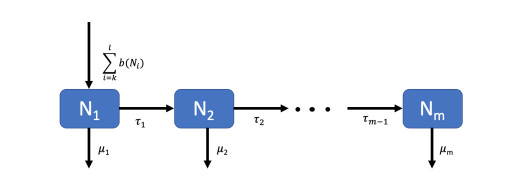
Citation: Qiuyi Su, Jianhong Wu. Impact of variability of reproductive ageing and rate on childhood infectious disease prevention and control: insights from stage-structured population models[J]. Mathematical Biosciences and Engineering, 2020, 17(6): 7671-7691. doi: 10.3934/mbe.2020390
[1] | Yicang Zhou, Zhien Ma . Global stability of a class of discrete age-structured SIS models with immigration. Mathematical Biosciences and Engineering, 2009, 6(2): 409-425. doi: 10.3934/mbe.2009.6.409 |
[2] | Yan-Xia Dang, Zhi-Peng Qiu, Xue-Zhi Li, Maia Martcheva . Global dynamics of a vector-host epidemic model with age of infection. Mathematical Biosciences and Engineering, 2017, 14(5&6): 1159-1186. doi: 10.3934/mbe.2017060 |
[3] | Jianxin Yang, Zhipeng Qiu, Xue-Zhi Li . Global stability of an age-structured cholera model. Mathematical Biosciences and Engineering, 2014, 11(3): 641-665. doi: 10.3934/mbe.2014.11.641 |
[4] | Sara Y. Del Valle, J. M. Hyman, Nakul Chitnis . Mathematical models of contact patterns between age groups for predicting the spread of infectious diseases. Mathematical Biosciences and Engineering, 2013, 10(5&6): 1475-1497. doi: 10.3934/mbe.2013.10.1475 |
[5] | Georgi Kapitanov . A double age-structured model of the co-infection of tuberculosis and HIV. Mathematical Biosciences and Engineering, 2015, 12(1): 23-40. doi: 10.3934/mbe.2015.12.23 |
[6] | Zhisheng Shuai, P. van den Driessche . Impact of heterogeneity on the dynamics of an SEIR epidemic model. Mathematical Biosciences and Engineering, 2012, 9(2): 393-411. doi: 10.3934/mbe.2012.9.393 |
[7] | Guihong Fan, Yijun Lou, Horst R. Thieme, Jianhong Wu . Stability and persistence in ODE modelsfor populations with many stages. Mathematical Biosciences and Engineering, 2015, 12(4): 661-686. doi: 10.3934/mbe.2015.12.661 |
[8] | Yijun Lou, Bei Sun . Stage duration distributions and intraspecific competition: a review of continuous stage-structured models. Mathematical Biosciences and Engineering, 2022, 19(8): 7543-7569. doi: 10.3934/mbe.2022355 |
[9] | Shanjing Ren, Lingling Li . Global stability mathematical analysis for virus transmission model with latent age structure. Mathematical Biosciences and Engineering, 2022, 19(4): 3337-3349. doi: 10.3934/mbe.2022154 |
[10] | James M. Hyman, Jia Li . Epidemic models with differential susceptibility and staged progression and their dynamics. Mathematical Biosciences and Engineering, 2009, 6(2): 321-332. doi: 10.3934/mbe.2009.6.321 |
Family planning involves consideration of the number (including the choice of zero) and spacing of children a family wishes to have. A number of factors can impact the family planning at the individual family level. At the population level the family planning and reproduction strategy including fertility, birth age and spacing of children, may be heavily influenced by economical conditions and societal resources which can be weighted heavily by the age-distribution of the entire population. In developing countries, policies like subsidizing education raise the earning power of women and the opportunity cost of having children, consequently lowers fertility [1]. Access to contraceptives may also yield lower fertility rates. In developed countries, the proportion of retired people is increasing, adding burden on the workforce population to support pensions and social programs. Increasing high skill migration may be an effective way to increase the return to education leading to lower fertility and a greater supply of highly skilled individuals [1], thus address the aging population problem.
A well-known example of family planning and age-distribution of population being significantly regulated by political and social-economic consideration is the China's one-child policy implemented for many years. In 1973, the Chinese government issued voluntary guidelines on fertility control to encourage later marriage, longer spacing between births, and fewer births overall [2,3]. In 1981, China's National Family Planning Commission proposed a population control policy advocating one child per couple, which was moderated in 1984, allowing most rural families a second child [2,3,4]. In 2002, the policy was incorporated in the Population and Family Planning Law, at the same time, a second child was permitted in some provinces if both husband and wife were from single-child families [2,3,5]. In 2013, the policy was relaxed to allow a second child if either spouse was from a single-child family [3,6]. In October of 2015, the Chinese government announced a two-child policy, effective from January 1 of 2016 [7]. The new policy that allows each couple have two children was proposed in order to help address the population aging issue. It was reported that, starting from May 2018, Chinese authorities were in the process of ending the population control policies [8].
A consequence of this recent change of the centralized population control policy after a long-term implementation of one-child per family policy is the obvious increasing of the family size, and substantial heterogeneity of the reproduction age and the spacing between the first and second child in those families with two children. This generates new close contact patterns in household and community level and thus any issue relevant to these contact patterns must be revisited. The control and prevention of childhood infectious diseases preventable by vaccine, such as pertussis, is one of these critical public health issues. Taking pertussis as an example, this childhood disease can be fatal in infants but infection can be prevented in other age groups with an effective vaccine. Pertussis vaccines wane over time, so those children who are expected to have younger siblings need to take a booster vaccine if (I) the prevalence of disease in the older age group and/or groups (recalling the potential heterogeneity of spacing between two children since females in multiple age groups may consider to give birth) is expected to be high; and (II) vaccine waning make this group of these groups less protected and more susceptible to the disease.
To the best of my knowledge, there is no study on impact of family planning and the scale of density-regulated birth rate on the long-term population demographic distribution and childhood disease dynamics. However, there are a few studies which imply the impact of demographic change on infectious disease dynamics. These work include studies on demographic transition and the dynamics of measles in China [9], the influence of demographic change on spread of infectious diseases [10], the impact of demographic transition on rubella transmission dynamics in China [11], the effects of demographic change and immigration on infectious diseases in Italy [12], the effects of demographic change on disease transmission and vaccine impact in a household structured population [13], the dynamical consequences of demographic change in a model of disease transmission [14] and the impact of demographic change on the estimated future burden of hepatitis B and seasonal influenza in the Netherlands [15].
This series of studies is dedicated to developing mathematical frameworks and analyses to examine the patterns of childhood infectious disease transmission, to identify prevalence of disease in different age groups, when female in multiple age intervals are giving birth to the second children. In this first paper of the series, we start with a simple stage-structured disease transmission model, and study the impact of family planning and the scale of density-regulated birth rate on the long-term population demographic distribution and infant disease incidence prevalence. We conduct our analyses by varying three parameters: birth rate, reproduction age interval(s), and the scale of the sub-population density regulation.
In particular, we introduce a multi-stage (m-stages) stratified model, where the population is divided by age into m groups (stages) with the i-th age stage spanning the age interval of length τi. Assume that females in the age groups, k,k+1,⋯,l-th groups give birth. For each group, we have the classical SIS epidemic-model, where the population is divided into the susceptible and the infectious. We consider the situation where the infectious period is much shorter than the period of each age stage. Therefore, the infectious individuals in the i-th age group Ii go back to the susceptible class Si before advancing to the (i+1)-th age group. The flowcharts of the demographic model and epidemiological model are shown in Figures 1 and 2 respectively.
Let Si(t) be the population of the susceptible of the ith age group, Ii(t) be the population of the infectious of the ith age group. Ni(t) denotes the total population of the ith age group at time t. The death rate of the ith age group is given by μi; σ is the recover rate; the birth rate of the ith productive group is a nonlinear function bi(Ni); βij is the transmission rate of the disease from stage j to stage i. The age-stratified epidemiological model is given by the following equations:
dS1(t)dt=l∑i=kbi(Ni(t))−l∑i=kbi(Ni(t−τ1))e−μ1τ1−μ1S1(t)−m∑i=1β1iS1(t)Ii(t)+σI1(t)dSh(t)dt=l∑i=kbi(Ni(t−h−1∑j=1τj))e−∑h−1j=1μjτj−l∑i=kbi(Ni(t−h∑j=1τj))e−∑hj=1μjτj−μhSh(t)−m∑i=1βhiSh(t)Ii(t)+σIh(t)for1<h<mdSm(t)dt=l∑i=kbi(Ni(t−m−1∑j=1τj))e−∑m−1j=1μjτj−μmSm(t)−m∑i=1βmiSm(t)Ii(t)+σIm(t)dIh(t)dt=m∑i=1βhiSh(t)Ii(t)−σIh(t)−μhIh(t)for1≤h≤m | (1.1) |
The demographic model is given by
dN1(t)dt=l∑i=kbi(Ni(t))−l∑i=kbi(Ni(t−τ1))e−μ1τ1−μ1N1(t)dNh(t)dt=l∑i=kbi(Ni(t−h−1∑j=1τj))e−∑h−1j=1μjτj−l∑i=kbi(Ni(t−h∑j=1τj))e−∑hj=1μjτj−μhNh(t)for1<h<mdNm(t)dt=l∑i=kbi(Ni(t−m−1∑j=1τj))e−∑m−1j=1μjτj−μmNm(t). | (1.2) |
In this section, we investigate the dynamics of the demographic model by studying the stability of equilibrium.
Linearizing (1.2) at the zero equilibrium gives
dN1(t)dt=l∑i=kb′i(0)Ni(t)−l∑i=kb′i(0)Ni(t−τ1)e−μ1τ1−μ1N1(t)dNh(t)dt=l∑i=kb′i(0)Ni(t−h−1∑j=1τj)e−∑h−1j=1μjτj−l∑i=kb′i(0)Ni(t−h∑j=1τj)e−∑hj=1μjτj−μhNh(t)for1<h<mdNm(t)dt=l∑i=kb′i(0)Ni(t−m−1∑j=1τj)e−∑m−1j=1μjτj−μmNm(t) | (2.1) |
Let λ be eigenvalue of the linear system (2.1). By calculation, the characteristic equation is given by
l∑i=kb′i(0)(1−e−(λ+μi)τi)e−λ∑i−1j=1τj−∑i−1j=1μjτjμi+λ=1 | (2.2) |
Now we make the following assumption:
(A1) The birth function takes the form bi(x)=pixq(x) where q(x) is a non-negative monotone decreasing function.
Note that pi is the maximal number of children a female in age group i could give per unit time, q(x) is the function which implies the restriction of resources, so assumption (A1) reflects the ecological consideration that the reproduction is linear in x only for small densities and decreases as a consequence of intra specific competition. For example, one well known birth function which takes the form in assumption (A1) is the Ricker function b(x)=pxe−qx.
With this assumption, we have the following theorem on local stability of the zero equilibrium.
Theorem 1. Under assumptions (A1), if ∑li=kpiq(0)e−∑i−1j=1μjτj(1−e−μiτi)μi>1, then the zero equilibrium is unstable; if ∑li=kpiq(0)e−∑i−1j=1μjτj(1−e−μiτi)μi<1, the zero equilibrium is stable.
Proof. Let G(λ):=∑li=kb′i(0)gi(λ), where gi(λ)=(1−e−(λ+μi)τi)e−λ∑i−1j=1τj−∑i−1j=1μjτjμi+λ. Then the characteristic equation (2.2) can be written as G(λ)=1. Calculating the derivative gives g′i(λ)<0 on (−μi,∞). Furthermore, gi(λ)→0 as λ→∞ and gi(λ)→+∞ as λ→−μi. From assumption (A1), we have b′i(0)=piq(0)>0. Therefore, G(λ) is monotone decreasing on (−μ,∞) where μ=min{μi,i=k,k+1,...,l}. Moreover, G(λ)→0 as λ→∞ and G(λ)→+∞ as λ→−μ.
If G(0)=∑li=kpiq(0)e−∑i−1j=1μjτj(1−e−μiτi)μi>1, G(λ)>1, since G(λ) is monotone decreasing on (−μ,∞) and limλ→∞G(λ)=0, the characteristic equation has a positive real root. So the zero equilibrium is unstable. If G(0)=∑li=kpiq(0)e−∑i−1j=1μjτj(1−e−μiτi)μi<1, G(λ)<1, since G(λ) is monotone decreasing on (−μ,∞), limλ→∞G(λ)=0 and limλ→−μG(λ)=+∞, the characteristic equation has a positive real root. So the zero equilibrium is stable.
Suppose that there is a positive equilibrium (N∗1,N∗2,...,N∗m), then we have
l∑i=kbi(N∗i)−l∑i=kbi(N∗i)e−μ1τ1−μ1N∗1=0l∑i=kbi(N∗i)e−∑h−1j=1μjτj−l∑i=kbi(N∗i)e−∑hj=1μjτj−μhN∗h=0for1<h<ml∑i=kbi(N∗i)e−∑m−1j=1μjτj−μmN∗m=0 | (2.3) |
From Eq (2.3) we derive
N∗1=1μ1l∑i=kbi(N∗i)(1−e−μ1τ1)N∗h=1μhl∑i=kbi(N∗i)e−h−1∑j=1μjτj(1−e−μhτh)for1<h<mN∗m=1μml∑i=kbi(N∗i)e−m−1∑j=1μjτj | (2.4) |
The conditions for existence of this positive equilibrium is given in the following theorem.
Theorem 2. Under assumption (A1) with limx→∞q(x)=0, the positive equilibrium (N∗1,N∗2,...,N∗m) exists and is unique if ∑li=kpiq(0)e−∑i−1j=1μjτj(1−e−μiτi)μi>1.
Proof. From Eq (2.4),
N∗h=μ1e−∑h−1j=1μjτj(1−e−μhτh)μh(1−e−μ1τ1)N∗1 | (2.5) |
for k≤h≤l. Equation (2.5) and the first equation in (2.4) imply that
μ1N∗11−e−μ1τ1=l∑i=kbi(μ1e−∑i−1j=1μjτj(1−e−μiτi)μi(1−e−μ1τ1)N∗1) | (2.6) |
From assumption (A1), bi(x)=pixq(x), so Eq (2.6) becomes
1=l∑i=kpie−∑i−1j=1μjτj(1−e−μiτi)μiq(μ1e−∑i−1j=1μjτj(1−e−μiτi)μi(1−e−μ1τ1)N∗1) | (2.7) |
So the positive equilibrium exists if there exists a positive N∗1 such that Eq (2.7) holds. Now let G(x)=∑li=kpie−∑i−1j=1μjτj(1−e−μiτi)μiq(μ1e−∑i−1j=1μjτj(1−e−μiτi)μi(1−e−μ1τ1)x). Since q(x) is monotone decreasing with respect to x, G(x) is monotone decreasing function. Furthermore, limx→∞G(x)=0. So G(x)=1 has a unique positive solution if and only if G(0)>1, i.e., ∑li=kpiq(0)e−∑i−1j=1μjτj(1−e−μiτi)μi>1.
Note that Theorems 1 and 2 imply that the positive equilibrium exists and is unique if and only if the zero equilibrium is unstable.
For the next, we study stability of this positive equilibrium.
We denote by C+m the non-negative cone of the Banach space of continuous functions Cm={φ=(φ1,φ2,...,φm):[−r,0]→Rmcontinuous}, where r=max{τ1,τ2,...,τm}, i.e. C+m={φ∈Cm:φi(θ)≥0forθ∈[−r,0],i=0,1,2,...,m}. By using the method of steps, it can be shown that for each φ∈C+m, there is a unique solution of (1.2) π(φ,t)=(N1(φ,t),N2(φ,t),...,Nm(φ,t))∈R+m through φ that is well defined and satisfies π(φ;.)|[−r,0]=φ.
In fact, by taking integral and making substitutions, system (1.2) can be written as
N1(t)=∫τ10e−μ1θl∑i=kbi(Ni(t−θ))dθNh(t)=∫∑hj=1τj∑h−1j=1τjl∑i=kbi(Ni(t−θ))e−∑h−1j=1μjτj−μh(θ−∑h−1j=1τj)dθ1<h<mNm(t)=∫∞∑m−1j=1τjl∑i=kbi(Ni(t−θ))e−∑m−1j=1μjτj−μm(θ−∑m−1j=1τj)dθ | (2.8) |
In what follows, we give a preliminary result, then we give a theorem on global stability of the positive equilibrium.
Lemma 1. Under assumption (A1), if the birth functions bi(x) are bounded for i=k,k+1,...,l, for every φ∈C+m with φi(0)>0, i=1,2,...,m, the solution π(φ;t) of (1.2) is bounded above for t>0.
Proof. Let N(t)=∑mi=1Ni(t). By adding up the m equations in Eq (1.2), we obtain
dNdt=l∑i=kbi(Ni(t))−m∑i=1μiNi(t)≤l∑i=kbi(Ni(t))−μN(t) |
where μ is the smallest death rate in the m age groups, i.e., μ=min{μi,i=1,2,...,m}. Since the birth functions bi(x) are bounded for i=k,k+1,...,l, there are Mi for i=1,2,...,m such that bi(Ni(t))≤Mi. Let M=∑li=kMi, then dNdt≤M−μN(t), which means that dNdt<0 when N>Mμ. So N is bounded, i.e., there is ˉN such that N(t)≤ˉN for t≥0. Therefore, Ni(t)≤ˉN for t≥0 for i=1,2,...,m. The solution π(φ;t) is bounded for t>0.
Theorem 3. Under assumption (A1) with limx→∞q(x)=0, if ∑li=kpiq(0)e−∑i−1j=1μjτj(1−e−μiτi)μi>1 and ∑li=k|b′i(N∗i)|e−∑i−1j=1μjτj(1+e−μiτi)μi<1, then the positive equilibrium (N∗1,N∗2,...,N∗m) is locally stable.
Proof. The linearized equations at the endemic equilirbium (N∗1,N∗2,...,N∗m) of system (1.2) is given by
dN1(t)dt=l∑i=kb′i(N∗i)Ni(t)−l∑i=kb′i(N∗i)Ni(t−τ1)e−μ1τ1−μ1N1(t)dNh(t)dt=l∑i=kb′i(N∗i)Ni(t−h−1∑j=1τj)e−∑h−1j=1τj−l∑i=kb′i(N∗i)Ni(t−h∑j=1τj)e−∑hj=1τj−μhNh(t)for1<h<mdNm(t)dt=l∑i=kb′i(N∗i)Ni(t−m−1∑j=1τj)e−∑m−1j=1μjτj−μmNm(t) | (2.9) |
Let λ be eigenvalue of the linear system (2.9). By calculation, the characteristic equation is given by
l∑i=kb′i(N∗i)(1−e−(λ+μi)τi)e−λ∑i−1j=1τj−∑i−1j=1μjτjμi+λ=1 | (2.10) |
Suppose that the characteristic equation (2.10) has an eigenvalue with non-negative real part, i.e., there exits λ=x+iy such that x≥0, then
|b′i(N∗i)(1−e−(λ+μi)τi)e−λ∑i−1j=1τj−∑i−1j=1μjτjμi+λ|=|b′i(N∗i)(1−e−(x+iy+μi)τi)e−(x+iy)∑i−1j=1τj−∑i−1j=1μjτjμi+x+iy|≤|b′i(N∗i)||1−e−(x+iy+μi)τi||e−(x+iy)∑i−1j=1τj−∑i−1j=1μjτj||μi+x+iy|≤|b′i(N∗i)||1−e−(x+μi)τi(cosyτi−isinyτi)||e−∑i−1j=1μjτj||μi+x+iy|=|b′i(N∗i)|√(1−e−(x+μi)τicosyτi)2+(e−(x+μi)τisinyτi)2|e−∑i−1j=1μjτj|√(x+μi)2+y2≤|b′i(N∗i)|√1+e−2(x+μi)−2e−(x+μi)τicosyτi|e−∑i−1j=1μjτj|μi≤|b′i(N∗i)|√1+e−2(x+μi)+2e−(x+μi)τi|e−∑i−1j=1μjτj|μi=|b′i(N∗i)|(1+e−(x+μi)τi)e−∑i−1j=1μjτjμi≤|b′i(N∗i)|(1+e−μiτi)e−∑i−1j=1μjτjμi | (2.11) |
Therefore, Eq (2.10) and inequality (2.11) indicate that
1=|l∑i=kb′i(N∗i)(1−e−(λ+μi)τi)e−λ∑i−1j=1τj−∑i−1j=1μjτjμi+λ|≤l∑i=k|b′i(N∗i)(1−e−(λ+μi)τi)e−λ∑i−1j=1τj−∑i−1j=1μjτjμi+λ|≤l∑i=k|b′i(N∗i)|(1+e−μiτi)e−∑i−1j=1μjτjμi | (2.12) |
which contradicts with the assumption that ∑li=k|b′i(N∗i)|e−∑i−1j=1μjτj(1+e−μiτi)μi<1. So the characteristic equation (2.10) has no eigenvalue with non-negative real part, the positive equilibrium (N∗1,N∗2,...,N∗m) is locally stable.
Theorem 4. Under assumption (A1) with limx→∞q(x)=0 and the birth functions bi(x) bounded for i=k,k+1,...,l, assume that μh=μ and τh=τ for some μ>0, τ>0 and all k≤h≤l. If ∑li=k|b′i(N∗i)|e−∑i−1j=1μjτj(1+e−μiτi)μi<1, then the positive equilibrium (N∗1,N∗2,...,N∗m) is globally stable. i.e., limt→∞π(φ;t)=(N∗1,N∗2,...,N∗m) for φ∈C+m with φi(0)>0.
Proof. Let {Ni(t)} be a solution of Eq (1.2). Since it's bounded, we can define
δi=lim inft→∞Ni(t),γi=lim supt→∞Ni(t) |
Let h be such that k≤h≤l, i.e. Nh is a productive group. There exists a sequence {tn} and a sequence {sn} such that limn→∞Nh(tn)=γh and limn→∞Nh(sn)=δh. So there exists some ϵ>0 such that δi−ϵ<Ni(tn)<γi+ϵ and δi−ϵ<Ni(sn)<γi+ϵ for n large enough for all k≤i≤l.
From the integrated equation (2.8),
Nh(tn)<∫∑hj=1τj∑h−1j=1τj∑li=kpi(γi+)q(δi−)e−∑h−1j=1μjτj−μh(θ−∑h−1j=1τj)dθ |
Let n→∞ and ϵ→0, the inequality becomes
γh≤∫∑hj=1τj∑h−1j=1τjl∑i=kpiγiq(δi)e−∑h−1j=1μjτj−μh(θ−∑h−1j=1τj)dθ=l∑i=kpiγiq(δi)e−∑h−1j=1μjτj+μh∑h−1j=1τj∫∑hj=1τj∑h−1j=1τje−μhθdθ=l∑i=kpiγiq(δi)(1−e−μhτh)e−∑h−1j=1μjτjμhq(δi) | (2.13) |
Now let A:=∑li=kpiγiq(δi), Eq (2.13) implies that
A=l∑i=kpiγiq(δi)≤l∑i=kpiA(1−e−μiτi)e−∑i−1j=1μjτjμiq(δi) |
which further implies that
l∑i=kpiq(δi)(1−e−μiτi)e−∑i−1j=1μjτjμi≥1 | (2.14) |
Let B:=∑li=kpiN∗iq(N∗i), from Eq (2.4)
B=l∑i=kpiN∗iq(N∗i)=l∑i=kpiB(1−e−μiτi)e−∑i−1j=1μjτjμiq(N∗i) |
so
l∑i=kpi(1−e−μiτi)e−∑i−1j=1μjτjμiq(N∗i)=1 | (2.15) |
From assumption (A1), q(x) is monotone decreasing, then Eqs (2.14) and (2.15) imply that there exists h1 such that k≤h1≤l and δh1≤N∗h1. From the integrated equation (2.8),
Nh(sn)>∫∑hj=1τj∑h−1j=1τjl∑i=kpi(δi−ϵ)q(γi+ϵ)e−∑h−1j=1μjτj−μh(θ−∑h−1j=1τj)dθ |
Following similar calculation as inequalities (2.13) and (2.14), we obtain
l∑i=kpiq(γi)(1−e−μiτi)e−∑i−1j=1μjτjμi≤1. | (2.16) |
From assumption (A1), q(x) is monotone decreasing, then Eqs (2.15) and (2.16) imply that there exists h2 such that k≤h2≤l and γh2≥N∗h2.
By substituting variables, from Eqs (2.4) and (2.8) we obtain
(Nk(t)−N∗k)e∑k−1j=1μjτj=∫∑kj=1τj∑k−1j=1τjl∑i=k(bi(Ni(t−θ)−bi(N∗i))e−μk(θ−∑k−1j=1τj)dθ | (2.17) |
and
(Nh(t)−N∗h)e∑h−1j=1μjτj=∫∑k−1j=1τj+τh∑k−1j=1τjl∑i=k(bi(Ni(t−θ)−bi(N∗i))e−μh(θ−∑k−1j=1τj)dθ | (2.18) |
Since μh=μ and τh=τ for k≤h≤l, Eqs (2.17) and (2.18) imply that
(Nk(t)−N∗k)e∑k−1j=1μjτj=(Nh(t)−N∗h)e∑h−1j=1μjτj | (2.19) |
for k≤h≤l.
Scenario 1: γk=N∗k
In this scenario, since the positive equilibrium is locally stable, δk=γk=N∗k, and limt→∞Nk(t)=N∗k. From Eq (2.19), limt→∞Nh(t)=N∗h for all k≤h≤l.
Scenario 2: γk>N∗k
In this scenario, since the positive equilibrium is locally stable, δk>N∗k, then Nk(t)>N∗k for t large enough. From Eq (2.19), Nh1(t)>N∗h1 for t large enough, which means that δh1≥N∗h1. Since we have δh1≤N∗h1 from previous discussion, δh1=N∗h1. Therefore, δh1=γh1=N∗h1, i.e., limt→∞Nh1(t)=N∗h1. From Eq (2.19), limt→∞Nh(t)=N∗h for all k≤h≤l.
Scenario 3: γk<N∗k
Then δk<N∗k and Nk(t)<N∗k for t large enough. From Eq (2.19), Nh2(t)<N∗h2 for t large enough, which implies that γh2≤N∗h2. Since we have γh2≥N∗h2 from previous discussion, γh2=N∗h2. i.e., limt→∞Nh2(t)=N∗h2. From Eq (2.19), limt→∞Nh(t)=N∗h for all k≤h≤l.
From discussion above, limt→∞Nh(t)=N∗h for all k≤h≤l. Then by the integral equation (2.8), limt→∞Nh(t)=N∗h for all 1≤h≤m.
Now we focus on the epidemic model, which is an ODE system given by
dIh(t)dt=m∑i=1βhi(Nh(t)−Ih(t))Ii(t)−σIh(t)−μhIh(t)for1≤h≤m | (3.1) |
Note that Eq (3.1) is derived from the last equation in (1.1) by replacing Sh(t) by Nh(t)−Ih(t).
Suppose that the population has reached the positive equilibrium, then system (3.1) is given by
dIh(t)dt=m∑i=1βhi(N∗h−Ih(t))Ii(t)−σIh(t)−μhIh(t)for1≤h≤m | (3.2) |
Let I=(I1,I2,...,Im)T, the flow of the solution of system (3.2) with initial value I0=(I01,I02,...,I0m) is given by ϕt(I0). Let V=(0,N∗1)×(0,N∗2)×...×(0,N∗m). We have the following conclusion
Theorem 5. If I0i∈(0,N∗i), then ϕti(I0)∈(0,N∗i), i.e., V is invariant under the flow ϕt.
Proof. Suppose that there is a smallest t0 such that there is j∈{1,2,...,m} such that Ij(t0)=0. Since I(t) is not constant 0, we have dIj(t)dt|t=t0=∑mi=1βhi(N∗h−Ih(t0))Ii(t0)>0. On the other hand, since t0 is the smallest s.t. Ij(t0)=0, dIj(t)dt|t=t0=limϵ→0Ij(t0−ϵ)−Ij(t0)−ϵ≤0, which is a contradiction. So Ij(t)>0 for all 1≤j≤m and all t>0 with initial value in V.
Similarly, suppose that there is a smallest t0 such that there is j∈{1,2,...,m} such that Ij(t0)=N∗j, then from Eq (3.2), dIjdt|t=t0=−σIh(t0)−μhIh(t0)<0. On the other hand, since t0 is the smallest s.t.Ij(t0)=N∗j, dIj(t)dt|t=t0=limϵ→0Ij(t0−ϵ)−Ij(t0)−ϵ≥0, which is a contradiction. So Ij(t)<0 for all 1≤j≤m and all t>0 with initial value in V.
Equation (3.2) has (0,0,...,0) as the disease-free equilibrium. Linearization around this equilibrium gives the following linear system
dIh(t)dt=m∑i=1βhiN∗hIi(t)−σIh(t)−μhIh(t) | (3.3) |
for 1≤h≤m Following the method in [16], we get
F=[β11N∗1β12N∗1⋯β1mN∗1β21N∗2β22N∗2⋯β2mN∗2⋮⋮⋱⋮βm1N∗mβm2N∗m⋯βmmN∗m] |
and
V=[σ+μ10⋯00σ+μ2⋯0⋮⋮⋱⋮00⋯σ+μm] |
Then we have
FV−1=[β11N∗1σ+μ1β12N∗1σ+μ2⋯β1mN∗1σ+μmβ21N∗2σ+μ1β22N∗2σ+μ2⋯β2mN∗2σ+μm⋮⋮⋱⋮βm1N∗mσ+μ1βm2N∗mσ+μ2⋯βmmN∗mσ+μm] |
In particular, if we assume that βij=αiλj, it can be calculated from induction that the characteristic equation of FV−1 is given by λm−1(λ−∑mi=1αiλiN∗iσ+μi)=0, then
R0=ρ(FV−1)=m∑i=1αiλiN∗iσ+μi |
The following theorem follows
Theorem 6. Assume that βij=αiλj. Then when ∑mi=1αiλiN∗iσ+μi<1, the disease-free equilibrium of system (3.2) is stable; when ∑mi=1αiλiN∗iσ+μi>1, the disease-free equilibrium of system (3.2) is unstable.
For the assumption βij=αiλj, if we assume that the population is homogeneously mixed, αi can be interpreted as susceptibility of age group i and λj can be interpreted as infectivity of age group j.
Suppose system (3.2) has a nontrivial equilibrium (I∗1,I∗2,...,I∗m), by plugging in the Eq (3.2) we derive
m∑i=1βhi(N∗h−I∗h)I∗i−σI∗h−μhI∗h=0 | (3.4) |
for 1≤h≤m. In particular, if we assume that βij=αiλj, then Eq (3.4) can be written as
(N∗h−I∗h)m∑i=1αhλiI∗i−σI∗h−μhI∗h=0 | (3.5) |
for 1≤h≤m. From Eq (3.5) we get
m∑i=1λiI∗i=(σ+μh)I∗hαh(N∗h−I∗h) | (3.6) |
for 1≤h≤m. and
σ+μhαh(N∗h/I∗h−1)=σ+μ1α1(N∗1/I∗1−1) |
Now let Mi=N∗iI∗i−1 and li=σ+μiαi, then
liMi=l1M1 |
Plugging I∗i=N∗iMi+1 into Eq (3.6) with h=1, we have
m∑i=1λiN∗iMi+1=l1M1 |
It follows that
m∑i=1λiN∗ili+l1/M1=1 |
Now define G:(0,N∗1)⟶R by
G(x)=m∑i=1λiN∗ili+l1/M | (3.7) |
where M=N∗1x−1. Then I∗1 is a solution of G(x)=1.
Note that G(x) is monotone non-increasing with respect to x, G(x)⟶0 as x⟶N∗1 and G(x)⟶∑mi=1λiN∗ili as x⟶0. So G(x)=1 has a solution in (0,N∗1) if and only if ∑mi=1λiN∗ili>1, and the solution is unique by monotonicity of G(x).
Note that R0=∑mi=1λiN∗ili. We conclude that
Theorem 7. Assume that βij=αiλj. The endemic equilibrium of system (3.2) exists and is unique if and only if R0=∑mi=1λiN∗ili>1.
Now we state the following theorem on global stability of the endemic equilibrium.
Theorem 8. Assume that βij=αiλj.Let V=(0,N∗1)×(0,N∗2)×...×(0,N∗m). If R0>1, then the endemic equilibrium {I∗i} of system (3.2) attracts all the forward orbits going through V.
Proof. Let F:V⟶Rm be defined by Fh(I1,I2,...,Im)=∑mi=1βhi(N∗h−Ih)Ii−σIh−μhIh.
It suffices to prove that
(H1) System (3.2) is cooperative, i.e., ∂Fh∂Ij≥0 for h≠j.
(H2) F is irreducible in the sense that the matrix [∂Fh∂Ij] is irreducible.
(H3) Solutions of Eq (3.2) with initial value (I01,I02,...,I0m) such that |I0h|≤N∗h are bounded.
Then by Theorems 1.5 and 2.4 in [17], (H1) and (H2) imply that system (3.2) doesn't have a non-constant periodic solution. By Theorem 1.1 in [17], (H1) and (H2) also imply that the solution flows of Eq (3.2) going through V have positive derivatives. Then by Theorem 4.1 in [17] and (H3) we conclude that almost all forward orbits of V converge to the endemic equilibrium {I∗i}.
(H1) ∂Fh∂Ij=βhj(N∗h−Ih)>0 for h≠j.
(H2) ∂Fh∂Ih=βhh(N∗h−Ih)−∑mi=1βhiIi−σ−μh. Now let A=[∂Fh∂Ij]. Ahj=∂Fh∂Ij>0 for h≠j by (H1). Suppose there is 1≤h≤m such that Ahh≤0, A2hh=∑mi=1AhiAih>0. Therefore, for each pair of indices h and j, there exists a natural number n such that Anhj is positive, which implies that the matrix A is irreducible.
(H3) It can be derived directly from Theorem 5.
Now if we look back on the original epidemic model (3.1), we have the following Theorem from Theorem 8.
Theorem 9. Under assumption (A1) with limx→∞q(x)=0, assume that βij=αiλj and μh=μ, τh=τ for some μ>0, τ>0 and all k≤h≤l.Let V=(0,N∗1)×(0,N∗2)×...×(0,N∗m). If ∑li=k|b′i(N∗i)|e−∑i−1j=1μjτj(1+e−μiτi)μi<1 and R0>1, then {I∗i} attracts all the forward orbits going through V in system (3.1).
Proof. From Theorem 4, limt→∞Nh(t)=N∗h for all 1≤h≤m. Denote Φ(t,s,x0) the solution of system (3.1) with x(s)=x0, and denote Θ(t,x0) the solution of system (3.2) with y(0)=x0. Then by Proposition 1.1 in [18], Φ is asymptotically autonomous semiflow with limit semiflow Θ. Let OΦ(s,x)={Φ(t,s,x):t≥s}, x∈V, then OΦ(s,x) has compact closure in V since it's bounded. Let ω=ω(s,x) which is the ω-limit set of OΦ(s,x). By Theorem 1.8 in [18], we conclude that ω is non-empty, compact and connected, and it attracts Φ(t,s,x). Moreover, ω is invariant for the semiflow Θ and is chain recurrent for Θ.
Now suppose that ω≠{I∗i}. There exists x=(x1,x2,...,xm)∈ω such that x≠{I∗i}. Let ϵ=d(x,{I∗i}). Since ω is compact, there exists T>0 such that d(Θ(t,x0),{I∗i})<ϵ2 for all x0∈ω and t≥T. By the definition of chain recurrence, there is an (ϵ2,T) chain from x to x, i.e., there is a sequence {x=x1,x2,...,xn+1=x;t1,t2,...,tn} for xi∈ω and ti≥T such that d(Θ(ti,xi),xi+1)<ϵ2. Then d(Θ(tn,xn),x)<ϵ2, which indicates that d(x,{I∗i})≤d(Θ(tn,xn),x)+d(Θ(tn,xn),{I∗i})<ϵ2+ϵ2=ϵ, which contradicts with ϵ=d(x,{I∗i}). Therefore, ω={I∗i}, i.e., {I∗i} attracts all the forward orbits going through V in system (3.1).
In this section, we assume that the birth rate of age group i is given by bi(Ni)=piNie−qN, we'll analyze how do demographic distribution N∗iN and infant disease rate at endemic equilibrium I∗1N∗1 change with birth parameters pi, q and productive age k.
By plugging bi(Ni)=piNie−qN into Eq (2.4), we have
l∑i=kpiN∗ie−qN=μhN∗he−∑h−1j=1μjτj(1−e−μhτh) |
for 1≤h≤m.
N∗iN∗h=μhe−∑i−1j=1μjτj(1−e−μiτi)μie−∑h−1j=1μjτj(1−e−μhτh) |
for 1<i,h<m. Combine the above two formulas, we have
l∑i=kpiμhe−∑i−1j=1μjτj(1−e−μiτi)μie−∑h−1j=1μjτj(1−e−μhτh)N∗he−qN=μhN∗he−∑h−1j=1μjτj(1−e−μhτh) |
Thus
l∑i=kpie−∑i−1j=1μjτj(1−e−μiτi)μi=eqN |
Solving for N gives
N=1qInl∑i=kpie−∑i−1j=1μjτj(1−e−μiτi)μi | (4.1) |
From Eq (2.4), we define
Q:=μ1N∗11−e−μ1τ1=μhN∗he−∑h−1j=1μjτj(1−e−μhτh)=μmN∗me−∑m−1j=1μjτj |
for 1≤h≤m. It follows that
N∗1=1−e−μ1τ1μ1QN∗h=e−∑h−1j=1μjτj(1−e−μhτh)μhQfor1<h<mN∗m=e−∑m−1j=1μjτjμmQ | (4.2) |
By plugging Eq (4.2) into N=∑mh=1N∗h, we have
N=(1−e−μ1τ1μ1+m−1∑h=1e−∑h−1j=1μjτj(1−e−μhτh)μh+e−∑m−1j=1μjτjμm)Q | (4.3) |
Equations (4.2) and (4.3) give
N∗1N=1−e−μ1τ1μ11−e−μ1τ1μ1+∑m−1h=1e−∑h−1j=1μjτj(1−e−μhτh)μh+e−∑m−1j=1μjτjμmN∗hN=e−∑h−1j=1μjτj(1−e−μhτh)μh1−e−μ1τ1μ1+∑m−1h=1e−∑h−1j=1μjτj(1−e−μhτh)μh+e−∑m−1j=1μjτjμmfor1<h<mN∗mN=e−∑m−1j=1μjτjμm1−e−μ1τ1μ1+∑m−1h=1e−∑h−1j=1μjτj(1−e−μhτh)μh+e−∑m−1j=1μjτjμm | (4.4) |
It's obvious from Eq (4.4) that change of pi, q and k don't make a change on the demographic distribution N∗hN for 1≤h≤m.
Proposition 10. Assume that the birth rate of age group i is given by bi(Ni)=piNie−qN. The stablized demographic distribution N∗h/N doesn't change with birth parameters pi, q and reproductive age k.
Note that change of pi, q and k does have an impact on the total number N and N∗h, though they don't influence the ratio N∗h/N.
It can be seen from Eq (4.1) that N increases as pi increases, decreases as q or k increases, so does N∗h for all 1≤h≤m.
Now we study the impact of changes of pi, q and k on the infant disease rate I∗1N∗1.
In the last section, we get that when R0>1, there is a endemic equilibrium of system 3.2 where I∗1 satisfies ∑mi=1λiN∗iNNli+l1M1=1 where li=σ+μiαi and Mi=N∗iI∗i−1. By taking derivative with respect to q, we get
m∑i=1λiN∗iNdNdq(li+l1M1)+l1M21dM1dqλiN∗i(li+l1M1)2=0 |
So
dM1dq=−m∑i=1λiN∗iNdNdq(li+l1M1)(li+l1M1)2/m∑i=1l1M21λiN∗i(li+l1M1)2>0 | (4.5) |
which means that N∗1I∗1 increases as q increases, so I∗1N∗1 decreases as q increases.
Similarly, we have
dM1dpi=−m∑i=1λiN∗iNdNdpi(li+l1M1)(li+l1M1)2/m∑i=1l1M21λiN∗i(li+l1M1)2<0 | (4.6) |
which means that N∗1I∗1 decreases as pi increases, so I∗1N∗1 increases as pi increases.
If k gets larger, N gets smaller as discussed above, N∗iN doesn't change, since we have ∑mi=1λiN∗iNNli+l1M1=1, l1M1 decreases thus M1=N∗1I∗1 increases. So I∗1N∗1 gets smaller. By the same argument, if k gets smaller, I∗1N∗1 gets larger.
In conclusion, we have
Proposition 11. Assume that the birth rate of age group i is given by bi(Ni)=piNie−qN. With all the other parameters fixed, the infant disease rate at endemic equilibrium I∗1N∗1 increases as birth rate pi increases, and decreases as the productive age k or q increases.
In this section, we assume that R0>1 and the birth rate of age group i is given by bi(Ni)=piNie−qN, we'll analyze how does disease distribution I∗iI∗ at endemic equilibrium change with birth parameters pi and q.
From Eq (3.6) we define H:=αh(N∗h/I∗h−1)σ+μh for any 1≤h≤m, then
I∗h=αh(σ+μh)H+αhN∗hNN | (5.1) |
and
I∗=m∑j=1I∗j=m∑j=1αj(σ+μj)H+αjN∗jNN | (5.2) |
Therefore,
I∗hI∗=αh(σ+μh)H+αhN∗hN∑mj=1αj(σ+μj)H+αjN∗jN | (5.3) |
Let Qh:=∑mj=1αj(σ+μj)((σ+μj)H+αj)2N∗jN−σ+μh(σ+μh)H+αh∑mj=1αj(σ+μj)H+αjN∗jN
Proposition 12. Assume that R0>1 and the birth rate of age group i is given by bi(Ni)=piNie−qN. With all the other parameters fixed, how I∗hI∗ changes with q, pi and k depends on the sign of Qh: I∗hI∗ increases as q increases if Qh>0 and decreases as q increases if Qh<0; I∗hI∗ decreases as pi increases if Qh>0 and increases as pi increases if Qh<0. In particular, if 1≤h≤m is such that αhσ+αh<αjσ+αj for all j≠h, I∗hI∗ decreases as q increases and increases as pi increases; if 1≤h≤m is such that αhσ+αh>αjσ+αj for all j≠h, I∗hI∗ increases as q increases and decreases as pi increases.
Proof. From Eq (5.3) and the conclusion we get that N∗jN doesn't change with q, pi or k
d(I∗hI∗)/dq=d(αh(σ+μh)H+αhN∗hN)/dq∑mj=1αj(σ+μj)H+αjN∗jN−d(∑mj=1αj(σ+μj)H+αjN∗jN)/dqαh(σ+μh)H+αhN∗hN(∑mj=1αj(σ+μj)H+αjN∗jN)2=−αh((σ+μh)H+αh)2N∗hN(σ+μh)dHdq∑mj=1αj(σ+μj)H+αjN∗jN+∑mj=1αj((σ+μj)H+αj)2N∗jN(σ+μj)dHdqαh(σ+μh)H+αhN∗hN(∑mj=1αj(σ+μj)H+αjN∗jN)2=(∑mj=1αj(σ+μj)((σ+μj)H+αj)2N∗jN−σ+μh(σ+μh)H+αh∑mj=1αj(σ+μj)H+αjN∗jN)αh(σ+μh)H+αhdHdqN∗hN(∑mj=1αj(σ+μj)H+αjN∗jN)2=Qhαh(σ+μh)H+αhdHdqN∗hN(∑mj=1αj(σ+μj)H+αjN∗jN)2 |
From last section d(N∗hI∗h)/dq>0, which implies that dHdq>0. So we have d(I∗hI∗)/dq>0 if Qh>0 and d(I∗hI∗)/dq<0 if Qh<0, which means that I∗hI∗ increases as q increases if Qh>0 and decreases as q increases if Qh<0. In particular, if 1≤h≤m is such that αhσ+αh<αjσ+αj for all j≠h, then σ+μh(σ+μh)H+αh>σ+μj(σ+μj)H+αj, which implies that Qh<0 thus d(I∗hI∗)/dq<0, I∗hI∗ decreases as q increases; if 1≤h≤m is such that αhσ+αh>αjσ+αj for all j≠h, then σ+μh(σ+μh)H+αh<σ+μj(σ+μj)H+αj, which implies that Qh>0 thus d(I∗hI∗)/dq>0, I∗hI∗ increases as q increases.
Similarly, we have
d(I∗hI∗)/dpi=Qhαh(σ+μh)H+αhdHdpiN∗hN(∑mj=1αj(σ+μj)H+αjN∗jN)2 |
From last section we have d(N∗hI∗h)/dpi<0, which implies that dHdpi<0. So we have d(I∗hI∗)/dpi<0 if Qh>0 and d(I∗hI∗)/dpi>0 if Qh<0, which means that I∗hI∗ decreases as pi increases if Qh>0 and increases as pi increases if Qh<0. In particular, if 1≤h≤m is such that αhσ+αh<αjσ+αj for all j≠h, then σ+μh(σ+μh)H+αh>σ+μj(σ+μj)H+αj, which implies that Qh<0 thus d(I∗hI∗)/dpi>0, I∗hI∗ increases as pi increases; if 1≤h≤m is such that αhσ+αh>αjσ+αj for all j≠h, then σ+μh(σ+μh)H+αh<σ+μj(σ+μj)H+αj, which implies that Qh>0 thus d(I∗hI∗)/dpi<0, I∗hI∗ decreases as pi increases.
In this section, we study how family planning strategies influence demographic distribution at equilibrium, basic reproduction number and infant disease rate.
For simplicity, we assume that there are only two productive age groups, the kth and (k+1)th group. We also assume that the birth function takes a more general form bi(Ni)=piq(Nk+Nk+1)Ni for i=k,k+1, where q is a decreasing function. Since the maximal children each female has per unit time in age group i, given by pi, are dependent on each other, more precisely, pk+pk+1=b for some constant b, which is the maximal children each female has per unit time, we assume that pk=bα and pk+1=b(1−α), then α indicates the tendency to have children at an earlier age. We study how α influence demographic distribution at equilibrium, basic reproduction number and infant disease rate.
From Eq (2.4), we have
N∗1=1μ1(bαq(N∗k+N∗k+1)N∗k+b(1−α)q(N∗k+N∗k+1)N∗k+1)(1−e−μ1τ1)N∗h=1μh(bαq(N∗k+N∗k+1)N∗k+b(1−α)q(N∗k+N∗k+1)N∗k+1)e−h−1∑j=1μjτj(1−e−μhτh)for1<h<mN∗m=1μm(bαq(N∗k+N∗k+1)N∗k+b(1−α)q(N∗k+N∗k+1)N∗k+1)e−m−1∑j=1μjτj | (6.1) |
Further calculation gives
Q=μ1N∗11−e−μ1τ1=μhN∗he−h−1∑j=1μjτj(1−e−μhτh)=μmN∗me−m−1∑j=1μjτj |
where
Q=bαq(N∗k+N∗k+1)N∗k+b(1−α)q(N∗k+N∗k+1)N∗k+1 |
This implies that α doesn't influence the demographic distribution at equilibrium.
Define
ai,k=μke−∑i−1j=1μjτj(1−e−μiτi)μie−∑k−1j=1μjτj(1−e−μkτk) |
for 1<i,k<m. By plugging bi(Ni)=piq(Nk+Nk+1)Ni into the equation for N∗k in Eq (6.1), we obtain
q((1+ak+1,k)N∗k)=μk(bα+b(1−α)ak+1,k)e−∑k−1j=1(1−e−μkτk) | (6.2) |
Define F(α):=bα+b(1−α)ak+1,k, then F′(α)=b(1−ak+1,k). From Eq (6.2), we have the following conclusions: If ak+1,k>1, then F′(α)<0, q is monotone increasing with respect to α, thus N∗k is monotone decreasing with respect to α; if ak+1,k<1, then F′(α)>0, q is monotone decreasing with respect to α, thus N∗k is monotone increasing with respect to α; if ak+1,k=1, then F′(α)=0, q doesn't change with α, thus N∗k doesn't change with α.
Note that the basic reproduction number is given by
R0=m∑i=1αiλiN∗iσ+μi=m∑i=1αiλiN∗iN∗kσ+μiN∗k | (6.3) |
So we have the following proposition.
Proposition 13. If ak+1,k>1, R0 decreases as α increases; if ak+1,k<1, R0 increases as α increases; if ak+1,k=1, R0 doesn't change with α.
Proposition 13 implies that if ak+1,k>1, the basic reproduction number decreases when more people are having children at an early age, if ak+1,k<1, the basic reproduction number increases when more people are having children at an early age, if ak+1,k=1, the basic reproduction number doesn't depend on tendency on birth age.
From previous calculation, we have
m∑i=1λiN∗iN∗kNk∗li+l1M1=1 | (6.4) |
where li=σ+μiαi, M1=N∗1I∗1−1. It implies that I∗1N∗1 is monotone increasing with respect to N∗k. So we have the following
Proposition 14. If ak+1,k>1, I∗1N∗1 decreases as α increases; if ak+1,k<1, I∗1N∗1 increases as α increases; if ak+1,k=1, I∗1N∗1 doesn't change with α.
Proposition 14 implies that if ak+1,k>1, the infant disease rate decreases when more people are having children at an early age, if ak+1,k<1, the infant disease rate increases when more people are having children at an early age, if ak+1,k=1, the infant disease rate doesn't depend on tendency of birth age.
We proposed a stage-structured model of childhood infectious disease transmission dynamics. The population demographics dynamics is governed by a certain family and population planning strategy which gives rise to nonlinear feedback delayed effects on the reproduction ageing and rate.
The long-term aging-profile of the population is described by the pattern and stability of equilibrium of the demographic model. For this demographic model, conditions on the birth functions and death rate were given to guarantee the existence and stability of the positive equilibrium. This implies conditions on birth function and age dependent death rate to reach a stable population. We also investigate the disease transmission dynamics, using the epidemic model when the population reaches the positive equilibrium (limiting equation). We establish conditions for the existence, uniqueness and global stability of the disease endemic equilibrium and prove the global stability of the endemic equilibrium for the original epidemic model with varying population demographics. Birth function, age-dependent death rate, recover rate and transmission coefficients are all involved in these conditions.
The global stability of the endemic equilibrium allows us to examine the effects of reproduction ageing and rate, under different family planning strategies, on the childhood infectious disease transmission dynamics. We find that increasing birth rate increases the infant disease rate and reproduction aging decreases the infant disease rate. We also find that reproduction ageing and rate doesn't change the demographic distribution at equilibrium.
We investigate impacts of family planning strategies on demographic distribution at equilibrium, basic reproduction number for childhood disease and infant disease rate. We find the conditions under which planning to have a child at an early age helps to decrease/increase the basic reproduction number and infant diseases rate. We also examine demographic distribution, diseases reproductive number, infant disease rate and age distribution of disease.
For original contributions, the model we propose is new as it is stage structured and the growth through age stages is described by time delay leading to nonlinear feedback, the idea of studying the impact of population policy and family planning strategy on disease transmission dynamics is also novel. This model can be modified to fit specific childhood diseases for specific purposes. For example, it can be modified to study the impact of China's second-child policy on pertussis transmission dynamics by incorporating more compartments to distinguish children from one-child and two-children families. The work can also be potentially used to inform targeted age group for optimal vaccine booster programs.
The authors declare no conflict of interest.
[1] | G. Casey, O. Galor, Population and Demography Perspective Paper, Copenhagen Consensus Center, 2014. |
[2] | S. Basten, Q. Jiang, Fertility in China: an uncertain future, Popul. Stud., 69 (2015), S97-S105. |
[3] | Q. Ke, L. Zhang, C. He, Z. Zhao, M. Qi, R. C. Griggs, et al., China's shift from population control to population quality, Neurology, 87 (2016), e85-e88. |
[4] | X. Yuan, The transformation and deduction of Chinese population, Chin. J. Popul. Sci., 01 (2000), 40-45. |
[5] | SINA, Spokesperson of the one-child policy committee: 11% or more of the population may have two children, 2007. Available from: http://news.sina.com.cn/c/2007-07-10/154513416121.shtml. |
[6] | China Daily, Most Chinese provincial areas relax one-child policy, 2014. Available from: http://www.chinadaily.com.cn/china/2014-07/10/content17706811.htm. |
[7] | BBC, China to end one-child policy and allow two, 2018. Available from: https://www.bbc.com/news/world-asia-34665539. |
[8] | D. Tang, The Times, China to scrap family planning rules as birthrate dwindles, 2018. Available from: https://www.thetimes.co.uk/article/china-to-scrap-family-planning-rulesas-birthrate-dwindles-x82kccgl3. |
[9] | S. Li, C. Ma, L. Hao, Q. Su, Z. An, F. Ma, et al., Demographic transition and the dynamics of measles in six provinces in China: A modeling study, PLoS Med., 14 (2017), e1002255. |
[10] | C. Connolly, R. Keil, S. H. Ali, Extended urbanisation and the spatialities of infectious disease: Demographic change, infrastructure and governance, Urban Stud., (2020), 1-19. |
[11] |
L. Gao, H. Hethcote, Simulations of rubella vaccination strategies in China, Math. Biosci., 202 (2006), 371-385. doi: 10.1016/j.mbs.2006.02.005
![]() |
[12] |
M. Iannelli, P. Manfredi, Demographic Change and Immigration in Agestructured Epidemic Models, Math. Popul. Stud., 14 (2007), 169-191. doi: 10.1080/08898480701426241
![]() |
[13] | N. Geard, K. Glass, J. M. McCaw, E. S. McBryde, K. B. Korb, M. J. Keeling, et al., The effects of demographic change on disease transmission and vaccine impact in a household structured population, Math. Popul. Stud., 13 (2015), 56-64. |
[14] | M. P. Dafilis, F. Frascoli, J. McVernon, J. M. Heffernan, J. M. McCaw, The dynamical consequences of seasonal forcing, immune boosting and demographic change in a model of disease transmission, J. Theor. Biol., 361 (2014), 124-132. |
[15] |
S. A. McDonald, A. van Lier, D. Plass, M. EE Kretzschmar The impact of demographic change on the estimated future burden of infectious diseases: examples from hepatitis B and seasonal influenza in the Netherlands, BMC Public Health, 12 (2012), 1046. doi: 10.1186/1471-2458-12-1046
![]() |
[16] |
P. van den Driessche, J. Watmough, Reproduction numbers and sub-threshold endemic equilibria for compartmental models of disease transmission, Math. Biosci., 180 (2002), 29-48. doi: 10.1016/S0025-5564(02)00108-6
![]() |
[17] |
M. W. Hirsch, System of differential equations that are competitive or cooperative II: convergence almost everywhere, SIAM J. Math. Anal., 16 (1985), 423-439. doi: 10.1137/0516030
![]() |
[18] |
K. Mischaikov, H. Smith, H. R. Thieme, Asymptotically autonomous semiflows: chain recurrence and Lyapunov functions, Trans. Am. Math. Soc., 347 (1995), 1669-1685. doi: 10.1090/S0002-9947-1995-1290727-7
![]() |
1. | Jingsong Wang, Zhen Tao, Kai Zhang, Shuai Wang, Weiguo Li, Infection Control-Based Construction of a Fever Outpatient Routine Management Model, 2022, 2022, 2090-2859, 1, 10.1155/2022/2902800 |