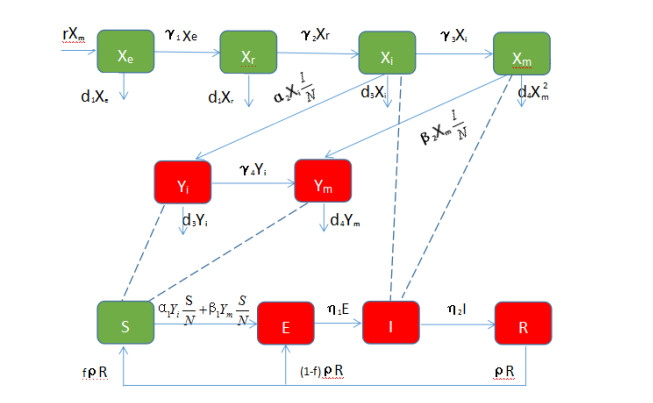
Citation: Yunbo Tu, Shujing Gao, Yujiang Liu, Di Chen, Yan Xu. Transmission dynamics and optimal control of stage-structured HLB model[J]. Mathematical Biosciences and Engineering, 2019, 16(5): 5180-5205. doi: 10.3934/mbe.2019259
[1] | Fumin Zhang, Zhipeng Qiu, Balian Zhong, Tao Feng, Aijun Huang . Modeling Citrus Huanglongbing transmission within an orchard and its optimal control. Mathematical Biosciences and Engineering, 2020, 17(3): 2048-2069. doi: 10.3934/mbe.2020109 |
[2] | Zhenzhen Liao, Shujing Gao, Shuixian Yan, Genjiao Zhou . Transmission dynamics and optimal control of a Huanglongbing model with time delay. Mathematical Biosciences and Engineering, 2021, 18(4): 4162-4192. doi: 10.3934/mbe.2021209 |
[3] | Chenwei Song, Rui Xu, Ning Bai, Xiaohong Tian, Jiazhe Lin . Global dynamics and optimal control of a cholera transmission model with vaccination strategy and multiple pathways. Mathematical Biosciences and Engineering, 2020, 17(4): 4210-4224. doi: 10.3934/mbe.2020233 |
[4] | Jia Li . Malaria model with stage-structured mosquitoes. Mathematical Biosciences and Engineering, 2011, 8(3): 753-768. doi: 10.3934/mbe.2011.8.753 |
[5] | Ning Yu, Xue Zhang . Discrete stage-structured tick population dynamical system with diapause and control. Mathematical Biosciences and Engineering, 2022, 19(12): 12981-13006. doi: 10.3934/mbe.2022606 |
[6] | Qiuyi Su, Jianhong Wu . Impact of variability of reproductive ageing and rate on childhood infectious disease prevention and control: insights from stage-structured population models. Mathematical Biosciences and Engineering, 2020, 17(6): 7671-7691. doi: 10.3934/mbe.2020390 |
[7] | Yujie Sheng, Jing-An Cui, Songbai Guo . The modeling and analysis of the COVID-19 pandemic with vaccination and isolation: a case study of Italy. Mathematical Biosciences and Engineering, 2023, 20(3): 5966-5992. doi: 10.3934/mbe.2023258 |
[8] | Tingting Xue, Long Zhang, Xiaolin Fan . Dynamic modeling and analysis of Hepatitis B epidemic with general incidence. Mathematical Biosciences and Engineering, 2023, 20(6): 10883-10908. doi: 10.3934/mbe.2023483 |
[9] | Pannathon Kreabkhontho, Watchara Teparos, Thitiya Theparod . Potential for eliminating COVID-19 in Thailand through third-dose vaccination: A modeling approach. Mathematical Biosciences and Engineering, 2024, 21(8): 6807-6828. doi: 10.3934/mbe.2024298 |
[10] | Alian Li-Martín, Ramón Reyes-Carreto, Cruz Vargas-De-León . Dynamics of a dengue disease transmission model with two-stage structure in the human population. Mathematical Biosciences and Engineering, 2023, 20(1): 955-974. doi: 10.3934/mbe.2023044 |
Huanglongbing (HLB), more commonly known as citrus greening disease, is one of the most dangerous and devastating diseases of citrus worldwide [1,2]. Two decades ago, it invaded the Western Hemisphere, primarily Florida and Brazil, where it has spread rapidly and caused major damage to global citrus production. It is estimated that citrus acreage in Florida has decreased by 40% and production by 49% since their historical peaks, all of which occurred in the last 20 years [3]. Up to 2015, 20% of Brazil's commercial citrus species has been infected with HLB, and the disease caused great damage to citrus industry by shortening tree lifespan and poor yield and quality [4]. In S˜ao Paulo, 64.1% of the commercial citrus blocks and 6.9% of the citrus trees were affected by HLB in 2012 [5]. In addition, Ganzhou is the top citrus producing area in Jiangxi Province, China, with an annual production of approximately 1.2 million tons [6,7]. However, the producing-area has also suffered from the widespread out of HLB and the output has significantly decreased in recent years [8]. The citrus acreage in Ganzhou had decreased from 2.48 million acres in 2013 to 1.00 million acres in 2018.
HLB is caused by phloem-restricted bacteria of the Candidatus Liberibacter group, which can be transmitted by two species of citrus psyllids, the Asian citrus psyllid (ACP), Diaphorina citri Kuwayama, and the African citrus psyllid, Trioza erytrae [3]. ACP is divided into eggs, 1st through 5th nymphal instars and adults [9]. The five nymphal instars of ACP can be differentiated by their distinct morphological characteristics [10]. Adults and nymphs are capable of acquiring the HLB pathogen after feeding on an infected plant for 30 min or longer [11]. Although the nymphs hardly move, they soon become Las-carrying (Candidatus Liberibacter asiaticus) adults with the ability to fly and transmit Las to other citrus plants. Thus the control period of vector psyllid should include the nymphal stages [10]. The results from [10] reported that psyllids can carry Las in either adult or nymphal stages, expect in the 1st and 2nd instars, the 3rd through 5th instars have stronger transmission ability than adults.
Generally a healthy citrus tree is inoculated by infected nymphs and adults, there is an incubation period in which the tree exhibits no symptoms but may act as a source of the disease [2]. The survey results from [12,13] indicated that the incubation period from grafting to development of HLB symptoms is 3 to 12 months under greenhouse conditions. Since incubation period is long and diagnosis is difficult, citrus trees can not be easily found in time after infection. This issue reduces the effectiveness of control strategy in which infected trees are removed to eliminate sources of HLB [14].
Recently, mathematical modeling has become an important and useful tool in understanding the epidemiology of vector-transmitted plant pathogens [15,16,17,18,19]. For citrus HLB, a few mathematical models currently exist which analyze how HLB spreads within individual trees [20], within a citrus grove [21,22,23,24,25], or from grove to grove [26]. In [21], the authors reviewed how mathematical models have yielded useful insights into controlling disease spread for vector-borne plant diseases, especially HLB. Note that, for citrus psyllid, different stages have different biological characters, such as reproductive potential, growth, temperature tolerance, transmission efficiency. Therefore, it is very necessary to consider the stage structure of psyllid in mathematical model, including eggs, the 1st and 2nd nymphal instars, the 3rd through 5th nymphal instars and adults. However, the stage structure of psyllid has not been discussed in the previous HLB models. Motivated by the preceding discussion, our first purpose of this paper is to formulate and investigate a HLB model, in which the incubation period of citrus tree and the stage structure of psyllid are taken into consideration.
There is no good source of genetic resistance to HLB in the genus citrus, and the disease cannot be cured once the trees are infected [2,27]. Current programs for HLB have focused on nutrient solution injection to reduce the infection of the bacteria, removal of infected trees to reduce sources of the disease and insecticide spraying control of the psyllid vector and planting with HLB-free nursery stock [2]. The second aim of this paper is to achieve awareness about the most desirable technique for minimizing the transmission of HLB by using the optimal control theory.
In this paper, different from the previous simple classification of psyllid into susceptible and infected populations, we consider that citrus psyllid in different stages have different abilities and ways to transmit HLB. Based on the above facts, in the next section, we establish a stage-structured HLB model, and obtain the basic reproductive number R0 of the model. In Section 3, we obtain the equivalent threshold condition T0 of the basic reproductive number R0, and prove extinction of the disease when R0≤1 while persistence of the disease when R0>1. In Section 4, we apply the optimal control technique to minimize the population of infected citrus trees, dead trees and total number of vector population. Different control strategies should be used for the elimination of infection in the population of citrus trees. In Section 5, we use numerical simulation to demonstrate and support the theoretical results. In the last section, we give a brief conclusion.
In this subsection, Citrus HLB model where transmission is via vector psyllids is formulated. The notation used in the mathematical model includes four states for citrus tree population. S denotes susceptible trees (individuals who can be infected by disease) and R represents dead trees. Due to the latency delay we split the infected trees into a latent stage, E (individuals who infected and asymptomatic but no infectious) and an infectious stage, I (individuals who have the ability to transmit the disease to others). Let N(t) be the total numbers of citrus tree population at time t in a grove, that is, N(t)=S(t)+E(t)+I(t)+R(t). Based on the fact that 3rd through 5th nymphal instars can transmit HLB, the citrus psyllid population is divided into six state variables. We let Xe represent eggs (produced by susceptible adults of psyllids), Xr denote 1st and 2nd nymphal instars (individuals who do not have the ability to transmit the disease to other susceptible trees), Xi and Yi denote 3rd through 5th susceptible and infected nymphal instars, respectively. Xm and Ym denote susceptible and infected adults of psyllid, respectively. We assume that the grove is subject to roguing and replanting management strategy. Moreover, we ignore the natural mortality rate of the citrus tree. The model flow diagram is depicted in Figure 1. Considering HLB transmission between citrus trees and psyllids, we establish the following HLB model.
dSdt=fρR−α1YiSN−β1YmSN,dEdt=α1YiSN+β1YmSN−η1E+(1−f)ρR,dIdt=η1E−η2I,dRdt=η2I−ρR,dXedt=rXm−γ1Xe−d1Xe,dXrdt=γ1Xe−γ2Xr−d2Xr,dXidt=γ2Xr−α2XiIN−γ3Xi−d3Xi,dXmdt=γ3Xi−β2XmIN−d4X2m,dYidt=α2XiIN−γ4Yi−d3Yi,dYmdt=γ4Yi+β2XmIN−d4Ym. | (1) |
In model (1), dead trees are rogued at a rate ρ and the corresponding spots are replanted with new trees. We assume that a proportion f∈[0,1] of the newly planted trees will be healthy and a proportion 1−f will become infected to a latent stage immediately. We also assume η1,γ1,γ3 and γ4 are the conversion rates, η2 is the disease-induced mortality rate of tree, r denotes oviposition rates of susceptible adult psyllid, d1 and d2 represent the natural mortality rates of eggs and 1st through2nd nymphal, respectively, d3 is the natural mortality rate of 3rd through 5th nymphal, d4 is the natural mortality rate of adult psyllid. Let α1 be the infection rate from 3rd through 5th infected nymphals to susceptible trees, α2 be the infection rate from infected trees to 3rd through 5th susceptible nymphals, β1 be the infection rate from infected adult psyllid to susceptible trees, and β2 be the infection rate from infected trees to susceptible adult psyllids.
Subject to the restriction dN(t)dt=dS(t)dt+dE(t)dt+dI(t)dt+dR(t)dt≡0, without loss of generality, let N(t)=1, and now S+E+I+R=1. Here S, E, I and R are defined separately as susceptibility rate, latent rate, infection rate and removal rate, respectively. Thus, system (1) can be reduced to the form:
dSdt=fρR−α1YiS−β1YmS,dEdt=α1YiS+β1YmS−η1E+(1−f)ρR,dIdt=η1E−η2I,dRdt=η2I−ρR,dXedt=rXm−γ1Xe−d1Xe,dXrdt=γ1Xe−γ2Xr−d2Xr,dXidt=γ2Xr−α2XiI−γ3Xi−d3Xi,dXmdt=γ3Xi−β2XmI−d4X2m,dYidt=α2XiI−γ4Yi−d3Yi,dYmdt=γ4Yi+β2XmI−d4Ym. | (2) |
From biological considerations, we study (2) in the closed set Γ={(S,E,I,R,Xe,Xr,Xi,Xm,Yi,Ym)∈R10|S,E,I,R≥0,S+E+I+R=1,Xe,Xr,Xi,Xm,Yi,Ym≥0}, which is invariant set under nonnegative initial conditions. It is easy to proof the boundedness of the solutions of system (2). We omit it.
The system (2) always exists a disease-free equilibrium (DFE) P0=(S0,0,0,0,X0e,X0r,X0i,X0m,0,0), where
S0=1,X0e=rγ1+d1X0m,X0r=rγ1(γ1+d1)(γ2+d2)X0m, |
X0i=rγ1γ2(γ1+d1)(γ2+d2)(γ3+d3)X0m,X0m=rγ1γ2γ3(γ1+d1)(γ2+d2)(γ3+d3)d4. |
The basic reproductive number R0 of an infectious disease is a fundamental concept in the study of disease transmissions. It represents the average number of secondary cases produced, in a completely susceptible population, by a typical infective individual [28]. If R0<1, then on average an infected individual produces less than one new infected individual over the course of its infectious period, and the infection cannot grow. Conversely, if R0>1, then each infected individual produces, on average, more than one new infection, and the disease can invade the population [29]. Diekmann et al. [29] define R0 as the spectral radius of the next generation matrix. That is, we rewrite the vector field of (2) as
dxidt=Fi(x)−Vi(x),i=1,2,...,10. |
and Vi(x)=V−i(x)−V+i(x), where x=(E,I,R,Yi,Ym,S,Xe,Xr,Xi,Xm),
F(x)=(α1YiS+β1YmS+(1−f)ρR00α2XiIβ2XmI00000)andV(x)=(η1Eη2I−η1EρR−η2Iγ4Yi+d3Yid4Ym−γ4Yiα1YiS+β1YmS−fρRγ1Xe+d1Xe−rXmγ2Xr+d2Xr−γ1Xeα2XiI+γ3Xi+d3Xi−γ2Xrβ2XmI+d4X2m−γ3Xi). |
F,V are defined as
F=(∂Fi∂xj(ˆP0))1≤i,j≤5, V=(∂Vi∂xj(ˆP0))1≤i,j≤5, |
where ˆP0=(0,0,0,0,0,S0,X0e,X0r,X0i,X0m). Thus
F=(00(1−f)ρα1β100000000000α2X0i0000β2X0m000)andV=(η10000−η1η20000−η2ρ00000γ4+d30000−γ4d4). |
Obviously, −V is cooperative. By simple computation, we get
FV−1=(1−f1−f1−fα1γ4+d3+β1γ4(γ4+d3)d4β1d40000000000α2X0iη2α2X0iη2000β2X0mη2β2X0mη2000). |
The eigenvalues of FV−1 are determined by
λ3[λ2−(1−f)λ−(α1γ4+d3+β1γ4(γ4+d3)d4)α2X0iη2−β2X0mη2β1d4]=0. | (3) |
It is easy to obtain that the spectral radius of FV−1 is:
ρ(FV−1)=R0=1−f2+√(1−f)24+R1+R2 , |
where
R1=(α1γ4+d3+β1γ4(γ4+d3)d4)α2η2X0iand R2=β1β2η2d4X0m. | (4) |
In order to give a more reasonable biological interpretation, we give an equivalent threshold quantity as
T0=R1+R2+1−f, |
where R1 and R2 are defined in (4). The biological meaning of quantity T0 can be explained as follows. Suppose that a single infected tree in stage E is introduced into a completely susceptible grove. The average number of secondary infections resulting from the psyllid in stage Xi (that is susceptible 3rd through 5th nymph) contact infected tree is,
R1=(α1γ4+d3+β1γ4(γ4+d3)d4)α2η2X0i. |
Further, the average number of secondary infections resulting from the psyllid in stage Xm (that is susceptible adult psyllid) contact infected tree is,
R2=β1β2η2d4X0m. |
The citrus tree will necessarily be removed in the R stage and will on average produce 1−f newly infected E trees. Thus the expected number of secondary infections is exactly T0. In the following, we will prove the equivalence of T0 and R0 for the local stability of the DFE P0.
Theorem 3.1. (ⅰ) R0<1 if and only if T0<1.
(ⅱ) T0<1 if and only if all eigenvalues of the Jacobian matrix of system (2) evaluated at DFE P0 have negative real parts.
Proof. It follows from (3) that the basic reproductive number R0 is the largest positive root of
ρ(λ)=λ2−(1−f)λ−(α1γ4+d3+β1γ4(γ4+d3)d4)α2X0iη2−β2X0mη2β1d4=λ2−(1−f)λ−R1−R2=0. |
Clearly, the leading coefficient of ρ(λ) is positive, and thus R0<1 if and only if
ρ(1)=f−R1−R2>0. |
Therefore, ρ(1)>0 is equivalent to T0<1 and the first assertion holds.
Next, we want to prove the second assertion. Calculating the Jacobian matrix of system (2) at the DFE P0:
J(P0)=(η10(1−f)ρα1β100000η1η2000000000η2ρ00000000α2X0i0γ4+d30000000β2X0m0γ4d40000000fρ−α1−β100000000000γ1+d100r000000γ1γ2+d2000−α2X0i00000γ2γ3+d300−β2X0m000000γ32d4X0m). |
Clearly, J(P0) can be seen as a block matrix of 2×2 and each block is a 5×5 matrix. The eigenvalues are determined by the following characteristic equation of J(P0):
p(λ)=λ(λ+γ1+d1)(λ+γ2+d2)(λ+γ3+d3)(λ+γ4+d3)(λ+d4)(λ+2d4X0m)×[(λ+η1)(λ+η2)(λ+ρ)−η1η2(1−f)ρ]−2η1rγ1γ2γ3λ(λ+ρ)×[β1γ4α2X0i−α1α2X0i(λ+d4)−β1β2X0m(λ+d4)(λ+γ4+d3)]=0. |
Any root λ of p(λ) with Re(λ)≥0 is also a root of q(λ) defined by
q(λ)=p(λ)λ(λ+η1)(λ+η2)(λ+ρ)(λ+γ1+d1)(λ+γ2+d2)(λ+γ3+d3)(λ+γ4+d3)(λ+d4)(λ+2d4X0m)=1−2η1β1rγ1γ2γ3γ4α2X0i(λ+η1)(λ+η2)(λ+γ1+d1)(λ+γ2+d2)(λ+γ3+d3)(λ+γ4+d3)(λ+d4)(λ+2d4X0m)−2η1α1rγ1γ2γ3α2X0i(λ+η1)(λ+η2)(λ+γ1+d1)(λ+γ2+d2)(λ+γ3+d3)(λ+γ4+d3)(λ+2d4X0m)−2η1β1rγ1γ2γ3β2X0m(λ+η1)(λ+η2)(λ+γ1+d1)(λ+γ2+d2)(λ+γ3+d3)(λ+2d4X0m)−η1η2(1−f)ρ(λ+η1)(λ+η2)(λ+ρ). |
Obviously, q(λ) is monotone increasing in λ when λ>0. It follows from that, since the leading coefficient of p(λ) is positive, we know that p(λ) has no positive real roots if and only if q(0)>0, which is equivalent to
(α1γ4+d3+β1γ4(γ4+d3)d4)α2η2X0i+β1β2η2d4X0m+1−f<1, |
that is, T0<1. Next, we need to prove all complex eigenvalues of J(P0) have negative real parts. Suppose the contrary. Then we define G(λ)=1−q(λ) and suppose p(λ)=0 with Re(λ)≥0 and Im(λ)≠0. Then G(λ)=1 where
G(λ)=2η1β1rγ1γ2γ3γ4α2X0i(λ+η1)(λ+η2)(λ+γ1+d1)(λ+γ2+d2)(λ+γ3+d3)(λ+γ4+d3)(λ+d4)(λ+2d4X0m)+2η1α1rγ1γ2γ3α2X0i(λ+η1)(λ+η2)(λ+γ1+d1)(λ+γ2+d2)(λ+γ3+d3)(λ+γ4+d3)(λ+2d4X0m)+2η1β1rγ1γ2γ3β2X0m(λ+η1)(λ+η2)(λ+γ1+d1)(λ+γ2+d2)(λ+γ3+d3)(λ+2d4X0m)+η1η2(1−f)ρ(λ+η1)(λ+η2)(λ+ρ). | (5) |
We claim |G(λ)|<G(Re(λ)). From (5), we have
|G(λ)|≤2η1β1rγ1γ2γ3γ4α2X0i|λ+η1||λ+η2||λ+γ1+d1||λ+γ2+d2||λ+γ3+d3||λ+γ4+d3||λ+d4||λ+2d4X0m|+2η1α1rγ1γ2γ3α2X0i|λ+η1||λ+η2||λ+γ1+d1||λ+γ2+d2||λ+γ3+d3||λ+γ4+d3||λ+2d4X0m|+2η1β1rγ1γ2γ3β2X0m|λ+η1||λ+η2||λ+γ1+d1||λ+γ2+d2||λ+γ3+d3||λ+2d4X0m)+η1η2(1−f)ρ|λ+η1||λ+η2||λ+ρ|<2η1β1rγ1γ2γ3γ4α2X0iΥ(Re(λ)+γ1+d1)(Re(λ)+γ2+d2)(Re(λ)+γ3+d3)(Re(λ)+γ4+d3)(Re(λ)+d4)(Re(λ)+2d4X0m)+2η1α1rγ1γ2γ3α2X0iΥ(Re(λ)+γ1+d1)(Re(λ)+γ2+d2)(Re(λ)+γ3+d3)(Re(λ)+γ4+d3)(Re(λ)+2d4X0m)+2η1β1rγ1γ2γ3β2X0mΥ(Re(λ)+γ1+d1)(Re(λ)+γ2+d2)(Re(λ)+γ3+d3)(Re(λ)+2d4X0m)+η1η2(1−f)ρΥ(Re(λ)+ρ)=G(Re(λ)), |
where Υ=(Re(λ)+η1)(Re(λ)+η2), and the second inequality is strict since Im(λ)≠0. Then we get T0<1⇔q(0)>0⇔G(0)<1, which implies G(Re(λ))<1 since G is decreasing in λ. Thus |G(λ)|<G(Re(λ))<1. This contradicts G(λ)=1.
Theorem 3.2. If R0<1, then the DFE P0 of system (2) is globally attractive.
Proof. Note that R0<1 implies that f>0. Suppose lim supt→∞E(t)=m>0. Then for every ε>0 there exists τ1>0, such that
E(t)≤m+ε,for all t≥τ1. | (6) |
It follows from the third equation of system (2) and (6) that
dI(t)dt≤η1(m+ε)−η2I2(t), |
for all t≥τ1. Then there exists τ2>τ1 such that
I(t)≤η1(m+ε)η2+ε, | (7) |
for all t≥τ2. It follows from the fourth equation of system (2) and (7) that
dR(t)dt≤η2(η1(m+ε)η2+ε)−ρR(t). |
for all t≥τ2. Thus there exists τ3>τ2 such that
R(t)≤η2ρ(η1(m+ε)η2+ε)+ε,for all t≥τ3. | (8) |
Further, from system (2), we have
dXedt=rXm−γ1Xe−d1Xe,dXrdt=γ1Xe−γ2Xr−d2Xr,dXidt≤γ2Xr−γ3Xi−d3Xi,dXmdt≤γ3Xi−d4X2m. | (9) |
Considering the auxiliary system of (9):
d˜Xedt=r˜Xm−γ1˜Xe−d1˜Xe,d˜Xrdt=γ1˜Xe−γ2˜Xr−d2˜Xr,d˜Xidt=γ2˜Xr−γ3˜Xi−d3˜Xi,d˜Xmdt=γ3˜Xi−d4˜X2m. | (10) |
Clearly, (10) is a quasi-monotone system, and the unique positive equilibrium (X0e,X0r,X0i,X0m) of system (10) is globally asymptotically stable if R0<1. By comparison theorem in differential equations, we obtain that there exists τ4>τ3 such that
Xe(t)≤X0e+ε,Xr(t)≤X0r+ε,Xi(t)≤X0i+ε,Xm(t)≤X0m+ε, | (11) |
for all t≥τ4. Substituting (7) and (11) into the ninth equation of system (2), we have that
dYi(t)dt≤α2(X0i+ε)(η1(m+ε)η2+ε)−(γ4+d3)Yi(t), |
for all t≥τ4. Then there exists τ5>τ4 such that
Yi(t)≤α2(X0i+ε)(η1(m+ε)η2+ε)γ4+d3+ε. | (12) |
for all t≥τ5. Substituting (7), (11) and (12) into the last equation of system (2), we get that
dYm(t)dt≤γ4(α2(X0i+ε)(η1(m+ε)η2+ε)γ4+d3+ε)+β2(X0m+ε)(η1(m+ε)η2+ε)−d4Ym(t) |
for all t≥τ5. Thus there exists τ6>τ5 such that
Ym(t)≤γ4(α2(X0i+ε)(η1(m+ε)η2+ε)γ4+d3+ε)+β2(X0m+ε)(η1(m+ε)η2+ε)d4+ε. | (13) |
for all t≥τ6. Now, substituting (8), (12) and (13) into the second equation of system (2), we get that for t≥τ6
dE(t)dt≤α1(α2(X0i+ε)(η1(m+ε)η2+ε)γ4+d3+ε)+β1(γ4(α2(X0i+ε)(η1(m+ε)η2+ε)γ4+d3+ε)+β2(X0m+ε)(η1(m+ε)η2+ε))d4+ε)−η1E(t)+(1−f)ρ(η2ρ(η1(m+ε)η2+ε)+ε). |
Then there exists τ7>τ6 such that
E(t)≤α1η1(α2(X0i+ε)(η1(m+ε)η2+ε)γ4+d3+ε)+β1η1(γ4(α2(X0i+ε)(η1(m+ε)η2+ε)γ4+d3+ε)+β2(X0m+ε)(η1(m+ε)η2+ε))d4+ε)+(1−f)ρη1(η2ρ(η1(m+ε)η2+ε)+ε). | (14) |
for all t≥τ7. Letting ε→0, the inequality (14) becomes
E(t)≤α1η1α2X0iη1mη2(γ4+d3)+β1η1(γ4α2X0iη1mη2(γ4+d3)d4+β2X0mη1mη2d4)+(1−f)η2η1η1mη2=(α1α2X0iη2(γ4+d3)+β1α2γ4X0iη2d4(γ4+d3)+β1β2X0mη2d4+1−f)m=T0m |
It follows from Theorem 3.1 that, R0<1 implies T0<1. Thus lim supt→∞E(t)<m, a contradiction. So m=0. Following (7), (8), (12)-(14) and the nonnegativity of the solutions, we have limt→∞E(t)=limt→∞I(t)=limt→∞R(t)=limt→∞Yi(t)=limt→∞Ym(t)=0. By the theory of asymptotically autonomous semiflows (see [30]), we have
limt→∞S(t)=S0, limt→∞Xe(t)=X0e, limt→∞Xr(t)=X0r, limt→∞Xi(t)=X0i, limt→∞Xm(t)=X0m. |
Therefore all nonnegative solutions converge to the DFE P0.
It follows from Theorem 3.1 that DFE is locally asymptotically stable as R0<1, while DFE is unstable as R0>1. Theorem 3.2 in subsection 3.2 illustrates the global stable result of DEF for the case R0<1.
Theorem 3.3. If R0>1, then the disease is uniformly persistent for system (2). That is, there is a positive constant ε0>0, such that
lim inft→∞E(t)>ε0, lim inft→∞I(t)>ε0, lim inft→∞R(t)>ε0, lim inft→∞Yi(t)>ε0, lim inft→∞Ym(t)>ε0. | (15) |
Proof. Denote ˜K={(S,E,I,R,Xe,Xr,Xi,Xm,Yi,Ym)∈R10+}, K0={(S,E,I,R,Xe,Xr,Xi,Xm,Yi,Ym)∈˜K:S≥0,E>0,I>0,R>0,Xe≥0,Xr≥0,Xi≥0,Xm≥0,Yi>0,Ym>0}, and ∂K0=˜K∖K0. Let u(t,t0,x0) be the unique solution of system (2) with the initial value x0=(S0,E0,I0,R0,Xe0,Xr0,Xi0,Xm0,Yi0,Ym0) at time t0.
Define poincaré map P:˜K→˜K associated with system (2) as follows:
P(x0)=u(t0+1,x0),∀x0∈˜K. |
Set
M∂={x0∈∂K0|Pm(x0)∈∂K0, ∀m∈Z+}. |
We claim that
M∂={(S,0,0,0,Xe,Xr,Xi,Xm,0,0)|S≥0,Xe≥0,Xr≥0,Xi≥0,Xm≥0}. |
Obviously, {(S,0,0,0,Xe,Xr,Xi,Xm,0,0)|S≥0,Xe≥0,Xr≥0,Xi≥0,Xm≥0}⊆M∂. Next, We want to show
M∂∖{(S,0,0,0,Xe,Xr,Xi,Xm,0,0)|S≥0,Xe≥0,Xr≥0,Xi≥0,Xm≥0}=∅. | (16) |
If (16) does not hold, then there exists a point (S0,E0,I0,R0,Xe0,Xr0,Xi0,Xm0,Yi0,Ym0)∈M∂∖{(S,0,0,0,Xe,Xr,Xi,Xm,0,0)|S≥0,Xe≥0,Xr≥0,Xi≥0,Xm≥0}. Next, for the five initial values E0,I0,R0,Yi0, and Ym0, we divided into four cases to discuss.
Case (i) One initial value is equal to zero, and the others are lager than zero. Without loss of generality, we choose E0=0,I0>0,R0>0,Yi0>0 and Ym0>0. It is obvious that S(t)>0,Yi(t)>0,Ym(t)>0 and R(t)>0 for any t>t0. Then from the second equation of system (2), we get dE(t)dt|t=t0=α1Yi(t0)S(t0)+β1Ym(t0)S(t0)+(1−f)ρR(t0)>0. Thus, (S,E,I,R,Xe,Xr,Xi,Xm,Yi,Ym)∉∂K0 for 0<t−t0≪1. This is a contradiction. The other subcases can be similarly proved.
Case (ii) Two initial values are equal to zero, and the others are larger than zero. Let E0=I0=0,R0>0,Yi0>0 and Ym0>0. It is obvious that S(t)>0,Yi(t)>0,Ym(t)>0, and R(t)>0 for any t>t0. From the second equation of system (2), we get dE(t)dt|t=t0=α1Yi(t0)S(t0)+β1Ym(t0)S(t0)+(1−f)ρR(t0)>0. So E(t)>0 for 0<t−t0≪1. This implies that I(t)>0 for 0<t−t0≪1. Therefore, we have (S,E,I,R,Xe,Xr,Xi,Xm,Yi,Ym)∉∂K0 for 0<t−t0≪1. This is a contradiction. Similarly, we can prove the other subcases.
Case (iii) Three initial values are equal to zero, and the others are larger than zero. Set E0=I0=R0=0,Yi0>0 and Ym0>0. Clearly, S(t)>0,Yi(t)>0 and Ym(t)>0, for any t>t0. It follows from the second equation of system (2) that dE(t)dt|t=t0=α1Yi(t0)S(t0)+β1Ym(t0)S(t0)>0. So E(t)>0 for 0<t−t0≪1. It follows from the third and fourth equations of system (2) that I(t)>0 and R(t)>0 for 0<t−t0≪1. Therefore, (S,E,I,R,Xe,Xr,Xi,Xm,Yi,Ym)∉∂K0 for 0<t−t0≪1. This is a contradiction. Similarly, we can prove the other subcases.
Case (iv) Four initial values are equal to zero, and the other is larger than zero. Set E0=I0=R0=Yi0=0 and Ym0>0. It is easy to see that S(t)>0 and Ym(t)>0 for all t>t0. From the second equation of system (2), we can get dE(t)dt|t=t0=α1Yi(t0)S(t0)>0, for 0<t−t0≪1. So E(t)>0 for 0<t−t0≪1. It follows from the third, fourth and ninth equations of system (2) that I(t)>0, R(t)>0 and Yi(t)>0 for 0<t−t0≪1. Thus, (S,E,I,R,Xe,Xr,Xi,Xm,Yi,Ym)∉∂K0 for 0<t−t0≪1. This is a contradiction. Similarly, we can prove the other subcases.
Thus
M∂={(S,0,0,0,Xe,Xr,Xi,Xm,0,0)|S≥0,Xe≥0,Xr≥0,Xi≥0,Xm≥0}. |
In the following, we proceed by contradiction to prove that there exists ξ>0 such that
lim supm→∞d(Pm(x0),P0)≥ξ,∀x0∈K0,m∈Z+. | (17) |
where P0=(S0,0,0,0,X0e,X0r,X0i,X0m,0,0).
It follows from Theorem 2 in [31] that R0>1⟺ρ(FV−1)>1⟺ρ(exp(F−V))>1. Therefore, if R0>1, we can choose ε1>0 sufficiently small such that
ρ(exp(F−V−Mε1))>1, | (18) |
where
Mε1=(000α1ε1β1ε100000000000α2ε10000β2ε1000). |
If (17) does not hold, then for any ζ>0, we have
lim supm→∞d(Pm(x0),P0)<ζ,for some x0∈K0. |
Without loss of generality, suppose that
d(Pm(x0),P0)<ζ,∀ζ>0,∀m∈Z+. |
By the continuity of the solution with respect to initial values, we have that there exists sufficiently small ε1>0 such that
‖u(t,Pm(x0))−u(t,P0)‖≤ε1,∀t∈[t0,t0+1],∀m∈Z+. | (19) |
For any t≥t0, there exists an integer l∈Z+ such that t−t0=l+ˆt, where ˆt∈[0,1). It follows from (19) that
‖u(t,Pm(x0))−u(t,P0)‖=‖u(t0+ˆt,Pm+l(x0))−u(t0+ˆt,P0)‖≤ε1. |
Therefore, we have
S(t)≥S0−ε1,Xi(t)≥X0i−ε1,Xm(t)≥X0m−ε1,for all t≥t0. | (20) |
From system (2) and inequality (20), we get
dE(t)dt≥α1Yi(S0−ε1)+β1Ym(S0−ε1)−η1E+(1−f)ρR,dI(t)dt=η1E−η2I,dR(t)dt=η2I−ρR,dYi(t)dt≥α2(X0i−ε1)I−γ4Yi−d3Yi,dYm(t)dt≥γ4Yi+β2(X0m−ε1)I−d4Ym. | (21) |
Obviously, system (21) is a quasi-monotonic system. Consider the following comparison system:
dˆZ(t)dt=(F−V−Mε1)ˆZ(t), | (22) |
where ˆZ(t)=(ˆE(t),ˆI(t),ˆR(t),^Yi(t),^Ym(t))T and
F−V−Mε1=(−η10(1−f)ρα1(S0−ε1)β1(S0−ε1)η1−η20000η2−ρ000α2(X0i−ε1)0−(γ4+d3)00β2(X0m−ε1)0γ4−d4) |
By [32], we know that there exists a positive vector v such that ˆZ(t)=vexp(ηt) is a solution of system (22), where η=lnρ(exp(F−V−Mε1)). From (18), we can get η>0 and thus ˆZ(t)→∞ as t→∞, that is, ˆE(t)→∞, ˆI(t)→∞, ˆR(t)→∞, ^Yi(t)→∞ and ^Ym(t)→∞ as t→∞. According to the comparison theorem in differential equations, we can easily obtain that
E(t)→∞, I(t)→∞, R(t)→∞, Yi(t)→∞, Ym(t)→∞, as t→∞ |
This contradicts with the boundedness of the solutions. Thus, we have proved that (17) holds and P is weakly uniformly persistent with respect to (K0,∂K0).
Obviously, the poincaré map P has a global attractor P0. P0 is an isolated invariant set in ˜K and WS(P0)⋂K0=∅, and it is acyclic in M∂. Every solution in M∂ converges to P0. According to Zhao [33], we derive that P is uniformly persistent with respect to (K0,∂K0). This implies that the solution of system (2) is uniformly persistent with respect to (K0,∂K0), that is, (15) holds.
Optimal control theory has been used to explore optimal control strategies for various infectious diseases [34,35,36]. The purpose of this section is to seek an optimal integrated strategy to prevent the spread of citrus HLB. In the following, we begin with the presentation of the optimal control problem for the transmission dynamics of HLB in order to derive nutrient solution injection, removal of infected trees and insecticide spraying strategies with minimal implementation cost. We will show that it is possible to implement control techniques while minimizing the cost of implementation of such measures.
In the host citrus trees population, the associated force of infections are reduced by factors of (1−u1), where u1 measures the precaution effort of nutrient solution injection. The control variable u2 represents the removing of infected trees. The control variable u3 shows the eradication effort of insecticide spraying. It follows that the reproduction rate of psyllid population (including egg, nymph, adult stages) is reduced by a factor of (1−u3). Based on the assumptions and extensions mentioned above, system (2) with control strategy can be improved as following forms:
dSdt=f(ρR+u2I)−α1(1−u1)YiS−β1(1−u1)YmS,dEdt=α1(1−u1)YiS+β1(1−u1)YmS−η1(1−u1)E+(1−f)(ρR+u2I),dIdt=η1(1−u1)E−η2I−u2I,dRdt=η2I−ρR,dXedt=r(1−u3)Xm−γ1Xe−d1Xe−r0u3Xe,dXrdt=γ1Xe−γ2Xr−d2Xr−r0u3Xr,dXidt=γ2Xr−α2XiI−γ3Xi−d3Xi−r0u3Xi,dXmdt=γ3Xi−β2XmI−d4X2m−r0u3Xm,dYidt=α2XiI−γ4Yi−d3Yi−r0u3Yi,dYmdt=γ4Yi+β2XmI−d4Ym−r0u3Ym, | (23) |
subject to nonnegative initial conditions, here r0 is a conversion rate.
For the optimal control problem of (23), we consider the control variables u(t)=(u1,u2,u3)∈U relative to the state variables S,E,I,R,Xe,Xr,Xi,Xm,Yi,Ym where control variables are bounded and measured with
U={(u1,u2,u3)|ui is Lebsegue measurable, 0≤ui(t)≤1,t∈[0,T],i=1,2,3}, | (24) |
where T represents the control period. Let V be the total number of psyllid population, that is, V=Xe+Xr+Xi+Xm+Yi+Ym. For the control problem, we now define the objective functional as
J(u1,u2,u3)=∫T0(A1I+A2R+A3V+B12u21+B22u22+B32u23)dt. | (25) |
subject to the control system (23). The objective is to minimize the cost functional (25). That is, the goal is minimizing the number of infected trees, dead trees and psyllid populations and the cost of implementing the control, by using possible minimal control variables ui(t) (i=1,2,3). We choose to model the control efforts via a linear combination of quadratic terms, u2i(t)(i=1,2,3). Further, the constants A1,A2,A3 and B1,B2,B3 represent a measure of the relative cost of the interventions over the interval [0,T]. In order to find an optimal control, u∗1,u∗2,u∗3 such that
J(u∗1,u∗2,u∗3)=minUJ(u1,u2,u3). | (26) |
where U is defined in (24) and subject to control system (23) with nonnegative initial conditions. Next, we use Pontryagin's Maximum Principle to solve this optimal control problem.
Following the idea of [37], we prove firstly the existence of the optimal control problem.
Theorem 4.1. For the objective functional J(u1,u2,u3)=∫T0(A1I+A2R+A3V+B12u21+B22u22+B32u23)dt, associated with model (23) defined in U, then there exists an optimal control u∗=(u∗1,u∗2,u∗3), such that J(u∗1,u∗2,u∗3)=minUJ(u1,u2,u3).
Proof. By Theorem Ⅲ.4.1 from [37], we only need to check the following assumptions:
(H1) The set of controls and corresponding state variables is nonempty.
(H2) The control set U is convex and closed.
(H3) Right hand side of each equation in control problem (23) is continuous, bound above by a sum of the bounded control and state, and can be written as a linear function of U with coefficients depending on time and the state.
(H4) There exist constants C1,C2>0 and β>1 such that the integrand of the objective functional L(y,u,t) is concave and satisfies
L(y,u,t)≥C1(|U1|2+|U2|2+|U3|2)β2−C2. |
Obviously, the state variables and the set of control are bounded and nonempty which confirm (H1). Note that the solutions are bounded, so the admissible control set is bounded and convex, which confirms (H2). The system is bilinear in control variables, so it confirms (H3) (since the solutions are bounded). The hypothesis (H4) can be verified as
A1I+A2R+A3V+12(B1u21+B2u22+B3u23)≥C1(|U1|2+|U2|2+|U3|2)β2−C2, |
where C1,C2>0,A1,A2,A3,B1,B2,B3>0,B4>0 and β>0. In view of the result given by Lukes [38], we have that there exists an optimal control strategy (u∗1,u∗2,u∗3) minimizing J(u1,u2,u3).
Next we explore the minimal value of J(u1,u2,u3). To accomplish this, we define the Lagrangian L and Hamiltonian H for the optimal control problem (23) as
L(I,R,V,u1,u2,u3)=A1I+A2R+A3V+12(B1u21+B2u22+B1u23), |
and
H(X,U,λ)=L(I,R,V,u1,u2,u3)+λ1[f(ρR+u2I)−α1(1−u1)YiS−β1(1−u1)YmS]+λ2[α1(1−u1)YiS+β1(1−u1)YmS−η1(1−u1)E+(1−f)(ρR+u2I)]+λ3[η1(1−u1)E−η2I−u2I]+λ4[η2I−ρR]+λ5[r(1−u3)Xm−γ1Xe−d1Xe−r0u3Xe]+λ6[γ1Xe−γ2Xr−d2Xr−r0u3Xr])+λ7[γ2Xr−α2XiI−γ3Xi−d3Xi−r0u3Xi]+λ8[γ3Xi−β2XmI−d4X2m−r0u3Xm]+λ9[α2XiI−γ4Yi−d3Yi−r0u3Yi]+λ10[γ4Yi+β2XmI−d4Ym−r0u3Ym], | (27) |
where X=(S,E,I,R,Xe,Xr,Xi,Xm,Yi,Ym), U=(u1,u2,u3) and λ=(λ1,λ2,λ3,...,λ10).
In this subsection, by using Pontryagin's Maximum Principle [39], we will obtain the optimal solution of the control system (23).
Let u∗1,u∗2 and u∗3 represent the optimal solution of the control problem (26), then there exists a nontrivial vector function λ(t)=(λ1(t),λ2(t),λ3(t),...,λ10(t)) satisfying three equalities:
(ⅰ) the state equation
dxdt=∂H(t,u∗1,u∗2,u∗3,λ(t))∂λ, |
(ⅱ) the optimality condition
0=∂H(t,u∗1,u∗2,u∗3,λ(t))∂u, |
(ⅲ) the adjoint equation
dλdt=−∂H(t,u∗1,u∗2,u∗3,λ(t))∂X. |
Now, we apply the necessary conditions to the Hamiltonian H given by (27). Following the results in [39], we can obtain the following conclusions.
Theorem 4.2. Let ˆy∗=(ˆS∗,ˆE∗,ˆI∗,ˆR∗,ˆX∗e,ˆX∗r,ˆX∗i,ˆX∗m,ˆY∗i,ˆY∗m) be an optimal solution associated with the optimal control strategy u∗(t)=(u∗1(t),u∗2(t),u∗3(t)) for the optimal control problem (26), then there exists adjoint variables λi,(i=1,2,...,10) satisfying
dλ1(t)dt=(λ1−λ2)[α1(1−u1)Yi+β1(1−u1)Ym]dλ2(t)dt=(λ2−λ3)η1(1−u1)dλ3(t)dt=−A1−fu2λ1−(1−f)u2λ2+(η2+u2)λ3−η2λ4+α2Xiλ7+β2Xmλ8−α2Xiλ9−β2Xmλ10dλ4(t)dt=−A2−fρλ1−(1−f)ρλ2+ρλ4dλ5(t)dt=−A3+(γ1+d1+γ0u3)λ5−γ1λ6dλ6(t)dt=−A3+(γ2+d2+γ0u3)λ6−γ2λ7dλ7(t)dt=−A3+(α2I+γ3+d3+γ0u3)λ7−γ3λ8−α2Iλ9dλ8(t)dt=−A3−r(1−u3)λ5+(β2I+2d4Xm+γ0u3)λ8−β2Iλ10dλ9(t)dt=−A3+(λ1−λ2)α1(1−u1)S+(γ4+d3+γ0u3)λ9−γ4λ10dλ10(t)dt=−A3+(λ1−λ2)β1(1−u1)S+(d4+γ0u3)λ10 |
with transversality conditions
λi(T)=0,i=1,2,...,10. |
Further, the control u∗1,u∗2,u∗3 are given by
u∗1=max{min{1,(λ2−λ1)(α1ˆY∗iˆS∗+β1ˆY∗mˆS∗)+(λ3−λ2)η1ˆE∗B1},0},u∗2=max{min{1,(λ2−λ1)fˆI∗+(λ3−λ2)ˆI∗B2},0},u∗3=max{min{1,rˆX∗mλ5+γ0ˆX∗eλ5+γ0ˆX∗rλ6+γ0ˆX∗iλ7+γ0ˆX∗mλ8+γ0ˆY∗iλ9+γ0ˆY∗mλ10B3},0}. | (28) |
Proof. To determine the adjoint equations and the transversality conditions we use the Hamiltonian (27). The adjoint system results from Pontryagin's Maximun Principle [39].
dλ1(t)dt=−∂H∂S,dλ2(t)dt=−∂H∂E,...,dλ10(t)dt=−∂H∂Ym, |
with λi(T)=0 (i=1,2,⋯,10).
To obtain the characterization of the optimal control given by (28), solving the equations
∂H∂u1=0,∂H∂u2=0,∂H∂u3=0, |
on the interior of the control set and applying the property of the control space U, we can derive (28) holds.
In this section, we use firstly the model (2) to simulate the data on the number of infected citrus trees of Yuan Orchard from June, 2015 to December, 2015. Yuan Orchard is located in Ganzhou, China, which is one of our monitoring sites for citrus HLB. The infected rates of trees I(t) are given in Table 1. Numerical simulation of I(t) is shown in Figure 2. In order to carry out the numerical simulations, we need to estimate the model parameters. We get these parameter values in three ways: some parameter values (η1,η2, r,γ1,γ2,γ3, d1,d2,d3,d4,r0) are obtained from the literature; some parameter values (ρ,f) are estimated; and other parameter values (α1,α2,β1,β2) are fitted by the MATLAB tool fminsearch, which is fitted by calculating the minimum sum of square (MSS) (see [40]):
MSS=11∑i=1(I(datai)−I(i))2. |
Date | 06/30 | 07/30 | 08/30 | 09/15 | 09/30 | 10/15 |
I(t) | 0.0318 | 0.053 | 0.1327 | 0.1946 | 0.215 | 0.2778 |
Date | 10/30 | 11/15 | 11/30 | 12/15 | 12/30 | |
I(t) | 0.354 | 0.354 | 0.3717 | 0.3716 | 0.407 |
By using the parameter values in Table 1, we can obtain α1=0.00494month−1,α2=0.00043month−1,β1=0.0097month−1 and β2=0.002258month−1 by fitting in simulations. All parameter values of the model are given in Table 2.
Parameter | Value | Unit | Reference | Parameter | Value | Unit | Reference |
η1 | 0.1667 | month−1 | [41] | d3 | 1.612 | month−1 | [9] |
η2 | 0.002258 | month−1 | [2] | d4 | 0.788 | month−1 | [9,42] |
ρ | 0.0791 | - | Estimation | f | 0.067 | month−1 | Estimation |
r | 62 | month | [9,42] | α1 | 0.00494 | month−1 | Fitting |
γ1 | 5.49625 | month−1 | [9] | α2 | 0.00043 | month−1 | Fitting |
γ2 | 5.376 | month−1 | [9] | β1 | 0.0097 | month−1 | Fitting |
γ3,γ4 | 2.188 | month−1 | [9] | β2 | 0.002258 | month−1 | Fitting |
d1 | 2.494 | month−1 | [9] | r0 | 6 | - | [43] |
d2 | 4.867 | month−1 | [9] |
Next, we numerically examine the effect of the optimal control strategy on the spread of citrus HLB in a population of trees and psyllids. In this simulation without control population is labeled with bold line and the control by a dashed line. The weight constant values in the objective functional are A1=800;A2=2000;A3=2;B1=200;B2=1;B3=50. The control u1, u2 and u3 are all used to optimize the objective function J. Figures 3 and 4 showed that the control strategy resulted in a decrease in the number of infected citrus trees I, dead citrus trees R, psyllids at each stage, Xe,Xr,Xi,Xm,Yi and Ym while an increase is observed in the number of susceptible citrus trees S. In Fig. 5, we can observe that the optimal control profile for u1, u2 and u3. Note that parameter values used in the numerical simulations are given in Table 1, and the initial conditions are taken as S(0)=0.6,E(0)=0.1,I(0)=0.2,R(0)=0.1, Xe(0)=100,Xr(0)=60,Xi(0)=60,Xm(0)=50,Yi(0)=30,Ym(0)=20.
In this paper, based on the mechanism and characteristics of citrus HLB transmission, we proposed a vector-borne plant disease model with stage structure in psyllids and studied the effect of intervention strategy in controlling the spread of HLB. We calculated the basic reproduction ratio R0 for the epidemic model, and showed that the disease would die out when R0<1, and the disease would be endemic when R0>1.
Moreover, by using the optimal control theory, we analyzed the intervention strategy, nutrient solution injection, removal of infected trees and insecticide spraying, to determine the optimal integrated strategy. Using the Pontryagin's Maximum Principle, we investigated the existence of the optimal control problem. In addition, we minimized the number of infected citrus trees, dead citrus trees and the total number of psyllid population, by using three control variables. Numerical simulations illustrated the effectiveness of the proposed control problem.
The research has been supported by the Natural Science Foundation of China (11561004) and the Key Science and Technology Program of Jiangxi Province (20143ACF60012).
The authors declare that there are no conflicts of interest regarding the publication of this paper.
[1] | D. Farnsworth, K. A. Grogan, A. H. C. V. Bruggen, et al., The potential economic cost and response to greening in Florida citrus, Agric. Appl. Econ. Assoc., 29 (2014): 1–6. |
[2] | J. M. Bove, Huanglongbing: A destructive, newly-emerging, century-old disease of citrus, J. Plant. Pathol., 88 (2006): 7–37. |
[3] | S. Alvarez, D. Solis and M. Thomas, Can Florida's citrus industry be saved while preserving the environment? An economic analysis for the bio-control of the Asian Citrus Psyllid, S. Agric. Econ. Assoc., 2015. |
[4] | H. Su, Research and health management of citrus Huanglongbing in Taiwan, In: Proc Intern Research Conference on Huanglongbing, Orlando, Florida, USA, (2008): 57–92. |
[5] | A. J. Ayres, J. B. Jr and J. M. Bové, The experience with Huanglongbing management in Brazil, Acta. Hortic., 1065 (2015): 55–62. |
[6] | W. Li, Y. N. Yao, L. Wu, et al., Detection and seasonal variations of Huanglongbing disease in navel orange trees using direct lonization mass spectrometry, J. Agric. Food. Chem. , 67 (2019): 2265–2271. |
[7] | C. Yang, H. Chen, H. Chen, et al., Antioxidant and anticancer activities of essential oil from Gannan navel orange peel, Molecules, 22 (2017): 1931–1400. |
[8] | G. Rao, L. Huang, M. H. Liu, et al., Identification of Huanglongbing-infected navel oranges based on laser-induced breakdown spectroscopy combined with different Chemometric methods, Appl. Optics., 57 (2018): 8738–8742. |
[9] | Y. H. Liu and J. H. Tsai, Effects of temperature on biology and life table parameters of the Asian citrus psyllid, Diaphorina Citri Kuwayama (Homoptera: Psyllidae), Ann. Appl. Biol., 137 (2000): 201–206. |
[10] | T. H. Hung, S. C. Hung, C. N. Chen, et al., Detection by PCR of Candidatus Liberibacter asiaticus,the bacterium causing citrus huanglongbing in vector psyllids: application to the study of vector pathogen relationships, Plant Pathol., 53 (2004): 96–102. |
[11] | F. Wu, Y. J. Cen, X. L. Deng, et al., Movement of Diaphorina citri(Hemiptera: Liviidae) adults between huanglongbing infected and healthy citrus, Flor. Entomol., 98 (2015): 410–416. |
[12] | W. Shen, S. E. Halbert, E. Dickstein, et al., Occurrence and ingrove distribution of citrus huang- longbing in north central Florida, J. Plant. Pathol., 95 (2003): 361–371. |
[13] | K. L. Manjunath, S. E. Halbert, C. Ramadugu, et al., Detection of Candidatus Liberibacter asiaticus in Diaphorina citri and its importance in the management of citrus huanglongbing in Florida, Phytopathology, 98 (2008): 387–396. |
[14] | E. E. G. Cardwell, L. L. Stelinski and P. A. Stansly, Biology and man agement of Asian Citrus Psyllid,vector of the Huanglongbing pathogens, Annu. Rev. Entomol., 58 (2013): 413–432. |
[15] | X. Z. Meng and Z. Q. Li, The dynamics of plant disease models with continuous and impulsive cultural control strategies, J. Theor. Biol., 266 (2010): 29–40. |
[16] | X. S. Zhang and J. Holt, Mathematical models of cross protection in the epidemiology of plant- virus disease, Anal. Theo. Plant. Pathol., 91 (2001): 924–934. |
[17] | S. J. Gao, L. J. Xia, Y. Liu, et al., A plant virus disease model with periodic environment and pulse roguing, Stud. Appl. Math., 136 (2016): 357–381. |
[18] | S. Y. Tang, Y. N. Xiao and R. A. Cheke, Dynamical analysis of plant disease models with cultural control strategies and economic thresholds, Math. Comput. Simul., 80 (2010): 894–921. |
[19] | M. S. Chan and M. J. Jeger, An analytical model of plant virus disease dynamics with roguing and replanting, J. Appl. Ecol., 31 (1994): 413–427. |
[20] | C. Chiyaka, B. H. Singer, S. E. Halbert, et al., Modeling huanglongbing transmission within a citrus tree, PNAS, 109 (2012): 12213–12218. |
[21] | R. A. Taylor, E. A. Mordecai, C. A. Gilligan, et al., Mathematical models are a powerful method to understand and control the spread of Huanglongbing, Peer J, 4 (2016): DOI 10.7717/peerj.2642. |
[22] | K. Jacobsen, J. Stupiansky and S. S. Pilyugin, Mathematical modeling of citrus groves infected by Huanglongbing, Math. Biosci. Eng, 10 (2013): 705–728. |
[23] | R. G. dA. Vilamiu, S. Ternes, G. A. Braga, et al., A model for Huanglongbing spread between citrus plants including delay times and human intervention, AIP. Conf. Proc., 1479 (2012): 2315–2319. |
[24] | S. J. Gao, L. Luo, S. X. Yan, et al., Dynamical behavior of a novel impulsive switching model for HLB with seasonal fluctuations, Complexity, 2018 (2018): 1–11. |
[25] | J.A. Lee, S. E. Halbert, W. O. Dawson, et al., Asymptomatic spread of Huanglongbing and implications for disease control, Proc. Natl. Acad. Sci. USA., 112 (2015): 7605–7610. |
[26] | M. Parry, G. J. Gibson, S. Parnell, et al., Bayesian inference for an emerging arboreal epidemic in the presence of control, Proc. Natl. Acad. Sci. USA., 111 (2014): 6258–6262. |
[27] | S. E. Halbert and K. L. Manjunath, Asian citrus psyllids (Sternorrhyncha: Psyllidae) and greening disease of citrus: A literature review and assessment of risk in Florida, Flor. Entomol., 87 (2004): 330–353. |
[28] | W. D. Wang and X. Q. Zhao, Threshold dynamics for compartmental epidemic models in periodic environments, J. Dyn. Differ. Equ., 20 (2008): 699–717. |
[29] | P. V. D. Driessche and J. Watmough, Reproduction numbers and sub-threshold endemic equilibria for compartmental models of disease transmission, Math. Biosci., 180 (2002): 29–48. |
[30] | H. R. Thieme, Convergence results and poincare-bendixson trichotomy for asymptotically au-tonomous differential equations, J. Math. Biol., 30 (1992): 755–763. |
[31] | P. V. D. Driessche and J. Watmough, Reproductive numbers and subthreshold endemic equilibria for compartmental models of disease transmission, Math. Biosci., 180 (2002): 29–48. |
[32] | D. Xu and X. Q. Zhao, Dynamics in a periodic competitive model with stage structure, J. Math. Anal. Appl., 311 (2005): 417–438. |
[33] | X. Zhao, Dynamical Systems in Population Biology, Springer, New York, NY, USA, 2003. |
[34] | T. K. Kar and A. Batabyal, Stability analysis and optimal control of an SIR epidemic model with vaccination, Biosystems, 104 (2011): 127–135. |
[35] | L. Y. Pang, S. G. Ruan, S. H. Liu, et al. , Transmission dynamics and optimal control of measles epidemics, Appl. Math. Comput., 256 (2015): 131–147. |
[36] | M. A. Khan, R. Khan, Y. Khan, et al., A mathematical analysis of Pine Wilt disease with variable population size and optimal control strategies, Chaos, Solitons and Fract., 108 (2018): 205–217. |
[37] | W. H. Fleming and R. W. Rishel, Deterministic and Stochastic Optimal Control, Springer-Verlag, New York, Berlin, 1975. |
[38] | D. L. Lukes, Differential equations: Classical to Controlled, Mathematics in Science and Engi-neering, Academic Press, New York, 1982. |
[39] | S. Lenhart and J. T. Workman, Optimal control applied to biological models, Mathematical and Computational Biology Series, Chapman & Hall/CRC Press, London/Boca Raton, 2007. |
[40] | X. Zhang, Y. Zhao and A. Neumann, Partial immunity and vaccination for influenza, J. Comput. Biol., 17 (2009): 1689–1696. |
[41] | T. Gottwald, Current epidemiological understanding of citrus Huanglongbing, Annu. Rev. Phy-topathol., 48 (2010): 119–139. |
[42] | K. S. P. Stelinski, R. H. Brlansky, T. A. Ebert, et al., Transmission parameters for Candidatus Liberibacter asiaticus by Asian citrus psyllid (Hemiptera: Psyllidae), J. Econ. Entomol., 103 (2010): 1531–1541. |
[43] | M. E. Rogers, General pest management considerations, Citrus. Ind., 89 (2008): 12–15. |
1. | Changyong Zhou, The status of citrus Huanglongbing in China, 2020, 45, 1983-2052, 279, 10.1007/s40858-020-00363-8 | |
2. | Zhenzhen Liao, Shujing Gao, Shuixian Yan, Genjiao Zhou, Transmission dynamics and optimal control of a Huanglongbing model with time delay, 2021, 18, 1551-0018, 4162, 10.3934/mbe.2021209 | |
3. | Yujiang Liu, Shujing Gao, Zhenzhen Liao, Di Chen, Dynamical behavior of a stage-structured Huanglongbing model with time delays and optimal control, 2022, 156, 09600779, 111830, 10.1016/j.chaos.2022.111830 | |
4. | Fumin Zhang, Zhipeng Qiu, Tao Feng, Yanfei Dai, Guihong Fan, Modeling the Importation and Local Transmission of Huanglongbing Disease: Bifurcation and Sensitivity Analysis, 2022, 32, 0218-1274, 10.1142/S0218127422501176 | |
5. | Jing Guo, Shujing Gao, Shuixian Yan, Zhenzhen Liao, Bifurcation and optimal control analysis of delayed models for huanglongbing, 2022, 15, 1793-5245, 10.1142/S1793524522500498 | |
6. | Fumin Zhang, Zhipeng Qiu, Aijun Huang, Yan Cheng, Guihong Fan, Global dynamics and bifurcation analysis of an insect-borne plant disease model with two transmission routes, 2022, 15, 1793-5245, 10.1142/S1793524522500553 | |
7. | Youquan Luo, Fumin Zhang, Yujiang Liu, Shujing Gao, Analysis and optimal control of a Huanglongbing mathematical model with resistant vector, 2021, 6, 24680427, 782, 10.1016/j.idm.2021.05.004 | |
8. | Tahir Khan, Roman Ullah, Gul Zaman, Jehad Alzabut, A mathematical model for the dynamics of SARS-CoV-2 virus using the Caputo-Fabrizio operator, 2021, 18, 1551-0018, 6095, 10.3934/mbe.2021305 | |
9. | Yunbo Tu, Xinzhu Meng, Abdullah Khames Alzahrani, Tonghua Zhang, Multi-objective optimization and nonlinear dynamics for sub-healthy COVID-19 epidemic model subject to self-diffusion and cross-diffusion, 2023, 175, 09600779, 113920, 10.1016/j.chaos.2023.113920 | |
10. | Shuimei Tang, Shujing Gao, Fumin Zhang, Yujiang Liu, Role of vector resistance and grafting infection in Huanglongbing control models, 2023, 8, 24680427, 491, 10.1016/j.idm.2023.04.006 | |
11. | Yujiang Liu, Shujing Gao, Di Chen, Bing Liu, Modeling the Transmission Dynamics and Optimal Control Strategy for Huanglongbing, 2024, 12, 2227-7390, 2648, 10.3390/math12172648 | |
12. | Ying Wang, Shujing Gao, Yujiang Liu, Huaiping Zhu, Modeling Study of the Effects of Ageratum conyzoides on the Transmission and Control of Citrus Huanglongbing, 2023, 12, 2223-7747, 3659, 10.3390/plants12203659 |
Date | 06/30 | 07/30 | 08/30 | 09/15 | 09/30 | 10/15 |
I(t) | 0.0318 | 0.053 | 0.1327 | 0.1946 | 0.215 | 0.2778 |
Date | 10/30 | 11/15 | 11/30 | 12/15 | 12/30 | |
I(t) | 0.354 | 0.354 | 0.3717 | 0.3716 | 0.407 |
Parameter | Value | Unit | Reference | Parameter | Value | Unit | Reference |
η1 | 0.1667 | month−1 | [41] | d3 | 1.612 | month−1 | [9] |
η2 | 0.002258 | month−1 | [2] | d4 | 0.788 | month−1 | [9,42] |
ρ | 0.0791 | - | Estimation | f | 0.067 | month−1 | Estimation |
r | 62 | month | [9,42] | α1 | 0.00494 | month−1 | Fitting |
γ1 | 5.49625 | month−1 | [9] | α2 | 0.00043 | month−1 | Fitting |
γ2 | 5.376 | month−1 | [9] | β1 | 0.0097 | month−1 | Fitting |
γ3,γ4 | 2.188 | month−1 | [9] | β2 | 0.002258 | month−1 | Fitting |
d1 | 2.494 | month−1 | [9] | r0 | 6 | - | [43] |
d2 | 4.867 | month−1 | [9] |
Date | 06/30 | 07/30 | 08/30 | 09/15 | 09/30 | 10/15 |
I(t) | 0.0318 | 0.053 | 0.1327 | 0.1946 | 0.215 | 0.2778 |
Date | 10/30 | 11/15 | 11/30 | 12/15 | 12/30 | |
I(t) | 0.354 | 0.354 | 0.3717 | 0.3716 | 0.407 |
Parameter | Value | Unit | Reference | Parameter | Value | Unit | Reference |
η1 | 0.1667 | month−1 | [41] | d3 | 1.612 | month−1 | [9] |
η2 | 0.002258 | month−1 | [2] | d4 | 0.788 | month−1 | [9,42] |
ρ | 0.0791 | - | Estimation | f | 0.067 | month−1 | Estimation |
r | 62 | month | [9,42] | α1 | 0.00494 | month−1 | Fitting |
γ1 | 5.49625 | month−1 | [9] | α2 | 0.00043 | month−1 | Fitting |
γ2 | 5.376 | month−1 | [9] | β1 | 0.0097 | month−1 | Fitting |
γ3,γ4 | 2.188 | month−1 | [9] | β2 | 0.002258 | month−1 | Fitting |
d1 | 2.494 | month−1 | [9] | r0 | 6 | - | [43] |
d2 | 4.867 | month−1 | [9] |