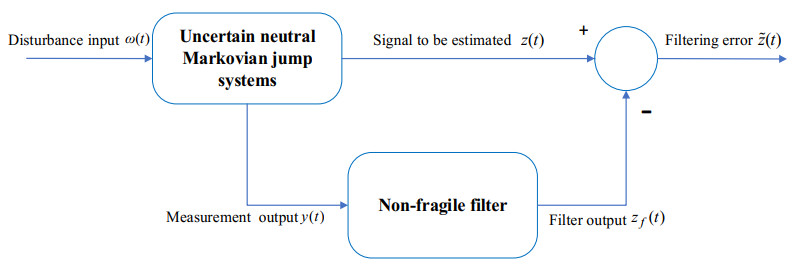
This paper deals with the problem of non-fragile H∞ filter design for a class of neutral Markovian jump systems with parameter uncertainties and time-varying delays. The parameter uncertainties are norm-bounded, and time-varying delays include state and neutral time-varying delays. First, by selecting the appropriate stochastic Lyapunov-Krasovskii functional and using the integral inequality technique, sufficient conditions are obtained to make the filtering error system not only stochastically stabilized, but also mode and delay dependent. Second, by the utilizing linear matrix inequality method, sufficient conditions are obtained for the filtering error system to be stochastically stable and to have a prescribed H∞ performance level γ. Based on this result, by processing the uncertainty terms, sufficient conditions for the existence of the filter are obtained, and mode-dependent filter parameters are given. Finally, by numerical simulation, the feasibility and validity of the theoretical results are verified.
Citation: Yakufu Kasimu, Gulijiamali Maimaitiaili. Non-fragile H∞ filter design for uncertain neutral Markovian jump systems with time-varying delays[J]. AIMS Mathematics, 2024, 9(6): 15559-15583. doi: 10.3934/math.2024752
[1] | Xipan Zhang, Changchun Shen, Dingju Xu . Reachable set estimation for neutral semi-Markovian jump systems with time-varying delay. AIMS Mathematics, 2024, 9(4): 8043-8062. doi: 10.3934/math.2024391 |
[2] | Weiwei Sun, Mengyang Qiu, Xinyu Lv . H∞ filter design for a class of delayed Hamiltonian systems with fading channel and sensor saturation. AIMS Mathematics, 2020, 5(4): 2909-2922. doi: 10.3934/math.2020188 |
[3] | Boonyachat Meesuptong, Peerapongpat Singkibud, Pantiwa Srisilp, Kanit Mukdasai . New delay-range-dependent exponential stability criterion and $ H_\infty $ performance for neutral-type nonlinear system with mixed time-varying delays. AIMS Mathematics, 2023, 8(1): 691-712. doi: 10.3934/math.2023033 |
[4] | Arthit Hongsri, Wajaree Weera, Thongchai Botmart, Prem Junsawang . Novel non-fragile extended dissipative synchronization of T-S fuzzy complex dynamical networks with interval hybrid coupling delays. AIMS Mathematics, 2023, 8(12): 28601-28627. doi: 10.3934/math.20231464 |
[5] | Wenlong Xue, Yufeng Tian, Zhenghong Jin . A novel nonzero functional method to extended dissipativity analysis for neural networks with Markovian jumps. AIMS Mathematics, 2024, 9(7): 19049-19067. doi: 10.3934/math.2024927 |
[6] | Yuxiao Zhao, Hong Lin, Xiaoyan Qiao . Persistence, extinction and practical exponential stability of impulsive stochastic competition models with varying delays. AIMS Mathematics, 2023, 8(10): 22643-22661. doi: 10.3934/math.20231152 |
[7] | Miao Zhang, Bole Li, Weiqiang Gong, Shuo Ma, Qiang Li . Matrix measure-based exponential stability and synchronization of Markovian jumping QVNNs with time-varying delays and delayed impulses. AIMS Mathematics, 2024, 9(12): 33930-33955. doi: 10.3934/math.20241618 |
[8] | Yuxin Lou, Mengzhuo Luo, Jun Cheng, Xin Wang, Kaibo Shi . Double-quantized-based $ H_{\infty} $ tracking control of T-S fuzzy semi-Markovian jump systems with adaptive event-triggered. AIMS Mathematics, 2023, 8(3): 6942-6969. doi: 10.3934/math.2023351 |
[9] | Yongxiang Zhu, Min Zhu . Well-posedness and order preservation for neutral type stochastic differential equations of infinite delay with jumps. AIMS Mathematics, 2024, 9(5): 11537-11559. doi: 10.3934/math.2024566 |
[10] | Baoyan Sun, Jun Hu, Yan Gao . Variance-constrained robust $ H_{\infty} $ state estimation for discrete time-varying uncertain neural networks with uniform quantization. AIMS Mathematics, 2022, 7(8): 14227-14248. doi: 10.3934/math.2022784 |
This paper deals with the problem of non-fragile H∞ filter design for a class of neutral Markovian jump systems with parameter uncertainties and time-varying delays. The parameter uncertainties are norm-bounded, and time-varying delays include state and neutral time-varying delays. First, by selecting the appropriate stochastic Lyapunov-Krasovskii functional and using the integral inequality technique, sufficient conditions are obtained to make the filtering error system not only stochastically stabilized, but also mode and delay dependent. Second, by the utilizing linear matrix inequality method, sufficient conditions are obtained for the filtering error system to be stochastically stable and to have a prescribed H∞ performance level γ. Based on this result, by processing the uncertainty terms, sufficient conditions for the existence of the filter are obtained, and mode-dependent filter parameters are given. Finally, by numerical simulation, the feasibility and validity of the theoretical results are verified.
In many practical systems, there are often sudden problems involving various noises, environmental disturbances, equipment failures, and maintenance. These factors can lead to sudden changes in system parameters and structure. Thus, for such complex problems, establishing an appropriate system model is essential. In order to solve such problems, Krasovskii and Lidskii first proposed Markovian jump systems [1]. Markovian jump systems as stochastic systems are also important mixing systems, and they are widely used in the fields of economics, biomedicine, manufacturing, power, aerospace, and networking [2,3,4,5]. Over the past decades, Markovian jump systems have been studied, focusing on the stability, control, and filtering of the system. In [6,7,8], the stability of neutral Markovian jump systems and stochastic singular Markovian jump systems were considered. In [9,10,11,12], problems of finite time H∞ control, robust and non-fragile H∞ control, and delay dependent control for Markovian jump systems with time-varying delays were studied. The problem of non-fragile H∞ filter design for a class of discrete singular Markovian jump systems with time-varying delays and measurement misspecification was considered based on extended passive theory in [13]. In [14], the problem of finite region asynchronous dissipative control was considered, with more attention paid to the transient behavior of a class of two-dimensional fuzzy Markovian jump systems. The problem of asynchronous deconvolution filter design for 2-D Markovian jump systems with random packet losses was addressed in [15].
Filtering is an important method for estimating system state information when the system is subject to disturbances. Filtering problems are fundamental in areas such as control and signal processing. Kalman and H∞ filtering are the more popular filtering methods. The Kalman filter is applicable when the disturbance in the system is Gaussian white noise or spectral density noise. The disadvantage of the Kalman filter is that if the understanding of the noise model or the measured noise is not accurate enough, these limit the scope of applications of the Kalman filtering technique. The H∞ filter applies to any signal where the measured noise is of bounded energy, and it does not have to require that the noise be Gaussian white noise. Therefore, H∞ filtering is widely used in many fields. The Riccati equation method and the linear matrix inequality (LMI) method are more popularly used in H∞ filter design. Among them, the Riccati equation method is widely used in systems with norm-bounded uncertainties, and the solution is relatively simple. However, the Riccati equation method uses an iterative approach and does not have a standardized solution, so it is relatively conservative [16]. The LMI method is more practical and less conservative than the Riccati method and is therefore widely used [17,18,19]. Through the study, it was found that either using classical optimal control to study system stability or using it to design controllers may lead to the emergence of fragile phenomena in the controllers, thereby leading to a decrease in the performance of the closed-loop system obtained by adding the controller, even making it difficult to maintain stability. Thus, the problem of non-fragile filtering has attracted the interest of scholars, and some research results have been obtained [20,21,22,23,24,25].
As is well known, time delays arise frequently in a variety of engineering systems, such as manufacturing systems, communication systems, networked control systems, chemical processing, and biological systems [13,26,27,32]. In fact, time delays are one of the most important reasons for system instability and poor performance. In recent decades, the study of stability and control of delayed systems has attracted the attention of many scholars. In [28], the stability analysis of time delay systems was investigated using single/multiple integral inequalities. The delay-dependent L2−L∞ filtering problem for stochastic systems with time delays was investigated in [29]. [30] discussed the delay-dependent H∞ filtering problem for a class of singular Markovian jump delay systems. Parameter uncertainties, like time delay, often lead to significant deterioration or even instability in the performance of the corresponding system. Therefore, it is necessary and reasonable to consider time delay and parameter uncertainties in the study of various control systems, including Markovian jump systems. Markovian jump systems with time delay and uncertainties have also been extensively studied [25,31,32].
Neutral delay as a special kind of time delay appears in the derivative of the system state. Since neutral systems can describe both the system state and the time delay of state differentiation, many control system models can be well modeled as neutral systems [33]. Thus, the neutral Markovian jump systems are also studied. For example, in [34], by utilizing the Lyapunov-Krasovskii generalization method, the problem of state estimation for uncertain neutral time-delay systems with Markovian jump parameters was studied. The robust H∞ filtering problem for uncertain Markovian jump-neutral distributed delay systems was studied in [35]. The problem of designing asynchronous H∞ controllers for neutral singular Markovian jump systems under dynamic event-triggered parties was studied in [36]. The existence of time delay affects the performance and stability of the dynamic system. Both state delay and neutral delay imply increased difficulty in modeling and controlling the system, and require more advanced control strategies and methods to deal with the time delay of the system. The mixed-delay-dependent L2−L∞ filter design for a class of neutral stochastic systems with time-varying delay was discussed in [37]. The problem of robust stability and H∞ filter design for neutral stochastic neural networks with parameter uncertainties and time delay was considered in [38]. In [39], the problem of robust H∞ filter design for a class of uncertain fuzzy-neutral stochastic systems with time-delay was investigated using the Takagi-Sugeno (T-S) fuzzy model. In recent years, there has been more research on filters for Markovian jump systems, but the problem of non-fragile H∞ filtering of Markovian jump systems with neutral delay has rarely been reported.
Based on the above discussions, this paper discusses the problem of non-fragile H∞ filter design for Markovian jump systems with parameter uncertainties and two kinds of time-varying delay. Mode-dependent non-fragile H∞ filters are obtained by utilizing delay and mode-dependent Lyapunov-Krasovskii functions and an integral inequality technique. The main contributions of this paper are as follows:
(1) The state delay and neutral delay are considered simultaneously for filtering design of uncertain Markovian jump systems.
(2) The designed filter is a non-fragile filter. Specifically, non-fragile H∞ filter is robust to parameter uncertainties and external disturbances.
(3) The non-fragile H∞ filtering conditions for uncertain Markovian jump systems with time-varying neutral delay and state delay are shown in terms of strict LMIs, which can be solved directly with the LMI toolbox and yield non-fragile H∞ filter parameters.
The remainder of this paper is organized as follows. The non-fragile H∞ filter design for uncertain neutral Markovian jump systems with time-varying delays problem formulation and preliminaries is formulated in Section 2. Section 3 presents stability analysis and the non-fragile H∞ filter design. Two numerical examples are provided in Section 4, and then we conclude this paper in Section 5.
Notation: (Ω,F,P) is a complete probability space, where Ω represents the sample space, F represents the σ -algebra of a subset of the sample space, and P represents the probability measure of F. L2[0,∞) is a square-integrable vector function in the [0,∞) space. ε{⋅} represents the mathematical expectation operator with respect to the given probability measure P. The symbol (∗) represents a term induced by symmetry in the linear matrix inequality (LMI). X>0(X⩾0) represents a positive definite (semi-positive) matrix, and X<0(X⩽0) represents a negative definite (semi-negative) matrix.
Given a complete probability space (Ω,F,P), we consider the following uncertain neutral Markovian jump systems with time-varying delays:
{˙x(t)=A(t,rt)x(t)+Ad(t,rt)x(t−d(t))+Ah(rt)˙x(t−h(t))+G1(rt)ω(t)y(t)=C(rt)x(t)+Cd(rt)x(t−d(t))+G2(rt)ω(t)z(t)=D(rt)x(t)x(t)=φ(t),t∈[−b,0] | (2.1) |
where x(t)∈Rn is the state vector, y(t)∈Rr is the measurement output vector, ω(t)∈Rp is the disturbance input vector, which belongs to L2[0,∞), and z(t)∈Rq is the signal vector to be estimated.
h(t) and d(t) are the time-varying delays which satisfy
0⩽h(t)⩽h,˙h(t)⩽ˉh<10⩽d(t)⩽d,˙d(t)⩽ˉd<1 | (2.2) |
φ(t) is a continuous real-valued initial function defined in the interval [−b,0], where b=max{d,h} is satisfied.
{rt} is a Markov process with right-continuous trajectories, taking values in a finite set rt=i∈M={1,2,...,N}. The transition rate matrix Π={λij} is given by
P{rt+ρ=j|rt=i}={λijρ+o(ρ),i≠j1+λijρ+o(ρ),i=j | (2.3) |
where ρ>0,limρ→0o(ρ)ρ=0, and λij⩾0 for i≠j is the transition rate from mode i at time t to mode j at time t+ρ, satisfying
λii=−N∑j=1,j≠iλij. | (2.4) |
For each rt∈M,
A(t,rt)=A(rt)+ΔA(t,rt)Ad(t,rt)=Ad(rt)+ΔAd(t,rt) | (2.5) |
where A(rt),Ad(rt),Ah(rt),C(rt),Cd(rt),D(rt),Dd(rt),G1(rt),G2(rt), and G3(rt) are known real constant matrices of appropriate dimensions, and where ΔA(t,rt) and ΔAd(t,rt) satisfy
ΔA(t,rt)=H1(rt)F(t,rt)K1(rt)ΔAd(t,rt)=H1(rt)F(t,rt)K2(rt) | (2.6) |
where F(t,rt),rt∈M are unknown matrices with Lebesgue measure satisfying
FT(t,rt)F(t,rt)⩽I,∀rt∈M, | (2.7) |
and H1(rt),K1(rt),andK2(rt),rt∈M, are known constant matrices with appropriate dimensions.
For convenience, for each rt=i∈M, A(t,rt) is replaced by Ai(t), G1(rt) is replaced by G1i, etc.
Now we consider a non-fragile filter for system (2.1) as follows:
{˙xf(t)=Af(t,rt)xf(t)+Bf(t,rt)y(t)zf(t)=Cf(rt)xf(t) | (2.8) |
where xf(t)∈Rn is the filter state vector; zf(t)∈Rq is the filter output vector; Af(t,rt),Bf(t,rt), Cf(t,rt) are the filter parameter matrices with appropriate dimension, for rt∈M
{Af(t,rt)=Af(rt)+ΔAf(t,rt)Bf(t,rt)=Bf(rt)+ΔBf(t,rt) | (2.9) |
where
{ΔAf(t,rt)=H2(rt)F(t,rt)K3(rt)ΔBf(t,rt)=H2(rt)F(t,rt)K4(rt) | (2.10) |
and H2(rt),K3(rt),andK4(rt),rt∈M, are known constant matrices with appropriate dimensions.
Let
˜x(t)=[xT(t),xTf(t)]T,˜z(t)=z(t)−zf(t) |
Then, we have the filtering error system
{˙˜x(t)=˜Ai˜x(t)+˜Adi˜x(t−d(t))+˜Ahi˙˜x(t−h(t))+˜Giω(t)˜z(t)=˜Ci˜x(t) | (2.11) |
where
˜Ai=ˉAi+ˉHiFi(t)ˉK1i,˜Adi=ˉAdi+ˉHiFi(t)ˉK2i |
ˉAi=[Ai0BfiCiAfi],ˉAdi=[Adi0BfiCdi0],˜Ahi=[Ahi000],˜Ci=[Di−Cfi], |
˜Gi=[G1iBfiG2i],ˉHi=[H1i00H2i],ˉK1i=[K1i0K4iCiK3i],ˉK2i=[K2i0K4iCi0]. |
The non-fragile H∞ filtering problem can be described as follows.
Problem description: Given the uncertain neutral Markovian jump system (2.1) with parameter uncertainties (2.6), find a non-fragile filter (2.8) such that the filtering error system (2.11) is stochastically stable and satisfies the following H∞ performance:
supω≠0‖˜z(t)‖2‖ω(t)‖2<γ |
where γ is a given positive scalar.
Figure 1 shows a flowchart describing non-fragile H∞ filtering of uncertain neutral Markovian jump systems with time-varying delays.
To support the research in this paper, the main definitions and lemmas used are presented in the following.
Definition 1. [7] The filtering error system (2.11) is said to be stochastically stable if for φ(t)∈Rn defined in the interval [−b,0] and r0∈M,
limt→∞ε{∫t0|˜x(α,φ,r0)|2dα}<∞ |
where ˜x(α,φ,r0) is the solution to the filtering error system (2.11) under the initial conditions.
Definition 2. [40] Under zero initial conditions, the filtering error system (2.11) satisfies the H∞ performance level γ>0, if for all nonzero ω(t)∈L2[0,∞), the following inequality holds:
limT→∞ε{∫T0|˜z(t)|2dt}⩽limT→∞γ2∫T0|ω(t)|2dt. |
Lemma 1. [41] For any matrices Q∈Rn×n and Z∈Rn×n satisfying [QZZTQ]⩾0 and a scalar d>0, there is satisfying 0⩽d(t)⩽d, such that the following integrals are well defined, then
−d∫tt−d(t)˙xT(s)Q˙x(s)ds⩽Ψ(t)ΞΨT(t) |
where
Ψ(t)=[xT(t)xT(t−d(t))xT(t−d)]T |
Ξ=[−QQ−ZZ−2Q+Z+ZTQ−Z−Q]. |
Lemma 2. (Bellman−Gronwall) Let k and f be continuous real-valued functions on [a,b] and the function g be integrable and nonnegative on [a,b].
If
k(t)⩽f(t)+∫tag(s)k(s)ds,a⩽t⩽b |
such that
k(t)⩽f(t)+∫tag(s)f(s)e∫tsg(τ)dτds,a⩽t⩽b |
if f(t) is a monotone non-decreasing function, then
k(t)⩽f(t)e∫tag(s)dsds,a⩽t⩽b. |
Lemma 3. (Schur Complement Lemma) If the matrices S11=ST11, S22=ST22, and S12 are symmetric matrices with appropriate dimensions, then the following LMIs are equivalent:
(1) S=[S11S12ST12S22]<0
(2) S11<0,S22−ST12S−111S12<0
(3) S22<0,S11−S12S−122ST12<0
Lemma 4. [11] Given appropriate dimensions, the matrices Π1i, Π2i, and Π3i, with Π1i=ΠT1i for all F(t) satisfying FT(t)F(t)⩽I, then
Π1i+Π2iF(t)Π3i+ΠT3iFT(t)ΠT2i<0 |
if and only if there exists a scalar a ε>0 such that
Π1i+εΠ2iΠT2i+ε−1ΠT3iΠ3i<0. |
In the following chapters, we base our analysis on the Lyapunov-Krasovskii functions to provide stability conditions for the filtering error system (2.11). Furthermore, we derive delay-dependent conditions for the existence of the non-fragile filter (2.8) and obtain sufficient conditions for the filtering error system (2.11) to be stochastically stabilized and achieve a certain H∞ performance level γ>0.
Theorem 1. Given the scalars γ>0,b>0,d>0,h>0,ˉd>0, and ˉh>0, the filtering error system (2.11) is stochastically stable if there exist matrices Pi>0,Q1i>0,Q2i>0,Q3>0,Q4>0,R1>0,R2>0, R3i>0, R4>0, and Zi such that the following matrix inequalities hold for all i∈M :
N∑j=1λijQ1j<R1 | (3.1.1) |
N∑j=1λijQ2j<R2 | (3.1.2) |
dN∑j=1λijR3j<R4 | (3.1.3) |
[R3iZi∗R3i]>0, | (3.1.4) |
Ωi=[Ωi11Ωi12Ωi13Ωi140∗Ωi22Ωi2300∗∗Ωi3300∗∗∗Ωi440∗∗∗∗Ωi55]+[˜ATi(t)˜ATdi(t)0˜AThi0]Λ[˜ATi(t)˜ATdi(t)0˜AThi0]T<0 | (3.1.5) |
where
Ωi11=N∑j=1λijPj+Pi˜Ai+˜ATiPi+Q1i+Q3+Q4+dR1−R3i;Ωi12=Pi˜Adi+R3i−Zi;Ωi13=Zi;Ωi14=Pi˜Ahi; |
Ωi22=−(1−ˉd)Q1i−2R3i+Zi+ZTi;Ωi23=R3i−Zi; |
Ωi33=−Q3−R3i;Ωi44=−(1−ˉh)Q2i;Ωi55=−Q4; |
Λ=Q2i+hR2+d2R3i+12d2R4. |
Proof. We will prove the stochastic stability of the filtering error system (2.11). First, we define a new process {(˜xt,rt),t⩾0} using {˜xt=x(t+θ),−2b⩽θ⩽0}, and then {(˜xt,rt),t⩾b} is a Markov process with initial state (ϕ(·),r0). Further, for all rt=i∈M, choose a stochastic Lyapunov-Krasovskii function candidate for system (2.11) as
V(˜xt,rt)=8∑i=1Vi(˜xt,rt) | (3.1.6) |
where
V1(˜xt,rt)=˜xT(t)P(rt)˜x(t) |
V2(˜xt,rt)=∫tt−d(t)˜xT(s)Q1(rt)˜x(s)ds; |
V3(˜xt,rt)=∫tt−h(t)˙˜xT(s)Q2(rt)˙˜x(s)ds; |
V4(˜xt,rt)=∫tt−d˜xT(s)Q3˜x(s)ds+∫tt−h˜xT(s)Q4˜x(s)ds; |
V5(˜xt,rt)=∫0−d∫tt+θ˜xT(s)R1˜x(s)dsdθ; |
V6(˜xt,rt)=∫0−h∫tt+θ˙˜xT(s)R2˙˜x(s)dsdθ; |
V7(˜xt,rt)=d∫0−d∫tt+α˙˜xT(s)R3(rt)˙˜x(s)dsdα; |
V8(˜xt,rt)=∫0−d∫0θ∫tt+α˙˜xT(s)R4˙˜x(s)dsdαdθ. |
Let L be the weak infinitesimal generator of the stochastic process {(˜xt,rt),t⩾b}. Based on (3.1.1)–(3.1.4), we obtain
LV1(˜xt,rt)=2˜xT(t)Pi˙˜x(t)+˜xT(t)(N∑j=1λijPj)˜x(t), | (3.1.7) |
LV2(˜xt,rt)⩽˜xT(t)Q1i˜x(t)−(1−ˉd)˜xT(t−d(t))Q1i˜x(t−d(t))+∫tt−d(t)˜xT(s)N∑j=1λijQ1j˜x(s)ds⩽˜xT(t)Q1i˜x(t)−(1−ˉd)˜xT(t−d(t))Q1i˜x(t−d(t))+∫tt−d(t)˜xT(s)R1˜x(s)ds | (3.1.8) |
LV3(˜xt,rt)⩽˙˜xT(t)Q2i˙˜x(t)−(1−ˉh)˙˜xT(t−h(t))Q2i˙˜x(t−h(t))+∫tt−h(t)˙˜xT(s)N∑j=1λijQ2j˙˜x(s)ds⩽˙˜xT(t)Q2i˙˜x(t)−(1−ˉh)˙˜xT(t−h(t))Q2i˙˜x(t−h(t))+∫tt−h(t)˙˜xT(s)R2˙˜x(s)ds | (3.1.9) |
LV4(˜xt,rt)=˜xT(t)(Q3+Q4)˜x(t)−˜xT(t−d)Q3˜x(t−d)−˜xT(t−h)Q4˜x(t−h) | (3.1.10) |
LV5(˜xt,rt)=d˜xT(t)R1˜x(t)−∫tt−d˜xT(s)R1˜x(s)ds⩽d˜xT(t)R1˜x(t)−∫tt−d(t)˜xT(s)R1˜x(s)ds | (3.1.11) |
LV6(˜xt,rt)=h˙˜xT(t)R2˙˜x(t)−∫tt−h˙˜xT(s)R2˙˜x(s)ds⩽h˙˜xT(t)R2˙˜x(t)−∫tt−h(x)˙˜xT(s)R2˙˜x(s)ds | (3.1.12) |
LV7(˜xt,rt)=d2˙˜xT(t)R3i˙˜x(t)−d∫tt−d˙˜xT(s)R3i˙˜x(s)ds+dN∑j=1λij∫0−d∫tt+α˙˜xT(s)R3j˙˜x(s)dsdα⩽d2˙˜xT(t)R3i˙˜x(t)−d∫tt−d(t)˙˜xT(s)R3i˙˜x(s)ds+∫0−d∫tt+α˙˜xT(s)R4˙˜x(s)dsdα; | (3.1.13) |
LV8(˜xt,rt)=12d2˙˜xT(t)R4˙˜x(t)−∫0−d∫tt+α˙˜xT(s)R4˙˜x(s)ds. | (3.1.14) |
According to Lemma 1, we have
−d∫tt−d(t)˙˜xT(s)R3i˙˜x(s)ds⩽ˉξT(t)Ξiˉξ(t) | (3.1.15) |
where
ˉξ(t)=[˜xT(t)˜xT(t−d(t))˜xT(t−d)˜xT(t−h(t))˜xT(t−h)ωT(t)]T |
Ξi=[−R3i(R3i−Zi)Zi000∗(−2R3i+Zi+ZiT)(R3i−Zi)000∗∗−R3i000∗∗∗000∗∗∗∗00∗∗∗∗∗0]. |
When ω(t)=0, we can obtain that
LV(˜xt,rt)⩽ξT(t)Ωiξ(t) | (3.1.16) |
where
ξ(t)=[˜xT(t)˜xT(t−d(t))˜xT(t−d)˙˜xT(t−h(t))˜xT(t−h)]T |
and we can find that (3.1.5) guarantees Ξi<0, which implies that there exists a scalar a>0 such that
LV(˜xt,rt)<−a|˜x(t)|2. | (3.1.17) |
for all ˜xt≠0. Then, for any t⩾b, by Dynkin's formula, we have
εV(˜xt,rt)−εV(˜xb,rb)⩽−aε∫tb|˜x(s)|2ds |
such that
ε∫tb|˜x(s)|2ds⩽a−1V(˜xb,rb). | (3.1.18) |
Based on the filtering error system (2.11), for all t>0 there exists scalars K1⩾0,K2⩾0,andK3⩾0 such that
|˜x(t)|=|˜x(0)+∫t0[˜Ai˜x(s)+˜Adi˜x(s−d(s))+˜Ahi˙˜x(s−h(s))]ds|⩽|˜x(0)|+K1∫t0[|˜x(s)|+|˜x(s−d(s))|+|˙˜x(s−h(s))|]ds⩽|˜x(0)|+K1∫t0[|˜x(s)|+K2|˜x(s−b)|+K3|˙˜x(s−b)|]ds |
where K1=maxi∈M{|˜Ai|,|˜Adi|,|˜Ahi|}. Then, for any 0⩽t⩽b, one obtains
|˜x(t)|⩽[K1(b+bK2+K3)+1]Sup−b⩽s⩽0|φ(s)|+K1∫t0|˜x(s)|ds. | (3.1.19) |
Utilizing Lemma 2 (Gronwall-Bellman Lemma), we obtain
|˜x(t)|⩽[K1(b+bK2+K3)+1]Sup−b⩽s⩽0|φ(s)|eK1b |
which implies
Sup0⩽t⩽b|˜x(t)|2⩽[K1(b+bK2+K3)+1]2[Sup−b⩽s⩽0|φ(s)|]2e2K1b. | (3.1.20) |
Using Eqs (3.1.6)–(3.1.20), we have
V(˜xb,rb)⩽K4[Sup−b⩽s⩽0|φ(s)|]2 |
where K4⩾0, and there exists a scalar α⩾0 such that
ε{∫t0|˜x(s)|2ds}⩽αε[Sup−b⩽s⩽0|φ(s)|]2. |
According to Definition 1, we obtained that the filtering error system (2.11) with ω(t)=0 is stochastically stabilized.
Remark 1. In the stability analysis, by using Lyapunov stability theory and integral inequality techniques, stability of uncertain neutral Markovian jump systems is guaranteed. It is worth noting that the proof of the conditions in the definition is obtained using Dynkin's formula and the Gronwall-Bellman Lemma. The proof process is similar to that of [12,41].
This paper investigates the problem of non-fragile H∞ filter design for neutral Markovian jump systems. Therefore, sufficient conditions are obtained for the filtering error system to have a H∞ performance level γ, and this condition is used to further find out the filtering parameters of the filter (2.8).
Theorem 2. The filtering error system (2.11) is stochastically stable and has an H∞ performance level γ if there exist matrices Pi>0,Q1i>0,Q2i>0,Q3>0,Q4>0,R1>0,R2>0, R3i>0,R4>0, and Zi such that the following matrix inequality holds for all i∈M :
˜Ωi=[Ωi11Ωi12Ωi13Ωi140Ωi16˜CTi∗Ωi22Ωi230000∗∗Ωi330000∗∗∗Ωi44000∗∗∗∗Ωi5500∗∗∗∗∗−γ2I0∗∗∗∗∗∗−I]+[˜ATi(t)˜ATdi(t)0˜AThi0˜GTi0]Λ[˜ATi(t)˜ATdi(t)0˜AThi0˜GTi0]T<0 | (3.2.1) |
where Ωi16=Pi˜Gi.
Proof. By applying the Schur complement lemma, we known that the matrix inequality (3.2.1) can ensure (3.1.5). Consequently, the filtering error system (2.11) with ω(t)=0 is stochastically stable by Theorem 1. When ω(t)≠0, under zero initial conditions the performance function J is as follows:
Define
\begin{ALIGN} J =& \int_0^T {({{\tilde z}^T}(} t)\tilde z(t) - {\gamma ^2}{\omega ^T}(t)\omega (t))dt \hfill \\ & = \int_0^T {({{\tilde z}^T}(} t)\tilde z(t) - {\gamma ^2}{\omega ^T}(t)\omega (t) + LV({{\tilde x}_t},{r_t}))dt - V({{\tilde x}_T},{r_T}) \hfill \\ & \leqslant \int_0^T {({{\tilde z}^T}(} t)\tilde z(t) - {\gamma ^2}{\omega ^T}(t)\omega (t) + LV({{\tilde x}_t},{r_t}))dt \hfill \\ \end{ALIGN} . |
If J satisfies condition J<0, then the filtering error system (2.11) is stochastically stable and meets the given H∞ performance level γ.
If the inequality (3.2.2) holds, then J<0.
˜zT(t)˜z(t)−γ2ωT(t)ω(t)+LV(˜xt,rt)⩽˜ξT(t)˜Ωi˜ξ(t)<0 | (3.2.2) |
where
˜ξ(t)=[ξT(t)ωT(t)]T. |
If ˜Ξi satisfies condition ˜Ξi<0, then the filtering error system (2.11) satisfies H∞ performance level γ, and system (2.1) is stochastically stable in the presence of a non-fragile H∞ filter (2.8).
According to Eq (3.2.2), we have
˜zT(t)˜z(t)−γ2ωT(t)ω(t)+LV(˜xt,rt)<0. |
Therefore, for any T>0, the following inequality is satisfied:
∫T0(˜zT(t)˜z(t)−γ2ωT(t)ω(t))dt⩽0. |
Letting T→∞, we have
limT→∞ε{∫T0|˜z(t)|2dt}⩽limT→∞γ2∫T0|ω(t)|2dt |
and then the filtering error system (2.11) can satisfy Definition 2.
Next, we begin to design a non-fragile H∞ filter for system (2.1).
Theorem 3. Given scalars γ>0,b>0,d>0,h>0,ˉd>0 and ˉh>0, suppose there exist matrices P1i>0,P3i>0,˜Q1i>0, ˜Q2i>0,˜Q3>0,˜Q4>0,˜R1>0,˜R2>0,˜R3i>0,˜R4>0,P2i,X1i,X2i,X3i,X4i X,Z1i,Z2i, Z3i,Z4i,˜Afi,˜Bfi, and ˜Cfi, where X is a non-singular matrix, such that the following linear matrix inequalities hold for all i∈M :
N∑j=1λij˜Q1j<˜R1, | (3.2.3) |
N∑j=1λij˜Q2j<˜R2, | (3.2.4) |
dN∑j=1λij˜R3j<˜R4 | (3.2.5) |
Pi=[P1iP2i∗P3i]>0, | (3.2.6) |
[˜R3i0Z1iZ2i∗εIZ3iZ4i∗∗˜R3i0∗∗∗εI]>0, | (3.2.7) |
Γi=[Γi1Γi2∗Γi3]<0, | (3.2.8) |
where
Γi1=[Γi111Γi112Γi113Γi114Γi115Γi116Γi117Γi118Γi119000Γi1113DTi∗Γi122Γi123Γi124Γi125Γi126Γi127Γi128Γi129000Γi1213−˜CTfi∗∗Γi133Γi134Γi135000Γi139000Γi13130∗∗∗Γi144Γi145000Γi149000Γi14130∗∗∗∗Γi155Γi156Γi157Γi158000000∗∗∗∗∗Γi166Γi167Γi168000000∗∗∗∗∗∗Γi1770000000∗∗∗∗∗∗∗Γi188000000∗∗∗∗∗∗∗∗Γi19900000∗∗∗∗∗∗∗∗∗Γi11010000∗∗∗∗∗∗∗∗∗∗−˜Q4000∗∗∗∗∗∗∗∗∗∗∗−2εI00∗∗∗∗∗∗∗∗∗∗∗∗−γ2I0∗∗∗∗∗∗∗∗∗∗∗∗∗−I], | (3.2.9) |
Γi3=−diag{εi1I,εi1I,ε−1i1I,ε−1i1I,εi2I,εi2I,ε−1i2I,ε−1i2I,εi3I,εi3I,ε−1i3I,ε−1i3I,}, |
Γi2=[Γi211Γi21209×609×6], | (3.2.10) |
where
Γi111=N∑j=1λijP1j+˜Q1i+˜Q3+˜Q4+d˜R1−˜R3i+X1iAi+˜BfiCi+ATiXT1i+CTi˜BTfi, |
Γi112=N∑j=1λijP2j+˜Afi+ATiXT2i+CTi˜BTfi,Γi113=P1i−X1i+ATiXT3i+CTi˜BTfi, |
Γi114=P2i−X+ATiXT4i+CTi˜BTfi,Γi115=X1iAdi+˜BfiCdi+˜R3i−Z1i, |
Γi116=−Z2i,Γi117=Z1i,Γi118=Z2i,Γi119=X1iAhi,Γi1113=X1iG1i+˜BfiG2i, |
Γi122=N∑j=1λijP3j+(4+d)εI+˜Afi+˜ATfi,Γi123=PT2i−X2i+˜ATfi, |
Γi124=P3i−X+˜ATfi,Γi125=X2iAdi+˜BfiCdi−Z3i,Γi126=εI−Z4i, |
Γi127=Z3i,Γi128=Z4i,Γi129=X2iAhi,Γi1213=X2iG1i+˜BfiG2i, |
Γi133=−XT3i−X3i+˜Q2i+h˜R2+d2˜R3i+12d2˜R4,Γi134=−XT4i−X, |
Γi135=X3iAdi+˜BfiCdi,Γi139=X3iAhi,Γi1313=X3iG1i+˜BfiG2i, |
Γi144=−XT−X+(1+h+32d2)εI,Γi145=X4iAdi+˜BfiCdi,Γi149=X4iAhi, |
Γi1413=X4iG1i+˜BfiG2i,Γi155=−(1−ˉd)˜Q1i−2˜R3i+Z1i+ZT1i,Γi156=Z2i+ZT3i, |
Γi157=˜R3i−Z1i,Γi158=−Z2i,Γi166=−(3−ˉd)εI+Z4i+ZT4i,Γi167=−Z3i, |
Γi168=εI−Z4i,Γi177=−˜Q3−˜R3i,Γi188=−3εI,Γi199=−(1−ˉh)˜Q2i,Γi1101=−(1−ˉh)εI, |
Γi211=[X1iH1iXH2iKT1iCTiKT4iKT1iCTiKT4iX2iH1iXH2i0KT3i0KT3i00000000000000KT2iCTdiKT4i00], |
Γi212=[000000000000X3iH1iXH2iX3iH1iXH2i00X4iH1iXH2iX4iH1iXH2i000000KT2iCTdiKT4i]. |
Then, there exists a mode-dependent H∞ filter (2.8) such that the filtering error system (2.11) is stochastically stable with a H∞ performance level γ. Moreover, the desired parameters of the mode-dependent filter are given by
Afi=X−1˜Afi,Bfi=X−1˜Bfi,Cfi=˜Cfi. | (3.2.11) |
Proof.
Select the following matrix
θi=[θi11θi12θi13θi14θi150θi17˜CTi∗θi22θi230θi250θi270∗∗θi33θi340000∗∗∗θi440000∗∗∗∗θi55000∗∗∗∗∗θi6600∗∗∗∗∗∗−γ2I0∗∗∗∗∗∗∗−I] | (3.2.12) |
where
θi11=N∑j=1λijPj+Q1i+Q3+Q4+dR1−R3i+WTi˜Ai+˜ATiWi, |
θi12=Pi−WTi+˜ATiNi,θi13=WTi˜Adi+R3i−Zi,θi14=Zi,θi15=WTi˜Ahi, |
θi17=WTi˜Gi,θi22=−Ni−NTi+Q2i+hR2+d2R3i+12d2R4,θi23=NTi˜Adi, |
θi25=NTi˜Ahi,θi27=NTi˜Gi,θi33=−(1−ˉd)Q1i−2R3i+Zi+ZTi, |
θi34=R3i−Zi,θi44=−Q3−R3i,θi55=−(1−ˉh)Q2i,θi66=−Q4. |
Pre- and post-multiplying θi by the matrix Φi and its transposition, respectively, gives
Φi=[I˜ATi0000000˜ATdiI00000000I00000˜AThi00I00000000I000˜GTi0000I00000000I] |
and for any i∈M, we can obtain
˜Ξi=ΦiθiΦTi. |
Thus, θi<0 implies ˜Ξi<0 such that
θi=Π1i+Π3iFi(t)Π2i+ΠT2iFTi(t)ΠT3i+Π5iFTi(t)Π4i+ΠT4iFi(t)ΠT5i+Π7iFi(t)Π6i+ΠT6iFTi(t)ΠT7i. |
where
Πi1=[ˉθi11ˉθi12ˉθi130θi150θi17˜CTi∗θi22ˉθi230θi250θi270∗∗θi3300000∗∗∗θi440000∗∗∗∗θi55000∗∗∗∗∗θi6600∗∗∗∗∗∗−γ2I0∗∗∗∗∗∗∗−I] |
ˉθi11=N∑j=1λijPj+Q1i+Q3+Q4+dR1−R3i+WTiˉAi+ˉATiWi,ˉθi12=Pi−WTi+ˉATiNi,ˉθi13=WTiˉAdi+R3i−Zi,θi23=NTiˉAdi,Πi2=[ˉK1i0ˉK2i00000],Πi3=[ˉHTiWi0000000]T,Πi4=[0ˉHTiNi000000],Πi5=[ˉK1i0000000]T, |
Πi6=[00ˉK2i00000],Πi7=[0ˉHTiNi000000]T. |
According to Lemma 4, for any ε1i>0,ε2i>0,ε3i>0,and˜θi<0, we have the following inequality:
˜θi=Π1i+ε−11iΠ3iΠT3i+ε1iΠT2iΠ2i+ε−12iΠ5iΠT5i+ε2iΠT4iΠ4i+ε−13iΠ7iΠT7i+ε3iΠT6iΠ6i<0. |
Choosing the matrices in ˜θi as
Pi=[P1iP2i∗P3i],WTi=[X1iXX2iX],NTi=[X3iXX4iX],Zi=[Z1iZ2iZ3iZ4i],Q1i=[˜Q1i00εI],Q2i=[˜Q2i00εI],Q3=[˜Q3002εI],Q4=[˜Q4002εI],R1=[˜R100εI],R2=[˜R200εI],R3i=[˜R3i00εI],R4=[˜R400εI]. |
and connecting those with (3.1.1)–(3.1.4) and (3.2.1), we obtain conditions (3.2.3)–(3.2.8) and filter parameters (3.2.11) in Theorem 3. This completes the proof.
Remark 2. In Theorem 3, using the linear matrix inequality method, non-fragile H∞ filtering conditions for uncertain neutral Markovian jump systems with time-varying state delay and neutral delay are given, where the non-fragile H∞ filtering conditions are mode- and delay-dependent strictly linear matrix inequalities to represent and obtain the mode-dependent filter parameters. These parameters can be solved directly with the LMI toolbox.
In this section, we use the LMI toolbox, solving for the values of the filter parameters. Using filter parameters and other known parameters, we can construct figures of system state, filter state, and filtering errors in order to verify the feasibility and validity of theoretical results.
Example 1. Consider the neutral Markovian jump system (2.1) with two modes and system parameters as follows:
A1=[−7.400−2.4],A2=[−5.90.31.6−10.7],Ad1=[−21.5400.55],Ad2=[−2.72.5−2.60.7],Ah1=[0.100−0.2],Ah2=[−0.20.11.2−0.1],G11=[0.340.14],G12=[0.5−0.3],D1=[0.2−0.1]D2=[0.3−0.2],C1=[0.10.3]C2=[40.2],Cd1=[0.2−0.3]Cd2=[0.1−0.2],H11=H12=[−0.010.0200.03],H21=H22=[0.010.01−0.020],K11=K12=[−0.030.020.010],G21=1.65G22=0.8,K21=K22=[−0.010.030.020.01],K31=K32=[0.010.02−0.030.01],K41=K42=[−0.030.02],Π=[−0.90.90.5−0.5],F1(t)=F2(t)=[cost00sint],ϵ11=ϵ12=ϵ13=0.9ϵ21=ϵ22=ϵ23=0.8,ϵ=1. |
Using the parameters above to solve the linear matrix inequalities (3.2.3)–(3.2.8) in Theorem 3, we obtain the H∞ performance level γ in Table 1.
ˉh=0.1,h=0.4 | ˉh=0.2,h=0.3 | ˉh=0.2,h=0.4 | ˉh=0.3,h=0.3 | |
[40] | ------ | 2.6408 | 2.7568 | 2.8694 |
Example1 | 0.9207 | 0.9213 | 0.9330 | 0.9334 |
In Table 1, it can be seen that there is a significant difference between the performance level obtained in this paper and the performance level in reference [40]. The H∞ performance level γ in this paper is smaller. This implies that the system proposed in this paper has stronger stability and better robustness. Furthermore, the performance of the non-fragile H∞ filter designed in this paper is better than the robust H∞ filter proposed in [40]. The filter designed in this paper includes uncertainty terms, therefore demonstrating a stronger resistance to uncertainties and parameter variations.
Letting d=0.2,h=0.4,ˉd=0.3,ˉh=0.2, and using Eq (3.2.11), we obtain the following mode-dependent filter parameters:
Af1=[−0.88090.02060.0221−1.0631],Bf1=[−0.0330−0.0154],Cf1=[−0.0618−0.0556],Af2=[−0.48770.02570.0254−0.4954],Bf2=[−0.0162−0.0101],Cf2=[−0.06520.0062]. |
When the transition rate matrix ∏ is known, the Markovian jump modes rt for the initial mode r0=1 is shown in Figure 2. Choosing the initial value x(t0)=[0.08−0.05]T, the time-varying delays are d(t)=max{0.3sin(t),0.2} and h(t)=max{0.2sin(t),0.4}, the disturbance input ω(t)=sin(t)e−0.06t, and Figures 3–5 show the system state x(t), filtering state xf(t), signal to be estimated z(t), filter output zf(t), and filtering error ˜z(t), respectively. From Figures 2–5, it can be seen that when the two subsystems are switched according to Markovian jump modes, the obtained filter tracks the real state smoothly, and can rapidly converge to zero over time. The filtering error system is guaranteed to be stochastically stable. Finally, it can be verified that our designed non-fragile H∞ filter is feasible.
Example 2. Consider the partial element equivalent circuit (PEEC) model [42] described by neutral Markovian jump system (2.1) with the following parameters:
A1=[−5000−8000−6],Ad1=[−0.60−0.5−0.3−0.90.10−0.2−0.8],Ah1=[0.20000.20000.2],G11=[0.05−0.020.07],G21=−0.3,C1=[0.40.50.8],Cd1=[0.40.10.2],D1=[0.20.050.1],H11=[0.10.2000.3−0.20.10.20.3],H21=[0.20.30.100.10.20.10.20.3],K11=[−0.700.10.4−0.3000.1−0.2],K21=[0.10.1000.20.10.200.1],K31=[−0.10.20.10.1−0.30.2−0.200.1],K41=[−0.10.20],ϵ11=ϵ12=ϵ13=0.9,A2=[−10000−12000−15],Ad2=[−0.75−0.20.3−0.1−0.600−0.2−0.7],Ah2=[−0.3000−0.3000−0.3],G12=[0.200.2],C2=[0.80.40.1],Cd2=[0.40.10.3],D2=[0.20.10.15],G22=−0.2,H12=[0.100.2−0.20.300.20.10.3],H22=[0.10.30.20.20.100.30.20.1],K12=[0.10−0.70−0.30.40.10−0.2],K22=[00.10.100.10.200.20.1],K32=[−0.10.10.20.2−0.30.10.10−0.2],K42=[−0.100.2],ϵ21=ϵ22=ϵ23=0.9,A3=[−6000−4000−8],Ad3=[−0.9−0.30−0.05−0.65−0.15−0.20−1],Ah3=[0.20000.20000.2],G13=[0.10.10.2],G23=−0.1, |
C3=[0.80.60.5],Cd3=[0.20.20.3],D3=[0.20.10.25],H13=[0.1−0.20−0.30.100.20.30.1],H23=[0.10−0.20.200.10.10.20.3],K13=[0.20−0.60−0.40.30.10.1−0.2],K23=[0.20.100.10.10.20−0.10.2],K33=[−0.200.10.100.20.1−0.1−0.2],K43=[−0.20.10.1],ϵ31=ϵ32=ϵ33=0.9,ϵ=0.094F1(t)=F2(t)=F3(t)=cost,Π=[−0.90.40.50.3−0.50.20.40.4−0.8]. |
Let d=0.25,h=0.2,ˉd=0.35,ˉh=0.25, and using the parameters above to solve the linear matrix inequalities (3.2.3)–(3.2.8) in Theorem 3, we obtain the H∞ performance level γmin=0.9595 and mode-dependent filter parameters as follows:
Af1=[−1.4480−0.0417−0.2536−0.0538−1.2574−0.1550−0.2509−0.1464−1.4312],Bf1=[−0.2019−0.0397−0.2269],Cf1=[0.00920.00220.0104],Af2=[−1.4099−0.0099−0.2306−0.0181−1.2337−0.1888−0.2149−0.1758−1.4363],Bf2=[−0.3306−0.0971−0.0911],Cf2=[0.00450.0064−0.0118],Af3=[−1.1677−0.00780.1115−0.0079−1.1451−0.11490.1063−0.1062−1.4207],Bf3=[−0.4528−0.3949−0.2829],Cf3=[0.0012−0.0103−0.0167]. |
The Markovian jump modes rt for the initial mode r0=1 is shown in Figure 6. Choosing the initial value x(t0)=[0.020.01−0.01]T, the time-varying delays are d(t)=max{0.35sin(t),0.25} and h(t)=max{0.25sin(t),0.2}, the disturbance input ω(t)=sin(t)e−0.05t, Figure 7 shows the PEEC model system state x(t), Figure 8 shows the state estimation xf(t), and Figure 9 shows the filtering error.
The problem of non-fragile H∞ filtering for uncertain neutral Markovian jump time-delay systems with known transition jump rates is investigated in this paper. Using the Lyapunov-Krasovskii function and integral inequality technique, a novel delay and mode dependent stability criterion was derived. Using the linear matrix inequality method, a sufficient condition for the existence of the filter is given, and a mode dependent non-fragile H∞ filter satisfying the H∞ performance level γ was designed. Two numerical examples have been provided to illustrate the effectiveness and usefulness of the results. In the future, the non-fragile H∞ filtering and control problems for uncertain neutral type singular Markovian jump systems with time-varying delays will be considered.
All authors contributed equally to this work. All authors read and approved the final manuscript.
The authors declare they have not used Artificial Intelligence (AI) tools in the creation of this article.
The work presented in this paper is supported by the NSFC under the grants 61462087 and 61751316 and the Special Education Project of Xinjiang under the grant 2022D03029.
The authors declare that there is no conflict of interest regarding the publication of this paper.
[1] | N. N. Krasovskii, Analytical design of controllers in systems with random attributes, Automat. Rem. Contr., 22 (1961), 1021–1025. |
[2] |
A. Friedman, Stochastic differential equations and applications, Stochastic differential equations, Berlin, Heidelberg: Springer Berlin Heidelberg, 77 (1975), 75–148. https://doi.org/10.1007/978-3-642-11079-5_2 doi: 10.1007/978-3-642-11079-5_2
![]() |
[3] | E. K. Boukas, Stochastic switching systems: Analysis and design, Springer Science & Business Media, 2007.https://doi.org/10.1007/0-8176-4452-0 |
[4] | O. L. V. Costa, M. D. Fragoso, R. P. Marques, Discrete-time Markov jump linear systems, Springer Science & Business Media, 2005.https://doi.org/10.1007/b138575 |
[5] |
O. L. V. Costa, M. V. Araujo, A generalized multi-period mean-variance portfolio optimization with Markov switching parameters, Automatica, 44 (2008), 2487–2497. https://doi.org/10.1016/j.automatica.2008.02.014 doi: 10.1016/j.automatica.2008.02.014
![]() |
[6] |
G. Zhuang, Q. Ma, B. Zhang, S. Y. Xu, J. W. Xia, Admissibility and stabilization of stochastic singular Markovian jump systems with time delays, Syst. Control Lett., 114 (2018), 1–10. https://doi.org/10.1016/j.sysconle.2018.02.004 doi: 10.1016/j.sysconle.2018.02.004
![]() |
[7] |
L. Xiong, J. Tian, X. Liu, Stability analysis for neutral Markovian jump systems with partially unknown transition probabilities, J. Franklin I., 349 (2012), 2193–2214. https://doi.org/10.1016/j.jfranklin.2012.04.003 doi: 10.1016/j.jfranklin.2012.04.003
![]() |
[8] |
X. H. Liu, H. S. Xi, On delay-range-dependent stochastic stability conditions of uncertain neutral delay Markovian jump systems, J. Appl. Math., 2013 (2013). https://doi.org/10.1155/2013/101485 doi: 10.1155/2013/101485
![]() |
[9] |
Y. Zhang, Y. Shi, P. Shi, Robust and non-fragile finite-time control for uncertain Markovian jump nonlinear systems, Appl. Math. Comput., 279 (2016), 125–138. https://doi.org/10.1016/j.amc.2016.01.012 doi: 10.1016/j.amc.2016.01.012
![]() |
[10] |
J. Cheng, H. Zhu, S. Zhong, Y. Zeng, X. C. Dong, Finite-time control for a class of Markovian jump systems with mode-dependent time-varying delays via new Lyapunov functionals, ISA T., 52 (2013), 768–774. https://doi.org/10.1016/j.isatra.2013.07.015 doi: 10.1016/j.isatra.2013.07.015
![]() |
[11] |
G. M. Zhuang, S. Y. Xu, J. W. Xia, Q. Ma, Z. Q. Zhang, Non-fragile delay feedback control for neutral stochastic Markovian jump systems with time-varying delays, Appl. Math. Comput., 355 (2019), 21–32. https://doi.org/10.1016/j.amc.2019.02.057 doi: 10.1016/j.amc.2019.02.057
![]() |
[12] |
S. Xu, J. Lam, X. Mao, Delay-dependent control and filtering for uncertain Markovian jump systems with time-varying delays, IEEE T. Circuits-I, 54 (2007), 2070–2077. https://doi.org/10.1109/TCSI.2007.904640 doi: 10.1109/TCSI.2007.904640
![]() |
[13] |
M. Sathishkumar, R. Sakthivel, C. Wang, B. Kaviarasan, S. M. Anthoni, Non-fragile filtering for singular Markovian jump systems with missing measurements, Signal Proces., 142 (2018), 125–136. https://doi.org/10.1016/j.sigpro.2017.07.012 doi: 10.1016/j.sigpro.2017.07.012
![]() |
[14] |
P. Cheng, S. P. He, W. Xie, W. D. Zhang, Finite-region dissipative control for 2-D fuzzy jump systems under hidden mode detection, IEEE T. Syst. Man Cy. S., 2023. https://doi.org/10.1109/TSMC.2023.3278746 doi: 10.1109/TSMC.2023.3278746
![]() |
[15] |
P. Cheng, H. Chen, S. He, W. Zhang, Asynchronous deconvolution filtering for 2-D Markov jump systems with packet loss compensation, IEEE T. Autom. Sci. Eng., 2023. https://doi.org/10.1109/TASE.2023.3292891 doi: 10.1109/TASE.2023.3292891
![]() |
[16] | H. Beikzadeh, H. D. Taghirad, Robust H∞ filtering for nonlinear uncertain systems using state-dependent Riccati equation technique, Proceedings of the 48h IEEE Conference on Decision and Control (CDC) held jointly with 2009 28th Chinese Control Conference, IEEE, 2009, 4438–4445.https://doi.org/10.1109/CDC.2009.5399746 |
[17] |
M. Abbaszadeh, H. J. Marquez, Dynamical robust H∞ filtering for nonlinear uncertain systems: An LMI approach, J. Franklin I., 347 (2010), 1227–1241. https://doi.org/10.1016/j.jfranklin.2010.05.016 doi: 10.1016/j.jfranklin.2010.05.016
![]() |
[18] |
H. D. Tuan, P. Apkarian, T. Q. Nguyen, Robust and reduced-order filtering: New LMI-based characterizations and methods, IEEE T. Signal Proces., 49 (2001), 2975–2984. https://doi.org/10.1109/78.969506 doi: 10.1109/78.969506
![]() |
[19] |
D. F. Coutinho, C. E. De Souza, K. A. Barbosa, A. Trofino, Robust linear H∞ filter design for a class of Encertain nonlinear systems: An LMI approach, SIAM J. Control Optim., 48 (2009), 1452–1472. https://doi.org/10.1137/060669504 doi: 10.1137/060669504
![]() |
[20] | L. Liang, Non-fragile H∞ filtering for fuzzy discrete-time systems with Markovian jump and data Loss, 2021 IEEE 10th Data Driven Control and Learning Systems Conference (DDCLS), IEEE, 2021, 1183–1188.https://doi.org/10.1109/DDCLS52934.2021.9455549 |
[21] |
W. Qi, Y. Zhou, L. Zhang, J. Cao, J. Cheng, Non-fragile H∞ SMC for Markovian jump systems in a finite-time, J. Franklin I., 358 (2021), 4721–4740. https://doi.org/10.1016/j.jfranklin.2021.04.010 doi: 10.1016/j.jfranklin.2021.04.010
![]() |
[22] | S. Yan, M. Shen, L. W. Li, B. C. Zheng, Non-fragile H∞ filtering for Markov jump systems with incomplete transition probabilities and intermittent measurements, 2018 Chinese Control And Decision Conference (CCDC), IEEE, 2018, 2133–2138.https://doi.org/10.1109/CCDC.2018.8407479 |
[23] | H. Zhang, S. Yan, M. Shen, Non-fragile H∞ filtering for discrete stochastic Markov jump systems with intermittent measurements, 2017 Eighth International Conference on Intelligent Control and Information Processing (ICICIP), IEEE, (2017), 135–140.https://doi.org/10.1109/ICICIP.2017.8113930 |
[24] |
M. Shen, J. H. Park, S. Fei, Event-triggered nonfragile H∞ filtering of Markov jump systems with imperfect transmissions, Signal Proces., 149 (2018), 204–213. https://doi.org/10.1016/j.sigpro.2018.03.015 doi: 10.1016/j.sigpro.2018.03.015
![]() |
[25] |
G. Zhuang, Y. Wei, Non-fragile H∞ filter design for uncertain stochastic nonlinear time-delay Markovian jump systems, Circ. Syst. Signal Pr., 33 (2014), 3389–3419. https://doi.org/10.1007/s00034-014-9809-2 doi: 10.1007/s00034-014-9809-2
![]() |
[26] |
Z. X. Li, H. Y. Su, Y. Gu, Z. G. Wu, H∞ filtering for discrete-time singular networked systems with communication delays and data missing, Int. J. Syst. Sci., 44 (2013), 604–614. https://doi.org/10.1080/00207721.2011.617892 doi: 10.1080/00207721.2011.617892
![]() |
[27] |
Z. Wu, H. Su, J. Chu, Delay-dependent H∞ filtering for singular Markovian jump time-delay systems, Signal Proces., 90 (2010), 1815–1824. https://doi.org/10.1016/j.sigpro.2009.11.029 doi: 10.1016/j.sigpro.2009.11.029
![]() |
[28] |
J. Chen, S. Xu, B. Zhang, Single/multiple integral inequalities with applications to stability analysis of time-delay systems, IEEE T. Autom. Contr., 62 (2016), 3488–3493. https://doi.org/10.1109/TAC.2016.2617739 doi: 10.1109/TAC.2016.2617739
![]() |
[29] |
Y. Chen, A. Xue, S. Zhou, New delay-dependent L2−L∞ filter design for stochastic time-delay systems, Signal Process., 89 (2009), 974–980. https://doi.org/10.1016/j.sigpro.2008.11.015 doi: 10.1016/j.sigpro.2008.11.015
![]() |
[30] |
G. Zhuang, J. Lu, M. Zhang, Robust H∞ filter design for uncertain stochastic Markovian jump Hopfield neural networks with mode-dependent time-varying delays, Neurocomputing, 127 (2014), 181–189. https://doi.org/10.1016/j.neucom.2013.08.016 doi: 10.1016/j.neucom.2013.08.016
![]() |
[31] |
G. Liu, S. Xu, J. H. Park, G. M. Zhuang, Reliable exponential filtering for singular Markovian jump systems with time-varying delays and sensor failures, Int. J. Robust Nonlin., 28 (2018), 4230–4245. https://doi.org/10.1002/rnc.4230 doi: 10.1002/rnc.4230
![]() |
[32] | W. Wang, S. Kong, G. Cui, Robust H∞ filtering for discrete-time Markovian jump systems with time-varying delay and parametric uncertainties, 2020 39th Chinese Control Conference (CCC). IEEE, 2020,932–937.https://doi.org/10.23919/CCC50068.2020.9188423 |
[33] |
R. Rabah, G. M. Sklyar, A. V. Rezounenko, On strong regular stabilizability for linear neutral type systems, J. Differ. Equations, 245 (2008), 569–593. https://doi.org/10.1016/j.jde.2008.02.041 doi: 10.1016/j.jde.2008.02.041
![]() |
[34] |
W. Chen, Q. Ma, B. Zhang, State estimation of neutral Markovian jump systems: A relaxed L-K functional approach, J. Franklin I., 355 (2018), 3659–3676. https://doi.org/10.1016/j.jfranklin.2018.01.041 doi: 10.1016/j.jfranklin.2018.01.041
![]() |
[35] |
Y. Li, J. Liu, Robust filtering for Markovian jump neutral systems with distributed delays, Syst. Sci. Control Eng., 4 (2016), 295–306. https://doi.org/10.1080/21642583.2016.1238326 doi: 10.1080/21642583.2016.1238326
![]() |
[36] |
H. Wang, Y. Wang, G. Zhuang, Asynchronous H∞ controller design for neutral singular Markov jump systems under dynamic event-triggered schemes, J. Franklin I., 358 (2021), 494–515. https://doi.org/10.1016/j.jfranklin.2020.10.034 doi: 10.1016/j.jfranklin.2020.10.034
![]() |
[37] |
Y. Yu, X. Tang, T. Li, S. M. Fei, Mixed-delay-dependent L2−L∞ filtering for neutral stochastic systems with time-varying delays, Int. J. Control Autom., 17 (2019), 2862–2870. https://doi.org/10.1007/s12555-019-0160-z doi: 10.1007/s12555-019-0160-z
![]() |
[38] |
M. Hua, H. Tan, J. Fei, J. J. Ni, Robust stability and H∞ filter design for neutral stochastic neural networks with parameter uncertainties and time-varying delay, Int. J. Mach. Learn. Cyb., 8 (2017), 511–524. https://doi.org/10.1007/s13042-015-0342-9 doi: 10.1007/s13042-015-0342-9
![]() |
[39] |
Y. Li, F. Deng, F. Xie, Robust delay-dependent H∞ filtering for uncertain Takagi-Sugeno fuzzy neutral stochastic time-delay systems, J. Franklin I., 356 (2019), 11561–11580. https://doi.org/10.1016/j.jfranklin.2019.02.043 doi: 10.1016/j.jfranklin.2019.02.043
![]() |
[40] |
G. Zhao, G. Zhuang, J. W. Xia, W. Sun, J. S. Zhao, M. S. Zhang, Mode-dependent H∞ filtering for time-varying delays neutral jump systems based on FWM technique, Int. J. Control Autom., 19 (2021), 2092–2104. https://doi.org/10.1007/s12555-020-0362-4 doi: 10.1007/s12555-020-0362-4
![]() |
[41] |
G. Zhuang, S. Xu, B. Zhang, H. L. Xu, Y. M. Chu, Robust H∞ deconvolution filtering for uncertain singular Markovian jump systems with time‐varying delays, Int. J. Robust Nonlin., 26 (2016), 2564–2585. https://doi.org/10.1002/rnc.3461 doi: 10.1002/rnc.3461
![]() |
[42] | D. Yue, Q. L. Han, A delay-dependent stability criterion of neutral systems and its application to a partial element equivalent circuit model, Proceedings of the 2004 American control conference, IEEE, 6 (2004), 5438–5442. |
1. | Yakufu Kasimu, Gulijiamali Maimaitiaili, Non-Fragile H∞ Deconvolution Filter Design for Uncertain Two-Dimensional Markovian Jump Systems With State-Varying Delays, 2025, 13, 2169-3536, 18863, 10.1109/ACCESS.2025.3533025 |
ˉh=0.1,h=0.4 | ˉh=0.2,h=0.3 | ˉh=0.2,h=0.4 | ˉh=0.3,h=0.3 | |
[40] | ------ | 2.6408 | 2.7568 | 2.8694 |
Example1 | 0.9207 | 0.9213 | 0.9330 | 0.9334 |
ˉh=0.1,h=0.4 | ˉh=0.2,h=0.3 | ˉh=0.2,h=0.4 | ˉh=0.3,h=0.3 | |
[40] | ------ | 2.6408 | 2.7568 | 2.8694 |
Example1 | 0.9207 | 0.9213 | 0.9330 | 0.9334 |