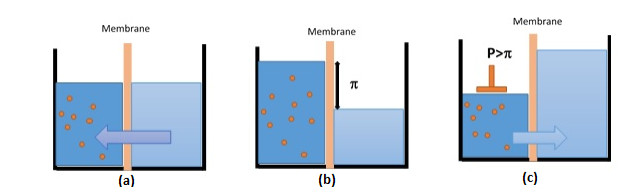
Clean water is a necessity for many organisms, especially human life. Due to many factors, there is a significant shortage of potable water. This has led to efforts involving recovering water from wastewater or the sea through different technologies. Recently, the desalination of seawater via the reverse osmosis system has shown to be a promising method for drinking water treatment and recovery. Such a technique relies on mathematical models based on many parameters, resulting in special PDEs to model the reverse osmosis system. This paper develops a numerical method to solve a reverse osmosis model. The governing PDE is converted into a Sylvester equation that is proved to be uniquely solvable, stable, consistent, and convergent. The numerical scheme developed is validated with experimental data from the literature, and some numerical simulations.
Citation: Saloua Helali, Anouar Ben Mabrouk, Mohamed Rashad, Nizar Bel Hadj Ali, Munirah A. Ȧlanazi, Marwah A. Alsharif, Elham M. Al-Ali, Lubna A. Alharbi, Manahil S. Mustafa. A Lyapunov-Sylvester numerical method for solving a reverse osmosis model[J]. AIMS Mathematics, 2024, 9(7): 17531-17554. doi: 10.3934/math.2024852
[1] | Siting Yu, Jingjing Peng, Zengao Tang, Zhenyun Peng . Iterative methods to solve the constrained Sylvester equation. AIMS Mathematics, 2023, 8(9): 21531-21553. doi: 10.3934/math.20231097 |
[2] | Imran Talib, Md. Nur Alam, Dumitru Baleanu, Danish Zaidi, Ammarah Marriyam . A new integral operational matrix with applications to multi-order fractional differential equations. AIMS Mathematics, 2021, 6(8): 8742-8771. doi: 10.3934/math.2021508 |
[3] | Cagnur Corekli . The SIPG method of Dirichlet boundary optimal control problems with weakly imposed boundary conditions. AIMS Mathematics, 2022, 7(4): 6711-6742. doi: 10.3934/math.2022375 |
[4] | Yumei Chen, Jiajie Zhang, Chao Pan . Numerical approximation of a variable-order time fractional advection-reaction-diffusion model via shifted Gegenbauer polynomials. AIMS Mathematics, 2022, 7(8): 15612-15632. doi: 10.3934/math.2022855 |
[5] | Abdur Rehman, Ivan Kyrchei, Muhammad Zia Ur Rahman, Víctor Leiva, Cecilia Castro . Solvability and algorithm for Sylvester-type quaternion matrix equations with potential applications. AIMS Mathematics, 2024, 9(8): 19967-19996. doi: 10.3934/math.2024974 |
[6] | Huiling Wang, Nian-Ci Wu, Yufeng Nie . Two accelerated gradient-based iteration methods for solving the Sylvester matrix equation AX + XB = C. AIMS Mathematics, 2024, 9(12): 34734-34752. doi: 10.3934/math.20241654 |
[7] | Haifa Bin Jebreen, Hongzhou Wang . On the effective method for the space-fractional advection-diffusion equation by the Galerkin method. AIMS Mathematics, 2024, 9(9): 24143-24162. doi: 10.3934/math.20241173 |
[8] | Yones Esmaeelzade Aghdam, Hamid Mesgarani, Zeinab Asadi, Van Thinh Nguyen . Investigation and analysis of the numerical approach to solve the multi-term time-fractional advection-diffusion model. AIMS Mathematics, 2023, 8(12): 29474-29489. doi: 10.3934/math.20231509 |
[9] | Fengxia Zhang, Ying Li, Jianli Zhao . The semi-tensor product method for special least squares solutions of the complex generalized Sylvester matrix equation. AIMS Mathematics, 2023, 8(3): 5200-5215. doi: 10.3934/math.2023261 |
[10] | Khalid K. Ali, Mohamed A. Abd El Salam, Mohamed S. Mohamed . Chebyshev fifth-kind series approximation for generalized space fractional partial differential equations. AIMS Mathematics, 2022, 7(5): 7759-7780. doi: 10.3934/math.2022436 |
Clean water is a necessity for many organisms, especially human life. Due to many factors, there is a significant shortage of potable water. This has led to efforts involving recovering water from wastewater or the sea through different technologies. Recently, the desalination of seawater via the reverse osmosis system has shown to be a promising method for drinking water treatment and recovery. Such a technique relies on mathematical models based on many parameters, resulting in special PDEs to model the reverse osmosis system. This paper develops a numerical method to solve a reverse osmosis model. The governing PDE is converted into a Sylvester equation that is proved to be uniquely solvable, stable, consistent, and convergent. The numerical scheme developed is validated with experimental data from the literature, and some numerical simulations.
Water scarcity is a pressing global challenge that has prompted innovative solutions to ensure a sustainable and reliable freshwater supply. As the world's population continues to grow and traditional water sources face increasing stress due to climate change, population density, and industrialization, the need for alternative water resources is imperative. One such solution at the forefront of addressing this issue is water desalination. Water desalination is the process of removing salt and other impurities from seawater or brackish water to make it suitable for human consumption, agriculture, and industrial purposes.
Reverse osmosis (RO) is currently the most advanced technique for desalinating brackish water and seawater, due to its excellent versatility, ease of use, and high efficiency. In particular, the RO process is distinguished by its ability to handle a variety of plant designs and capacities, as well as its high salt rejection rate of 99% and recovery rate of up to 40%.
The process of osmosis occurs by applying pulsating pressure through the feed water that penetrates the fine pores of semi-permeable membranes. In practice, the reverse osmosis semi-permeable membranes are competitive, especially for seawater desalination. Recently, with the need to recycle many materials and wastes, reverse osmosis process has been implemented in municipal wastewater treatment.
Such a technique relies on mathematical models based on advection-diffusion PDEs to model the reverse osmosis system. Recall, that the concentration distribution process is always known as a time-dependent function depending on two-dimensional space variables, leading to a parabolic partial differential equation known as the advection-diffusion equation. The advection process describes heat transfer resulting from flow, while the diffusion phenomenon is due to the propagation of heat as a wave from higher-temperature water to lower-temperature water.
In the literature, many numerical methods have been applied to approximate solutions of the advection-diffusion or the reverse osmosis, such as one-dimensional discrete models based on Riemann sum numerical integration methods combined with the stretching transformations ([18,26,27,28]), or fractional differential equations combined with wavelets ([2,3,4,6,21]). Hadadian et al. [20] developed a mathematical simulation model for the RO process based on the mass, material, and energy balances considering the concentration polarization. Chen and Qin [10] developed a mathematical model to separate glucose from water through a reverse osmosis membrane. They optimized the model parameters, including the hydraulic permeability constant, reflection coefficient, and solute transport coefficient. Elnour et al. [15] developed an algorithm for designing RO systems. The algorithm is validated using operational data from a local plant and was found to be capable of simulating the actual plant operation with an average error of less than 5% for the majority of the system variables. Maure and Mungkasi [28] studied a mathematical equation in a reverse osmosis system of salt water at a given position and time. They converted the partial differential equation into ordinary differential equations with a transformation method. Noeiaghdam et al. [29] presented a novel mathematical method to find the optimal solution for the RO process. They applied the single exponential and double exponential decay to find the approximate solution of the RO.
In the present paper, numerical methods are developed to approximate the solutions of the continuous problem governing a reverse osmosis model by replacing the spacial partial derivatives with 2-dimensional finite-difference approximators to transform the continuous boundary-value problem into a linear algebraic system. The resulting method is analyzed for error, stability, solvability, and convergence. The method is shown to be more performant relative to tridiagonal systems. It transforms the continuous problem to the so-called Lyapunov-Sylvester equation of the form AX+XB=C. Thus, the problem then involves the study of the linear operator L(X)=AX+XB defined on an appropriate matrix space, for fixed matrices A, B, and C depending on the discretization method and the problem parameters. The authors refer to the most recent work due to [23] for the inversion of such operators.
To check the approximate solution, we always adapt a small space step-size, or equivalently, a large discrete system, which hurts the increase in the algorithms' time of finding the solution. In this case, many methods such as Krylov's are applied to reduce the time of reckoning. In [30], for example, two ways have been investigated based on Krylov's method for solving a large-scale system of differential equations, by applying in one way an exponential projection technique, and in the other by dropping the original large-size system to a small-size one. In [31], a close problem and technique to the present paper are used related, especially, to the Sylvester matrix. More precisely, large-scale differential Sylvester matrix equations characterized by low-rank right-hand sides are investigated via an extended global Arnoldi process. The authors first approximated the exponential matrix in the exact solution via a global extended Krylov method, and secondly, they applied an extended Arnoldi algorithm to get a low-rank approximation of the Sylvester equation solution.
In the same direction, the authors in [32,33] investigated a large-scale generalized Sylvester equation by projecting the initial problem onto a block Krylov subspace to get a small-scale problem, which is then solved via an orthogonality Galerkin condition and Rosenbrock or the so-called backward differential formula method. The same authors applied both a modified global Arnoldi algorithm and an extended global Arnoldi algorithm for solving a large-scale Sylvester system of ordinary differential equations. The basic idea also involves a projection technique on the extended global Krylov or global Krylov subspaces. The main property of the systems investigated is the possibility of a non-invertible corresponding matrix, which requires the projection of a matrix exponential function to get solvable low-dimensional systems.
Again using Krylov's method in one of its variants, a numerical approach was developed in [34] to solve a large differential Stein matrix equation in a nonsymmetric case. The authors used an extended block Arnoldi algorithm and a backward differentiation formula. In [35], a Lyapunov differential equation was studied by applying an extended nonsymmetric block Lanczos method, which allows for the transformation of the original large-scale problem into a small-scale system using the extended block Krylov subspace projection and the extended nonsymmetric block Lanczos algorithm. Next, backward differentiation formula is applied to solve the low-dimensional Lyapunov equation. We conclude from these studies that the strange structures in dynamical systems need compatible tools to be well investigated, such as fractional derivatives, which, although being old concepts, have recently seen renewed interest in many fields.
Many problems and models involving PDEs are re-considered in the fractional calculus framework, where more adequate solutions to real-world problems in nature are provided accordingly. In [36], an exponential-cotangent derivative was involved in the well-known Riemann-Liouville approach to study the solutions of some fractional differential equations using the Laplace transform. The theoretical findings are applied to an SIR-type model. In [37], the problem of stability was investigated for a class of conformable linear systems in an infinite-dimensional form. α-exponential stability, asymptotic stability, and uniform stability variants have been studied via conformable fractional derivatives and semigroup theory. Sadek et al, [38] studied a Lyapunov-Sylvester type differential equation using the Bernstein polynomials collocation method. As in the present paper, this technique allowed for the transformation of the original problem to a linear system of algebraic equations, which is then solved by using an iterative method.
In [39], a fascinating link between the frameworks of fractional calculus and the Lyapunov-Sylvester equation was given. The authors considered a class of fractional differential matrix equations and applied some fractional backward differentiation formulas techniques to numerically approximate the solutions of the original problem. An important problem when investigating PDEs concerns the controllability of the provided scheme or solution, and especially the observation of strange behaviors such as eventual chaotic or fractal behavior. In this way, in [40], the authors proposed a control technique for a non-homogeneous continuous-time fractal dynamical system derived from the Lyapunov equation.
The paper is organized as follows. In Section 2, some basic ideas from [23] on the invertibility of operators of the form L(X)=AX+XB are recalled. In Section 3, the discrete scheme is introduced. The continuous model describing the reverse osmosis process is converted into a discrete algebraic system involving the Lyapunov-Sylvester equation in a discrete form. The solvability of the discrete problem obtained in Section 3 is investigated in Section 4. Section 5 is devoted to the convergence, consistency, and stability of the method. Section 6 is concerned with the experimental results and their discussion. Section 7 is an appendix devoted to some preliminary results, some comments on existing models, and some mathematical proofs. Section 8 is a conclusion.
The resolution of the so-called Lyapunov equation
AX+XAT=C, | (2.1) |
where A and C are given matrices and X is an unknown one, is often of interest, and has been the object of some studies until the 60's [22]. However, these studies have been characterized by restrictive aspects. For example, in [22], a Kronecker product-based method was developed to resolve Eq (2.1). The method consisted of a recursive scheme using the well-known Caylay result, and it gives explicit solutions in 2 and 3-dimensional cases. Such cases are not complicated and a verification or a computation can be proved with simpler and more direct methods than the one in [22]. The equation has been re-considered by many authors due to its relation and application in PDEs, the theory of stability, and dynamical systems. Kohaupt has focused on general forms of the cited equation. The author in [23] considered the matrix eigenvalue problem
AX+XB=μX. | (2.2) |
A large study of this problem has been provided, and special cases, such as B=AT, B=A∗, A, and B diagonalizable, etc., have been discussed. The main result of [23] is presented in the following theorem.
Theorem 2.1. [23] Let A∈Cn×n and B∈Cm×m both be diagonalizable. Let αi be the eigenvalues of A and ui be the associated right eigenvectors for i=1,...,n. Let βi be the eigenvalues of B and vi be the associated left eigenvectors for i=1,...,m, i.e.,
Aui=αiui,i=1,...,n,andviB=βivi,i=1,...,m. |
Then,
λij=αi+βj,i=1,...,n,; j=1,...,m, |
are the mn eigenvalues of (2.2) and
Wi,j=uivj,i=1,...,n,; j=1,...,m, |
are the associated eigenmatrices.
Osmosis is essentially a natural process that occurs when water molecules migrate from a solution with low solute concentration (low osmotic pressure) to a solution with high solute concentration (high osmotic pressure) via a semipermeable membrane (Figure 1(a)). The osmosis process is said to be at an equilibrium condition when the chemical potentials across the membrane are equal (Figure 1(b)). The flow of water molecules can be halted or reversed by applying external pressure to the solution (the feed solution) that has a higher concentration. If the applied pressure differential is greater than the osmotic pressure difference across the membrane, water molecules are compelled to flow against the direction of the natural osmosis phenomenon. Reverse osmosis is the process occurring in this case, s illustrated by Figure 1(c). The symbol P designates the process or external pressure, while the symbol π is the osmotic pressure.
The feed water stream separates into two streams in a continuous RO process, as represented in Figure 2. Water molecules that have passed through the membrane constitute the first stream. Permeate or product water is the name of this low-solute stream. A smaller number of water molecules and the rejected solutes are the constituents of the second stream. This stream is known as brine, concentrate, or reject because it contains more solutes than feed. The feed channel is characterized by three parameters: feed flow rate Fd, concentration Cd, and pressure Pd ([24,25,41,42,43,44,45]).
The permeate channel and the feed channel are located on opposite sides of the membrane. The feed channel is filled with feed solution at one end, while the rejected solution emerges at the other. The fluid flow in the feed channel is parallel to the x-coordinate, while in the permeate channel, it is parallel to the y-coordinate. Therefore, a two-dimensional advection-diffusion equation will be investigated as a governing model for the reverse osmosis phenomenon, which is expressed by the following time-dependent 2D-model,
{∂C∂t+y∂C∂x=αΔC,(t,x,y)∈(0,∞)×Ω,C(t,0,y)=C(t,x,∞)=Cd,(t,x,y)∈(0,∞)×Ω,−D∂C∂y(t,x,0)=qC(t,x,0),(t,x)∈(0,∞)×Ω1,C(0,x,y)=Cd,(x,y)∈Ω, | (3.1) |
where (x,y) is the pair of R2-space variables, and C=C(t,x,y) is the concentration of salt solution in a semipermeable membrane at point (x,y) and the instant t. The quantity ∂C∂t is the time derivative of C(t,x,y), ∂C∂x is the derivative of C(t,x,y) relative to the first space variable x, and ΔC=∂2C∂y2+∂2C∂x2 is the Laplacian of C(t,x,y) resulting from the Laplace operator applied to it. The real number parameter q represents the water flow rates in semipermeable distribution, D is the salt diffusivity in water, δ is the distance from the semipermeable boundary to the center of the channel, cd is the concentration away from semipermeable membranes, ν0 is the horizontal velocity measured at a distance δ from the semipermeable boundary, α=Dδν0. The time-dependent two-dimensional advection-diffusion equation describes the concentration of a salt solution. The schematic illustration given in Figure 3 below explains the phenomenon, where v(y) is the speed of the fluid flowing through the duct, parallel to the x-axis. The domain Ω=Ω1×Ω2 is a rectangular strip as in Figure 3. Here, we recall that the components Ω1 and Ω2 are in general different, (for example, Ω1=[0,Lx] and Ω2=[0,Ly]). Numerically, we may take two different steps hx=LxN in x and hy=LyN in y with the same number of discretization points N, or we may also take two different sizes Nx and Ny leading to hx=LxNx in x and hy=LyNy in y. However, it is well known in numerical analysis that these facts do not have a great effect on the approximations. For this, we restrict ourselves to an 'artificial' square region for the discretization as well as the numerical developments.
From a physical or natural interpretation point of view, we take a semi-infinite region in the R2+ quadrant. At t=0, the system is assumed to be still at rest, which means that the concentration of salts is still the same as the seawater to be filtered, which in turn leads to a natural initial condition C(0,x,y)=C0. The notation or condition x=∞ means that we are 'very' far from the semi-permeable membrane at y=0, and the 'artificial' condition y=∞ means that we are 'very' far from the membrane. In our case, we assume that the concentration away from the membrane is the same as that before flowing in the filter. In the discrete and numeric validation, we symbolize this as
x=∞⟺x=Lxandy=∞⟺y=Ly. |
The numerical method is based on a simple classical 2-dimensional finite difference scheme, where the differential operators are estimated as follows. Let N∈N and s satisfying Ns=L1−L0 be a space step. For the reasons raised above, without loss of generality, we take in our numerical solutions L0=0 and L1=L. Next, consider the net space
Ωs={(xj,ym);xj=L0+js, ym=L0+ms, 0≤j,m≤N}. |
Let u=(uj,m) be a mesh function defined on Ωs. Next, we introduce the operators
∇+xuj,m=uj+1,m−uj,msand∇−xuj,m=uj,m−uj−1,ms, |
and
∇+yuj,m=uj,m+1−uj,msand∇−yuj,m=uj,m−uj,m−1s. |
The first-order derivatives in x and y are approximated by
∂u∂x≃12(∇+xuj,m+∇−xuj,m),and∂u∂y≃12(∇+yuj,m+∇−yuj,m), |
respectively, while the second-order operators in x and y are
Δx=∇+x∇−x=∇−x∇+xandΔy=∇+y∇−y=∇−y∇+y, |
and, finally, the Laplace operator is
Δ=Δx+Δy. |
Let l=Δt be the time step and tn=t0+nl, n∈N be the discrete time grid. For (j,m)∈I={0,1,…,N}2 and n≥0, Cnj,m will be the net function C(tn,xj,ym) (also used to designate the numerical solution). The following discrete approximations will be applied for the time derivatives,
∂C∂t≃12∇tCnj,m=Cn+1j,m−Cn−1j,m2l. |
Our numerical scheme is based on a calibrating method which consists of a barycenter ponderations according to the time for the final space derivative to be applied in the approximation model. Precisely, we set
^Cnj,m=μCn+1j,m+(1−2μ)Cnj,m+μCn−1j,m, |
where the weight parameter μ∈[0,1].
The calibration method is usually used to allow for the best numerical approximation of the real solution. As this solution is, in fact, unknown, we did not know a priori which time position is the closer to it. The variation of the weights allows us to investigate this problem. See for example [7,8,9,11,12]
As a result, the approximate solution Cnj,m is defined as a solution to the discrete problem
{∇tCnj,m+ym(∇+x^Cnj,m+∇−x^Cnj,m)=2αΔ^Cnj,m,0≤j,m≤N,n≥0,C0j,m=Cn0,m=Cnj,N=Cd,0≤j,m≤N,n≥0,−D(∇+yCnj,0+∇−yCnj,0)=2qCnj,0,0≤j≤N,n≥0. | (3.2) |
For the rest of the paper, for n≥0, denote by Cn=(Cnj,m)0≤j,m≤N the matrix with coefficients Cnj,m.
The first result in this paper concerns the solvability of the system (3.2) above, and is stated as follows.
Theorem 4.1. System (3.2) is uniquely solvable.
Proof. Denote
β=2qhD,σ=2αls2,σm=lyms,λ=μσ,andη=(1−2μ)σ. |
From system (3.2), it is obtained that
Cn+1j,m−Cn−1j,m=σm[μ(Cn+1j−1,m−Cn+1j+1,m)+(1−2μ)(Cnj−1,m−Cnj+1,m)+μ(Cn−1j−1,m−Cn−1j+1,m)]+σ[μ(Cn+1j−1,m−4Cn+1j,m+Cn+1j+1,m+Cn+1j,m−1+Cn+1j,m+1)+(1−2μ)(Cnj−1,m−4Cnj,m+Cnj+1,m+Cnj,m−1+Cnj,m+1)+μ(Cn−1j−1,m−4Cn−1j,m+Cn−1j+1,m+Cn−1j,m−1+Cn−1j,m+1)]. |
Now, denote
αm=μ(σ+σm),βm=μ(σ−σm), |
and, similarly,
am=(1−2μ)(σ+σm),bm=(1−2μ)(σ−σm), |
and regrouping the terms in n+1, n, and n−1, we obtain
Cn+1j,m=αmCn+1j−1,m−2λCn+1j,m+βmCn+1j+1,m+λ[Cn+1j,m−1−2Cn+1j,m+Cn+1j,m+1]+amCnj−1,m−2ηCnj,m+bmCnj+1,m+η[Cnj,m−1−2Cnj,m+Cnj,m+1]αmCn−1j−1,m−2λCn−1j,m+βmCn−1j+1,m+λ[Cn−1j,m−1−2Cn−1j,m+Cn−1j,m+1]+Cn−1j,m. | (4.1) |
Otherwise, we may write this system as the inner product of vectors as
Cn+1j,m=[αm−2λβm][Cn+1j−1,mCn+1j,mCn+1j+1,m]T+λ[Cn+1j,m−1Cn+1j,mCn+1j,m+1][1−21]T+[am−2ηbm][Cnj−1,mCnj,mCnj+1,m]T+η[Cnj,m−1Cnj,mCnj,m+1][1−21]T+[αm−2λβm][Cn−1j−1,mCn−1j,mCn−1j+1,m]T+λ[Cn−1j,m−1Cn−1j,mCn−1j,m+1][1−21]T+Cn−1j,m, | (4.2) |
where the upper script [.]T designates the transpose. Next, let Am, Bm, and R be the tridiagonal matrices of size (N+1,N+1) whom coefficients are, respectively,
Am(j−1,j)=αm,Am(j,j)=−2λ,Am(j+1,j)=βm, |
Bm(j−1,j)=am,Bm(j,j)=−2η,Bm(j+1,j)=βm, |
and
R(j,j)=−2,R(j−1,j)=R(j+1,j)=1. |
System (4.2) above may be transformed into a Lyapunov-Sylvester form as
(I−Am)Cn+1−λCn+1R=BmCn+ηCnR+(I+Am)Cn−1+λCn−1R, | (4.3) |
where I=IN+1 is the identity matrix of size N+1. Now, if we denote for two matrices A,B∈MN+1(C), the Lyapunov-Sylvester operator
LA,B(X)=AX+XB,∀X∈MN+1(C), |
we obtain a dynamic Lyapunov-Sylvester equation of the form
LI−Am,−λR(Cn+1)=LBm,ηR(Cn)+LI+Am,λR(Cn−1). | (4.4) |
Notice that the matrices (I−Am) and λR have different eigenvalues (see Appendix 7.1, Lemma 7.1). As a consequence, due to Theorem 2.1 (see also [23]), the linear Lyapunov-Sylvester operator LI−Am,−λR is invertible. So, the result follows.
To show the consistency of the numerical scheme, we use the local truncation error due to (3.2). The result is given in the following lemma.
Lemma 5.1. The numerical scheme is consistent at the order (l2+s2).
Proof. To show this lemma, we will compute the principal part due to the truncation error applied to (3.2). To do this, we start by evaluating the necessary elements of the discrete scheme. So, by assuming that C is sufficiently regular, standard computations yield that
Cn+1j+1,m=[C+∂C∂xs+∂2C∂x2s22+∂3C∂x3s36]+[∂C∂t+∂2C∂x∂ts+∂3C∂x2∂ts22+∂4C∂x3∂ts36]l+[∂2C∂t2+∂3C∂x∂t2s+∂4C∂x2∂t2s22+∂5C∂x3∂t2s36]l22+[∂3C∂t3+∂4C∂x∂t3s+∂5C∂x2∂t3s22+∂6C∂x3∂t3s36]l36+o(l2+s2). | (5.1) |
Similarly, we have
Cn+1j−1,m=[C−∂C∂xs+∂2C∂x2s22−∂3C∂x3s36]+[∂C∂t−∂2C∂x∂ts+∂3C∂x2∂ts22−∂4C∂x3∂ts36]l+[∂2C∂t2−∂3C∂x∂t2s+∂4C∂x2∂t2s22−∂5C∂x3∂t2s36]l22+[∂3C∂t3−∂4C∂x∂t3s+∂5C∂x2∂t3s22−∂6C∂x3∂t3s36]l36+o(l2+s2). | (5.2) |
By canceling the terms in s (taking s=0), we obtain
Cn+1j,m=C+∂C∂tl+∂2C∂t2l22+∂3C∂t3l36+o(l2+s2). | (5.3) |
Now, by replacing x with y in Eqs (5.1) and (5.2) above, we deduce that
Cn+1j,m+1=[C+∂C∂ys+∂2C∂y2s22+∂3C∂y3s36]+[∂C∂t+∂2C∂y∂ts+∂3C∂y2∂ts22+∂4C∂y3∂ts36]l+[∂2C∂t2+∂3C∂y∂t2s+∂4C∂y2∂t2s22+∂5C∂y3∂t2s36]l22+[∂3C∂t3+∂4C∂y∂t3s+∂5C∂y2∂t3s22+∂6C∂y3∂t3s36]l36+o(l2+s2). | (5.4) |
Similarly, we have
Cn+1j,m−1=[C−∂C∂ys+∂2C∂y2s22−∂3C∂y3s36]+[∂C∂t−∂2C∂y∂ts+∂3C∂y2∂ts22−∂4C∂y3∂ts36]l+[∂2C∂t2−∂3C∂y∂t2s+∂4C∂y2∂t2s22−∂5C∂y3∂t2s36]l22+[∂3C∂t3−∂4C∂y∂t3s+∂5C∂y2∂t3s22−∂6C∂y3∂t3s36]l36+o(l2+s2). | (5.5) |
Now, by canceling l (taking l=0) in all the equations above, we get
{Cnj+1,m=C+∂C∂xs+∂2C∂x2s22+∂3C∂x3s36+o(l2+s2),Cnj−1,m=C−∂C∂xs+∂2C∂x2s22−∂3C∂x3s36+o(l2+s2),Cnj,m+1=C+∂C∂ys+∂2C∂y2s22+∂3C∂y3s36+o(l2+s2),Cnj,m−1=C−∂C∂ys+∂2C∂y2s22−∂3C∂y3s36+o(l2+s2). | (5.6) |
Now, as previously, by replacing l with −l in the equations above, we get
Cn−1j+1,m=[C+∂C∂xs+∂2C∂x2s22+∂3C∂x3s36]−[∂C∂t+∂2C∂x∂ts+∂3C∂x2∂ts22+∂4C∂x3∂ts36]l+[∂2C∂t2+∂3C∂x∂t2s+∂4C∂x2∂t2s22+∂5C∂x3∂t2s36]l22−[∂3C∂t3+∂4C∂x∂t3s+∂5C∂x2∂t3s22+∂6C∂x3∂t3s36]l36+o(l2+s2). | (5.7) |
In the same way, we get
Cn−1j−1,m=[C−∂C∂xs+∂2C∂x2s22−∂3C∂x3s36]−[∂C∂t−∂2C∂x∂ts+∂3C∂x2∂ts22−∂4C∂x3∂ts36]l+[∂2C∂t2−∂3C∂x∂t2s+∂4C∂x2∂t2s22−∂5C∂x3∂t2s36]l22−[∂3C∂t3−∂4C∂x∂t3s+∂5C∂x2∂t3s22−∂6C∂x3∂t3s36]l36+o(l2+s2). | (5.8) |
Similarly, by canceling s in the last equation, we get
Cn−1j,m=C−∂C∂tl+∂2C∂t2l22−∂3C∂t3l36+o(l2+s2). | (5.9) |
Now, by interchanging the role of j and m in (5.7), we obtain
Cn−1j,m+1=[C+∂C∂ys+∂2C∂y2s22+∂3C∂y3s36]−[∂C∂t+∂2C∂y∂ts+∂3C∂y2∂ts22+∂4C∂y3∂ts36]l+[∂2C∂t2+∂3C∂y∂t2s+∂4C∂y2∂t2s22+∂5C∂y3∂t2s36]l22−[∂3C∂t3+∂4C∂y∂t3s+∂5C∂y2∂t3s22+∂6C∂y3∂t3s36]l36+o(l2+s2). | (5.10) |
Now, by replacing l with −l in (5.10), we obtain
Cn−1j,m−1=[C−∂C∂ys+∂2C∂y2s22−∂3C∂y3s36]−[∂C∂t−∂2C∂y∂ts+∂3C∂y2∂ts22−∂4C∂y3∂ts36]l+[∂2C∂t2−∂3C∂y∂t2s+∂4C∂y2∂t2s22−∂5C∂y3∂t2s36]l22−[∂3C∂t3−∂4C∂y∂t3s+∂5C∂y2∂t3s22−∂6C∂y3∂t3s36]l36+o(l2+s2). | (5.11) |
All the equations above permit us to deduce the following principal part of the truncation error as
L(x,y,t)=[2ym∂3C∂x3−α(∂4C∂x4+∂4C∂y4)]s212+[13∂3C∂t3+2μym∂3C∂x∂t2−α(∂4C∂x2∂t2+∂4C∂y2∂t2)]l22+o(l2+s2), |
which tends to 0 as (l,s) tends to 0. This means that the method is consistent.
To examine the stability of the numerical method, we will apply the Von-Neumann technique by investigating the impact of the scheme on an isolated Fourier mode.
Denote Cnj,m as the solution of the numerical scheme, and ˇCnj,m as the numerical value obtained subject to computer round-off errors. Next, denote
Snj,m=Cnj,m−ˇCnj,m=enlψeijsφxeimsφy=XnYjZm, |
where ψ=ψ1+iψ2 is a complex number, φx and φy are real, and i2=−1. By applying the Von-Neumann criterion for stability, we shall show that
|X|≤1. | (5.12) |
Indeed, from (3.2) or its developed form (4.1), after canceling Xn−1Yj−1Zm−1, and regrouping the terms in X2 and X, it holds that
X2[Z(βmY2−(1+2λ)Y+αm)+λY(Z−1)2]+X[Z(βmY2−2λY+αm)+λY(Z−1)2]+Z(βmY2+(1−2λ)Y+αm)+λY(Z−1)2=0. | (5.13) |
Now, notice that
(Z−1)2=4Zsin2(sφy2). |
Equation (5.13) may be written in a simplified form
A(Y,Z)X2+B(Y,Z)X+C(Y,Z)=0, | (5.14) |
where
{A(Y,Z)=βmY2+(1−2λ(2−cos(sφy)))Y+αm,B(Y,Z)=βmY2−2λ(2−cos(sφy))Y+αm,C(Y,Z)=βmY2+(1−2λcos(sφy))Y+αm. | (5.15) |
To establish the Von-Neumann criterion for stability, it suffices to show that
|X|=|eiψ|≤|B(Y,Z)||A(Y,Z)|≤1. | (5.16) |
Let us now examine the difference |B(Y,Z)|2−|A(Y,Z)|2. Careful computations yield that
|B(Y,Z)|2−|A(Y,Z)|2=(3μ−1)(μ−1)[2(σ2−σ2m)cos(2sφx)−8σ2(2−cos(sφy))cos(sφx)+2σ2+2σ2m+4σ2(2−cos(sφy))2]+8μσ(2−cos(sφx)−cos(sφy))−1. | (5.17) |
For 0≤μ<13, from (5.17), we get
|B(Y,Z)|2−|A(Y,Z)|2≤64(3μ−1)(μ−1)σ2+16σ−1. |
By denoting
σ0=√1+(3μ−1)(μ−1)−18(3μ−1)(μ−1), |
we obtain
σ≤σ0⟹|B(Y,Z)|2−|A(Y,Z)|2≤0. |
The left-hand term reads
ls2≤σ02α. | (5.18) |
Next, for 13<μ<1, from (5.17), we get
|B(Y,Z)|2−|A(Y,Z)|2≤−20(3μ−1)(μ−1)σ2+16σ−1. |
By denoting
σ1=4−√16−5(3μ−1)(μ−1)10(3μ−1)(μ−1), |
we obtain
σ≤σ1⟹|B(Y,Z)|2−|A(Y,Z)|2≤0, |
which, similar to the previous case, gives
ls2≤σ12α. | (5.19) |
Finally, for μ∈{13,1}, Eq (5.17) yields that
|B(Y,Z)|2−|A(Y,Z)|2≤16σ−1. |
This leads to
ls2≤132α. | (5.20) |
Thus, the stability follows as a result of (5.18), (5.19), and (5.20).
To show the convergence of the numerical scheme, here we apply the Fourier mode concept combined with the Vonn-Neuman criterion to establish a control on the time and space steps. Indeed, denote
ˆσ=min(σ0,σ1,116). |
The intersection of (5.18), (5.19), and (5.20) gives
ls2≤ˆσ2α. | (5.21) |
Taking l=sη+2 for some η>0 small enough (which is always possible), we get
s≤(ˆσ2α)1/η⟺l≤(ˆσ2α)(2+η)/η. |
Thus, convergence holds.
In this section, we will test the mathematical model above for salt rejection, and mainly for inorganic salts such as NaCl or Na2SO4. We take the parameter values h=10−3, D=10−9, ν0=10−3, and q=10−3. The numerical tests and simulations are conducted using MATLAB R2018a on a Dell Latitude 5530, Windows 10 64-bit Operating System, x64-based processor, 12th Gen Intel(R) Core(TM) i7-1255U 1.70 GHz, and 16.0 GB RAM.
In existing models based on the advection-diffusion equation, many constraints always applied, such as the dependence of the liquid (as well as the concentration of salts) flux speed on the perpendicular direction of the membrane (the x-axis here), and thus the concentration acceleration in the same direction. In this case, the model obtained (reviewed next in Appendix 7.2) may be converted via the well-known stretching or self-similar transformations into an ODE, which may be solved even for exact solutions.
The idea may be explained according to the speed of the liquid, the proportions of the different pollutants, such as salts, and due to many factors related to the filtration/osmosis system (the nature or the homogeneity of the membrane, and so on), which affects the result(s) of the osmosis. This task resembles the water in valleys and rivers, as it brings with it many types of pollutants and materials. Relative to the speed of feed water and according to the quantity, the percentages, and the quality of materials in the seawater, several sediments are formed on both sides of the membrane. Over time, these sediments cause the stream itself to narrow. Thus, we are faced with a phenomenon of interconnected parameters and dimensions, where the parameters, factors, and dimensions, that are initially neglected, excluded, and considered ineffective, come back to influence the model and the entire phenomenon.
Figure 4 illustrates the variation of the concentration C of the salt on the semi-permeable boundary with the distance x along the plate. Notice easily that this curve joins well with existing works, such as [18] or its variants [26,27,28].
We notice from Figure 4 that, when relaxing the parameter t, and thus getting a stationary solution C(t,x,y)=C(x,y), we come back to the classical equilibrium discussed in [18]. In this case, at the boundary of the membrane y=0, the phenomenon of the precipitation of the salts may persist, even being small, but as time passes, we notice effectively that this small amount of salts may have an impact on the whole process. Moreover, model (7.1) may lead to ambiguities relative to the boundary conditions assumed to be satisfied there, such as C(0,y)=C(x,∞)=C0, which cannot be fulfilled via solution (7.4). In such a case, simple computations permit us to get C(x,y)∼C0y, whenever x→0 or y→∞. The last approximations may contradict the physical reality of the whole process. These remarks mean that, for model (7.1) to approach reality, some artificial conditions should be added, which may again affect the compatibility with the real process. Moreover, this again raises the impact of neglecting some parameters or some parts in the real 2D time-dependent model, and thus motivates our approach in considering the whole 2D time-dependent model (3.1). Examinations of the complete model have led to Figures 5, 6, and 7.
In Figure 5, the time-wise behavior of the salt concentration is illustrated at some interior points (x,y), and compared to the concentration at the boundary point (0,0), where it is initially c0. We notice that the RO process works well at interior points (especially far from the boundary y=0), as the concentration of the salt decreases naturally until reaching a value less than half in a reduced time interval.
In Figures 6 and 7, the profile of the concentration of salts is investigated for a fixed time instants when the process is active. Notice from these figures that the concentration is somehow perturbed at the boundaries and takes a quasi-linear asymptotic profile at the interior of the channel. A logical explanation of this behavior is that, effectively, it is not completely true that the concentration of salts is omitted from the liquid, but, in contrast, the effect of the perpendicular direction to the flow of the osmosis is real, and it induces precipitation of the salts along the boundary of the membrane, which becomes considerable over time, and thus has to be taken into consideration in any model. Taking it into consideration in our opinion needs a whole revision of the system, such as the membranes, and their components, homogeneity, heterogeneity, construction materials, temperature, and also the permeability flow, which may not be constants, and may depend on the salts' precipitation along the process.
Besides, with the discovery and rapid development of modern technologies, such as nano-scales and nanomaterials, the variations in scales and levels that were not observable in classical technology are now well detected by modern instruments. This means that the ignorance of many parameters in the equations or models governing physical and/or chemical phenomena such as reverse osmosis no longer has any motivation or justification, as a maybe major part of these phenomena takes place in macro and sometimes nano levels. This motivates our involvement of both the variations according to the time and space variables in the model, especially the second-order space derivatives which explain, even if small, the acceleration of the process propagation in the space, and the time derivative which reflects the rate of the process in time.
Lemma 7.1. The matrices A and λR above have different eigenvalues.
Proof. For the sake of simplicity and clearness, we will develop the first two special cases of 2×2- and 3×3-matrices. In the first, we have to investigate the intersection of the spectra of the matrices
I−Am=(1+2λ−β0−α11+2λ)andR=(2−1−12). |
It is easy to see that the eigenvalues of λR are λ and 3λ. But, notice that both det((1−λ)I−Am)≠0, and det((1−3λ)I−Am)≠0. Hence, λ and 3λ are not eigenvalues of (I−Am). Therefore, Theorem 2.1 (see also [23]) implies that the linear Lyapunov-Sylvester operator LI−Am,−λR is invertible.
For the 3×3-case, we get
I−Am=(1+2λ−β00−α11+2λ−β10−α21+2λ)andR=(2−10−12−10−12). |
It is easy to see that λR has the only eignevalues 2λ, (2−√2)λ and (2+√2)λ. It is easy to check that det(A−xI)≠0, for x∈{2λ,(2−√2)λ,(2+√2)λ}. Thus, the lemma is proved.
In the existing works, as criticized in the introduction, approximate models have been investigated, characterized by two major drawbacks. First, such models ignore or neglect the role of some variables, based on assumptions on the minerals' concentration, such as, salts, which is assumed to be rapidly varying according to the y-direction, compared to the x-one, allowing one to neglect the diffusion in the x-direction. Second, such models assume that an equilibrium in time exists, and thus a stationary variant is investigated. In this case, we get the following governing equation to the reverse osmosis model:
y∂C∂x=α∂2C∂y2. | (7.1) |
However, this model may be solved for a general solution by utilizing the classical change of variables ζ=y3√x, which, by denoting C(x,y)=3√xφ(ζ), permits one to transform Eq (7.1) into
φ″+ζ23αφ′−ζ3αφ=0. | (7.2) |
Notice that this last equation has a solution φ0(ζ)=ζ. To find a general solution, denoting φ(ζ)=ζϕ(ζ), and substituting in (7.2), we get
ζϕ″+(2+ζ33α)ϕ′=0, | (7.3) |
which is a classical ordinary differential equation whose solution is
ϕ1(ζ)=13√9α∫∞3√9αζexp(−s3)s2ds. |
The general solutions of (7.2) reads
φ(ζ)=K0φ0(ζ)+K1ζϕ1(ζ), | (7.4) |
where K0 and K1 are constants.
The aim of the present paper is to develop a numerical method to solve a reverse osmosis model, where the governing (1+2)-dimensional diffusion-convection PDE due to the salt concentration in the osmosis membrane is converted into a Sylvester equation that is proved to be uniquely solvable, stable, consistent and convergent. The numerical scheme developed was validated with experimental data from the literature, and via some numerical simulations. The objective of the present paper is therefore twofold. As a result, many future directions may be raised.
Related to the Lyapunov-Sylvester algebraic system, an interesting direction that interested us is to link fractional derivatives to convection-diffusion evolutionary problems by considering fractional derivatives operators for the time and space variables in the PDE. With the LS equation, in algebra and especially when the operators LA,B are defined for A,B belongs to some ring R by
LA,B(X)=AX+XB,X∈R, |
we often call them generalized derivations on the ring R. These have been studied in the context of algebras in some normed spaces. Indeed, a generalized derivation of an algebra R is any map of the form LA,B where A and B are fixed elements in R. In the theory of operator algebras, they are considered as an important class of so-called elementary operators L(X)=n∑i=1AiXBi, where, as previously, the Ai's and the Bi's are elements of the algebra R. Remark that the operator LA,B satisfies
LA,B(XY)=LA,B(X)Y+XDB(Y), |
where DB(Y)=YB−BY is the so-called inner derivation. Remark that
DB(XY)=DB(X)Y+XDB(Y) |
which looks like the well-known product derivation rule on function spaces (fg)′=f′g+fg′. Hence, the nomination of derivation on algebraic structures. So, it is questionable that an integration operator IA,B could or could not be defined so that one has for any X and Y in R,
LA,B(X)=Y⟺IA,B(Y)=X. |
Taking the simple example, A=(0121) and B=(1102), the associated Lyapunov-Sylvester operator LA,B is invertible for the ring M2(R), but non-invertible on M2(Z/3Z). A second example, for A=(1221) and B=(1121), leads to an invertible operator on M2(R), but its inverse has no longer the same form. A general form that may be expected is
L−1(X)=m∑i=1AiXBi+n∑i=1CiXTBi. |
So, here also, what could be the minimum values of the parameters m and n given the operator L−1? Could we expect a signature-type (n,m) for these operators?
We now return to the physical phenomenon subject of the application, which deals with the RO model. In this context, water represents an essential factor for the existence of all creatures in this universe. Even wastewater or brackish water constitute suitable environments for the life of several types of organisms, such as bacteria, fishes, marine plants, and so on. This is in addition to its basic role in environmental balance, such as maintaining temperatures and climate.
Clean, fresh, and/or potable water is the most important type of water and liquids overall, due to its essential role in the continuation of the life of the most important type of beings in the universe, the human, and such a role is irreplaceable by other liquids.
With the increase in demand for potable water due to frequent use, accompanied by a shortage of natural sources such as rain, water napes, and many other reasons, humans have resorted to inventing several ways to benefit from other water sources, such as rivers and seas, thus confronting the problem of desalinating water and extracting impurities from it to make it suitable for drinking in particular.
The present paper investigates one of the methods applied to extract fresh water from seawater using the reverse osmosis process. A numerical method is developed to solve a two-dimensional time-dependent original mathematical model of a reverse osmosis process. The governing PDE is transformed to a Lyapunov-Sylvester algebraic system, (which has been solved in its original geometric space 3D-dimension Euclidean space), without transforming it into a 1-dimensional space as in the majority of existing works dealing with higher-dimensional PDEs. The system is investigated for solvability, stability, consistency, and convergence. Besides, experimental results have been provided based on simulation results, and eventually compared with existing studies. The findings showed that the RO may be suitable for the aim of fresh water extraction, whenever it is accompanied by many improvements, related to the space parameters for the used models, such as the dimensions, the time factor, the materials used for the physical RO system, the permeability of the membrane walls, and also the way of controlling the system during its progress.
On another side, RO, or more generally desalination tools, may also be linked to energy consumption, which in turn needs optimal models to reduce such consumption. The membrane module configuration, for example, could balance the flux and, hence, minimize energy consumption. New technologies using nanofiltration for pressure-driven membrane processes are linked to both the diffusion and the convection through the pores and/or charged membrane. To understand more and improve these facts, the scientific community needs to involve related parameters into the mathematical models such as the present convection-diffusion one. Optimality based on the membrane properties, such as porosity and geometry, is still limited in comparison to the use of substances' properties ([1,5,13,14,16,17,19].
S. Helali: Supervision, Conceptualization, Investigation, Writing–original draft, Writing–review and editing, Funding acquisition; A. Ben Mabrouk: Conceptualization, Methodology, Formal analysis, Investigation, Writing–original draft, Writing–review and editing, Visualization; M. Rashad: Conceptualization, Formal Analysis; N. Bel Haj Ali: Conceptualization, Formal analysis; M. A. Alsharif: Formal analysis; M. A. Alanazi: Writing–review and editing, E. M. Al-Ali: Methodology, Writing–review and editing; L. A. Alharbi: Writing–review and editing; M. S. Mustafa: Writing–review and editing. All authors have read and agreed to the published version of the manuscript.
The authors declare they have not used Artificial Intelligence (AI) tools in the creation of this article.
The authors would like to thank and extend their appreciation to the deanship of scientific research at the University of Tabuk, KSA, and the Deputyship for Research & Innovation, Ministry of Education, Saudi Arabia for funding this research work through the project number (0097-1443-S).
The author declares here that no conflicts of interest in this paper.
[1] |
H. Abdallah, M. S. Shalaby, M. A. Saad, A. M. Shaban, Supporting Polyvinylchloride Polymeric Blend Membrane with Coated Woven Fabric, J. Membr. Sci. Res., 4 (2018), 174–180. http://doi.org/10.22079/JMSR.2018.81167.1176 doi: 10.22079/JMSR.2018.81167.1176
![]() |
[2] |
B. Absar, O. Belhamiti, Modeling and computer simulation of a reverse osmosis desalination plant-case study of Bousfer plant-Algeria, Desalin. Water Treat., 51 (2013), 5942–5953. http://doi.org/10.1080/19443994.2013.770192 doi: 10.1080/19443994.2013.770192
![]() |
[3] |
B. Absar, S. E. M. L. Kadi, O. Belhamiti, Reverse osmosis modeling with the orthogonal collocation on finite element method, Desalin. Water Treat., 21 (2010), 23–32. https://doi.org/10.5004/DWT.2010.1162 doi: 10.5004/DWT.2010.1162
![]() |
[4] | H. Ali Merina, O. Belhamiti, Simulation Study of Nonlinear Reverse Osmosis Desalination System Using Third and Fourth Chebyshev Wavelet Methods, MATCH Commun. Math. Comput. Chem., 75 (2016), 629–652. |
[5] |
D. Ariono, M. Purwasasmit, I. G. Wenten, Brine Effluents: Characteristics, Environmental Impacts, and Their Handling, J. Eng. Technol. Sci., 48 (2016), 367–387. https://doi.org/10.5614/j.eng.technol.sci.2016.48.4.1 doi: 10.5614/j.eng.technol.sci.2016.48.4.1
![]() |
[6] |
O. Belhamiti, B. Absar, A Numerical Study of Fractional Order Reverse Osmosis Desalination Model using Legendre Wavelet Approximation, Iran. J. Math. Chem., 8 (2017), 345–364. http://doi.org/10.22052/ijmc.2017.86494.1289 doi: 10.22052/ijmc.2017.86494.1289
![]() |
[7] |
A. Ben Mabrouk, M. Ayadi, Lyapunov type operators for numerical solutions of PDEs, Appl. Math. Comput., 204 (2008), 395–407. http://doi.org/10.1016/j.amc.2008.06.061 doi: 10.1016/j.amc.2008.06.061
![]() |
[8] | A. Bezia, A. Ben Mabrouk, K. Betina, Lyapunov-sylvesters operators for (2+1)-Boussinesq equation, Electron. J. Differ. Equations, 268 (2016), 1–19. |
[9] |
A. Bezia, A. Ben Mabrouk, Finite difference method for (2+1)-Kuramoto-Sivashinsky equation, J. Part. Diff. Eq., 31 (2018), 193–213. http://doi.org/10.4208/jpde.v31.n3.1 doi: 10.4208/jpde.v31.n3.1
![]() |
[10] |
C. Chen, H. Qin, A Mathematical Modeling of the Reverse Osmosis Concentration Process of a Glucose Solution, Processes, 7 (2019), 271. http://doi.org/10.3390/pr7050271 doi: 10.3390/pr7050271
![]() |
[11] | R. Chteoui, A. Ben Mabrouk, A Generalized Lyapunov-Sylvester Computational Method for Numerical Solutions of NLS Equation with Singular Potential, Anal. Theory Appl., 33 (2017), 333–354. |
[12] |
R. Chteoui, A. F. Aljohani, A. Ben Mabrouk, Lyapunov–Sylvester computational method for numerical solutions of a mixed cubic-superlinear Schrödinger system, Eng. Comput., 38 (2022), 1081–1094. http://doi.org/10.1007/s00366-020-01264-9 doi: 10.1007/s00366-020-01264-9
![]() |
[13] | B. Djebedjian, H. Gad, I. Khaled, M. A. Rayan, Optimization of Reverse Osmosis Desalination System Using Genetic Algorithms Technique, Twelfth International Water Technology Conference, 2008, 1047–1067 |
[14] | A. Djordjevich, S. Savović, A. Janićijević, Explicit Finite-Difference Solution of Two-Dimensional Solute Transport with Periodic Flow in Homogenous Porous Media, J. Hydrol. Hydromech., 65 (2017), 426–432. |
[15] |
M. Elnour, N. Meskin, K. M. Khan, R. Jain, S. Zaidi, H. Siddiqui, Full-Scale Seawater Reverse Osmosis Desalination Plant Simulator, IFAC-PapersOnLine, 53 (2020), 16561–16568. http://doi.org/10.1016/j.ifacol.2020.12.780 doi: 10.1016/j.ifacol.2020.12.780
![]() |
[16] |
A. M. Farooque, S. Al-Jeshi, M. O. Saeed, A. Alreweli, Inefficacy of Osmotic Backwash Induced by Sodium Chloride Salt Solution in Controlling SWRO Membrane Fouling, Appl. Water Sci., 4 (2014), 407–424. http://doi.org/10.1007/s13201-014-0158-x doi: 10.1007/s13201-014-0158-x
![]() |
[17] |
K. P. Fattah, A. K. Al-Tamimi, W. Hamweyah, F. Iqbal, Evaluation of Sustainable Concrete Produced with Desalinated Reject Brine, Int. J. Sustain. Built Environ., 6 (2017), 183–190. http://doi.org/10.1016/j.ijsbe.2017.02.004 doi: 10.1016/j.ijsbe.2017.02.004
![]() |
[18] | G. R. Fulford, P. Broadbridge, Industrial Mathematics: Case Studies in the Diffusion of Heat and Matter, Cambridge: Cambridge University Press, 2002. |
[19] | R. M. Garud, S. V. Kore, V. S. Kore, G. S. Kulkarni, A Short Review on Process and Applications of Reverse Osmosis, Univ. J. Environ. Res. Technol., 1 (2011), 233–238. |
[20] |
Z. Hadadian, S. Zahmatkesh, M. Ansari, A. Haghighi, E. Moghimipour, Mathematical and experimental modeling of reverse osmosis (RO) process, Korean J. Chem. Eng., 38 (2021), 366–379. http://doi.org/10.1007/s11814-020-0697-9 doi: 10.1007/s11814-020-0697-9
![]() |
[21] | M. Hamou Maamar, O. Belhamiti, New (0,2) Jacobi multi-wavelets adaptive method for numerical simulation of gas separations using hollow fiber membranes, Commun. Appl. Nonlinear Anal., 22 (2015), 61–81. |
[22] | A. Jameson, Solution of equation AX+XB=C by inversion of an M×M or N×N matrix, SIAM J. Appl. Math., 16 (1968), 1020–1023. |
[23] |
L. Kohaupt, Solution of the matrix eigenvalue problem VA+AV∗=μV with applications to the study of free linear dynamical systems, J. Comput. Appl. Math., 213 (2008), 142–165. http://doi.org/10.1016/j.cam.2007.01.001 doi: 10.1016/j.cam.2007.01.001
![]() |
[24] | J. Kucera, Reverse Osmosis. Industrial Applications and Processes, Salem: Scrivener Publishing, 2010. |
[25] |
T. W. Lion, R. J. Allen, Osmosis in a minimal model system, J. Chem. Phys., 137 (2012), 244911. http://doi.org/10.1063/1.4770271 doi: 10.1063/1.4770271
![]() |
[26] | O. P. Maure, Aspek Matematis dan Aspek Pendidikan pada Suatu Model Pemurnian Air dalam Sistem Osmosis Terbalik, 2019. Available from: https://repository.usd.ac.id/35192. |
[27] |
O. P. Maure, S. Mungkasi, Application of Numerical Integration in Solving a Reverse Osmosis Model, AIP Conf. Proc., 2202 (2019), 020043. http://doi.org/10.1063/1.5141656 doi: 10.1063/1.5141656
![]() |
[28] | O. P. Maure, S. Mungkasi, On Modelling of Water Distillation in a Reverse Osmosis Process, Proceedings of the 2nd International Conference of Science and Technology for the Internet of Things, ICSTI 2019, 2019. http://doi.org/10.4108/eai.20-9-2019.2292098 |
[29] |
S. Noeiaghdam, D. Sidorov, A. Zamyshlyaeva, A. Tynda, A. Dreglea, A Valid Dynamical Control on the Reverse Osmosis System Using the CESTAC Method, Mathematics, 9 (2020), 48. http://doi.org/10.3390/math9010048 doi: 10.3390/math9010048
![]() |
[30] |
L. Sadek, T. H. Alaoui, Numerical methods for solving large-scale systems of differential equations, Ricerche. Mat., 72 (2023), 785–802. http://doi.org/10.1007/s11587-021-00585-1 doi: 10.1007/s11587-021-00585-1
![]() |
[31] |
E. M. Sadek, A. H. Bentbib, L. Sadek, H. T. Alaoui, Global extended Krylov subspace methods for large-scale differential Sylvester matrix equations, J. Appl. Math. Comput., 62 (2020), 157–177. http://doi.org/10.1007/s12190-019-01278-7 doi: 10.1007/s12190-019-01278-7
![]() |
[32] | L. Sadek, H. T. Alaoui, The extended block Arnoldi method for solving generalized differential Sylvester equations, J. Math. Model., 8 (2020), 189–206. |
[33] |
L. Sadek, H. T. Alaoui, Application of MGA and EGA algorithms on large-scale linear systems of ordinary differential equations, J. Comput. Sci., 62 (2022), 101719. http://doi.org/10.1016/j.jocs.2022.101719 doi: 10.1016/j.jocs.2022.101719
![]() |
[34] |
L. Sadek, E. M. Sadek, T. H. Alaoui, On Some Numerical Methods for Solving Large Differential Nonsymmetric Stein Matrix Equations, Math. Comput. Appl., 27 (2022), 69. http://doi.org/10.3390/mca27040069 doi: 10.3390/mca27040069
![]() |
[35] |
L. Sadek, H. T. Alaoui, The extended nonsymmetric block Lanczos methods for solving large-scale differential Lyapunov equations, Math. Model. Comput., 8 (2021), 526–536. http://doi.org/10.23939/mmc2021.03.526 doi: 10.23939/mmc2021.03.526
![]() |
[36] |
L. Sadek, A Cotangent Fractional Derivative with the Application, Fractal Fract., 7 (2023), 444. http://doi.org/10.3390/fractalfract7060444 doi: 10.3390/fractalfract7060444
![]() |
[37] |
L. Sadek, Stability of conformable linear infinite-dimensional systems, Int. J. Dyn. Control, 11 (2023), 1276–1284. http://doi.org/10.1007/s40435-022-01061-w doi: 10.1007/s40435-022-01061-w
![]() |
[38] |
L. Sadek, A. S. Bataineh, O. R. Isik, H. T. Alaoui, I. Hashim, A numerical approach based on Bernstein collocation method: Application to differential Lyapunov and Sylvester matrix equations, Math. Comput. Simul., 212 (2023), 475–488. http://doi.org/10.1016/j.matcom.2023.05.011 doi: 10.1016/j.matcom.2023.05.011
![]() |
[39] |
L. Sadek, Fractional BDF Methods for Solving Fractional Differential Matrix Equations, Int. J. Appl. Comput. Math, 8 (2022), 238. http://doi.org/10.1007/s40819-022-01455-6 doi: 10.1007/s40819-022-01455-6
![]() |
[40] |
L. Sadek, Controllability and observability for fractal linear dynamical systems, J. Vib. Control, 29 (2023), 4730–4740. http://doi.org/10.1177/10775463221123354 doi: 10.1177/10775463221123354
![]() |
[41] | R. F. Spellman, Reverse Osmosis. A Guide for the Nonengineering Professional, Boca Raton: CRC Press, 2015. http://doi.org/10.1201/b18732 |
[42] |
E. W. Tow, D. M. Warsinger, A. M. Trueworthy, J. Swaminathan, G. P. Thiel, S. M. Zubair, et al., Comparison of Fouling Propensity Between Reverse Osmosis, Forward Osmosis, and Membrane Distillation, J. Membrane Sci., 556 (2018), 352–364. http://doi.org/10.1016/j.memsci.2018.03.065 doi: 10.1016/j.memsci.2018.03.065
![]() |
[43] | M. E. Williams, A Review of Reverse Osmosis Theory, 2003. Available from: http://www.wescinc.com/RO$_-$Theory.pdf. |
[44] | S. J. Wimalawansa, Purification of Contaminated Water with Reverse Osmosis: Effective Solution of Providing Clean Water for Human Needs in Developing Countries, Int. J. Emerging Technol. Adv. Eng., 3 (2013), 75–89. |
[45] | BYJU'S, Reverse osmosis. Available from: //byjus.com/chemistry/reverse-osmosis. |