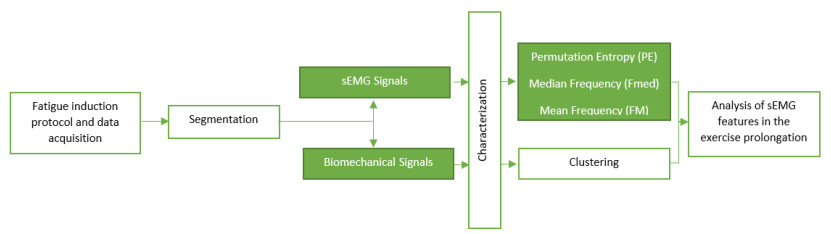
Citation: J. Murillo-Escobar, Y. E. Jaramillo-Munera, D. A. Orrego-Metaute, E. Delgado-Trejos, D. Cuesta-Frau. Muscle fatigue analysis during dynamic contractions based on biomechanical features and Permutation Entropy[J]. Mathematical Biosciences and Engineering, 2020, 17(3): 2592-2615. doi: 10.3934/mbe.2020142
[1] | Baohua Hu, Yong Wang, Jingsong Mu . A new fractional fuzzy dispersion entropy and its application in muscle fatigue detection. Mathematical Biosciences and Engineering, 2024, 21(1): 144-169. doi: 10.3934/mbe.2024007 |
[2] | Shaojun Zhu, Jinhui Zhao, Yating Wu, Qingshan She . Intermuscular coupling network analysis of upper limbs based on R-vine copula transfer entropy. Mathematical Biosciences and Engineering, 2022, 19(9): 9437-9456. doi: 10.3934/mbe.2022439 |
[3] | Ge Zhu, Xu Zhang, Xiao Tang, Xiang Chen, Xiaoping Gao . Examining and monitoring paretic muscle changes during stroke rehabilitation using surface electromyography: A pilot study. Mathematical Biosciences and Engineering, 2020, 17(1): 216-234. doi: 10.3934/mbe.2020012 |
[4] | Konki Sravan Kumar, Daehyun Lee, Ankhzaya Jamsrandoj, Necla Nisa Soylu, Dawoon Jung, Jinwook Kim, Kyung Ryoul Mun . sEMG-based Sarcopenia risk classification using empirical mode decomposition and machine learning algorithms. Mathematical Biosciences and Engineering, 2024, 21(2): 2901-2921. doi: 10.3934/mbe.2024129 |
[5] | Bei Liu, Wenbin Tan, Xian Zhang, Ziqi Peng, Jing Cao . Recognition study of denatured biological tissues based on multi-scale rescaled range permutation entropy. Mathematical Biosciences and Engineering, 2022, 19(1): 102-114. doi: 10.3934/mbe.2022005 |
[6] | Chengliang Wu, Weiya Hao, Wei He, Xiaofei Xiao, Xuhong Li, Wei Sun . Biomechanical and neuromuscular strategies on backward somersault landing in artistic gymnastics: A case study. Mathematical Biosciences and Engineering, 2019, 16(5): 5862-5876. doi: 10.3934/mbe.2019293 |
[7] | Xiebing Chen, Yuliang Ma, Xiaoyun Liu, Wanzeng Kong, Xugang Xi . Analysis of corticomuscular connectivity during walking using vine copula. Mathematical Biosciences and Engineering, 2021, 18(4): 4341-4357. doi: 10.3934/mbe.2021218 |
[8] | Feng You, Yunbo Gong, Xiaolong Li, Haiwei Wang . R2DS: A novel hierarchical framework for driver fatigue detection in mountain freeway. Mathematical Biosciences and Engineering, 2020, 17(4): 3356-3381. doi: 10.3934/mbe.2020190 |
[9] | Xiaobo Zhang, Donghai Zhai, Yan Yang, Yiling Zhang, Chunlin Wang . A novel semi-supervised multi-view clustering framework for screening Parkinson's disease. Mathematical Biosciences and Engineering, 2020, 17(4): 3395-3411. doi: 10.3934/mbe.2020192 |
[10] | Fengjie Liu, Monan Wang, Yuzheng Ma . Multiscale modeling of skeletal muscle to explore its passive mechanical properties and experiments verification. Mathematical Biosciences and Engineering, 2022, 19(2): 1251-1279. doi: 10.3934/mbe.2022058 |
Muscle fatigue is a reduction in the maximum voluntary force induced by exercise [1]. This topic has been addressed by different exercise physiology researchers, as it has a great impact on athlete performance, and is an important risk factor for the occurrence of overload injuries that, in some cases, can be irreversible [2,3]. It has been shown that adequate physical conditioning can help avoid fatigue [4]. Such conditioning involves modifying or avoiding exercises or tasks that cause fatigue, in turn preventing muscle injuries. Muscle fatigue causes numerous injuries in workers who perform mechanical or strenuous tasks, generally associated with overload efforts. For example, in Colombia, 38.04% of the population declares to be working in conditions of fatigue most of the time [5], and according to occupational disease data, between 2009 and 2012, an average 88.25% of the reported accidents were associated with musculoskeletal injuries. Surface electromyography (sEMG) has been used for muscle fatigue analysis and many researchers have studied the changes during prolonged exercise. In this regard, there are some important topics to address, such as contraction type, fatigue states and sEMG features.
The study of muscle fatigue has been addressed mainly for isometric contractions [6,7,8,9], where the length of the muscle does not change during exercise. However, dynamic contractions (where the length of the muscle changes during exercise), represent a more realistic view of many daily activities as well as sports (i.e. walk, carry objects, heavy lifting, run, cycle, among others), but these contractions have not been explored in depth. Dynamic contractions are more challenging; first, the muscle movement adds low frequency noise and artifacts to the sEMG, as well as hindering its acquisition and processing. Second, the activation of sEMG is not continuous, which is why it is necessary in many cases to segment the signal. Finally, the performance of sEMG features decrease in this signal.
Most of the work in this field approaches muscle fatigue as a two state process: Fatigue and Non-Fatigue [10,11]. However, some authors propose to address this problem in three states (Non-Fatigue, Transition-to-Fatigue, and Fatigue), because to introduce the transient-to-fatigue state before the onset of fatigue allows the development of different prediction and/or detection algorithms of muscle fatigue. In [8] they used this approach to detect the Transition-to-Fatigue state, and based on it made predictions regarding how long it takes the bicep muscles to reach the Fatigue state during isometric contractions. Other studies have used sEMG and deep learning to detect muscle fatigue states [12]. Comparisons between classifiers has also been conducted, showing that Auto-Regressive Models has the best performance for detecting the three states of muscle fatigue, while Support Vector Machines, are more useful for Fatigue detection [13].
Many studies have reported on the performance of different indexes in time domain, spectral domain or time-frequency domain [9]. The spectral domain indexes have been specially used in studies working with dynamic contractions [14,15,16]; however, of late, time-frequency indexes have become a trend which seems to be more effective [17,18]. Generally many studies relate several indexes like Mean Frequency (FM), Median Frequency (Fmed), Dimitrov's spectral index (Flnsmk), the Energy of the Detail Coefficients (EDCk) of Wavelet transform, and even some time domain indexes like Zero Crossing (ZC), Root Mean Square (RMS), Waveform Lenght (WL), and so forth [2,13,14,19,20,21]. Non-linear parameters, which are not as commonly used, can also be implemented in the analysis of muscle fatigue [9]. The use of Non-linear paremeters was proposed by Nieminen and Takala (1996) [9] who suggested that sEMG signals could be better modeled as outputs of a nonlinear dynamic system, which opens up more possibilities in the assessment of muscle fatigue and new techniques like entropy analysis, fractal analysis and recurrence quantification analysis. Permutation Entropy (PE) is a recently proposed non-linear parameter that has been proved in many biomedical applications [11,22]; however, there are limited studies assessing its performance in sEMG signals during conditions of muscle fatigue. PE is interesting to consider in this application, because unlike other non-linear features, PE has low computational complexity and is almost parameter free.
The aim of this paper is to asses the performance of PE in distinguishing between three muscle states (Non-Fatigue, Transition-to-Fatigue and Fatigue) during dynamic contractions using sEMG and biomechanical signals.
This study was divided into 5 stages, described in Figure 1. First, a muscle fatigue induction protocol was established in order to acquire the data. Second, the signals were segmented to obtain each muscle contraction. Third, the signals were characterized, including time and frequency features and PE. Then, clustering based on biomechanical features was used to label the signals of each contraction into three classes: Non-Fatigue, Transition-to-Fatigue, and Fatigue. Finally, we assessed the feature performance in distinguishing among the three muscle states.
Nine healthy young adults (5 women and 4 men) aged 21 +/- 3, who do not frequently perform physical activity participated in this study. All subjects with injuries in upper limbs and cardiovascular or neuromuscular pathologies were excluded. This study was approved by the Ethics Committee for Scientific Research of the Instituto Tecnologico Metropolitano of Medellin. All the participants signed an informed consent which explained the study and the risks of their participation.
This study focuses on muscle fatigue induced in the deltoid muscle, as the shoulder is considered a critical joint in musculoskeletal injuries, therefore the study of the deltoid muscle group has been useful in implementing algorithms for muscle fatigue detection during dynamic contractions [14,23]. For that reason, we propose a lateral dumbbell lifting exercise that produces a right or obtuse angle between the arm and the trunk. During this exercise, the back is held straight without any lateral movement of the trunk. In this protocol, the participants were seated during the exercise and posture was visually controlled by an instructor.
The load during the exercise, was determined by the Maximum Repetition Value (MRV) of each participant and was computed by the Brzycki expression [24] as:
MRV=w1.0278−0.278Cn, | (2.1) |
where w is the weight of the dumbbell and Cn is the number of contractions. Each individual warmed-up and was given an adjustable dumbbell (21.15 +/- 2.25 lb for men, and 10.2 +/- 1.2 lb for women). At that point, they were asked to perform as many lateral dumbbell lifts as they could with each arm (Figure 2)
Once the MRV was determined, the participants performed a first round of the exercise, at 60% of the MRV with each arm as long as they could or until they started to express fatigue. After a 30-minute break, they repeated the routine with 50% of the MRV. A total of 4 tests per participant were conducted. We used a 25-beats-per-minute metronome to control the cadence of the contractions, and participants were asked to perform as many contractions as possible with the beat of the metronome.
PowerLab 16/35 (AD Instruments Inc.) equipment with a sampling frequency of 2KHz and a Notch filter for deleting the line interference was used for acquiring sEMG signals. Subsequently, a butterworth pass band filter of second order between 30-300 Hz was applied, based on the methodology proposed in [25] in order to improve burst detection. PowerLab was connected to the participant with Ag/AgCl surface electrodes of a 20 mm diameter disc, placed on the half zone of the lateral deltoid with a 20 mm inter-electrode distance and with a reference electrode in the back at the height of the C7. The study area was previously cleaned with alcohol and was not shaved.
Each test was recorded with Basler high-speed cameras to capture the biomechanical signals. Reflective markers were placed on the ribs, forearm, and Acromion of the participants (Figure 2). Kinovea software was used to track the markers and obtain their position signals.
sEMG segmentation is an important task in the analysis of dynamic contractions as the muscle activation is not continuous, unlike isometric contractions where a simple moving window is enough to analyze fatigue progression. However, in dynamic contractions it is necessary to obtain the segments of muscle activation to avoid rest segments, where muscles fibers are not activated (sEMG does not carry any information), enabling us to analyze the fatigue progression based only on the activation segments. Therefore, it was necessary to implement a preprocessing stage to segment the sEMG and biomechanical signals (angle, angular speed, and angular acceleration).
The threshold-based method proposed in [25] was used for contraction (burst) segmentation. According to their method, initially, a passband filter between 30 and 300 Hz was applied. Subsequently, we used the Teager-Kaiser (TKEO) energy coefficient (ψ) defined as 2.2:
ψ[xn]=x2n−xn+1xn−1 | (2.2) |
where X is a sEMG signal of length N, defined as X={x1,x2,⋯,xN}. Afterwards, the signals were rectified and filtered with a 50-Hz low-pass filter. Finally, burst detection was carried out based on the Approximate Generalized Likelihood Ratio (AGLR) method [26,27,28]. AGLR is based on statistical testing of the null hypotheses H0 (sEMG signal is not a burst) and the alternate hypothesis H1 (sEMG signal is a burst), describing the statistical properties of a series of sEMG samples and uses a thresholding approach defined as [25]:
AGLRn=ln(k∏n=1p1(xn)|H1p0(xn)|H0)<>h | (2.3) |
where p1 and p0, represent the probability that sEMG segments correspond to a contraction (burst) or not, respectively.
Algorithm 1, describes the pseudocode for the segmentation of a sEMG signal. Parameters μ0, σ0, μ1 and σ1, refer to the mean and deviation of univariate Gaussian Probability Density Function, which were obtained after manually segmenting 6 bursts and 6 baseline signals of each subject. Parameters k (window length) and Th (Threshold) of the AGLR method where set to 300 and 15 respectively after a heuristic search (results of this task are not shown in this publication, as it is not relevant to the main objective).
![]() |
After segmentation, we removed the border of the sEMG segments in order to ensure that all segments had the same length (1000 points, equivalent to 0.5 seconds of signal) and to avoid border effects related to the beginning and the end of the contraction.
The reflective markers were placed on each participant forming a triangle between marker 1 on the forearm (M1), marker 2 on the acromion (M2) and marker 3 on the ribs (M3), (Figure 3), in order to obtain the angle between the arm and the trunk during exercise.
Kinovea software was used to track the positions of the three markers. Kinovea returns the position of the marker as Cartesian coordinates (based on pixels) in every frame of the video (500 frames per second). Then, we computed the distance d1 (distance between forearm and ribs), d2 (distance between forearm and acromion) and d3 (distance between acromion and ribs) in pixels using the Euclidean norm. Distances d2 and d3 are constant, representing the anthropometric measurements of any subject. However, these distances presented small changes between frames, due to tracking errors and involuntary subject movement, for this reason, we set d2 and d3 as the average distance during all frames.
Being θn the angle signal formed between the trunk and the forearm, it was computed using law of cosines as:
θn=cos−1(d22+d32−d12n2d2d3) | (2.4) |
where d1n is the distance between forearm and ribs, in the moment n.
Biomechanical signals angular velocity (ωn) and angular acceleration (αn) were computed as the first and second derivative of θn. Then, the signals were filtered using third order Butterworth low-pass filters at cut-off frequencies of 100 Hz (for θn and ωn) and 30 Hz (for αn).
Algorithm 2 shows the process for computing biomechanical signals θn, ωn and αn. Inputs M1, M2 and M3 are the matrix of dimensions N×2, that represent the Cartesian position at N time of the markers according to the figure 3.
Finally, every contraction in the signals (θ, ω and α) was segmented. For this purpose, we employed a segmentation approach based on a threshold for the θ signal, and used the same times to construct a remaining signal, as follows:
Maskn={θn>Th,1θn<Th,0 | (2.5) |
when Maskn=1 refers to a contraction, in this work we used the 25thpercentile of θ as the threshold (Th) (Figure 4).
Assuming that biomechanical changes are due to muscle fatigue progression, we propose to obtain features from θ, ω and α as described in Table 1.
Feature | Abbreviation | Formula |
Maximum angle | Maxθ | maxθn |
Mean angle | Meanθ | 1N∑Nn=1θn |
Integral of angle | Intθ | ∑Nn=1θn |
Maximum velocity | Maxω | maxωn |
Minimum velocity | Minω | minωn |
Mean velocity | Meanω | 1N∑Nn=1ωn |
Integral of velocity | Intω | ∑Nn=1ωn |
Mean speed | Mean|ω| | 1N∑Nn=1|ω| |
Maximum acceleration | Maxα | maxαn |
Minimum acceleration | Minα | minαn |
Mean acceleration | Meanα | 1N∑Nn=1αn |
Integral of acceleration | Intα | ∑Nn=1αn |
Each of the biomechanical features obtained was normalized intra-subject, as some biomechanical variables such as Maxθ and Maxω present great variability between subjects. Next, the features were correlated with the contraction number in order to identify biomechanical features related to muscle fatigue progression. In this study, values with an absolute correlation greater or equal to 70% were chosen as relevant biomechanical features and were used for clustering.
![]() |
We employed an unsupervised learning algorithm in order to label the contraction into three groups, which has a direct relation with changes in the biomechanical variables of the subject, for practical effects we refer to these different stages as: Non-Fatigue, Transition-to-Fatigue, and Fatigue, although this does not involve a clinical diagnosis of muscle fatigue. Most of the literature in this topic suggests that muscle fatigue has two stages (Non-Fatigue and Fatigue) [11,29]; however, other works show that using a three stage approach allows for the development of a prediction system able to detect fatigue before its clinical appearance [30]. Therefore, we implemented a type of agglomerative hierarchical clustering based on Ward's method. The clustering procedure was conducted using the contractions obtained from all the tests. In agglomerative clustering, initially every point (in this application, a point is a contraction) in the data set is an individual cluster and clusters are progressively merged according to a similarity criterion. Ward's method states that a cluster should be fused with the cluster that causes the least increase in within-cluster sum of squares [31]. Formally, the within-cluster sum of squares is defined as the sum of the squares distance between points in the cluster and the centroid of the cluster. The resulting sum of squares that joins clusters a and b can be computed as [32]:
√2nanb(na+nb)||¯xa−¯xb|| | (2.6) |
where na and nb are the number of elements in the clusters, ¯xa, and ¯xb are the cluster centroids.
Once the fragments of the sEMG signal were obtained and labeled as described in Section 2.3, we computed different sEMG features PE, RMS, ZC, FM and Fmed to confirm that the changes in biomechanical variables were due to muscle fatigue progression. Then, we evaluated if there was a statistically-significant difference between the sEMG features of the three groups. For this purpose, we used an Analysis of Variance (ANOVA) to establish the existence of a statistical difference between the 3 groups (Non-Fatigue, Transition-to-Fatigue, and Fatigue). Subsequently, we performed a post-hoc analysis using the Less Significant Difference Test (LSD) to directly compare pairs of groups, i.e. Non-Fatigue vs Transition-to-Fatigue, Transition-to-Fatigue vs. Fatigue, and Non-Fatigue vs. Fatigue.
Finally, we carried out a Receive Operator Curve (ROC) analysis, which uses logistic regression as classifier, in order to assess the discriminant capacity of the more promising sEMG features to distinguish between Non-fatigue, Transition-to-Fatigue and Fatigue.
Entropy measures are very common for quantifying the complexity of a time series; however, common approaches, such as Shannon entropy, neglect to take into account effects from the temporal order of the values in the time series. Bandt and Pompe proposed in [33], a natural encoding which reflects the rank order of successive (xn) in sequences of length (N). In this regard, given an sEMG signal X={x1,x2,⋯xN}, if we take signal segments of m points (Embedded dimension) with a time delay of τ as Si={Xi,Xi+τ,Xi+2τ,⋯Xi+τ(m−1)}. Sorting Si in ascending order as Xi+r0<Xi+r1<⋯<Xi+r(m−1), we can obtain its ordinal pattern as πj={r0,r1,⋯r(m−1)} [34]. Then, the set of ordinal patterns is Π={π1,π2,⋯πm!}. The permutation entropy (PE) is computed using the Shannon form, but taking the relative frequency of the ordinal patterns as:
PE=m!∑j=1−p′(πj)log2p′(πj) | (2.7) |
where (p′(πj)) represents the relative frequencies of the ordinal pattern πj. PE essentially measures information based on the occurrence or absence of certain permutation patterns of length m, in the ranks of values of a time series [35]. A detailed explanation of PE calculation can be found in [35].
In this study, after computation, PE values were normalized intra-subject with the aim of reducing the impact of between subject differences, and we conducted an exhaustive search of the embedded dimension m (between 2 and 10) and the time delay τ (between 1 and 10).
We analyzed features in the time domain, such as the Root Mean Square (RMS) which is commonly used in studies on muscle fatigue [9,14,21]. We applied fast Fourier transform to sEMG signals and computed features such as FM and Fmed which are considered traditional indicators of muscle fatigue [9,16,36]) in each of the Burst obtained. These features are very common in muscle fatigue analysis, and some studies have shown that during static contractions the FM usually decreases [9,15], and Fmed has been shown to have a similar behavior [14,29]. All the features described were computed according to table 2 and were normalized intra-subject to reduce the impact of between subject difference.
Feature | Equation |
Permutation Entropy (PE) | ∑m!j=1−p′(πj)log2p′(πj) |
Root Mean Square (RMS) | √1N∑Nn=1|x2n| |
Mean Frecuency (MF) | ∑Fmaxf=FminfS[f]∑Fmaxf=FminS[f] |
Median Frecuency (Fmed) | ∑Fmedf=FminS[f]=∑Fmaxf=FmedS[f] |
p′(πj) is the relative frequency of the ordinal pattern πj X is a sEMG signal as X={x1,x2,⋯xN} S[f] is the power spectrum density of a signal at frequency f |
The data for this study was obtained from only nine subjects; however, this does not seem to be a disadvantage, as several studies have used a similar number of subjects [37,38,39] or less [12,21,40,41,42]. This number of subjects is feasible as several samples are recorded per subject; for example, in isometric exercises long signals can be recorded [42] and it is possible to apply windows of time in order to increase the number of samples per subject [12]. On the other hand, muscle activity during dynamic contractions can be analyzed by means of a contraction set, as these signals change greatly over time, like in [14], where the authors did an analysis of different parameters based on their behaviour in each contraction of a given exercise.
In this study, the nine subjects performed 2 tests, first, with 60 % of the MRV and then with 50 %. This process was repeated for each arm, obtaining 4 signals per subject, for a total of 36 signals. Additionally, signals were treated as a set of contractions, thus obtaining a collection of 950 samples that include sEMG and position signals.
The segmentation process is described step by step in Figure 5 and Algorithm 1. (a) presents the original signal obtained in one of the tests. (b) shows the signal after applying the 30–300Hz bandpass filter. (c) is the signal after applying the TKEO. (d) plots the signal of TKEO after passing through a 50-Hz low pass filter and rectification. Finally, (e) describes the way the signal is segmented using the approximate generalized likelihood ratio (AGLR) method.
The AGLR method using the TKEO seems to be an adequate method for sEMG signal segmentation. In another study [25], the inclusion of the TKEO in signal conditioning significantly reduced the detection error of muscle activation, which is reflected in the precision of fragmentation of the sEMG burst. However, in this study, intra-subject preprocessing was necessary since a general model presented significant errors in burst detection. By adopting this method, we were able to segment all the contractions in sEMG signals.
Figure 6 shows the filtering process of biomechanical signals. Noise can be observed due to the marker tracking process in Kinovea and the involuntary movements of the subject. The applied filters present a suitable correction of the signal under study.
The proposed segmentation method is based on thresholding using the 25th percentile of θ signal, which has shown to be effective because only one of the 36 tests had to be manually segmented. Since the signals are equal in time, the same mask was applied to the three of them. In Figure 6, we can observe the preprocessing stage applied to the biomechanical signals. (a), (b), and (c) show the signals obtained from the motion analysis system using the methods described in Section 2.3.2. In turn, (d), (e), and (f) are filtered biomechanical signals with low pass filters. Finally, (g), (h), and (i) present the resulting segmentation applied to biomechanical signals.
As a result of the segmentation process, we obtained 944 contractions associated with sEMG bursts with an average duration of 1.34 s (2683 points approximately); however, they were trimmed to a duration of 0.5 seconds, to avoid border effects. Finally, the same number of biomechanical signals (θ, ω and α) were obtained from 36 trials.
Several biomechanical features were calculated in this study, in order to identify which of them are related to fatigue progression. We identified features with a Pearson's correlation greater than or equal to 70%. This correlation analysis is summarized in Table 3. The results show that all features obtained from θ are related to fatigue progression, while Maxω is the only feature related to angular velocity. On the other hand, angular acceleration features were not related to fatigue progression.
Biomechanical features | Correlation |
Max |
0.82 |
Int |
0.81 |
Mean |
0.81 |
Max |
0.7 |
Mean |
0.68 |
Min |
0.55 |
Int |
0.46 |
Max |
0.31 |
Mean |
0.3 |
Min |
0.23 |
Int |
0.22 |
Mean |
0.15 |
Figure 7 shows the results of the clustering process and the evolution of states within the exercise progression. Furthermore, the clustering process identified the 3 following groups:
Group 1 (Non-Fatigue): these are the contractions at the beginning of the test, indicating that muscles are in an optimal state during the contractions and the biomechanical conditions have not been compromised (Non-Fatigue).
Group 2 (Transition-to-Fatigue): these are the contraction in the middle of Group 1 and Group 3, we assume this group of contractions corresponds to a slight change in the biomechanical features of the subject. This stage was called Transition-to-Fatigue.
Group 3 (Fatigue): these are the contractions at the end of the exercise. During these contractions the subject can not continue the exercise and there is a big change in the biomechanical features, indicating the possible presence of muscle fatigue.
In other hand, Figure 7 (b) shows the results of the correct labeling of the signals, while Figure 7 (c) highlights some clustering errors. We identified that 72.3 % of the signals have some mislabeled contractions; however, this represents just 8.06 % of the contractions.
It is important to state that this labeling is not proof of clinical fatigue, but shows a significant variation in the subject's biomechanical variables, which can lead to possible musculoskeletal injuries during sport or work-related movements. Although this labeling process can not confirm the presence of clinical fatigue in the muscle, it is still relevant as it can identify changes in the biomechanical variables, possibly preventing injuries.
Such variability in biomechanical features is difficult to quantify between subjects [43], which means that every subject has their own range of motion according to their age and physical condition, among other factors. For this reason, we evaluated the changes in biomechanical features after intra-subject normalization. Figure 8 shows the distribution of 2 biomechanical features Maxθ & Maxω) after intra-subject normalization. From both box-plots it is clear that biomechanical features decrease with fatigue progression, however Maxω shows a larger variability than Maxθ.
Several researches have used strategies for labeling muscle fatigue stages, based on biomechanical or kinematic features, but the majority have not demonstrated it clinically, as it is an invasive and expensive procedure. For instance, some authors have assumed a fatigue stage after a set of intensive exercises [44,45] or have carried out the same activity until the participants were exhausted [46,47,48]. Others have implemented a threshold when a biomechanical variable such as force, velocity, or distance has decreased enough to assume muscle fatigue [6,49]. Our labeling method is more reliable than establishing a threshold for a biomechanical variable or than assuming muscle fatigue after an exercise set, as it not make any assumptions but rather groups the contractions according to their biomechanical similarities.
In order to confirm that the biomechanical changes used to labeled the contractions were due to muscle fatigue progression, we computed sEMG features and compared them between the three muscle fatigue states. Figure 9 shows a Heat-map of the ANOVA test p-values between Non-Fatigue, Transition-to-Fatigue and Fatigue for PE using different values of m and τ (Embedded dimension and time delay). The greatest statistical difference was obtained with m=7 and τ=6. Taking into account that the sEMG length was of 1.34 s (i.e. N=2683) and the recommendation N>>m! stated in [33], we found that with m=7 (m!=5040) the number of possible ordinal patterns is larger than the number of signal points, indicating that the recommendation of N>>m! is not fulfilled and therefore, the obtained PE values are not a good approximation of sEMG complexity. However, these results confirm that for classification purposes, it is not necessary for this rule to be satisfied as was demonstrated in [34].
On the other hand, Figure 10 shows the behaviour of the PE in different subjects. In this figure the mean value of PE from all the tests applied to each subject was extracted in each of the assessed states (Non Fatigue, Transition-to-Fatigue and Fatigue), and they were superimposed in order to evidence inter-subject trends. It can be observed thaty in all the subjects the PE value decreases progressively, in some subjects with a higher slope than others. The mean PE value (intra-subject normalized) for all subjects was 0.75 in Non-Fatigue state, 0.54 for Transition-to-Fatigue and 0.37 for Fatigue. The PE value decreased around 50.6% from Non-fatigue to Fatigue contractions, indicating a clear reduction in sEMG complexity in the presence of fatigue.
Table 4 shows a comparison of the ANOVA test results of each sEMG feature and LSD between the groups defined in Section 3.2, considering p << 0.01 as statically significant difference. Results show that biomechanical changes are due to the progression in muscle fatigue and the retrieved groups from the clustering correspond to different states of the muscle during exercise, as the statistical test revealed that all the sEMG features exhibited changes in at least 2 of the 3 comparisons. Additionally, these findings are comparable to other muscle fatigue studies during dynamic contractions [9,14] where the Fmed and ZC shows statistically significant differences between stages of rest and after exercises, with decreasing behavior. This result is contrary to [50] who reported a increment of Fmed during the fatiguing exercise.
Feauture | ANOVA test | LSD | ||
G1 vs G2 | G1 vs G3 | G2 vs G3 | ||
PE | p<<0.01 | p<<0.01 | p<<0.01 | p<<0.01 |
Fmed | p<<0.01 | p<<0.01 | p<<0.01 | p<<0.01 |
FM | p<<0.01 | 0.0074 | p<<0.01 | 0.25 |
RMS | p<<0.01 | 0.4856 | 0.0073 | p<<0.01 |
A ROC analysis was applied to the more promising features (PE and Fmed) in order to observe how they distinguish between the different classes, the Area Under Receive Operator Curve (AUROC) help us to analyze the performance of our algorithm in the classification task. As shown in Figure 11, the performance of PE is slightly higher than Fmed, although both features have a high performance for distinguish between Non Fatigue and Fatigue states. This result was expected as several studies have previously found significant changes between these stages [9,51,52].The worst performance was obtained when the algorithm distinguished between Transition-to-Fatigue and Fatigue, possibly due to the fact that the biomechanical changes were not so important in this case; however, we observed that PE performs better than Fmed in these two stages and both are greater than FM (AUROC = 0, 54). The use of both features (PE and Fmed) as inputs does not improve the classification performance between the groups. 11 sEMG muscle fatigue features and 6 mathematical operators
Authors like [14,29] describe the behavior of some frequency and time domain features. We found correspondence in the Fmed feature because (as can be observed from Table 4) it is highly variable with respect to the three groups of muscle fatigue. However, FM and RMS fail to identify the 3 states of muscular fatigue and only differentiate between Non-Fatigue and Fatigue. Another study presents the behavior of PE in the evaluation of muscle fatigue, finding that the PE value decreases with exercise prolongation [11]. We obtained similar results; however, we demonstrated a constant change of PE during exercise, while [11] only considered the first and last of the six equal segments using time scales. Therefore, this could indicate that PE is the most adequate feature for assessing muscle fatigue in the three states.
Other authors have analyzed the behavior of several biomechanical variables in terms of the evolution of a repetitive task, finding that biomechanical variables can adapt with muscular fatigue progression [44,53]. In addition, another works have demonstrated how the muscle fatigue influences directly the biomechanical and kinematic behavior of some joints [49,54]. Similar results have been found in this work because changes in variables like angle and velocity enabled to identify the three states of muscle fatigue progression. Thus, these results suggest a relation between the subject's biomechanical variables and muscle fatigue progression.
The classification accuracy of PE between transitions between states can be seen in Table 5, as well as in other reports in the literature. Initially, our method did not perform as well as other approaches, however, there are several reason behind this. Firstly, the highest performances are obtained for isometric contractions, while our method works in dynamics contractions, the latter which are more challenging to analyze due to non-stationary signal and the movement artifacts [9]. Secondly, most studies only distinguish between Non-Fatigue and Fatigue, while our study aims to classify between the three states. Thirdly, most muscle fatigue analyses has been conducted in biceps muscle, while our approach is with the deltoid muscle. Finally, it is important to clarify that our method uses a single feature to classify the signals, this means that logistic regression classifier is acting as a simple thresholding approach. In this regard, other methods such SVM or k-NN represent a more robust framework for classifying the signals, but their use is justified when one is using multiple features.
States | Reference | Contraction Type | Muscle | Classification method | Features | Accuracy |
Non-Fatigue vs Transition-to-Fatigue | [30] | Isometric | Biceps | LDA | 1D spectro-composite feature | 90.37% |
[12] | Isometric | Biceps | deep belief networks | Raw Signal | 66.32% | |
In this Work | Dynamic | Deltoid | Logistic Regression | PE | 71.0% | |
Transition-to-Fatigue vs Fatigue | In this Work | Dynamic | Deltoid | Logistic Regression | PE | 68.0% |
Non-Fatigue vs Fatigue | [12] | Isometric | Biceps | DBNs | Raw Signal | 97.60% |
[30] | Isometric | Biceps | Logistic Regression | 1D spectro-composite feature | 84.8% | |
[55] | Dynamic | Biceps | k-NN | Multifractal features | 88% | |
[56] | Dynamic | Biceps | SVM | Renyi entropy | 86.7% | |
[57] | Dynamic | Biceps | Logistic Regression | Multifractal features-Infomation Gain | 84% | |
[57] | Dynamic | Biceps | k-NN | Multifractal features-Infomation Gain | 82% | |
[55] | Dynamic | Biceps | Logistic Regression | Multifractal features | 82% | |
[58] | Dynamic | Biceps | LDA | Pseudo-wavelet | 78.45% | |
In this Work | Dynamic | Deltoid | Logistic Regression | PE | 80.0% |
PE offers a low computational complexity cost compared to other non-linear descriptors like Lyapunov exponents or Correlation dimension [33]. However, when the embedded dimension is large (usually above m>12), the number of possible ordinal patterns (Permutation) increases dramatically and the memory consumption required to maintain the counting of those pattern is insufficient in most machines. Despite the above, for this particular application we found that an embedded dimension of 7 is optimal for characterizing the EMG signal in order to detect muscle fatigue. This means that the number of possible ordinal patterns is only 5040 and the required memory to maintain the counting is around 20.1 Kilo bytes (using an integer of 32 bits for counting), such memory capacity is available in low cost devices such as an Arduino UNO (32 KB). Regarding computational complexity, in the literature there are several proposals for computing PE in a fast and robust way, such as Piek et al. that proposed a PE algorithm based on a binary vector representation of ordinal patterns and achieved a computational complexity of O(m2⋅N⋅logN) [59], their implementation is based on bit shift operations which are very efficient in execution time, resulting in an attractive possibility for using PE in real time application.
This work presents the analysis of muscle fatigue progression, during dynamic contractions, based on Permutation Entropy and biomechanical features. Results reveal that biomechanical features can be used to distinguish between different stages of muscle fatigue, mainly, features derived from angle (θ) and angular velocity (ω). Additionally, Permutation Entropy (PE) of surface electromyography (sEMG) signal, had the best discriminant capacity between Muscle Fatigue, Transition-to-Fatigue and Non-fatigue, over-performing classical sEMG features as Mean and Median Frequency (Fmed and MF), and Root Mean Square (RMS).
In future work, it would be valuable to look into the use of PE for predicting muscle fatigue from sEMG signals.
The authors have no conflicts of interest to report.
[1] | S. C. Gandevia, Spinal and supraspinal factors in human muscle fatigue, Physiol. Rev., 81 (2001), 1725-1789. |
[2] | A. Phinyomark, S. Thongpanja, H. Hu, P. Phukpattaranont, C. Limsakul, The usefulness of mean and median frequencies in electromyography analysis, in Computational Intelligence in Electromyography Analysis - A Perspective on Current Applications and Future Challenges, IntechOpen Limited, London, 2012, 195-220. |
[3] | A. Ascensão, J. Magalhães, J. Oliveira, J. Duarte, J. Soares, Fisiologia da fadiga muscular. Delimitação conceptual, modelos de estudo e mecanismos de fadiga de origem central e periférica, Rev. Por. de Ciências do Desp., 3 (2003), 108-123. |
[4] | S. D. Mair, A. V. Seaber, R. R. Glisson, W. E. Garrett, The role of fatigue in susceptibility to acute muscle strain injury, Am. J. Sports Med., 24 (1996), 137-143. |
[5] | M. Corchuelo, M. Soler, L. Lozano, Informe ejecutivo de la segunda Encuesta nacional de condiciones de seguridad y salud en el trabajo en el sistema general de Riesgos Laborales de Colombia, Ministerio de Trabajo, Republica de Colombia, 2013, 1-56. |
[6] | S. P. Arjunan, D. K. Kumar, G. Naik, Computation and evaluation of features of surface electromyogram to identify the force of muscle contraction and muscle fatigue, Biomed. Res. Int., 2014 (2014), 1-6. |
[7] | C. Rocha, B. S. Geres, H. U. Kuriki, R. D. Faria, N. Filho, Análise da reprodutibilidade de parâmetros no domínio da frequência do sinal EMG utilizados na caracterização da fadiga muscular localizada Materiais e Métodos, Motriz-revista de Ed. Fís., 18 (2012), 456-464. |
[8] | F. Sepulveda, M. R. Al-mulla, M. Colley, sEMG techniques to detect and predict localised muscle fatigue, in EMG methods for evaluating muscle and nerve function, IntechOpen Limited, London, 2012, 157-186. |
[9] | M. González-Izal, A. Malanda, E. Gorostiaga, M. Izquierdo, Electromyographic models to assess muscle fatigue, J. Electromyogr. Kinesiol., 22 (2012), 501-512. |
[10] | D. R. Bueno, J. M. Lizano, L. Montano, Muscular fatigue detection using sEMG in dynamic contractions, in Annual International Conference of the IEEE Engineering in Medicine and Biology Society (EMBS 2015), 2015, 494-497. |
[11] | R. S. Navaneethakrishna, Multiscale feature based analysis of surface EMG signals under fatigue and non-fatigue conditions, in Annual International Conference of the IEEE Engineering in Medicine and Biology Society (EMBS 2014), 2014, 4627-4630. |
[12] | M. Tian, Y. Ozturk, S. Sun, Y. Su, Measurement of upper limb muscle fatigue using deep belief networks, J. Mech. Med. Biol., 16 (2016) 1-18. |
[13] | N. A. Kamaruddin, P. I. Khalid, A. Z. Shaameri, The use of surface electromyography in muscle fatigue assessments: A review, Jurnal Teknologi, 74 (2015), 1-5. |
[14] | D. R. Bueno, J. M. Lizano, L. Montano, Muscular fatigue detection using sEMG in dynamic contractions, in Annual International Conference of the IEEE Engineering in Medicine and Biology Society (EMBS 2015), 2015, 494-497. |
[15] | H. J. Hwang, W. H. Chung, J. H. Song, J. K. Lim, H. S. Kim, Prediction of biceps muscle fatigue and force using electromyography signal analysis for repeated isokinetic dumbbell curl exercise, J. Mech. Sci. Technol., 30 (2016), 5329-5336. |
[16] | S. Thongpanja, A. Phinyomark, P. Phukpattaranont, C. Limsakul, A feasibility study of fatigue and muscle contraction indices based on EMG time-dependent spectral analysis, Proced. Eng., 32 (2012), 239-245. |
[17] | M. Vitor-Costa, H. Bortolotti, T. Camala, R. da Silva, T. Ahrao, A. de Moraes, et al., EMG spectral analysis of incremental exercise in cyclists and non-cyclists using Fourier and Wavelet transforms, Rev. Bras. Cineantropom. Desempenho, 14 (2012), 660-670. |
[18] | S. K. Chowdhury, A. D. Nimbarte, M. Jaridi, R. C. Creese, Discrete Wavelet transform analysis of surface electromyography for the fatigue assessment of neck and shoulder muscles, J. Electromyogr. Kinesiol., 23 (2013), 995-1003. |
[19] | B. M. Idrees, O. Farooq, Estimation of Muscle Fatigue Using Wavelet Decomposition, in Fifth International Conference on Digital Information Processing and Communications (ICDIPC 2015), 2015, 267-271. |
[20] | M. Sarillee, M. Hariharan, M. N. Anas, M. I. Omar, M. N. Aishah, Y. Ck, et al., Classification of muscle fatigue condition using multi-sensors, in 2015 IEEE International Conference on Control System, Computing and Engineering (ICCSCE), 2015, 27-29. |
[21] | D. R. Rogers, D. T. MacIsaac, A comparison of EMG-based muscle fatigue assessments during dynamic contractions, J. Electromyogr. Kinesiol., 23 (2013), 1004-1011. |
[22] | D. Cuesta-Frau, M. Varela-Entrecanales, A. Molina-Picó, B. Vargas, Patterns with equal values in Permutation Entropy: Do they really matter for biosignal classification?, Complexity, 2018 (2018), 1-16. |
[23] | S. A. Rawashdeh, D. A. Rafeldt, T. L. Uhl, J. E. Lumpp, Wearable motion capture unit for shoulder injury prevention, in 2015 IEEE 12th International Conference on Wearable and Implantable Body Sensor Networks (BSN), 2015, 1-6. |
[24] | M. Brzycki, Strength testing: Predicting a one-rep max from repetitions to fatigue, J. Phys. Educ. Recreat. Dance, 64 (1993), 88-90. |
[25] | S. Solnik, P. Rider, K. Steinweg, P. Devita, T. Hortobgyi, Teager-Kaiser energy operator signal conditioning improves EMG onset detection, Eur. J. Appl. Physiol., 110 (2010), 489-498. |
[26] | G. Staude, Onset detection in surface electromyographic signals: A systematic comparison of methods, EURASIP J. Adv. Sig. Pr., 2001 (2001), 67-81. |
[27] | G. H. Staude, Precise onset detection of human motor responses using a whitening filter and the Log-Likelihood-Ratio Test, IEEE Trans. Biomed. Eng., 48 (2001), 1292-1305. |
[28] | G. Staude, W. Wolf, Objective motor response onset detection in surface myoelectric signals, Med. Eng. Phys., 21 (1999), 449-467. |
[29] | P. Bhat, A. Gupta, A novel approach to detect localized muscle fatigue during isometric exercises, in 2016 IEEE 13th International Conference on Wearable and Implantable Body Sensor Networks (BSN), 2016, 224-229. |
[30] | F. Mohamed, Al-Mulla, M. Colley, An autonomous wearable system for predicting and detecting localised muscle fatigue, Sensors, 11 (2011), 1542-1557. |
[31] | B. Everitt, S. Landau, M. Leese, D. Stahl, Hierarchical clustering, in Cluster Analysis, 5th edition, 2011, 71-110. |
[32] | T. Strauss, M. J. Von Maltitz, Generalising Ward's method for use with Manhattan distances, PLoS ONE, 12 (2017), 1-21. |
[33] | C. Bandt, B. Pompe, Permutation Entropy: A natural complexity measure for time series, Phys. Rev. Lett., 88 (2002). |
[34] | D. Cuesta-Frau, J. P. Murillo-Escobar, D. A. Orrego, E. Delgado-Trejos, Embedded dimension and time series length. Practical influence on permutation entropy and its applications, Entropy, 21 (2019), 1-25. |
[35] | M. Riedl, A. Müller, N. Wessel, Practical considerations of permutation entropy: A tutorial review, Eur. Phys. J., 222 (2013), 249-262. |
[36] | F. N. Jamaluddin, S. A. Ahmad, S. B. M. Noor, W. Z. W. Hassan, A. Yaacob, Y. Adam, Performance of DWT and SWT in muscle fatigue Detection, in 2015 IEEE Student Symposium in Biomedical Engineering and Sciences (ISSBES), Shah Alam, Malaysia, 2015, 50-53. |
[37] | K. B. Smale, M. S. Shourijeh, D. L. Benoit, Use of muscle synergies and Wavelet transforms to identify fatigue during squatting, J. Electromyogr. Kinesiol., 28 (2016), 158-166. |
[38] | L. Kahl, U. G. Hofmann, Comparison of algorithms to quantify muscle fatigue in upper limb muscles based on sEMG signals, Med. Eng. Phys., 38 (2016), 1260-1269. |
[39] | T. W. Beck, X. Ye, N. P. Wages, Local muscle endurance is associated with fatigue-based changes in electromyographic spectral properties, but not with conduction velocity, J. Electromyogr. Kinesiol., 25 (2015), 451-456. |
[40] | R. B. Graham, M. P. Wachowiak, B. J. Gurd, The assessment of muscular effort, fatigue, and physiological adaptation using EMG and wavelet analysis, PLoS ONE, 10 (2015), 1-13. |
[41] | E. F. Shair, T. N. S. T. Zawawi, A. R. Abdullah, N. H. Shamsudin, sEMG Signals analysis using time-frequency distribution for symmetric and asymmetric lifting, in 2015 International Symposium on Technology Management and Emerging Technologies (ISTMET), 2015, 233-237. |
[42] | M. Asefi, S. Moghimi, H. Kalani, A. Moghimi, Dynamic modeling of sEMG-force relation in the presence of muscle fatigue during isometric contractions, Biomed. Signal Proc. Control, 28 (2016), 41-49. |
[43] | A. Samani, C. Pontonnier, G. Dumont, P. Madeleine, Shoulder kinematics and spatial pattern of Trapezius electromyographic activity in real and virtual environments, PLoS ONE, 10 (2015), 1-18. |
[44] | P. Bonato, P. Boissy, U. Della Croce, S. H. Roy, Changes in the surface EMG signal and the biomechanics of motion during a repetitive lifting task, IEEE Tran. on Neu. Sys. and Rehab. Eng., 10 (2002), 38-47. |
[45] | S. Gafner, V. Hoevel, I. M. Punt, S. Schmid, L. Allet, Hip-abductor fatigue influences sagittal plane ankle kinematics and shank muscle activity during a single-leg forward jump, J. Electromyogr. Kinesiol., 43 (2018), 75-81. |
[46] | E. Coventry, K. M. O. Connor, B. A. Hart, J. E. Earl, K. T. Ebersole, The effect of lower extremity fatigue on shock attenuation during single-leg landing, Clin. Biomech., 21 (2006), 1090-1097. |
[47] | J. Augustsson, R. Thomeé, C. Lindén, M. Folkesson, R. Tranberg, J. Karlsson, Single-leg hop testing following fatiguing exercise: Reliability and biomechanical analysis, Scand. J. Med. Sci. Sports., 16 (2006), 111-120. |
[48] | J. L. R. Jayalath, N. Weerakkody, R. Bini, Effects of fatigue on ankle biomechanics during jumps: A systematic review, J. Electromyogr. Kinesiol., 42 (2018), 81-91. |
[49] | K. F. Orishimo, I. J. Kremenic, Effect of Fatigue on Single-Leg Hop Landing Biomechanics, J. Appl. Biomech., 22 (2006), 245-254. |
[50] | C. M. Davidson, G. De Vito, M. M. Lowery, Effect of oral glucose supplementation on surface EMG during fatiguing dynamic exercise, in 2016 38th Annual International Conference of the IEEE Engineering in Medicine and Biology Society (EMBC), 2016, 3498-3502. |
[51] | S. Zhang, A novel portable one lead ECG monitor with low-cost and long-time recording based on NUC501, in 2010 Chinese Control and Decision Conference, 2010, 276-279 |
[52] | D. Sun, E. Koutsos, P. Georgiou, Comparison of sEMG bit-stream modulators for cross-correlation based muscle fatigue estimation, in 2016 IEEE International Symposium on Circuits and Systems (ISCAS), 2016, 838-841. |
[53] | K. S. Urbinati, A. D. Vieira, C. Papcke, R. Pinheiro, P. Nohama and M. Scheeren, Physiological and biomechanical fatigue responses in Karate: A case study, Open Sports Sci. J., 10 (2017), 286-293. |
[54] | G. C. Lessi, R. S. Silva, F. V. Serrão, Comparison of the effects of fatigue on kinematics and muscle activation between men and women after anterior cruciate ligament reconstruction, Phys. Ther. Sport., 31 (2018), 29-34. |
[55] | K. Marri, R. Swaminathan, Classification of muscle fatigue in dynamic contraction using surface electromyography signals and multifractal singularity spectral analysis, J. Dyn. Sys. Meas. Control, 138 (2017), 1-10. |
[56] | N. Makaram, R. Swaminathan, A binary bat approach for identification of fatigue condition from sEMG signals, in International Conference on Swarm, Evolutionary, and Memetic Computing, 2014, 480-489. |
[57] | K. Marri, R. Swaminathan, Classification of muscle fatigue using surface electromyography signals and multifractals, in 2015 12th International Conference on Fuzzy Systems and Knowledge Discovery (FSKD), New Jersey, EEUU, 2015, 669-674. |
[58] | F. Sepulveda, M. Al-Mulla, B. A. Bader, Optimal elbow angle for extracting sEMG signals during fatiguing dynamic contraction, Computers, 4 (2015), 251-264. |
[59] | A. B. Piek, I. Stolz, K. Keller, Algorithmics, possibilities and limits of ordinal pattern based entropies, Entropy, 21 (2019), 1-24. |
1. | Inyeol Yun, Jinpyeo Jeung, Yonghun Song, Yoonyoung Chung, Non-Invasive Quantitative Muscle Fatigue Estimation Based on Correlation Between sEMG Signal and Muscle Mass, 2020, 8, 2169-3536, 191751, 10.1109/ACCESS.2020.3029792 | |
2. | Inyeol Yun, Jinpyeo Jeung, Hyungsub Lim, Jieun Kang, Sangyeop Lee, Seongmin Park, Suwon Seong, Soojin Park, Kilwon Cho, Yoonyoung Chung, Stable Bioelectric Signal Acquisition Using an Enlarged Surface-Area Flexible Skin Electrode, 2021, 2637-6113, 10.1021/acsaelm.1c00095 | |
3. | Shaojun Zhu, Jinhui Zhao, Yating Wu, Qingshan She, Intermuscular coupling network analysis of upper limbs based on R-vine copula transfer entropy, 2022, 19, 1551-0018, 9437, 10.3934/mbe.2022439 | |
4. | Victor C.H. Chan, Gwyneth B. Ross, Allison L. Clouthier, Steven L. Fischer, Ryan B. Graham, The role of machine learning in the primary prevention of work-related musculoskeletal disorders: A scoping review, 2022, 98, 00036870, 103574, 10.1016/j.apergo.2021.103574 | |
5. | Hong-qi Xu, Yong-tai Xue, Zi-jian Zhou, Koon Teck Koh, Xin Xu, Ji-peng Shi, Shou-wei Zhang, Xin Zhang, Jing Cai, Retentive capacity of power output and linear versus non-linear mapping of power loss in the isotonic muscular endurance test, 2021, 11, 2045-2322, 10.1038/s41598-021-02116-2 | |
6. | Bei Liu, Wenbin Tan, Xian Zhang, Ziqi Peng, Jing Cao, Recognition study of denatured biological tissues based on multi-scale rescaled range permutation entropy, 2022, 19, 1551-0018, 102, 10.3934/mbe.2022005 | |
7. | Yue Ren, Bingquan Luo, Jun Chu, Fahd Abd Algalil, Biomechanical Research on Special Ability of Long Jump Take-Off Muscle Based on Multisource Information Fusion, 2022, 2022, 1754-2103, 1, 10.1155/2022/2556087 | |
8. | Philippe Ravier, Antonio Dávalos, Meryem Jabloun, Olivier Buttelli, The Refined Composite Downsampling Permutation Entropy Is a Relevant Tool in the Muscle Fatigue Study Using sEMG Signals, 2021, 23, 1099-4300, 1655, 10.3390/e23121655 | |
9. | Hao Tang, Shurun Wang, Qi Tan, Bin Wang, A Double Threshold Adaptive Method for Robust Detection of Muscle Activation Intervals From Surface Electromyographic Signals, 2022, 71, 0018-9456, 1, 10.1109/TIM.2022.3169751 | |
10. | E. Pereira-Montiel, E. Pérez-Giraldo, J. Mazo, D. Orrego-Metaute, E. Delgado-Trejos, D. Cuesta-Frau, J. Murillo-Escobar, Automatic sign language recognition based on accelerometry and surface electromyography signals: A study for Colombian sign language, 2022, 71, 17468094, 103201, 10.1016/j.bspc.2021.103201 | |
11. | Chih-Kun Hsiao, Yi-Jung Tsai, Chih-Wei Lu, Jen-Chou Hsiung, Hao-Yuan Hsiao, Yung-Chuan Chen, Yuan-Kun Tu, Effects of Kinesio taping on forearm supination/pronation performance fatigability, 2022, 23, 1471-2474, 10.1186/s12891-022-05068-4 | |
12. | Divya Sasidharan, Venugopal G, S Ramakrishnan, 2022, Muscle Fatigue Analysis by Visualization of Dynamic Surface EMG Signals Using Markov Transition Field, 978-1-7281-2782-8, 3611, 10.1109/EMBC48229.2022.9871981 | |
13. | Xiaoguang Liu, Xu Cui, Tie Liang, Cunguang Lou, Hongrui Wang, Xiuling Liu, Characteristics analysis of muscle function network and its application to muscle compensatory in repetitive movement, 2023, 83, 17468094, 104639, 10.1016/j.bspc.2023.104639 | |
14. | Joshua Liddy, Michael Busa, Considerations for Applying Entropy Methods to Temporally Correlated Stochastic Datasets, 2023, 25, 1099-4300, 306, 10.3390/e25020306 | |
15. | Yuriy Prylutskyy, Dmytro Nozdrenko, Olga Gonchar, Svitlana Prylutska, Kateryna Bogutska, Eric Täuscher, Peter Scharff, Uwe Ritter, Rohit Sharma, The Residual Effect of C60 Fullerene on Biomechanical and Biochemical Markers of the Muscle Soleus Fatigue Development in Rats, 2023, 2023, 1687-4129, 1, 10.1155/2023/2237574 | |
16. | DIVYA SASIDHARAN, G. VENUGOPAL, RAMAKRISHNAN SWAMINATHAN, COMPLEXITY ANALYSIS OF SURFACE ELECTROMYOGRAPHY SIGNALS UNDER FATIGUE USING HJORTH PARAMETERS AND BUBBLE ENTROPY, 2023, 23, 0219-5194, 10.1142/S0219519423400511 | |
17. | Shurun Wang, Hao Tang, Bin Wang, Jia Mo, A Novel Approach to Detecting Muscle Fatigue Based on sEMG by Using Neural Architecture Search Framework, 2023, 34, 2162-237X, 4932, 10.1109/TNNLS.2021.3124330 | |
18. | Na Li, Rui Zhou, Bharath Krishna, Ashirbad Pradhan, Hyowon Lee, Jiayuan He, Ning Jiang, Non-invasive Techniques for Muscle Fatigue Monitoring: A Comprehensive Survey, 2024, 56, 0360-0300, 1, 10.1145/3648679 | |
19. | Divya Sasidharan, Venugopal Gopinath, Ramakrishnan Swaminathan, A Proposal to Analyze Muscle Dynamics Under Fatiguing Contractions Using Surface Electromyography Signals and Fuzzy Recurrence Network Features, 2023, 22, 0219-4775, 10.1142/S0219477523500335 | |
20. | Beāte Banga, Alexei Katashev, Modris Greitāns, 2023, Chapter 4, 978-3-031-37131-8, 25, 10.1007/978-3-031-37132-5_4 | |
21. | Baohua Hu, Yong Wang, Jingsong Mu, A new fractional fuzzy dispersion entropy and its application in muscle fatigue detection, 2023, 21, 1551-0018, 144, 10.3934/mbe.2024007 |
Feature | Abbreviation | Formula |
Maximum angle | Maxθ | maxθn |
Mean angle | Meanθ | 1N∑Nn=1θn |
Integral of angle | Intθ | ∑Nn=1θn |
Maximum velocity | Maxω | maxωn |
Minimum velocity | Minω | minωn |
Mean velocity | Meanω | 1N∑Nn=1ωn |
Integral of velocity | Intω | ∑Nn=1ωn |
Mean speed | Mean|ω| | 1N∑Nn=1|ω| |
Maximum acceleration | Maxα | maxαn |
Minimum acceleration | Minα | minαn |
Mean acceleration | Meanα | 1N∑Nn=1αn |
Integral of acceleration | Intα | ∑Nn=1αn |
Feature | Equation |
Permutation Entropy (PE) | ∑m!j=1−p′(πj)log2p′(πj) |
Root Mean Square (RMS) | √1N∑Nn=1|x2n| |
Mean Frecuency (MF) | ∑Fmaxf=FminfS[f]∑Fmaxf=FminS[f] |
Median Frecuency (Fmed) | ∑Fmedf=FminS[f]=∑Fmaxf=FmedS[f] |
p′(πj) is the relative frequency of the ordinal pattern πj X is a sEMG signal as X={x1,x2,⋯xN} S[f] is the power spectrum density of a signal at frequency f |
Biomechanical features | Correlation |
Max |
0.82 |
Int |
0.81 |
Mean |
0.81 |
Max |
0.7 |
Mean |
0.68 |
Min |
0.55 |
Int |
0.46 |
Max |
0.31 |
Mean |
0.3 |
Min |
0.23 |
Int |
0.22 |
Mean |
0.15 |
Feauture | ANOVA test | LSD | ||
G1 vs G2 | G1 vs G3 | G2 vs G3 | ||
PE | p<<0.01 | p<<0.01 | p<<0.01 | p<<0.01 |
Fmed | p<<0.01 | p<<0.01 | p<<0.01 | p<<0.01 |
FM | p<<0.01 | 0.0074 | p<<0.01 | 0.25 |
RMS | p<<0.01 | 0.4856 | 0.0073 | p<<0.01 |
States | Reference | Contraction Type | Muscle | Classification method | Features | Accuracy |
Non-Fatigue vs Transition-to-Fatigue | [30] | Isometric | Biceps | LDA | 1D spectro-composite feature | 90.37% |
[12] | Isometric | Biceps | deep belief networks | Raw Signal | 66.32% | |
In this Work | Dynamic | Deltoid | Logistic Regression | PE | 71.0% | |
Transition-to-Fatigue vs Fatigue | In this Work | Dynamic | Deltoid | Logistic Regression | PE | 68.0% |
Non-Fatigue vs Fatigue | [12] | Isometric | Biceps | DBNs | Raw Signal | 97.60% |
[30] | Isometric | Biceps | Logistic Regression | 1D spectro-composite feature | 84.8% | |
[55] | Dynamic | Biceps | k-NN | Multifractal features | 88% | |
[56] | Dynamic | Biceps | SVM | Renyi entropy | 86.7% | |
[57] | Dynamic | Biceps | Logistic Regression | Multifractal features-Infomation Gain | 84% | |
[57] | Dynamic | Biceps | k-NN | Multifractal features-Infomation Gain | 82% | |
[55] | Dynamic | Biceps | Logistic Regression | Multifractal features | 82% | |
[58] | Dynamic | Biceps | LDA | Pseudo-wavelet | 78.45% | |
In this Work | Dynamic | Deltoid | Logistic Regression | PE | 80.0% |
Feature | Abbreviation | Formula |
Maximum angle | Maxθ | maxθn |
Mean angle | Meanθ | 1N∑Nn=1θn |
Integral of angle | Intθ | ∑Nn=1θn |
Maximum velocity | Maxω | maxωn |
Minimum velocity | Minω | minωn |
Mean velocity | Meanω | 1N∑Nn=1ωn |
Integral of velocity | Intω | ∑Nn=1ωn |
Mean speed | Mean|ω| | 1N∑Nn=1|ω| |
Maximum acceleration | Maxα | maxαn |
Minimum acceleration | Minα | minαn |
Mean acceleration | Meanα | 1N∑Nn=1αn |
Integral of acceleration | Intα | ∑Nn=1αn |
Feature | Equation |
Permutation Entropy (PE) | ∑m!j=1−p′(πj)log2p′(πj) |
Root Mean Square (RMS) | √1N∑Nn=1|x2n| |
Mean Frecuency (MF) | ∑Fmaxf=FminfS[f]∑Fmaxf=FminS[f] |
Median Frecuency (Fmed) | ∑Fmedf=FminS[f]=∑Fmaxf=FmedS[f] |
p′(πj) is the relative frequency of the ordinal pattern πj X is a sEMG signal as X={x1,x2,⋯xN} S[f] is the power spectrum density of a signal at frequency f |
Biomechanical features | Correlation |
Max |
0.82 |
Int |
0.81 |
Mean |
0.81 |
Max |
0.7 |
Mean |
0.68 |
Min |
0.55 |
Int |
0.46 |
Max |
0.31 |
Mean |
0.3 |
Min |
0.23 |
Int |
0.22 |
Mean |
0.15 |
Feauture | ANOVA test | LSD | ||
G1 vs G2 | G1 vs G3 | G2 vs G3 | ||
PE | p<<0.01 | p<<0.01 | p<<0.01 | p<<0.01 |
Fmed | p<<0.01 | p<<0.01 | p<<0.01 | p<<0.01 |
FM | p<<0.01 | 0.0074 | p<<0.01 | 0.25 |
RMS | p<<0.01 | 0.4856 | 0.0073 | p<<0.01 |
States | Reference | Contraction Type | Muscle | Classification method | Features | Accuracy |
Non-Fatigue vs Transition-to-Fatigue | [30] | Isometric | Biceps | LDA | 1D spectro-composite feature | 90.37% |
[12] | Isometric | Biceps | deep belief networks | Raw Signal | 66.32% | |
In this Work | Dynamic | Deltoid | Logistic Regression | PE | 71.0% | |
Transition-to-Fatigue vs Fatigue | In this Work | Dynamic | Deltoid | Logistic Regression | PE | 68.0% |
Non-Fatigue vs Fatigue | [12] | Isometric | Biceps | DBNs | Raw Signal | 97.60% |
[30] | Isometric | Biceps | Logistic Regression | 1D spectro-composite feature | 84.8% | |
[55] | Dynamic | Biceps | k-NN | Multifractal features | 88% | |
[56] | Dynamic | Biceps | SVM | Renyi entropy | 86.7% | |
[57] | Dynamic | Biceps | Logistic Regression | Multifractal features-Infomation Gain | 84% | |
[57] | Dynamic | Biceps | k-NN | Multifractal features-Infomation Gain | 82% | |
[55] | Dynamic | Biceps | Logistic Regression | Multifractal features | 82% | |
[58] | Dynamic | Biceps | LDA | Pseudo-wavelet | 78.45% | |
In this Work | Dynamic | Deltoid | Logistic Regression | PE | 80.0% |