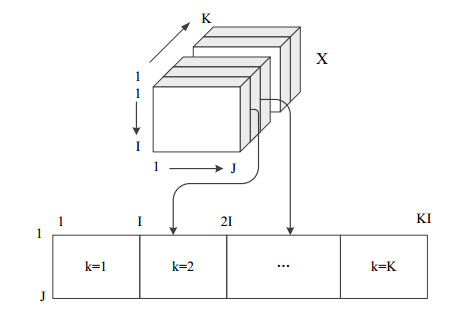
Citation: Xiaochen Sheng, Weili Xiong. Soft sensor design based on phase partition ensemble of LSSVR models for nonlinear batch processes[J]. Mathematical Biosciences and Engineering, 2020, 17(2): 1901-1921. doi: 10.3934/mbe.2020100
[1] | Xiaoshan Qian, Lisha Xu, Xinmei Yuan . Dynamic correction of soft measurement model for evaporation process parameters based on ARMA. Mathematical Biosciences and Engineering, 2024, 21(1): 712-735. doi: 10.3934/mbe.2024030 |
[2] | Xiaoshan Qian, Lisha Xu, Xinmei Yuan . Soft-sensing modeling of mother liquor concentration in the evaporation process based on reduced robust least-squares support-vector machine. Mathematical Biosciences and Engineering, 2023, 20(11): 19941-19962. doi: 10.3934/mbe.2023883 |
[3] | Ping Wang, Qiaoyan Sun, Yuxin Qiao, Lili Liu, Xiang Han, Xiangguang Chen . Online prediction of total sugar content and optimal control of glucose feed rate during chlortetracycline fermentation based on soft sensor modeling. Mathematical Biosciences and Engineering, 2022, 19(10): 10687-10709. doi: 10.3934/mbe.2022500 |
[4] | Wei Zou, Yanxia Shen, Lei Wang . Design of robust fuzzy iterative learning control for nonlinear batch processes. Mathematical Biosciences and Engineering, 2023, 20(11): 20274-20294. doi: 10.3934/mbe.2023897 |
[5] | Mei-Ling Huang, Zong-Bin Huang . An ensemble-acute lymphoblastic leukemia model for acute lymphoblastic leukemia image classification. Mathematical Biosciences and Engineering, 2024, 21(2): 1959-1978. doi: 10.3934/mbe.2024087 |
[6] | Naila Naz, Muazzam A Khan, Suliman A. Alsuhibany, Muhammad Diyan, Zhiyuan Tan, Muhammad Almas Khan, Jawad Ahmad . Ensemble learning-based IDS for sensors telemetry data in IoT networks. Mathematical Biosciences and Engineering, 2022, 19(10): 10550-10580. doi: 10.3934/mbe.2022493 |
[7] | Peng-Yeng Yin, Chih-Chun Tsai, Rong-Fuh Day, Ching-Ying Tung, Bir Bhanu . Ensemble learning of model hyperparameters and spatiotemporal data for calibration of low-cost PM2.5 sensors. Mathematical Biosciences and Engineering, 2019, 16(6): 6858-6873. doi: 10.3934/mbe.2019343 |
[8] | Natalya Shakhovska, Vitaliy Yakovyna, Valentyna Chopyak . A new hybrid ensemble machine-learning model for severity risk assessment and post-COVID prediction system. Mathematical Biosciences and Engineering, 2022, 19(6): 6102-6123. doi: 10.3934/mbe.2022285 |
[9] | Wangping Xiong, Yimin Zhu, Qingxia Zeng, Jianqiang Du, Kaiqi Wang, Jigen Luo, Ming Yang, Xian Zhou . Dose-effect relationship analysis of TCM based on deep Boltzmann machine and partial least squares. Mathematical Biosciences and Engineering, 2023, 20(8): 14395-14413. doi: 10.3934/mbe.2023644 |
[10] | Ji-Ming Wu, Wang-Ren Qiu, Zi Liu, Zhao-Chun Xu, Shou-Hua Zhang . Integrative approach for classifying male tumors based on DNA methylation 450K data. Mathematical Biosciences and Engineering, 2023, 20(11): 19133-19151. doi: 10.3934/mbe.2023845 |
In chemical processes, accurate real-time predictions of product quality are highly desirable, which is critical to realize successful process control, monitoring and optimization [1,2]. However, due to the costs of online analyzer and offline laboratory analysis, the process often encounters the great challenge of lacking reliable quality estimation. Soft sensing technique, which aims to construct theoretical or statistical models that can describe the functional relationship between process variables (easy-to-measure variables) and quality variables (difficult-to-measure variables), is proposed to address this issue and attracts much attention in both academia and industry. Generally, soft sensors can be classified into three groups: Model-driven, data-driven and mixed models [3,4,5,6]. Compared with model-driven method, data-driven one does not require in-depth mechanical knowledge of processes and only relies on recorded process datasets, which shows great flexibility and low complexity. Many dynamical models, such as nonlinear autoregressive with exogenous inputs (NARX) [7], and data-driven models, such as partial least squares (PLS), artificial neural networks (ANN), support vector machine (SVM), and Gaussian process regression (GPR) [8,9,10,11,12], have been successfully applied to online quality prediction.
Batch processes play an important role in the production of food, drugs, special chemicals and biological industrial products, which have high requirements for product quality and safe operation. In addition to the nonlinear and time-varying characteristics, other distinct characteristics, such as instability, finite duration, and batch-to-batch variations, are quite different from those of continuous processes [13,14]. It is difficult to construct accurate predictive models as the operating conditions vary. Furthermore, datasets obtained from batch processes are high-dimensional, including different batches, variables, and sampling time. Thus, they cannot be directly used for modeling and need to be preprocessed. Generally, multidimensional datasets contain abundant process information that can contribute to informative models, but it may also lead to information redundancy and complex model structure. Thus, dimension reduction and significant feature information extraction are crucial in satisfactory soft sensor development. Multiway principal component analysis (MPCA) [15,16,17] and multiway PLS (MPLS) [18,19] have been successfully applied in the fault diagnosis and soft sensing for batch processes. MPCA can be used to realize data analysis and preprocessing. Variable-wise unfolding method, which tends to keep the track of variables and retain the overall change information of process variables in batch and time, is introduced to obtain the two-dimensional datasets. Then, ordinary PCA is applied to dimensionality reduction and extract maximum amount of process information, making it more effective to soft sensor modeling.
Traditional nonlinear soft sensors can achieve a universal generalization performance in quality prediction of chemical processes. However, many of them rely on a single global model under the assumption that the operating phases and conditions are constant in the whole process. With operating conditions or product demands changing, processes exhibit apparent multiphase behaviors while different phases present various process characteristics, thereby resulting in the poor regression accuracy of global models.
Ensemble learning has been investigated and developed to be an effective tool to improve the generalization performance of soft sensors, especially for multiphase or multimode batch processes [20]. Under ensemble learning framework, the process dataset is partitioned into several local domains, then a series of local high-performance models are constructed and integrated to make a final quality prediction. Instead of global model construction, ensemble model based soft sensors can greatly enhance estimation accuracy and maintain satisfactory performance for a long time even though process characteristics change. The first step of ensemble learning method is to generate subsets from process data samples. Several popular data partition approaches include bagging [21], boosting [22], clustering [23] and the subspace method [24]. Clustering based methods, such as K-means, fuzzy C-means (FCM) [25], and Gaussian mixture model (GMM) [26], have been widely used and have shown their effectiveness in data clustering for multiphase processes. For example, Wang et al. used GMM to create local partitions and verified the feasibility and reliability of the proposed soft sensor [26]. However, this method only considers one batch of process data and does not take multiphase characteristics into account. In addition, the dataset length of each batch may not be equal because of the complex operating conditions in actual processes. Prediction combination is another important step of ensemble learning method. Traditional approaches for this purpose are averaging, voting, Bayesian inference, and learning method [2,20,26]. Bayesian fusion method has been proven to be a natural fit for ensemble model combination due to its strong statistical learning and analytical abilities from datasets [27,28]. It can remarkably and effectively utilize the limited process information.
Motivated to address the aforementioned issues, a novel ensemble learning based soft sensor, namely ensemble least squares support vector regression (LSSVR) [29,30] based on GMM method (GMM-LSSVR), is developed in this paper for the quality prediction of multiphase/multimode nonlinear batch processes. Firstly, MPCA is applied to data unfolding and information extraction for original 3-dimensional process datasets. In this method, the feature vectors corresponding to the large feature values are selected to form a subspace, where original datasets are mapped, then the preprocessed low-dimensional data matrix can be obtained for soft sensor modeling. Secondly, the Bayesian information criterion (BIC) [31] technique is introduced to determine the optimal number of Gaussian components for phase partition. And the newly obtained datasets are divided into several different subsets by GMM method to produce ensemble components. Thirdly, the grid search (GS) [32] method is used to generate all possible parameter pairs (σ, γ) due to its significant search effect and easy implementation. Meanwhile, ten-fold cross-validation [33] technique is employed to calculate the average relative error and evaluate the optimality of each pair. In such cases, an optimal parameter pair can be determined for each local LSSVR model, which greatly contributes to reliability enhancement. Finally, the Bayesian inference strategy is used to estimate the posterior probability of each test sample with respect to different operation dynamics and multiple models are combined with posterior probability based weightings for the final prediction.
The remainder of this paper is organized as follows. Section 2 briefly reviews LSSVR model, MPCA and GMM methods. Section 3 presents some details of the proposed novel soft sensor, including its modeling method, parameters determination, and combination strategy. Section 4 evaluates the effectiveness of the modeling method via simulation results in a batch process. Finally, Section 5 draws the conclusions of this paper.
The LSSVR model is modified from support vector regression (SVR) [29]. Instead of inequality constraints applied, LSSVR uses equality constraints in the optimization problem in order to turn the convex quadratic optimization procedure into the solution of linear equations, which has shown its great ability in dealing with significant nonlinearity in batch processes. Thus, LSSVR is applied to construct local models upon the several partitioned regions in this paper.
Given
{minJ(ωω,ζ)=12ωωTωω+γ12ζζTζζs.t.y=ZTωω+bI+ζζ | (1) |
where
By introducing Lagrange multipliers
L(ωω,b,ζ,αα)=J(ωω,ζ)−ααT(ZTωω+bI+ζζ−y) | (2) |
The following linear equations can be obtained by referring to the Karush-Kuhn-Tucker (KKT) condition for optimality.
{∂L∂ωω=0⇒ωω=Zαα∂L∂b=0⇒ααTI=0∂L∂ζζ=0⇒αα=γζζ∂L∂αα=0⇒ZTωω+bI+ζζ−y=0 | (3) |
Then, a linear system can be described by simplifying equations and eliminating
[bαα]=[01T1H]−1[0y] | (4) |
where
Ki,j=φ(xi)Tφ(xj)=k(xi,xj), ∀(i,j)∈NN×NN | (5) |
In this work, the Gaussian kernel function is adopted to be the kernel function of LSSVR:
f\left( {\bf{x}} \right) = \varphi {\left( {\bf{x}} \right)^{\rm{T}}}{{\bf{ \pmb{\mathsf{ ω}} }}^*} + {b^*} = \varphi {\left( {\bf{x}} \right)^{\rm{T}}}{\bf{Z}}{{\bf{ \pmb{\mathsf{ α}} }}^*} + {b^*} = \sum\limits_{i = 1}^N {{\bf{ \pmb{\mathsf{ α}} }}_i^*\varphi {{\left( {\bf{x}} \right)}^{\rm{T}}}\varphi \left( {{{\bf{x}}_i}} \right)} + {b^*} = \sum\limits_{i = 1}^N {{\bf{ \pmb{\mathsf{ α}} }}_i^*k\left( {{\bf{x}}, {{\bf{x}}_i}} \right)} + {b^*} | (6) |
In batch processes, the collected datasets are related to batches, variables and sampling time. Excessive data information may lead to information redundancy and deteriorate the estimation performance of soft sensor models. MPCA method has been proven to be effective in dimensionality reduction and widely used in data preprocessing of batch processes.
The dataset of a batch process can be given as a three-dimensional matrix
In this way, the original dataset can be rewritten into a new KI-dimensional variable space, then the new data matrix is preprocessed by
\left\{ \begin{array}{l} {\overline x _{i, j, k}} = \frac{{{x_{i, j, k}} - {{\overline x }_j}}}{{{s_{j, k}}}} \\ {\overline x _j} = \frac{1}{{KI}}\sum\limits_{k = 1}^K {\sum\limits_{i = 1}^I {{x_{i, j, k}}} } \\ {s_{j, k}} = \sqrt {\frac{1}{{KI}}\sum\limits_{k = 1}^K {\sum\limits_{i = 1}^I {{{\left( {{x_{i, j, k}} - {{\overline x }_j}} \right)}^2}} } } \\ \end{array} \right. | (7) |
where
For the standard dataset
{\bf{X}}\left( {{\bf{KI}}{\rm{ \times }}{\bf{J}}} \right) = {\bf{T}}{{\bf{P}}^{\rm{T}}} + {\bf{E}} | (8) |
where
As an effective probabilistic approach for data clustering, GMM is widely used for process monitoring and soft sensor application. The main purpose of GMM method is to identify and localize phase of data samples in batch processes.
Consider a training dataset consisting of
p({\bf{x}}\mathit{\boldsymbol{| \boldsymbol{\varTheta} }}) = \sum\limits_{g = 1}^G {{\pi _g}p({\bf{x}}\mathit{\boldsymbol{|}}{\mathit{\boldsymbol{ \boldsymbol{\varTheta} }}_g})} | (9) |
where
\sum\limits_{g = 1}^G {{\pi _g} = 1} , \ \ 0 \leqslant {\pi _g} \leqslant 1 | (10) |
And
p({\bf{x}}\mathit{\boldsymbol{|}}{\mathit{\boldsymbol{ \boldsymbol{\varTheta} }}_g}) = \frac{1}{{\sqrt {{{(2\pi )}^m}\left| {{\sum _g}} \right|} }} \times \exp \left[ { - \tfrac{1}{2}{{\left( {{\bf{x}}\mathit{\boldsymbol{ - }}{\mu _g}} \right)}^{\rm{T}}}{\sum _g}^{ - 1}\left( {{\bf{x}}\mathit{\boldsymbol{ - }}{\mu _g}} \right)} \right] | (11) |
Assume that data samples follow a mixture of a finite number of Gaussian distributions, it can be seen that each Gaussian component has three parameters (
L({\bf{x}}\mathit{\boldsymbol{| \boldsymbol{\varTheta} }}) = \log \mathop \prod \limits_{i = 1}^N \sum\limits_{g = 1}^G {{\pi _g}p({\bf{x}}\mathit{\boldsymbol{|}}{\mathit{\boldsymbol{ \boldsymbol{\varTheta} }}_g})} = \sum\limits_{i = 1}^N {\log } \sum\limits_{g = 1}^G {{\pi _g}p({\bf{x}}\mathit{\boldsymbol{|}}{\mathit{\boldsymbol{ \boldsymbol{\varTheta} }}_g})} | (12) |
Then, expectation maximization (EM) algorithm, is introduced to estimate the optimal parameters by iterative calculation, which consist of E step and M step:
E step: Evaluate the posterior probability that ith training data samples, which belongs to the gth Gaussian component by using current parameter values.
p({C_g}|{{\bf{x}}_i}) = \frac{{{\pi _g}p({{\bf{x}}_i}|{\mathit{\Theta} _g})}}{{\sum\limits_{g = 1}^G {{\pi _g}p({{\bf{x}}_i}|{\mathit{\Theta} _g})} }}, \ \ i = 1, 2, \cdots N | (13) |
M step: Obtain the corresponding likelihood function via the posterior probability calculated by E step. Re-estimate the parameters using the current value.
{\mu _g} = \frac{{\sum\limits_{i = 1}^N {p\left( {{C_g}\left| {{{\bf{x}}_i}} \right.} \right)} {{\bf{x}}_i}}}{{\sum\limits_{i = 1}^N {p\left( {{C_g}\left| {{{\bf{x}}_i}} \right.} \right)} }} | (14) |
{\pi _g} = \frac{{\sum\limits_{i = 1}^N {p\left( {{C_g}\left| {{{\bf{x}}_i}} \right.} \right)} }}{N} | (15) |
{\sum _g} = \frac{{\sum\limits_{i = 1}^N {p\left( {{C_g}\left| {{{\bf{x}}_i}} \right.} \right)} \left( {{{\bf{x}}_i} - {{\bf{ \pmb{\mathsf{ μ}} }}_g}} \right){{\left( {{{\bf{x}}_i} - {{\bf{ \pmb{\mathsf{ μ}} }}_g}} \right)}^{\rm{T}}}}}{{\sum\limits_{i = 1}^N {p\left( {{C_g}\left| {{{\bf{x}}_i}} \right.} \right)} }} | (16) |
The parameter estimation process is not completed until the convergence is satisfied. For batch processes, the number of Gaussian components of GMM model corresponds to the number of stages of the process. Moreover, the mixing coefficient of each Gaussian component for a data sample is determined by the average posterior probability of the sample with respect to the corresponding component.
Parameter determination is an important step of model construction, and it can directly affect the generalization behavior of regression models. The multi-model parameter optimization method shows its strong superiority in tackling parametric uncertainty problems when industrial processes are complex and time-varying [34].
LSSVR models need to determine regularization coefficients and kernel parameters. The commonly used methods for parameter determination include GS and swarm intelligence optimization [36,37,38,39]. In this study, the parameters of the LSSVR model are determined by ten-fold cross-validation and GS methods. First, for the parameter pair (σ, γ) that needs to be determined, GS method is used to form the grid in the given parameter selection interval. Second, the average relative error (Eq. (17)) of the corresponding model is calculated by ten-fold cross-validation method at the grid point. Finally, the parameter pair with the minimum error value is selected as an optimal pair.
\delta = \frac{1}{N}\sum\limits_{i = 1}^N {\frac{{\left| {{y_i} - {{\widehat y}_i}} \right|}}{{{y_i}}}} | (17) |
where
The steps of LSSVR parameter determination are presented as follows:
Step 1: Assign an initial value to σ and γ.
Step 2: Determine the search range of σ and γ.
Step 3: Determine the grid point position of the first cross-validation calculation according to the initial value.
Step 4: Select ten-fold cross-validation as the objective function of grid point calculation. Then calculate the errors of all grid points.
Step 5: Compare the error results and determine an optimal parameter pair.
Some traditional soft sensors construct a global regression model for quality prediction; it ignores the multiphase and multistage characteristics of batch processes. Fortunately, ensemble learning based local modeling methods, which can better meet the requirements of prediction accuracy by combining multiple local models, have drawn increasing attention to improving the performance of soft sensors. Therefore, a novel soft sensor, referred to as ensemble LSSVR based on GMM (GMM-LSSVR), is proposed for quality prediction in multiphase batch processes. First, MPCA is employed to data preprocessing, including three-dimensional data unfolding and dimensionality reduction. And GMM method is applied to divide the preprocessed dataset into multiple local domains. Then, several local LSSVR models are constructed for all identified subsets. Meanwhile, optimal hyperparameters are determined by combining ten-fold cross-validation with GS method. Finally, according to the posterior probability of the new sample to each operation period (Eq (18)), the high-performance predictions of local LSSVR models are integrated to produce the overall prediction results by using the Bayesian inference and finite mixture mechanism, as shown in Eq (19).
p({S_g}|{{\bf{x}}_q}) = \frac{{{\pi _g}p({{\bf{x}}_q}|{\mathit{\Theta} _g})}}{{\sum\limits_{g = 1}^G {{\pi _g}p({{\bf{x}}_q}|{\mathit{\Theta} _g})} }} | (18) |
{y_p} = \sum\limits_{g = 1}^G {y_q^gp\left( {{S_g}\left| {{{\bf{x}}_q}} \right.} \right)} | (19) |
where
When GMM method is applied, the BIC technique is introduced to determine the number of Gaussian components in an intuitive and persuasive way, which can be formulated as
{\rm{BIC}} = - 2\log L({\bf{x}}\left| \mathit{\boldsymbol{ \boldsymbol{\varTheta} }} \right.) + dlog(N) | (20) |
where
Figure 2 illustrates the online prediction steps of test samples based on GMM-LSSVR method. The proposed soft sensor modeling strategy is shown in Figure 3.
Penicillin fermentation process is a typical microbial fermentation reaction and is often used to be a benchmark process for monitoring, controlling, and quality prediction. This process is a complex multivariable coupled biochemical procedure and often contains significant nonlinearity and time-varying behavior, which can be generally divided into three stages: growth, penicillin synthesis and autolysis stages [20]. Figure 4 shows the flow diagram of penicillin fermentation process. During the whole cultivation process, bacterial growth and antibiotic synthesis process are completed under suitable fermentation conditions such as temperature, pH, and oxygen concentration and so on. Considering the costs of offline chemical analysis and hardware sensors, designing a high-performance soft sensor plays an important role in real-time estimation of penicillin concentration.
A simulation platform named PenSim has been widely used to simulate fed-batch penicillin fermentation process under different operating conditions [20]. In this study, all process data samples for experiments are collected via running the PenSim platform. There are total 16 process variables in the simulation plant, and 11 highly related variables are selected as input variables, which are listed in Table 1. The typical trend plots of input and quality variables are depicted in Figure 5. The entire duration of each batch is set as 400 hours, while the sampling interval is set as 1 hour. Under the normal operating condition, a total of 4 training batches (named as Batches 1 to 4) are obtained for soft sensor model construction, while the additional 2 test batches (named as Batches 5 and 6) are collected for model performance evaluation.
NO. | Variable description (Unit) | NO. | Variable description (Unit) |
1 | Aeration rate (L/h) | 7 | Carbon dioxide concentration (g/L) |
2 | Agitator power (W) | 8 | PH (-) |
3 | Substrate feed rate (L/h) | 9 | Fermenter temperature (K) |
456 | Substrate feed temperature (K)Dissolved oxygen concentration (g/L)Culture volume (L) | 1011 | Generated heat (kcal)Cooling water flow rate (L/h) |
For model construction, 100 data samples are collected evenly from Batches 1 to 4, respectively. As a result, training dataset is composed of 400 samples, while additional 200 samples that collected evenly from Batch 5 compose the test dataset 1, and other 200 samples from Batch 6 compose the test dataset 2. Here, two test datasets are used for model evaluation: test dataset 1 in Batch 5 and test dataset 2 in Batch 6 with noisy condition. Suppose that the measure noise is the zero-mean Gaussian noise with variance of 0.01, the dataset 2 is used to study the behavior of the proposed soft sensor model under noisy measure environment. In order to show the sampling strategy more intuitively, for examples, we collect the aeration rate (one of the input variables) values every 4 hours in the training Batch 1. The sampling time plots of aeration rate are illustrated in Figure 6, where the red points represent the data samples selected for modeling. Figure 6a–d and e–f gives the sampling time of aeration rate in the training batches and test batches, respectively. The sampling time plots of other input variables are like that of aeration rate.
Then, MPCA is applied to data preprocessing. Firstly, two-dimensional modeling datasets can be obtained from original multidimensional datasets by variable-wise data unfolding method. Then, PCA, as a well-known technique in statistics and machine learning, is used to compress the input variables, and extract the most important information of the process. The relationship between principal components number and cumulative contribution rate for input dataset is illustrated in Figure 7. In this study, the principal component number can be set as 7 because the corresponding cumulative contribution rate achieves 0.98. As a result, the dimensionally reduced data is obtained and imported into soft sensor models for training.
The BIC value is calculated according to the obtained data matrix to determine the optimal number of Gaussian components. The relationship between the number of Gaussian components and BIC values is shown in Figure 8. When the number of Gaussian components is small, BIC values decrease dramatically. As the number increases, which changes from 3 to 6, BIC values change smoothly. In order to simplify model structure as much as possible and prevent the model from overfitting, the optimal number of Gaussian components is set as 3.
Four soft sensor models have been constructed in the following study:
(ⅰ) GPR: A global GPR model constructed from the preprocessed dataset.
(ⅱ) LSSVR: A global LSSVR model constructed from the preprocessed dataset.
(ⅲ) GMM-GPR: An ensemble model based on several local GPR models constructed from local preprocessed datasets that are obtained by using GMM method.
(ⅳ) GMM-LSSVR: An ensemble model based on several local LSSVR models constructed from local preprocessed datasets that are obtained by using GMM method.
To verify the prediction capabilities of the soft sensors with penicillin concentration, three performance indices including root-mean-square error (RMSE), tracking precision (TP) and coefficient of determination (R2) are used, which are defined as follows:
{\rm{RMSE}} = \sqrt {\frac{1}{N}\sum\limits_{i = 1}^N {{{\left( {{y_i} - {{\widehat y}_i}} \right)}^2}} } | (21) |
{\rm{TP}} = 1 - \frac{{\sigma _{error}^2}}{{\sigma _{true}^2}} | (22) |
{{\rm{R}}^{\rm{2}}} = 1 - \frac{{\sum\limits_{i = 1}^N {{{\left( {{{\widehat y}_i} - {y_i}} \right)}^2}} }}{{\sum\limits_{i = 1}^N {{{\left( {{y_i} - {{\overline y }_i}} \right)}^2}} }} | (23) |
where
Table 2 shows the quantitative comparison of the performance indicators for different four soft sensors. The comparison of global modeling and local learning methods shows that ensemble GPR model and ensemble LSSVR model perform better than global GPR and global LSSVR, respectively, because the RMSE value of the former is smaller than that of the latter. Clearly, GMM based multiple models can accurately and effectively describe the multiphase characteristics of batch process and enhance the ability of model interpretation. Therefore, for penicillin fermentation process, multi-model modeling has higher estimation accuracy and smaller prediction error. Similarly, by comparing GMM-GPR model with GMM-LSSVR model, it can be found that the ensemble LSSVR model based soft sensor has higher prediction accuracy and better tracking effect for penicillin concentration, whereas the ensemble GPR model based soft sensor has bigger RMSE values and smaller TP values. This result shows that, although the prediction performance of GMM- ensemble GPR model is improved compared with the global GPR model, poor predictions for test samples are still observed. As presented, the prediction performance of GMM-GPR model is far inferior to that of GMM-LSSVR model. Despite the presence of noise, as studied for dataset 2 in Batch 6 with noise, GMM-LSSVR based soft sensor still outperforms other different soft sensors. Three performance indicators can demonstrate the feasibility and superiority of the proposed method.
Method | Batch 5 with no noise | Batch 6 with noise | ||||
RMSE | TP | R2 | RMSE | TP | R2 | |
GPR | 0.0101 | 0.9996 | 0.9995 | 0.0206 | 0.9982 | 0.9980 |
LSSVR | 0.0119 | 0.9994 | 0.9993 | 0.0224 | 0.9981 | 0.9977 |
GMM-GPR | 0.0094 | 0.9996 | 0.9996 | 0.0177 | 0.9986 | 0.9985 |
GMM-LSSVR | 0.0039 | 0.9999 | 0.9999 | 0.0125 | 0.9993 | 0.9993 |
To present the regression performance of different soft sensors, the prediction results of penicillin concentration for global modeling and local learning methods is depicted in detail in Figures 9 and 10. As shown in Figure 9, the prediction curve of penicillin concentration by GMM-LSSVR model is more in line with the true value curve, thereby showing that the predicted value of penicillin concentration in this method is closer to the true value, and the prediction accuracy is also significantly higher than that of global LSSVR model. Furthermore, the prediction error of GMM-LSSVR model for test samples is reduced, and its generalization performance is better compared with that of GMM-GPR model. Similar analysis conclusions can be made according to the quality prediction results of Batch 6, which is given in Figure 10. This soft sensor modeling method can effectively improve the prediction capability and regression accuracy of global LSSVR model and can better complete the prediction of penicillin concentration.
To further illustrate the effectiveness of the proposed method, Figure 11 shows the comparison of the prediction errors of penicillin concentration for four soft sensors. It can be clearly seen that whether there is noise or not, the prediction error of the GMM-LSSVR model fluctuates less near 0, showing that the prediction results of the model are more consistent with the real results, and the tracking ability is stronger. Compared with different modeling methods, the GMM-LSSVR based soft sensor provides an accurate prediction of the true value of penicillin concentration and has good regression performance. In addition, the scatter plots of prediction results for penicillin concentration is presented in Figure 12. Compared with other scatters, the red asterisk scatters that correspond to GMM-LSSVR are more compactly distributed in the diagonal line, which shows that the proposed method can further improve the tracking performance and regression accuracy of the soft sensor. It can deliver reliable and accurate estimation of quality variable despite the presence of noise.
A smart soft sensor based on ensemble LSSVR models is proposed to deal with nonlinear, time-varying, and multiphase characteristics in batch processes. First, MPCA method is applied to be an effective tool for data unfolding and dimensionality reduction. Then, the new obtained dataset can be partitioned into several local regions, where local LSSVR models are constructed. Second, local LSSVR models are constructed for each operation period, respectively. Meanwhile, GS method and ten-fold cross-validation procedure are introduced to local model parameter determination. In this way, each local LSSVR model with a pair of optimal parameters can provide superior regression accuracy. Finally, an ensemble regression model is established by combining different local models by Bayesian fusion strategy and we can obtain the final prediction for test samples from ensemble LSSVR model online. Detailed analyses and comparative studies for penicillin fermentation process show that the proposed soft sensor is feasible and can deliver reliable and accurate quality prediction. In addition, we may be able to improve our future work for soft sensor development by applying cellular neural network approach.
This work was supported by the National Natural Science Foundation of China (Grant No.61773182), and the Subtopics of National Key Research and Development Program of China (Grant No.2018YFC1603705-03).
The authors declare no conflict of interest in this paper.
[1] | X. Wang, H. Liu, Soft sensor based on stacked auto-encoder deep neural network for air preheater rotor deformation prediction, Adv. Eng. Inf., 36 (2018), 112-119. |
[2] | W. Shao, X. Tian, Adaptive soft sensor for quality prediction of chemical processes based on selective ensemble of local partial least squares models, Chem. Eng. Res. Des., 95 (2015), 113-132. |
[3] | B. Bidar, J. Sadeghi, F, Shahraki, M. M. Khalilipour, Data-driven soft sensor approach for online quality prediction using state dependent parameter models, Chemom. Intell. Lab. Syst., 162 (2017), 130-141. |
[4] | J. Zheng, Z. Song, Semisupervised learning for probabilistic partial least squares regression model and soft sensor application, J. Process Control, 64 (2018), 123-131. |
[5] | D. Wang, J. Liu, R. Srinivasan, Data-Driven soft sensor approach for quality prediction in a refining process, IEEE Trans. Ind. Inf., 6 (2010), 11-17. |
[6] | H. Kaneko, M. Arakawa, K. Funatsu, Development of a new soft sensor method using independent component analysis and partial least squares, AIChE J., 55 (2010), 87-98. |
[7] | M. Zounemat-Kermani, D. Stephan, R. Hinkelmann, Multivariate NARX neural network in prediction gaseous emissions within the influent chamber of wastewater treatment plants, Atmos. Pollut. Res., 10 (2019), 1812-1822. |
[8] | R. Sharmin, U. Sundararaj, S. Shah, L.V. Griend, Y. J. Sun, Inferential sensors for estimation of polymer quality parameters: Industrial application of a PLS-based soft sensor for a LDPE plant, Chem. Eng. Sci., 61 (2006), 6372-6384. |
[9] | M. J. Willis, G. A. Montague, C. Di. Massimo, M. T. Tham, A. J. Morris, Artificial neural networks in process estimation and control, Automatica, 28 (1992), 1181-1187. |
[10] | C. Cortes, V. Vapnik, Support Vector Network, Mach. Learn., 20 (1995), 273-297. |
[11] | J. Tan, S. Li, Z. Zhang, C. X. Chen, W. Chen, H. Tang, et al., Identification of hormone binding proteins based on machine learning methods, Math. Biosci. Eng., 16 (2019), 2466-2480. |
[12] | W. Xiong, W. Zhang, B. Xu, B. Huang, JITL based MWGPR soft sensor for multi-mode process with dual-updating strategy, Comput. Chem. Eng., 90 (2016), 260-267. |
[13] | P. Facco, F. Bezzo, M. Barolo, Nearest-neighbor method for the automatic maintenance of multivariate statistical soft sensors in batch processing, Ind. Eng. Chem. Res., 49 (2010), 2336-2347. |
[14] | V. Ferreira, F. A. A. Souza, R. Araujo, Semi-supervised soft sensor and feature ranking based on co-regularised least squares regression applied to a polymerization batch process, IEEE Int. Conf. Ind. Inf., 2017, 257-262. |
[15] | P. Nomikos, J. F. Macgregor, Monitoring batch processes using multi-way principal component analysis, AIChE J., 40 (2010), 1361-1375. |
[16] | Z. Lv, Q. Jiang, X. Yan, Batch process monitoring based on multisubspace multiway principal component analysis and time-series Bayesian inference, Ind. Eng. Chem. Res., 53 (2014), 6457-6466. |
[17] | L. P. L. De Oliveira, D. Marcondes Filho, Monitoring batch processes with an incomplete set of variables, Int. J. Adv. Manuf. Technol., 94 (2018), 2515-2523. |
[18] | C. Flavio, H. M. M. Van, Fast multiway partial least squares regression, IEEE Trans. Biomed. Eng., 66 (2019), 433-443. |
[19] | X. Chen, X. Gao, Y. Zhang, Y. Qi, Enhanced batch process monitoring and quality prediction based on multi-phase multi-way partial least squares, IEEE International Conference on Intelligent Computing and Intelligent Systems, 2010. Available from: https://ieeexplore_ieee.xilesou.top/abstract/document/5658834. |
[20] | H. Jin, X. Chen, L. Wang, K. Yang, L. Wu, Adaptive soft sensor development based on online ensemble Gaussian process regression for nonlinear time-varying batch processes, Ind. Eng. Chem. Res., 54 (2015), 7320-7345. |
[21] | M. Sun, H. Yang, Gaussian process ensemble soft-sensor modeling based on improved Bagging algorithm, CIESC J., 67 (2016), 1386-1391. |
[22] | C. Wang, X. Bai, Boosting learning algorithm for stock price forecasting, IOP Conf. Ser.: Mater. Sci. Eng., 322 (2018). |
[23] | S. Tasnim, A. Rahman, A. M. T. Oo, M. E. Haque, Wind power prediction using cluster based ensemble regression, Int. J. Comput. Intell. Appl., 16 (2017), 1750026. |
[24] | L. A. Gabralla, A. Abraham, Prediction of oil prices using bagging and random subspace, Proceedings of the Fifth International Conference on Innovations in Bio-Inspired Computing and Applications IBICA 2014, 2014. Available from: https://link_springer.xilesou.top/. |
[25] | Y. Sun, Y. He, X. Ji, Soft sensor modeling of the penicillin fermentation based on FCM clustering and LS-SVM, 2010 International Conference on Computer Application & System Modeling, 2010. Available from: https://ieeexplore_ieee.xilesou.top/abstract/document/5622912. |
[26] | L. Wang, H. Jin, X. Chen, J. Dai, K. Yang, D. Zhang, Soft Sensor Development based on the hierarchical ensemble of Gaussian process regression models for nonlinear and non-Gaussian chemical processes, Ind. Eng. Chem. Res., 55 (2016), 7704-7719. |
[27] | L. Yao, Z. Ge, Online updating soft sensor modeling and industrial application based on selectively integrated moving window approach, IEEE Trans. Instrum. Meas., 66 (2017), 1985-1993. |
[28] | H. Kaneko, K. Funatsu, Adaptive soft sensor based on online support vector regression and Bayesian ensemble learning for various states in chemical plants, Chemom. Intell. Lab. Syst., 137 (2014), 57-66. |
[29] | Y. Liu, J. Chen, Integrated soft sensor using just-in-time support vector regression and probabilistic analysis for quality prediction of multi-grade processes, J. Process Control, 23 (2013), 793-804. |
[30] | S. Xu, X. An, X. Qiao, L. Zhu, L. Li, Multi-output least-squares support vector regression machines, Pattern Recognit. Lett., 34 (2013), 1078-1084. |
[31] | A. P. Verbyla, A note on model selection using information criteria for general linear models estimated using REML, Aust. N. Z. J. Stat., 61 (2019), 39-50. |
[32] | N. Pillai, S. L. Schwartz, T. Ho, A. Dokoumetzidis, R. Bies, I. Freedman, Estimating parameters of nonlinear dynamic systems in pharmacology using chaos synchronization and grid search, J. Pharmacokinet. Pharmacodyn., 46 (2019), 193-210. |
[33] | V. Vakharia, R. Gujar, Prediction of compressive strength and portland cement composition using cross-validation and feature ranking techniques, Constr. Build. Mater., 225 (2019), 292-301. |
[34] | P. Arena; S. Baglio, L. Fortuna, G. Manganaro, Self-organization in a two-layer CNN, IEEE Trans. Circuits Syst. I Fundam. Theory Appl., 45 (1998), 157-162. |
[35] | M. Wang, H. Chen, H. Li, Z. Cai, X. Zhao, C. Tong, et al., Grey wolf optimization evolving kernel extreme learning machine: application to bankruptcy prediction, Eng. Appl. Artif. Intell., 63 (2017), 54-68. |
[36] | S. W. Lin, K. C. Ying, S. C. Chen, Z. J. Lee, Particle swarm optimization for parameter determination and feature selection of support vector machines, Expert Syst. Appl., 35 (2018), 1817-1824. |
[37] | C. Wu, F. Yang, Y. Wu, R. Han, Prediction of crime tendency of high-risk personnel using C5.0 decision tree empowered by particle swarm optimization, Math. Biosci. Eng., 16 (2019), 4135-4150. |
[38] | X. Kong, X. Che, R. Su, C. Zhang, Q. Yao, X. Shi, A new technique for rapid assessment of eutrophication status of coastal waters using a support vector machine, Chin. J. Oceanol. Limnol., 36 (2018), 1-14. |
1. | Mariano M. Perdomo, Carlos I. Sanseverinatti, Luis A. Clementi, Jorge R. Vega, 2022, Sensor Inferencial Multi-modelo Aplicado a un Proceso Simulado para la Producción Continua de Látex para Caucho, 978-1-6654-8014-7, 1, 10.1109/ARGENCON55245.2022.9939849 | |
2. | Yan-Ning Sun, Wei Qin, Hong-Wei Xu, Run-Zhi Tan, Zhan-Luo Zhang, Wen-Tian Shi, A multiphase information fusion strategy for data-driven quality prediction of industrial batch processes, 2022, 608, 00200255, 81, 10.1016/j.ins.2022.06.057 | |
3. | Nobuhito Yamada, Hiromasa Kaneko, Adaptive soft sensor ensemble for selecting both process variables and dynamics for multiple process states, 2021, 219, 01697439, 104443, 10.1016/j.chemolab.2021.104443 | |
4. | Wangwang Zhu, Zhengjiang Zhang, Yi Liu, Dynamic Data Reconciliation for Improving the Prediction Performance of the Data-Driven Model on Distributed Product Outputs, 2022, 61, 0888-5885, 18780, 10.1021/acs.iecr.2c02536 | |
5. | Hongyu Tang, Zhenli Yang, Feng Xu, Qi Wang, Bo Wang, Soft Sensor Modeling Method Based on Improved KH-RBF Neural Network Bacteria Concentration in Marine Alkaline Protease Fermentation Process, 2022, 194, 0273-2289, 4530, 10.1007/s12010-022-03934-4 | |
6. | Joyce Chen Yen Ngu, Wan Sieng Yeo, 2022, Prediction Of Dissolved Oxygen Using Least Square Support Vector Regression Model, 978-1-6654-8663-7, 70, 10.1109/GECOST55694.2022.10010638 | |
7. | Feng Xu, Kaihao Hu, Ali Mohsin, Jie Wu, Lihuan Su, Yuan Wang, Rong Ben, Hao Gao, Xiwei Tian, Ju Chu, Recent advances in the biosynthesis and production optimization of gentamicin: A critical review, 2024, 2405805X, 10.1016/j.synbio.2024.11.003 | |
8. | Ling Zhao, Jinlin Zhu, Zheng Zhang, Zhenping Xie, Furong Gao, 2023, A Novel Semi-supervised Two-dimensional Dynamic Soft Sensor for Quality Prediction in Batch Processes, 979-8-3503-2529-4, 1, 10.1109/IAI59504.2023.10327600 | |
9. | Jameson Malang, Wan Sieng Yeo, Zhen Yang Chua, Jobrun Nandong, Agus Saptoro, A. Saptoro, R. Nagarajan, J.S.Y. Lau, Y.Y. Tiong, V. Rowtho, C.P. Selvan, A. Tan, C. Koh, A comparison study between different kernel functions in the least square support vector regression model for penicillin fermentation process, 2023, 377, 2261-236X, 01025, 10.1051/matecconf/202337701025 | |
10. | Wenlong Li, Xi Wang, Houliu Chen, Xu Yan, Haibin Qu, In-Line Vis-NIR Spectral Analysis for the Column Chromatographic Processes of the Ginkgo biloba L. Leaves. Part II: Batch-to-Batch Consistency Evaluation of the Elution Process, 2022, 9, 2297-8739, 378, 10.3390/separations9110378 | |
11. | Wei Zou, Yanxia Shen, Lei Wang, Design of robust fuzzy iterative learning control for nonlinear batch processes, 2023, 20, 1551-0018, 20274, 10.3934/mbe.2023897 | |
12. | Na Lu, Bo Wang, Xianglin Zhu, Soft Sensor Modeling Method for the Marine Lysozyme Fermentation Process Based on ISOA-GPR Weighted Ensemble Learning, 2023, 23, 1424-8220, 9119, 10.3390/s23229119 | |
13. | Xinmin Zhang, Bocun He, Hongyu Zhu, Zhihuan Song, Information Complementary Fusion Stacked Autoencoders for Soft Sensor Applications in Multimode Industrial Processes, 2024, 20, 1551-3203, 106, 10.1109/TII.2023.3257307 | |
14. | Yi Shan Lee, Junghui Chen, A novel reinforced incomplete cyber-physics ensemble with error compensation learning for within-batch quality prediction, 2025, 65, 14740346, 103172, 10.1016/j.aei.2025.103172 |
NO. | Variable description (Unit) | NO. | Variable description (Unit) |
1 | Aeration rate (L/h) | 7 | Carbon dioxide concentration (g/L) |
2 | Agitator power (W) | 8 | PH (-) |
3 | Substrate feed rate (L/h) | 9 | Fermenter temperature (K) |
456 | Substrate feed temperature (K)Dissolved oxygen concentration (g/L)Culture volume (L) | 1011 | Generated heat (kcal)Cooling water flow rate (L/h) |
Method | Batch 5 with no noise | Batch 6 with noise | ||||
RMSE | TP | R2 | RMSE | TP | R2 | |
GPR | 0.0101 | 0.9996 | 0.9995 | 0.0206 | 0.9982 | 0.9980 |
LSSVR | 0.0119 | 0.9994 | 0.9993 | 0.0224 | 0.9981 | 0.9977 |
GMM-GPR | 0.0094 | 0.9996 | 0.9996 | 0.0177 | 0.9986 | 0.9985 |
GMM-LSSVR | 0.0039 | 0.9999 | 0.9999 | 0.0125 | 0.9993 | 0.9993 |
NO. | Variable description (Unit) | NO. | Variable description (Unit) |
1 | Aeration rate (L/h) | 7 | Carbon dioxide concentration (g/L) |
2 | Agitator power (W) | 8 | PH (-) |
3 | Substrate feed rate (L/h) | 9 | Fermenter temperature (K) |
456 | Substrate feed temperature (K)Dissolved oxygen concentration (g/L)Culture volume (L) | 1011 | Generated heat (kcal)Cooling water flow rate (L/h) |
Method | Batch 5 with no noise | Batch 6 with noise | ||||
RMSE | TP | R2 | RMSE | TP | R2 | |
GPR | 0.0101 | 0.9996 | 0.9995 | 0.0206 | 0.9982 | 0.9980 |
LSSVR | 0.0119 | 0.9994 | 0.9993 | 0.0224 | 0.9981 | 0.9977 |
GMM-GPR | 0.0094 | 0.9996 | 0.9996 | 0.0177 | 0.9986 | 0.9985 |
GMM-LSSVR | 0.0039 | 0.9999 | 0.9999 | 0.0125 | 0.9993 | 0.9993 |