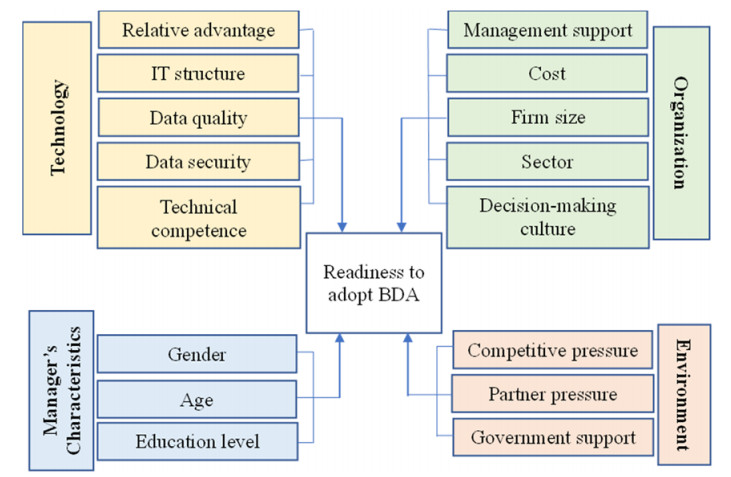
Recently, fuzzy dispersion entropy (DispEn) has attracted much attention as a new nonlinear dynamics method that combines the advantages of both DispEn and fuzzy entropy. However, it suffers from limitation of insensitivity to dynamic changes. To solve this limitation, we proposed fractional fuzzy dispersion entropy (FFDispEn) based on DispEn, a novel fuzzy membership function and fractional calculus. The fuzzy membership function was defined based on the Euclidean distance between the embedding vector and dispersion pattern. Simulated signals generated by the one-dimensional (1D) logistic map were used to test the sensitivity of the proposed method to dynamic changes. Moreover, 29 subjects were recruited for an upper limb muscle fatigue experiment, during which surface electromyography (sEMG) signals of the biceps brachii muscle were recorded. Both simulated signals and sEMG signals were processed using a sliding window approach. Sample entropy (SampEn), DispEn and FFDispEn were separately used to calculate the complexity of each frame. The sensitivity of different algorithms to the muscle fatigue process was analyzed using fitting parameters through linear fitting of the complexity of each frame signal. The results showed that for simulated signals, the larger the fractional order q, the higher the sensitivity to dynamic changes. Moreover, DispEn performed poorly in the sensitivity to dynamic changes compared with FFDispEn. As for muscle fatigue detection, the FFDispEn value showed a clear declining tendency with a mean slope of −1.658 × 10−3 as muscle fatigue progresses; additionally, it was more sensitive to muscle fatigue compared with SampEn (slope: −0.4156 × 10−3) and DispEn (slope: −0.1675 × 10−3). The highest accuracy of 97.5% was achieved with the FFDispEn and support vector machine (SVM). This study provided a new useful nonlinear dynamic indicator for sEMG signal processing and muscle fatigue analysis. The proposed method may be useful for physiological and biomedical signal analysis.
Citation: Baohua Hu, Yong Wang, Jingsong Mu. A new fractional fuzzy dispersion entropy and its application in muscle fatigue detection[J]. Mathematical Biosciences and Engineering, 2024, 21(1): 144-169. doi: 10.3934/mbe.2024007
[1] | Fang Liu, Yanfei Du . Spatiotemporal dynamics of a diffusive predator-prey model with delay and Allee effect in predator. Mathematical Biosciences and Engineering, 2023, 20(11): 19372-19400. doi: 10.3934/mbe.2023857 |
[2] | Kawkab Al Amri, Qamar J. A Khan, David Greenhalgh . Combined impact of fear and Allee effect in predator-prey interaction models on their growth. Mathematical Biosciences and Engineering, 2024, 21(10): 7211-7252. doi: 10.3934/mbe.2024319 |
[3] | Yun Kang, Sourav Kumar Sasmal, Amiya Ranjan Bhowmick, Joydev Chattopadhyay . Dynamics of a predator-prey system with prey subject to Allee effects and disease. Mathematical Biosciences and Engineering, 2014, 11(4): 877-918. doi: 10.3934/mbe.2014.11.877 |
[4] | Mengyun Xing, Mengxin He, Zhong Li . Dynamics of a modified Leslie-Gower predator-prey model with double Allee effects. Mathematical Biosciences and Engineering, 2024, 21(1): 792-831. doi: 10.3934/mbe.2024034 |
[5] | Juan Ye, Yi Wang, Zhan Jin, Chuanjun Dai, Min Zhao . Dynamics of a predator-prey model with strong Allee effect and nonconstant mortality rate. Mathematical Biosciences and Engineering, 2022, 19(4): 3402-3426. doi: 10.3934/mbe.2022157 |
[6] | A. Q. Khan, I. Ahmad, H. S. Alayachi, M. S. M. Noorani, A. Khaliq . Discrete-time predator-prey model with flip bifurcation and chaos control. Mathematical Biosciences and Engineering, 2020, 17(5): 5944-5960. doi: 10.3934/mbe.2020317 |
[7] | Yuhong Huo, Gourav Mandal, Lakshmi Narayan Guin, Santabrata Chakravarty, Renji Han . Allee effect-driven complexity in a spatiotemporal predator-prey system with fear factor. Mathematical Biosciences and Engineering, 2023, 20(10): 18820-18860. doi: 10.3934/mbe.2023834 |
[8] | Kunlun Huang, Xintian Jia, Cuiping Li . Analysis of modified Holling-Tanner model with strong Allee effect. Mathematical Biosciences and Engineering, 2023, 20(8): 15524-15543. doi: 10.3934/mbe.2023693 |
[9] | Claudio Arancibia–Ibarra, José Flores . Modelling and analysis of a modified May-Holling-Tanner predator-prey model with Allee effect in the prey and an alternative food source for the predator. Mathematical Biosciences and Engineering, 2020, 17(6): 8052-8073. doi: 10.3934/mbe.2020408 |
[10] | Yongli Cai, Malay Banerjee, Yun Kang, Weiming Wang . Spatiotemporal complexity in a predator--prey model with weak Allee effects. Mathematical Biosciences and Engineering, 2014, 11(6): 1247-1274. doi: 10.3934/mbe.2014.11.1247 |
Recently, fuzzy dispersion entropy (DispEn) has attracted much attention as a new nonlinear dynamics method that combines the advantages of both DispEn and fuzzy entropy. However, it suffers from limitation of insensitivity to dynamic changes. To solve this limitation, we proposed fractional fuzzy dispersion entropy (FFDispEn) based on DispEn, a novel fuzzy membership function and fractional calculus. The fuzzy membership function was defined based on the Euclidean distance between the embedding vector and dispersion pattern. Simulated signals generated by the one-dimensional (1D) logistic map were used to test the sensitivity of the proposed method to dynamic changes. Moreover, 29 subjects were recruited for an upper limb muscle fatigue experiment, during which surface electromyography (sEMG) signals of the biceps brachii muscle were recorded. Both simulated signals and sEMG signals were processed using a sliding window approach. Sample entropy (SampEn), DispEn and FFDispEn were separately used to calculate the complexity of each frame. The sensitivity of different algorithms to the muscle fatigue process was analyzed using fitting parameters through linear fitting of the complexity of each frame signal. The results showed that for simulated signals, the larger the fractional order q, the higher the sensitivity to dynamic changes. Moreover, DispEn performed poorly in the sensitivity to dynamic changes compared with FFDispEn. As for muscle fatigue detection, the FFDispEn value showed a clear declining tendency with a mean slope of −1.658 × 10−3 as muscle fatigue progresses; additionally, it was more sensitive to muscle fatigue compared with SampEn (slope: −0.4156 × 10−3) and DispEn (slope: −0.1675 × 10−3). The highest accuracy of 97.5% was achieved with the FFDispEn and support vector machine (SVM). This study provided a new useful nonlinear dynamic indicator for sEMG signal processing and muscle fatigue analysis. The proposed method may be useful for physiological and biomedical signal analysis.
The vigorous advancement of internet technology continues to generate large volumes of data through several sources, such as media, the cloud, the Web, the Internet of Things and databases [1]. The aggregation of these sources is referred to as big data, and companies are looking to process and analyze these huge data sets to extract benefits [2]. As a result, numerous studies have shown the benefits of BDA in organizations. Specifically, the use of BDA enhances the prediction of future product development trends, which improves the decision-making process [2,3,4,5], and enhances supply chain systems [6]. BDA prediction is also paramount in the promotion of firm performance [7,8,9], improvement of marketing efficiency [5,10] and prediction of market trends [5,11]. The momentousness of BDA adoption culminates with the development of a sustainable dynamic economic system that takes advantage of current contextual demands [12]. Evidence shows that firms that succeed in implementing BDA primarily graduate into major cross-national corporations. Examples of such corporations include Google, Apple, Twitter, Uber, Walmart, Amazon, IBM Watson, Rolls-Royce, Toyota and others [13]. Despite the benefits of BDA for firms and economic performance, numerous companies still encounter an assortment of barriers that inhibit the adoption of BDA, especially by SMEs [14,15,16]. For most developing economies, SMEs are pivotal in economic development and validation of BDA implementation. However, Coleman et al. [17] indicated that SMEs are still slow in implementing BDA, as they are faced with several barriers in the application of big data [17,18]. Del Vecchio et al. [19] pointed out the challenges and benefits of big data for SMEs. Noonpakdee et al. [20] presented barriers when Thailand SMEs adopted big data. Similarly, Chuah and Thurusamry [21] mentioned the challenges of SMEs in Malaysia using BDA. In addition, Mangla et al. [22] demonstrated the performance of SMEs' adoptions of BDA in India. Park and Kim [23] and Maroufkhani et al. [9] identified drivers of big data adoption among Korean and Iranian SMEs. However, the majority of these studies concentrate on the advantages and efficiency of BDA adoption, as well as the challenges that SMEs face when performing BDA. Previous research examining the factors influencing the use of BDA by SMEs is still scarce. With limited studies on BDA application by SMEs, such as in Vietnam, it becomes very difficult for SMEs to adopt BDA. The Technology-Organization-Environment (TOE) framework is composed of technology, organization and environment pillars [24]. It is considered to be the most comprehensive and flexible approach for examining company decisions on the adoption and implementation of information technology-based innovations [25]. Therefore, this study applies the TOE framework and four data mining algorithms (CHAID, Bayesian networks, neural networks and C5.0) to identify the predictors of readiness to adopt BDA by SMEs. The study was guided by the following objectives:
1) To identify the best model for the predicting factors' influences on the readiness to adopt BDA among SMEs and
2) To predict the key factors that affect the readiness to adopt BDA in SMEs.
The findings will be useful for managers, policymakers and providers to understand the influences of BDA adoption readiness. Managers can, therefore, build competitive strategies to enhance company performance through the use of BDA. Additionally, the study proves new techniques that can be used to predict the factors influencing enterprise readiness to adopt BDA.
Big data includes both structured and unstructured large volumes of data, and their analysis requires specific processing. The key features of the big data process are categorized into 3 Vs: (i) volume, (ii) velocity and (iii) variety. In this case, volume depicts the amount of information in the dataset, while velocity refers to the rate at which data are created. Variety indicates the different forms of data that are created. Zhong et al. [26] added two more Vs, verification and value, to characterize big data as a "5Vs" data source. In this case, verification concerns bad data that need to be verified, whereas value addresses the economic and social costs of application. On the other hand, Saggi and Jain [27] classified big data features into volume, velocity, variety, valence, veracity, variability and value to produce the "7Vs" classification. The valence is related to the complexity of the data, and veracity reflects accuracy within the dataset, while the inconsistencies in all data are mostly responsible for variability.
Ideally, BDA involves two components, big data and business analytics [5]. The former provides the foundation for informational and technological analysis for business activities, whereas the latter provides valuable insights necessary for the improvement of the decision process in the business unit. This has a multidisciplinary benefit that promotes firm business performance [28]. For example, big data has been adopted in the manufacturing sector [9], the health care sector [29], the service sector [26] and the hospitality industry [30]. Dubey et al. [31] argued that BDA presents unequivocal and fundamental impact effects on the swiftness of supply chains and competitive advantage. Previous studies that presented benefits, challenges and performance applied big data in SMEs [17,19,20,22,32,33]. For example, Park and Kim [23] used the analytic hierarchy process and regression analysis and found that benefits received, technological abilities, financial abilities and data quality are the major factors predicting the intention to apply big data among Korean companies. Mangla et al. [22] applied structural equation modeling (SEM) to show that BDA increased project performance in Indian SMEs. Similarly, Maroufkhani et al. [9] and Lutfi et al. [34] also used partial least squares structural equation modeling (PLS-SEM) to identify the elements impacting the intentions of Iranian SMEs and Jordanian SMEs to use BDA. In addition, Sun et al. [35], Maroufkhani et al. [36] and Baig et al. [37] used a review of related articles to figure out drivers of an organization's inclination toward the utilization of big data for businesses purposes. Clearly, most of the previous studies on factors affecting the intentions of BDA adoption used latent variables. This leads to the limitation of independent factors [38]. The observed variables (e.g., demographic variables, sector, firm size) are rarely included in the research model. This research works to bridge this gap.
The TOE framework is useful in revealing the drivers of decisions to embrace new information technology [24]. It is a threefold framework consisting of technology, organization and environment. The technology pillar defines factors associated with tools, software, IT infrastructure, etc. which affect decisions to apply big data by individuals and/or organizations. The organization pillar defines the capacity of a firm to acquire competence in the employment of multiple resources required for the operation of information systems in firms. The environmental pillar consists of multiple industrial features, e.g., competitors and vendor support, directly or indirectly affecting the operations of enterprises. The TOE framework is considered to be flexible and is widely used in technology application studies amongst companies [39]. Some previous studies on BDA adoption have applied the TOE framework. Sun et al. [35] and Baig et al. [37] laid out a synopsis of the determinants of big data adoption using the TOE framework. Park et al. [40] and Park and Kim [23] applied the TOE framework to ascertain the drivers of big data adoption among Korean companies. Similarly, Lai et al. [41], used the TOE framework to identify the determinants of BDA adoption by Chinese firms. Maroufkhani et al. [9] applied the framework to find out the determinants of BDA application among SMEs in Iranian. However, previous studies evaluating factors affecting BDA mostly refer to latent variables without considering observed variables. Therefore, the present study extends the TOE framework to understand the drivers of BDA adoption. The research model of this study is shown in Figure 1.
The technology pillar involves intra- and inter-organizational drivers that influence company decisions to embrace new information technology [42]. In this dimension, the first factor mentioned is the relative advantage, which outlines the level to which the new proposed technology provides greater benefit for firms [43]. According to Ghobakhloo et al. [44], SMEs are only willing to embrace new technology if the said advantages outweigh the performance of existing technology. IT infrastructure is salient for organizational competitiveness [30], reflecting a firm's ability to operationalize information systems. However, SMEs often lack IT resources, undercutting their abilities for data collection and analysis [19]. According to Wang and Wang [32], the lack of IT specialists is a major drawback for most SMEs in attaining flexibility in IT infrastructure usage. Data quality is an important factor leading to the success of enterprises' BDA adoption.
Big data stockpiles could be structured, semi-structured or unstructured. Organizations must choose specific software to ensure the quality of the data as well as the efficiency of BDA [14]. Park and Kim [23] mentioned that data quality has a great influence on big data adoption decisions among Korean firms. The security issue is critical for firms' decisions to adopt BDA. Third parties are privy to personal and company information, thus exposing individuals and companies to cybercrime [45]. Therefore, data security is a key factor affecting the decisions of enterprises to adopt BDA [35]. Technical competence refers to expertise, which is a prerequisite for analyzing big data by employees. Yadegaridehkordi et al. [30] indicated that enough knowledge for staff to analyze information technology is an important factor affecting the application of innovation in organizations. Alharthi et al. [14] concluded that staff lack of BDA skills is a barrier when companies adopt BDA.
The organizational dimension represents different organizational conditions that affect readiness toward the adoption of BDA. The first element is management support, which is critically vital in the adoption of an innovation [46]. If managers realize the benefits of BDA adoption, they can allocate the resources needed for implementation. By contrast, if management does not see the profits of BDA adoption, they will oppose the application of that data [47]. Second, the adoption of BDA is attached to a cost factor to maintain and develop the application of big data [35]. In this regard, company development-related costs are usually funded through support from the financial institution. Such support tends to be limited for SMEs compared to larger firms, thereby undermining the adoption of BDA by small companies [17]. Hence, firm size is considered an essential driver for the adoption of technological innovations [24]. The type of industry is another driver believed to influence the intentions to apply new technology in enterprises. Gangwar [48] pointed out that there was a significant difference between the manufacturing and the service sectors regarding BDA. Finally, decision-making culture is another factor that influences the adoption of BDA. More often than not, organizations that apply an evidence-based decision-making culture embrace big data analytics to develop evidence that enhances managers' competence for strategic decision-making, thereby improving enterprise profitability [35].
Environmental factors include external factors that the organization may encounter [49]. Factors such as competition pressure, partner pressure and government support are perceived as external drivers of big data adoption by SMEs [23]. Competitive pressure outlines the extent to which competitors affect organizational decisions towards the adoption of new technologies [24]. The role of competition pressure is widely acknowledged in the literature on IT adoption [50,51]. Zhu et al. [52] revealed the importance of the pressure from trading partners in influencing company decisions to adopt and utilize new information technology. In addition, the government also plays a fundamental role in influencing the adoption of information technology. If the government exudes a strong political will and ensures a good institutionally enabling environment for the enrollment of big data technology, firms are often encouraged to develop internal policies for the adoption and implementation of BDA. Such a positive relationship has been confirmed by numerous studies [35,41]. Government support and policy include the provision of public data, fostering of experts, protecting intellectual property and regulation for privacy and security that affect the use of big data by firms [53].
Rojas-Méndez et al. [54] demonstrated that demographic variables (gender, age, education level) are important factors for predicting people's willingness to adopt the technology. In this regard, the manager's level of education is the most important demographic characteristic affecting the application of technology [54]. Parasuraman and Colby [55] pointed out that there is a need for studies focusing on factors such as age, education level, occupation and demographic characteristics to assess the readiness to use the new technology of each person. For this reason, the manager's characteristics dimension is included to predict the determinants of big data adoption by SMEs.
Data mining includes many different algorithms used mainly for classification purposes. CHAID analysis is an algorithm that develops a predictive model that merges predictors that best explain the response variable [56]. A Bayesian network is a probability-based graphical model that represents expertise about an uncertain domain, where individual nodes correspond to some random variable, and each edge represents the conditional probability for the corresponding random variables [56,57]. Neural networks are a set of connected input/output units where each connection has a distinct weight associated with each other [56]. One of the most often used decision tree inducers is the C5.0 model, which divides the sample according to the field that delivers the most information gained at each level.
The four algorithms have some differences. Neural networks are widely used because of their ability to produce results quickly, although their capacity for problem-solving is limited. The CHAID model uses simple predictions based on the frequency distribution of potential problems. The C5.0 model is considered an algorithm with outstanding performance and high accuracy [58].
The data mining technique is applied in research to collect data from questionnaires and predict factors affecting the research problem. For instance, Cortez and Silva [59] collected data from 788 students in a public school in Portugal by questionnaire. The questionnaire included 37 items that mentioned demographics, social and school information. Four algorithms, consisting of decision trees, random tree, neural networks and support vector machines, were used to predict students' mathematics and Portuguese grades in this study. Yukselturk et al. [60] predicted dropout students through four algorithms: k-nearest neighbor, decision tree, naive Bayes and neural network. In that study, data was collected from 189 students in Turkey. The questionnaire included ten variables to predict students who drop out of courses. Applying the data mining technique, the researcher can easily discover unexpected factors [61]. However, studies using the data mining technique to predict factors affecting the adoption of BDA have still not been found.
The questionnaire was literature-based and collected comments from professionals and managers of SMEs. The questionnaire was partitioned into three sections. Section A used thirty-five items collecting data on determinants of readiness to implement big data among SMEs. Section B consisted of nine items assessing the readiness to apply BDA. The first two sections used a seven-point agreement Likert-scale, ranging from 1 for "Strongly Disagree" to 7 for "Strongly Agree." Section C collected data on the respondents' socio-economic characteristics.
The subjects of this study are SMEs involved in manufacturing and service provision. The manufacturing and service sectors are two areas that have important roles in the economies of each country [62]. Manufacturing refers to the activities of people using tools and machines to convert raw materials into finished products, transport them to suppliers and recycle used products [26,63]. Services include areas such as retail, finance, tourism, health, accommodation services, restaurants, etc., whereby the service sector provides services to consumers. The questionnaire was emailed to Vietnamese managers of SMEs that met the eligibility criterion of the study. A total sample of 240 managers of manufacturing and service provider companies participated in the study. The data were collected during the period from September to December 2020.
Table 1 shows the respondents' demographic analysis. The gender proportion showed that the majority of respondents were males (72.5%), followed by females (27.5%). Age distribution was such that the majority of the respondents were aged 30 to 45 (57.9%), with those aged ≥ 46 accounting for 29.2%, and those aged < 30 accounted for 12.9%. The descriptive statistics revealed that 46.7% of managers hold bachelor's degrees, 39.2% hold post-graduate degrees, and only 14.2% have college or vocational training. Firm size showed that the majority of participants were small enterprises (82.5%), and medium enterprises accounted for 17.5%. Among these firms, 50.8% were manufacturing firms, and 49.2% were service firms.
Variable | Type | Frequency | Percentage (%) |
Gender | Male | 174 | 72.5 |
Female | 66 | 27.5 | |
Age | < 30 | 31 | 12.9 |
30–45 | 139 | 57.9 | |
≥ 46 | 70 | 29.2 | |
Education level | College education | 34 | 14.2 |
Bachelor's degree | 112 | 46.7 | |
Master's degree or above | 94 | 39.2 | |
Role of respondent | Chief Executive Officer | 85 | 35.4 |
Executive management | 91 | 37.9 | |
IT management | 64 | 26.7 | |
Sector | Manufacturing | 122 | 50.8 |
Service | 118 | 49.2 | |
Firm size | Small enterprise | 198 | 82.5 |
Medium enterprise | 42 | 17.5 |
In this study, each variable is measured by at least three items based on references. To be more specific, the variables are relative advantage (four items) [51], IT infrastructure (three items) [20], data quality (three items) [41], data security (three items) [64], technical competence (four items) [65], management support (three items) [66], cost (three items) [51], decision-making culture (three items) [35], competitive pressure (three items) [67], partner pressure (three items) [67], government support (three items) [26] and readiness to apply BDA in SMEs (nine items) [37,55,68].
To assess the reliability and validity of latent variables, Cronbach's α value, composite reliability (CR), average variance extracted (AVE) of all constructs and factor loadings of items are shown in Table A1. A preliminary dataset analysis of External Factor Analysis (EFA) was carried out. The KMO (Kaiser-Meyer-Olkin) value was 0.814, being greater than the critical value (0.7) [69], and the Bartlett sphericity test's significant value was p = 0.000, indicating that factor analysis is suitable for the original dataset. Cronbach's α value was computed to assess the reliability of the questionnaire. The reliability test indicated that the value of Cronbach's α for the latent variables ranged between 0.626 and 0.867. According to Hair et al. [70], if the Cronbach's α value is greater than 0.700 (0.600 acceptable), the questionnaire has good internal consistency. Therefore, the questionnaire for this study was found to be consistent and reliable.
All factor loadings (from 0.520 to 0.865) were higher than the acceptable limit (0.5) [69]. The CR of all constructs indicated good internal consistency, being higher than 0.7 [71]. All constructs, except for data quality (0.457) and management support (0.471), had AVE values higher than 0.5, indicating good convergent validity. Taking into consideration the Fornell and Larcker [72] proposal that an AVE value equal to 0.4 can be acceptable if the CR value is greater than 0.6, the data quality and management support variables were accepted in this study because they had a CR value high of 0.7. This proves that all latent variables in this study have acceptable convergent values.
To predict the factors' influences on BDA adoption readiness, the dependent variable (readiness to apply big data in SMEs) was divided into two options based on an average of nine items that identify the readiness to apply BDA among SMEs. The first option was coded "1 = Low readiness, " with the mean values of the nine items < 6.0, and "2 = High readiness" was used with the mean values of the nine items ≥ 6.0. Table 2 and Table 3 present the sixteen independent (input) variables and the dependent (target) variable.
No. | Variable | Data type | Description | |
Technology dimension | ||||
1 | Relative advantage | Continuous | Mean value | |
2 | IT infrastructure | Continuous | Mean value | |
3 | Data quality | Continuous | Mean value | |
4 | Data security | Continuous | Mean value | |
5 | Technical competence | Continuous | Mean value | |
Organization dimension | ||||
6 | Management support | Continuous | Mean value | |
7 | Cost | Continuous | Mean value | |
8 | Firm size | Nominal | 1 = "Small", 2 = "Medium" | |
9 | Sector | Nominal | 1 = "Manufacturing", 2 = "Service" | |
10 | Decision-making culture | Continuous | Mean value | |
Environment dimension | ||||
11 | Competitive pressure | Continuous | Mean value | |
12 | Partner pressure | Continuous | Mean value | |
13 | Government support | Continuous | Mean value | |
Manager's characteristics dimension | ||||
14 | Gender | Nominal | 1 = "Male", 2 = "Female" | |
15 | Age | Nominal | 1 = " < 30", 2 = "30–45", 3 = "≥ 46" | |
16 | Education level | Nominal | 1 = "High school, College/Vocational education", 2 = "Bachelor's degree", 3 = "Master's degree, or above" |
Category | Frequency | Percentage (%) |
1 (Low readiness) | 119 | 49.6 |
2 (High readiness) | 121 | 50.4 |
Total | 240 | 100.0 |
This study used four data mining algorithms that were run through the Statistical Package for Social Sciences (SPSS) 18 software (IBM, Armonk, NY, USA). The algorithms used for the prediction of factors' influences on the adoption readiness of BDA include CHAID, Bayesian networks, neural networks and C5.0. These algorithms are commonly applied in studies that analyze data collected from questionnaires.
CHAID algorithm
CHAID is one of the pioneer algorithms that partition data into multiple subgroups [73]. However, this method does not allow for data pruning. CHAID applies the chi-square independence test to identify the splitting rule for each node. This test performs an automatic split categorization of independent categorical variables from continuous variables. Super-classes are then produced through the merging of the input variables based on statistical analogy, maintaining them if they are statistically dissimilar. A comparative analysis between the super-classes and the target variable is done to assess dependency using the chi-square independence test. The super-class that shows the highest significance is then selected as the splitting criteria for the node.
Bayesian networks algorithm
The Bayesian network is popular, being used in multiple research fields [74]. This method combines qualitative and quantitative variables. A Bayesian network is a directed graph with an additional set of probability distributions. Here, the graph represents the qualitative aspect, whereas the probability distributions represent the quantitative part. In the graph, the nodes denote dubious factors, while the arcs address the presence of a causal connection between two factors. Bayesian networks are very effective in predictive studies. The structure makes inferences from Bayesian networks robust, reduces the differences of estimated parameters and is also robust against overfitting.
Neural network algorithm
Neural networks are modeled from brain functionality. They use numerous connected receptor units that accept messages from other units, processing them and conveying the new message to other units. However, the output of the neural network is difficult to retrace; hence, interpretation becomes hard. These disadvantages are overridden by the complexity and flexibility of the algorithm, transforming it into a robust and comprehensive discriminator that is applicable to resolve varied problems compared to other methods [56].
C5.0 algorithm
The C5.0 algorithm evolved from the C4.5 algorithm as formulated by Ross Quinlan [75]. The algorithm has the capacity to segment data into multiple subgroups. The C5.0 possesses pruning ability, selecting splitting rules through an impurity measure [56]. The pros of the C5.0 algorithm include its robustness in handling missing data points and several input columns. In addition, the method requires shorter training sessions for estimates and uses normal enhancement techniques to improve the accuracy of the classification function.
This study sought to categorize response variables into two options (Low readiness and High readiness); then, a partition node was inserted to segregate the data into training (70%) and testing (30%) sets. The performance of models was assessed through the confusion matrix (Table 4). Next, the performance of models was analyzed using the attributes of accuracy, precision, recall, specificity, F-measure and area under the receiver operating characteristic (ROC) curve (AUC) and k-fold cross-validation.
Confusion Matrix of Readiness Low or High |
Predicted value | ||
Low readiness | High readiness | ||
Observed value | Low readiness | True Negative (TN) | False Positive (FP) |
High readiness | False Negative (FN) | True Positive (TP) |
In Table 4, true positive and true negative present the number of correct positive and correct negative samples predicted by the model. False positive and false negative stand for the number of wrong positive and wrong negative samples [76,77].
Accuracy is judging the overall correct rate, that is, that the actual category is consistent with the predicted category [76,77].
Accuracy=TP+TNTP+FP+FN+TN | (1) |
Precision is judging how much of the recall is true, that is, how much of the actual truth is accurately predicted to be true [76].
Precision=TPTP+FP | (2) |
Recall is the proportion of true positives to the total number of true positives and false negatives [76,77].
Recall=TPR=TPTP+FN | (3) |
1−Recall=FPR=FPFP+TN | (4) |
Specificity is the correct rate of judgment that is true, that is, the ratio of true to true among predictions [76].
Specificity=TNTN+FP | (5) |
F-measure: The harmonic mean of the precision and precision performance measurements is used to calculate the precision recovery curve. A high F-measurement result suggests that the categorization quality is excellent [76].
F−measure=2×PrecisionxRecallPrecision+Recall | (6) |
AUC: The ROC is a two-dimensional diagram of the false positive rate (FPR) on the horizontal axis versus the true positive rate (TPR) on the vertical axis. Based on Eqs (3) and (4), the TPR and FPR values of the cut-off points between 0 and 1 are calculated, and then the diagram is plotted by joining these data points. The area under the curve (AUC) is an appropriate measure if its value always varies between 0.5 and 1. The AUC is the standard to evaluate the model performance [78]. More specifically, the model performance is evaluated as acceptable (0.7 < = AUC < 0.8), good (0.8 < AUC < 0.9) or outstanding (AUC ≥ 0.9) discrimination [79].
k-fold cross-validation: In a comparative analysis of various forecast models, the total collection data is commonly divided into training and testing subsets, and thoroughly expecting models are analyzed based on their precision in the test data set. By dividing the information into designing and testing datasets, a decision of doing a single split or multiple splits can be made, which is regularly called k-fold cross-validation. To estimate the performances of classifiers, a stratified 10-fold cross-validation approach is used. Empirical studies showed that 10 folds seem to be an optimal number [80]. In this study, each fold of data included 24 cases (240 cases/10 = 24 cases) and was used once to test the performance of the classifier.
To be clearer, the research process of this study is shown in Figure 2.
Data were collected from 240 managers of Vietnamese SMEs. A total of sixteen input variables (eleven latent variables and five observed variables) were analyzed through the data mining technique. The performances of prediction models were evaluated through the four classification models. The best performance was revealed by the C5.0 model, predicting readiness to apply big data with more accuracy. Therefore, C5.0 was employed to predict the five observed variables' (firm size, sector, gender, age and education level) impacts on the readiness to apply BDA. Finally, the C5.0 procedure was illustrated as a decision tree.
As shown in Table 5, the correctness values of the predictions of CHAID, Bayesian networks, neural network and C5.0 for training data were 83.32, 82.93, 81.10 and 87.20%, respectively. The results of the correct predictions on the testing data were 68.42, 85.53, 71.05 and 89.47%, respectively. Hence, these models have high prediction accuracy.
Model type | Title | Training | Testing | AUC | |||
Training | Testing | ||||||
CHAID | Correct | 135 | 83.32% | 52 | 68.42% | 0.891 | 0.747 |
Wrong | 29 | 17.68% | 24 | 31.58% | |||
Total | 164 | 76 | |||||
Bayesian networks | Correct | 136 | 82.93% | 65 | 85.53% | 0.941 | 0.910 |
Wrong | 28 | 17.07% | 11 | 14.47% | |||
Total | 164 | 76 | |||||
Neural network | Correct | 133 | 81.10% | 54 | 71.05% | 0.861 | 0.815 |
Wrong | 31 | 18.90% | 22 | 28.95% | |||
Total | 164 | 76 | |||||
C5.0 | Correct | 143 | 87.20% | 68 | 89.47% | 0.893 | 0.939 |
Wrong | 21 | 12.80% | 8 | 10.53% | |||
Total | 164 | 76 |
Moreover, the training data showed an AUC value range of 0.861 to 0.941, while the test set ranged from 0.747 to 0.939. Hence, the models were considered good in discriminating the predictors [79]. The stream of four models is shown in Figure 3.
The ROC curve is also used for the evaluation of the classification algorithms. The ROC curve visualizes the false positive rate against the true positive rate. The false positive rate result will change according to the classification threshold value, and the best classification result model can be selected according to the area under the ROC curve. A larger area means the model has a better classification effect. In Figure 4, the results show that on training data, Bayesian networks are the best model, and for testing data, C5.0 is the best model.
The coincidence matrix and the evaluation results of the four models are shown in Table 6. It is clear that the resulting values in all four models for accuracy, precision, recall, specificity and F-measure were higher than 0.7, excepting accuracy, precision, recall, specificity and F-measure values on the testing data of the CHAID model and precision and specificity values on the testing data of the neural network model, which were approximately 0.7. This proves that the four models used in this study have good classification quality. Specifically, C5.0 is the model with the highest performance evaluation, followed by Bayesian networks, neural networks, and (the lowest) the CHAID model.
Model type | Partition | Title | Coincidence matrix | Accuracy | Precision | Recall | Specificity | F-measure | |
Low readiness |
High readiness |
||||||||
CHAID | Training | Low readiness | 69 | 9 | 0.8232 | 0.8800 | 0.7674 | 0.8846 | 0.8199 |
High readiness | 20 | 66 | |||||||
Total | 89 | 75 | |||||||
Testing | Low readiness | 28 | 13 | 0.6842 | 0.6486 | 0.6857 | 0.6829 | 0.6667 | |
High readiness | 11 | 24 | |||||||
Total | 39 | 37 | |||||||
Bayesian networks | Training | Low readiness | 70 | 8 | 0.8293 | 0.8919 | 0.7674 | 0.8974 | 0.8250 |
High readiness | 20 | 66 | |||||||
Total | 90 | 74 | |||||||
Testing | Low readiness | 37 | 4 | 0.8553 | 0.8750 | 0.8000 | 0.9024 | 0.8358 | |
High readiness | 7 | 28 | |||||||
Total | 44 | 32 | |||||||
Neural network | Training | Low readiness | 65 | 13 | 0.8110 | 0.8395 | 0.7907 | 0.8333 | 0.8144 |
High readiness | 18 | 68 | |||||||
Total | 83 | 81 | |||||||
Testing | Low readiness | 27 | 14 | 0.7105 | 0.6585 | 0.7714 | 0.6585 | 0.7105 | |
High readiness | 8 | 27 | |||||||
Total | 35 | 41 | |||||||
C5.0 | Training | Low readiness | 67 | 11 | 0.8720 | 0.8736 | 0.8837 | 0.8590 | 0.8786 |
High readiness | 10 | 76 | |||||||
Total | 77 | 87 | |||||||
Testing | Low readiness | 36 | 5 | 0.8947 | 0.8649 | 0.9143 | 0.8780 | 0.8889 | |
High readiness | 3 | 32 | |||||||
Total | 39 | 37 |
Similarly, the results of the 10-fold cross-validation for the four models are shown in Table A2, which indicated that C5.0 is the model with the highest average accuracy among the four selected tested models. Accordingly, the accuracy average for the 10-fold cross-validation of the C5.0 model is 0.885, followed by Bayesian networks (0.833) and neural networks (0.772), making the CHAID model (0.679) the lowest. This can be explained because C5.0 has higher memory performance than other algorithms and then can generate more precise rules. CHAID, an algorithm, applies the chi-square independence test that is suitable for categorical data. However, the input variables of this study are mostly continuous variables. To improve the precision of the model, this algorithm must perform by grouping data into categories. That is the reason why CHAID performs with the least precise predictions.
Predictor importance is a sensitivity analysis technique. It is used to identify the more important variables and/or omit the least important variables in the forecasting model [76].
The important drivers of readiness to adopt BDA are presented in Table 7. In CHAID and Bayesian networks, the most important variable was the management support variable. Conversely, in the neural network, the cost variable was the most critical. The most critical variable in the C5.0 model was data quality. The predictors of four algorithms rank the predictors from most important to least essential based on the total value (total relative importance value for each attribute).
Variable | Technique | Total value | |||
CHAID | Bayesian networks | Neural network | C5.0 | ||
Management support | 0.3566 | 0.3627 | 0.1179 | 0.2029 | 1.0401 |
Data quality | 0.2079 | 0.2007 | 0.0973 | 0.2073 | 0.7132 |
Firm size | 0.1485 | 0.0675 | 0.0000 | 0.1099 | 0.3259 |
Data security | 0.0954 | 0.0455 | 0.1398 | 0.0000 | 0.2807 |
Cost | 0.0000 | 0.0000 | 0.1613 | 0.0779 | 0.2392 |
Sector | 0.0759 | 0.0293 | 0.0516 | 0.0685 | 0.2253 |
Competitive pressure | 0.0028 | 0.0936 | 0.0610 | 0.0297 | 0.1871 |
Partner pressure | 0.0000 | 0.0728 | 0.0775 | 0.0000 | 0.1503 |
Gender | 0.0000 | 0.0000 | 0.0410 | 0.0958 | 0.1368 |
Government support | 0.0254 | 0.0000 | 0.0720 | 0.0000 | 0.0974 |
Technical competence | 0.0000 | 0.0507 | 0.0443 | 0.0000 | 0.0950 |
IT infrastructure | 0.0000 | 0.0000 | 0.0000 | 0.0747 | 0.0747 |
Age | 0.0028 | 0.0000 | 0.0000 | 0.0541 | 0.0569 |
Decision-making culture | 0.0000 | 0.0349 | 0.0000 | 0.0000 | 0.0349 |
Education level | 0.0343 | 0.0000 | 0.0000 | 0.0000 | 0.0343 |
Relative advantage | 0.0000 | 0.0288 | 0.0000 | 0.0000 | 0.0288 |
To get an overview of the gauge result of the four models, we consolidated the values of the four models. The mix of these prescient models is known as aggregation-based sensitivity examination and is suggested in light of the fact that it produces hearty, exact models [76,81]. As a result, the sixteen input variables were categorized into four dimensions—technology dimension (relative advantage, IT infrastructure, data quality, data security, technical competence), organization dimension (top management support, cost, sector, firm size, decision making culture), environment dimension (competitive pressure, partner pressure, government support) and manager's characteristics dimension (gender, age, education level)—that have an impact on the readiness of BDA adoption. The major predictor variables for BDA adoption among Vietnam SMEs were identified to be management support, data quality, firm size, data security and cost.
Based on the results of the evaluation of the four forecasting models, the C5.0 is the model with the highest predictive accuracy. Therefore, the authors used the C5.0 model to evaluate in detail the observed variables affecting the readiness to use BDA in SMEs. The output variable was the readiness to apply BDA among SMEs (Low readiness and High readiness), and input variables were firm size, sector, gender, age and education level. The stream of the C5.0 model is presented in Figure 5.
The process of the C5.0 model consists of five input observed variables. This model used the whole dataset, with the result of a correct prediction percentage of 73.75% and an AUC value of 0.758. This proves that the model has high-performance measurements. The results of the model represented three descriptors splitting nodes (firm size, sector and age).
Figure 6 illustrates the results of the decision tree of the C5.0 model. The first splitting node of readiness to apply BDA in SMEs was firm size. In node 1, the proportion of small companies that are not ready to adopt BDA is 57.58%, while the number of small companies with high readiness is lower (42.42%). Next, node 1 diverged into nodes 2 and 3. In node 2, 69.83% of manufacturing companies were still not ready to adopt BDA, and only 30.17% of companies had high readiness. In node 3, the rate of the services companies' readiness to apply BDA is high, 59.76%, and the figure for low willingness companies was 40.24%. Next, node 3 diverged into nodes 4 and 5. In node 4, 70.97% of service companies with leaders under 46 have a high level of readiness to adopt BDA, whereas only 29.03% of service companies have low readiness. Otherwise, in node 5, with leaders aged 46 and over, the percentage of companies willing to adopt BDA (25.00%) was lower than the percentage of companies that were not ready to adopt BDA (75.00%). Finally, in node 6, the majority of medium companies have a high willingness to adopt BDA (88.09%), whereas only 11.91% of medium enterprises have low readiness.
The findings of the current study demonstrated that sixteen factors of four dimensions (technology, organization, environment and manager's characteristics) have impacts on the readiness to adopt BDA. Furthermore, management support, data quality, firm size, data security and cost were revealed as major predictors of the readiness to apply BDA among Vietnamese SMEs. In addition, medium-sized companies in the service sector are assessed to have higher readiness to apply BDA than other SMEs. In addition, the results of the C5.0 model indicated that firm size, sector and age do have an impact on the BDA adoption readiness.
The results of the study show that management support is the strongest decisive factor in the readiness to apply BDA among Vietnamese SMEs. The result is similar to findings from previous studies such as Sun et al. [35], Maroufkhani et al. [9], Lai et al. [41], Asiaei and Rahim [82]. The support of managers will create favorable conditions for the company in maintaining and using technology [82]. Realizing the benefits of big data, management can allocate the resources needed for adoption and implementation. By contrast, if the management does not see the benefits of big data for businesses, they will oppose its adoption [47].
Generally, data is supposed to be an important input when companies adopt BDA. To perform a successful BDA, data quality is extremely important. Firms have abundant data sources and have high accuracy that will contribute to applying big data readiness. In this study, data quality is a strong factor of BDA adoption, which is consistent with the findings of Park and Kim [23].
Not surprisingly, firm size affected the readiness of BDA adoption. This is consistent with the results of Sohaib et al. [83] and Alshamaila et al. [84]. To be more specific, medium enterprises have higher readiness to adopt BDA than small enterprises. This can be explained by medium-sized companies having larger revenue and more employees than small companies. Therefore, they have many advantages when investing in BDA applications.
Data security was also predicted as a strong influencing factor in this study. Big data includes a lot of personal information [14]; hence, it is of serious concern among firms when deciding to adopt BDA. The influence of data security in technology adoption was also found in many previous studies, such as in software-as-a-service adoption [85], cloud computing [51,83] and big data adoption [23,35,37].
Cost is one of the five factors that are predicted to have an important influence on the readiness of SMEs to adopt BDA. This finding is similar to Park and Kim [23] and Sun et al. [35], who found that cost is an important factor in maintaining and developing the analysis of big data in enterprises. In addition, costs for big data adoption can be a barrier for companies to implementing big data [17,86].
The classification results of the C5.0 model with five observed variables show that the service sector has a higher readiness to apply BDA than the manufacturing sector. This result is consistent with Gangwar [48], who identified factors influencing big data adoption in Indian companies. This is because service organizations like wholesalers, retailers and lodging providers have early access to information technology systems and high-quality human resources to analyze large amounts of data. Moreover, in the context of the complicated development of the COVID-19 pandemic, wholesale and retail companies in Vietnam have had a rapid shift from traditional shopping to online shopping. As a result, organizations must develop suggestion systems and find ways to respond to client information as quickly as possible. Hence, service SMEs are better prepared to adopt BDA. Manufacturing companies are stated to be encountering numerous obstacles, such as a lack of infrastructure and BDA tools, when it comes to using BDA to optimize supply chains [86].
The findings show that small service firms with managers under the age of 46 have a higher readiness to adopt BDA than those firms with older managers. This can be explained by young managers being bolder in adopting new technology, while older managers consider more carefully the necessary conditions when applying BDA, such as information technology, high-quality human resources and finance. In addition, in the implementation of new technologies, some of the older leaders have a lagging mindset, fear of risk and fear of change. This is consistent with the findings of Badri et al. [87], who mentioned that elderly teachers are thought to show less technology readiness than younger teachers.
Applying BDA plays an important role in helping organizations improve competitiveness, enhance supply chains, optimize logistics and improve business performance. Based on the data mining technique, the findings of the study show that the C5.0 model is the best model to predict factors affecting BDA adoption readiness in SMEs. Five factors have the greatest influence on the readiness to adopt BDA: management support, data quality, firm size, data security and cost. Moreover, an important finding of this study is that the age of managers also affects the readiness to adopt BDA.
This study is useful to managers of SMEs, providers and policymakers in developing better policies and strategies for the adoption of BDA. In terms of managers, the volume of data generated in organizations is growing exponentially. So, how to effectively analyze big data is a matter of great interest to organizations today. The proposed model can assist businesses in determining their readiness to adopt BDA. Furthermore, the findings of the study assist managers in increasing their awareness of the elements affecting the enterprise's readiness to use big data. For example, this research shows that management support is the most important factor influencing BDA adoption readiness. As a result, before deciding to embrace BDA, SME management should be proactive in studying to increase their knowledge of the technology and developing a clear strategy. In terms of service providers, the outcomes of this study reveal that SMEs should prioritize data quality, data security and cost factors when preparing to embrace BDA. SMEs, on the other hand, are having financial challenges. As a result, plans for developing BDA tools, hardware, software and other products that meet the needs of providers' clients in emerging and underdeveloped countries should be formed. In addition, when implementing BDA, suppliers must improve services to support SMEs. In terms of policymakers, the survey revealed that the service sector is more prepared to use big data than the manufacturing sector and that medium-sized businesses are more prepared to use big data than small businesses. As a result, the government should have policies in place to assist each sort of business.
Thanks to the great benefits that BDA contributes to business development, a huge number of businesses are interested in BDA. This study has made significant contributions that help practitioners and researchers understand the importance of influencing factors on the readiness to apply big data in SMEs. First, instead of using traditional analytical methods to perform information-based sensitivity analysis, as shown in previous studies, well-known data mining algorithms were used to develop predictive models in this study. Second, this study explored factors that have strong impacts on the readiness to adopt BDA among SMEs. From these findings, the research model is expected to be a useful reference for practitioners in developing countries and the scientific community for doing future related research.
In addition to the study findings, this study also demonstrates some limitations. First is the limitation on the number of samples when using the data mining technique. Therefore, future studies should be conducted with larger sample sizes. Second, the numbers of input variables and prediction algorithms are limited. In future investigations, the number of input variables should increase, and different forecasting algorithms may be used to evaluate the predictive model's findings.
The authors would like to thank all respondents who spent valuable time answering questionnaires and the insightful comments of the reviewers.
The authors declare there is no conflict of interest.
Variable | Item number | Factor loadings | Cronbach α | CR | AVE |
Relative advantage | 4 | 0.530–0.865 | 0.807 | 0.805 | 0.519 |
IT structure | 3 | 0.617–0.848 | 0.798 | 0.809 | 0.590 |
Data quality | 3 | 0.569–0.728 | 0.691 | 0.714 | 0.457 |
Data security | 3 | 0.705–0.756 | 0.716 | 0.764 | 0.520 |
Technical competence | 4 | 0.744–0.816 | 0.867 | 0.867 | 0.620 |
Management support | 3 | 0.520–0.781 | 0.707 | 0.722 | 0.471 |
Cost | 3 | 0.755–0.849 | 0.798 | 0.843 | 0.643 |
Decision-making culture | 3 | 0.615–0.846 | 0.746 | 0.768 | 0.528 |
Competitive pressure | 3 | 0.787–0.842 | 0.856 | 0.856 | 0.664 |
Partner pressure | 3 | 0.690–0.717 | 0.626 | 0.751 | 0.501 |
Government support | 3 | 0.703–0.727 | 0.719 | 0.757 | 0.509 |
Readiness to adopt big data | 9 | 0.684–0.810 | 0.773 | 0.909 | 0.526 |
*Note: CR: Composite Reliability, AVE: Average Variance Extracted |
Fold No. | CHAID | Bayesian networks | Neural network | C5.0 | ||||||||
Confusion matrix | Accuracy | Confusion matrix | Accuracy | Confusion matrix | Accuracy | Confusion matrix | Accuracy | |||||
1 | 12 | 5 | 0.649 | 13 | 4 | 0.784 | 12 | 5 | 0.703 | 14 | 3 | 0.892 |
11 | 9 | 4 | 16 | 6 | 14 | 1 | 19 | |||||
2 | 21 | 11 | 0.729 | 28 | 4 | 0.847 | 26 | 6 | 0.780 | 27 | 5 | 0.881 |
5 | 22 | 5 | 22 | 7 | 20 | 2 | 25 | |||||
3 | 28 | 13 | 0.684 | 37 | 4 | 0.855 | 27 | 14 | 0.711 | 36 | 5 | 0.895 |
11 | 24 | 7 | 28 | 8 | 27 | 3 | 32 | |||||
4 | 32 | 21 | 0.615 | 49 | 4 | 0.846 | 36 | 17 | 0.692 | 45 | 8 | 0.875 |
25 | 26 | 12 | 39 | 15 | 36 | 5 | 46 | |||||
5 | 53 | 10 | 0.736 | 58 | 5 | 0.840 | 46 | 17 | 0.720 | 55 | 8 | 0.888 |
23 | 39 | 15 | 47 | 18 | 44 | 6 | 56 | |||||
6 | 46 | 25 | 0.697 | 64 | 7 | 0.828 | 51 | 20 | 0.752 | 62 | 9 | 0.890 |
19 | 55 | 18 | 56 | 16 | 58 | 7 | 67 | |||||
7 | 66 | 13 | 0.669 | 71 | 20 | 0.828 | 65 | 14 | 0.761 | 69 | 9 | 0.883 |
41 | 43 | 8 | 64 | 25 | 59 | 10 | 75 | |||||
8 | 84 | 12 | 0.728 | 87 | 23 | 0.836 | 62 | 34 | 0.692 | 83 | 13 | 0.887 |
41 | 58 | 9 | 76 | 26 | 73 | 9 | 90 | |||||
9 | 93 | 14 | 0.657 | 96 | 11 | 0.833 | 86 | 21 | 0.681 | 93 | 14 | 0.884 |
60 | 49 | 25 | 84 | 48 | 61 | 11 | 98 | |||||
10 | 62 | 55 | 0.627 | 105 | 12 | 0.835 | 44 | 18 | 0.732 | 101 | 16 | 0.877 |
33 | 86 | 27 | 92 | 22 | 65 | 13 | 106 | |||||
Average | 0.679 | 0.833 | 0.722 | 0.885 | ||||||||
Confusion matrix illustrates the classification of the cases in the test dataset. In the confusion matrix, the columns represent the actual cases, and the rows represent the predicted. Accuracy = (TP + TN)/(TP + FP + TN + FN). |
[1] |
V. Khodadadi, F. N. Rahatabad, A. Sheikhani, N. J. Dabanloo, Nonlinear analysis of biceps surface EMG signals for chaotic approaches, Chaos Soliton Fract., 166 (2023), 112965. https://doi.org/10.1016/j.chaos.2022.112965 doi: 10.1016/j.chaos.2022.112965
![]() |
[2] |
X. Zhang, P. Zhou, Sample entropy analysis of surface EMG for improved muscle activity onset detection against spurious background spikes, J. Electromyogr. Kinesiology, 22 (2012), 901–907. https://doi.org/10.1016/j.jelekin.2012.06.005 doi: 10.1016/j.jelekin.2012.06.005
![]() |
[3] |
Z. Brari, S. Belghith, A new algorithm for largest Lyapunov exponent determination for noisy chaotic signal studies with application to Electroencephalographic signals analysis for epilepsy and epileptic seizures detection, Chaos Soliton Fract., 165 (2022), 112757. https://doi.org/10.1016/j.chaos.2022.112757 doi: 10.1016/j.chaos.2022.112757
![]() |
[4] |
S. B. He, K. H. Sun, R. X. Wang, Fractional fuzzy entropy algorithm and the complexity analysis for nonlinear time series, Eur. Phys. J. Special Topics, 227 (2018), 943–957. https://doi.org/10.1140/epjst/e2018-700098-x doi: 10.1140/epjst/e2018-700098-x
![]() |
[5] |
K. Harezlak, P. Kasprowski, Application of time-scale decomposition of entropy for eye movement analysis, Entropy, 22 (2020), 68. https://doi.org/10.3390/e22020168 doi: 10.3390/e22020168
![]() |
[6] |
S. Jia, B. Ma, W. Guo, Z. S. Li, A sample entropy based prognostics method for lithiumion batteries using relevance vector machine, J. Manuf. Sys., 61 (2021), 773–781. https://doi.org/10.1016/j.jmsy.2021.03.019 doi: 10.1016/j.jmsy.2021.03.019
![]() |
[7] |
J. Richman, J. Moorman, Physiological time-series analysis using approximate entropy and sample entropy, Am. J. Physiol. Heart Circ. Physiol., 278 (2000), H2039–H2049. https://doi.org/10.1152/ajpheart.2000.278.6.H2039 doi: 10.1152/ajpheart.2000.278.6.H2039
![]() |
[8] |
W. T. Chen, Z. Z. Wang, H. B. Xie, W. X. Yu, Characterization of surface EMG signal based on fuzzy entropy, IEEE Trans. Neural Syst. Rehabil. Eng., 15 (2007), 266–272. https://doi.org/10.1109/TNSRE.2007.897025 doi: 10.1109/TNSRE.2007.897025
![]() |
[9] |
M. Rostaghi, H. Azami, Dispersion Entropy: A measure for time-series analysis, IEEE Signal Proc. Let., 23 (2016), 610–614. https://doi.org/10.1109/LSP.2016.2542881 doi: 10.1109/LSP.2016.2542881
![]() |
[10] |
S. B. Jiao, B. Geng, Y. X. Li, Q. Zhang, Q. Wang, Fluctuation-based reverse dispersion entropy and its applications to signal classification, Appl. Acoust., 175 (2021), 107857. https://doi.org/10.1016/j.apacoust.2020.107857 doi: 10.1016/j.apacoust.2020.107857
![]() |
[11] |
H. Azami, M. Rostaghi, D. Abásolo, J. Escudero, Refined composite multiscale dispersion entropy and its application to biomedical signals, IEEE Trans. Bio-med. Eng., 64 (2017), 2872–2879. https://doi.org/10.1109/TBME.2017.2679136 doi: 10.1109/TBME.2017.2679136
![]() |
[12] |
S. Sharma, S. K. Tiwari, A novel feature extraction method based on weighted multi-scale fluctuation based dispersion entropy and its application to the condition monitoring of rotary machines, Mech. Syst. Signal Pr., 171 (2022), 108909. https://doi.org/10.1016/j.ymssp.2022.108909 doi: 10.1016/j.ymssp.2022.108909
![]() |
[13] |
C. J. Li, Y. C. Wu, H. J. Lin, J. M. Li, F. Zhang, Y. X. Yang, ECG denoising method based on an improved VMD algorithm, IEEE Sens J., 22 (2022), 22725–22733. https://doi.org/10.1109/JSEN.2022.3214239 doi: 10.1109/JSEN.2022.3214239
![]() |
[14] |
B. García-Martínez, A. Fernández-Caballero, R. Alcaraz, A. Martínez-Rodrigo, Application of dispersion entropy for the detection of emotions with electroencephalographic signals, IEEE T. Cogn. Dev. Syst., 14 (2022), 1179–1187. https://doi.org/10.1109/TCDS.2021.3099344 doi: 10.1109/TCDS.2021.3099344
![]() |
[15] |
E. Kafantaris, T. Y. M. Lo, J. Escudero, Stratified multivariate multiscale dispersion entropy for physiological signal analysis, IEEE Trans. Bio-med Eng., 70 (2023), 1024–1035. https://doi.org/10.1109/TBME.2022.3207582 doi: 10.1109/TBME.2022.3207582
![]() |
[16] |
Q. F. Wang, Y. Xiao, S. Wang, W. C. Liu, X. J. Liu, A method for constructing automatic rolling bearing fault identification model based on refined composite multi-scale dispersion entropy, IEEE Access, 9 (2021), 86412–86428. https://doi.org/10.1109/ACCESS.2021.3089251 doi: 10.1109/ACCESS.2021.3089251
![]() |
[17] |
M. Rostaghi, M. M. Khatibi, M. R. Ashory, H. Azami, Fuzzy dispersion entropy: A nonlinear measure for signal analysis, IEEE Trans. Fuzzy Syst., 30 (2021), 3785–3796. https://doi.org/10.1109/TFUZZ.2021.3128957 doi: 10.1109/TFUZZ.2021.3128957
![]() |
[18] |
Y. X. Li, B. Geng, B. Z. Tang, Simplified coded dispersion entropy: A nonlinear metric for signal analysis, Nonlinear Dyn., 111 (2023), 9327–9344. https://doi.org/10.1007/s11071-023-08339-4 doi: 10.1007/s11071-023-08339-4
![]() |
[19] |
J. P. Ugarte, J. A. Tenreiro Machado, C. Tobón, Fractional generalization of entropy improves the characterization of rotors in simulated atrial fibrillation, Appl. Math. Comput., 425 (2022), 127077. https://doi.org/10.1016/j.amc.2022.127077 doi: 10.1016/j.amc.2022.127077
![]() |
[20] |
A. D. Crescenzo, S. Kayal, A. Meoli, Fractional generalized cumulative entropy and its dynamic version, Commun. Nonlinear Sci., 102 (2021), 105899. https://doi.org/10.1016/j.cnsns.2021.105899 doi: 10.1016/j.cnsns.2021.105899
![]() |
[21] |
Y. Wang, P. J. Shang, Complexity analysis of time series based on generalized fractional order cumulative residual distribution entropy, Phys. A, 537 (2020), 122582. https://doi.org/10.1016/j.physa.2019.122582 doi: 10.1016/j.physa.2019.122582
![]() |
[22] |
J. T. Machado, Fractional order generalized information, Entropy, 16 (2014), 2350–2361. https://doi.org/10.3390/e16042350 doi: 10.3390/e16042350
![]() |
[23] |
S. R. Wang, H. Tang, B. Wang, J. Mo, Analysis of fatigue in the biceps brachii by using rapid refined composite multiscale sample entropy, Biomed. Signal Process., 67 (2021), 102510. https://doi.org/10.1016/j.bspc.2021.102510 doi: 10.1016/j.bspc.2021.102510
![]() |
[24] |
I. Yun, J. Jeung, Y. Song, Y. Chung, Non-Invasive quantitative muscle fatigue estimation based on correlation between sEMG signal and muscle mass, IEEE Access, 8 (2020), 191751–191757. https://doi.org/10.1109/ACCESS.2020.3029792 doi: 10.1109/ACCESS.2020.3029792
![]() |
[25] |
D. R. Rogers, D. T. MacIsaac, EMG-based muscle fatigue assessment during dynamic contractions using principal component analysis, J. Electromyogr. Kinesiology, 21 (2011), 811–818. https://doi.org/10.1016/j.jelekin.2011.05.002 doi: 10.1016/j.jelekin.2011.05.002
![]() |
[26] |
J. R. Mota-Carmona, F. Pérez-Escamirosa, A. Minor-Martínez, R. M. Rodríguez-Reyna, Muscle fatigue detection in upper limbs during the use of the computer mouse using discrete wavelet transform: A pilot study, Biomed. Signal Process., 76 (2022), 103711. https://doi.org/10.1016/j.bspc.2022.103711 doi: 10.1016/j.bspc.2022.103711
![]() |
[27] |
B. K. Barry, R. M. Enoka, The neurobiology of muscle fatigue: 15 years later, Integr. Comput. Biol., 47 (2007), 465–473. https://doi.org/10.1093/icb/icm047 doi: 10.1093/icb/icm047
![]() |
[28] |
W. K. Xu, B. Chu, E. Rogers, Iterative learning control for robotic-assisted upper limb stroke rehabilitation in the presence of muscle fatigue, Control Eng. Pract., 31 (2014), 63–72. https://doi.org/10.1016/j.conengprac.2014.05.009 doi: 10.1016/j.conengprac.2014.05.009
![]() |
[29] |
F. F. Wang, E. M. Yiu, Is surface Electromyography (sEMG) a useful tool in identifying muscle tension dysphonia? An integrative review of the current evidence, J. Voice, 10 (2021). https://doi.org/10.1016/j.jvoice.2021.10.006 doi: 10.1016/j.jvoice.2021.10.006
![]() |
[30] |
J. Hussain, K. Sundaraj, F. L. Yin, L. C. Kiang, S. Sundaraj, M. A. Ali, A systematic review on fatigue analysis in triceps brachii using surface electromyography, Biomed. Signal Process., 40 (2018), 396–414. https://doi.org/10.1016/j.bspc.2017.10.008 doi: 10.1016/j.bspc.2017.10.008
![]() |
[31] |
S. E. Jero, K. D. Bharathi, P. A. Karthick, S. Ramakrishnan, Muscle fatigue analysis in isometric contractions using geometric features of surface electromyography signals, Biomed. Signal Process., 68 (2021), 102603. https://doi.org/10.1016/j.bspc.2021.102603 doi: 10.1016/j.bspc.2021.102603
![]() |
[32] | G. Y. Zhang, E. Morin, Y. X. Zhang, S. A. Etemad, Non-invasive detection of low-level muscle fatigue using surface EMG with wavelet decomposition, in 40th Annual International Conference of the IEEE Engineering in Medicine and Biology Society (EMBC), (2018), 5648–5651. https://doi.org/10.1109/EMBC.2018.8513588 |
[33] |
M. Cifrek, V. Medved, S. Tonković, S. Ostojić, Surface EMG based muscle fatigue evaluation in biomechanics, J Clin. Biomech., 24 (2009), 327–340. https://doi.org/10.1016/j.clinbiomech.2009.01.010 doi: 10.1016/j.clinbiomech.2009.01.010
![]() |
[34] |
W. W. Hu, Y. C. Huang, C. P. Li, Improved algorithm of muscle fatigue detection using linear regression analysis, Electron. Lett., 49 (2013), 89–91. https://doi.org/10.1049/el.2012.2316 doi: 10.1049/el.2012.2316
![]() |
[35] |
H. B. Xie, Z. Z. Wang, Mean frequency derived via Hilbert-Huang transform with application to fatigue EMG signal analysis, Comput. Methods Prog. Biomed., 82 (2006), 114–120. https://doi.org/10.1016/j.cmpb.2006.02.009 doi: 10.1016/j.cmpb.2006.02.009
![]() |
[36] |
H. B. Xie, J. Y. Guo, Y. P. Zheng, Fuzzy approximate entropy analysis of chaotic and natural complex systems: detecting muscle fatigue using electromyography signals, Ann. Biomed. Eng., 38 (2010), 1483–1496. https://doi.org/10.1007/s10439-010-9933-5 doi: 10.1007/s10439-010-9933-5
![]() |
[37] |
A. Shoeibi, M. Khodatars, M. Jafari, N. Ghassemi, P. Moridian, R. Alizadehsani, et al., Diagnosis of brain diseases in fusion of neuroimaging modalities using deep learning: A review, Inf. Fusion, 93 (2023), 85–117. https://doi.org/10.1016/j.inffus.2022.12.010 doi: 10.1016/j.inffus.2022.12.010
![]() |
[38] |
J. Murillo-Escobar, Y. E. Jaramillo-Munera, D. A. Orrego-Metaute, E. Delgado-Trejos, D. Cuesta-Frau, Muscle fatigue analysis during dynamic contractions based on biomechanical features and Permutation Entropy, Math. Biosci. Eng., 17 (2020), 2592–2615. https://doi.org/10.3934/mbe.2020142 doi: 10.3934/mbe.2020142
![]() |
[39] |
S. E. Jero, K. D. Bharathi, P. A. Karthick, S. Ramakrishnan, Muscle fatigue analysis in isometric contractions using geometric features of surface electromyography signals, Biomed. Signal Process., 68 (2021), 102603. https://doi.org/10.1016/j.bspc.2021.102603 doi: 10.1016/j.bspc.2021.102603
![]() |
[40] |
P. A. Karthick, D. M. Ghosh, S. Ramakrishnan, Surface electromyography based muscle fatigue detection using high-resolution time-frequency methods and machine learning algorithms, Comput. Methods Prog. Biomed., 154 (2018), 45–56. https://doi.org/10.1016/j.cmpb.2017.10.024 doi: 10.1016/j.cmpb.2017.10.024
![]() |
[41] |
J. H. Wang, Y. N. Sun, S. M. Sun. Recognition of muscle fatigue status based on improved wavelet threshold and CNN-SVM, IEEE Access, 8 (2020), 207914–207922. https://doi.org/10.1109/ACCESS.2020.3038422 doi: 10.1109/ACCESS.2020.3038422
![]() |
[42] |
J. H. Wang, S. M. Sun, Y. N. Sun, A muscle fatigue classification model based on LSTM and improved wavelet packet threshold, Sensors, 21 (2021), 6369. https://doi.org/10.3390/s21196369 doi: 10.3390/s21196369
![]() |
[43] | A. Shoeibi, M. Rezaei, N. Ghassemi, Z. Namadchian, A. Zare, J. M. Gorriz, Automatic diagnosis of schizophrenia in EEG signals using functional connectivity features and CNN-LSTM model, in Proceedings of the International Work-Conference on the Interplay Between Natural and Artificial Computation (IWINAC), (2022), 63–73. https://doi.org/10.1007/978-3-031-06242-1_7 |
[44] |
M. Jafari, D. Sadeghi, A. Shoeibi, H. Alinejad-Rokny, A. Beheshti, D. López-García, et al., Empowering precision medicine: AI-Driven schizophrenia diagnosis via EEG signals: A comprehensive review from 2002–2023, Appl. Intell., 2023 (2023), 1–45. https://doi.org/10.1007/s10489-023-05155-6 doi: 10.1007/s10489-023-05155-6
![]() |
[45] |
P. Chawla, S. B. Rana, H. Kaur, K. Singh, R. Yuvaraj, M. Murugappan, A decision support system for automated diagnosis of Parkinson's disease from EEG using FAWT and entropy features, Biomed. Signal Process., 79 (2023), 104116. https://doi.org/10.1016/j.bspc.2022.104116 doi: 10.1016/j.bspc.2022.104116
![]() |
[46] |
N. Makaram, P. A. Karthick, R. Swaminathan, Analysis of dynamics of EMG signal variations in fatiguing contractions of muscles using transition network approach, IEEE Trans. Instrum. Meas., 70 (2021), 4003608. https://doi.org/10.1109/TIM.2021.3063777 doi: 10.1109/TIM.2021.3063777
![]() |
[47] |
C. Tepe, M. C. Demir, Real-time classification of EMG Myo armband data using support vector machine, IRBM, 43 (2022), 300–308. https://doi.org/10.1016/j.irbm.2022.06.001 doi: 10.1016/j.irbm.2022.06.001
![]() |
[48] |
X. J. Wang, D. P. Dong, X. K. Chi, S. P. Wang, Y. N. Miao, M. L. An, et al., sEMG-based consecutive estimation of human lower limb movement by using multi-branch neural network, Biomed. Signal Process., 68 (2021), 102781. https://doi.org/10.1016/j.bspc.2021.102781 doi: 10.1016/j.bspc.2021.102781
![]() |
[49] |
J. R. Potvin, L. R. Bent, A validation of techniques using surface EMG signals from dynamic contractions to quantify muscle fatigue during repetitive tasks, J. Electromyogr. Kinesiology, 7 (1997), 131–139. https://doi.org/10.1016/S1050-6411(96)00025-9 doi: 10.1016/S1050-6411(96)00025-9
![]() |
[50] |
K. Dragomiretskiy, D. Zosso, Variational mode decomposition, IEEE Trans. Signal Process., 62 (2014), 531–544. https://doi.org/10.1109/TSP.2013.2288675 doi: 10.1109/TSP.2013.2288675
![]() |
[51] |
H. Ashraf, U. Shafiq, Q. Sajjad, A. Waris, O. Gilani, M. Boutaayamou, et al., Variational mode decomposition for surface and intramuscular EMG signal denoising, Biomed. Signal Process., 82 (2023), 104560. https://doi.org/10.1016/j.bspc.2022.104560 doi: 10.1016/j.bspc.2022.104560
![]() |
[52] |
S. H. Ma, B. Lv, C. Lin, X. J. Sheng, X. Y. Zhu, EMG signal filtering based on variational mode decomposition and sub-band thresholding, IEEE J. Biomed. Health., 25 (2021), 47–58. https://doi.org/10.1109/JBHI.2020.2987528 doi: 10.1109/JBHI.2020.2987528
![]() |
[53] |
D. L. Donoho, De-noising by soft-thresholding, IEEE Trans. Inf. Theory, 41 (1995), 613–627. https://doi.org/10.1109/18.382009 doi: 10.1109/18.382009
![]() |
[54] |
H. Ashraf, A. Waris, S. O. Gilani, M. U. Tariq, H. Alquhayz, Threshold parameters selection for empirical mode decomposition-based EMG signal denoising, Intell. Autom. Soft Comput., 27 (2021), 799–815. https://doi.org/10.32604/iasc.2021.014765 doi: 10.32604/iasc.2021.014765
![]() |
[55] |
S. Phatak, S. S. Rao, Logistic map: A possible random-number generator, Phys. Rev. E, 51 (1995), 3670. https://doi.org/10.1103/PhysRevE.51.3670 doi: 10.1103/PhysRevE.51.3670
![]() |
[56] |
L. Kahl, U. G. Hofmann, Comparison of algorithms to quantify muscle fatigue in upper limb muscles based on sEMG signals, Med. Eng. Phys., 38 (2016), 1260–1269. https://doi.org/10.1016/j.medengphy.2016.09.009 doi: 10.1016/j.medengphy.2016.09.009
![]() |
[57] |
Y. X. Li, B. Z. Tang, B. Geng, S. B. Jiao, Fractional order fuzzy dispersion entropy and its application in bearing fault diagnosis, Fractal Fract., 6 (2022), 544. https://doi.org/10.3390/fractalfract6100544 doi: 10.3390/fractalfract6100544
![]() |
[58] |
E. Z. Song, Y. Ke, C. Yao, Q. Dong, L. P. Yang, Fault diagnosis method for high-pressure common rail injector based on IFOA-VMD and hierarchical dispersion entropy, Entropy, 21 (2019), 923. https://doi.org/10.3390/e21100923 doi: 10.3390/e21100923
![]() |
[59] |
X. A. Yan, Y. D. Xu, M. P. Jia, Intelligent fault diagnosis of rolling-element bearings using a self-adaptive hierarchical multiscale fuzzy entropy, Entropy, 23 (2021), 1128. https://doi.org/10.3390/e23091128 doi: 10.3390/e23091128
![]() |
[60] |
R. J. Zhou, X. Wang, J. Wan, N. X. Xiong, EDM-Fuzzy: An Euclidean distance based multiscale fuzzy entropy technology for diagnosing faults of industrial systems, IEEE Trans. Ind. Inf., 17 (2021), 4046–4054. https://doi.org/10.1109/TII.2020.3009139 doi: 10.1109/TII.2020.3009139
![]() |
[61] |
A. Shoeibi, N. Ghassemi, M. Khodatars, P. Moridian, A. Khosravi, A. Zare, et al., Automatic diagnosis of schizophrenia and attention deficit hyperactivity disorder in RS-fMRI modality using convolutional autoencoder model and interval type-2 fuzzy regression, Cognit. Neurodyn., 17 (2023), 1501–1523. https://doi.org/10.1007/s11571-022-09897-w doi: 10.1007/s11571-022-09897-w
![]() |
[62] |
H. M. Qassim, W. Z. W. Hasan, H. R. Ramli, H. H. Harith, L. N. I. Mat, L. I. Ismail, Proposed fatigue index for the objective detection of muscle fatigue using surface electromyography and a double-step binary classifier, Sensors, 22 (2022), 1900. https://doi.org/10.3390/s22051900 doi: 10.3390/s22051900
![]() |
[63] |
K. D. Bharathi, P. A. Karthick, S. Ramakrishnan, Automated detection of muscle fatigue conditions from cyclostationary based geometric features of surface electromyography signals, Comput. Methods Biomech. Biomed. Eng., 25 (2022), 320–332. https://doi.org/10.1080/10255842.2021.1955104 doi: 10.1080/10255842.2021.1955104
![]() |
[64] |
N. Makaram, P. A. Karthick, V. Gopinath, R. Swaminathan, Surface Electromyography-based muscle fatigue analysis using binary and weighted visibility graph features, Fluctuation Noise Lett., 20 (2021), 2150016. https://doi.org/10.1142/S0219477521500164 doi: 10.1142/S0219477521500164
![]() |
[65] |
D. B. Krishnamani, P. A. Karthick, R. Swaminathan, Variational mode decomposition based differentiation of fatigue conditions in muscles using surface electromyography signals, IET Signal Process., 14 (2021), 745–753. https://doi.org/10.1049/iet-spr.2020.0315 doi: 10.1049/iet-spr.2020.0315
![]() |
[66] |
D. Sasidharan, V. Gopinath, R. Swaminathan, A proposal to analyze muscle dynamics under fatiguing contractions using surface Electromyography signals and fuzzy recurrence network features, Fluctuation Noise Lett., 22 (2023), 2350033. https://doi.org/10.1142/S0219477523500335 doi: 10.1142/S0219477523500335
![]() |
[67] |
D. Sasidharan, V. Gopinath, R. Swaminathan, Complexity analysis of surface Electromyography signals under fatigue using Hjorth parameters and bubble entropy, J. Mech. Med. Biol., 23 (2023), 2340051. https://doi.org/10.1142/S0219519423400511 doi: 10.1142/S0219519423400511
![]() |
[68] |
A. Greco, G. Valenza, A. Bicchi, M. Bianchi, E. P. Scilingo, Assessment of muscle fatigue during isometric contraction using autonomic nervous system correlates, Biomed. Signal Process., 51 (2019), 42–49. https://doi.org/10.1016/j.bspc.2019.02.007 doi: 10.1016/j.bspc.2019.02.007
![]() |
[69] |
W. D. Wang, H. H. Li, D. Z. Kong, M. H. Xiao, P. Zhang, A novel fatigue detection method for rehabilitation training of upper limb exoskeleton robot using multi-information fusion, Int. J. Adv. Robot Syst., 17 (2020), 1–11. https://doi.org/10.1177/1729881420974295 doi: 10.1177/1729881420974295
![]() |
[70] |
S. R. Wang, H. Tang, B. Wang, J. Mo, A novel approach to detecting muscle fatigue based on sEMG by using neural architecture search framework, IEEE Trans. Neural Network Learn., 34 (2023), 4932–4943. https://doi.org/10.1109/TNNLS.2021.3124330 doi: 10.1109/TNNLS.2021.3124330
![]() |
[71] |
S. K. Chen, K. L. Xu, X. W. Yao, J. Ge, L. Li, S. Y. Zhu, et al., Information fusion and multi-classifier system for miner fatigue recognition in plateau environments based on electrocardiography and electromyography signals, Comput. Methods Programs Biomed., 211 (2021), 106451. https://doi.org/10.1016/j.cmpb.2021.106451 doi: 10.1016/j.cmpb.2021.106451
![]() |
[72] |
S. K. Chen, K. L. Xu, X. W. Yao, S. Y. Zhu, B. H. Zhang, H. D. Zhou, et al., Psychophysiological data-driven multi-feature information fusion and recognition of miner fatigue in high-altitude and cold areas, Comput. Biol. Med., 133 (2021), 104413. https://doi.org/10.1016/j.compbiomed.2021.104413 doi: 10.1016/j.compbiomed.2021.104413
![]() |
[73] |
Q. Liu, Y. Liu, C. S. Zhang, Z. L. Ruan, W. Meng, Y. L. Cai, et al., SEMG-based dynamic muscle fatigue classification using SVM with improved whale optimization algorithm, IEEE Int. Things, 8 (2021), 16835–16844. https://doi.org/10.1109/JIOT.2021.3056126 doi: 10.1109/JIOT.2021.3056126
![]() |
[74] |
J. X. Liu, Q. Tao, B. Wu, Dynamic muscle fatigue state recognition based on deep learning fusion model, IEEE Access, 11 (2023), 95079–95091. https://doi.org/10.1109/ACCESS.2023.3309741 doi: 10.1109/ACCESS.2023.3309741
![]() |
[75] | J. R. Suganthi, K. Rajeswari, Evaluation of muscle fatigue based on SEMG using deep learning techniques, in 2023 5th International Conference on Inventive Research in Computing Applications (ICIRCA), (2023), 1–6. https://doi.org/10.1109/ICIRCA57980.2023.10220926 |
[76] |
Y. Q. Zhang, S. Y. Chen, W. P. Cao, P. Guo, D. R. Gao, M. Q. Wang, et al., MFFNet: Multi-dimensional feature fusion network based on attention mechanism for sEMG analysis to detect muscle fatigue, Exp. Syst. Appl., 185 (2021), 115639. https://doi.org/10.1016/j.eswa.2021.115639 doi: 10.1016/j.eswa.2021.115639
![]() |
[77] |
Y. K. Dang, Z. T. Liu, X. X. Yang, L. Q. Ge, S. Miao, A fatigue assessment method based on attention mechanism and surface electromyography, Int. Things Cyber-Phys. Syst., 3 (2023), 112–120. https://doi.org/10.1016/j.iotcps.2023.03.002 doi: 10.1016/j.iotcps.2023.03.002
![]() |
1. | F. A. Rihan, H. J. Alsakaji, C. Rajivganthi, Stability and Hopf Bifurcation of Three-Species Prey-Predator System with Time Delays and Allee Effect, 2020, 2020, 1076-2787, 1, 10.1155/2020/7306412 | |
2. | Heba Alsakaji, Fathalla A. Rihan, Rajivganthi Chinnathambi, Dynamics of a Three Species Predator-Prey Delay Differential Model with Allee Effect and Holling Type-II Functional Response, 2018, 1556-5068, 10.2139/ssrn.3273687 | |
3. | Jai Prakash Tripathi, Partha Sarathi Mandal, Ashish Poonia, Vijay Pal Bajiya, A widespread interaction between generalist and specialist enemies: The role of intraguild predation and Allee effect, 2021, 89, 0307904X, 105, 10.1016/j.apm.2020.06.074 | |
4. | Dingyong Bai, Yun Kang, Shigui Ruan, Lisha Wang, Dynamics of an intraguild predation food web model with strong Allee effect in the basal prey, 2021, 58, 14681218, 103206, 10.1016/j.nonrwa.2020.103206 | |
5. | Liyun Lai, Zhenliang Zhu, Fengde Chen, Stability and Bifurcation in a Predator–Prey Model with the Additive Allee Effect and the Fear Effect, 2020, 8, 2227-7390, 1280, 10.3390/math8081280 | |
6. | Hua Liu, Yong Ye, Yumei Wei, Weiyuan Ma, Ming Ma, Kai Zhang, Pattern Formation in a Reaction-Diffusion Predator-Prey Model with Weak Allee Effect and Delay, 2019, 2019, 1076-2787, 1, 10.1155/2019/6282958 | |
7. | Yong Ye, Yi Zhao, Bifurcation Analysis of a Delay-Induced Predator–Prey Model with Allee Effect and Prey Group Defense, 2021, 31, 0218-1274, 2150158, 10.1142/S0218127421501583 | |
8. | R. P. GUPTA, DINESH K. YADAV, ROLE OF ALLEE EFFECT AND HARVESTING OF A FOOD-WEB SYSTEM IN THE PRESENCE OF SCAVENGERS, 2022, 30, 0218-3390, 149, 10.1142/S021833902250005X | |
9. | Hafizul Molla, Sahabuddin Sarwardi, Stacey R. Smith, Mainul Haque, Dynamics of adding variable prey refuge and an Allee effect to a predator–prey model, 2022, 61, 11100168, 4175, 10.1016/j.aej.2021.09.039 | |
10. | Prahlad Majumdar, Sabyasachi Bhattacharya, Susmita Sarkar, Uttam Ghosh, On optimal harvesting policy for two economically beneficial species mysida and herring: a clue for conservation biologist through mathematical model, 2022, 0228-6203, 1, 10.1080/02286203.2022.2064708 | |
11. | Xiaofen Lin, Hua Liu, Xiaotao Han, Yumei Wei, Stability and Hopf bifurcation of an SIR epidemic model with density-dependent transmission and Allee effect, 2022, 20, 1551-0018, 2750, 10.3934/mbe.2023129 | |
12. | Xiaqing He, Zhenliang Zhu, Jialin Chen, Fengde Chen, Dynamical analysis of a Lotka Volterra commensalism model with additive Allee effect, 2022, 20, 2391-5455, 646, 10.1515/math-2022-0055 | |
13. | Dipankar Kumar, Md. Mehedi Hasan, Gour Chandra Paul, Dipok Debnath, Nayan Mondal, Omar Faruk, Revisiting the spatiotemporal dynamics of a diffusive predator-prey system: An analytical approach, 2023, 44, 22113797, 106122, 10.1016/j.rinp.2022.106122 | |
14. | Ali Yousef, Fatma Bozkurt, Thabet Abdeljawad, Qualitative Analysis of a Fractional Pandemic Spread Model of the Novel Coronavirus (Covid-19), 2020, 66, 1546-2226, 843, 10.32604/cmc.2020.012060 | |
15. | Sangeeta Saha, Guruprasad Samanta, Switching effect on a two prey–one predator system with strong Allee effect incorporating prey refuge, 2024, 17, 1793-5245, 10.1142/S1793524523500122 | |
16. | Anuj Kumar Umrao, Prashant K. Srivastava, Bifurcation Analysis of a Predator–Prey Model with Allee Effect and Fear Effect in Prey and Hunting Cooperation in Predator, 2023, 0971-3514, 10.1007/s12591-023-00663-w | |
17. | Dingyong Bai, Jianhong Wu, Bo Zheng, Jianshe Yu, Hydra effect and global dynamics of predation with strong Allee effect in prey and intraspecific competition in predator, 2024, 384, 00220396, 120, 10.1016/j.jde.2023.11.017 | |
18. | S. Biswas, D. Pal, G.S. Mahapatra, Harvesting effect on prey-predator system with strong Allee effect in prey and herd behaviour in both, 2023, 37, 0354-5180, 1561, 10.2298/FIL2305561B | |
19. | Ruma Kumbhakar, Mainul Hossain, Nikhil Pal, Dynamics of a two-prey one-predator model with fear and group defense: A study in parameter planes, 2024, 179, 09600779, 114449, 10.1016/j.chaos.2023.114449 | |
20. | Qun Zhu, Fengde Chen, Impact of Fear on Searching Efficiency of First Species: A Two Species Lotka–Volterra Competition Model with Weak Allee Effect, 2024, 23, 1575-5460, 10.1007/s12346-024-01000-4 | |
21. | Samim Akhtar, Nurul Huda Gazi, Sahabuddin Sarwardi, Mathematical modelling and bifurcation analysis of an eco-epidemiological system with multiple functional responses subjected to Allee effect and competition, 2024, 26667207, 100421, 10.1016/j.rico.2024.100421 |
Variable | Type | Frequency | Percentage (%) |
Gender | Male | 174 | 72.5 |
Female | 66 | 27.5 | |
Age | < 30 | 31 | 12.9 |
30–45 | 139 | 57.9 | |
≥ 46 | 70 | 29.2 | |
Education level | College education | 34 | 14.2 |
Bachelor's degree | 112 | 46.7 | |
Master's degree or above | 94 | 39.2 | |
Role of respondent | Chief Executive Officer | 85 | 35.4 |
Executive management | 91 | 37.9 | |
IT management | 64 | 26.7 | |
Sector | Manufacturing | 122 | 50.8 |
Service | 118 | 49.2 | |
Firm size | Small enterprise | 198 | 82.5 |
Medium enterprise | 42 | 17.5 |
No. | Variable | Data type | Description | |
Technology dimension | ||||
1 | Relative advantage | Continuous | Mean value | |
2 | IT infrastructure | Continuous | Mean value | |
3 | Data quality | Continuous | Mean value | |
4 | Data security | Continuous | Mean value | |
5 | Technical competence | Continuous | Mean value | |
Organization dimension | ||||
6 | Management support | Continuous | Mean value | |
7 | Cost | Continuous | Mean value | |
8 | Firm size | Nominal | 1 = "Small", 2 = "Medium" | |
9 | Sector | Nominal | 1 = "Manufacturing", 2 = "Service" | |
10 | Decision-making culture | Continuous | Mean value | |
Environment dimension | ||||
11 | Competitive pressure | Continuous | Mean value | |
12 | Partner pressure | Continuous | Mean value | |
13 | Government support | Continuous | Mean value | |
Manager's characteristics dimension | ||||
14 | Gender | Nominal | 1 = "Male", 2 = "Female" | |
15 | Age | Nominal | 1 = " < 30", 2 = "30–45", 3 = "≥ 46" | |
16 | Education level | Nominal | 1 = "High school, College/Vocational education", 2 = "Bachelor's degree", 3 = "Master's degree, or above" |
Category | Frequency | Percentage (%) |
1 (Low readiness) | 119 | 49.6 |
2 (High readiness) | 121 | 50.4 |
Total | 240 | 100.0 |
Confusion Matrix of Readiness Low or High |
Predicted value | ||
Low readiness | High readiness | ||
Observed value | Low readiness | True Negative (TN) | False Positive (FP) |
High readiness | False Negative (FN) | True Positive (TP) |
Model type | Title | Training | Testing | AUC | |||
Training | Testing | ||||||
CHAID | Correct | 135 | 83.32% | 52 | 68.42% | 0.891 | 0.747 |
Wrong | 29 | 17.68% | 24 | 31.58% | |||
Total | 164 | 76 | |||||
Bayesian networks | Correct | 136 | 82.93% | 65 | 85.53% | 0.941 | 0.910 |
Wrong | 28 | 17.07% | 11 | 14.47% | |||
Total | 164 | 76 | |||||
Neural network | Correct | 133 | 81.10% | 54 | 71.05% | 0.861 | 0.815 |
Wrong | 31 | 18.90% | 22 | 28.95% | |||
Total | 164 | 76 | |||||
C5.0 | Correct | 143 | 87.20% | 68 | 89.47% | 0.893 | 0.939 |
Wrong | 21 | 12.80% | 8 | 10.53% | |||
Total | 164 | 76 |
Model type | Partition | Title | Coincidence matrix | Accuracy | Precision | Recall | Specificity | F-measure | |
Low readiness |
High readiness |
||||||||
CHAID | Training | Low readiness | 69 | 9 | 0.8232 | 0.8800 | 0.7674 | 0.8846 | 0.8199 |
High readiness | 20 | 66 | |||||||
Total | 89 | 75 | |||||||
Testing | Low readiness | 28 | 13 | 0.6842 | 0.6486 | 0.6857 | 0.6829 | 0.6667 | |
High readiness | 11 | 24 | |||||||
Total | 39 | 37 | |||||||
Bayesian networks | Training | Low readiness | 70 | 8 | 0.8293 | 0.8919 | 0.7674 | 0.8974 | 0.8250 |
High readiness | 20 | 66 | |||||||
Total | 90 | 74 | |||||||
Testing | Low readiness | 37 | 4 | 0.8553 | 0.8750 | 0.8000 | 0.9024 | 0.8358 | |
High readiness | 7 | 28 | |||||||
Total | 44 | 32 | |||||||
Neural network | Training | Low readiness | 65 | 13 | 0.8110 | 0.8395 | 0.7907 | 0.8333 | 0.8144 |
High readiness | 18 | 68 | |||||||
Total | 83 | 81 | |||||||
Testing | Low readiness | 27 | 14 | 0.7105 | 0.6585 | 0.7714 | 0.6585 | 0.7105 | |
High readiness | 8 | 27 | |||||||
Total | 35 | 41 | |||||||
C5.0 | Training | Low readiness | 67 | 11 | 0.8720 | 0.8736 | 0.8837 | 0.8590 | 0.8786 |
High readiness | 10 | 76 | |||||||
Total | 77 | 87 | |||||||
Testing | Low readiness | 36 | 5 | 0.8947 | 0.8649 | 0.9143 | 0.8780 | 0.8889 | |
High readiness | 3 | 32 | |||||||
Total | 39 | 37 |
Variable | Technique | Total value | |||
CHAID | Bayesian networks | Neural network | C5.0 | ||
Management support | 0.3566 | 0.3627 | 0.1179 | 0.2029 | 1.0401 |
Data quality | 0.2079 | 0.2007 | 0.0973 | 0.2073 | 0.7132 |
Firm size | 0.1485 | 0.0675 | 0.0000 | 0.1099 | 0.3259 |
Data security | 0.0954 | 0.0455 | 0.1398 | 0.0000 | 0.2807 |
Cost | 0.0000 | 0.0000 | 0.1613 | 0.0779 | 0.2392 |
Sector | 0.0759 | 0.0293 | 0.0516 | 0.0685 | 0.2253 |
Competitive pressure | 0.0028 | 0.0936 | 0.0610 | 0.0297 | 0.1871 |
Partner pressure | 0.0000 | 0.0728 | 0.0775 | 0.0000 | 0.1503 |
Gender | 0.0000 | 0.0000 | 0.0410 | 0.0958 | 0.1368 |
Government support | 0.0254 | 0.0000 | 0.0720 | 0.0000 | 0.0974 |
Technical competence | 0.0000 | 0.0507 | 0.0443 | 0.0000 | 0.0950 |
IT infrastructure | 0.0000 | 0.0000 | 0.0000 | 0.0747 | 0.0747 |
Age | 0.0028 | 0.0000 | 0.0000 | 0.0541 | 0.0569 |
Decision-making culture | 0.0000 | 0.0349 | 0.0000 | 0.0000 | 0.0349 |
Education level | 0.0343 | 0.0000 | 0.0000 | 0.0000 | 0.0343 |
Relative advantage | 0.0000 | 0.0288 | 0.0000 | 0.0000 | 0.0288 |
Variable | Item number | Factor loadings | Cronbach α | CR | AVE |
Relative advantage | 4 | 0.530–0.865 | 0.807 | 0.805 | 0.519 |
IT structure | 3 | 0.617–0.848 | 0.798 | 0.809 | 0.590 |
Data quality | 3 | 0.569–0.728 | 0.691 | 0.714 | 0.457 |
Data security | 3 | 0.705–0.756 | 0.716 | 0.764 | 0.520 |
Technical competence | 4 | 0.744–0.816 | 0.867 | 0.867 | 0.620 |
Management support | 3 | 0.520–0.781 | 0.707 | 0.722 | 0.471 |
Cost | 3 | 0.755–0.849 | 0.798 | 0.843 | 0.643 |
Decision-making culture | 3 | 0.615–0.846 | 0.746 | 0.768 | 0.528 |
Competitive pressure | 3 | 0.787–0.842 | 0.856 | 0.856 | 0.664 |
Partner pressure | 3 | 0.690–0.717 | 0.626 | 0.751 | 0.501 |
Government support | 3 | 0.703–0.727 | 0.719 | 0.757 | 0.509 |
Readiness to adopt big data | 9 | 0.684–0.810 | 0.773 | 0.909 | 0.526 |
*Note: CR: Composite Reliability, AVE: Average Variance Extracted |
Fold No. | CHAID | Bayesian networks | Neural network | C5.0 | ||||||||
Confusion matrix | Accuracy | Confusion matrix | Accuracy | Confusion matrix | Accuracy | Confusion matrix | Accuracy | |||||
1 | 12 | 5 | 0.649 | 13 | 4 | 0.784 | 12 | 5 | 0.703 | 14 | 3 | 0.892 |
11 | 9 | 4 | 16 | 6 | 14 | 1 | 19 | |||||
2 | 21 | 11 | 0.729 | 28 | 4 | 0.847 | 26 | 6 | 0.780 | 27 | 5 | 0.881 |
5 | 22 | 5 | 22 | 7 | 20 | 2 | 25 | |||||
3 | 28 | 13 | 0.684 | 37 | 4 | 0.855 | 27 | 14 | 0.711 | 36 | 5 | 0.895 |
11 | 24 | 7 | 28 | 8 | 27 | 3 | 32 | |||||
4 | 32 | 21 | 0.615 | 49 | 4 | 0.846 | 36 | 17 | 0.692 | 45 | 8 | 0.875 |
25 | 26 | 12 | 39 | 15 | 36 | 5 | 46 | |||||
5 | 53 | 10 | 0.736 | 58 | 5 | 0.840 | 46 | 17 | 0.720 | 55 | 8 | 0.888 |
23 | 39 | 15 | 47 | 18 | 44 | 6 | 56 | |||||
6 | 46 | 25 | 0.697 | 64 | 7 | 0.828 | 51 | 20 | 0.752 | 62 | 9 | 0.890 |
19 | 55 | 18 | 56 | 16 | 58 | 7 | 67 | |||||
7 | 66 | 13 | 0.669 | 71 | 20 | 0.828 | 65 | 14 | 0.761 | 69 | 9 | 0.883 |
41 | 43 | 8 | 64 | 25 | 59 | 10 | 75 | |||||
8 | 84 | 12 | 0.728 | 87 | 23 | 0.836 | 62 | 34 | 0.692 | 83 | 13 | 0.887 |
41 | 58 | 9 | 76 | 26 | 73 | 9 | 90 | |||||
9 | 93 | 14 | 0.657 | 96 | 11 | 0.833 | 86 | 21 | 0.681 | 93 | 14 | 0.884 |
60 | 49 | 25 | 84 | 48 | 61 | 11 | 98 | |||||
10 | 62 | 55 | 0.627 | 105 | 12 | 0.835 | 44 | 18 | 0.732 | 101 | 16 | 0.877 |
33 | 86 | 27 | 92 | 22 | 65 | 13 | 106 | |||||
Average | 0.679 | 0.833 | 0.722 | 0.885 | ||||||||
Confusion matrix illustrates the classification of the cases in the test dataset. In the confusion matrix, the columns represent the actual cases, and the rows represent the predicted. Accuracy = (TP + TN)/(TP + FP + TN + FN). |
Variable | Type | Frequency | Percentage (%) |
Gender | Male | 174 | 72.5 |
Female | 66 | 27.5 | |
Age | < 30 | 31 | 12.9 |
30–45 | 139 | 57.9 | |
≥ 46 | 70 | 29.2 | |
Education level | College education | 34 | 14.2 |
Bachelor's degree | 112 | 46.7 | |
Master's degree or above | 94 | 39.2 | |
Role of respondent | Chief Executive Officer | 85 | 35.4 |
Executive management | 91 | 37.9 | |
IT management | 64 | 26.7 | |
Sector | Manufacturing | 122 | 50.8 |
Service | 118 | 49.2 | |
Firm size | Small enterprise | 198 | 82.5 |
Medium enterprise | 42 | 17.5 |
No. | Variable | Data type | Description | |
Technology dimension | ||||
1 | Relative advantage | Continuous | Mean value | |
2 | IT infrastructure | Continuous | Mean value | |
3 | Data quality | Continuous | Mean value | |
4 | Data security | Continuous | Mean value | |
5 | Technical competence | Continuous | Mean value | |
Organization dimension | ||||
6 | Management support | Continuous | Mean value | |
7 | Cost | Continuous | Mean value | |
8 | Firm size | Nominal | 1 = "Small", 2 = "Medium" | |
9 | Sector | Nominal | 1 = "Manufacturing", 2 = "Service" | |
10 | Decision-making culture | Continuous | Mean value | |
Environment dimension | ||||
11 | Competitive pressure | Continuous | Mean value | |
12 | Partner pressure | Continuous | Mean value | |
13 | Government support | Continuous | Mean value | |
Manager's characteristics dimension | ||||
14 | Gender | Nominal | 1 = "Male", 2 = "Female" | |
15 | Age | Nominal | 1 = " < 30", 2 = "30–45", 3 = "≥ 46" | |
16 | Education level | Nominal | 1 = "High school, College/Vocational education", 2 = "Bachelor's degree", 3 = "Master's degree, or above" |
Category | Frequency | Percentage (%) |
1 (Low readiness) | 119 | 49.6 |
2 (High readiness) | 121 | 50.4 |
Total | 240 | 100.0 |
Confusion Matrix of Readiness Low or High |
Predicted value | ||
Low readiness | High readiness | ||
Observed value | Low readiness | True Negative (TN) | False Positive (FP) |
High readiness | False Negative (FN) | True Positive (TP) |
Model type | Title | Training | Testing | AUC | |||
Training | Testing | ||||||
CHAID | Correct | 135 | 83.32% | 52 | 68.42% | 0.891 | 0.747 |
Wrong | 29 | 17.68% | 24 | 31.58% | |||
Total | 164 | 76 | |||||
Bayesian networks | Correct | 136 | 82.93% | 65 | 85.53% | 0.941 | 0.910 |
Wrong | 28 | 17.07% | 11 | 14.47% | |||
Total | 164 | 76 | |||||
Neural network | Correct | 133 | 81.10% | 54 | 71.05% | 0.861 | 0.815 |
Wrong | 31 | 18.90% | 22 | 28.95% | |||
Total | 164 | 76 | |||||
C5.0 | Correct | 143 | 87.20% | 68 | 89.47% | 0.893 | 0.939 |
Wrong | 21 | 12.80% | 8 | 10.53% | |||
Total | 164 | 76 |
Model type | Partition | Title | Coincidence matrix | Accuracy | Precision | Recall | Specificity | F-measure | |
Low readiness |
High readiness |
||||||||
CHAID | Training | Low readiness | 69 | 9 | 0.8232 | 0.8800 | 0.7674 | 0.8846 | 0.8199 |
High readiness | 20 | 66 | |||||||
Total | 89 | 75 | |||||||
Testing | Low readiness | 28 | 13 | 0.6842 | 0.6486 | 0.6857 | 0.6829 | 0.6667 | |
High readiness | 11 | 24 | |||||||
Total | 39 | 37 | |||||||
Bayesian networks | Training | Low readiness | 70 | 8 | 0.8293 | 0.8919 | 0.7674 | 0.8974 | 0.8250 |
High readiness | 20 | 66 | |||||||
Total | 90 | 74 | |||||||
Testing | Low readiness | 37 | 4 | 0.8553 | 0.8750 | 0.8000 | 0.9024 | 0.8358 | |
High readiness | 7 | 28 | |||||||
Total | 44 | 32 | |||||||
Neural network | Training | Low readiness | 65 | 13 | 0.8110 | 0.8395 | 0.7907 | 0.8333 | 0.8144 |
High readiness | 18 | 68 | |||||||
Total | 83 | 81 | |||||||
Testing | Low readiness | 27 | 14 | 0.7105 | 0.6585 | 0.7714 | 0.6585 | 0.7105 | |
High readiness | 8 | 27 | |||||||
Total | 35 | 41 | |||||||
C5.0 | Training | Low readiness | 67 | 11 | 0.8720 | 0.8736 | 0.8837 | 0.8590 | 0.8786 |
High readiness | 10 | 76 | |||||||
Total | 77 | 87 | |||||||
Testing | Low readiness | 36 | 5 | 0.8947 | 0.8649 | 0.9143 | 0.8780 | 0.8889 | |
High readiness | 3 | 32 | |||||||
Total | 39 | 37 |
Variable | Technique | Total value | |||
CHAID | Bayesian networks | Neural network | C5.0 | ||
Management support | 0.3566 | 0.3627 | 0.1179 | 0.2029 | 1.0401 |
Data quality | 0.2079 | 0.2007 | 0.0973 | 0.2073 | 0.7132 |
Firm size | 0.1485 | 0.0675 | 0.0000 | 0.1099 | 0.3259 |
Data security | 0.0954 | 0.0455 | 0.1398 | 0.0000 | 0.2807 |
Cost | 0.0000 | 0.0000 | 0.1613 | 0.0779 | 0.2392 |
Sector | 0.0759 | 0.0293 | 0.0516 | 0.0685 | 0.2253 |
Competitive pressure | 0.0028 | 0.0936 | 0.0610 | 0.0297 | 0.1871 |
Partner pressure | 0.0000 | 0.0728 | 0.0775 | 0.0000 | 0.1503 |
Gender | 0.0000 | 0.0000 | 0.0410 | 0.0958 | 0.1368 |
Government support | 0.0254 | 0.0000 | 0.0720 | 0.0000 | 0.0974 |
Technical competence | 0.0000 | 0.0507 | 0.0443 | 0.0000 | 0.0950 |
IT infrastructure | 0.0000 | 0.0000 | 0.0000 | 0.0747 | 0.0747 |
Age | 0.0028 | 0.0000 | 0.0000 | 0.0541 | 0.0569 |
Decision-making culture | 0.0000 | 0.0349 | 0.0000 | 0.0000 | 0.0349 |
Education level | 0.0343 | 0.0000 | 0.0000 | 0.0000 | 0.0343 |
Relative advantage | 0.0000 | 0.0288 | 0.0000 | 0.0000 | 0.0288 |
Variable | Item number | Factor loadings | Cronbach α | CR | AVE |
Relative advantage | 4 | 0.530–0.865 | 0.807 | 0.805 | 0.519 |
IT structure | 3 | 0.617–0.848 | 0.798 | 0.809 | 0.590 |
Data quality | 3 | 0.569–0.728 | 0.691 | 0.714 | 0.457 |
Data security | 3 | 0.705–0.756 | 0.716 | 0.764 | 0.520 |
Technical competence | 4 | 0.744–0.816 | 0.867 | 0.867 | 0.620 |
Management support | 3 | 0.520–0.781 | 0.707 | 0.722 | 0.471 |
Cost | 3 | 0.755–0.849 | 0.798 | 0.843 | 0.643 |
Decision-making culture | 3 | 0.615–0.846 | 0.746 | 0.768 | 0.528 |
Competitive pressure | 3 | 0.787–0.842 | 0.856 | 0.856 | 0.664 |
Partner pressure | 3 | 0.690–0.717 | 0.626 | 0.751 | 0.501 |
Government support | 3 | 0.703–0.727 | 0.719 | 0.757 | 0.509 |
Readiness to adopt big data | 9 | 0.684–0.810 | 0.773 | 0.909 | 0.526 |
*Note: CR: Composite Reliability, AVE: Average Variance Extracted |
Fold No. | CHAID | Bayesian networks | Neural network | C5.0 | ||||||||
Confusion matrix | Accuracy | Confusion matrix | Accuracy | Confusion matrix | Accuracy | Confusion matrix | Accuracy | |||||
1 | 12 | 5 | 0.649 | 13 | 4 | 0.784 | 12 | 5 | 0.703 | 14 | 3 | 0.892 |
11 | 9 | 4 | 16 | 6 | 14 | 1 | 19 | |||||
2 | 21 | 11 | 0.729 | 28 | 4 | 0.847 | 26 | 6 | 0.780 | 27 | 5 | 0.881 |
5 | 22 | 5 | 22 | 7 | 20 | 2 | 25 | |||||
3 | 28 | 13 | 0.684 | 37 | 4 | 0.855 | 27 | 14 | 0.711 | 36 | 5 | 0.895 |
11 | 24 | 7 | 28 | 8 | 27 | 3 | 32 | |||||
4 | 32 | 21 | 0.615 | 49 | 4 | 0.846 | 36 | 17 | 0.692 | 45 | 8 | 0.875 |
25 | 26 | 12 | 39 | 15 | 36 | 5 | 46 | |||||
5 | 53 | 10 | 0.736 | 58 | 5 | 0.840 | 46 | 17 | 0.720 | 55 | 8 | 0.888 |
23 | 39 | 15 | 47 | 18 | 44 | 6 | 56 | |||||
6 | 46 | 25 | 0.697 | 64 | 7 | 0.828 | 51 | 20 | 0.752 | 62 | 9 | 0.890 |
19 | 55 | 18 | 56 | 16 | 58 | 7 | 67 | |||||
7 | 66 | 13 | 0.669 | 71 | 20 | 0.828 | 65 | 14 | 0.761 | 69 | 9 | 0.883 |
41 | 43 | 8 | 64 | 25 | 59 | 10 | 75 | |||||
8 | 84 | 12 | 0.728 | 87 | 23 | 0.836 | 62 | 34 | 0.692 | 83 | 13 | 0.887 |
41 | 58 | 9 | 76 | 26 | 73 | 9 | 90 | |||||
9 | 93 | 14 | 0.657 | 96 | 11 | 0.833 | 86 | 21 | 0.681 | 93 | 14 | 0.884 |
60 | 49 | 25 | 84 | 48 | 61 | 11 | 98 | |||||
10 | 62 | 55 | 0.627 | 105 | 12 | 0.835 | 44 | 18 | 0.732 | 101 | 16 | 0.877 |
33 | 86 | 27 | 92 | 22 | 65 | 13 | 106 | |||||
Average | 0.679 | 0.833 | 0.722 | 0.885 | ||||||||
Confusion matrix illustrates the classification of the cases in the test dataset. In the confusion matrix, the columns represent the actual cases, and the rows represent the predicted. Accuracy = (TP + TN)/(TP + FP + TN + FN). |