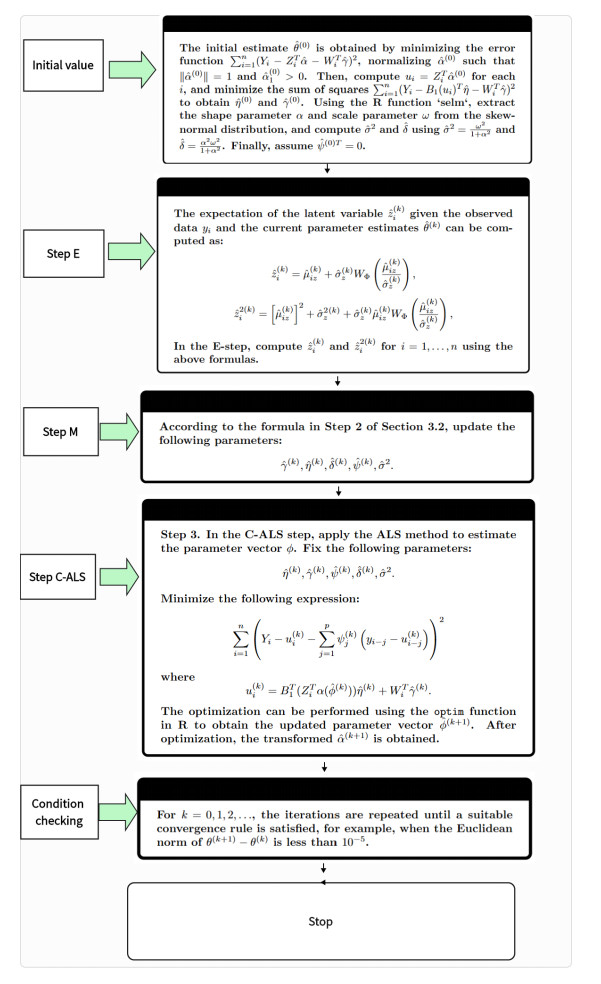
This study investigates the conditional Hyers–Ulam stability of a first-order nonlinear h-difference equation, specifically a discrete logistic model. Identifying bounds on both the relative size of the perturbation and the initial population size is an important issue for nonlinear Hyers–Ulam stability analysis. Utilizing a novel approach, we derive explicit expressions for the optimal lower bound of the initial value region and the upper bound of the perturbation amplitude, surpassing the precision of previous research. Furthermore, we obtain a sharper Hyers–Ulam stability constant, which quantifies the error between true and approximate solutions, thereby demonstrating enhanced stability. The Hyers–Ulam stability constant is proven to be in terms of the step-size h and the growth rate but independent of the carrying capacity. Detailed examples are provided illustrating the applicability and sharpness of our results on conditional stability. In addition, a sensitivity analysis of the parameters appearing in the model is also performed.
Citation: Douglas R. Anderson, Masakazu Onitsuka. A discrete logistic model with conditional Hyers–Ulam stability[J]. AIMS Mathematics, 2025, 10(3): 6512-6545. doi: 10.3934/math.2025298
[1] | Musong Gu, Chen Peng, Zhao Li . Traveling wave solution of (3+1)-dimensional negative-order KdV-Calogero-Bogoyavlenskii-Schiff equation. AIMS Mathematics, 2024, 9(3): 6699-6708. doi: 10.3934/math.2024326 |
[2] | Yanxia Hu, Qian Liu . On traveling wave solutions of a class of KdV-Burgers-Kuramoto type equations. AIMS Mathematics, 2019, 4(5): 1450-1465. doi: 10.3934/math.2019.5.1450 |
[3] | Yuanqing Xu, Xiaoxiao Zheng, Jie Xin . New non-traveling wave solutions for (3+1)-dimensional variable coefficients Date-Jimbo-Kashiwara-Miwa equation. AIMS Mathematics, 2021, 6(3): 2996-3008. doi: 10.3934/math.2021182 |
[4] | Hussain Gissy, Abdullah Ali H. Ahmadini, Ali H. Hakami . The solitary wave phenomena of the fractional Calogero-Bogoyavlenskii-Schiff equation. AIMS Mathematics, 2025, 10(1): 420-437. doi: 10.3934/math.2025020 |
[5] | Yunmei Zhao, Yinghui He, Huizhang Yang . The two variable (φ/φ, 1/φ)-expansion method for solving the time-fractional partial differential equations. AIMS Mathematics, 2020, 5(5): 4121-4135. doi: 10.3934/math.2020264 |
[6] | Hayman Thabet, Subhash Kendre, James Peters . Travelling wave solutions for fractional Korteweg-de Vries equations via an approximate-analytical method. AIMS Mathematics, 2019, 4(4): 1203-1222. doi: 10.3934/math.2019.4.1203 |
[7] | M. Ali Akbar, Norhashidah Hj. Mohd. Ali, M. Tarikul Islam . Multiple closed form solutions to some fractional order nonlinear evolution equations in physics and plasma physics. AIMS Mathematics, 2019, 4(3): 397-411. doi: 10.3934/math.2019.3.397 |
[8] | Xiaoli Wang, Lizhen Wang . Traveling wave solutions of conformable time fractional Burgers type equations. AIMS Mathematics, 2021, 6(7): 7266-7284. doi: 10.3934/math.2021426 |
[9] | Feiting Fan, Xingwu Chen . Dynamical behavior of traveling waves in a generalized VP-mVP equation with non-homogeneous power law nonlinearity. AIMS Mathematics, 2023, 8(8): 17514-17538. doi: 10.3934/math.2023895 |
[10] | Chun-Ku Kuo, Dipankar Kumar, Chieh-Ju Juan . A study of resonance Y-type multi-soliton solutions and soliton molecules for new (2+1)-dimensional nonlinear wave equations. AIMS Mathematics, 2022, 7(12): 20740-20751. doi: 10.3934/math.20221136 |
This study investigates the conditional Hyers–Ulam stability of a first-order nonlinear h-difference equation, specifically a discrete logistic model. Identifying bounds on both the relative size of the perturbation and the initial population size is an important issue for nonlinear Hyers–Ulam stability analysis. Utilizing a novel approach, we derive explicit expressions for the optimal lower bound of the initial value region and the upper bound of the perturbation amplitude, surpassing the precision of previous research. Furthermore, we obtain a sharper Hyers–Ulam stability constant, which quantifies the error between true and approximate solutions, thereby demonstrating enhanced stability. The Hyers–Ulam stability constant is proven to be in terms of the step-size h and the growth rate but independent of the carrying capacity. Detailed examples are provided illustrating the applicability and sharpness of our results on conditional stability. In addition, a sensitivity analysis of the parameters appearing in the model is also performed.
With advances in information technology, functional data collected as curves or images is common in econometrics and biomedicine. However, the classical statistical method does not perform well when applied directly to such data, which has driven the development of functional data analysis (FDA). Researchers have extensively studied the functional regression model as an essential part of the FDA. For example, Cardot et al. [1] introduced the functional linear model, and due to the flexibility of semi-parametric models, significant research on functional semi-parametric regression has been conducted since 2008. For instance, Şentürk et al. [2] extended the traditional varying-coefficient model to functional data, proposing the functional varying-coefficient model. Aneiros-Perez et al. [3] proposed the semi-functional partially linear model, combining the advantages of semi-linear modeling and the nonparametric form of functional data. Zhou et al. [4] introduced the semi-functional linear model, studied the spline estimation of functional coefficients and nonparametric functions, and derived the convergence rate of these spline estimates.
When the dimensionality of multivariate covariates becomes excessively high, the curse of dimensionality inevitably emerges. The single-index model captures the key features of high-dimensional data by searching for a univariate index of multivariate covariates, modeling the effects of the covariates in a nonparametric manner. Härdle et al. [5] applied the single-index model to discrete choice analysis in econometrics and dose-response modeling in biostatistics. Zou et al. [6] studied the M-estimators for the single-index model, approximated the unknown link function with B-splines, and obtained the M-estimators for both the parametric and nonparametric components in a single step. They also proved the asymptotic normality of the estimators. In functional data analysis, to avoid the curse of dimensionality while preserving the advantages of nonparametric smoothing, Yu et al. [7] combined the single-index model with functional linear regression models and proposed the single-index partially functional linear regression model.
However, the above functional regression models assume that the errors are independent. In reality, data often exhibits autocorrelation, such as in financial series or photovoltaic system outputs. For regression analysis of such data, it is necessary to assume that the errors are autocorrelated. To address this issue, Dabo-Niang et al. [8] proposed the functional semi-parametric partially linear model with autoregressive errors and obtained estimates of these coefficients using generalized least squares, proving the consistency and asymptotic normality of the estimators. Yang et al. [9] proposed a robust estimation method for the semi-functional linear model with autoregressive errors. Xiao et al. [10] introduced the partial functional linear model with autoregressive errors, estimated multivariate regression parameters, and functional regression coefficients using generalized least squares, and proved the asymptotic normality of multivariate coefficients and the optimal convergence rate of the functional regression parameters. When the dimensionality of multivariate covariates in a partially functional linear model becomes excessively high, the curse of dimensionality often arises. To address this issue, this paper introduces a single-index partially functional linear model with autoregressive errors for analyzing autocorrelated data.
The research above assumes that the error terms follow a normal distribution. However, in practice, the data may show skewness. To address this skewness issue, Azzalini et al. [11] proposed the skew-normal (SN) distribution, which accommodates skewness and includes the normal distribution as a particular case. Xiao et al. [12] proposed a new asymmetric Huber regression (AHR) estimation method for analyzing the partially functional linear models with skewed data and derived the asymptotic properties of the proposed estimator. In multivariate data analysis, Ferreira et al. [13] proposed an estimation method for the partially linear model with first-order autoregressive (AR(1)) skew-normal errors and conducted local influence analysis. Liu et al. [14] introduced a Bayesian local influence method for detecting influential observations in the partially linear model with first-order autoregressive skew-normal errors and performed local influence analysis. Ferreira et al. [15] extended the autoregressive order to p-order, proposing the partially linear model with p-order autoregressive (AR) skew-normal errors and deriving the maximum likelihood estimator using the EM algorithm. We extend this autoregressive error distribution to models involving functional data. We investigate the parameter estimation problem for the single-index partially functional linear model with p-order autoregressive skew-normal errors.
In the study of parameter estimation for functional data models with autoregressive errors, Chen et al. [16] investigated a functional partial linear model with autoregressive errors, used weighted least squares to estimate spline coefficients, and derived theoretical properties such as the convergence rates of the function regression parameters and nonparametric function estimates for scalar predictor variables. Wang et al. [17] studied a multivariate functional linear model with autoregressive errors, employed least squares to estimate the function coefficients and autoregressive coefficients, and proved the asymptotic properties of the proposed estimators. Inspired by the study of Ferreira et al. [15], this paper proposes a parameter estimation method based on the EM algorithm for the single-index partially functional linear model with p-order autoregressive skew-normal errors. Due to the constraints on the single-index vector, the standard EM algorithm cannot be directly applied. Therefore, a new EM algorithm is proposed, which introduces a conditional adaptive least squares (CALS) step after the conventional EM steps. This step handles the constraint issue through reparameterization and uses the adaptive least squares method to estimate the single-index vector.
The main objective of sensitivity analysis is to assess the impact of perturbations in the model or data on parameter estimates. A commonly used method is case deletion, which evaluates the influence of each observation on the parameter estimates. However, this method does not directly capture the impact of other perturbations in the model. To address this limitation, Cook [18] proposed the local influence method, which studies the sensitivity of the log-likelihood to small perturbations in parts of the model and is computationally simpler. Building upon Cook's pioneering work, Zou et al. [19] extended this method to partially linear single-index models. In the study of autocorrelated data, Ferreira et al. [13] used the EM algorithm to estimate the unknown parameters in partially linear models with first-order autoregressive AR(1) skew-normal errors and conducted a local influence analysis using the conditional expectation of the complete-data log-likelihood function. Inspired by these works, we extend the local influence method to the single-index partially functional linear regression models with p-order autoregressive skew-normal errors.
The structure of this paper is as follows. Section 2 describes in detail the single-index partially functional linear model with p-order autoregressive skew-normal errors. Section 3 introduces the proposed EM-CALS algorithm, compares it with the TSILS, and presents a residual analysis based on conditional quantiles. Section 4 conducts local influence analysis using the Q-function in the EM algorithm. Section 5 evaluates the efficiency of the EM-CALS algorithm through simulation studies. Section 6 demonstrates the application of the proposed method using actual data from grid-connected photovoltaic systems. Section 7 provides some discussions and summarizes the contributions of this paper.
Common statistical regression models often assume that the error terms follow normal or other symmetric distributions. However, in practice, data often exhibits skewness. If a symmetric distribution assumption is maintained in such cases, it may lead to inefficient estimates. To better reflect the characteristics of the data, it is more reasonable to assume a skewed distribution, such as the skew-normal distribution, for the error terms, which can improve the model's estimation performance. The following section provides an introduction to skew-normal distribution.
The SN distribution was proposed by Azzalini [11], which is an effective extension of the normal distribution. The skewness coefficient of the skew-normal distribution ranges from −0.995 to 0.995, and the maximum kurtosis can reach 3.869. By introducing the skewness parameter, this distribution can flexibly accommodate skewed data, making it suitable for a wider range of data modeling scenarios. In the parameter estimation below, we refer to the method of Ferreira et al. [15] and propose the EM-CALS algorithm for estimating unknown parameters and functions. We adopt the parameterized form of the skew-normal distribution proposed by Sahu et al. [20] to facilitate the direct use of analytical expressions in the E and M steps.
We will briefly introduce the Sahu-type skew-normal distribution and discuss some of its properties, which will be used in the EM-CALS algorithm.
The probability density function (pdf) and cumulative distribution function (cdf) of the Sahu-type skew-normal distribution are as follows:
f(y∣μ,σ2,δ)=2√σ2+δ2ϕ(y−μ√σ2+δ2)Φ(δσy−μ√σ2+δ2), | (2.1) |
FY(y;μ,σ2,δ)=2Φ((y−μ√σ2+δ2,0);0,Ω), | (2.2) |
where μ is the location parameter, σ2 is the scale parameter, and δ is the skewness parameter. ϕ and Φ are the pdf and cdf of the standard normal distribution N(0,1), respectively, Ω=(1−δ1−δ11), where δ1=δ√σ2+δ2. If the random variable Y follows a skew-normal distribution with parameters μ,σ2,δ, we denote it as Y∼SN(μ,σ2,δ). The skew-normal distribution simplifies to the normal distribution when δ=0.
If Y∼SN(μ,σ2,δ), the expectation and variance of Y are as follows:
E[Y]=μ+bδ,Var(Y)=σ2+(1−b2)δ2, | (2.3) |
where b=√2π.
Additionally, the stochastic representation of Y is given by Yd=μ+δ|X0|+σX1, where X0 and X1 are independent random variables N(0,1).
In regression analysis of autocorrelated data, autoregressive error structures are commonly used for modeling. Traditional studies often assume that the errors follow a normal distribution. However, when the data exhibits skewness, setting the error distribution to a skewed distribution, such as the skew-normal distribution, can effectively improve the efficiency of parameter estimation.
The single-index model, as a dimensionality reduction method, was combined with the functional linear model by Yu et al. [7] to propose the single-index partially functional linear model. In this model, the error structure is based on autoregressive errors under the skew-normal distribution when modeling autocorrelated data with skewness.
The single-index partially functional linear model with AR(p) skew-normal errors, denoted as SIPFLM-SNAR(p), is defined as follows:
yi=g(Z⊤iα)+∫Tβ(t)Xi(t)dt+εi,εi=ei+p∑j=1ψjεi−j,ei∼SN(−bδ,σ2,δ), | (2.4) |
let yi represent the observed response values for i=1,2,…,n, and Zi denote an l -dimensional vector of covariates. The parameter vector α=(α1,…,αl)⊤ is an unknown vector satisfying the constraint ‖α‖=1. For identification purposes, we assume that the first component of α1 is positive. The function g(⋅) is an unknown univariate link function, and {X(t):t∈T} represents a zero-mean random element in the Hilbert space H=L2(T), where H denotes the space of all square-integrable functions on T. The inner product in H is defined as ⟨x,y⟩=∫Tx(t)y(t)dt for any x,y∈H, with the corresponding norm ‖x‖=⟨x,x⟩1/2. The autoregressive parameters are denoted as ψ1,…,ψp, and the error terms ei are independent random variables, each following a skew-normal distribution with zero mean and constant variance, as described in Eq (2.3). We assume that ε0=ε−1=⋯=ε−(p−1)=0. When ψ1=⋯=ψp=0 and δ=0, model (2.4) reduces to the single-index partially functional linear model as discussed by Yu et al. [7]. To define the function g(⋅), we let its domain be [a1,b1], where a1 and b1 are the infimum and supremum of the set {Z⊤α}, respectively. For simplicity, we assume that T=[0,1].
Following the approach of Ferreira et al. [15], for i=1,…,n, we assume that
Yi∣(yi−1,…,yi−p)∼SN(ui+p∑j=1ψj(yi−j−ui−j)−bδ,σ2,δ), |
where ui=g(Z⊤iα)+∫10β(t)Xi(t)dt.
Given the flexibility and local control of the B-spline, we choose to represent the smooth functions g(u) and β(t) using B-splines (deBoor [21]). First, consider g(u), which is expressed as
g(u)≈N1∑j=1B1j,l1(u)ηj, |
where B1j,l1(u) denotes the normalized B-spline basis function of degree l1, and ηj are the coefficients to be estimated.
B1j,0(u)={1,κj≤u<κj+1,0,otherwise, |
and
B1j,l1(u)=wjl1B1j,l1−1(u)+(1−wj+1,l1)B1j+1,l1−1(u),l1>0, |
where wjl1(u)=u−κjκj+l1−κj. To approximate the link function g(⋅), we partition the interval [a1,b1] as a1=κ0<κ1<⋯<κk1+1=b1, and use κi as the knots. We use N1=k1+l1+1 as the normalized B-spline basis functions of degree l1 to approximate g(⋅), forming a linear spline space. Following the idea of Yu et al. [7], the basis functions are defined using a cubic B-spline with evenly distributed knots, where l1=3. In this case, we have the approximation: g(u)≈∑N1j=1B1j,3(u)ηj. To simplify, we let B1j=B1j,3(u), and, thus, the approximation becomes: g(u)≈∑N1j=1B1j(u)ηj.
We place these basis functions into the vector B1(u)=(B11(u),…,B1N1(u))⊤. Then, we approximate g(⋅) on the interval [a1,b1] using B1(⋅). Similarly, we approximate slope function β(t) using the same method. Let B2(t)=(B21(t),…,B2N2(t))⊤ be the vector of normalized B-spline basis functions of degree l2 on the interval [0,1], containing k2 internal knots, where N2=k2+l2+1. Thus, we have β(t)≈∑N2j=1B2j,l2(u)γj. This can be rewritten in matrix form as
g(u)≈B⊤1(u)η,β(t)≈B⊤2(t)γ, |
where η=(η1,…,ηN1)⊤ and γ=(γ1,…,γN2)⊤.
To estimate the slope function β(⋅) and the link function g(⋅), we employ B-spline approximation. First, the degrees of spline functions must be determined. Inspired by the idea of Huang et al. [22], cubic splines with equally spaced knots are selected. The choice of the number of knots and basis functions is also crucial. Too many knots and basis functions may increase the complexity of the model, and although the fitting error may decrease, it could lead to overfitting by capturing noise, resulting in unreliable parameter estimates. On the other hand, too few knots and basis functions may fail to capture the complexity of the data, leading to underfitting. We need to select the numbers of basis functions, N1 and N2. For this purpose, the Akaike information criterion (AIC) and Bayesian information criterion (BIC) are commonly used to select the truncation parameters. In this study, the optimal values of N1 and N2 are determined by minimizing the BIC criterion, which is defined as follows:
BIC(N1,N2)=−2L(ˆθ,N1,N2)+(N1+N2+l+p+1)ln(n), |
where L(ˆθ,N1,N2) denotes the log-likelihood function evaluated at ˆθ for fixed N1 and N2.
We define W=⟨X(t),B2(t)⟩=(∫10X(t)B21(t)dt,…,∫10X(t)B2N2(t)dt)⊤, and Wi=⟨Xi(t),B2(t)⟩, ψ=(ψ1,…,ψp)⊤. The model (2.4) simplifies to
yi≈B⊤1(Z⊤iα)η+W⊤iγ+εi,εi=ei+p∑j=1ψjεi−j,ei∼SN(−bδ,σ2,δ),i=1,…,n. | (2.5) |
The unknown parameters in this model are ˜θ=(α⊤,η⊤,γ⊤,ψ⊤,δ,σ2)⊤, where the l -dimensional single-index vector α must satisfy the constraints ‖α‖=1 and α1>0. Inspired by Yu and Ruppert [26], we also handle the constraint on the single-index parameter through reparameterization.
Inspired by the work of Yu et al. [7], let ϕ represent a parameter vector of dimension l−1, and define α=α(ϕ)=(√1−‖ϕ‖2,ϕ⊤)⊤. Given that the true parameter vector ϕ0 must satisfy the constraint ‖ϕ0‖≤1, we assume the strict inequality ‖ϕ0‖<1. As a result, the function α(ϕ) is infinitely differentiable with respect to ϕ. The Jacobian matrix of α(ϕ) with respect to ϕ is expressed as
Jϕ≡(−(1−‖ϕ‖2)−1/2ϕ⊤Il−1), |
where Il is the l×l identity matrix. After reparameterization, the unknown parameters are θ=(ϕ⊤,η⊤,γ⊤,ψ⊤,δ,σ2)⊤.
According to Eq (2.5), for the parameter θ=(ϕ⊤,η⊤,γ⊤,ψ⊤,δ,σ2)⊤∈Rp∗, where ψ=(ψ1,…,ψp) and p∗=N1+N2+l+p+1, the log-likelihood function of the observed data can be expressed as
ℓ(θ)=nlog(2√2π)−n2log(σ2+δ2)−12(σ2+δ2)n∑i=1(yi−ξi+bδ)2+n∑i=1log{Φ(Bi)}, | (3.1) |
where Bi=δσ(σ2+δ2)1/2(yi−ξi+bδ), ξi=B⊤1(Z⊤iα)η+W⊤iγ+∑pj=1ψj(yi−j−B⊤1(Z⊤i−jα)η−W⊤i−jγ), B⊤1(Z⊤iα) and W⊤i represent the i -th row of B1(Z⊤α) and W, respectively.
To estimate the parameter θ, we need to maximize the log-likelihood function. However, directly maximizing this objective function can be challenging, so numerical methods are necessary. Given the stochastic representation of the skew-normal distribution, the EM algorithm is particularly useful for addressing this problem.
The EM algorithm, introduced by Dempster et al. [23], is widely utilized for solving maximum likelihood estimation problems. One of the key advantages of the EM algorithm is its ability to efficiently handle maximum likelihood estimation issues that involve missing data or latent variables through an iterative approach. This capability makes it particularly effective for managing complex models, especially when the data is incomplete or the underlying structure is not well-defined.
Let TN(μ,σ2;0,+∞) represent the truncated normal distribution with parameters μ and σ2, which is supported on the interval (0,+∞) (Johnson et al. [24]). By utilizing the stochastic representation of the Sahu-type skew-normal distribution, we can derive:
Yi∣Zi=zi,yi−1,…,yi−pind∼N(ξi−bδ+δzi,σ2),Ziiid∼TN(0,1;(0,+∞)),i=1,…,n. | (3.2) |
The EM algorithm treats latent variables z=(z1,…,zn)⊤ as unobserved data and y=(y1,…,yn)⊤ as observed data. Using Eq (3.2), we obtain the joint distribution of (Yi,Zi) as
f(yi,zi;yi−1,…,yi−p,θ)=2ϕ(yi∣ξi+δzi−bδ,σ2)ϕ(zi)I(0,+∞)(zi)=2ϕ(yi∣ξi−bδ,σ2+δ2)ϕ(zi∣μiz,σ2z)I(0,+∞)(zi)=f(yi∣yi−1,…,yi−p)f(zi∣yi,yi−1,…,yi−p), | (3.3) |
where μiz=δσ2+δ2(yi−ξi+bδ),σ2z=σ2σ2+δ2; thus, using Eq (3.3), we obtain Zi∣yi,θ∼TN(μiz,σ2z;(0,+∞)).
Using the properties of the truncated normal distribution, we can obtain the following conditional expectation:
E[Zi∣yi]=μiz+σzWΦ(μizσz),E[Z2i∣yi]=μ2iz+σ2z+σzμizWΦ(μizσz), | (3.4) |
where WΦ(u)=ϕ(u)Φ(u).
Using Eq (3.3), the joint distribution of yc=(y,z), we obtain the logarithmic likelihood function for the complete data:
ℓc(θ|yc)=C−n2log(σ2)−12σ2n∑i=1[(yi−ξi)2−2δ(yi−ξi)(zi−b)+δ2(b2−2bzi+z2i)], |
where C is a constant independent of the unknown parameters.
In the EM algorithm, the Q-function is the expectation of the complete logarithmic likelihood function of the data, given the observed data y and the current parameter estimates ˆθ(k). Specifically, it is defined as
Q(θ∣ˆθ(k))=E[ℓc(θ∣yc)∣y,ˆθ(k)]∝−n2log(σ2)−12σ2n∑i=1[(yi−ξi)2−2δ(yi−ξi)(ˆz(k)i−b)+δ2(b2−2bˆz(k)i+^z2(k)i)], |
where ˆz(k)i=E[Zi∣yi,ˆθ(k)] and ^z2i(k)=E[Z2i∣yi,ˆθ(k)].
Based on Eq (3.4), the expectation of the latent variable z given the observed data y and the current parameter estimates ˆθ(k) can be computed as follows:
ˆz(k)i=ˆμ(k)iz+ˆσ(k)zWΦ(ˆμ(k)izˆσ(k)z), | (3.5) |
^z2(k)i=[ˆμ(k)iz]2+^σ2(k)z+ˆσ(k)zˆμ(k)izWΦ(ˆμ(k)izˆσ(k)z), | (3.6) |
for any i=1,…,n, where ˆμ(k)iz=ˆδ(k)^σ2(k)+ˆδ(k)2(yi−ˆξ(k)i+bˆδ(k)), ^σ2(k)z=^σ2(k)^σ2(k)+ˆδ(k)2, ˆξ(k)i=ˆu(k)i+∑pj=1ˆψ(k)j(yi−j−ˆu(k)i−j), ˆu(k)i=B⊤1(Z⊤iα(k))η(k)+W⊤iγ(k).
Let A=A(ψ) be an n×n matrix, given by
A=(100⋯00⋯000−ψ110⋯00⋯000−ψ2−ψ11⋯00⋯000⋮⋮⋮⋱⋮⋮⋱⋮⋮⋮−ψp−ψp−1−ψp−2⋯−ψ11⋯0000−ψp−ψp−1⋯−ψ2−ψ11⋯00⋮⋮⋮⋱⋮⋮⋱⋮⋮⋮0⋯0⋯00⋯−ψ2−ψ11). |
Let ¯θ=(η⊤,γ⊤,ψ⊤,δ,σ2)⊤, where the M-step updates the parameter ¯θ by maximizing Q(θ∣ˆθ(k)), yielding a new estimate ¯θ(k+1), and the i th row of A is A⊤i.
The EM algorithm is preferred for its simplicity and stability. However, when parameters are subject to constraints, maximizing the Q-function can become extremely difficult, complicating the M-step. Keiji Takai [25] proposed a constrained EM algorithm that utilizes a projection method, but challenges still arise when addressing nonlinear constraints in single-index models. In particular, the unit norm constraint ‖α‖=1 requires solving nonlinear equations, calculating gradients, and inverting matrices, which becomes increasingly complex in high-dimensional parameter spaces and can lead to numerical instability, thereby complicating optimization. Furthermore, selecting the appropriate step size in the P-step of the constrained EM algorithm is challenging, especially under high-dimensional nonlinear constraints, where finding a suitable step size is often difficult. This frequently results in slow convergence and reduced efficiency.
For the constraint ‖α‖=1 and α1>0, Yu and Ruppert [26] addressed this issue using a reparameterization approach. Additionally, Yu et al. [7] applied the least squares method to estimate the single-index vector in the single-index partially functional linear model. Inspired by these works, we also use the least squares method to compute the single-index vector. Furthermore, since the least squares method converges faster than the EM algorithm and is simpler for handling nonlinear constraints, we choose to use the least squares method as a substitute for maximum likelihood estimation (MLE). Furthermore, given that the error terms exhibit an autoregressive structure and do not meet the assumption of normality in the error distribution, we employ the ALS to estimate the single-index vector α. The ALS method effectively addresses autocorrelation among the error terms, providing robust parameter estimates by accounting for dependencies among errors. Additionally, ALS can adapt to skewed distributions and nonzero expectations of errors, leading to more accurate and stable parameter estimates. Within the framework of the EM algorithm for this model, the E-step and M-step are utilized to estimate other complex parameters. In contrast, the single-index vector α is estimated by introducing a CALS step. In this CALS step, we estimate ϕ using ALS while holding the current estimates of the other parameters fixed, and then we obtain the estimate of the single-index vector α(ϕ) through a transformation.
Similar to Ferreira et al.[15], for i=1,…,n, we assume that
Yi∣(yi−1,…,yi−p)∼SN(ui+p∑j=1ψj(yi−j−ui−j)−bδ,σ2,δ), | (3.7) |
where ui=B⊤1(Z⊤iα(ϕ))η+W⊤iγ.
Given the current estimates of ¯θ, namely, η=η(t), γ=γ(t), ψ=ψ(t), δ=δ(t), and σ2=σ2(t), and the observed data Y, the ALS method can be employed to estimate the single-index vector α. Using the conditional expectation formula (2.3) for the truncated normal distribution, we can compute its conditional expectation as follows:
ˆYi=E(Yi∣yi−1,…,yi−p,η,γ,ψ,δ,σ2)=ui+p∑j=1ψj(yi−j−ui−j). |
In ALS, the parameter ϕ is obtained by minimizing the sum of squared residuals
ˆϕALS=argminϕn∑i=1U2i, |
where the residual Ui is defined as: Ui=Yi−E(Yi∣yi−1,…,yi−p,η,γ,ψ,δ,σ2). Finally, the estimate of the single-index vector α is obtained by transforming ˆϕALS into α(ˆϕALS).
Step 0. Start from ˆθ(0)=(ˆα(0)⊤,ˆη(0)⊤,ˆγ(0)⊤,ˆψ(0)⊤,ˆδ(0),^σ2(0))⊤, for example, by minimizing ∑ni=1(Yi−Z⊤iα−W⊤iγ)2 and normalizing ˆα(0) such that ‖ˆα(0)‖=1 and ˆα(0)1>0, to obtain the estimate of ˆα(0), where ˆα(0)1 is the first component of ˆα(0). Given the initial single-index vector ˆα(0), we compute {ui=Z⊤iˆα(0),i=1,…,n}. Then, minimize the error sum of squares function:
n∑i=1(Yi−B⊤1(ui)η−W⊤iγ)2, |
to optimize (η⊤,γ⊤)⊤, yielding ˆη(0) and ˆγ(0). In B1(u), the basis functions use k1 equidistant points as nodes within the domain of g(⋅). Once ˆη(0) and ˆγ(0) are obtained, fit r=Y−B1(u)ˆη(0)−Wˆγ(0) using the R function selm, extract the shape parameter α and scale parameter ω from the skew-normal distribution, and use the relationships σ2=ω21+α2 and δ=α2ω21+α2 to obtain ˆδ(0) and ^σ2(0). Assume ˆψ(0)⊤=0. After completing the above steps, the initial estimate is ˆθ(0)=(ˆα(0)⊤,ˆη(0)⊤,ˆγ(0)⊤,ˆψ(0)⊤,ˆδ(0),^σ2(0))⊤.
Step 1. In the E-step, calculate z(k)i and z2(k)i for i=1,…,n using formulas (3.5) and (3.6).
Step 2. In the M-step, update (ˆγ(k)⊤,ˆη(k)⊤,ˆδ(k),ˆψ(k)⊤,ˆσ2(k))⊤. When k>1 : ˆα(k)=α(ˆϕ(k)), and when k=0 : ˆα(0)=ˆα(0). The iteration formulas are as follows:
ˆγ(k+1)=[(ˆA(k)W)⊤(ˆA(k)W)]−1[(ˆA(k)W)⊤(ˆA(k)Y−ˆA(k)B1(Z⊤ˆα(k))ˆη(k)−ˆδ(k)(ˆz(k)−b))],ˆη(k+1)=[(ˆA(k)B1(Z⊤ˆα(k)))⊤(ˆA(k)B1(Z⊤ˆα(k)))]−1[(ˆA(k)B1(Z⊤ˆα(k)))⊤(ˆA(k)Y−ˆA(k)Wˆγ(k)−ˆδ(k)(ˆz(k)−b))],ˆδ(k+1)=∑ni=1(yi−ˆξ(k)i)(ˆz(k)i−b)∑ni=1(b2−2bˆz(k)i+^z2i(k)),ˆψ(k+1)j=1∑ni=1r2i−jn∑i=1[ri−p∑l=1,l≠jˆψ(k)lri−l−ˆδ(k)(ˆz(k)i−b)]ri−j,j=1,…,p,^σ2(k+1)=1nn∑i=1[(yi−ˆξ(k)i)2−2ˆδ(k)(yi−ˆξ(k)i)(ˆz(k)i−b)+ˆδ(k)2(b2−2bˆz(k)i+^z2i(k))], |
where ri=yi−u(k)i=yi−B⊤1(Z⊤iˆα(k))ˆη(k)−W⊤iˆγ(k),i=1,…,n, and r0=r−1=⋯=r−(p−1)=0, ˆz(k)=(ˆz(k)1,ˆz(k)2,…,ˆz(k)n)⊤,fork=0,1,2,…. Finally, the matrix ˆA(k) is generated by the following formula: ˆA(k)=A(ˆψ(k)).
The basis function B1(u) uses k1 equidistant points within the interval [a1,b1], but in practice, the interval [a1,b1] is unknown. We generate the B-spline basis function for each given ˆα(k) using the minimum and maximum values of Z⊤iˆα(k) as boundary points. Beyond this interval, g(⋅) may be defined in any reasonable manner without altering the results.
Step 3. In the CALS step, apply the ALS method to estimate the parameter vector ϕ. Fix (ˆη(k)⊤,ˆγ(k)⊤,ˆψ(k)⊤,ˆδ(k),ˆσ2(k))⊤ and minimize ∑ni=1(Yi−u(k)i−∑pj=1ψ(k)j(yi−j−u(k)i−j))2, where u(k)i=B⊤1(Z⊤iα(ˆϕ(k)))ˆη(k)+W⊤iˆγ(k), to obtain ˆϕ(k+1). This step can be optimized using the optim function in R, and then the transformed ˆα(k+1) is obtained.
Step 4. Repeat Steps 1 to 3 until the convergence criterion is satisfied, where ||¯θ(k+1)−¯θ(k)|| is less than 10−5, and represent the final estimates of α,η,γ,ψ,δ,σ2as ˆα,ˆη,ˆγ,ˆψ,ˆδ,^σ2, respectively.
The following is the flowchart of the EM-CALS algorithm (see Figure 1):
Inspired by the work of Yang et al. [27], we use the TSILS to estimate the unknown parameters in the single-index partially functional linear regression model with p-order autoregressive skew-normal errors. This section compares the proposed EM-CALS algorithm with the TSILS.
The two-step iterative least squares estimation is as follows:
Step 0. Start with the initial estimate ˆ¯θ(0)=(ˆα(0)⊤,ˆη(0)⊤,ˆγ(0)⊤,ˆψ(0)⊤,ˆδ(0),ˆσ2(0))⊤, for example, by minimizing the objective function: ∑ni=1(Yi−Z⊤iα−W⊤iγ)2, and normalizing ˆα(0) such that ‖ˆα(0)‖=1 and ˆα(0)1>0, where ˆα(0)1 is the first component of ˆα(0), to obtain the estimate of ˆα(0). Given the initial single-index vector ˆα(0), we compute {ui=Z⊤iˆα(0),i=1,…,n}. Then, obtain ˆUi=(ˆW⊤i,B⊤i)⊤, and compute ˆθ(0)=(ˆγ(0)⊤,ˆη(0)⊤)⊤ based on the least squares method: θ(0)=(ˆU⊤ˆU)−1ˆU⊤Y, where Y=(Y1,Y2,…,Yn)⊤ and ˆU⊤=(ˆU⊤1,ˆU⊤2,…,ˆU⊤n). Once ˆη(0) and ˆγ(0) are obtained, fit r=Y−B1(u)ˆη(0)−Wˆγ(0) using the R function selm. Extract the shape parameter α and scale parameter ω from the skew-normal distribution, and use the relationships: σ2=ω21+α2,δ=α2ω21+α2 to obtain ˆδ(0) and ˆσ2(0). Assume ˆψ(0)⊤=0. After completing the above steps, the initial estimate is:
ˆ¯θ(0)=(ˆα(0)⊤,ˆη(0)⊤,ˆγ(0)⊤,ˆψ(0)⊤,ˆδ(0),ˆσ2(0))⊤. |
Step 1. Update ˜V(k), ˜H(k):
˜V(k)=(ˆε(k)p+1⋮ˆε(k)n),˜H(k)=(ˆε(k)p⋯ˆε(k)1⋮⋮ˆε(k)n−1⋯ˆε(k)n−p), |
where ˆε(k)t=Yt−ˆU⊤tˆθ(k−1), t=1,2,⋯,n.
Step 2. Compute ˆψ(k):
ˆψ(k)=(˜H(k)⊤˜H(k))−1˜H(k)⊤˜V(k). |
Step 3. Update V(k)t, H(k)t:
V(k)t=Yt−p∑l=1ψ(k)lYt−l,H(k)t=ˆUt−p∑l=1ψ(k)lˆUt−l. |
Step 4. Compute θ(k):
ˆθ(k)=(H(k)⊤H(k))−1H(k)⊤V(k), |
where
V(k)=(V(k)p+1⋮V(k)n),H(k)=(H(k)p+1⋮H(k)n). |
Step 5. In this step, apply the ALS method to estimate the parameter vector ϕ. Fix (ˆη(k)⊤,ˆγ(k)⊤,ˆψ(k)⊤,ˆδ(k),ˆσ2(k))⊤ and minimize ∑ni=1(Yi−u(k)i−∑pj=1ψ(k)j(yi−j−u(k)i−j))2, where u(k)i=B⊤1(Z⊤iα(ˆϕ(k)))ˆη(k)+W⊤iˆγ(k), to obtain ˆϕ(k+1). This step can be optimized using the optim function in R, and then the transformed ˆα(k+1) is obtained.
Step 6. In B1(u), the basis functions use k1 equidistant points as nodes within the domain of g(⋅). Once ˆη(k) and ˆγ(k) are obtained, fit r=Y−B1(u)ˆη(k)−Wˆγ(k) using the R function selm, extract the shape parameter α and scale parameter ω from the skew-normal distribution, and use the relationships σ2=ω21+α2 and δ=α2ω21+α2 to obtain ˆδ(k+1) and ^σ2(k+1).
Step 7. Repeat Steps 1 to 6 until the convergence criterion is satisfied, where ||¯θ(k+1)−¯θ(k)|| is smaller than 10−5.
This section verifies the statistical performance of the proposed algorithm through simulation experiments. The experimental setup is as follows: We generate simulated data with the sample size n=1000 and conduct 100 independent repeated experiments to eliminate randomness. For objective performance evaluation, all comparative experiments are implemented under identical simulated data conditions, with estimation accuracy and computational efficiency quantitatively analyzed through mean square error (MSE) and root MSE (RMSE) metrics.
To evaluate the performance of the proposed algorithm, we compute both the RMSE and the MSE, which are defined as follows:
RMSE=√1nn∑i=1(Yi−ˆYi)2,MSE=1nn∑i=1(Yi−ˆYi)2, |
where Yi is the true value of the i -th observation, ˆYi is the estimated value for the i -th observation, and n is the total number of samples.
To assess the performance of the estimated link function g(⋅) and slope function β(⋅), we adopt the root of the average squared errors (RASE), as introduced by Peng et al. [28], which is defined as follows:
RASE1=(1K1K1∑k=1(ˆg(uk)−g(uk))2)1/2, |
RASE2=(1K2K2∑k=1(ˆβ(tk)−β(tk))2)1/2, |
where {uk,k=1,…,K1} and {tk,k=1,…,K2} are the grid points uniformly distributed on the domains of g(⋅) and β(⋅), respectively. In the two examples below, we choose K1=K2=200.
The dataset in the simulation experiments is generated according to the following model:
Y=sin(u)+∫10β(t)X(t)dt+ε. |
In the single-index component, we use the design of Lin et al. [29], g(u)=sin(u), where u=Z⊤α, α=(0.2,−0.7)⊤√0.53, and let Z=(Z1,Z2)⊤, where Ziiid.∼Unif(0,1), for i=1,2. In the functional linear component, we follow the design of Yu et al. [30], with the slope function set as β(t)=√2sin(πt2)+3√2sin(3πt2), and X(t)=∑50j=1ξjvj(t). Here, ξj follows a normal distribution with mean 0 and variance λj=((j−0.5)π)−2 and vj(t)=√2sin((j−0.5)πt). The random error ε satisfies the model εi=ei+∑pl=1ψlεi−l, where ei∼SN(−bδ,σ2,δ), with δ=1 and σ2=0.2. This part studies the 1-order autoregressive error structure (AR(1)), where we set ψ=0.5.
The computational efficiency of the two algorithms was quantitatively evaluated through average user central processing unit (CPU) time per iteration monitoring. The EM-CALS algorithm demonstrated a mean user time of 80.765 s per iteration, compared to 75.486 s for the TSILS implementation. This observed time difference aligns with the established computational complexity characteristics of EM algorithms versus least squares methods, where EM-based approaches typically require more intensive computations due to their iterative latent variable estimation process.
As shown in Table 1, EM-CALS outperforms TSILS across all metrics, particularly excelling in RASE1 and RASE2. The EM-CALS algorithm shows significant improvement over the TSILS algorithm in terms of the mean values of RMSE, MSE, RASE1, and RASE2, with decreases of 1.19%, 2.45%, 38.00%, and 51.35%, respectively, demonstrating its significant advantage in enhancing prediction accuracy. However, in terms of computational time, TSILS is found to be faster than EM-CALS. Therefore, EM-CALS is better suited for tasks that prioritize accuracy, while TSILS may remain relevant for scenarios where computational efficiency is the primary concern.
RMSE | MSE | RASE1 | RASE2 | ||||||||
Algorithms | Mean | Var | Mean | Var | Mean | Var | Mean | Var | |||
EM-CALS | 0.747 | 0.000 | 0.558 | 0.001 | 0.498 | 0.073 | 0.072 | 0.001 | |||
TSILS | 0.756 | 0.000 | 0.572 | 0.001 | 0.801 | 0.151 | 0.148 | 0.006 |
If the data is correlated, Dunn and Smyth [31] suggested using conditional residuals to ensure the independence and asymptotic normality of the quantile residuals. These residuals can be derived from the conditional residuals defined in model (2.5) as follows:
rqi=yi−B⊤1(Z⊤iˆα)ˆη−W⊤iˆγ−p∑j=1ˆψj(yi−j−B⊤1(Z⊤i−jˆα)ˆη−W⊤i−jˆγ)={yi−B⊤1(Z⊤iˆα)ˆη−W⊤iˆγ,i=1,…,p,yi−B⊤1(Z⊤iˆα)ˆη−W⊤iˆγ−∑pj=1ˆψj(yi−j−B⊤1(Z⊤i−jˆα)ˆη−W⊤i−jˆγ),i=p+1,…,n, |
where Wi=⟨Xi(t),B2(t)⟩.
Using the expression for the cdf of a skew-normal distribution as presented in Eq (2.2), the conditional quantile residual can be defined as follows:
tqi=Φ−1(FY(rqi;−bˆδ,^σ2,ˆδ)),i=1,…,n. |
According to the research by Dunn and Smyth [31], if the parameter θ can be consistently estimated, the distribution of tqi will asymptotically approach a standard normal distribution. Therefore, the conditional quantile residual can be used to analyze deviations from the error assumptions and identify potential outliers.
Inspired by Ferreira's work [13], we use the conditional expectation of the complete-data log-likelihood function to conduct a local influence analysis.
The perturbation model is defined as M={f(yc,θ,ω):ω∈Ω}, where ω=(ω1,…,ωn) represents a perturbation vector that varies within an open region Ω⊂Rn. The function f(yc,θ,ω) is the pdf of the complete data yc perturbed by ω and ℓc(θ,ω∣yc)=logf(yc,θ,ω). Let ˆθ(ω) be the maximum of the function Q(θ,ω∣ˆθ)=E[ℓc(θ,ω∣Yc)∣y,ˆθ]. It is assumed that there exists a ω0 such that ℓc(θ,ω0|Yc)=ℓc(θ|Yc) for all θ. The influence graph is defined as α(ω)=(ω⊤,fQ(ω))⊤, where fQ(ω) is the Q-displacement function, defined as: fQ(ω)=2[Q(ˆθ|ˆθ)−Q(ˆθ(ω)|ˆθ)].
Zhu et al. [33] pointed out that, at the parameter point ω0, the normal curvature CfQ,d of α(w) along the direction of the unit vector d effectively characterizes the local behavior of the Q-displacement function.
The normal curvature CfQ,d of α(w) is defined as:
CfQ,d=−2d⊤¨Qω0dand−¨Qω0=△⊤ω0(−¨Qθ(ˆθ))−1△ω0, |
where ¨Qθ(ˆθ)=∂2Q(θ|ˆθ)∂θ∂θ⊤|θ=ˆθand △ω=∂2Q(θ,ω|ˆθ)∂θ∂ω⊤|θ=ˆθ(ω).
Following Cook's approach, we construct a measure of influence by using the spectral decomposition of the symmetric matrix −2¨Qω0=∑nk=1ξkeke⊤k. Let {(ξ1,e1),…,(ξn,en)} be the eigenvalue-eigenvector pairs of the matrix −2¨Qω0, where the first q eigenvalues satisfy ξ1≥⋯≥ξq>0 and ξq+1=⋯=ξn=0, and {e1,…,en} forms an orthonormal basis. Based on the methods of Zhu et al.[33], the aggregated contribution vector M(0)l is defined as the normalized weighted sum of the components corresponding to all nonzero eigenvalues:
M(0)l=q∑k=1˜ξke2kl, |
where ˜ξk=ξk√∑qj=1ξ2j represents the normalized eigenvalue weights, and e2k=(e2k1,…,e2kn) is the square of the components of the eigenvector ek.
To identify influential cases, we perform a preliminary evaluation by inspecting the graph of {M(0)l,l=1,…,n}. Following Ferreira's approach[13], we use 1n+c∗S as a benchmark, and consider the l -th case as influential if M(0)l exceeds this benchmark. Here, c∗ is a constant selected based on the specific application, and S is the standard deviation of the vector {M(0)l,l=1,…,n}.
Let A be an n×n matrix. The result of ∂A∂ψi is that the elements on the i -th lower off-diagonal are all −1, and the other elements are 0. The result of ∂AT∂ψi is that the elements on the i -th upper off-diagonal are all −1, and the other elements are 0. The k -th row of ∂A∂ψi is ∂Ak⊤∂ψi.
When i=2, the results are shown below:
∂A∂ψ2=[000⋯0000⋯0−100⋯00−10⋯0⋮⋱⋮⋮⋮0⋯−100]n×n, |
∂A⊤∂ψ2=[00−10⋯0000−1⋯0⋮⋮⋮⋮⋱⋮0000⋯−10000⋯00000⋯0]n×n. |
To obtain the diagnostic measures of the SIPFLM-SNAR(P), based on the approach of Zhu and Lee [33], it is necessary to compute ¨Qθ(ˆθ)=∂2Q(θ|ˆθ)∂θ∂θ⊤, where θ=(ηT,γT,σ2,δ,ψT)T. ¨Qθ(ˆθ) has elements given by the following expression:
∂2Q(θ|ˆθ)∂η∂η⊤=−1σ2(AB1(Z⊤α))⊤AB1(Z⊤α),∂2Q(θ∣ˆθ)∂γ∂η⊤=−1σ2(AW)⊤AB1(Z⊤α),∂2Q(θ|ˆθ)∂σ2∂η=−1σ4(AB1(Z⊤α))⊤(y−ξ−δ(ˆz−b1n)),∂2Q(θ|ˆθ)∂δ∂η=−1σ2(AB1(ZTα))⊤(ˆz−b1n),∂2Q(θ|ˆθ)∂ψi∂η=1σ2(AB1(Z⊤α))⊤⋅∂A∂ψir+1σ2B⊤1(Z⊤α)⋅∂A⊤∂ψi(y−ξ−δ(ˆz−b1n)),i=1,…,p,∂2Q(θ|ˆθ)∂γ∂γ⊤=−1σ2(AW)⊤AW, |
∂2Q(θ|ˆθ)∂σ2∂γ=−1σ4(AW)⊤(y−ξ−δ(ˆz−b1n)),∂2Q(θ|ˆθ)∂δ∂γ=−1σ2(AW)⊤(ˆz−b1n),∂2Q(θ|ˆθ)∂ψi∂γ=1σ2(AW)⊤⋅∂A∂ψir+1σ2(W⊤∂A⊤∂ψi)(y−ξ−δ(ˆz−b1n)),i=1,…,p,∂2Q(θ|ˆθ)∂σ4=n2σ4−1σ6n∑i=1[(yi−ξi)2−2δ(yi−ξi)(ˆzi−b)+δ2(b2−2b^zi+^z2i)],∂2Q(θ|ˆθ)∂δ∂σ2=1σ4n∑i=1[−(yi−ξi)(^zi−b)+δ(b2−2b^zi+^z2i)],∂2Q(θ|ˆθ)∂ψi∂σ2=1σ4r⊤(∂A⊤∂ψi)Ar−δσ4r⊤∂A⊤∂ψi(ˆz−b1n),i=1,…,p,∂2Q(θ|ˆθ)∂δ2=−1σ2n∑i=1(b2−2b^zi+^z2i),∂2Q(θ|ˆθ)∂ψi∂δ=1σ2r⊤∂A⊤∂ψi(ˆz−b1n),i=1,…,p,∂2Q(θ|ˆθ)∂ψj∂ψi=−12σ2r⊤(∂A⊤∂ψi⋅∂A∂ψj+∂A⊤∂ψj⋅∂A∂ψi)r,i=1,…,p,j=1,…,p, |
where 1n is a vector of ones, and ξ=(ξ1,…,ξn), r=y−B1(Z⊤α)η−Wγ, ˆz=(ˆz1,…,ˆzn)⊤.
In the above formula, ∂2Q(θ|ˆθ)∂ψi∂η represents the i -th column of ∂2Q(θ|ˆθ)∂ψ⊤∂η, and ∂2Q(θ|ˆθ)∂ψj∂ψi corresponds to the (i,j) -th element of ∂2Q(θ|ˆθ)∂ψ∂ψ⊤; other symbols follow the same pattern.
In this section, we consider the three usual perturbation schemes in local influence for the SIPFLM-SNAR(P) proposed in this work.
This section examines whether differentially weighted observations influence the maximum likelihood estimation of θ. The perturbed Q-function is written as: Q(θ,ω|ˆθ)=∑ni=1ωiQi(θ|ˆθ). In this case, ω0=(1,…,1)=1n and ∂Q(θ,ω|ˆθ)∂ωi=Qi(θ|ˆθ), and △ω0 has elements ∂Qi(θ|ˆθ)∂θ,i=1,…,n.
∂Qi(θ|ˆθ)∂η=1σ2(B⊤1(Z⊤α)Ai)(yi−ξi−δ(^zi−b)),∂Qi(θ|ˆθ)∂γ=1σ2(W⊤Ai)(yi−ξi−δ(^zi−b)),∂Qi(θ|ˆθ)∂σ2=−12σ2+12σ4[(yi−ξi)2−2δ(yi−ξi)(ˆzi−b)+δ2(b2−2bˆzi+^z2i)],∂Qi(θ|ˆθ)∂δ=1σ2[(yi−ξi)(ˆzi−b)−δ(b2−2bˆzi+^z2i)],∂Qi(θ|ˆθ)∂ψk=−1σ2(ATir−δ(ˆzi−b))(∂Ai⊤∂ψk)r,k=1,…,p. |
Inspired by Ferreira's work [13], we consider an additive perturbation given by
yiω=yi+Syωi,i=1,…,n, |
where Sy is the standard deviation of y. In this case, ω0=0:n×1, and by replacing yi with yiω in the Q-function, we obtain Q(θ,ω∣ˆθ).
It follows that the matrix
∂2Q(θ,ω|ˆθ)∂θ∂ω⊤|ω=ω0, |
where
∂2Q(θ,ω∣ˆθ)∂η∂ω⊤|ω=ω0=Syσ2(AB1(ZTα))⊤A,∂2Q(θ,ω∣ˆθ)∂γ∂ω⊤|ω=ω0=Syσ2(AW)⊤A,∂2Q(θ,ω∣ˆθ)∂σ2∂ω|ω=ω0=Syσ4(A⊤Ar−δA⊤(ˆz−b1n)),∂2Q(θ,ω∣ˆθ)∂δ∂ω|ω=ω0=Syσ2A⊤(ˆz−b1n),∂2Q(θ,ω∣ˆθ)∂ψi∂ω|ω=ω0=−Syσ2[(∂A⊤∂ψi⋅A+A⊤⋅∂A∂ψi)r−(δ∂A⊤∂ψi)(ˆz−b1n)]. |
Inspired by the research of Zou et al. [19], we consider the following perturbation.
We perturb Zi as follows: Zi+ljω⊤ki,whereω∈Rn,lj∈Rl,ki∈Rn, where lj and ki are unit vectors with their j -th and i -th elements equal to 1, respectively. It means that only the j -th covariate is being perturbed. In this case, ω0=0:n×1. By replacing Zi in the Q-function with Zi+ljω⊤ki, we obtain: Q(θ,ω∣ˆθ).
∂2Q(θ,ω∣ˆθ)∂η∂ω⊤|ω=ω0=1σ2[n∑i=1[(−B1(Z⊤iα)+p∑l=1ψlB1(Z⊤i−lα))⋅(ciα⊤ljk⊤i−p∑l=1ψl(ci−lα⊤ljk⊤i−l))+(yi−ξi−δ(^zi−b))⋅(˙B1(Z⊤iα)α⊤ljk⊤i−p∑l=1ψl˙B1(Z⊤i−lα)α⊤ljk⊤i−l)]], |
∂2Q(θ,ω∣ˆθ)∂γ∂ω⊤|ω=ω0=1σ2[n∑i=1(−Wi+p∑l=1ψlWi−l)(ciα⊤ljk⊤i−p∑l=1ψl(ci−lα⊤ljk⊤i−l))],∂2Q(θ,ω∣ˆθ)∂δ∂ω⊤|ω=ω0=1σ2[n∑i=1−(^zi−b)(ciα⊤ljk⊤i−p∑l=1ψl(ci−lα⊤ljk⊤i−l))],∂2Q(θ,ω∣ˆθ)∂σ2∂ω⊤|ω=ω0=−1σ4[n∑i=1(yi−ξi−δ(^zi−b))(ciα⊤ljk⊤i−p∑l=1ψl(ci−lα⊤ljk⊤i−l))],∂2Q(θ,ω∣ˆθ)∂ψk∂ω⊤|ω=ω0=−1σ2[n∑i=1[ri−k(ciα⊤ljk⊤i−p∑l=1ψl(ci−lα⊤ljk⊤i−l))+(yi−ξi−δ(^zi−b))(ci−kα⊤ljk⊤i−k)]], |
where ci is given by ci=˙B⊤1(Z⊤iα)η, and it is important to note that all terms must have indices greater than 0; otherwise, the term will be equal to 0.
In this section, we examine the properties of the proposed estimator using two simulation examples. We consider three different sample sizes: n=100, 300, and 700. Each example is repeated 300 times.
Additionally, we utilize MSE, bias, and variance (var) values to assess the performance of parameter estimation. For instance, in the case of δ, the MSE, bias, and var values are calculated using the following equations:
MSE(ˆδ)=1300300∑j=1(ˆδj−δ)2, | (5.1) |
Bias(ˆδ)=1300300∑j=1(ˆδj−δ), | (5.2) |
Var(ˆδ)=1300300∑j=1(ˆδj−¯δ)2, | (5.3) |
where δ is the true value, and ¯δ is the mean of {ˆδj}j=1,…,300. To assess the performance of the estimated link function g(⋅) and slope function β(⋅), we adopt the RASE as a metric.
Following the idea of Yu et al. [7], the simulation experiment uses a cubic B-spline with evenly distributed knots. To simplify the computational complexity and ensure numerical stability, the numbers of spline basis functions, N1 and N2, are selected by minimizing the BIC.
The dataset for the first example is generated according to the following model:
Y=sin(u)+∫10β(t)X(t)dt+ε. |
In the single-index component, we use the design of Lin et al.[29], g(u)=sin(u), where u=Z⊤α, α=(0.2,−0.7)⊤√0.53, and let Z=(Z1,Z2)⊤, where Ziiid∼Unif(0,1), for i=1,2. In the functional linear component, we follow the design of Yu et al. [30], with the slope function set as β(t)=√2sin(πt2)+3√2sin(3πt2), and X(t)=∑50j=1ξjvj(t). Here, ξj follows a normal distribution with mean 0 and variance λj=((j−0.5)π)−2, and vj(t)=√2sin((j−0.5)πt). The random error ε satisfies the model εi=ei+∑pl=1ψlεi−l, where ei∼SN(−bδ,σ2,δ), with σ2=0.2.
To assess the algorithm's robustness, we examine its performance under various error structures by adjusting the autoregressive coefficient, order, and skewness. We focus on three cases: Case 1, where we have a 1-order autoregressive structure (AR(1)) with δ=0.7; Case 2, where we have a 2-order autoregressive structure (AR(2)) with δ=0.7; and Case 3, where we again use a 1-order autoregressive structure (AR(1)) but with δ=1. For the autoregressive structures, we set ψ=0.5 for AR(1), and ψ1=−0.7, ψ2=0.2 for AR(2).
Tables 2 and 3 present the MSE, Var, and bias for the parameters α, ψ, σ2, and δ, along with the sample mean, median, and variance of RASEj (for j=1,2) at different sample sizes. It is evident that as the sample size n increases from 100 to 300 and further to 700, both the MSE and the sample statistics (mean, median, and variance) of RASEj show a decreasing trend. Based on the above results, it can be seen that the B-splines provide asymptotically unbiased estimates for the nonparametric components. Although the bias of α1 and ψ fluctuates slightly, it shows a decreasing trend as the sample size increases, while the bias of the other parameters consistently decreases as the sample size grows. Figures 2 and 3 present the true curves and the fitted curves based on the estimates. It is evident that as the sample size increases, the estimated curves gradually approach the true curves, indicating that the algorithm's fitting performance for the nonparametric components improves with larger sample sizes. Overall, the simulation results demonstrate that the proposed estimation method is effective.
n | Criterion | Mean | Median | Var |
100 | RASE1 | 1.266 | 1.037 | 1.106 |
RASE2 | 0.297 | 0.188 | 0.092 | |
300 | RASE1 | 0.695 | 0.646 | 0.104 |
RASE2 | 0.131 | 0.110 | 0.017 | |
700 | RASE1 | 0.486 | 0.433 | 0.044 |
RASE2 | 0.073 | 0.066 | 0.001 |
n=100 | n=300 | n=700 | ||||||||||
Parameter | True Value | MSE | Var | Bias | MSE | Var | Bias | MSE | Var | Bias | ||
α1 | 0.2√0.53 | 0.025 | 0.025 | 0.009 | 0.013 | 0.013 | 0.017 | 0.006 | 0.006 | 0.005 | ||
α2 | −0.7√0.53 | 0.471 | 0.403 | 0.261 | 0.065 | 0.063 | 0.045 | 0.001 | 0.001 | 0.005 | ||
ψ | 0.5 | 0.009 | 0.009 | 0.001 | 0.002 | 0.002 | -0.002 | 0.001 | 0.001 | 0 | ||
σ2 | 0.2 | 0.011 | 0.011 | -0.024 | 0.004 | 0.004 | 0.005 | 0.001 | 0.011 | -0.002 | ||
δ | 0.7 | 0.112 | 0.104 | -0.091 | 0.031 | 0.028 | -0.053 | 0.008 | 0.008 | -0.013 |
To validate the stability of the algorithm, Case 2 extends the error structure from 1-order autoregressive (AR(1)) to 2-order autoregressive (AR(2)), increasing the complexity of the error model. As shown in Tables 4 and 5, the bias and MSE of the parameters α, ψ, and δ, as well as the sample mean, median, and variance of RASEj (for j=1,2), decrease as the sample size increases. Although the bias of σ2 fluctuates slightly, its MSE still decreases with increasing sample size. This indicates that as the sample size grows, the accuracy and stability of the parameter estimates improve. From Figures 4 and 5, it can be observed that the estimated curves gradually approach the true curves.
n | Criterion | Mean | Median | Var |
100 | RASE1 | 1.149 | 0.923 | 0.591 |
RASE2 | 0.336 | 0.170 | 0.120 | |
300 | RASE1 | 0.620 | 0.553 | 0.097 |
RASE2 | 0.149 | 0.086 | 0.053 | |
700 | RASE1 | 0.436 | 0.397 | 0.025 |
RASE2 | 0.083 | 0.055 | 0.022 |
n=100 | n=300 | n=700 | ||||||||||
Parameter | True Value | MSE | Var | Bias | MSE | Var | Bias | MSE | Var | Bias | ||
α1 | 0.2√0.53 | 0.031 | 0.031 | 0.027 | 0.010 | 0.010 | 0.007 | 0.005 | 0.005 | -0.006 | ||
α2 | −0.7√0.53 | 0.800 | 0.606 | 0.440 | 0.268 | 0.246 | 0.147 | 0.102 | 0.099 | 0.054 | ||
ψ1 | -0.7 | 0.018 | 0.016 | -0.050 | 0.004 | 0.003 | -0.012 | 0.001 | 0.001 | -0.003 | ||
ψ2 | 0.2 | 0.020 | 0.015 | -0.066 | 0.004 | 0.003 | -0.017 | 0.001 | 0.001 | -0.007 | ||
σ2 | 0.2 | 0.014 | 0.014 | -0.007 | 0.005 | 0.005 | 0.011 | 0.003 | 0.003 | 0.009 | ||
δ | 0.7 | 0.156 | 0.128 | -0.167 | 0.057 | 0.050 | -0.084 | 0.040 | 0.037 | -0.055 |
To evaluate the performance of the proposed algorithm under data with varying skewness, Case 3 systematically increased the skewness parameter δ from 0.7 to 1. As shown in Tables 6 and 7, the bias and mean squared error (MSE) of the parameters α, ψ, and δ, as well as the sample mean, median, and variance of RASEj (for j=1,2), decrease with increasing sample size across different sample sizes. Although the bias of σ2 fluctuates slightly, it remains at a low level (ranging from -0.001 to -0.004). Additionally, as seen in Figures 6 and 7, the estimated functions progressively approach the true functions as the sample size increases.
n | Criterion | Mean | Median | Var |
100 | RASE1 | 1.149 | 0.923 | 0.591 |
RASE2 | 0.336 | 0.170 | 0.120 | |
300 | RASE1 | 0.620 | 0.553 | 0.097 |
RASE2 | 0.149 | 0.086 | 0.053 | |
700 | RASE1 | 0.436 | 0.397 | 0.025 |
RASE2 | 0.083 | 0.055 | 0.022 |
n=100 | n=300 | n=700 | ||||||||||
Parameter | True Value | MSE | Var | Bias | MSE | Var | Bias | MSE | Var | Bias | ||
α1 | 0.2√0.53 | 0.038 | 0.037 | 0.030 | 0.018 | 0.017 | 0.021 | 0.009 | 0.009 | 0.007 | ||
α2 | −0.7√0.53 | 0.674 | 0.530 | 0.378 | 0.180 | 0.168 | 0.109 | 0.001 | 0.001 | 0.007 | ||
ψ | 0.5 | 0.009 | 0.009 | 0.009 | 0.002 | 0.002 | -0.001 | 0.001 | 0.001 | 0 | ||
σ2 | 0.2 | 0.018 | 0.017 | -0.034 | 0.004 | 0.004 | -0.001 | 0.001 | 0.001 | -0.004 | ||
δ | 1 | 0.108 | 0.104 | -0.060 | 0.014 | 0.013 | -0.028 | 0.005 | 0.004 | -0.007 |
From the algorithm's performance under AR(1) and AR(2) error structures, as well as varying levels of skewness, it can be observed that the proposed estimation method demonstrates good consistency and effectiveness in complex error structures. Furthermore, the estimation accuracy improves significantly as the sample size increases.
To systematically evaluate the time complexity and efficiency of the algorithm, this paper measures the average runtime of the EM-CALS algorithm under Cases 1–3 scenarios with sample sizes of n=100, 300, and 700. As shown in Table 8, the experiment execution time does not directly increase or decrease with the increase in sample size. This is related to the more flexible convergence criteria applied in the EM-CALS algorithm.
n | User | System | Elapsed | |
100 | Case 1 | 146.328 | 2.723 | 151.925 |
Case 2 | 189.914 | 2.816 | 196.018 | |
Caee 3 | 143.048 | 2.206 | 148.332 | |
300 | Case 1 | 123.117 | 2.297 | 127.611 |
Case 2 | 256.243 | 3.896 | 263.757 | |
Case 3 | 50.093 | 0.829 | 51.795 | |
700 | Case 1 | 135.031 | 2.781 | 140.028 |
Case 2 | 203.583 | 3.370 | 207.119 | |
Case 3 | 82.259 | 1.514 | 84.915 |
The dataset is generated based on the following model:
Y=−2(u−1)2+1+∫10β(t)X(t)dt+ε, |
where u=Z⊤α, Z=(Z1,Z2)⊤, and Ziiid∼Unif(0,1), i=1,2. The single-index vector is α=(α1,α2)⊤=(√33,√63)⊤. For the slope function, the generation of X(t) and ε follows the same design as in Example 1.
To evaluate the robustness of the algorithm, we adopt the same design as in Example 1. By varying the skewness parameter, autoregressive coefficients, and orders, the algorithm's performance is assessed under different error structures. Specifically, we consider three cases: Case 4, which involves a 1-order autoregressive structure (AR(1)) with δ=0.7; Case 5, which uses a 2-order autoregressive structure (AR(2)) with δ=0.7; and Case 6, which again employs a 1-order autoregressive structure (AR(1)), but with δ=1. For the autoregressive structures, we set ψ=0.5 for AR(1), and ψ1=−0.7, ψ2=0.2 for AR(2).
From Tables 9 and 10, it is evident that as the sample size n increases, the sample mean, median, and variance of RASE 1 and RASE 2 gradually decrease. This trend indicates that the algorithm's performance in fitting the nonparametric components improves with larger sample sizes. Additionally, the MSE values for all parameters also decline as n increases. The Var consistently decreases with larger n, indicating improved stability of the estimators. Although the bias of some parameters, such as α1, shows slight fluctuations across different sample sizes, these variations remain small and are generally kept at low levels. These fluctuations may be linked to the complexity of the error terms.
n | Criterion | Mean | Median | Var |
100 | RASE1 | 1.269 | 1.073 | 0.644 |
RASE2 | 0.199 | 0.160 | 0.113 | |
300 | RASE1 | 0.706 | 0.625 | 0.182 |
RASE2 | 0.104 | 0.099 | 0.002 | |
700 | RASE1 | 0.478 | 0.429 | 0.041 |
RASE2 | 0.070 | 0.067 | 0.001 |
n=100 | n=300 | n=700 | ||||||||||
Parameter | True Value | MSE | Var | Bias | MSE | Var | Bias | MSE | Var | Bias | ||
α1 | √33 | 0.017 | 0.017 | -0.010 | 0.005 | 0.005 | 0.001 | 0.002 | 0.002 | 0.004 | ||
α2 | √63 | 0.030 | 0.030 | -0.022 | 0.002 | 0.002 | -0.005 | 0.001 | 0.001 | -0.004 | ||
ψ | 0.5 | 0.010 | 0.010 | 0.002 | 0.002 | 0.002 | -0.001 | 0.001 | 0.001 | 0 | ||
σ2 | 0.2 | 0.011 | 0.011 | -0.029 | 0.004 | 0.004 | 0.006 | 0.001 | 0.001 | -0.002 | ||
δ | 0.7 | 0.112 | 0.106 | -0.079 | 0.033 | 0.030 | -0.057 | 0.008 | 0.008 | -0.014 |
Figures 8 and 9, it is evident that as the sample size n increases, the performance of the fitted functions β(t) and g(t) using B-splines improves significantly. When n=100, a noticeable deviation exists between the fitted curves and the true curves. However, as n increases to 300 and 700, the fitted curves progressively converge to the true curves. This indicates that larger sample sizes allow the algorithm to more effectively capture the characteristics of the true curves, leading to improved estimation accuracy and convergence.
The analysis of Tables 11 and 12 reveals that as the sample size n increases, the algorithm's performance in fitting the nonparametric components improves consistently. This improvement is reflected in reducing the sample mean, median, and variance of RASE1 and RASE2. Moreover, as the sample size grows, the MSE and the variance of the parameters also decrease. For the bias of the parameters α1 and σ2, although there are some fluctuations, the values remain within a relatively small range overall. Increasing the sample size results in more precise and stable estimation outcomes.
n | Criterion | Mean | Median | Var |
100 | RASE1 | 1.097 | 0.908 | 0.559 |
RASE2 | 0.333 | 0.139 | 0.766 | |
300 | RASE1 | 0.618 | 0.540 | 0.095 |
RASE2 | 0.085 | 0.064 | 0.062 | |
700 | RASE1 | 0.438 | 0.403 | 0.024 |
RASE2 | 0.049 | 0.047 | 0 |
n=100 | n=300 | n=700 | ||||||||||
Parameter | True Value | MSE | Var | Bias | MSE | Var | Bias | MSE | Var | Bias | ||
α1 | \frac{\sqrt{3}}{3} | 0.022 | 0.021 | -0.026 | 0.004 | 0.004 | -0.003 | 0.001 | 0.001 | -0.004 | ||
\alpha_2 | \frac{\sqrt{6}}{3} | 0.157 | 0.149 | -0.091 | 0.012 | 0.012 | -0.008 | 0.001 | 0.001 | 0.001 | ||
\psi_1 | -0.7 | 0.019 | 0.016 | -0.054 | 0.004 | 0.004 | -0.012 | 0.001 | 0.001 | -0.004 | ||
\psi_2 | 0.2 | 0.020 | 0.016 | -0.068 | 0.004 | 0.003 | -0.017 | 0.001 | 0.001 | -0.007 | ||
\sigma^2 | 0.2 | 0.013 | 0.013 | -0.011 | 0.006 | 0.005 | 0.015 | 0.003 | 0.003 | 0.007 | ||
\delta | 0.7 | 0.153 | 0.127 | -0.159 | 0.068 | 0.058 | -0.101 | 0.036 | 0.033 | -0.050 |
In Figures 10 and 11, it is evident that as the sample size n increases, the algorithm's performance to fit \beta(t) and g(t) improves significantly. When the sample size n increases to 300 and 700, the fitted curves approach the accurate curves more closely, indicating that the accuracy of the estimation improves with larger sample sizes.
Tables 13 and 14 present the MSE, Var, and bias for the parameters \boldsymbol{\alpha} , \boldsymbol{\psi} , \sigma^2 , and \delta , along with the sample mean, median, and variance of \mathrm{RASE}_j (for j = 1, 2 ) at different sample sizes. These results demonstrate conclusions consistent with Case 5. Simulation experiments using three distinct parameter configurations in Example 2 indicate that the proposed algorithm demonstrates strong adaptability in the single-index partially linear model, even with complex autoregressive error structures. As illustrated in Figures 12 and 13, the estimated curves gradually approach the true curve as the sample size increases.
n | Criterion | Mean | Median | Var |
100 | RASE _1 | 1.538 | 1.238 | 1.284 |
RASE _2 | 0.319 | 0.211 | 0.408 | |
300 | RASE _1 | 0.771 | 0.701 | 0.232 |
RASE _2 | 0.125 | 0.117 | 0.003 | |
700 | RASE _1 | 0.527 | 0.467 | 0.069 |
RASE _2 | 0.083 | 0.080 | 0.001 |
n = 100 | n = 300 | n = 700 | ||||||||||
Parameter | True Value | MSE | Var | Bias | MSE | Var | Bias | MSE | Var | Bias | ||
\alpha_1 | \frac{\sqrt{3}}{3} | 0.030 | 0.029 | -0.016 | 0.007 | 0.007 | -0.001 | 0.003 | 0.003 | 0.005 | ||
\alpha_2 | \frac{\sqrt{6}}{3} | 0.104 | 0.099 | -0.071 | 0.003 | 0.003 | -0.006 | 0.001 | 0.001 | -0.006 | ||
\psi | 0.5 | 0.010 | 0.010 | 0.003 | 0.002 | 0.002 | 0 | 0.001 | 0.001 | 0 | ||
\sigma^2 | 0.2 | 0.019 | 0.018 | -0.035 | 0.004 | 0.004 | -0.001 | 0.001 | 0.001 | -0.005 | ||
\delta | 1 | 0.107 | 0.104 | -0.053 | 0.015 | 0.014 | -0.028 | 0.004 | 0.004 | -0.008 |
To systematically evaluate the time complexity and efficiency of the algorithm, the average runtime of the EM-CALS algorithm is measured under Cases 4–6 scenarios in Example 2, with sample sizes of n = 100 , 300, and 700, following the same approach as in Example 1. As shown in Table 15, the experiment execution time does not directly increase or decrease with the increase in sample size. This is related to the more flexible convergence criteria applied in the EM-CALS algorithm.
n | User | System | Elapsed | |
100 | Case 4 | 126.892 | 2.917 | 194.251 |
Case 5 | 193.034 | 2.961 | 199.880 | |
Caee 6 | 111.520 | 2.566 | 173.009 | |
300 | Case 4 | 75.457 | 1.741 | 109.605 |
Case 5 | 168.304 | 2.418 | 172.591 | |
Case 6 | 32.806 | 0.786 | 48.882 | |
700 | Case 4 | 65.384 | 1.678 | 68.649 |
Case 5 | 177.791 | 2.418 | 172.591 | |
Case 6 | 48.716 | 1.256 | 56.743 |
With the rapid advancement of photovoltaic (PV) technology, it has gained significant popularity in grid-connected applications. The power output of PV systems is affected by various factors, including solar irradiance, ambient temperature, sunlight intensity, and installation angle. Given the fluctuations in solar irradiance and environmental conditions, the power output of PV systems inherently exhibits temporal variability. Wang, Su, and Shu [32] analyzed grid-connected power generation data from Macau in 2011 using partial functional linear regression under the assumption of independent errors. Xiao and Wang [12] extended this analysis by employing a partial functional linear model with autoregressive errors on the same dataset.
In this study, we analyzed the Macau 2018 PV power generation dataset provided by Qiu [34]. This dataset comprises solar power generation data collected from a 3-kilowatt rooftop PV plant at the University of Macau throughout 2018, with measurements taken at 30-second intervals. Additionally, it includes public weather report data from Macau, with weather variables recorded hourly. The system is located on Coloane Island in the Macau Special Administrative Region (SAR) (latitude 22° 100000'N, longitude 113° 330000'E). The data was recorded from January 1, 2018 to December 31, 2018. Due to various factors, such as meteorological conditions, maintenance, or instrument malfunctions, some data entries were missing. After employing standard preprocessing techniques to eliminate missing values and outliers, we retained 356 days of data. The total output power for the following day was selected as the response variable Y (kW), while the hourly output power from the previous day served as the functional predictor. Several meteorological variables were also considered as multivariate predictors. Specifically, Z_1 represents daily average cloud cover, Z_2 refers to daily precipitation, Z_3 denotes the daily average temperature, and Z_4 refers to total solar radiation. Figure 14 illustrates the behavior of the functional predictor variables X_i(t) , where all functional predictors exhibit similar patterns. Before modeling, Figures 15–18 depict the relationships between daily average cloud cover, daily precipitation, daily average temperature, and daily solar radiation with daily output power, respectively. The fitted curves were derived using the lasso method.
To evaluate model performance, we split the dataset into two subsamples: A training subsample \{Y_t, Z_t, X_t(u)\}_{t = 1}^{300} for parameter estimation and a test subsample \{Y_t, Z_t, X_t(u)\}_{t = 301}^{356} for validating prediction quality. We quantify forecasting accuracy through two metrics: the MSE and mean relative squared error (MRSE), defined, respectively, as
\begin{align} \text{MSE} & = \frac{1}{56}\sum\limits_{t = 301}^{356}\left(Y_{t} - \hat{Y}_{t}\right)^{2}, \end{align} | (6.1) |
\begin{align} \text{MRSE} & = \frac{1}{56}\sum\limits_{t = 301}^{356}\frac{\left(Y_{t} - \hat{Y}_{t}\right)^{2}}{\operatorname{Var}(Y)}, \end{align} | (6.2) |
where \operatorname{Var}(Y) denotes the variance of the response variable over the test set, Y_i represents the true value of the i -th observation, and \hat{Y}_i is the predicted value for the i -th observation from the model.
As shown in Figures 15–18, the daily average cloud cover ( Z_1 ) negatively impacts output power, as seen in Figure 15. Clouds obstruct sunlight, reducing effective radiation reaching PV panels and significantly lowering generation efficiency. Daily precipitation ( Z_2 ) demonstrates a negative nonlinear relationship with output power. As precipitation increases, the power output decreases. Heavier rainfall is typically accompanied by cloudy conditions, which reduce solar radiation. This attenuation of solar radiation, caused by both cloud cover and rain, leads to a decrease in power generation. The daily average temperature ( Z_3 ) has a positive but insignificant effect on output power. While higher temperatures are generally associated with ample sunlight that aids power generation, the efficiency of PV panels actually decreases at elevated temperatures. This results in only a weak positive impact of temperature on output power. In contrast, total solar radiation ( Z_4 ) shows a strong positive correlation with output power. Higher radiation levels enhance photon absorption, leading to a direct increase in power generation, in accordance with the fundamental principles of PV energy conversion.
Figures 15–18 illustrate that the relationships between daily average cloud cover, daily precipitation, daily average temperature, and daily solar radiation with output power are nonlinear. Consequently, traditional linear models are insufficient for accurately capturing these complex interactions. The single-index model provides the necessary flexibility to account for nonlinear relationships among multiple covariates. By integrating the effects of several covariates into a single index, this model is particularly effective for modeling the nonlinear dynamics between output power and the relevant variables. Therefore, we utilize the single-index partially functional linear regression model for our analysis.
The residual analysis was performed using the single-index partially functional linear regression model introduced by Yu et al. [7]. The residuals were then evaluated with the Shapiro-Wilk test to check for normality. The results revealed a W statistic of 0.77008 and a p-value less than 2.2 \times 10^{-16} , which led to the rejection of the null hypothesis. This indicates that the residuals do not follow a normal distribution. Furthermore, the Q-Q plot shown in Figure 19 suggests that while the residuals approximate a normal distribution in the central region, they exhibit significant deviations in the tails, indicating heavy-tailed characteristics and a departure from normality. Given the observed skewness and heavy tails in the residuals, the SN distribution, as proposed by Azzalini [11], was adopted to more accurately model errors. The SN distribution introduces a skewness parameter, enabling it to effectively capture asymmetry and accommodate data with moderate skewness and kurtosis. As a result, the assumption of normal errors was replaced with the skew-normal error distribution.
Based on the model and algorithm proposed by Yu et al. [7], the residual sequence is obtained. Subsequently, the Ljung-Box (LB) test statistic, along with the autocorrelation function (ACF) and partial autocorrelation function (PACF) plots, are utilized to test for the presence of 1-order to 5-order serial correlation.
Figures 20 and 21 illustrate the PACF and the ACF of the residuals, respectively. Both functions reveal significant deviations from zero at a lag of 2, indicating that the residuals display an AR(2) structure. As shown in Table 16, when h = 2 , the p -value of the LB test statistic is the smallest and is less than the given significance level of 0.05, leading to the rejection of the null hypothesis and indicating that the errors follow an AR(2) structure. Considering the AR(2) structure and skewness of the errors, we propose a single-index partially functional linear model with AR(2) skew-normal errors, referred to as SIPFLM-SNAR(2). For comparative analysis, we also created two additional models: one that includes normal AR(2) errors, called SIPFLM-NAR(2), and another that assumes independent errors.
h-order | |||||
h=1 | h=2 | h=3 | h=4 | h=5 | |
p values | 0.768 | 0.026 | 0.061 | 0.091 | 0.050 |
Table 17 summarizes the estimated values of the relevant parameters and MSE and MRSE. The results indicate that the proposed SIPFLM-SNAR(2) model achieves a lower MSE and MRSE compared to the SIPFLM-NAR(2) and SIPFLM models, demonstrating its superior predictive accuracy.
Parameter | Indep. Normal | AR(2) -Normal | AR(2) -Skew-Normal |
\alpha_1 | 0.001 | 0.649 | 0.646 |
\alpha_2 | 0.074 | 0.049 | 0.051 |
\alpha_3 | -0.996 | -0.757 | -0.760 |
\alpha_4 | 0.048 | 0.053 | 0.054 |
\gamma_1 | -1.095 | -0.687 | -0.688 |
\gamma_2 | 2.212 | 1.563 | 1.567 |
\gamma_3 | -1.917 | -1.490 | -1.497 |
\gamma_4 | 0.625 | 0.573 | 0.582 |
\eta_1 | 0.548 | 0.642 | 0.646 |
\eta_2 | 5.524 | 5.751 | 5.741 |
\eta_3 | 14.573 | 14.609 | 14.608 |
\eta_4 | 15.032 | 15.018 | 15.007 |
\psi_1 | – | 0.115 | 0.114 |
\psi_2 | – | 0.194 | 0.194 |
\sigma^2 | 2.061 | 2.916 | 2.958 |
\delta | – | – | 0.086 |
MSE | 5.168 | 3.190 | 3.161 |
MRSE | 0.314 | 0.194 | 0.192 |
Total Sample Size | 356 | 356 | 356 |
Training Set Size | 300 | 300 | 300 |
Test Set Size | 56 | 56 | 56 |
This paper addresses parameter estimation for the single-index partially functional linear models with p -order autoregressive skew-normal errors. We propose an EM-CALS algorithm to estimate the model's parametric and nonparametric components. The method includes analytical expressions for the E-step and M-step. To handle the nonlinear constraint imposed on the single-index coefficient, specifically the unit norm constraint, we incorporate a CALS step into the algorithm. This modification reduces the parameter estimation complexity and improves the algorithm's stability. To demonstrate the performance advantages of the EM-CALS algorithm, we compare it with the TSILS algorithm. Compared to the TSILS algorithm, the EM-CALS algorithm shows significant improvements in the mean values of RMSE, MSE, RASE _{1} , and RASE _{2} , with decreases of 1.19%, 2.45%, 38%, and 51.35%, respectively, demonstrating its clear advantage in enhancing prediction accuracy. Additionally, we perform a residual analysis based on conditional residuals, following the methodology described by Dunn and Smyth [31].
The proposed model performs local influence analysis using the Q-function in the EM algorithm. The impact of case-weight perturbation, response variable perturbation, and explanatory variable perturbation on the model is examined, and the analytical expression of the Hessian matrix is derived.
The performance of the EM-CALS algorithm is validated through simulation studies that assess its behavior under various scenarios, including changes in the autoregressive order of the errors and the nonparametric function in the single-index component. The results indicate that while some parameters show fluctuations in bias, their MSE decreases with larger sample sizes. This suggests an improvement in model fitting performance. The proposed estimation method demonstrates good stability and accuracy, particularly when larger sample sizes are used.
Furthermore, the model is applied to a dataset from a PV system for power prediction. The findings reveal that the SIPFLM-SNAR(2) model effectively captures asymmetry and temporal dependence in the response variable, making it highly useful in scenarios involving functional data and multidimensional scalar predictors.
Despite the positive results of this study, several limitations remain. First, the asymptotic properties and convergence rate of the EM-CALS estimators have yet to be proven. Future research could explore the asymptotic normality and convergence speed of this algorithm. Second, the simulations in this study mainly focus on low-dimensional cases, and the performance of the algorithm in high-dimensional single-index models has not been fully examined. As the model's dimensionality increases, ensuring the accuracy of estimations and improving the computational efficiency of the algorithm will present significant challenges. Future research can expand the scope of simulations to further validate the applicability of the algorithm in high-dimensional settings while considering additional potential influencing factors, thereby enhancing the robustness and broader applicability of the algorithm.
Lijie Zhou: Investigation, formal analysis, validation, software, data curation, writing-original draft, writing-review and editing; Liucang Wu: methodology, validation; Bin Yang: Conceptualization, funding acquisition, investigation, resources, validation, data curation, writing-original draft, writing-review and editing. All authors have read and approved the final version of the manuscript for publication.
The authors declare they have not used Artificial Intelligence (AI) tools in creating this article.
The research was supported by the National Nature Science Foundation of China (No.12261051), the Key project of Yunnan Province Basic Research Program (Grant No.202401AS070061), the Yunnan Provincial Department of Education Science Research Fund (Grant No.2024J0087), and the General Project of Yunnan Province Basic Research Program(Grant No.202401AT070321).
The authors declare that there is no conflict of interest.
[1] | J. Brzdęk, D. Popa, I. Raşa, B. Xu, Ulam stability of operators, Academic Press, 2018. |
[2] | A. K. Tripathy, Hyers–Ulam stability of ordinary differential equations, Chapman and Hall/CRC, 2021. https://doi.org/10.1201/9781003120179 |
[3] |
A. R. Baias, F. Blaga, D. Popa, On the best Ulam constant of a first order linear difference equation in Banach spaces, Acta Math. Hungar., 163 (2021), 563–575. https://doi.org/10.1007/s10474-020-01098-3 doi: 10.1007/s10474-020-01098-3
![]() |
[4] |
S. N. Bora, M. Shankar, Ulam–Hyers stability of second-order convergent finite difference scheme for first- and second-order nonhomogeneous linear differential equations with constant coefficients, Results Math., 78 (2023), 17. https://doi.org/10.1007/s00025-022-01791-5 doi: 10.1007/s00025-022-01791-5
![]() |
[5] |
K. Chen, Y. Si, Ulam type stability for the second-order linear Hahn difference equations, Appl. Math. Lett., 160 (2025), 109355. https://doi.org/10.1016/j.aml.2024.109355 doi: 10.1016/j.aml.2024.109355
![]() |
[6] |
D. M. Kerekes, B. Moşneguţu, D. Popa, On Ulam stability of a second order linear difference equation, AIMS Math., 8 (2023), 20254–20268. https://doi.org/10.3934/math.20231032 doi: 10.3934/math.20231032
![]() |
[7] |
A. Novac, D. Otrocol, D. Popa, Ulam stability of a linear difference equation in locally convex spaces, Results Math., 76 (2021), 33. https://doi.org/10.1007/s00025-021-01344-2 doi: 10.1007/s00025-021-01344-2
![]() |
[8] |
Y. Shen, Y. Li, The z-transform method for the Ulam stability of linear difference equations with constant coefficients, Adv. Differ. Equations, 2018 (2018), 396. https://doi.org/10.1186/s13662-018-1843-0 doi: 10.1186/s13662-018-1843-0
![]() |
[9] |
C. Buşe, V. Lupulescu, D. O'Regan, Hyers–Ulam stability for equations with differences and differential equations with time-dependent and periodic coefficients, Proc. R. Soc. Edinburgh Sect. A, 150 (2020), 2175–2188. https://doi.org/10.1017/prm.2019.12 doi: 10.1017/prm.2019.12
![]() |
[10] |
D. Popa, I. Raşa, A. Viorel, Approximate solutions of the logistic equation and Ulam stability, Appl. Math. Lett., 85 (2018), 64–69. https://doi.org/10.1016/j.aml.2018.05.018 doi: 10.1016/j.aml.2018.05.018
![]() |
[11] |
M. Onitsuka, Conditional Ulam stability and its application to the logistic model, Appl. Math. Lett., 122 (2021), 107565. https://doi.org/10.1016/j.aml.2021.107565 doi: 10.1016/j.aml.2021.107565
![]() |
[12] |
M. Onitsuka, Approximate solutions of generalized logistic equation, Discrete Contin. Dyn. Syst., 29 (2024), 4505–4526. https://doi.org/10.3934/dcdsb.2024053 doi: 10.3934/dcdsb.2024053
![]() |
[13] |
L. Backes, D. Dragičeviū, M. Onitsuka, M. Pituk, Conditional Lipschitz shadowing for ordinary differential equations, J. Dyn. Differ. Equations, 36 (2024), 3535–3552. https://doi.org/10.1007/s10884-023-10246-6 doi: 10.1007/s10884-023-10246-6
![]() |
[14] |
S. M. Jung, Y. W. Nam, Hyers–Ulam stability of Pielou logistic difference equation, J. Nonlinear Sci. Appl., 10 (2017), 3115–3122. https://doi.org/10.22436/jnsa.010.06.26 doi: 10.22436/jnsa.010.06.26
![]() |
[15] |
Y. W. Nam, Hyers–Ulam stability of elliptic Möbius difference equation, Cogent Math. Stat., 5 (2018), 1492338. https://doi.org/10.1080/25742558.2018.1492338 doi: 10.1080/25742558.2018.1492338
![]() |
[16] |
Y. W. Nam, Hyers–Ulam stability of hyperbolic Möbius difference equation, Filomat, 32 (2018), 4555–4575. https://doi.org/10.2298/fil1813555n doi: 10.2298/fil1813555n
![]() |
[17] |
Y. W. Nam, Hyers–Ulam stability of loxodromic Möbius difference equation, Appl. Math. Comput., 356 (2019), 119–136. https://doi.org/10.1016/j.amc.2019.03.033 doi: 10.1016/j.amc.2019.03.033
![]() |
[18] |
A. S. Ackleh, Y. M. Dib, S. R. J. Jang, A three-stage discrete-time population model: continuous versus seasonal reproduction, J. Biol. Dyn., 1 (2007), 291–304. https://doi.org/10.1080/17513750701605440 doi: 10.1080/17513750701605440
![]() |
[19] | L. L. Albu, Non-linear models: applications in economics, SSRN Electron. J., 2006. https://doi.org/10.2139/ssrn.1565345 |
[20] | M. Bohner, A. Peterson, Advances in dynamic equations on time scales, Birkhäuser, 2003. https://doi.org/10.1007/978-0-8176-8230-9 |
[21] |
J. Cushing, S. Henson, A periodically forced Beverton–Holt equation, J. Differ. Equations Appl., 8 (2002), 1119–1120. https://doi.org/10.1080/1023619031000081159 doi: 10.1080/1023619031000081159
![]() |
[22] |
S. Elaydi, R. J. Sacker, Global stability of periodic orbits of non-autonomous difference equations and population biology, J. Differ. Equations, 208 (2005), 258–273. https://doi.org/10.1016/j.jde.2003.10.024 doi: 10.1016/j.jde.2003.10.024
![]() |
[23] | M. Bohner, A. Peterson, Dynamic equations on time scales: an introduction with applications, Birkhäuser, 2001. https://doi.org/10.1007/978-1-4612-0201-1 |
RMSE | MSE | \text{RASE}_{1} | \text{RASE}_{2} | ||||||||
Algorithms | Mean | Var | Mean | Var | Mean | Var | Mean | Var | |||
EM-CALS | 0.747 | 0.000 | 0.558 | 0.001 | 0.498 | 0.073 | 0.072 | 0.001 | |||
TSILS | 0.756 | 0.000 | 0.572 | 0.001 | 0.801 | 0.151 | 0.148 | 0.006 |
n | Criterion | Mean | Median | Var |
100 | RASE _1 | 1.266 | 1.037 | 1.106 |
RASE _2 | 0.297 | 0.188 | 0.092 | |
300 | RASE _1 | 0.695 | 0.646 | 0.104 |
RASE _2 | 0.131 | 0.110 | 0.017 | |
700 | RASE _1 | 0.486 | 0.433 | 0.044 |
RASE _2 | 0.073 | 0.066 | 0.001 |
n = 100 | n = 300 | n = 700 | ||||||||||
Parameter | True Value | MSE | Var | Bias | MSE | Var | Bias | MSE | Var | Bias | ||
\alpha_1 | \frac{0.2}{\sqrt{0.53}} | 0.025 | 0.025 | 0.009 | 0.013 | 0.013 | 0.017 | 0.006 | 0.006 | 0.005 | ||
\alpha_2 | \frac{-0.7}{\sqrt{0.53}} | 0.471 | 0.403 | 0.261 | 0.065 | 0.063 | 0.045 | 0.001 | 0.001 | 0.005 | ||
\psi | 0.5 | 0.009 | 0.009 | 0.001 | 0.002 | 0.002 | -0.002 | 0.001 | 0.001 | 0 | ||
\sigma^2 | 0.2 | 0.011 | 0.011 | -0.024 | 0.004 | 0.004 | 0.005 | 0.001 | 0.011 | -0.002 | ||
\delta | 0.7 | 0.112 | 0.104 | -0.091 | 0.031 | 0.028 | -0.053 | 0.008 | 0.008 | -0.013 |
n | Criterion | Mean | Median | Var |
100 | RASE _1 | 1.149 | 0.923 | 0.591 |
RASE _2 | 0.336 | 0.170 | 0.120 | |
300 | RASE _1 | 0.620 | 0.553 | 0.097 |
RASE _2 | 0.149 | 0.086 | 0.053 | |
700 | RASE _1 | 0.436 | 0.397 | 0.025 |
RASE _2 | 0.083 | 0.055 | 0.022 |
n = 100 | n = 300 | n = 700 | ||||||||||
Parameter | True Value | MSE | Var | Bias | MSE | Var | Bias | MSE | Var | Bias | ||
\alpha_1 | \frac{0.2}{\sqrt{0.53}} | 0.031 | 0.031 | 0.027 | 0.010 | 0.010 | 0.007 | 0.005 | 0.005 | -0.006 | ||
\alpha_2 | \frac{-0.7}{\sqrt{0.53}} | 0.800 | 0.606 | 0.440 | 0.268 | 0.246 | 0.147 | 0.102 | 0.099 | 0.054 | ||
\psi_1 | -0.7 | 0.018 | 0.016 | -0.050 | 0.004 | 0.003 | -0.012 | 0.001 | 0.001 | -0.003 | ||
\psi_2 | 0.2 | 0.020 | 0.015 | -0.066 | 0.004 | 0.003 | -0.017 | 0.001 | 0.001 | -0.007 | ||
\sigma^2 | 0.2 | 0.014 | 0.014 | -0.007 | 0.005 | 0.005 | 0.011 | 0.003 | 0.003 | 0.009 | ||
\delta | 0.7 | 0.156 | 0.128 | -0.167 | 0.057 | 0.050 | -0.084 | 0.040 | 0.037 | -0.055 |
n | Criterion | Mean | Median | Var |
100 | RASE _1 | 1.149 | 0.923 | 0.591 |
RASE _2 | 0.336 | 0.170 | 0.120 | |
300 | RASE _1 | 0.620 | 0.553 | 0.097 |
RASE _2 | 0.149 | 0.086 | 0.053 | |
700 | RASE _1 | 0.436 | 0.397 | 0.025 |
RASE _2 | 0.083 | 0.055 | 0.022 |
n = 100 | n = 300 | n = 700 | ||||||||||
Parameter | True Value | MSE | Var | Bias | MSE | Var | Bias | MSE | Var | Bias | ||
\alpha_1 | \frac{0.2}{\sqrt{0.53}} | 0.038 | 0.037 | 0.030 | 0.018 | 0.017 | 0.021 | 0.009 | 0.009 | 0.007 | ||
\alpha_2 | \frac{-0.7}{\sqrt{0.53}} | 0.674 | 0.530 | 0.378 | 0.180 | 0.168 | 0.109 | 0.001 | 0.001 | 0.007 | ||
\psi | 0.5 | 0.009 | 0.009 | 0.009 | 0.002 | 0.002 | -0.001 | 0.001 | 0.001 | 0 | ||
\sigma^2 | 0.2 | 0.018 | 0.017 | -0.034 | 0.004 | 0.004 | -0.001 | 0.001 | 0.001 | -0.004 | ||
\delta | 1 | 0.108 | 0.104 | -0.060 | 0.014 | 0.013 | -0.028 | 0.005 | 0.004 | -0.007 |
n | User | System | Elapsed | |
100 | Case 1 | 146.328 | 2.723 | 151.925 |
Case 2 | 189.914 | 2.816 | 196.018 | |
Caee 3 | 143.048 | 2.206 | 148.332 | |
300 | Case 1 | 123.117 | 2.297 | 127.611 |
Case 2 | 256.243 | 3.896 | 263.757 | |
Case 3 | 50.093 | 0.829 | 51.795 | |
700 | Case 1 | 135.031 | 2.781 | 140.028 |
Case 2 | 203.583 | 3.370 | 207.119 | |
Case 3 | 82.259 | 1.514 | 84.915 |
n | Criterion | Mean | Median | Var |
100 | RASE _1 | 1.269 | 1.073 | 0.644 |
RASE _2 | 0.199 | 0.160 | 0.113 | |
300 | RASE _1 | 0.706 | 0.625 | 0.182 |
RASE _2 | 0.104 | 0.099 | 0.002 | |
700 | RASE _1 | 0.478 | 0.429 | 0.041 |
RASE _2 | 0.070 | 0.067 | 0.001 |
n = 100 | n = 300 | n = 700 | ||||||||||
Parameter | True Value | MSE | Var | Bias | MSE | Var | Bias | MSE | Var | Bias | ||
\alpha_1 | \frac{\sqrt{3}}{3} | 0.017 | 0.017 | -0.010 | 0.005 | 0.005 | 0.001 | 0.002 | 0.002 | 0.004 | ||
\alpha_2 | \frac{\sqrt{6}}{3} | 0.030 | 0.030 | -0.022 | 0.002 | 0.002 | -0.005 | 0.001 | 0.001 | -0.004 | ||
\psi | 0.5 | 0.010 | 0.010 | 0.002 | 0.002 | 0.002 | -0.001 | 0.001 | 0.001 | 0 | ||
\sigma^2 | 0.2 | 0.011 | 0.011 | -0.029 | 0.004 | 0.004 | 0.006 | 0.001 | 0.001 | -0.002 | ||
\delta | 0.7 | 0.112 | 0.106 | -0.079 | 0.033 | 0.030 | -0.057 | 0.008 | 0.008 | -0.014 |
n | Criterion | Mean | Median | Var |
100 | RASE _1 | 1.097 | 0.908 | 0.559 |
RASE _2 | 0.333 | 0.139 | 0.766 | |
300 | RASE _1 | 0.618 | 0.540 | 0.095 |
RASE _2 | 0.085 | 0.064 | 0.062 | |
700 | RASE _1 | 0.438 | 0.403 | 0.024 |
RASE _2 | 0.049 | 0.047 | 0 |
n = 100 | n = 300 | n = 700 | ||||||||||
Parameter | True Value | MSE | Var | Bias | MSE | Var | Bias | MSE | Var | Bias | ||
\alpha_1 | \frac{\sqrt{3}}{3} | 0.022 | 0.021 | -0.026 | 0.004 | 0.004 | -0.003 | 0.001 | 0.001 | -0.004 | ||
\alpha_2 | \frac{\sqrt{6}}{3} | 0.157 | 0.149 | -0.091 | 0.012 | 0.012 | -0.008 | 0.001 | 0.001 | 0.001 | ||
\psi_1 | -0.7 | 0.019 | 0.016 | -0.054 | 0.004 | 0.004 | -0.012 | 0.001 | 0.001 | -0.004 | ||
\psi_2 | 0.2 | 0.020 | 0.016 | -0.068 | 0.004 | 0.003 | -0.017 | 0.001 | 0.001 | -0.007 | ||
\sigma^2 | 0.2 | 0.013 | 0.013 | -0.011 | 0.006 | 0.005 | 0.015 | 0.003 | 0.003 | 0.007 | ||
\delta | 0.7 | 0.153 | 0.127 | -0.159 | 0.068 | 0.058 | -0.101 | 0.036 | 0.033 | -0.050 |
n | Criterion | Mean | Median | Var |
100 | RASE _1 | 1.538 | 1.238 | 1.284 |
RASE _2 | 0.319 | 0.211 | 0.408 | |
300 | RASE _1 | 0.771 | 0.701 | 0.232 |
RASE _2 | 0.125 | 0.117 | 0.003 | |
700 | RASE _1 | 0.527 | 0.467 | 0.069 |
RASE _2 | 0.083 | 0.080 | 0.001 |
n = 100 | n = 300 | n = 700 | ||||||||||
Parameter | True Value | MSE | Var | Bias | MSE | Var | Bias | MSE | Var | Bias | ||
\alpha_1 | \frac{\sqrt{3}}{3} | 0.030 | 0.029 | -0.016 | 0.007 | 0.007 | -0.001 | 0.003 | 0.003 | 0.005 | ||
\alpha_2 | \frac{\sqrt{6}}{3} | 0.104 | 0.099 | -0.071 | 0.003 | 0.003 | -0.006 | 0.001 | 0.001 | -0.006 | ||
\psi | 0.5 | 0.010 | 0.010 | 0.003 | 0.002 | 0.002 | 0 | 0.001 | 0.001 | 0 | ||
\sigma^2 | 0.2 | 0.019 | 0.018 | -0.035 | 0.004 | 0.004 | -0.001 | 0.001 | 0.001 | -0.005 | ||
\delta | 1 | 0.107 | 0.104 | -0.053 | 0.015 | 0.014 | -0.028 | 0.004 | 0.004 | -0.008 |
n | User | System | Elapsed | |
100 | Case 4 | 126.892 | 2.917 | 194.251 |
Case 5 | 193.034 | 2.961 | 199.880 | |
Caee 6 | 111.520 | 2.566 | 173.009 | |
300 | Case 4 | 75.457 | 1.741 | 109.605 |
Case 5 | 168.304 | 2.418 | 172.591 | |
Case 6 | 32.806 | 0.786 | 48.882 | |
700 | Case 4 | 65.384 | 1.678 | 68.649 |
Case 5 | 177.791 | 2.418 | 172.591 | |
Case 6 | 48.716 | 1.256 | 56.743 |
h-order | |||||
h=1 | h=2 | h=3 | h=4 | h=5 | |
p values | 0.768 | 0.026 | 0.061 | 0.091 | 0.050 |
Parameter | Indep. Normal | AR(2) -Normal | AR(2) -Skew-Normal |
\alpha_1 | 0.001 | 0.649 | 0.646 |
\alpha_2 | 0.074 | 0.049 | 0.051 |
\alpha_3 | -0.996 | -0.757 | -0.760 |
\alpha_4 | 0.048 | 0.053 | 0.054 |
\gamma_1 | -1.095 | -0.687 | -0.688 |
\gamma_2 | 2.212 | 1.563 | 1.567 |
\gamma_3 | -1.917 | -1.490 | -1.497 |
\gamma_4 | 0.625 | 0.573 | 0.582 |
\eta_1 | 0.548 | 0.642 | 0.646 |
\eta_2 | 5.524 | 5.751 | 5.741 |
\eta_3 | 14.573 | 14.609 | 14.608 |
\eta_4 | 15.032 | 15.018 | 15.007 |
\psi_1 | – | 0.115 | 0.114 |
\psi_2 | – | 0.194 | 0.194 |
\sigma^2 | 2.061 | 2.916 | 2.958 |
\delta | – | – | 0.086 |
MSE | 5.168 | 3.190 | 3.161 |
MRSE | 0.314 | 0.194 | 0.192 |
Total Sample Size | 356 | 356 | 356 |
Training Set Size | 300 | 300 | 300 |
Test Set Size | 56 | 56 | 56 |
RMSE | MSE | \text{RASE}_{1} | \text{RASE}_{2} | ||||||||
Algorithms | Mean | Var | Mean | Var | Mean | Var | Mean | Var | |||
EM-CALS | 0.747 | 0.000 | 0.558 | 0.001 | 0.498 | 0.073 | 0.072 | 0.001 | |||
TSILS | 0.756 | 0.000 | 0.572 | 0.001 | 0.801 | 0.151 | 0.148 | 0.006 |
n | Criterion | Mean | Median | Var |
100 | RASE _1 | 1.266 | 1.037 | 1.106 |
RASE _2 | 0.297 | 0.188 | 0.092 | |
300 | RASE _1 | 0.695 | 0.646 | 0.104 |
RASE _2 | 0.131 | 0.110 | 0.017 | |
700 | RASE _1 | 0.486 | 0.433 | 0.044 |
RASE _2 | 0.073 | 0.066 | 0.001 |
n = 100 | n = 300 | n = 700 | ||||||||||
Parameter | True Value | MSE | Var | Bias | MSE | Var | Bias | MSE | Var | Bias | ||
\alpha_1 | \frac{0.2}{\sqrt{0.53}} | 0.025 | 0.025 | 0.009 | 0.013 | 0.013 | 0.017 | 0.006 | 0.006 | 0.005 | ||
\alpha_2 | \frac{-0.7}{\sqrt{0.53}} | 0.471 | 0.403 | 0.261 | 0.065 | 0.063 | 0.045 | 0.001 | 0.001 | 0.005 | ||
\psi | 0.5 | 0.009 | 0.009 | 0.001 | 0.002 | 0.002 | -0.002 | 0.001 | 0.001 | 0 | ||
\sigma^2 | 0.2 | 0.011 | 0.011 | -0.024 | 0.004 | 0.004 | 0.005 | 0.001 | 0.011 | -0.002 | ||
\delta | 0.7 | 0.112 | 0.104 | -0.091 | 0.031 | 0.028 | -0.053 | 0.008 | 0.008 | -0.013 |
n | Criterion | Mean | Median | Var |
100 | RASE _1 | 1.149 | 0.923 | 0.591 |
RASE _2 | 0.336 | 0.170 | 0.120 | |
300 | RASE _1 | 0.620 | 0.553 | 0.097 |
RASE _2 | 0.149 | 0.086 | 0.053 | |
700 | RASE _1 | 0.436 | 0.397 | 0.025 |
RASE _2 | 0.083 | 0.055 | 0.022 |
n = 100 | n = 300 | n = 700 | ||||||||||
Parameter | True Value | MSE | Var | Bias | MSE | Var | Bias | MSE | Var | Bias | ||
\alpha_1 | \frac{0.2}{\sqrt{0.53}} | 0.031 | 0.031 | 0.027 | 0.010 | 0.010 | 0.007 | 0.005 | 0.005 | -0.006 | ||
\alpha_2 | \frac{-0.7}{\sqrt{0.53}} | 0.800 | 0.606 | 0.440 | 0.268 | 0.246 | 0.147 | 0.102 | 0.099 | 0.054 | ||
\psi_1 | -0.7 | 0.018 | 0.016 | -0.050 | 0.004 | 0.003 | -0.012 | 0.001 | 0.001 | -0.003 | ||
\psi_2 | 0.2 | 0.020 | 0.015 | -0.066 | 0.004 | 0.003 | -0.017 | 0.001 | 0.001 | -0.007 | ||
\sigma^2 | 0.2 | 0.014 | 0.014 | -0.007 | 0.005 | 0.005 | 0.011 | 0.003 | 0.003 | 0.009 | ||
\delta | 0.7 | 0.156 | 0.128 | -0.167 | 0.057 | 0.050 | -0.084 | 0.040 | 0.037 | -0.055 |
n | Criterion | Mean | Median | Var |
100 | RASE _1 | 1.149 | 0.923 | 0.591 |
RASE _2 | 0.336 | 0.170 | 0.120 | |
300 | RASE _1 | 0.620 | 0.553 | 0.097 |
RASE _2 | 0.149 | 0.086 | 0.053 | |
700 | RASE _1 | 0.436 | 0.397 | 0.025 |
RASE _2 | 0.083 | 0.055 | 0.022 |
n = 100 | n = 300 | n = 700 | ||||||||||
Parameter | True Value | MSE | Var | Bias | MSE | Var | Bias | MSE | Var | Bias | ||
\alpha_1 | \frac{0.2}{\sqrt{0.53}} | 0.038 | 0.037 | 0.030 | 0.018 | 0.017 | 0.021 | 0.009 | 0.009 | 0.007 | ||
\alpha_2 | \frac{-0.7}{\sqrt{0.53}} | 0.674 | 0.530 | 0.378 | 0.180 | 0.168 | 0.109 | 0.001 | 0.001 | 0.007 | ||
\psi | 0.5 | 0.009 | 0.009 | 0.009 | 0.002 | 0.002 | -0.001 | 0.001 | 0.001 | 0 | ||
\sigma^2 | 0.2 | 0.018 | 0.017 | -0.034 | 0.004 | 0.004 | -0.001 | 0.001 | 0.001 | -0.004 | ||
\delta | 1 | 0.108 | 0.104 | -0.060 | 0.014 | 0.013 | -0.028 | 0.005 | 0.004 | -0.007 |
n | User | System | Elapsed | |
100 | Case 1 | 146.328 | 2.723 | 151.925 |
Case 2 | 189.914 | 2.816 | 196.018 | |
Caee 3 | 143.048 | 2.206 | 148.332 | |
300 | Case 1 | 123.117 | 2.297 | 127.611 |
Case 2 | 256.243 | 3.896 | 263.757 | |
Case 3 | 50.093 | 0.829 | 51.795 | |
700 | Case 1 | 135.031 | 2.781 | 140.028 |
Case 2 | 203.583 | 3.370 | 207.119 | |
Case 3 | 82.259 | 1.514 | 84.915 |
n | Criterion | Mean | Median | Var |
100 | RASE _1 | 1.269 | 1.073 | 0.644 |
RASE _2 | 0.199 | 0.160 | 0.113 | |
300 | RASE _1 | 0.706 | 0.625 | 0.182 |
RASE _2 | 0.104 | 0.099 | 0.002 | |
700 | RASE _1 | 0.478 | 0.429 | 0.041 |
RASE _2 | 0.070 | 0.067 | 0.001 |
n = 100 | n = 300 | n = 700 | ||||||||||
Parameter | True Value | MSE | Var | Bias | MSE | Var | Bias | MSE | Var | Bias | ||
\alpha_1 | \frac{\sqrt{3}}{3} | 0.017 | 0.017 | -0.010 | 0.005 | 0.005 | 0.001 | 0.002 | 0.002 | 0.004 | ||
\alpha_2 | \frac{\sqrt{6}}{3} | 0.030 | 0.030 | -0.022 | 0.002 | 0.002 | -0.005 | 0.001 | 0.001 | -0.004 | ||
\psi | 0.5 | 0.010 | 0.010 | 0.002 | 0.002 | 0.002 | -0.001 | 0.001 | 0.001 | 0 | ||
\sigma^2 | 0.2 | 0.011 | 0.011 | -0.029 | 0.004 | 0.004 | 0.006 | 0.001 | 0.001 | -0.002 | ||
\delta | 0.7 | 0.112 | 0.106 | -0.079 | 0.033 | 0.030 | -0.057 | 0.008 | 0.008 | -0.014 |
n | Criterion | Mean | Median | Var |
100 | RASE _1 | 1.097 | 0.908 | 0.559 |
RASE _2 | 0.333 | 0.139 | 0.766 | |
300 | RASE _1 | 0.618 | 0.540 | 0.095 |
RASE _2 | 0.085 | 0.064 | 0.062 | |
700 | RASE _1 | 0.438 | 0.403 | 0.024 |
RASE _2 | 0.049 | 0.047 | 0 |
n = 100 | n = 300 | n = 700 | ||||||||||
Parameter | True Value | MSE | Var | Bias | MSE | Var | Bias | MSE | Var | Bias | ||
\alpha_1 | \frac{\sqrt{3}}{3} | 0.022 | 0.021 | -0.026 | 0.004 | 0.004 | -0.003 | 0.001 | 0.001 | -0.004 | ||
\alpha_2 | \frac{\sqrt{6}}{3} | 0.157 | 0.149 | -0.091 | 0.012 | 0.012 | -0.008 | 0.001 | 0.001 | 0.001 | ||
\psi_1 | -0.7 | 0.019 | 0.016 | -0.054 | 0.004 | 0.004 | -0.012 | 0.001 | 0.001 | -0.004 | ||
\psi_2 | 0.2 | 0.020 | 0.016 | -0.068 | 0.004 | 0.003 | -0.017 | 0.001 | 0.001 | -0.007 | ||
\sigma^2 | 0.2 | 0.013 | 0.013 | -0.011 | 0.006 | 0.005 | 0.015 | 0.003 | 0.003 | 0.007 | ||
\delta | 0.7 | 0.153 | 0.127 | -0.159 | 0.068 | 0.058 | -0.101 | 0.036 | 0.033 | -0.050 |
n | Criterion | Mean | Median | Var |
100 | RASE _1 | 1.538 | 1.238 | 1.284 |
RASE _2 | 0.319 | 0.211 | 0.408 | |
300 | RASE _1 | 0.771 | 0.701 | 0.232 |
RASE _2 | 0.125 | 0.117 | 0.003 | |
700 | RASE _1 | 0.527 | 0.467 | 0.069 |
RASE _2 | 0.083 | 0.080 | 0.001 |
n = 100 | n = 300 | n = 700 | ||||||||||
Parameter | True Value | MSE | Var | Bias | MSE | Var | Bias | MSE | Var | Bias | ||
\alpha_1 | \frac{\sqrt{3}}{3} | 0.030 | 0.029 | -0.016 | 0.007 | 0.007 | -0.001 | 0.003 | 0.003 | 0.005 | ||
\alpha_2 | \frac{\sqrt{6}}{3} | 0.104 | 0.099 | -0.071 | 0.003 | 0.003 | -0.006 | 0.001 | 0.001 | -0.006 | ||
\psi | 0.5 | 0.010 | 0.010 | 0.003 | 0.002 | 0.002 | 0 | 0.001 | 0.001 | 0 | ||
\sigma^2 | 0.2 | 0.019 | 0.018 | -0.035 | 0.004 | 0.004 | -0.001 | 0.001 | 0.001 | -0.005 | ||
\delta | 1 | 0.107 | 0.104 | -0.053 | 0.015 | 0.014 | -0.028 | 0.004 | 0.004 | -0.008 |
n | User | System | Elapsed | |
100 | Case 4 | 126.892 | 2.917 | 194.251 |
Case 5 | 193.034 | 2.961 | 199.880 | |
Caee 6 | 111.520 | 2.566 | 173.009 | |
300 | Case 4 | 75.457 | 1.741 | 109.605 |
Case 5 | 168.304 | 2.418 | 172.591 | |
Case 6 | 32.806 | 0.786 | 48.882 | |
700 | Case 4 | 65.384 | 1.678 | 68.649 |
Case 5 | 177.791 | 2.418 | 172.591 | |
Case 6 | 48.716 | 1.256 | 56.743 |
h-order | |||||
h=1 | h=2 | h=3 | h=4 | h=5 | |
p values | 0.768 | 0.026 | 0.061 | 0.091 | 0.050 |
Parameter | Indep. Normal | AR(2) -Normal | AR(2) -Skew-Normal |
\alpha_1 | 0.001 | 0.649 | 0.646 |
\alpha_2 | 0.074 | 0.049 | 0.051 |
\alpha_3 | -0.996 | -0.757 | -0.760 |
\alpha_4 | 0.048 | 0.053 | 0.054 |
\gamma_1 | -1.095 | -0.687 | -0.688 |
\gamma_2 | 2.212 | 1.563 | 1.567 |
\gamma_3 | -1.917 | -1.490 | -1.497 |
\gamma_4 | 0.625 | 0.573 | 0.582 |
\eta_1 | 0.548 | 0.642 | 0.646 |
\eta_2 | 5.524 | 5.751 | 5.741 |
\eta_3 | 14.573 | 14.609 | 14.608 |
\eta_4 | 15.032 | 15.018 | 15.007 |
\psi_1 | – | 0.115 | 0.114 |
\psi_2 | – | 0.194 | 0.194 |
\sigma^2 | 2.061 | 2.916 | 2.958 |
\delta | – | – | 0.086 |
MSE | 5.168 | 3.190 | 3.161 |
MRSE | 0.314 | 0.194 | 0.192 |
Total Sample Size | 356 | 356 | 356 |
Training Set Size | 300 | 300 | 300 |
Test Set Size | 56 | 56 | 56 |