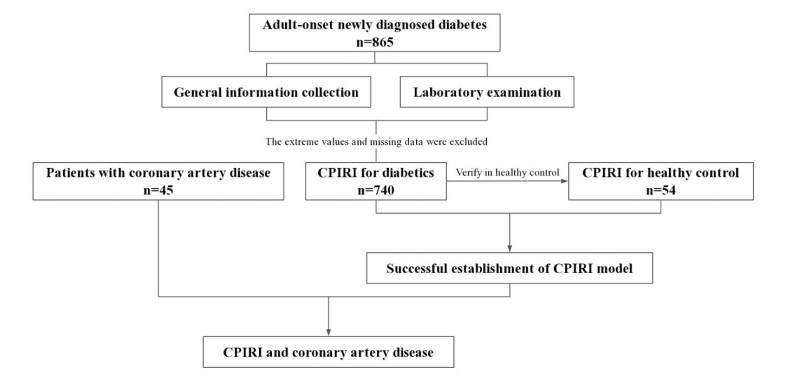
Insulin resistance is a major risk factor for coronary artery disease (CAD). The C-peptide-to-insulin ratio (C/I) is associated with hepatic insulin clearance and insulin resistance. The current study was designed to establish a novel C/I index (CPIRI) model and provide early risk assessment of CAD.
A total of 865 adults diagnosed with new-onset diabetes mellitus (DM) within one year and 54 healthy controls (HC) were recruited to develop a CPIRI model. The CPIRI model was established with fasting C/I as the independent variable and homeostasis model assessment of insulin resistance (HOMA-IR) as the dependent variable. Associations between the CPIRI model and the severity of CAD events were also assessed in 45 hyperglycemic patients with CAD documented via coronary arteriography (CAG) and whom underwent stress echocardiography (SE) and exercise electrocardiography test (EET).
Fasting C-peptide/insulin and HOMA-IR were hyperbolically correlated in DM patients and HC, and log(C/I) and log(HOMA-IR) were linearly and negatively correlated. The respective correlational coefficients were −0.83 (p < 0.001) and −0.76 (p < 0.001). The equations CPIRI(DM) = 670/(C/I)2.24 + 0.25 and CPIRI(HC) = 670/(C/I)2.24 − 1 (F = 1904.39, p < 0.001) were obtained. Patients with insulin resistance exhibited severe coronary artery impairment and myocardial ischemia. In CAD patients there was no significant correlation between insulin resistance and the number of vessels involved.
CPIRI can be used to effectively evaluate insulin resistance, and the combination of CPIRI and non-invasive cardiovascular examination is of great clinical value in the assessment of CAD.
Citation: Hao Dai, Qi Fu, Heng Chen, Mei Zhang, Min Sun, Yong Gu, Ningtian Zhou, Tao Yang. A novel numerical model of combination levels of C-peptide and insulin in coronary artery disease risk prediction[J]. Mathematical Biosciences and Engineering, 2021, 18(3): 2675-2687. doi: 10.3934/mbe.2021136
[1] | Ziyu Jin, Ning Li . Diagnosis of each main coronary artery stenosis based on whale optimization algorithm and stacking model. Mathematical Biosciences and Engineering, 2022, 19(5): 4568-4591. doi: 10.3934/mbe.2022211 |
[2] | Li Cai, Qian Zhong, Juan Xu, Yuan Huang, Hao Gao . A lumped parameter model for evaluating coronary artery blood supply capacity. Mathematical Biosciences and Engineering, 2024, 21(4): 5838-5862. doi: 10.3934/mbe.2024258 |
[3] | Anarina L. Murillo, Jiaxu Li, Carlos Castillo-Chavez . Modeling the dynamics of glucose, insulin, and free fatty acids with time delay: The impact of bariatric surgery on type 2 diabetes mellitus. Mathematical Biosciences and Engineering, 2019, 16(5): 5765-5787. doi: 10.3934/mbe.2019288 |
[4] | Xintong Wu, Yingyi Geng, Xinhong Wang, Jucheng Zhang, Ling Xia . Continuous extraction of coronary artery centerline from cardiac CTA images using a regression-based method. Mathematical Biosciences and Engineering, 2023, 20(3): 4988-5003. doi: 10.3934/mbe.2023231 |
[5] | Benchawan Wiwatanapataphee, Yong Hong Wu, Thanongchai Siriapisith, Buraskorn Nuntadilok . Effect of branchings on blood flow in the system of human coronary arteries. Mathematical Biosciences and Engineering, 2012, 9(1): 199-214. doi: 10.3934/mbe.2012.9.199 |
[6] | Danilo T. Pérez-Rivera, Verónica L. Torres-Torres, Abraham E. Torres-Colón, Mayteé Cruz-Aponte . Development of a computational model of glucose toxicity in the progression of diabetes mellitus. Mathematical Biosciences and Engineering, 2016, 13(5): 1043-1058. doi: 10.3934/mbe.2016029 |
[7] | Nattawan Chuchalerm, Wannika Sawangtong, Benchawan Wiwatanapataphee, Thanongchai Siriapisith . Study of Non-Newtonian blood flow - heat transfer characteristics in the human coronary system with an external magnetic field. Mathematical Biosciences and Engineering, 2022, 19(9): 9550-9570. doi: 10.3934/mbe.2022444 |
[8] | Micaela Morettini, Christian Göbl, Alexandra Kautzky-Willer, Giovanni Pacini, Andrea Tura, Laura Burattini . Former gestational diabetes: Mathematical modeling of intravenous glucose tolerance test for the assessment of insulin clearance and its determinants. Mathematical Biosciences and Engineering, 2020, 17(2): 1604-1615. doi: 10.3934/mbe.2020084 |
[9] | Jiaxu Li, Yang Kuang . Systemically modeling the dynamics of plasma insulin in subcutaneous injection of insulin analogues for type 1 diabetes. Mathematical Biosciences and Engineering, 2009, 6(1): 41-58. doi: 10.3934/mbe.2009.6.41 |
[10] | Javad Hassannataj Joloudari, Hamid Saadatfar, Mohammad GhasemiGol, Roohallah Alizadehsani, Zahra Alizadeh Sani, Fereshteh Hasanzadeh, Edris Hassannataj, Danial Sharifrazi, Zulkefli Mansor . FCM-DNN: diagnosing coronary artery disease by deep accuracy fuzzy C-means clustering model. Mathematical Biosciences and Engineering, 2022, 19(4): 3609-3635. doi: 10.3934/mbe.2022167 |
Insulin resistance is a major risk factor for coronary artery disease (CAD). The C-peptide-to-insulin ratio (C/I) is associated with hepatic insulin clearance and insulin resistance. The current study was designed to establish a novel C/I index (CPIRI) model and provide early risk assessment of CAD.
A total of 865 adults diagnosed with new-onset diabetes mellitus (DM) within one year and 54 healthy controls (HC) were recruited to develop a CPIRI model. The CPIRI model was established with fasting C/I as the independent variable and homeostasis model assessment of insulin resistance (HOMA-IR) as the dependent variable. Associations between the CPIRI model and the severity of CAD events were also assessed in 45 hyperglycemic patients with CAD documented via coronary arteriography (CAG) and whom underwent stress echocardiography (SE) and exercise electrocardiography test (EET).
Fasting C-peptide/insulin and HOMA-IR were hyperbolically correlated in DM patients and HC, and log(C/I) and log(HOMA-IR) were linearly and negatively correlated. The respective correlational coefficients were −0.83 (p < 0.001) and −0.76 (p < 0.001). The equations CPIRI(DM) = 670/(C/I)2.24 + 0.25 and CPIRI(HC) = 670/(C/I)2.24 − 1 (F = 1904.39, p < 0.001) were obtained. Patients with insulin resistance exhibited severe coronary artery impairment and myocardial ischemia. In CAD patients there was no significant correlation between insulin resistance and the number of vessels involved.
CPIRI can be used to effectively evaluate insulin resistance, and the combination of CPIRI and non-invasive cardiovascular examination is of great clinical value in the assessment of CAD.
Abbreviations: CAD: coronary artery disease; C/I: the C-peptide-to-insulin ratio; CPIRI: C-peptide to insulin ratio index; DM: diabetes mellitus; HC: healthy controls; HOMA-IR: homeostasis model assessment of insulin resistance; CAG: coronary arteriography; SE: stress echocardiography; EET: exercise electrocardiography test; HGP: hepatic glucose production; T2DM: type 2 diabetes mellitus; HbA1c: Glycated hemoglobin; WMA: wall motion abnormalities; LCA: left coronary artery; RCA: right coronary artery; BMI: body mass index; NDDM: newly diagnosed diabetes mellitus; NAFLD: non-alcoholic fatty liver disease
Coronary artery disease (CAD) results in substantial mortality worldwide [1]. Several non-invasive and reliable methods for coronary artery disease screening and diagnosis have been developed, including stress echocardiography (SE) and the exercise electrocardiography test (EET) [2]. Various mechanisms inherently associated with insulin resistance are involved in coronary artery disease in both diabetic and non-diabetic subjects, including changes in vascular endothelium [3], adipokines [4] and other cytokines [5], the opioid system [6,7], ubiquitin-proteasome deregulation [8], carbonic anhydrase activation [9], oxidative stress and inflammatory activity [10,11]. Insulin resistance is considered a major risk factor for CAD [12].
The concept of insulin resistance can be traced back to clinical observations described by Himsworth in 1936 [13]. It is usually defined as insulin-mediated glucose processing and/or inhibition of hepatic glucose production (HGP), and the sensitivity of adipose tissue lipolysis and/or reduced reactivity [14,15]. The liver plays an important role in coordinating systemic metabolic homeostasis [16,17]. In patients with hepatic insulin resistance insulin cannot effectively stimulate the liver to absorb glucose, nor can it fully regulate HGP in fasting and post-prandial states. Excessive HGP results in inadequate glucose uptake by muscle tissues, which leads to hyperglycemia.
Several methods including direct, indirect, and surrogate indicators are currently employed to assess insulin resistance. The vast majority of insulin resistance indicators assess the relationship between glucose and insulin. Among them, the homeostasis model assessment of insulin resistance (HOMA-IR) index is the most widely used to evaluate the degree of insulin resistance. First proposed by Matthews et al. [18,19] in 1985 with a standard value of 1.0, HOMA-IR is based on analyses of feedback regulation between the liver and pancreatic-islet tissue in homeostasis, in turn reflecting the balance between insulin secretion and hepatic glycogen output. Notably however, variation in the blood glucose level affects the assessment of insulin resistance. One of the aims of the current study was to establish a novel model for insulin resistance evaluation.
There are differences in metabolic kinetics between insulin and C-peptide, though both are secreted into the portal vein in equimolar quantities. Specifically, the clearance of C-peptide from circulation is slower than that of insulin, and a large portion of insulin is cleared via hepatic metabolism, whereas the remainder enters the periphery and has a half-life of only 2–3 min. The liver is therefore the primary organ responsible for insulin clearance, whereas C-peptide is mainly cleared via the kidney. The half-life of C-peptide is approximately 30 min [20,21,22]. Accordingly, the C-peptide-to-insulin ratio (C/I) reflects hepatic insulin clearance [23], and hepatic insulin clearance is closely related to insulin resistance [24,25].
Early diagnosis of diabetes mellitus (DM) as well as intensive glycemic control are of great importance in reducing the incidence of cardiovascular events, and previous studies have confirmed that the fasting state facilitates the acquisition of sufficient critical data to accurately evaluate insulin resistance [26]. The present study incorporated fasting C/I into a novel C/I index (CPIRI) based on HOMA-IR in newly diagnosed DM patients and healthy controls (HC) to determine insulin resistance levels, and investigated relationships between insulin resistance and CAD, with the aim of generating a new insulin resistance risk prediction model for use in patients with suspected CAD.
The current investigation was a population-based cross-sectional study that included 865 people aged from 18 to 84 years who had been diagnosed with DM within 1 year at the First Affiliated Hospital with Nanjing Medical University, and 54 HC with normal glucose tolerance. DM was diagnosed in accordance with the World Health Organization 1999 criteria. Exclusion criteria for DM patients were a history of pancreatic exocrine disease, gestational DM, secondary DM, severe systemic disease, severe heart failure, critical liver/kidney dysfunction, hematologic disease, and mental illness. After excluding DM patients with incomplete data and those found to have extreme (1%) outlying data, a CPIRI model was generated based on 740 DM patients and 54 HC. In addition, 45 patients with CAD confirmed via coronary arteriography (CAG) and a history of type 2 DM (T2DM) were enrolled in the study, and relationships between CPIRI and CAD severity (by CAG/SE/EET) were investigated. A flow-chart representation of the study is shown in Figure 1.
The study protocol was approved by the ethics committee of the First Affiliated Hospital with Nanjing Medical University (2015-SR-071) in Nanjing, China. All participants provided written informed consent.
General patient information and complete medical histories were obtained using questionnaires specifically developed for this study. Anthropometric details were collected by experienced researchers. After overnight fasting, blood samples were collected and metabolic profiles were obtained via colorimetric assays with an automatic biochemical analyzer (AU5800; Beckman, Coulter, Fullerton, CA, USA). Levels of insulin and C-peptide were determined via an automatic electrochemical luminescence immunoassay (Roche, Basel, Switzerland). Glycated hemoglobin (HbA1c) concentrations were measured using a Variant II system (Bio-Rad Laboratories, Hercules, CA, USA). Abdominal ultrasound imaging was performed using an EPIC7 L12-3 ultrasound machine (Philips, Amsterdam, Netherlands). HOMA-IR was calculated via the following formula [18]:
HOMA−IR=fastinginsulin(mIU/L)×fastingglucose(mmol/L)/22.5 | (1) |
All patients with suspected CAD accepted SE as previously described [2]. Briefly, they were treated using a treadmill protocol (GE T2100) or dobutamine infusion. Short axis and apical 4-chamber, 3-chamber and 2-chamber images were obtained (iE33 Philips Medical Systems, Eindhoven, the Netherlands). Imaging of rest, peak exercise, postexercise and recovery were evaluated as described above. Information on exercise capacity, blood pressure, heart rate and rhythm changes were supervised and analyzed, as well as wall motion abnormalities (WMA) analysis as final interpretation. Sonovue, an ultrasound contrast agent (Bracco, Milan, Italy), was administered by intravenous bolus injection (0.3–0.5 mL) and flushed with saline. The final results were interpreted by professional cardiologists. A 17-segment left-ventricle model was evaluated by a four-point score (1: normal; 2: hypokinetic; 3: akinetic; 4: dyskinetic motion), which was used to analyze the WMA and wall thickening. A negative judgment was defined as normal ST segments at baseline, exercise and recovery stages. Indeed, peak exercise was defined as patients achieving the age-predicted target heart rate at a workload of at least 7 Metabolic Equivalents Regional WMA at rest or randomized exercise stages were deemed to be positive SE.
EET results were interpreted by three expert cardiologists. A treadmill and the Bruce protocol were used for evaluation. Parameters and judgments were as described above.
Standard techniques were used to perform CAG. A visual quantitative scoring system was used to evaluate stenosis, with positive defined as ≥ 50% luminal diameter narrowing in one or more epicardial coronary arteries or major branches. A cutoff value of 50% was used because it has previously been shown to be prognostic.
Statistical analysis was performed using SPSS 23.0 (IBM, Armonk, NY, USA). Quantitative data were confirmed to be normally distributed via the Shapiro–Wilk test, and were expressed as means ± the standard deviation. Categorical variables were expressed as frequencies or percentages. Mean values from two groups were compared using Levene's test of homogeneity of variances. Student's t-test was used to compare datasets with equal variance, and the t' test was used to compare datasets with unequal variance. If Levene's test revealed homogeneity of variance, one-way analysis was performed to compare means of multiple groups. If Levene's test revealed heterogeneity of variance, the Welch test was used. Comparisons of classification variables between groups were evaluated using the Pearson χ2 test. General linear regression was used to formulate the CPIRI model. p < 0.05 was considered statistically significant.
Patients with insulin resistance who suffered from cardiovascular attack underwent SE, EET, and CAG. All cardiovascular tests confirmed that insulin resistance resulted in severe coronary artery impairment and myocardial ischemia. As shown in Figure 2, SE and EET depicted severer ST depression in V1–V6 leads and elevated ST in aVR lead (Figure 2A). Severe WMA (Figure 2B) suggested myocardial ischemia, which was confirmed by CAG (Figure 2C).
The main clinical and metabolic characteristics of the newly diagnosed DM patients in the study are shown in Table 1. Their mean age was approximately 48 years. Male patients accounted for a larger proportion of the newly diagnosed DM than in the HC group (57.8% vs. 50.0%, p < 0.001). Diabetic patients suffered more serious metabolic disorders, as evidenced by increased total cholesterol, triglycerides, low density lipoprotein, apolipoprotein A, body mass index (BMI), waist circumference, and aminotransferase, and reduced levels of high-density lipoprotein and albumin. All aforementioned differences were statistically significant (p < 0.05).
HC | NDDM | P | |
Age | 29.59 ± 8.93 | 47.92 ± 14.39 | < 0.001 |
Gender (male%) | 50.0% | 57.8% | < 0.001 |
BMI (kg/m2) | 21.75 ± 2.38 | 25.57 ± 3.99 | < 0.001 |
Waist (cm) | 78.30 ± 8.10 | 91.36 ± 11.60 | < 0.001 |
HbA1c (%) | 5.26 ± 0.27 | 9.47 ± 2.91 | < 0.001 |
Total cholesterol (mmol/L) | 4.59 ± 0.91 | 5.01 ± 1.41 | < 0.001 |
Triglyceride (mmol/L) | 0.94 ± 0.42 | 2.08 ± 2.22 | < 0.001 |
High density lipoprotein (mmol/L) | 1.39 ± 0.35 | 1.10 ± 0.38 | < 0.001 |
Low density lipoprotein (mmol/L) | 2.80 ± 0.62 | 3.27 ± 0.96 | < 0.001 |
Apolipoprotein A (mg/L) | 137.82 ± 134.89 | 213.67 ± 232.43 | < 0.001 |
Alanine aminotransferase (U/L) | 18.54 ± 11.91 | 36.94 ± 34.31 | < 0.001 |
Aspartate aminotransferase (U/L) | 22.49 ± 7.21 | 29.23 ± 21.37 | < 0.001 |
Gamma-glutamyl transpeptidase (U/L) | 18.85 ± 11.07 | 50.14 ± 59.55 | < 0.001 |
Alkaline phosphatase (U/L) | 69.10 ± 20.32 | 95.67 ± 37.60 | < 0.001 |
Uric acid (μmol/L) | 322.58 ± 95.81 | 314.99 ± 92.82 | 0.550 |
Blood urea nitrogen (mmol/L) | 4.98 ± 1.32 | 5.17 ± 1.86 | 0.457 |
Serum creatinine (μmol/L) | 66.01 ± 14.95 | 62.37 ± 14.96 | 0.075 |
Albumin (g/L) | 44.49 ± 3.06 | 40.92 ± 5.64 | < 0.001 |
Fasting C/I and HOMA-IR were hyperbolically dependent in both DM patients and HC (Figure 3), whereas log(C/I) was linearly negatively correlated with log(HOMA-IR). The correlational coefficients were −0.83 in the DM group (p < 0.001) and −0.76 in the HC group (p < 0.001). Thus, CPIRI was constructed using log(C/I) as the independent variable and log(HOMA-IR) as the dependent variable. After excluding patients with incomplete data and those with extreme (1%) outlying data the following equation was obtained after analysis of 740 DM patients:
log(CPIRI)=6.51−2.24×log(C/I)(F=1904.39,p<0.001) | (2) |
After logarithm conversion, the original CPIRI model was obtained:
CPIRI=671.8/(C/I)2.24 | (3) |
When the original values for CPIRI and HOMA-IR in DM patients were paired for analyses using Student's t-test however, the differences in values between the two indices was 0.239 and was significant (t = 4.17, p < 0.001). Correction of the constant and coefficient simplification in the original equation yielded the corrected equation:
CPIRI(DM)=670/(C/I)2.24+0.25 | (4) |
Paired Student's t-test results for CPIRI(DM) and HOMA IR were not statistically significant (t = 0.20, p = 0.84), and the two indicators were significantly correlated in DM patients (Pearson's correlational coefficient = 0.77, p < 0.001).
HC data were analyzed using the CPIRI(DM) model to verify its application in a population with normal glucose tolerance. In a paired t-test there was a significant difference between CPIRI(DM) and HOMA-IR values in HC (t = −11.23, p < 0.001), thus CPIRI(DM) is not suitable for application in healthy populations. Consequently, the following model applicable in healthy populations was constructed:
CPIRI(HC)=670/(C/I)2.24−1 | (5) |
In a paired t-test there was no significant difference between CPIRI(HC) and HOMA IR values in HC (t = −1.22, p = 0.229), and the two indicators were significantly correlated (Pearson's correlational coefficient = 0.80, p < 0.001).
CPIRI and HOMA-IR values were compared in HC and DM subjects. Both indices were significantly higher in DM patients than in HC (CPIRI 2.50 ± 1.80 vs. 1.50 ± 1.44; HOMA-IR 2.50 ± 2.40 vs. 1.42 ± 0.88, p < 0.05) (Figure 4). In general, the novel CPIRI model could be used to distinguish the degree of insulin resistance in HC and DM patients with accuracy identical to that observed with HOMA-IR. There was no significant difference between the two indicators in an identical population, whereas the correlation between them was high. Therefore, a CPIRI model for evaluating DM patients and HC was successfully established.
Whether CPIRI could distinguish different degrees of insulin resistance was then assessed. DM patients were subetaouped based on BMI cutoffs of 24 kg/m2 and 28 kg/m2. CPIRI and HOMA-IR values increased with BMI (CPIRI 2.20 ± 1.84 vs. 2.41 ± 1.59 vs. 2.95 ± 1.85; HOMA-IR 1.82 ± 1.90 vs. 2.45 ± 2.06 vs. 3.34 ± 2.93; p < 0.05) (Figure S1A). CPIRI and HOMA-IR values were higher in DM patients with a history of non-alcoholic fatty liver disease (NAFLD) than in those without a history of NAFLD (CPIRI 2.14 ± 1.36 vs. 1.80 ± 1.23; HOMA-IR 1.89 ± 1.35 vs. 1.40 ± 1.30; p < 0.05) (Figure S1B).
The relationship between insulin-resistant state and CAD severity was investigated. CPIRI was positively associated with increasing extension (1-2-3-vessel disease) in CAD, though not statistically significantly (Figure S2).
Multiple studies indicate that several non-invasive examinations such as echocardiography, electrocardiography, cardiac magnetic resonance imaging, myocardial perfusion imaging, SE, EET, and coronary computed tomography angiography are of diagnostic value in patients with CAD [27]. We previously confirmed that either SE or EET analysis can be used to optimize CAD diagnosis, and that combination analysis may reduce the prevalence of CAD as well as medical costs [2]. In the current study there was a direct association between insulin resistance and CAD determined via multiple imaging modalities. Patients with insulin resistance were more likely to exhibit WMAs than those without insulin resistance via either SE or EET. Given that insulin resistance is likely an independent predictor of the development of cardiovascular disease in the general population [28] and in patients with T2DM [29], a better understanding of how it can be accurately and conveniently calculated could have a profound effect on CAD.
The vast majority of insulin resistance indicators assess the relationship between glucose and insulin, whereas C-peptide is typically used as a marker to indicate β-cell function. Fluctuations in insulin and plasma glucose alone are inadequate as an index of insulin resistance however, especially in patients with T2DM, because β-cell loss and inadequate insulin secretion can lead to inappropriately low insulin concentrations. Given this we attempted to generate a model of insulin resistance incorporating C-peptide.
By virtue of the physiological relationship between C-peptide and insulin metabolism, peripheral molar C/I reflects changes in hepatic metabolic clearance rates of insulin, and it performs best under steady-state conditions due to differences in the half-lives of C-peptide and insulin when they are secreted in response to stimuli [23,30]. The relationship between impaired insulin clearance and T2DM was initially reported in 1949 [31]. Since then, several studies have identified defective insulin clearance as a critical hallmark in the pathogenesis of hyperinsulinemia [32]. Generally speaking, hepatic insulin clearance could precede and therefore contribute to the hyperinsulinemia of obesity, suggesting a mutual interaction between insulin resistance and insulin clearance in the liver [33]. Notably, variation of hepatic glucoregulation and insulin clearance occurred independently of changes in peripheral insulin resistance as measured by the hyperinsulinemic-euglycemic clamp [34]. HOMA-IR reflects the dynamic balance between insulin secretion and hepatic glycogen output, which is closely related to hepatic insulin resistance. Taking this phenomenon into consideration, we believed that the CPIRI may shed new light on the evaluation of insulin resistance with C/I as the independent variable and HOMA-IR as the dependent variable.
In the current study 865 newly diagnosed DM patients and 54 HC were used to create a CPIRI model, and diabetics had more pervasive and severe metabolic abnormalities than individuals with normal glucose levels (Table 1). Because fasting insulin has a skewed distribution, log(HOMA-IR) can normalize the skewness of insulin level [26]. In the present study log(C/I) was linearly negatively correlated with log(HOMA-IR) both in DM patients and HC, with respective correlational coefficients of −0.83 (p < 0.001) and −0.76 (p < 0.001). As a result, we generated the CPIRI as an exponential equation rather than a linear equation. The model applicable for DM patients was CPIRI(DM) = 670/(C/I)2.24 + 0.25, and the model applicable for HC was CPIRI(HC) = 670/(C/I)2.24 – 1. CPIRI and HOMA-IR values were compared in HC and DM patients, and both indices were significantly higher in DM patients (CPIRI 2.50 ± 1.80 vs. 1.50 ± 1.44; HOMA-IR 2.50 ± 2.40 vs. 1.42 ± 0.88; p < 0.05). A novel CPIRI model with accuracy identical to that observed with HOMA-IR was successfully established by estimating the severity of insulin resistance among HC and DM patients.
The CPIRI model can eliminate the effects of fluctuations in plasma glucose as well as glucotoxicity on insulin secretion and insulin resistance levels, because it reflects insulin resistance via dynamic changes in C/I. Based on HOMA-IR the CPIRI was designed and further optimized in the present study, and it can be widely used in epidemiologic studies and clinical research. Calculating the CPIRI also requires fewer blood-collection tubes than HOMA-IR (one instead of two), which may facilitate a more concise practical procedure and improve patient satisfaction; and hence, adherence.
In the current study C-peptide was detected using two monoclonal antibodies that recognize human C-peptide but exhibit cross-reactivity with the C-chain or the cleavage product of proinsulin. The concentration of proinsulin and the cleavage product is 100 fold lower than that of C-peptide. Consequently, cross-reactivity does not significantly influence C-peptide detection [20,35]. Thus the CPIRI is a reliable model for evaluating insulin resistance.
In the present study CPIRI values increased significantly with increasing BMI (2.20 ± 1.84 vs. 2.41 ± 1.59 vs. 2.95 ± 1.85, p < 0.05), and it was higher in DM patients with a history of NAFLD than in those with no history of NAFLD (2.14 ± 1.36 vs. 1.80 ± 1.23, p < 0.05). There is a positive association between BMI and the risk of CAD [36], and previous epidemiological studies have shown that NAFLD is associated with CAD [37]. Consequently, the CPIRI model has important implications in CAD.
Significant correlations between the number of vessels involved and insulin levels have been reported [38,39], suggesting that the severity of atherosclerosis is positively correlated with insulin resistance. In the current study the degree of insulin resistance determined via the CPIRI was positively associated with increasing extension (1-2-3 vessel disease) in CAD. The difference was not statistically significant however, possibly due to the sample size. Consequently, the findings of the present study suggest that insulin resistance might be considered a risk factor for CAD, which is concordant with previous studies [40].
The current study had several limitations. The CPIRI model is not applicable when insulin concentration is extremely low, because the independent variable C/I is a ratio and insulin is the denominator. The sample size was relatively small, limiting conclusive interpretation of the results. Lastly, the cross-sectional study design itself has inherent limitations. Follow-up of the study population will help to clarify cause effect relationships between CPIRI and CAD.
To our knowledge, the current study is the first to evaluate insulin resistance via a combination of C-peptide and insulin levels in both diabetics and subjects with normal glucose tolerance. The combination of a mathematical model of insulin resistance and non-invasive cardiovascular examination is of great clinical value for the prediction of CAD risk, as well as the diagnosis and treatment of CAD events.
This work was supported by grants from the National Natural Science Foundation of China (81530026, 81830023), and Jiangsu Provincial Special Program of Medical Science (BE2017753).
The authors declare that they have no competing interests.
[1] |
D. J. Hunter, K. S. Reddy, Noncommunicable diseases, N. Engl. J. Med., 369 (2013), 1336–1343. doi: 10.1056/NEJMra1109345
![]() |
[2] | Q. Huang, J. H. Wang, D. F. Li, J. H. Zhao, X. J. Feng, N. T. Zhou, Exercise electrocardiography combined with stress echocardiography for predicting myocardial ischemia in adults, Exp. Ther. Med., 21 (2021), 130. |
[3] | K. E. Bornfeldt, I. Tabas, Insulin resistance, hyperglycemia, and atherosclerosis, Cell Metab., 14 (2011), 575-585. |
[4] | F. C. Sasso, P. C. Pafundi, R. Marfella, P. Calabrò, F. Piscione, F. Furbatto, et al., Adiponectin and insulin resistance are related to restenosis and overall new PCI in subjects with normal glucose tolerance: the prospective AIRE Study, Cardiovasc. Diabetol., 18 (2019), 24. |
[5] | K. Esposito, M. Ciotola, F. C. Sasso, D. Cozzolino, F. Saccomanno, R. Assaloni, et al., Effect of a single high-fat meal on endothelial function in patients with the metabolic syndrome: role of tumor necrosis factor-alpha, Nutr. Metab. Cardiovasc. Dis., 17 (2007), 274–279. |
[6] | H. A. Qazmooz, H. N. Smesam, R. F. Mousa, H. K. Al-Hakeim, M. Maes, Trace element, immune and opioid biomarkers of unstable angina, increased atherogenicity and insulin resistance: Results of machine learning, J. Trace. Elem. Med. Biol., 64 (2021), 126703. |
[7] | D. Cozzolino, G. Sessa, T. Salvatore, F.C. Sasso, D. Giugliano, P. J. Lefebvre, R. Torella, et al., The involvement of the opioid system in human obesity: a study in normal weight relatives of obese people, J. Clin. Endocrinol. Metab., 81 (1996), 713–718. |
[8] |
R. Marfella, M. D'. Amico, C. D. Filippo, M. Siniscalchi, F. C. Sasso, F. Ferraraccio, et al., The possible role of the ubiquitin proteasome system in the development of atherosclerosis in diabetes, Cardiovasc. Diabetol., 6 (2007), 35. doi: 10.1186/1475-2840-6-35
![]() |
[9] | D. Torella, G. M. Ellison, M. Torella, C. Vicinanza, I. Aquila, C. Iaconetti, et al., Carbonic anhydrase activation is associated with worsened pathological remodeling in human ischemic diabetic cardiomyopathy, J. Am. Heart Assoc., 3 (2014), e000434. |
[10] |
R. Marfella, F. Ferraraccio, M. R. Rizzo, M. Portoghese, M. Barbieri, C. Basilio, et al., Innate immune activity in plaque of patients with untreated and L-thyroxine-treated subclinical hypothyroidism, J. Clin. Endocrinol. Metab., 96 (2011), 1015–1020. doi: 10.1210/jc.2010-1382
![]() |
[11] |
R. Marfella, F. C. Sasso, M. Siniscalchi, P. Paolisso, M. R. Rizzo, F. Ferraro, et al., Peri-procedural tight glycemic control during early percutaneous coronary intervention is associated with a lower rate of in-stent restenosis in patients with acute ST-elevation myocardial infarction, J. Clin. Endocrinol. Metab., 97 (2012), 2862–2871. doi: 10.1210/jc.2012-1364
![]() |
[12] |
G. Reaven, Insulin resistance and coronary heart disease in nondiabetic individuals, Arterioscler. Thromb. Vasc. Biol., 32 (2012), 1754–1759. doi: 10.1161/ATVBAHA.111.241885
![]() |
[13] |
H. P. Himsworth, Diabetes mellitus: its differentiation into insulin-sensitive and insulin-insensitive types, Int. J. Epidemiol., 42 (2013), 1594–1598. doi: 10.1093/ije/dyt203
![]() |
[14] |
S. E. Kahn, R. L. Hull, K. M. Utzschneider, Mechanisms linking obesity to insulin resistance and type 2 diabetes, Nature, 444 (2006), 840–846. doi: 10.1038/nature05482
![]() |
[15] |
B. B. Kahn, J. S. Flier, Obesity and insulin resistance, J. Clin. Invest., 106 (2000), 473–481. doi: 10.1172/JCI10842
![]() |
[16] | C. J. Ramnanan, D. S. Edgerton, G. Kraft, A. D. Cherrington, Physiologic action of glucagon on liver glucose metabolism, Diabetes Obes. Metab., 13 (2011), 118–125. |
[17] |
H. V. Lin, D. Accili, Hormonal regulation of hepatic glucose production in health and disease, Cell Metab., 14 (2011), 9–19. doi: 10.1016/j.cmet.2011.06.003
![]() |
[18] |
D. R. Matthews, J. P. Hosker, A. S. Rudenski, B. A. Naylor, D. F. Treacher, R. C. Turner, Homeostasis model assessment: insulin resistance and beta-cell function from fasting plasma glucose and insulin concentrations in man, Diabetologia, 28 (1985), 412–419. doi: 10.1007/BF00280883
![]() |
[19] |
T. M. Wallace, J. C. Levy, D. R. Matthews, Use and abuse of HOMA modeling, Diabetes Care, 27 (2004), 1487–1495. doi: 10.2337/diacare.27.6.1487
![]() |
[20] |
J. Wahren, A. Kallas, A. A. Sima, The clinical potential of C-peptide replacement in type 1 diabetes, Diabetes, 61 (2012), 761–772. doi: 10.2337/db11-1423
![]() |
[21] |
O. K. Faber, H. Kehlet, S. Madsbad, C. Binder, Kinetics of human C-peptide in man, Diabetes, 27 (1978), 207–209. doi: 10.2337/diab.27.1.S207
![]() |
[22] |
G. L. C. Yosten, C. Maric-Bilkan, P. Luppi, J. Wahren, Physiological effects and therapeutic potential of proinsulin C-peptide, Am. J. Physiol. Endocrinol. Metab., 307 (2014), E955–968. doi: 10.1152/ajpendo.00130.2014
![]() |
[23] |
R. R. Henry, G. Brechtel, K. Griver, Secretion and hepatic extraction of insulin after weight loss in obese noninsulin-dependent diabetes mellitus, J. Clin. Endocrinol. Metab., 66 (1988), 979–986. doi: 10.1210/jcem-66-5-979
![]() |
[24] | J. Jimenez, S. Zuniga-Guajardo, B. Zinman, A. Angel, Effects of weight loss in massive obesity on insulin and C-peptide dynamics: sequential changes in insulin production, clearance, and sensitivity, J. Clin. Endocrinol. Metab., 64 (1987), 661–668. |
[25] | M. T. Meistas, S. Margolis, A. A. Kowarski, Hyperinsulinemia of obesity is due to decreased clearance of insulin, Am. J. Physiol., 245 (1983), E155–159. |
[26] | A. Katz, S. S. Nambi, K. Mather, A. D. Baron, D. A. Follmann, G. Sullivan, et al., Quantitative insulin sensitivity check index: a simple, accurate method for assessing insulin sensitivity in humans, J. Clin. Endocrinol. Metab., 85 (2000), 2402–2410. |
[27] |
I. R. Mordi, A. A. Badar, R. J. Irving, J. R. Weir-McCall, J. G. Houston, C. C. Lang, Efficacy of noninvasive cardiac imaging tests in diagnosis and management of stable coronary artery disease, Vasc. Health Risk Manag., 13 (2017), 427–437. doi: 10.2147/VHRM.S106838
![]() |
[28] |
E. Bonora, S. Kiechl, J. Willeit, F. Oberhollenzer, G. Egger, J. B. Meigs, et al., Insulin resistance as estimated by homeostasis model assessment predicts incident symptomatic cardiovascular disease in caucasian subjects from the general population: the Bruneck study, Diabetes Care, 30 (2007), 318–324. doi: 10.2337/dc06-0919
![]() |
[29] |
E. Bonora, G. Formentini, F. Calcaterra, S. Lombardi, F. Marini, L. Zenari, et al., HOMA-estimated insulin resistance is an independent predictor of cardiovascular disease in type 2 diabetic subjects: prospective data from the Verona Diabetes Complications Study, Diabetes Care, 25 (2002), 1135–1141. doi: 10.2337/diacare.25.7.1135
![]() |
[30] |
K. S. Polonsky, A. H. Rubenstein, C-peptide as a measure of the secretion and hepatic extraction of insulin: Pitfalls and limitations, Diabetes, 33 (1984), 486–494. doi: 10.2337/diab.33.5.486
![]() |
[31] | R. H. Broh-Kahn, I. A. Mirsky, The inactivation of insulin by tissue extracts; the effect of fasting on the insulinase content of rat liver, Arch. Biochem., 20 (1949), 10–14. |
[32] |
O. Pivovarova, W. Bernigau, T. Bobbert, F. Isken, M. Möhlig, J. Spranger, et al., Hepatic insulin clearance is closely related to metabolic syndrome components, Diabetes Care, 36(2013), 3779–3785. doi: 10.2337/dc12-1203
![]() |
[33] | C. W. Hilton, H. Mizuma, F. Svec, C. Prasad, Relationship between plasma cyclo (His-Pro), a neuropeptide common to processed protein-rich food, and C-peptide/insulin molar ratio in obese women, Nutr. Neurosci., 4 (2001), 469–474. |
[34] |
A. M. Lundsgaard, K. A. Sjøberg, L. D. Høeg, J. Jeppesen, A. B. Jordy, A. K. Serup, et al., Opposite Regulation of Insulin Sensitivity by Dietary Lipid Versus Carbohydrate Excess, Diabetes, 66 (2017), 2583–2595. doi: 10.2337/db17-0046
![]() |
[35] |
T. M. Zilkens, A. M. Eberle, H. Schmidt-Gayk, Immunoluminometric assay (ILMA) for intact human proinsulin and its conversion intermediates, Clin. Chim. Acta., 247 (1996), 23–37. doi: 10.1016/0009-8981(95)06217-3
![]() |
[36] |
N. Li, P. T. Katzmarzyk, R. Horswell, Y. Zhang, W. Li, W. Zhao, et al., BMI and coronary heart disease risk among low-income and underinsured diabetic patients, Diabetes Care, 37 (2014), 3204–3212. doi: 10.2337/dc14-1091
![]() |
[37] |
M. C. G. J. Brouwers, N. Simons, C. D. A. Stehouwer, A. Isaacs, Non-alcoholic fatty liver disease and cardiovascular disease: assessing the evidence for causality, Diabetologia, 63 (2020), 253–260. doi: 10.1007/s00125-019-05024-3
![]() |
[38] |
I. Kowalska, J. Prokop, H. Bachórzewska-Gajewska, B. Telejko, I. Kinalskal, W. Kochman, et al., Disturbances of glucose metabolism in men referred for coronary arteriography: Postload glycemia as predictor for coronary atherosclerosis, Diabetes Care, 24 (2001), 897–901. doi: 10.2337/diacare.24.5.897
![]() |
[39] |
F. C. Sasso, O. Carbonara, R. Nasti, B. Campana, R. Marfella, M. Torella, et al., Glucose metabolism and coronary heart disease in patients with normal glucose tolerance, Jama, 291 (2004), 1857–1863. doi: 10.1001/jama.291.15.1857
![]() |
[40] |
T. Strisciuglio, R. Izzo, E. Barbato, G. D. Gioia, I. Colaiori, A. Fiordelisi, et al., Insulin resistance predicts severity of coronary atherosclerotic disease in non-diabetic patients, J. Clin. Med., 9 (2020), 2144. doi: 10.3390/jcm9072144
![]() |
![]() |
![]() |
HC | NDDM | P | |
Age | 29.59 ± 8.93 | 47.92 ± 14.39 | < 0.001 |
Gender (male%) | 50.0% | 57.8% | < 0.001 |
BMI (kg/m2) | 21.75 ± 2.38 | 25.57 ± 3.99 | < 0.001 |
Waist (cm) | 78.30 ± 8.10 | 91.36 ± 11.60 | < 0.001 |
HbA1c (%) | 5.26 ± 0.27 | 9.47 ± 2.91 | < 0.001 |
Total cholesterol (mmol/L) | 4.59 ± 0.91 | 5.01 ± 1.41 | < 0.001 |
Triglyceride (mmol/L) | 0.94 ± 0.42 | 2.08 ± 2.22 | < 0.001 |
High density lipoprotein (mmol/L) | 1.39 ± 0.35 | 1.10 ± 0.38 | < 0.001 |
Low density lipoprotein (mmol/L) | 2.80 ± 0.62 | 3.27 ± 0.96 | < 0.001 |
Apolipoprotein A (mg/L) | 137.82 ± 134.89 | 213.67 ± 232.43 | < 0.001 |
Alanine aminotransferase (U/L) | 18.54 ± 11.91 | 36.94 ± 34.31 | < 0.001 |
Aspartate aminotransferase (U/L) | 22.49 ± 7.21 | 29.23 ± 21.37 | < 0.001 |
Gamma-glutamyl transpeptidase (U/L) | 18.85 ± 11.07 | 50.14 ± 59.55 | < 0.001 |
Alkaline phosphatase (U/L) | 69.10 ± 20.32 | 95.67 ± 37.60 | < 0.001 |
Uric acid (μmol/L) | 322.58 ± 95.81 | 314.99 ± 92.82 | 0.550 |
Blood urea nitrogen (mmol/L) | 4.98 ± 1.32 | 5.17 ± 1.86 | 0.457 |
Serum creatinine (μmol/L) | 66.01 ± 14.95 | 62.37 ± 14.96 | 0.075 |
Albumin (g/L) | 44.49 ± 3.06 | 40.92 ± 5.64 | < 0.001 |
HC | NDDM | P | |
Age | 29.59 ± 8.93 | 47.92 ± 14.39 | < 0.001 |
Gender (male%) | 50.0% | 57.8% | < 0.001 |
BMI (kg/m2) | 21.75 ± 2.38 | 25.57 ± 3.99 | < 0.001 |
Waist (cm) | 78.30 ± 8.10 | 91.36 ± 11.60 | < 0.001 |
HbA1c (%) | 5.26 ± 0.27 | 9.47 ± 2.91 | < 0.001 |
Total cholesterol (mmol/L) | 4.59 ± 0.91 | 5.01 ± 1.41 | < 0.001 |
Triglyceride (mmol/L) | 0.94 ± 0.42 | 2.08 ± 2.22 | < 0.001 |
High density lipoprotein (mmol/L) | 1.39 ± 0.35 | 1.10 ± 0.38 | < 0.001 |
Low density lipoprotein (mmol/L) | 2.80 ± 0.62 | 3.27 ± 0.96 | < 0.001 |
Apolipoprotein A (mg/L) | 137.82 ± 134.89 | 213.67 ± 232.43 | < 0.001 |
Alanine aminotransferase (U/L) | 18.54 ± 11.91 | 36.94 ± 34.31 | < 0.001 |
Aspartate aminotransferase (U/L) | 22.49 ± 7.21 | 29.23 ± 21.37 | < 0.001 |
Gamma-glutamyl transpeptidase (U/L) | 18.85 ± 11.07 | 50.14 ± 59.55 | < 0.001 |
Alkaline phosphatase (U/L) | 69.10 ± 20.32 | 95.67 ± 37.60 | < 0.001 |
Uric acid (μmol/L) | 322.58 ± 95.81 | 314.99 ± 92.82 | 0.550 |
Blood urea nitrogen (mmol/L) | 4.98 ± 1.32 | 5.17 ± 1.86 | 0.457 |
Serum creatinine (μmol/L) | 66.01 ± 14.95 | 62.37 ± 14.96 | 0.075 |
Albumin (g/L) | 44.49 ± 3.06 | 40.92 ± 5.64 | < 0.001 |