gradually returned to normal and the size of susceptible population increases to S02 when the second wave emerges. The time of the susceptible population change, denoted by T3, depends on the region. For Beijing and Xinjiang, we let it be the same as T1. For Hong Kong SAR, it is the time when the local restriction policy is released. Thus, the number of susceptible is given by the following piecewise function
S0={S01,t<T3,S02,t≥T3. | (2.4) |
We use the discrete stochastic model (2.1) with the input parameter pE(T2−τ) to fit the data of the two waves of outbreaks in Beijing and Xinjiang using the least square method. The data fitted include the number of reported confirmed cases, asymptomatic cases and recovered cases. For the epidemic in Hong Kong SAR, there were still sporadic reports of confirmed cases after the first wave. The reason for the second wave in Hong Kong SAR is likely the increase in the number of susceptible population due to lifted restriction of interventions. We use the model (2.1) without the input parameter pE(T2−τ) to fit the data in Hong Kong SAR. Parameter values obtained from the fitting are listed in Tables 1 and 2. The population size of susceptible in the three places is less than the entire population of those places. Here the susceptible population refers to those who may contact with the infected cases. The stochastic simulations provide good fits to the data in these three palaces, see Figure 2(d–f), Figure 3(d–f) and Figure 4(d–f). The corresponding contact rate c(t), quarantine rate q(t) and the susceptible population change S0(t) are shown in Figure 2(a–c), Figure 3(e–g) and Figure 4(a–c), respectively.
Initial values | Definition | Values | Source | |||
Beijing | Xinjiang | Hong Kong SAR | ||||
S01 | The value of susceptible population in the first wave | 5.0014×103 | 9.4767×103 | 7.3327×103 | Estimated | |
S02 | The value of the susceptible in the second wave | 6.0119×103 | 4.9377×104 | 2×104 | Estimated | |
E(0) | The initial value of exposed population | 8.0747 | 8.0209 | 6.0401 | Estimated | |
I(0) | The initial value of infected symptomatic population | 4.0902 | 3.0368 | 6.0316 | Estimated | |
A(0) | The initial value of infected asymptomatic population | 5.0848 | 4.0312 | 2.0328 | Estimated | |
Sq(0) | The initial value of quarantined susceptible population | 49.7473 | 49.9653 | 45.7412 | Estimated | |
Eq(0) | The initial value of quarantined exposed population | 20.0155 | 5.0235 | 13.1394 | Estimated | |
HI(0) | The initial value of confirmed and hospitalized symptomatic population | 1 | 3 | 5 | Data | |
1 | 3 | 5 | Data | |||
HA(0) | The initial value of confirmed and hospitalized asymptomatic population | 0 | 1 | 0 | Data | |
0 | 1 | 0 | Data | |||
R(0) | The initial value of recovered population | 0 | 0 | 0 | Data |
The emergence of the second wave is influenced by the number of input exposed individuals and how long the infection has been spreading before the report of confirmed cases. We conduct numerical simulations to study the risk of having a second wave. The occurrence of a second wave is evaluated by the maximum number of confirmed cases in 500 stochastic simulations. We denote the average number by MH and choose a threshold value 30. If the MH value exceeds 30, it will be regarded as a second wave. The result shows that not all the scenarios result in a second wave. From 500 stochastic simulations, we calculate the probability of the occurrence of a second wave, which is denoted by Prop.
In Figures 5 and 6, we explore the effect of varying the input parameter on the risk of second wave in Beijing and Xinjiang, respectively. The range of the parameter pE is set to [0,30] at time T2−τ, and the time delay parameter τ is within the range [0,20]. From Figure 5(a), we find that both the number of input exposed individuals and the time between initial entry and subsequent confirmation affect the severity of the second wave. The average maximum value of the second wave peak can reach 1600 cases in Beijing. Increasing the number of input exposed individuals can expand the scale of the disease spread. A larger time delay τ implies that the disease had spread for a longer time without any interventions before its detection. This poses a substantial challenge for the subsequent control of the disease.
We provide the parameter region of a second wave occurrence in Figure 5(b). The deep blue points represent the parameter range of the occurrence of a second wave, while the deep red points represent the parameter range of no second wave. The simulation shows that a second outbreak would not take place when less than three exposed cases were imported. If the infection induced by the imported cases can be quickly identified, then the chance of having a second wave decreases. Figure 5(c) further shows the probability of the occurrence of the second wave under the same parameter range in Figure 5(a). Large values of pE and τ will make a second wave inevitable. We have the similar conclusion from the simulation for Xinjiang (see Figure 6). The scale of the second wave is larger than Beijing with the same parameter range because the average maximum value of possible second wave peak can reach 5000 cases in the worst scenario.
Figure 9(a) shows the average result of 500 stochastic simulations of the model (2.1) with six different susceptible populations in Hong Kong SAR. As the susceptible population increases, the average maximum value of the second wave peak also increases. Interestingly, the probability of the occurrence of the second wave remains almost the same for different susceptible populations (see Figure 9(b)). Numerical results on the effect of varying the susceptible population size in Beijing and Xinjiang are shown in Figures 7 and 8, respectively. Based on the simulations in these two places, we have a conclusion similar to Hong Kong SAR. This analysis suggests that the susceptible population size plays a minor role in leading to the second wave when the other parameters are fixed.
COVID-19, a highly contagious disease first reported in December 2019, has been spreading globally for more than one year. Some countries/regions have mitigated the outbreak by various measures but are still at risk of recurrence. In this paper, we constructed a stochastic discrete-time compartmental epidemic model to analyze the risk of the occurrence of a second or future wave of outbreak. Compared with a deterministic system, a stochastic model is able to include the random factors in the spread of an infectious disease, particularly when the number of initial infected individuals is small. This is the case when a new wave of outbreak occurs. This discrete model can more intuitively describe the flow between any two compartments. The transition between two compartments is not deterministic and assumed to obey binomial distributions in our model. The change between two compartments in one time step corresponds to the daily data. Thus, using the discrete stochastic model facilitates full use of the data from multiple sources, thereby improving the reliability of the parameter estimation results.
To describe the change in the intensity of control measures in response to the COVID-19 pandemic, we adopt time-varying contact rate and quarantine rate in the model. There are a few possible factors that may lead to a second wave, including import exposed cases, asymptomatic cases, and the presence of viruses in the environment such as the frozen food chain. The common characteristic of these factors is that the transmission is silent and difficult to be identified. We find that the time between the exposed case entry and the confirmation of subsequent infection plays a critical role in the occurrence of the second wave.
The cause of the second-wave outbreak in Beijing and Xinjiang is mainly the imported cases and an increase in the susceptible population due to relaxed interventions. The model provided a good fit to the data of the second wave in Beijing in June 2020. Based on the fitting, the value of input exposed cases is estimated to be 6 and the time from exposed individual entry to the detection of infection is 17 days. The size of susceptible population increases from 5.001×103 in the first wave to 6.012×103 in the second wave. For Xinjiang where the second wave of the epidemic occurred in July 2020, the value of input exposed cases is estimated to be 7 and the time from entry to detection is 16 days. The change in the number of susceptible people is greater than in Beijing.
Hong Kong SAR also experienced a second wave in July 2020. Unlike Beijing and Xinjiang, there were occasional reports of infected cases all the time in Hong Kong SAR after the first wave and the main cause of the second wave is likely to be the increase in the number of susceptible people. Our modeling result suggests that in a region where the infection is not cleared (e.g., in Hong Kong SAR) susceptible people will increase as the control measures are lifted and this may lead to a second wave. If there is no case for a long time (e.g., in Beijing and Xinjiang), it is necessary to screen imported cases and viruses (e.g., via the food chain), which may be the major cause of the second wave.
On the basis of the fitting to the data in Beijing and Xinjiang, we further evaluated the possibility of having a future outbreak and its severity. Because there were no confirmed cases for a long time after the first wave in Beijing and Xinjiang, the contact rate returned to the normal level, as shown in Figures 2 and 3. If there are imported exposed cases, the time to detect the infection is shown to be critical in leading to the second wave. The simulation shown in Figure 5 and Figure 6 indicates that the second wave is determined by the number of imported exposed individuals and the time needed to detect them. The results suggest that if the imported exposed cases are less than three, then the number of confirmed cases will be below the threshold 30 we set, which would not be considered as a second wave. If the values of imported exposed individuals and the time lag in detection are larger (e.g., in the red region in Figures 5 and 6), a second wave will emerge. The more imported exposed cases and the longer for the infection to be detected, the more likely a second wave will occur. Once a confirmed case is found, it is imperative to track the trajectory of that case and the contacted persons. The information obtained from this study can be used to evaluate the possibility (i.e., the possibility of infected cases above a threshold level) and scale of a future wave of outbreak.
By investigating the effect of the susceptible population on the second wave in Beijing, Xinjiang and Hong Kong SAR, we found that the larger the susceptible population size, the more infections if the second wave occurs. However, the susceptible population size itself does not affect the probability of the occurrence of a second wave. This result suggests that imported cases might be an important factor leading to the occurrence of a second wave in a place where the epidemic has been well controlled. Once a case is found, reducing the number of susceptible people will help control the disease spread in the second wave.
Our study cannot predict when a second or future wave of COVID-19 would take place. When a new wave occurs, the model can be used to predict the scale or severity of the outbreak. This is based on the fitting of the model to existing data. If the data are not sufficient for fitting, then the power of the model prediction would be limited. Lastly, the model does not include the influence of vaccination on the disease spread. How the vaccine rollout influences the emergence of future waves remains to be further investigated.
In summary, we established a stochastic modeling framework that incorporates control measures at different stages of the epidemic and potential causes for the second wave emerged in Beijing, Xinjiang, and Hong Kong SAR. Because infected people without symptoms are contagious and the virus attached to goods is difficult to be detected, comprehensive measures are still imperative to curb the COVID-19 pandemic. It is necessary to screen the imported cases in flights and to detect the virus that may be transported by various routes. If a confirmed case is found, the contact of the case should be thoroughly tracked and the close contacts should be quarantined. Finally, it is important to continue protective measures such as wearing masks and avoiding large-scale gathering to reduce the number of susceptible people. This will make the future wave of outbreak less severe if it takes place.
This work was finished when the first author visited the University of Florida in 2020. This research was partially supported by the National Natural Science Foundation of China (grant numbers: 12031010(ST), 11631012(ST)) and by the Fundamental Research Funds for the Central Universities (grant numbers: 2018CBLZ001(SH), GK201901008(ST)). L. Rong is supported by the National Science Foundation (grant number: DMS-1950254).
No conflict of interest.
[1] |
B. Hu, H. Guo, P. Zhou, Z. L. Shi, Characteristics of SARS-CoV-2 and COVID-19, Nat. Rev. Microbiol., 19 (2021), 141–154. doi: 10.1038/s41579-020-00459-7
![]() |
[2] | H. Lu, C. Stratton, Y. Tang, Outbreak of pneumonia of unknown etiology in Wuhan, China: The mystery and the miracle, J. Med. Virol., 92 (2021), 401–402. |
[3] | World Health Organization. Naming the coronavirus disease (COVID-19) and the virus that causes it, 2020. Available from: https://www.who.int/emergencies/diseases/novel-coronavirus-2019/technical-guidance/naming-the-coronavirus-disease-(covid-2019)-and-the-virus-that-causes-it. |
[4] |
M. Shereen, S. Khan, A. Kazmi, N. Bashir, R. Siddique, COVID-19 infection: Origin, transmission, and characteristics of human coronaviruses, J. Adv. Res., 24 (2020), 91–98. doi: 10.1016/j.jare.2020.03.005
![]() |
[5] |
C. Ngonghala, E. Iboi, A. Gumel, Could masks curtail the post-lockdown resurgence of COVID-19 in the US?, Math. Biosci., 329 (2020), 108452. doi: 10.1016/j.mbs.2020.108452
![]() |
[6] |
B. Korber, W. Fischer, S. Gnanakaran, H. Yoon, J. Theiler, W. Abfalterer, D. C. Montefiori, Spike mutation pipeline reveals the emergence of a more transmissible form of SARS-CoV-2, Cell, 182 (2020), 812–827. doi: 10.1016/j.cell.2020.06.043
![]() |
[7] |
S. Wang, Y. Pan, Q. Wang, H. Miao, A. N. Brown, L. Rong, Modeling the viral dynamics of SARS-CoV-2 infection, Math. Biosci., 328 (2020), 108438. doi: 10.1016/j.mbs.2020.108438
![]() |
[8] |
M. Kraemer, C. Yang, B. Gutierrez, C. Wu, B. Klein, D. Pigott, et al., The effect of human mobility and control measures on the COVID-19 epidemic in China, Science, 368 (2020), 493–497. doi: 10.1126/science.abb4218
![]() |
[9] |
S. Liu, L. Yang, C. Zhang, Y. T. Xiang, Z. Liu, S. Hu, et al., Online mental health services in China during the COVID-19 outbreak, Lancet Psychiatry, 7 (2020), e17–e18. doi: 10.1016/S2215-0366(20)30077-8
![]() |
[10] |
Y. Han, H. Yang, The transmission and diagnosis of 2019 novel coronavirus infection disease (COVID-19): A Chinese perspective, J. Med. Virol., 92 (2020), 639–644. doi: 10.1002/jmv.25749
![]() |
[11] |
N. Zhu, D. Zhang, W. Wang, X. Li, B. Yang, J. Song, et al., A novel coronavirus from patients with pneumonia in China, 2019, N. Engl. J. Med., 382 (2020), 727–733. doi: 10.1056/NEJMoa2001017
![]() |
[12] |
J. Chen, H. He, W. Cheng, Y. Liu, Z. Sun, C. Chai, et al., Potential transmission of SARS-CoV-2 on a flight from Singapore to Hangzhou, China: an epidemiological investigation, Travel Med. Infect. Dis., 36 (2020), 101816. doi: 10.1016/j.tmaid.2020.101816
![]() |
[13] | F. Jiang, Z. Zhao, X. Shao, Time series analysis of COVID-19 infection curve: A change-point perspective, J. Econom., (2020). |
[14] | World Health Organization, COVID-19 Weekly Epidemiological Update. Online Version, (Accessed 22 Novermber 2020). |
[15] |
Y. Bai, L. Yao, T. Wei, F. Tian, D. Y. Jin, L. Chen, et al., Presumed asymptomatic carrier transmission of COVID-19, JAMA, 323 (2020), 1406–1407. doi: 10.1001/jama.2020.2565
![]() |
[16] | World Health Organization. Coronavirus disease 2019 (COVID-19): situation report, 82, (2020). |
[17] |
J. Al-Tawfiq, Asymptomatic coronavirus infection: MERS-CoV and SARS-CoV-2 (COVID-19), Travel Med. Infect. Dis., 35 (2020), 101608. doi: 10.1016/j.tmaid.2020.101608
![]() |
[18] |
X. Yu, R. Yang, COVID-19 transmission through asymptomatic carriers is a challenge to containment, Influenza Other Respir. Viruses, 14 (2020), 474–475. doi: 10.1111/irv.12743
![]() |
[19] |
H. Nishiura, T. Kobayashi, T. Miyama, A. Suzuki, S. M. Jung, K. Hayashi, et al., Estimation of the asymptomatic ratio of novel coronavirus infections (COVID-19), Int. J. Infect. Dis., 94 (2020), 154–155. doi: 10.1016/j.ijid.2020.03.020
![]() |
[20] |
J. Hirshberg, M. Stout, N. Raghuraman, Coronavirus disease 2019 infection among asymptomatic and symptomatic pregnant women: two weeks of confirmed presentations to an affiliated pair of New York City hospitals, Am. J. Obstet. Gynecol. MFM, 2 (2020), 100118. doi: 10.1016/j.ajogmf.2020.100118
![]() |
[21] |
G. Suda, K. Ogawa, M. Kimura, O. Maehara, T. kitagataya, M. ohara, et al., Time-dependent changes in the seroprevalence of COVID-19 in asymptomatic liver disease outpatients in an area in Japan undergoing a second wave of COVID-19, Hepatol. Res., 50 (2020), 1196–1200. doi: 10.1111/hepr.13551
![]() |
[22] |
L. Nikolai, C. Meyer, P. Kremsner, T. Velavan, Asymptomatic SARS Coronavirus 2 infection: Invisible yet invincible, Int. J. Infect. Dis., 100 (2020), 112–116. doi: 10.1016/j.ijid.2020.08.076
![]() |
[23] |
F. Ye, S. Xu, Z. Rong, R. Xu, X. Liu, P. Deng, et al., Delivery of infection from asymptomatic carriers of COVID-19 in a familial cluster, Int. J. Infect. Dis., 92 (2020), 133–138. doi: 10.1016/j.ijid.2020.01.010
![]() |
[24] | B. Dickens, J. Koo, J. Lim, H. Sun, H. Clapham, A. Wilder-Smith, et al., Strategies at points of entry to reduce importation risk of COVID-19 cases and reopen travel, J. Travel Med., 27 (2020), 1–8. |
[25] |
L. Zhang, H. Yang, K. Wang, Y. Zhan, L. Bian, Measuring imported case risk of COVID-19 from inbound international flights–-A case study on China, J. Air Transp. Manag., 89 (2020), 101918. doi: 10.1016/j.jairtraman.2020.101918
![]() |
[26] | Z. Zhang, L. Li, P. Qin, K. Li, Y. Huang, L. Luo, et al., Countries of origin of imported COVID-19 cases into China and measures to prevent onward transmission, J. Travel Med., 27 (2020), 1–3. |
[27] |
O. Adegboye, A. Adekunle, A. Pak, E. Gayawan, D. Leung, D. Rojas, et al., Change in outbreak epicenter and its impact on the importation risks of COVID-19 progression: a modelling study, Travel Med. Infect. Dis., 40 (2021), 101988. doi: 10.1016/j.tmaid.2021.101988
![]() |
[28] |
C. Cruz, R. Ganly, Z. Li, S. Gietel-Basten, Exploring the young demographic profile of COVID-19 cases in Hong Kong: Evidence from migration and travel history data, PLoS ONE, 15 (2020), e0235306. doi: 10.1371/journal.pone.0225487
![]() |
[29] |
Z. Liang, M. Pang, X. Yang, J. Li, Y. Wang, Z. Li, et al., Weekly assessment of the COVID-19 pandemic and risk of importation-China, April 8, 2020, China CDC Weekly, 2 (2020), 270–276. doi: 10.46234/ccdcw2020.070
![]() |
[30] |
J. Han, X. Zhang, S. He, P. Jia, Can the coronavirus disease be transmitted from food? A review of evidence, risks, policies and knowledge gaps, Environ. Chem. Lett., 19 (2021), 5–16. doi: 10.1007/s10311-020-01101-x
![]() |
[31] |
D. Glass, European and US lockdowns and second waves during the COVID-19 pandemic, Math. Biosci., 330 (2020), 108472. doi: 10.1016/j.mbs.2020.108472
![]() |
[32] |
C. Galanakis, The food systems in the era of the coronavirus (COVID-19) pandemic crisis, Foods, 9 (2020), 523. doi: 10.3390/foods9040523
![]() |
[33] |
A. Aleta, D. Martïn-Corral, A. Piontti, M. Ajelli, M. Litvinova, M. Chinazzi, et al., Modelling the impact of testing, contact tracing and household quarantine on second waves of COVID-19, Nat. Hum. Behav., 4 (2020), 964–971. doi: 10.1038/s41562-020-0931-9
![]() |
[34] | V. Grech, S. Cuschieri, COVID-19: A global and continental overview of the second wave and its (relatively) attenuated case fatality ratio, Early Hum. Dev., (2020), 105211. |
[35] | Y. Ho, Y. Chen, S. H. Hung, C. Huang, P. Po, C. Chan, et al., Social distancing 2.0 with privacy-preserving contact tracing to avoid a second wave of COVID-19, arXiv preprint arXiv: 2006.16611. |
[36] |
M. Renardy, M. Eisenberg, D. Kirschner, Predicting the second wave of COVID-19 in Washtenaw County, MI, J. Theor. Biol., 507 (2020), 110461. doi: 10.1016/j.jtbi.2020.110461
![]() |
[37] | K. Friston, T. Parr, P. Zeidman, A. Razi, G. Flandin, J. Daunizeau, et al., Effective immunity and second waves: a dynamic causal modelling study, Wellcome Open Res., 5 (2020). |
[38] |
H. Zhao, Z. Feng, Staggered release policies for COVID-19 control: Costs and benefits of relaxing restrictions by age and risk, Math. Biosci., 326 (2020), 108405. doi: 10.1016/j.mbs.2020.108405
![]() |
[39] |
J. Panovska-Griffiths, C. Kerr, R. Stuart, D. Mistry, D. Klein, R. Viner, et al., Determining the optimal strategy for reopening schools, the impact of test and trace interventions, and the risk of occurrence of a second COVID-19 epidemic wave in the UK: a modelling study, Lancet Child Adolesc. Health, 4 (2020), 817–827. doi: 10.1016/S2352-4642(20)30250-9
![]() |
[40] |
L. Löpez, X. Rodö, The end of social confinement and COVID-19 re-emergence risk, Nat. Hum. Behav., 4 (2020), 746–755. doi: 10.1038/s41562-020-0908-8
![]() |
[41] |
G. Fan, Z. Yang, Q. Lin, S. Zhao, L. Yang, D. He, Decreased case fatality rate of COVID-19 in the second wave: A study in 53 countries or regions, Transbound. Emerg. Dis., 68 (2021), 213–215. doi: 10.1111/tbed.13819
![]() |
[42] | J. Jia, S. Liu, J. Ding, G. Liao, Y. Wei, R. Zhang, et al., The impact of imported cases on the control of COVID-19 in China (in Chinese), SCIENTA SINICA Mathematica, 50 (2020), 1–14. |
[43] |
P. Lekone, B. Finkenstädt, Statistical inference in a stochastic epidemic SEIR model with control intervention: Ebola as a case study, Biometrics, 62 (2006), 1170–1177. doi: 10.1111/j.1541-0420.2006.00609.x
![]() |
[44] |
S. Tang, B. Tang, N. Bragazzi, F. Xia, T. Li, S. He, et al., Analysis of COVID-19 epidemic traced data and stochastic discrete transmission dynamic model (in Chinese), SCIENTA SINICA Mathematica, 50 (2020), 1071–1086. doi: 10.1360/SSM-2020-0053
![]() |
[45] |
S. He, S. Tang, L. Rong, A discrete stochastic model of the COVID-19 outbreak: Forecast and control, Math. Biosci. Eng., 17 (2020), 2792–2804. doi: 10.3934/mbe.2020153
![]() |
[46] |
H. Tian, Y. Liu, Y. Li, C. Wu, B. Chen, M. Kraemer, et al., An investigation of transmission control measures during the first 50 days of the COVID-19 epidemic in China, Science, 368 (2020), 638–642. doi: 10.1126/science.abb6105
![]() |
[47] |
B. Tang, X. Wang, Q. Li, N. Bragazzi, S. Tang, Y. Xiao, et al., Estimation of the transmission risk of the 2019-nCoV and its implication for public health interventions, J. Clin. Med., 9 (2020), 462. doi: 10.3390/jcm9020462
![]() |
1. | Timotei István Erdei, Rudolf Krakó, Géza Husi, Design of a Digital Twin Training Centre for an Industrial Robot Arm, 2022, 12, 2076-3417, 8862, 10.3390/app12178862 | |
2. | Cheng-Long Wang, Shasha Gao, Xue-Zhi Li, Maia Martcheva, Modeling Syphilis and HIV Coinfection: A Case Study in the USA, 2023, 85, 0092-8240, 10.1007/s11538-023-01123-w | |
3. | Yujie Sheng, Jing-An Cui, Songbai Guo, The modeling and analysis of the COVID-19 pandemic with vaccination and isolation: a case study of Italy, 2023, 20, 1551-0018, 5966, 10.3934/mbe.2023258 | |
4. | Hao Wang, Di Zhu, Shiqi Li, Robert A. Cheke, Sanyi Tang, Weike Zhou, Home quarantine or centralized quarantine? A mathematical modelling study on the COVID-19 epidemic in Guangzhou in 2021, 2022, 19, 1551-0018, 9060, 10.3934/mbe.2022421 | |
5. | Israel Edem Agbehadji, Bankole Osita Awuzie, Alfred Beati Ngowi, COVID-19 Pandemic Waves: 4IR Technology Utilisation in Multi-Sector Economy, 2021, 13, 2071-1050, 10168, 10.3390/su131810168 | |
6. | Tianheng Xie, Jianfang Zhang, Xiangtao Li, Data-Driven Intelligent Risk System in the Process of Financial Audit, 2022, 2022, 1563-5147, 1, 10.1155/2022/9054209 | |
7. | Katica Tomic, 2023, Chapter 11, 978-3-031-13752-5, 301, 10.1007/978-3-031-13753-2_11 | |
8. | Shasha Gao, Mingwang Shen, Xueying Wang, Jin Wang, Maia Martcheva, Libin Rong, A multi-strain model with asymptomatic transmission: Application to COVID-19 in the US, 2023, 565, 00225193, 111468, 10.1016/j.jtbi.2023.111468 | |
9. | 德玉 郭, A Dynamics Model of COVID-19 with Transition from the Asymptomatic to the Symptomatic Infected Individuals, 2023, 12, 2324-7991, 2457, 10.12677/AAM.2023.125248 | |
10. | Kaijing Chen, Fengying Wei, Xinyan Zhang, Hao Jin, Zuwen Wang, Yue Zuo, Kai Fan, Epidemiological feature analysis of SVEIR model with control strategy and variant evolution, 2024, 9, 24680427, 689, 10.1016/j.idm.2024.03.005 | |
11. | Shasha Gao, Pant Binod, Chidozie Williams Chukwu, Theophilus Kwofie, Salman Safdar, Lora Newman, Seoyun Choe, Bimal Kumar Datta, Wisdom Kwame Attipoe, Wenjing Zhang, P. van den Driessche, A mathematical model to assess the impact of testing and isolation compliance on the transmission of COVID-19, 2023, 8, 24680427, 427, 10.1016/j.idm.2023.04.005 |
Parameters | Definition | Values | Source | |||
Beijing | Xinjiang | Hong Kong SAR | ||||
c(t) | c0 | Contact rate at the initial time | 17.0142 | 14.0025 | 11.9175 | Estimated |
cb | Minimum contact rate under the most strict control strategies | 1.0869 | 2.0468 | 3.0460 | Estimated | |
r1 | Exponential decreasing rate of contact rate in the first period | 0.2196 | 0.2049 | 0.0703 | Estimated | |
r2 | Exponential increasing rate of contact rate in the second period | 0.0506 | 0.0200 | 0.0324 | Estimated | |
r3 | Exponential decreasing rate of contact rate in the third period | 0.2241 | 0.3096 | 0.1445 | Estimated | |
β | Probability of transmission per contact | 0.2801 | 0.2722 | 0.1504 | Estimated | |
q(t) | q0 | Quarantined rate at the initial time | – | 0.2820 | – | Estimated |
qm | Maximum quarantined rate with control strategies | – | 0.7083 | – | Estimated | |
r4 | Exponential increasing rate of quarantined rate in the first period | – | 0.2027 | – | Estimated | |
r5 | Exponential decreasing rate of quarantined rate in the second period | – | 0.1010 | – | Estimated | |
r6 | Exponential increasing rate of quarantined rate in the third period | – | 0.2028 | – | Estimated | |
q | Quarantined rate | 0.2935 | – | 0.4866 | Estimated | |
ρ | Ratio of symptomatic infection | 0.5142 | 0.5520 | 0.5529 | Estimated | |
σ | Transition rate of exposed individuals to the infected class | 1/5 | 1/5 | 1/5 | [47] | |
λ | Rate at which the quarantined uninfected contacts were released into the wider community | 1/14 | 1/14 | 1/14 | [47] | |
δI | Constant transition rate of symptomatic infected | 0.3474 | 0.2008 | 0.0999 | Estimated | |
δA | Constant transition rate of asymptomatic infected | 0.2860 | 0.2006 | 0.3027 | Estimated | |
δq | Constant transition rate of quarantined exposed | 0.3599 | 0.2816 | 0.2571 | Estimated | |
θ | Correction factor for transmission probability of asymptomatic infectious | 0.5919 | 0.5031 | 0.5041 | Estimated | |
k | Conversion rate from asymptomatic infected to symptomatic infected | 0.6124 | 0.6221 | 0.5026 | Estimated | |
γI | Recovery rate of infected individuals | 0.0701 | 0.1632 | 0.0799 | Estimated | |
γA | Recovery rate of asymptotic infected individuals | 0.0906 | 0.1629 | 0.2393 | Estimated | |
τ | The time of importation of the first case in the second wave | 17 | 16 | – | Estimated | |
pE(T2−τ) | The number of exposed cases entered at the time T2−τ in the second wave | 6 | 7.02 | – | Estimated | |
α | Disease-induced death rate | 0 | 0 | 0 | Assumed | |
– means the parameter is not included in that place. |
Initial values | Definition | Values | Source | |||
Beijing | Xinjiang | Hong Kong SAR | ||||
S01 | The value of susceptible population in the first wave | 5.0014×103 | 9.4767×103 | 7.3327×103 | Estimated | |
S02 | The value of the susceptible in the second wave | 6.0119×103 | 4.9377×104 | 2×104 | Estimated | |
E(0) | The initial value of exposed population | 8.0747 | 8.0209 | 6.0401 | Estimated | |
I(0) | The initial value of infected symptomatic population | 4.0902 | 3.0368 | 6.0316 | Estimated | |
A(0) | The initial value of infected asymptomatic population | 5.0848 | 4.0312 | 2.0328 | Estimated | |
Sq(0) | The initial value of quarantined susceptible population | 49.7473 | 49.9653 | 45.7412 | Estimated | |
Eq(0) | The initial value of quarantined exposed population | 20.0155 | 5.0235 | 13.1394 | Estimated | |
HI(0) | The initial value of confirmed and hospitalized symptomatic population | 1 | 3 | 5 | Data | |
1 | 3 | 5 | Data | |||
HA(0) | The initial value of confirmed and hospitalized asymptomatic population | 0 | 1 | 0 | Data | |
0 | 1 | 0 | Data | |||
R(0) | The initial value of recovered population | 0 | 0 | 0 | Data |
Parameters | Definition | Values | Source | |||
Beijing | Xinjiang | Hong Kong SAR | ||||
c(t) | c0 | Contact rate at the initial time | 17.0142 | 14.0025 | 11.9175 | Estimated |
cb | Minimum contact rate under the most strict control strategies | 1.0869 | 2.0468 | 3.0460 | Estimated | |
r1 | Exponential decreasing rate of contact rate in the first period | 0.2196 | 0.2049 | 0.0703 | Estimated | |
r2 | Exponential increasing rate of contact rate in the second period | 0.0506 | 0.0200 | 0.0324 | Estimated | |
r3 | Exponential decreasing rate of contact rate in the third period | 0.2241 | 0.3096 | 0.1445 | Estimated | |
β | Probability of transmission per contact | 0.2801 | 0.2722 | 0.1504 | Estimated | |
q(t) | q0 | Quarantined rate at the initial time | – | 0.2820 | – | Estimated |
qm | Maximum quarantined rate with control strategies | – | 0.7083 | – | Estimated | |
r4 | Exponential increasing rate of quarantined rate in the first period | – | 0.2027 | – | Estimated | |
r5 | Exponential decreasing rate of quarantined rate in the second period | – | 0.1010 | – | Estimated | |
r6 | Exponential increasing rate of quarantined rate in the third period | – | 0.2028 | – | Estimated | |
q | Quarantined rate | 0.2935 | – | 0.4866 | Estimated | |
ρ | Ratio of symptomatic infection | 0.5142 | 0.5520 | 0.5529 | Estimated | |
σ | Transition rate of exposed individuals to the infected class | 1/5 | 1/5 | 1/5 | [47] | |
λ | Rate at which the quarantined uninfected contacts were released into the wider community | 1/14 | 1/14 | 1/14 | [47] | |
δI | Constant transition rate of symptomatic infected | 0.3474 | 0.2008 | 0.0999 | Estimated | |
δA | Constant transition rate of asymptomatic infected | 0.2860 | 0.2006 | 0.3027 | Estimated | |
δq | Constant transition rate of quarantined exposed | 0.3599 | 0.2816 | 0.2571 | Estimated | |
θ | Correction factor for transmission probability of asymptomatic infectious | 0.5919 | 0.5031 | 0.5041 | Estimated | |
k | Conversion rate from asymptomatic infected to symptomatic infected | 0.6124 | 0.6221 | 0.5026 | Estimated | |
γI | Recovery rate of infected individuals | 0.0701 | 0.1632 | 0.0799 | Estimated | |
γA | Recovery rate of asymptotic infected individuals | 0.0906 | 0.1629 | 0.2393 | Estimated | |
τ | The time of importation of the first case in the second wave | 17 | 16 | – | Estimated | |
pE(T2−τ) | The number of exposed cases entered at the time T2−τ in the second wave | 6 | 7.02 | – | Estimated | |
α | Disease-induced death rate | 0 | 0 | 0 | Assumed | |
– means the parameter is not included in that place. |
Initial values | Definition | Values | Source | |||
Beijing | Xinjiang | Hong Kong SAR | ||||
S01 | The value of susceptible population in the first wave | 5.0014×103 | 9.4767×103 | 7.3327×103 | Estimated | |
S02 | The value of the susceptible in the second wave | 6.0119×103 | 4.9377×104 | 2×104 | Estimated | |
E(0) | The initial value of exposed population | 8.0747 | 8.0209 | 6.0401 | Estimated | |
I(0) | The initial value of infected symptomatic population | 4.0902 | 3.0368 | 6.0316 | Estimated | |
A(0) | The initial value of infected asymptomatic population | 5.0848 | 4.0312 | 2.0328 | Estimated | |
Sq(0) | The initial value of quarantined susceptible population | 49.7473 | 49.9653 | 45.7412 | Estimated | |
Eq(0) | The initial value of quarantined exposed population | 20.0155 | 5.0235 | 13.1394 | Estimated | |
HI(0) | The initial value of confirmed and hospitalized symptomatic population | 1 | 3 | 5 | Data | |
1 | 3 | 5 | Data | |||
HA(0) | The initial value of confirmed and hospitalized asymptomatic population | 0 | 1 | 0 | Data | |
0 | 1 | 0 | Data | |||
R(0) | The initial value of recovered population | 0 | 0 | 0 | Data |