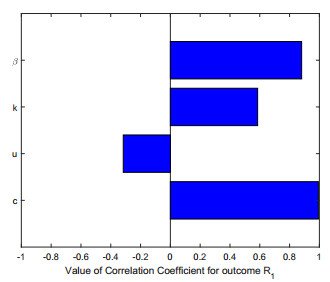
Citation: Jian Ren, Rui Xu, Liangchen Li. Global stability of an HIV infection model with saturated CTL immune response and intracellular delay[J]. Mathematical Biosciences and Engineering, 2021, 18(1): 57-68. doi: 10.3934/mbe.2021003
[1] | Xuejuan Lu, Lulu Hui, Shengqiang Liu, Jia Li . A mathematical model of HTLV-I infection with two time delays. Mathematical Biosciences and Engineering, 2015, 12(3): 431-449. doi: 10.3934/mbe.2015.12.431 |
[2] | Cuicui Jiang, Kaifa Wang, Lijuan Song . Global dynamics of a delay virus model with recruitment and saturation effects of immune responses. Mathematical Biosciences and Engineering, 2017, 14(5&6): 1233-1246. doi: 10.3934/mbe.2017063 |
[3] | A. M. Elaiw, A. S. Shflot, A. D. Hobiny . Stability analysis of general delayed HTLV-I dynamics model with mitosis and CTL immunity. Mathematical Biosciences and Engineering, 2022, 19(12): 12693-12729. doi: 10.3934/mbe.2022593 |
[4] | Ting Guo, Zhipeng Qiu . The effects of CTL immune response on HIV infection model with potent therapy, latently infected cells and cell-to-cell viral transmission. Mathematical Biosciences and Engineering, 2019, 16(6): 6822-6841. doi: 10.3934/mbe.2019341 |
[5] | Jiawei Deng, Ping Jiang, Hongying Shu . Viral infection dynamics with mitosis, intracellular delays and immune response. Mathematical Biosciences and Engineering, 2023, 20(2): 2937-2963. doi: 10.3934/mbe.2023139 |
[6] | Xiaohong Tian, Rui Xu, Jiazhe Lin . Mathematical analysis of an age-structured HIV-1 infection model with CTL immune response. Mathematical Biosciences and Engineering, 2019, 16(6): 7850-7882. doi: 10.3934/mbe.2019395 |
[7] | Pensiri Yosyingyong, Ratchada Viriyapong . Global dynamics of multiple delays within-host model for a hepatitis B virus infection of hepatocytes with immune response and drug therapy. Mathematical Biosciences and Engineering, 2023, 20(4): 7349-7386. doi: 10.3934/mbe.2023319 |
[8] | Yu Yang, Gang Huang, Yueping Dong . Stability and Hopf bifurcation of an HIV infection model with two time delays. Mathematical Biosciences and Engineering, 2023, 20(2): 1938-1959. doi: 10.3934/mbe.2023089 |
[9] | Cameron Browne . Immune response in virus model structured by cell infection-age. Mathematical Biosciences and Engineering, 2016, 13(5): 887-909. doi: 10.3934/mbe.2016022 |
[10] | Yan Wang, Minmin Lu, Daqing Jiang . Viral dynamics of a latent HIV infection model with Beddington-DeAngelis incidence function, B-cell immune response and multiple delays. Mathematical Biosciences and Engineering, 2021, 18(1): 274-299. doi: 10.3934/mbe.2021014 |
Mathematical modeling and analysis of HIV pathogenesis are crucial in understanding the transmission mechanism of HIV. Many authors are interested in studying the global stability of HIV infection models (see, for example, [1,2,3,4]). In addition, the analysis of mathematical models contributes to developing new antiviral drugs and designing effective therapies for HIV infection (see, for example, [5,6,7]). Since cytotoxic T lymphocytes (CTLs) play a significant role in the within-host anti-HIV defense by attacking infected cells in HIV infection, in 1996, Nowak and Bangham [8] proposed the following HIV model describing the interaction between host cells, free viruses and CTLs:
˙x(t)=λ−dx(t)−βx(t)v(t),˙y(t)=βx(t)v(t)−ay(t)−py(t)z(t),˙v(t)=ky(t)−uv(t),˙z(t)=cy(t)z(t)−bz(t). | (1.1) |
Here, x(t), y(t), v(t) and z(t) are the concentrations of uninfected cells, infected cells, free viruses and CTLs at time t, respectively. The parameter λ denotes the production rate of uninfected cells. Uninfected cells, infected cells, free viruses and CTLs die at rate dx(t), ay(t), uv(t) and bz(t), respectively. The term βx(t)v(t) is the rate at which uninfected cells are infected by free viruses, and the term py(t)z(t) represents the rate for infected cells to be killed by CTLs. Free viruses are released from infected cells at rate ky(t) and CTL immune response is activated by infected cells at rate cy(t)z(t).
We note that system (1.1) assumes that CTL immune response is activated at bilinear rate. However, in [9], De Boer suggested that bilinear rate cannot model several immune responses that are together controlling a chronic infection, and proposed immune response functions with saturation effect. In [10], Jiang and Wang considered saturated immune response function cy(t)z(t)/(h+z(t)) to replace the bilinear rate, here h is a saturation constant.
It is assumed in system (1.1) that as soon as the free viruses enter a target cell, the target cell is immediately infected and new free viruses are produced simultaneously. In [11], a mathematical model with the intracellular phase of the viral life-cycle was first proposed by Herz et al. There is a fixed time delay τ between infection of a cell and production of new free viruses. Some researchers have incorporated the intracellular delay into HIV infection models and investigated the effect of the intracellular delay on HIV infection dynamics (see, for example, [12,13,14,15]).
On the other hand, system (1.1) assumes that the infection rate of virus-to-cell is bilinear. In fact, however, in [16], Ebert et al. observed a nonlinear relationship between parasite dose and infection rate in experiments. Furthermore, in [17], Regoes et al. showed that the infection rate is a sigmoidal function. In [18], Song and Neumann considered the infection rate with saturation effect, βxvp/(1+αvq), where p, q and α are positive constants, and investigated the global stability of the viral model with saturated infection rate βxv/(1+αv).
Inspired by the biological reasons mentioned above, in this paper, we study the effect of saturated infection rate, intracellular delay and saturated CTL immune response on the dynamics of HIV infection. To this end, we consider the following delay differential equations:
˙x(t)=λ−dx(t)−βx(t)v(t)1+αv(t),˙y(t)=βe−mτx(t−τ)v(t−τ)1+αv(t−τ)−ay(t)−py(t)z(t),˙v(t)=ky(t)−uv(t),˙z(t)=cy(t)z(t)h+z(t)−bz(t). | (1.2) |
Here, τ denotes the time between viral entry into a cell and production of free viruses; e−mτ is the probability of cellular survival from time t−τ to time t. All parameters of system (1.2) are positive constants.
We assume that the initial condition of system (1.2) satisfies:
x(θ)=ϕ1(θ), y(0)=y0>0, v(θ)=ϕ2(θ), z(0)=z0>0,ϕ1(θ)≥0, ϕ2(θ)≥0, θ∈[−τ,0), ϕi(0)>0 (i=1,2), | (1.3) |
where (ϕ1(θ),ϕ2(θ))∈C([−τ,0],R2+0), R2+0={(x1,x2):xi≥0,i=1,2}.
According to the basic theory of functional differential equations [19], we can prove that system (1.2) has a unique solution (x(t),y(t),v(t),z(t)) that satisfies the initial condition (1.3). Furthermore, it is easy to show that under the initial condition (1.3), all solutions of system (1.2) are defined on [0,+∞) and are positive for all t≥0.
The main goal of this paper is to make a complete mathematical analysis of system (1.2) and investigate its dynamical behaviors. This paper is organized as follows. In the next section, we calculate the reproduction numbers of system (1.2) and discuss the existence of feasible equilibria. In Section 3, we study the local asymptotic stability of each of feasible equilibria. The global asymptotic stability of each of feasible equilibria is investigated in Section 4. In Section 5, we perform sensitivity analyses to illustrate the effects of parameters on the immunity-activated reproduction number R1 and the viral load.
It is clear that system (1.2) always admits an infection-free equilibrium E0(λ/d,0,0,0).
Now, we calculate the immunity-inactivated reproduction number of system (1.2). According to the method of next generation matrix proposed by van den Driessche and Watmough [20], we have
F=(βe−mτx(t−τ)v(t−τ)1+αv(t−τ)0) and V=(ay(t)+py(t)z(t)−ky(t)+uv(t)). |
Sequentially, we obtain that
F=(0βλe−mτd00) and V=(a0−ku). |
Hence the next generation matrix is
FV−1=(βkλe−mτaudβλe−mτud00). |
We therefore derive the immunity-inactivated reproduction number
R0=ρ(FV−1)=βkλe−mτaud, |
representing the number of second infected cells produced by a infective cell in a whole susceptible population [20]. It is easy to prove that if R0>1, system (1.2) has a unique immunity-inactivated equilibrium E1(x1,y1,v1,0), here
x1=λ(u+αky1)d(u+αky1)+βky1, v1=ky1u, y1=ud(R0−1)k(αd+β). |
Denote
R1=cud(R0−1)bkh(αd+β), |
where R1 is called immunity-activated reproduction number of system (1.2). Besides, we can show that if R1>1, system (1.2) has an immunity-activated equilibrium E2(x2,y2,v2,z2), where
x2=(a+pz2)(cu+αbk(h+z2))cβke−mτ, y2=b(h+z2)c, v2=bk(h+z2)cu, |
and
z2=−(bk(αd+β)(a+hp)+cdpu)+√Δ2bpk(αd+β), |
here,
Δ=(bk(αd+β)(a+hp)+cdpu)2+4ab2k2hp(αd+β)2(R1−1). |
In this section, we study the local dynamics of system (1.2).
Theorem 3.1. If R0<1, the infection-free equilibrium E0(λ/d,0,0,0) of system (1.2) is locally asymptotically stable; if R0>1, E0 is unstable.
Proof. By calculation, we have the following characteristic equation of system (1.2) at E0:
(ξ+b)(ξ+d)[(ξ+a)(ξ+u)−βkλe−(m+ξ)τd]=0. | (3.1) |
Clearly, Eq. (3.1) has negative real roots ξ=−b and ξ=−d, and other roots are governed by the following equation:
(ξ+a)(ξ+u)−βkλe−(m+ξ)τd=0. | (3.2) |
Substituting R0 into Eq. (3.2), we can rewrite (3.2) as
(ξa+1)(ξu+1)=R0e−ξτ. | (3.3) |
Next, we verify that all roots of Eq. (3.3) have negative real parts. By contradiction, let ξ1=Reξ1+iImξ1 with Reξ1≥0 be a root of Eq. (3.3). It follows that
|ξ1a+1|≥1>R0, |ξ1u+1|≥|e−ξ1τ|. |
Further, we have
|(ξ1a+1)(ξ1u+1)|>|R0e−ξ1τ|, |
which contradicts (3.3). Hence, we conclude that if R0<1, all roots of Eq. (3.1) have negative real parts. Accordingly, E0 is locally asymptotically stable. On the other hand, if R0>1, we denote
G(ξ)=(ξ+a)(ξ+u)−βkλe−(m+ξ)τd. | (3.4) |
It is easy to see that G(0)=au(1−R0)<0 and G(ξ)→+∞ as ξ→+∞. Noting that G(ξ) is a continuous function in respect to ξ, it follows that if R0>1, Eq. (3.1) has a positive real root. Thus, E0 is unstable.
Theorem 3.2. If R1<1<R0, the immunity-inactivated equilibrium E1(x1,y1,v1,0) of system (1.2) is locally asymptotically stable.
Proof. By calculation, we have the following characteristic equation of system (1.2) at E1:
[ξ−(cy1h−b)][(ξ+d+βv11+αv1)(ξ+a)(ξ+u)−βkx1e−(m+ξ)τ(1+αv1)2(ξ+d)]=0. | (3.5) |
Since
R1=1b(cy1h−b)+1<1, | (3.6) |
Eq. (3.5) has a negative real root ξ=cy1h−b, and the remaining roots are determined by the following equation:
(ξ+d+βv11+αv1)(ξ+a)(ξ+u)=(ξ+d)βkx1e−(m+ξ)τ(1+αv1)2. | (3.7) |
We claim that all roots of Eq. (3.7) have negative real parts. Assume the contrary, Eq. (3.7) has a root ξ2=Reξ2+iImξ2 with Reξ2≥0. It is easy to show that
|(ξ2+a)(ξ2+u)|≥au=βkx1e−mτ1+αv1>βkx1e−mτ(1+αv1)2. | (3.8) |
Furthermore, we have
|ξ2+dξ2+d+βv11+αv1βkx1e−(m+ξ2)τ(1+αv1)2|<βkx1e−mτ(1+αv1)2, |
which contradicts (3.7). Consequently, if R1<1<R0, all roots of Eq. (3.5) have negative real parts. Accordingly, E1 is locally asymptotically stable.
Theorem 3.3. If R1>1, the immunity-activated equilibrium E2(x2,y2,v2,z2) of system (1.2) is locally asymptotically stable.
Proof. The characteristic equation of system (1.2) at E2 is:
(ξ+d+βv21+αv2)(ξ+u)[pcy2z2h+z2+(ξ+b−chy2(h+z2)2)(ξ+a+pz2)]=(ξ+d)(ξ+b−chy2(h+z2)2)βkx2e−(m+ξ)τ(1+αv2)2. | (3.9) |
In the following, we show that all roots of Eq. (3.9) have negative real parts. If Eq. (3.9) has a root ξ3 with nonnegative real part, we obtain that
|ξ3+dξ3+d+βv21+αv2(ξ3+b−chy2(h+z2)2)βkx2e−(m+ξ3)τ(1+αv2)2|<βkx2e−mτ(1+αv2)2|ξ3+b−chy2(h+z2)2|. |
From the expression of E2, we derive that u(a+pz2)=βkx2e−mτ1+αv2. Thus, we have
|(ξ3+u)[pcy2z2h+z2+(ξ3+b−chy2(h+z2)2)(ξ3+a+pz2)]|>u(a+pz2)|ξ3+b−chy2(h+z2)2|>βkx2e−mτ(1+αv2)2|ξ3+b−chy2(h+z2)2|. | (3.10) |
This leads to a contradiction. Therefore, all roots of Eq. (3.9) have negative real parts if R1>1. Accordingly, E2 is locally asymptotically stable.
In this section, we establish the global dynamics of system (1.2).
Now, we discuss the boundedness of solutions, and have the following result.
Lemma 4.1. All solutions of system (1.2) with initial condition (1.3) are ultimately bounded for all t≥0.
Proof. Let (x(t),y(t),v(t),z(t)) be any solution of system (1.2) satisfying the initial condition (1.3). Denote B(t)=x(t−τ)+emτy(t).
Differentiating B(t) with respect to t, we obtain that
˙B(t)=λ−dx(t−τ)−aemτy(t)−pemτy(t)z(t)≤λ−[dx(t−τ)+aemτy(t)]≤λ−min{a,d}B(t), |
which implies that lim supt→∞B(t)≤λmin{a,d}. Then x(t) and y(t) are ultimately bounded. From the third and the fourth equations of system (1.2), we derive that
˙v(t)=ky(t)−uv(t)≤ke−mτλmin{a,d}−uv(t), |
˙z(t)≤cy(t)−bz(t)≤ce−mτλmin{a,d}−bz(t), |
and thus
lim supt→∞v(t)≤ke−mτλumin{a,d}, lim supt→∞z(t)≤ce−mτλbmin{a,d}. |
Hence v(t) and z(t) are ultimately bounded. Therefore, the positively invariant set for system (1.2) is given by:
Ω={(x,y,v,z)|x+emτy≤λmin{a,d},v≤ke−mτλumin{a,d},z≤ce−mτλbmin{a,d}}. |
Next, we define a function g(x)=x−1−lnx, where g(1)=0 and g(x) attains its minimum at x=1.
Theorem 4.2. If R0<1, the infection-free equilibrium E0(λ/d,0,0,0) of system (1.2) is globally asymptotically stable.
Proof. Let (x(t),y(t),v(t),z(t)) be any positive solution of system (1.2) satisfying the initial condition (1.3). Define
V1(t)=x−x0−x0lnx(t)x0+emτy(t)+aemτkv(t)+phemτcz(t)+∫tt−τβx(s)v(s)1+αv(s)ds, |
where x0=λ/d. Calculating the time derivative of V1(t) along positive solutions of system (1.2), we have
˙V1(t)=dx0(2−x(t)x0−x0x(t))+βx0v(t)1+αv(t)−auemτkv(t)−phbemτcz(t)+pemτ(hh+z(t)−1)y(t)z(t)≤dx0(2−x(t)x0−x0x(t))+(R0−1)auemτkv(t)−phbemτcz(t)+pemτ(hh+z(t)−1)y(t)z(t). | (4.1) |
Since R0<1, we have ˙V1(t)≤0, and ˙V1(t)=0 if and only if x=x0, y=v=z=0. It is easy to show that M0={E0}⊂Ω is the largest invariant subset of {(x(t),y(t),v(t),z(t)):˙V1(t)=0}. In addition, from Theorem 3.1, we know that if R0<1, E0 is locally asymptotically stable. Thus, by LaSalle's invariance principle [19], we conclude that E0 is globally asymptotically stable.
Theorem 4.3. If R1<1<R0, the immunity-inactivated equilibrium E1(x1,y1,v1,0) of system (1.2) is globally asymptotically stable.
Proof. Let (x(t),y(t),v(t),z(t)) be any positive solution of system (1.2) satisfying the initial condition (1.3). Define
V2(t)=x(t)−x1−x1lnx(t)x1+emτ(y(t)−y1−y1lny(t)y1)+βx1v1ky1(1+αv1)(v(t)−v1−v1lnv(t)v1)+pemτy1bz(t)+βx1v11+αv1∫tt−τg(x(t)v(t)(1+αv1)x1v1(1+αv(t)))ds. |
Differentiating V2(t) along positive solutions of system (1.2), we obtain that
˙V2(t)=(1−x1x(t))(λ−dx(t)−βx(t)v(t)1+αv(t))+emτ(1−y1y(t))(βe−mτx(t−τ)v(t−τ)1+αv(t−τ)−ay(t)−py(t)z(t))+βx1v1ky1(1+αv1)(1−v1v(t))(ky(t)−uv(t))+pemτy1b(cy(t)z(t)h+z(t)−bz(t))+βx1v11+αv1[g(x(t)v(t)(1+αv1)x1v1(1+αv(t)))−g(x(t−τ)v(t−τ)(1+αv1)x1v1(1+αv(t−τ)))]. | (4.2) |
Combining λ=dx1+βx1v11+αv1, βe−mτx1v11+αv1=ay1 and ky1=uv1 with (4.2), we have
˙V2(t)=dx1(2−x(t)x1−x1x(t))−βx1v11+αv1[g(x1x(t))+g(v1y(t)y1v(t))]−βx1v11+αv1[g(x(t−τ)v(t−τ)(1+αv1)y1x1v1(1+αv(t−τ))y(t))+g(1+αv(t)1+αv1)]−βx1v11+αv1α(v(t)−v1)2v1(1+αv1)(1+αv(t))+pemτb(cy1h+z(t)−b)y(t)z(t). | (4.3) |
From (3.6), we have cy1h+z(t)−b<cy1h−b<0. Thus ˙V2(t)≤0, and ˙V2(t)=0 if and only if x=x1, y=y1, v=v1, z=0. It is easy to see that the largest invariant subset of {(x(t),y(t),v(t),z(t)):˙V2(t)=0} is the singleton M1={E1}⊂Ω. Furthermore, from Theorem 3.2, we know that if R1<1<R0, E1 is locally asymptotically stable. According to LaSalle's invariance principle [19], E1 is globally asymptotically stable.
Theorem 4.4. If R1>1, the immunity-activated equilibrium E2(x2,y2,v2,z2) of system (1.2) is globally asymptotically stable.
Proof. Let (x(t),y(t),v(t),z(t)) be any positive solution of system (1.2) satisfying the initial condition (1.3). Define
V3(t)=x−x2−x2lnx(t)x2+emτ(y(t)−y2−y2lny(t)y2)+βx2v2ky2(1+αv2)(v(t)−v2−v2lnv(t)v2)+pemτy2b(z(t)−z2−z2lnz(t)z2)+βx2v21+αv2∫tt−τg(x(t)v(t)(1+αv2)x2v2(1+αv(t)))ds. |
Calculating the derivative of V3(t) along positive solutions of system (1.2), we obtain that
˙V3(t)=(1−x2x(t))(λ−dx(t)−βx(t)v(t)1+αv(t))+emτ(1−y2y(t))(βe−mτx(t−τ)v(t−τ)1+αv(t−τ)−ay(t)−py(t)z(t))+βx2v2ky2(1+αv2)(1−v2v(t))(ky(t)−uv(t))+pemτy2b(1−z2z(t))(cy(t)z(t)h+z(t)−bz(t))+βx2v21+αv2[g(x(t)v(t)(1+αv2)x2v2(1+αv(t)))−g(x(t−τ)v(t−τ)(1+αv2)x2v2(1+αv(t−τ)))]. | (4.4) |
Substituting λ=dx2+βx2v21+αv2, βe−mτx2v21+αv2=ay2+py2z2, ky2=uv2 and cy2z2h+z2=bz2 into (4.4), we get
˙V3(t)=dx2(2−x(t)x2−x2x(t))−βx2v21+αv2[g(x2x(t))+g(v2y(t)y2v(t))]−βx2v21+αv2[g(x(t−τ)v(t−τ)(1+αv2)y2x2v2(1+αv(t−τ))y(t))+g(1+αv(t)1+αv2)]−βx2v21+αv2α(v(t)−v2)2v2(1+αv2)(1+αv(t))−pemτ(z(t)−z2)2h+z(t)y(t). | (4.5) |
Obviously, ˙V3(t)≤0 and ˙V3(t)=0 if and only if x=x2, y=y2, v=v2, z=z2. M2={E2}⊂Ω is the largest invariant subset of {(x(t),y(t),v(t),z(t)):˙V3(t)=0}. From Theorem 3.3, if R1>1, E2 is locally asymptotically stable. Hence, using LaSalle's invariance principle [19], we obtain that E2 is globally asymptotically stable.
In this section, we perform sensitivity analyses to show the effects of parameters β, k, u and c on the immunity-activated reproduction number R1 and the viral load.
We choose the parameter values as follows [21,22,23]:
λ=46 cells ml−1 day−1, d=0.0046 day−1, β=4.8×10−7 ml virion−1 day−1,m=1.39 day−1, τ=0.5 day, a=0.01 day−1, p=0.00094 ml cells−1 day−1,k=11.349 virion cells−1 day−1, u=0.25 day−1, c=0.01 day−1, b=0.5 day−1. | (5.1) |
Meanwhile, we assume α=0.15 ml virion−1 and h=0.01 cells ml−1.
First, using the methods of Latin Hypercube Sampling and Partial Rank Correlation Coefficients (PRCCs) developed in [24], we investigate the sensitivity of the immunity-activated reproduction number R1 on the parameters β, k, u and c, which have important effects on HIV infection (see Figure 1). From Figure 1, we see that β, k and c are positively correlated with R1 while u are negatively correlated with R1. In addition, we obtain that decreasing the activation rate of CTL immune response and the infection rate of virus-to-cell infection can more effectively reduce R1.
Second, we study the sensitivity of the viral load with respect to the parameters β, k, u and c (see Figure 2). In Figure 2, it is easy to see that β, k and c are positively correlated with the viral load while u are negatively correlated with the viral load. Besides, we get that decreasing the release rate of free viruses or increasing the death rate of free viruses can more effectively reduce the viral load, which is helpful in controling the viral infection.
The authors wish to thank the Editor and the reviewers for their valuable comments that greatly improved the presentation of this work.
This work was supported by the National Natural Science Foundation of China (Nos. 11871316, 11801340), and the Natural Science Foundation of Shanxi Province (Nos. 201801D121006, 201801D221007).
The authors have no conflicts of interest in this paper.
[1] |
A. M. Elaiw, Global properties of a class of HIV models, Nonlinear Anal. RWA, 11 (2010), 2253-2263. doi: 10.1016/j.nonrwa.2009.07.001
![]() |
[2] |
L. Wang, M. Y. Li, Mathematical analysis of the global dynamics of a model for HIV infection of CD4+ T cells, Math. Biosci., 200 (2006), 44-57. doi: 10.1016/j.mbs.2005.12.026
![]() |
[3] |
L. Cai, X. Li, M. Ghosh, B. Guo, Stability analysis of an HIV/AIDS epidemic model with treatment, J. Comput. Appl. Math., 229 (2009), 313-323. doi: 10.1016/j.cam.2008.10.067
![]() |
[4] | J. Xu, Y. Geng, Y. Zhou, Global dynamics for an age-structured HIV virus infection model with cellular infection and antiretroviral therapy, Appl. Math. Comput., 305 (2017), 62-83. |
[5] |
M. A. Nowak, S. Bonhoeffer, G. M. Shaw, R. M. May, Anti-viral drug treatment: dynamics of resistance in free virus and infected cell populations, J. Theor. Biol., 184 (1997), 203-217. doi: 10.1006/jtbi.1996.0307
![]() |
[6] |
M. Y. Li, L. Wang, Backward bifurcation in a mathematical model for HIV infection in vivo with anti-retroviral treatment, Nonlinear Anal. RWA, 17 (2014), 147-160. doi: 10.1016/j.nonrwa.2013.11.002
![]() |
[7] |
G. P. Samanta, Permanence and extinction of a nonautonomous HIV/AIDS epidemic model with distributed time delay, Nonlinear Anal. RWA, 12 (2011), 1163-1177. doi: 10.1016/j.nonrwa.2010.09.010
![]() |
[8] |
M. A. Nowak, C. R. M. Bangham, Population dynamics of immune responses to persistent viruses, Science, 272 (1996), 74-79. doi: 10.1126/science.272.5258.74
![]() |
[9] | R. De Boer, Which of our modeling predictions are robust?, PLoS Comput. Biol., 8 (2012), e1002593. |
[10] | C. Jiang, W. Wang, Complete classification of global dynamics of a virus model with immune responses, Discrete Contin. Dyn. Syst. Ser. B, 19 (2014), 1087-1103. |
[11] |
A. V. M. Herz, S. Bonhoeffer, R. M. Anderson, R. M. May, M. A. Nowak, Viral dynamics in vivo: Limitations on estimates of intracellular delay and virus decay, Proc. Natl. Acad. Sci. USA, 93 (1996), 7247-7251. doi: 10.1073/pnas.93.14.7247
![]() |
[12] |
A. M. Elaiw, I. A. Hassanien, S. A. Azoz, Global stability of HIV infection models with intracellular delays, J. Korean Math. Soc., 49 (2012), 779-794. doi: 10.4134/JKMS.2012.49.4.779
![]() |
[13] |
B. Reddy, J. Yin, Quantitative intracellular kinetics of HIV type 1, AIDS Res. Hum. Retrovir., 15 (1999), 273-283. doi: 10.1089/088922299311457
![]() |
[14] | A. S. Perelson, A. U. Neumann, M. Markowitz, J. M. Leonard, D. D. Ho, HIV-1 dynamics in vivo: Virion clearance rate, infected cell life-span, and viral generation time, Science, 271 (1996), 1582-1586. |
[15] |
J. E. Mittler, B. Sulzer, A. U. Neumann, A. S. Perelson, Influence of delayed viral production on viral dynamics in HIV-1 infected patients, Math. Biosci., 152 (1998), 143-163. doi: 10.1016/S0025-5564(98)10027-5
![]() |
[16] |
D. Ebert, C. D. Zschokke-Rohringer, H. J. Carius, Dose effects and density-dependent regulation of two microparasites of Daphnia magna, Oecologia, 122 (2000), 200-209. doi: 10.1007/PL00008847
![]() |
[17] |
R. R. Regoes, D. Ebert, S. Bonhoeffer, Dose-dependent infection rates of parasites produce the Allee effect in epidemiology, Proc. R. Soc. Lond. Ser. B, 269 (2002), 271-279. doi: 10.1098/rspb.2001.1816
![]() |
[18] |
X. Song, A. U. Neumann, Global stability and periodic solution of the viral dynamics, J. Math. Anal. Appl., 329 (2007), 281-297. doi: 10.1016/j.jmaa.2006.06.064
![]() |
[19] | J. K. Hale, S. Verduyn Lunel, Introduction to Functional Differential Equations, Springer, New York, 1993. |
[20] |
P. van den Driessche, J. Watmough, Reproduction numbers and sub-threshold endemic equilibria for compartmental models of disease transmission, Math. Biosci., 180 (2002), 29-48. doi: 10.1016/S0025-5564(02)00108-6
![]() |
[21] |
M. Stafford, L. Corey, Y. Cao, E. Daar, D. Ho, A. Perelson, Modeling plasma virus concentration during primary HIV infection, J. Theor. Biol., 203 (2000), 285-301. doi: 10.1006/jtbi.2000.1076
![]() |
[22] |
K. Pawelek, S. Liu, F. Pahlevani, L. Rong, A model of HIV-1 infection with two time delays: Mathematical analysis and comparison with patient data, Math. Biosci., 235 (2012), 98-109. doi: 10.1016/j.mbs.2011.11.002
![]() |
[23] | J. Wang, M. Guo, X. Liu, Z. Zhao, Threshold dynamics of HIV-1 virus model with cell-to-cell transmission, cell-mediated immune responses and distributed delay, Appl. Math. Comput., 291 (2016), 149-161. |
[24] |
S. Marino, I. B. Hogue, C. J. Ray, A methodology for performing global uncertainty and sensitivity analysis in systems biology, J. Theor. Biol., 254 (2008), 178-196. doi: 10.1016/j.jtbi.2008.04.011
![]() |
1. | Yan Yang, Rui Xu, Mathematical analysis of a delayed HIV infection model with saturated CTL immune response and immune impairment, 2022, 68, 1598-5865, 2365, 10.1007/s12190-021-01621-x | |
2. | Jiawei Deng, Ping Jiang, Hongying Shu, Viral infection dynamics with mitosis, intracellular delays and immune response, 2022, 20, 1551-0018, 2937, 10.3934/mbe.2023139 | |
3. | Noura H. AlShamrani, Reham H. Halawani, Wafa Shammakh, Ahmed M. Elaiw, Global Properties of HIV-1 Dynamics Models with CTL Immune Impairment and Latent Cell-to-Cell Spread, 2023, 11, 2227-7390, 3743, 10.3390/math11173743 | |
4. | Elsayed Dahy, Ahmed M. Elaiw, Aeshah A. Raezah, Hamdy Z. Zidan, Abd Elsattar A. Abdellatif, Global Properties of Cytokine-Enhanced HIV-1 Dynamics Model with Adaptive Immunity and Distributed Delays, 2023, 11, 2079-3197, 217, 10.3390/computation11110217 | |
5. | Zihao Hu, Junxian Yang, Qiang Li, Song Liang, Dongmei Fan, Mathematical analysis of stability and Hopf bifurcation in a delayed HIV infection model with saturated immune response, 2024, 47, 0170-4214, 9834, 10.1002/mma.10097 | |
6. | Zhiqi Zhang, Yuming Chen, Xia Wang, Libin Rong, Dynamic analysis of a latent HIV infection model with CTL immune and antibody responses, 2024, 17, 1793-5245, 10.1142/S1793524523500791 | |
7. | Noura H. AlShamrani, Reham H. Halawani, Ahmed M. Elaiw, Effect of Impaired B-Cell and CTL Functions on HIV-1 Dynamics, 2023, 11, 2227-7390, 4385, 10.3390/math11204385 | |
8. | Lili Lv, Junxian Yang, Zihao Hu, Dongmei Fan, Dynamics Analysis of a Delayed HIV Model With Latent Reservoir and Both Viral and Cellular Infections, 2024, 0170-4214, 10.1002/mma.10655 | |
9. | Surya Prakash, Anuj Kumar Umrao, Prashant K. Srivastava, Bifurcation and stability analysis of within host HIV dynamics with multiple infections and intracellular delay, 2025, 35, 1054-1500, 10.1063/5.0232978 |