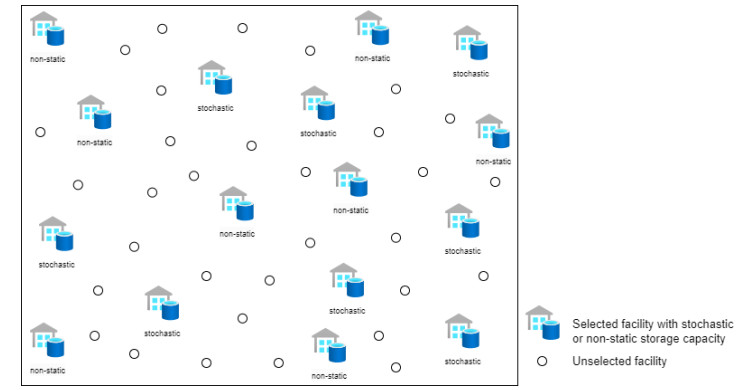
In this paper, the asymptotic behavior of solutions to a fractional stochastic nonlocal reaction-diffusion equation with polynomial drift terms of arbitrary order in an unbounded domain was analysed. First, the stochastic equation was transformed into a random one by using a stationary change of variable. Then, we proved the existence and uniqueness of solutions for the random problem based on pathwise uniform estimates as well as the energy method. Finally, the existence of a unique pullback attractor for the random dynamical system generated by the transformed equation is shown.
Citation: Ruonan Liu, Tomás Caraballo. Random dynamics for a stochastic nonlocal reaction-diffusion equation with an energy functional[J]. AIMS Mathematics, 2024, 9(4): 8020-8042. doi: 10.3934/math.2024390
[1] | Yuyang Zhao, Yang Liu . Output controllability and observability of mix-valued logic control networks. Mathematical Modelling and Control, 2021, 1(3): 145-156. doi: 10.3934/mmc.2021013 |
[2] | Hongli Lyu, Yanan Lyu, Yongchao Gao, Heng Qian, Shan Du . MIMO fuzzy adaptive control systems based on fuzzy semi-tensor product. Mathematical Modelling and Control, 2023, 3(4): 316-330. doi: 10.3934/mmc.2023026 |
[3] | Yunsi Yang, Jun-e Feng, Lei Jia . Recent advances of finite-field networks. Mathematical Modelling and Control, 2023, 3(3): 244-255. doi: 10.3934/mmc.2023021 |
[4] | Lei Wang, Xinyun Liu, Ting Li, Jiandong Zhu . Skew-symmetric games and symmetric-based decomposition of finite games. Mathematical Modelling and Control, 2022, 2(4): 257-267. doi: 10.3934/mmc.2022024 |
[5] | Naiwen Wang . Solvability of the Sylvester equation AX−XB=C under left semi-tensor product. Mathematical Modelling and Control, 2022, 2(2): 81-89. doi: 10.3934/mmc.2022010 |
[6] | Lusong Ding, Weiwei Sun . Neuro-adaptive finite-time control of fractional-order nonlinear systems with multiple objective constraints. Mathematical Modelling and Control, 2023, 3(4): 355-369. doi: 10.3934/mmc.2023029 |
[7] | Daizhan Cheng, Ying Li, Jun-e Feng, Jianli Zhao . On numerical/non-numerical algebra: Semi-tensor product method. Mathematical Modelling and Control, 2021, 1(1): 1-11. doi: 10.3934/mmc.2021001 |
[8] | Daizhan Cheng, Zhengping Ji, Jun-e Feng, Shihua Fu, Jianli Zhao . Perfect hypercomplex algebras: Semi-tensor product approach. Mathematical Modelling and Control, 2021, 1(4): 177-187. doi: 10.3934/mmc.2021017 |
[9] | Jianhua Sun, Ying Li, Mingcui Zhang, Zhihong Liu, Anli Wei . A new method based on semi-tensor product of matrices for solving reduced biquaternion matrix equation l∑p=1ApXBp=C and its application in color image restoration. Mathematical Modelling and Control, 2023, 3(3): 218-232. doi: 10.3934/mmc.2023019 |
[10] | Naveen Kumar, Km Shelly Chaudhary . Position tracking control of nonholonomic mobile robots via H∞-based adaptive fractional-order sliding mode controller. Mathematical Modelling and Control, 2025, 5(1): 121-130. doi: 10.3934/mmc.2025009 |
In this paper, the asymptotic behavior of solutions to a fractional stochastic nonlocal reaction-diffusion equation with polynomial drift terms of arbitrary order in an unbounded domain was analysed. First, the stochastic equation was transformed into a random one by using a stationary change of variable. Then, we proved the existence and uniqueness of solutions for the random problem based on pathwise uniform estimates as well as the energy method. Finally, the existence of a unique pullback attractor for the random dynamical system generated by the transformed equation is shown.
Real-world applications of combinatorial optimization algorithms in fields such as logistics, manufacturing, finance, telecommunications, and computer science are vast and diverse, demonstrating the broad impact and significance of solving these complex optimization challenges. In the CDP, the objective is to maximize the dispersion of facilities while considering a capacity constraint that must meet the minimum capacity demand [17]. This NP-hard problem has been defined by [29] in order to model a type of facility location problem with dispersion. Hence, the goal is to choose a subset of facilities with the objective of maximizing their dispersion according to a specified distance measure [9]. The concept of diversity focuses on the measurement of the distances between facilities, which is customized to suit the particular requirements of the application at hand [20]. In the traditional CDP, the quantities demanded and the capacities of the facilities are assumed to be deterministic and known without any ambiguity. Nonetheless, in numerous real-world scenarios, particularly in logistics and transportation, the demand for goods or services may experience randomness, which means that the facilities' capacities might be influenced by uncertain factors. The stochastic capacitated dispersion problem (SCDP) introduces a further layer of realism by incorporating uncertainties. Actually, in many practical situations, the capacity of facilities may not always remain fixed due to a myriad of reasons, such as equipment failures, maintenance requirements, or unforeseen changes in available resources. As such, the SCDP presents a more comprehensive and accurate representation of real-world complexities in supply chain and distribution systems. One of the main challenges in choosing a facility location is ensuring it can handle customer demand. In other words, the capacity of operational facilities should ideally surpass the total demand they need to serve. This condition frequently arises in various logistics-related problems, regardless of the decision level [12].
Furthermore, the capacity parameter in the CDP may also need to be non-static to better reflect real-world scenarios. In real case studies, certain nodes may have limited capacity due to factors such as physical space, equipment availability, or regulatory restrictions. For instance, in a transportation network, some distribution centers might have zero capacity due to maintenance or temporary closures, while others operate at their maximum capacity. This implies that some facilities might unexpectedly exhibit zero capacity, determined by a black box mechanism that considers environmental, operational, or market-related variables [13]. Thus, in this paper, a new stochastic and non-static capacitated dispersion problem (SNS-CDP) is proposed. In the SNS-CDP, the capacities of the facilities may be stochastic or non-static, and a sim-learnheuristic solution approach is proposed to address the complexities introduced in the stochastic and non-static components. Figure 1 illustrates a simplified SNS-CDP where the blue shapes show the selected facilities and the white small circles show the unselected ones. In this figure, facilities are depicted with stochastic or non-static storage capacities.
Authors such as [4,27], among many others, have explained why it is important to consider random variables (following certain probability distributions) when modeling real-life capacities (or other inputs of the optimization problem). Assuming these real-life capacities as deterministic values might not properly reflect the uncertainty that characterizes real-life operations. Hence, the main contribution of this paper is designing an innovative sim-learnheuristic algorithm that hybridizes a metaheuristic algorithm, simulation, and machine learning in order to solve the novel SNS-CDP. By defining the type of each facility, the algorithm is able to address the random capacities as well as the non-static ones. In addition to its contribution to the field of facility location and optimization, the proposed sim-learnheuristic algorithm holds significant potential for broader applications in logistics, supply chain management, manufacturing, and telecommunication networks, where both the stochastic and non-static capabilities should be considered to produce solutions closer to reality. The rest of this paper is organized as follows: Section 2 presents related work on the CDP. The mathematical formulation is described in Section 3. In Section 4, our solution methodology is proposed. Following that, in Section 5, we present the numerical findings achieved through the experimentation of our method. Lastly, Section 6 summarizes the main contributions of the paper and outlines potential lines for future research.
Since the stochastic and non-static CDP have not been studied before, we provide some references on the classical version of the CDP as well as on some stochastic or dynamic versions of the more general facility location problem (FLP).
Despite the CDP not having been extensively explored in the scientific literature, there are several works on its deterministic version. For instance, Marti et al. [19] presented a heuristic based on the scatter search methodology to solve the CDP. The results demonstrate the superiority of the proposed model and heuristic over existing methods. In another paper, Marti et al. [26] proposed heuristics based on the greedy randomized adaptive search procedure (GRASP) and variable neighborhood descent (VND) methodologies to the CDP. Gomez et al. [12] proposed a multi-start biased-randomized algorithm to solve the deterministic CDP efficiently. Compared to the available instances' solutions in the literature, the proposed approach is highly competitive. In another paper, Mladenovic et al. [24] presented a variable neighborhood search VNS-based heuristics to solve the deterministic CDP. The proposed heuristics are basic VNS, general VNS, and general skewed VNS, and their efficiency has been assessed on benchmark instances. Also, Lu et al. [17] proposed a fast greedy construction parameter-free heuristic algorithm based on tabu search. Additionally, Landete et al. [16] explored the CDP from a mathematical perspective. In this work, the CDP is considered through various mathematical formulations. The study enhanced the formulations by incorporating valid inequalities and variable-fixing procedures. Computational experiments demonstrated the practicality of the proposed approaches for solving diverse instances of the problem in its deterministic version. More recently, Rosati et al. [28] proposed a multi-neighborhood simulated annealing for the CDP. This paper considers two compact mathematical models to achieve good bounds on various instance sizes.
The majority of CDP literature predominantly concentrates on the deterministic variant, while the stochastic and non-static CDP models have not been extensively addressed yet. However, a recent research by [11] presented a new simulation-based metaheuristic approach for solving the stochastic CDP. This paper introduces two models for the CDP with stochastic capacities, incorporating soft constraints and penalty costs for violating the total capacity constraint. There has been some research conducted on stochastic and/or dynamic FLPs. For instance, Bieniek [3] addressed the single source capacitated FLP with general stochastic demands. They proposed a unified solution for determining facility locations and customer allocations. Additionally, some papers have employed actual case studies on the dynamic FLP. For instance, Mišković, et al. [23] introduced a robust variant of the dynamic FLP, focusing on optimizing the emergency service network of police special forces units in Serbia. The paper also used the variable neighborhood search method with an efficient local search procedure to solve real-life problem instances. Jena et al. [15] addressed a multi-period FLP with multiple commodities and capacity levels, allowing for facility relocation and temporary closures. The study proposed a formulation for the problem and a hybrid heuristic that combines Lagrangian relaxation and a restricted mixed-integer programming model. In another paper, Marufuzzaman et al. [22] addressed the capacitated dynamic FLP, which aims to satisfy customer demands at minimum cost by determining facility opening, closing, or retention over time. To tackle this challenging problem, the paper proposed a unique hybrid solution algorithm, combining a rolling horizon algorithm with an accelerated Benders decomposition approach. Pearce et al. [25] proposed an approach to solve the budget-constrained dynamic uncapacitated facility location and network design problem optimally. The problem involves constructing or expanding a network and placing facilities within a given budget to meet demands. The study utilizes disaggregated Benders decomposition within a branch-and-cut framework. In another work, Wang et al. [31] introduced a dynamic k-level FLP considering the time factors, and proposed a combinatorial primal-dual approximation algorithm that finds a constant factor approximate solution. Additionally, Oliveira et al. [6] investigated two variants of a dynamic multi-period two-level uncapacitated FLP, where first-level plants serve scattered clients over time via second-level facilities. The study proposed a decomposition approach using Benders decomposition and GRASP. Finally, Silva et al. [30] focused on the dynamic FLP with modular capacities, aiming to minimize location and demand allocation costs over a planning horizon. They proposed three linear relaxation-based heuristics and an evolutionary heuristic that hybridizes a genetic algorithm with a variable neighborhood descent metaheuristic. Recently, Xu et al. [32] explored two ensemble methods for decision-making in stochastic mixed-integer FLP with limited computational budgets, focusing on variance reduction and probabilistic guarantees. Additionally, Ala et al. [1] exploreed the dynamic capacitated FLP for mobile renewable energy charging stations using two-stage stochastic programming. Finally, Aydin et al. [2] addressed the capacitated reliable facility location problem under uncertainty by developing two novel algorithms that integrate sample average approximation and progressive hedging algorithm.
In this section, the mathematical formulation of the deterministic CDP proposed by [29] is presented; then, the stochastic and non-static formulations derived from the deterministic model are proposed.
The CDP is mathematically defined as follows: Consider a graph G=(V,E), where V represents a set of n nodes (facilities), and E is a set of edges connecting these nodes. Each node i∈V has an average capacity denoted by ci, and for every pair of nodes i and j, connected by an edge (i,j)∈E, their distance is represented by dij (dij=dji). The objective of the CDP is to choose a subset of V, such that the total capacity of the selected nodes is equal to or exceeds a given value B, while simultaneously maximizing the minimum distance among all pairs of selected facilities. Additionally, we introduce a binary variable xi that is equal to 1 when facility i is selected, and 0 otherwise. Based on these definitions, the mathematical model of the deterministic CDP is the following:
maxmini,j∈V,i<jdijxixj | (3.1) |
s.t.:∑i∈Vcixi≥B | (3.2) |
xi∈{0,1},∀i∈V. | (3.3) |
Equation (3.1) represents the objective function aiming to maximize the minimum distance among the selected pairs of facilities. Additionally, constraints (3.2) guarantee that the selected nodes meet the minimum capacity requirement (B). Finally, constraints (3.3) refer to the nature of the xi variable, {enforcing that xi is a binary decision variable. This restriction ensures that xi=1 when facility i is selected and xi=0 when it is not}. It is assumed that ci represents the deterministic capacity of facility i.
In the stochastic model of CDP, it is assumed that the storage capacity of each facility is not known in advance. Instead, it is modeled using a probability distribution function. To achieve this, each facility is associated with an independent parameter, denoted by Ci (for all i∈V), which follows a non-negative probability distribution, such as the Weibull or log-normal distributions. The formulations of the stochastic variant are as follows:
maxmini,j∈V,i<jdijxixj | (3.4) |
s.t.: P(∑i∈VCixi≥B)≥α | (3.5) |
xi∈{0,1}∀i∈V. | (3.6) |
In this model, constraints (3.5) state that the selected facilities collectively have a capacity greater than or equal to the specified minimum requirement with a probability of at least α. When faced with a specific scenario, if the system is incapable of fulfilling the requested demand, there are no available backup or recourse actions that can be employed to revise the situation. Consequently, it becomes essential to calculate the probability of the solution's failure, which refers to the likelihood of the system not being able to provide the necessary service as required. This computation allows us to assess the reliability and performance of the system in uncertain situations, helping to identify potential shortcomings and make informed decisions to improve its overall effectiveness.
In the non-static scenario, we assume that the storage capacities of the facilities are neither deterministic nor stochastic subject to a predefined probability distribution function. We refer to it as non-static because the parameter is defined in a manner that is not mathematically dynamic, meaning it does not depend on time. However, it is also not entirely static. Therefore, in this paper, the term "non-static" denotes the specific nature of the parameter. In this scenario, the probability of obtaining a capacity for node i is assumed to follow a logistic regression model. According to [13], the formulations of the non-static component are as follows:
maxmini,j∈V,i<jdijxixj | (3.7) |
s.t.: ∑i∈Vpicixi≥B | (3.8) |
xi∈{0,1}∀i∈V. | (3.9) |
In this model, constraint (3.8) ensures that the total capacity of the selected nodes meets the minimum required threshold. This constraint incorporates a probability term pi following a Bernoulli distribution, pi∼Ber(ϕ(ci,sdi,odi)) where ϕ is a sigmoid function of the logistic regression model. The function ϕ is defined as:
ϕ(ci,sdi,odi)=11+exp(−(β0+β1ci+β2sdi+β3odi))∀i∈V. | (3.10) |
In Eq (3.10), the function ϕ is a black box model that imitates the behavior of the real world. In this function, the parameter ci represents the deterministic capacity of node i, sdi denotes the seasonal demand at node i, and odi indicates the operational disruption associated with node i. In addition, β1, β2, and β3 are dependent coefficients, while the β0 is the intercept term. In this model, the variables sdi and odi are binary variables, taking on values of either 0 or 1. A value of 1 signifies a promising condition, while a value of 0 denotes a non-promising condition.
In the stochastic-non-static scenario, we recognize that the capacities of facilities do not all follow the same statistical pattern. Some facilities' capacities are modeled stochastically, incorporating variability and uncertainty through a probability distribution function. For other facilities, capacities are considered non-static, meaning they can either be zero or match the deterministic capacity based on the variabilities of seasonal demand and operational disruption. Unlike stochastic capacities, non-static capacities are not described by probability distributions but are assumed to be influenced by a black box model.
In this section, our proposed solution approach is presented. Since the problem described in the previous section is NP-hard, an approximation algorithm is defined to address the complexities of the problem. To address the solution approach for the SNS-CDP, a sim-learnheuristic methodology is proposed. We utilize the power of metaheuristics to handle the deterministic variant of the problem effectively. Additionally, a simulation technique is integrated with the metaheuristics to address the SCDP. Finally, a machine learning component is incorporated to account for the non-static CDP. In the following subsections, each component of our sim-learnheuristic solution approach is described.
To address the deterministic version of the CDP, a novel hybrid metaheuristic is proposed. Our metaheuristic involves two distinct phases: The initial phase focuses on generating a solution, while the subsequent phase aims to improve it. During the first phase, a constructive heuristic is developed that can adapt based on the specific instance being addressed. This method is repeatedly employed in a classic multi-start pattern to create multiple solutions. In the second phase, the solutions undergo further improvement using a multi-start biased-randomized (MSBR) algorithm. This approach potentially refines the solutions to obtain higher-quality results.
The constructive heuristic, proposed by [10], involves adding elements to a partial solution in a greedy manner. In order to randomly select elements from a list of promising candidates based on the distance objective function, it is incorporated into the GRASP framework [7]. To further enhance these methods, we introduce biased-randomization (BR) techniques [14], enabling the addition of nodes. These BR techniques utilize skewed probability distributions, transforming deterministic heuristics into probabilistic algorithms. As a result, this algorithm can rapidly generate numerous high-quality solutions for the problem.
Our constructive heuristic using a BR technique starts with an initial empty solution S. During the execution of the algorithm, nodes from set V are added to solution S, all while ensuring that the capacity constraint remains unfulfilled. The instance I, which is going to be solved, encompasses important information, such as the capacity of each node (facility) i (ci), the minimum required capacity level (B), and the distances between pairs of nodes (dij). The other parameter of the algorithm is δ, which acts as a balancing factor between the distance metric and the capacity aspect within the governing greedy function. Equation (4.1) provides the mathematical expression to calculate the hi, where ds is the shortest distance between i and any other nodes j in S, and ci is the capacity of node i. Parameter δ manages the balance between distance and capacity considerations.
hi=δ⋅ds(i,j)+(1−δ)⋅ci. | (4.1) |
A flowchart of the algorithm steps is presented in Figure 2. In the algorithm, the procedure begins by initializing an empty set S to represent the evolving solution. The edges within the input graph are sorted in descending order based on their distances, forming the list edges−list, which aids edge selection. A biased-randomized selection is used to choose the edge (i0, j0) from edges−list using a geometric probability distribution function, and its nodes i0 and j0 are added to S. Then, the candidate list CL is formed, comprising nodes from V excluding those in S. The total weight of the solution S is set to the distance between i0 and j0. An iterative process begins, where nodes in CL are sorted in descending order based on a certain criterion, and a node i∗ is chosen using a geometric selection strategy. A biased random selection of the elements with the geometric probability distribution based on the h values provided by Eq (4.1) returns the element i∗ to be added to the solution. Node i∗ is added to S and the solution's total weight is updated. Then, the node i is removed from CL. This iterative process continues until S becomes feasible. Finally, the algorithm returns the evolved solution S as the output.
In the non-static model, a white box is applied in order to provide predictions to deal with the uncertainty of the problem. The white box is defined as a function that approximates the black box. In this regard, for the first two nodes, the Eq (4.2) should be used to calculate the hi instead of Eq (4.1), where prob(i) is the probability of facility i covering the capacity.
hi=δ⋅ds(i,j)+1−δ2⋅(prob(i)⋅ci+prob(j)⋅cj). | (4.2) |
In the algorithm, after generating the S set, the black box updates the capacities of the nodes regarding the facilities condition variables. In the non-static model, the candidate list evaluates the nodes and ranks them in order of preference based on an evaluation function. The evaluation function, defined in Eq (4.3), considers several key factors. The term min_distance(i) represents the minimum distance between node i and the selected facilities. The term max_min_distance is the maximum value of these minimum distances among all candidates in the candidate list. The capacity of a candidate facility is denoted by ci, while max_cap represents the maximum capacity among all candidate facilities. Additionally, prob(i) signifies the probability that facility i will cover the required capacity. The value of δ∈(0,1) is a tuning parameter that is selected based on a value that produces the best outcome.
eval(i)=δ⋅min_distance(i)max_min_distance(V∖S)+(1−δ)⋅ci⋅prob(i)max_cap(V∖S). | (4.3) |
Figure 3 shows the framework of the MSBR algorithm. This algorithm takes the initial solution as its input, denoted as S, which is generated through the constructive method, and a parameter representing the maximum number of iterations. The algorithm starts by putting the set S in an initial solution set, S0. Subsequently, the algorithm sets the counter to zero. The core of the algorithm emerges in a loop, where each iteration increments the counter by one unit. Within this iterative process, the algorithm first identifies the oldest node from S, effectively removing it from S set. Subsequently, it proceeds to select a node, denoted as u∗, with the maximum minimum distance to S. This selected node, u∗, is then incorporated into the solution set S. Following this adjustment, the algorithm evaluates the quality of the updated solution set by calculating the objective function value, denoted as f(S). If the new objective function value is better than the value associated with the initial solution set, f(S0), the algorithm updates the set S0 and resets the counter to zero. If, however, the new solution does not obtain an improvement, the algorithm continues its iterative loop. This iterative process persists until the algorithm reaches the maximum specified number of iterations. Upon reaching this threshold, the final solution set S0 becomes the output of the algorithm, representing the best solution achieved throughout the optimization process. The computational complexity of the MSBR is O(n2). This complexity arises from the necessity to sort the edge list in increasing distance order and the iterative process of selecting and removing nodes based on the geometric probability distribution until the capacity constraint is satisfied. The second-phase improvement has a complexity of O(nlogn) per iteration, due to the management of the list and neighborhood explorations. Therefore, the overall complexity of the algorithm can be described as O(n2+itmax⋅nlogn), where itmax denotes the maximum number of iterations without improvement.
Our two-phase algorithm comprising the construction and improvement phases, which encompass the constructive step, as well as MSBR improvements, is described in the previous paragraphs. We collectively refer to this two-phase algorithm, MSBR methodology in the rest of this paper. The subsequent subsection describes the sim-learnheuristic algorithm and the integration of MSBR with our simulation and machine-learning frameworks.
As it has been explained in Section 3, the non-static part of the solution works based on black box and white box mechanisms. The black box imitates the behavior of real life; however, the white box approximates the black box. A multivariate logistic regression machine learning method is applied to predict categorical values based on input variables described in Section 3. The variables used in the logistic regression model are seasonal demand, operational disruption, and deterministic capacity of each facility. This method estimates the probability of a binary dependent variable by modeling the relationship between the dependent and independent variables. The non-static capacities can be zero if the black box model predicts zero or equal to the deterministic capacity, as described in Eq (3.10). The values provided by the black box model are stored in the white box model, which then adjusts the candidate list as outlined in the constructive heuristic section. This process ensures that the capacities in our non-static component are continuously updated.
In Section 4.1.2, the MSBR algorithm specifically adjusted for addressing the deterministic variant of the CDP is introduced. However, it is not able to deal with the inherent uncertainty prevalent in real-world problems. In this section, the MSBR is improved by incorporating a simulation framework. Algorithm 1 shows the simulation procedure employed within the sim-learnheuristic approach. As mentioned before, in our SNS-CDP, the facilities are categorized into two distinct groups based on their characteristics: Facilities with stochastic or non-static capacities, which are determined through simulation. First, for stochastic facilities, the capacity is determined by generating random numbers utilizing a probability distribution function, specifically- the log-normal distribution function with location μ and scale σ parameters. As discussed in [5,18], among others, the choice of a log-normal distribution for modeling facility capacities is justified by its suitability in representing positively skewed data, which is typical in capacity-related scenarios. Notice that the specific parameters of the log-normal will be obtained from real-life observations using best-fit statistical techniques. The log-normal distribution is frequently used in practical applications where values are strictly positive and exhibit variability across several orders of magnitude. This characteristic makes it an ideal fit for modeling the stochastic nature of facility capacities in our problem. Second, black box and white box mechanisms are employed to generate the non-static capacities of the corresponding facilities, utilizing three independent variables: ci, sdi, and odi, along with their respective coefficients.
In the context of simulation (Algorithm 1), the key inputs to this algorithm include the solution, its associated nodes, nodes' types, and the maximum number of simulations. The algorithm starts by initializing an empty list named capacity. Subsequently, a loop is initiated to iterate through each of the specified maximum simulation iterations. Within this loop, a new variable named all_nodes_capacity is created. The primary objective is to populate this list with capacities associated with all facilities in all the iterations. Within another loop, facilities marked for opening_ as determined by the MSBR algorithm_ are considered to be given the capacity values based on their type. If the facility type is stochastic, the capacity is generated as a random number using the log-normal distribution function. In cases where the facility type is non-static, a black_box function generates a probability value based on deterministic capacity, seasonal demand, and operational disruption variables. The resulting capacities are then stored using the white_box function. Upon completing capacity assignments, the capacities of all facilities are aggregated into the capacity list, and the cumulative capacities are stored in the all_nodes_capacity variable. A major constraint enforced throughout this process is that the collective capacities of all facilities must meet or exceed a predefined demand threshold. However, in instances where this constraint is not met, the algorithm encounters a failure. In the final step, the total capacity is appended to the capacity list. Consequently, this list accumulates different total capacity values across different iterations. Finally, the reliability and the final solution are determined. Table 1 describes all the parameters used in the Algorithm 1.
Algorithm 1 Simulation module |
1: fail ← 0 2: capacity ← [] 3: while simulation ≤ max_simulations do 4: all_nodes_capacity ← 0 5: for each node in solution do 6: if dict_type[node] is 1 then 7: node_capacity ← StochasticValue[node] 8: else 9: probValue ← simulate_blackbox(capacity, operation_disruption, seasonal_demand) 10: node_capacity ← probValue × capacity 11: Add node_capacity to white_box 12: end if 13: Add node_capacity to all_nodes_capacity 14: end for 15: if all_nodes_capacity < max_demand then 16: fail = fail+1 17: end if 18: Append all_nodes_capacity to capacity 19: end while 20: Update reliability = (max_simulations - fail) / max_simulations |
Variable | Description |
fail | Counter for fails |
capacity | List storing the capacities of all nodes after each simulation |
max_simulations | Maximum number of simulations to run |
all_nodes_capacity | Sum of capacities for all nodes in the current simulation |
solution | Set of nodes selected to be established |
dict_type | Dictionary indicating the type of each node |
StochasticValue | Pre-determined function to generate stochastic using log-normal function |
simulate_blackbox | Function to calculate probability value based on inputs |
operation_disruption | Input parameter for the fit_blackbox function |
seasonal_demand | Input parameter for the fit_blackbox function |
probValue | Probability value obtained from fit_blackbox |
white_box | Data structure to store intermediate results for nodes not of type 1 |
node_capacity | Capacity of the current node |
max_demand | Maximum demand that all nodes' capacity must meet |
reliability | Reliability metric for the solution |
It is well-established in stochastic literature that excellent solutions obtained for the deterministic version of an optimization problem carry over its quality to its stochastic counterpart, albeit with an understanding that some level of variability comes with the random components [8]. The sim-learnheuristic uses the strength of deterministic optimization to obtain solutions that exhibit a remarkable degree of stability to the stochastic-non-static counterpart. The sim-learnheuristic employs a multi-step process to address SNS-CDP. In the initial stage, it transforms the stochastic-non-static problem into a deterministic problem by substituting expected values for stochastic-non-static parameters. Subsequently, the problem is resolved using the MSBR algorithm, the metaheuristic component, followed by refinement through a short simulation enhanced by the log-normal distribution function and machine learning techniques. This process iterates until the best solutions are achieved within the designated time frame. The pool of the best stochastic and non-static solutions is dynamically updated during this phase. In the next phase, a simulation with a high number of replications is applied to the solutions. This long simulation enables us to attain a more accurate estimation of the solutions. Finally, in the last step, the algorithm computes the reliability and assesses risks associated with the solutions, providing valuable statistical tools for result analysis. Figure 4 shows our sim-learnheuristic approach for solving the SNS-CDP. Based on the mathematical formulations provided for the stochastic CDP in Section 3, specifically the constraint 3.5, the reliability of a solution S is the probability that this solution is feasible. This approach guarantees a comprehensive optimization process, resulting in top-quality solutions with strong reliability assessments.
In this section, we explore four distinct variants of the CDP, each characterized by specific assumptions regarding facility behavior. In the first scenario, a deterministic version of the CDP is considered. In the second scenario, it is assumed that the capacity of the facilities follows a log-normal distribution function to define a stochastic scenario. In the third scenario, it is assumed that the capacities of the nodes are non-static using the whitebox_black box mechanism and the multivariate logistic regression model. Finally, in the combination scenario, there are two types of facilities. It is assumed that facilities with odd identification numbers are non-static, while the even ones are stochastic facilities. Additionally, each variant of the CDP has been solved twice. First, we consider the deterministic solution in stochastic, non-static, and stochastic-non-static environments. Then, we solve the stochastic, non-static, and stochastic-non-static problems using MSBR-simulation, MSBR-machine learning, and MSBR-simulation-machine learning, respectively.
Two groups of instances are considered to be solved. First, the GKD benchmark, constructed using Euclidean distances, with node coordinates generated from a uniform distribution ranging between 0 and 10. This dataset is originally from [21] and comprises two subsets: GKD-b (instances with 50 and 150 nodes) and GKD-c (instances with 500 nodes). The MDG benchmark incorporates real numbers randomly selected from a uniform distribution between 0 and 1000. This dataset, initially presented by [7], consists of instances with 500 nodes. In the scenarios with the stochastic capacities (scenarios two and four), the capacity of each facility is depicted as a random variable, denoted as ^Ci, using the log-normal probability distribution function with two parameters (μ, σ) that are determined using the deterministic mean capacity parameter of the facilities (ci). Additionally, the number of short and long simulation iterations in these scenarios is set at 100 and 1000, respectively.
Two sets of parameters for the stochastic and non-static problems have been defined. Table 2 shows the corresponding values for the black box coefficients β0, β1, β2, β3, and σ level of the log-normal function in low and high scenarios. Figure 5 visualizes the probability of selecting a new node using the black box model based on the chosen set of coefficients for the high and low scenarios. Three different values for the capacity, namely 10, 50, and 100, are considered. Figures 5(a)–(c) depict the visualization with the high-level set of coefficients, while Figures 5(d)–(f) show the visualization with the low-level set of coefficients. Seasonal demand and operational disruption are considered binary variables, each taking values of either 0 or 1. In Figure 5, the cells (0,0) show the probability of selecting a node under the non-promising condition. Conversely, the cells (1,1) represent the probability of selecting a node when both variables are in the promising condition.
non-static component | stochastic component | ||||
β0 | β1 | β2 | β3 | σ | |
High | -1.5 | 0.005 | 2.0 | 1.0 | 10.0 |
Low | -0.7 | 0.010 | 0.8 | 1.0 | 5.0 |
The algorithms are implemented using Python 3.10.5 and executed on an Intel(R) Core(TM)i3 CPU @ 2.00GHz with 8 GB of RAM. The sim-learnheuristic code is provided at: https://github.com/Elnazghorbani/Sim-learnheuristic_CDP. We imposed a maximum execution time of 60s for smaller and medium-sized instances (those with fewer than 500 nodes) and extended it to 180s for larger instances with 500 nodes. Tables 3 and 4 represent the results achieved for solving the different variants of the CDP for the high and low levels of coefficients, respectively. In the first column of both tables, the instances are introduced, then the deterministic solution using the MSBR algorithm is presented. The deterministic solution in the stochastic environment column reveals the performance of the deterministic solution when tested in a stochastic environment, along with its reliability (percentage of the feasibility of the solution) measure. Then, in the next column, the stochastic objective function values generated by the MSBR-simulation(sim) algorithm are presented. Similar columns are repeated for the non-static and stochastic-non-static scenarios. The solutions in the columns MSBR-machine learning(ml) and MSBR-sim-ml are provided considering the solo stochastic and the stochastic-non-static scenarios, respectively. Finally, the gaps(%) between the MSBR-sim-ml solution and deterministic solution in a non-static environment (a), MSBR-sim (b), and MSBR-ml (c) for all the instances are calculated to compare the sim-learnheuristic with other methodologies.
Instance | Deterministic Sol. | Solution to Stochastic Problem | Solution to Non-Static Problem | Solution to Stochastic- Non-Static Problem | Gaps% | |||||||||||
Deterministic Sol. in Stochastic Env. | Reliability | MSBR-Sim Sol. (a) | Reliability | Deterministic Sol. in Non-Static Env. | Reliability | MSBR-ML Sol. (b) | Reliability | Deterministic Sol. in Stochastic- Non-Static Env. (c) | Reliability | MSBR-Sim-ML Sol. (d) | Reliability | (d)-(c) | (d)-(a) | (d)-(b) | ||
GKD-b_11_n50_b02_m5.txt | 147.2 | 83.5 | 0.56 | 142.5 | 1.00 | 76.5 | 0.90 | 111.3 | 0.79 | 86.3 | 0.59 | 143.4 | 0.99 | 39.8 | 0.6 | 22.4 |
GKD-b_12_n50_b02_m5.txt | 178.1 | 144.1 | 0.81 | 170.4 | 1.00 | 45.1 | 0.25 | 168.8 | 1.00 | 84.4 | 0.47 | 166.8 | 0.99 | 49.4 | -2.2 | -1.2 |
GKD-b_13_n50_b02_m5.txt | 96.1 | 58.4 | 0.61 | 91.7 | 1.00 | 84.6 | 0.96 | 92.4 | 0.96 | 61.8 | 0.64 | 89.2 | 0.97 | 30.7 | -2.9 | -3.7 |
GKD-b_14_n50_b02_m5.txt | 84.6 | 64.3 | 0.76 | 81.2 | 1.00 | 28.3 | 0.34 | 69.3 | 0.94 | 59.3 | 0.70 | 79.6 | 0.99 | 25.5 | -2.0 | 13.0 |
GKD-b_15_n50_b02_m5.txt | 154.9 | 111.4 | 0.72 | 147.3 | 1.00 | 57.2 | 0.37 | 121.7 | 0.83 | 58.1 | 0.38 | 143.7 | 0.98 | 59.6 | -2.5 | 15.3 |
GKD-b_16_n50_b02_m15.txt | 77.7 | 55.2 | 0.71 | 76.1 | 0.98 | 40.8 | 0.53 | 67.0 | 0.95 | 46.1 | 0.59 | 60.8 | 0.87 | 24.2 | -25.3 | -10.2 |
GKD-b_17_n50_b02_m15.txt | 41.8 | 21.6 | 0.52 | 35.8 | 0.98 | 23.2 | 0.56 | 33.8 | 0.98 | 13.5 | 0.32 | 26.9 | 0.76 | 50.0 | -33.0 | -25.5 |
GKD-b_18_n50_b02_m15.txt | 108.5 | 69.0 | 0.64 | 104.0 | 1.00 | 99.8 | 0.92 | 103.4 | 1.00 | 69.1 | 0.64 | 96.7 | 0.99 | 28.5 | -7.5 | -6.9 |
GKD-b_19_n50_b02_m15.txt | 119.1 | 112.0 | 0.96 | 114.6 | 1.00 | 64.3 | 0.54 | 108.6 | 0.97 | 87.4 | 0.73 | 110.6 | 0.99 | 21.0 | -3.6 | 1.8 |
GKD-b_20_n50_b02_m15.txt | 115.3 | 58.1 | 0.50 | 107.4 | 1.00 | 105.8 | 0.92 | 106.0 | 1.00 | 59.6 | 0.52 | 104.9 | 0.97 | 43.2 | -2.4 | -1.0 |
GKD-b_41_n150_b02_m15.txt | 163.9 | 92.1 | 0.56 | 156.2 | 1.00 | 87.9 | 0.54 | 152.8 | 1.00 | 93.6 | 0.58 | 152.8 | 1.00 | 38.8 | -2.2 | 0.0 |
GKD-b_42_n150_b02_m15.txt | 84.0 | 66.3 | 0.79 | 74.0 | 0.97 | 7.1 | 0.08 | 75.8 | 1.00 | 19.7 | 0.24 | 79.9 | 1.00 | 75.3 | 7.4 | 5.1 |
GKD-b_43_n150_b02_m15.txt | 62.5 | 39.5 | 0.63 | 57.2 | 0.99 | 6.4 | 0.10 | 58.2 | 1.00 | 12.6 | 0.20 | 54.7 | 1.00 | 76.9 | -4.6 | -6.5 |
GKD-b_44_n150_b02_m15.txt | 100.6 | 63.8 | 0.63 | 95.4 | 0.99 | 0.1 | 0.00 | 91.7 | 1.00 | 5.0 | 0.10 | 95.9 | 1.00 | 94.8 | 0.5 | 4.4 |
GKD-b_45_n150_b02_m15.txt | 104.4 | 59.0 | 0.57 | 99.4 | 1.00 | 1.3 | 0.01 | 89.6 | 0.91 | 17.6 | 0.17 | 94.4 | 0.95 | 81.3 | -5.3 | 5.1 |
GKD-b_46_n150_b02_m45.txt | 123.3 | 69.3 | 0.56 | 116.6 | 0.99 | 62.6 | 0.51 | 111.7 | 1.00 | 55.9 | 0.45 | 115.7 | 1.00 | 51.7 | -1.0 | 3.5 |
GKD-b_47_n150_b02_m45.txt | 162.8 | 112.3 | 0.69 | 150.0 | 0.99 | 100.3 | 0.62 | 148.5 | 1.00 | 95.1 | 0.58 | 153.8 | 1.00 | 38.2 | 2.5 | 3.4 |
GKD-b_48_n150_b02_m45.txt | 99.8 | 70.1 | 0.70 | 94.3 | 0.97 | 4.0 | 0.04 | 86.1 | 0.99 | 17.7 | 0.18 | 93.1 | 1.00 | 81.0 | -1.2 | 7.5 |
GKD-b_49_n150_b02_m45.txt | 166.3 | 113.6 | 0.68 | 165.4 | 1.00 | 85.0 | 0.51 | 158.7 | 0.99 | 107.1 | 0.64 | 152.1 | 0.94 | 29.6 | -8.7 | -4.3 |
GKD-b_50_n150_b02_m45.txt | 110.1 | 65.7 | 0.60 | 105.4 | 0.99 | 90.8 | 0.83 | 100.9 | 0.96 | 65.0 | 0.59 | 98.8 | 0.94 | 34.3 | -6.7 | -2.1 |
MDG-b_01_n500_b02_m50.txt | 52.0 | 31.0 | 0.60 | 34.0 | 0.99 | 0.0 | 0.00 | 23.0 | 0.96 | 1.2 | 0.02 | 23.1 | 0.97 | 94.8 | -47.1 | 0.2 |
MDG-b_02_n500_b02_m50.txt | 43.6 | 25.4 | 0.58 | 25.9 | 1.00 | 4.1 | 0.09 | 29.2 | 0.97 | 14.7 | 0.34 | 26.4 | 1.00 | 44.2 | 1.9 | -10.6 |
MDG-b_03_n500_b02_m50.txt | 43.6 | 24.5 | 0.56 | 29.7 | 0.96 | 0.0 | 0.00 | 26.4 | 0.98 | 1.1 | 0.03 | 29.7 | 0.99 | 96.2 | 0.1 | 11.3 |
MDG-b_04_n500_b02_m50.txt | 44.5 | 29.3 | 0.71 | 31.6 | 1.00 | 0.0 | 0.00 | 24.0 | 1.00 | 3.6 | 0.08 | 21.4 | 0.63 | 83.3 | -47.7 | -12.4 |
MDG-b_05_n500_b02_m50.txt | 46.5 | 25.1 | 0.69 | 32.0 | 0.96 | 0.1 | 0.00 | 23.4 | 1.00 | 6.2 | 0.13 | 26.5 | 1.00 | 76.5 | -20.7 | 11.7 |
MDG-b_06_n500_b02_m50.txt | 45.3 | 28.7 | 0.63 | 35.0 | 1.00 | 7.5 | 0.17 | 33.2 | 0.98 | 20.6 | 0.46 | 30.3 | 0.99 | 32.0 | -15.7 | -9.7 |
MDG-b_07_n500_b02_m50.txt | 42.6 | 24.8 | 0.58 | 34.6 | 0.99 | 0.0 | 0.00 | 21.0 | 0.86 | 13.7 | 0.32 | 24.5 | 1.00 | 44.0 | -41.2 | 14.4 |
MDG-b_08_n500_b02_m50.txt | 48.0 | 29.6 | 0.80 | 38.4 | 0.99 | 23.9 | 0.50 | 41.3 | 1.00 | 23.9 | 0.70 | 33.6 | 0.93 | 28.8 | -14.6 | -23.1 |
MDG-b_09_n500_b02_m50.txt | 49.4 | 37.6 | 0.76 | 38.3 | 1.00 | 15.4 | 0.31 | 32.9 | 0.98 | 26.3 | 0.53 | 26.5 | 1.00 | 0.8 | -44.5 | -24.3 |
MDG-b_10_n500_b02_m50.txt | 50.9 | 34.5 | 0.68 | 37.5 | 1.00 | 0.0 | 0.00 | 28.7 | 1.00 | 2.2 | 0.04 | 27.1 | 0.98 | 91.9 | -38.3 | -5.7 |
GKD-c_01_n500_b02_m50.txt | 9.1 | 6.9 | 0.76 | 8.4 | 1.00 | 1.9 | 0.21 | 8.5 | 0.98 | 4.9 | 0.54 | 8.6 | 1.00 | 43.4 | 2.4 | 0.9 |
GKD-c_02_n500_b02_m50.txt | 9.3 | 6.0 | 0.64 | 9.0 | 0.97 | 1.9 | 0.20 | 4.6 | 0.52 | 3.9 | 0.42 | 8.3 | 0.97 | 52.5 | -8.8 | 44.1 |
GKD-c_03_n500_b02_m50.txt | 9.2 | 7.0 | 0.76 | 8.6 | 0.99 | 3.4 | 0.37 | 8.5 | 1.00 | 5.6 | 0.61 | 8.3 | 0.99 | 32.4 | -3.4 | -2.1 |
GKD-c_04_n500_b02_m50.txt | 9.0 | 5.1 | 0.57 | 8.6 | 0.99 | 1.4 | 0.15 | 8.4 | 1.00 | 2.8 | 0.31 | 8.4 | 0.98 | 66.8 | -2.3 | 0.3 |
GKD-c_05_n500_b02_m50.txt | 9.0 | 7.3 | 0.81 | 8.8 | 0.98 | 4.0 | 0.44 | 8.4 | 1.00 | 6.2 | 0.69 | 8.5 | 1.00 | 27.3 | -3.7 | 1.2 |
GKD-c_06_n500_b02_m50.txt | 9.0 | 6.1 | 0.67 | 8.7 | 0.99 | 1.8 | 0.20 | 7.5 | 0.90 | 4.2 | 0.46 | 8.5 | 0.99 | 51.0 | -2.1 | 11.9 |
GKD-c_07_n500_b02_m50.txt | 9.1 | 8.0 | 0.88 | 8.7 | 1.00 | 4.1 | 0.46 | 8.3 | 0.97 | 6.3 | 0.70 | 8.6 | 0.99 | 26.4 | -0.8 | 3.5 |
GKD-c_08_n500_b02_m50.txt | 9.3 | 6.7 | 0.72 | 8.9 | 1.00 | 4.6 | 0.49 | 8.6 | 1.00 | 5.8 | 0.62 | 8.9 | 1.00 | 35.0 | 0.0 | 3.4 |
GKD-c_09_n500_b02_m50.txt | 9.0 | 7.0 | 0.78 | 8.7 | 1.00 | 2.8 | 0.31 | 0.6 | 0.35 | 5.9 | 0.66 | 8.7 | 0.99 | 31.9 | 0.2 | 92.9 |
GKD-c_10_n500_b02_m50.txt | 9.1 | 6.8 | 0.75 | 8.8 | 1.00 | 0.0 | 0.00 | 8.4 | 0.97 | 1.2 | 0.13 | 8.7 | 1.00 | 86.6 | -1.2 | 3.0 |
Average | 71.5 | 47.2 | 0.68 | 65.2 | 0.99 | 28.7 | 0.34 | 60.3 | 0.94 | 31.9 | 0.43 | 61.7 | 0.97 | 50.5 | -9.7 | 3.3 |
Instance | Deterministic Sol. | Solution to Stochastic Problem | Solution to Non-Static Problem | Solution to Stochastic- Non-Static Problem | Gaps% | |||||||||||
Deterministic Sol. in Stochastic Env. | Reliability | MSBR-Sim Sol. (a) | Reliability | Deterministic Sol. in Non-Static Env. | Reliability | MSBR-ML Sol. (b) | Reliability | Deterministic Sol. in Stochastic- Non-Static Env. (c) | Reliability | MSBR-Sim-ML Sol. (d) | Reliability | (d)-(c) | (d)-(a) | (d)-(b) | ||
GKD-b_11_n50_b02_m5.txt | 147.2 | 83.5 | 0.57 | 142.8 | 1.00 | 140.0 | 0.95 | 142.9 | 1.00 | 89.4 | 0.61 | 137.3 | 1.00 | 34.9 | -4.0 | -4.1 |
GKD-b_12_n50_b02_m5.txt | 178.1 | 144.1 | 0.81 | 170.4 | 1.00 | 153.2 | 0.86 | 171.4 | 1.00 | 146.9 | 0.83 | 166.1 | 1.00 | 11.6 | -2.6 | -3.2 |
GKD-b_13_n50_b02_m5.txt | 96.1 | 58.4 | 0.61 | 91.9 | 1.00 | 65.2 | 1.00 | 92.2 | 1.00 | 62.1 | 0.65 | 92.2 | 1.00 | 32.7 | 0.3 | 0.0 |
GKD-b_14_n50_b02_m5.txt | 84.6 | 64.3 | 0.76 | 81.2 | 1.00 | 75.2 | 0.89 | 80.6 | 1.00 | 70.4 | 0.83 | 81.2 | 1.00 | 13.3 | 0.0 | 0.7 |
GKD-b_15_n50_b02_m5.txt | 154.9 | 111.4 | 0.72 | 147.3 | 1.00 | 143.6 | 0.93 | 150.0 | 0.98 | 114.0 | 0.74 | 148.7 | 0.99 | 23.3 | 0.9 | -0.9 |
GKD-b_16_n50_b02_m15.txt | 77.7 | 55.2 | 0.71 | 76.1 | 0.98 | 66.6 | 0.97 | 74.9 | 1.00 | 65.5 | 0.84 | 71.3 | 0.94 | 8.1 | -6.9 | -5.1 |
GKD-b_17_n50_b02_m15.txt | 41.8 | 21.6 | 0.52 | 35.8 | 0.98 | 26.2 | 0.97 | 39.8 | 0.98 | 22.3 | 0.53 | 34.6 | 1.00 | 35.5 | -3.4 | -15.0 |
GKD-b_18_n50_b02_m15.txt | 108.5 | 69.0 | 0.64 | 103.9 | 1.00 | 75.1 | 0.99 | 104.3 | 1.00 | 71.3 | 0.66 | 103.7 | 0.99 | 31.2 | -0.2 | -0.6 |
GKD-b_19_n50_b02_m15.txt | 119.1 | 114.6 | 0.96 | 115.3 | 1.00 | 115.2 | 0.97 | 115.3 | 0.96 | 110.2 | 0.98 | 111.6 | 1.00 | 1.2 | -3.3 | -3.3 |
GKD-b_20_n50_b02_m15.txt | 115.3 | 58.1 | 0.50 | 107.3 | 1.00 | 60.1 | 1.00 | 111.3 | 1.00 | 61.3 | 0.53 | 111.8 | 1.00 | 45.1 | 4.0 | 0.5 |
GKD-b_41_n150_b02_m15.txt | 163.9 | 92.1 | 0.56 | 156.2 | 1.00 | 154.0 | 0.95 | 156.0 | 0.99 | 106.1 | 0.65 | 154.4 | 0.99 | 31.3 | -1.1 | -1.0 |
GKD-b_42_n150_b02_m15.txt | 84.0 | 66.3 | 0.79 | 74.0 | 0.97 | 75.5 | 0.90 | 76.3 | 1.00 | 71.7 | 0.85 | 79.0 | 1.00 | 9.2 | 6.3 | 3.4 |
GKD-b_43_n150_b02_m15.txt | 62.5 | 39.5 | 0.63 | 57.2 | 0.99 | 57.4 | 0.92 | 59.8 | 0.97 | 40.9 | 0.66 | 58.5 | 0.99 | 30.0 | 2.2 | -2.3 |
GKD-b_44_n150_b02_m15.txt | 100.6 | 63.8 | 0.63 | 95.4 | 0.99 | 55.3 | 0.55 | 98.9 | 1.00 | 51.7 | 0.51 | 95.6 | 0.99 | 45.9 | 0.2 | -3.4 |
GKD-b_45_n150_b02_m15.txt | 104.4 | 59.0 | 0.57 | 99.4 | 1.00 | 66.3 | 0.64 | 102.4 | 1.00 | 56.2 | 0.54 | 101.5 | 0.98 | 44.7 | 2.1 | -0.9 |
GKD-b_46_n150_b02_m45.txt | 123.3 | 69.3 | 0.56 | 116.6 | 0.99 | 90.1 | 0.73 | 114.3 | 0.97 | 65.1 | 0.53 | 115.4 | 1.00 | 43.6 | -1.1 | 0.9 |
GKD-b_47_n150_b02_m45.txt | 162.8 | 112.3 | 0.69 | 150.0 | 0.99 | 144.1 | 0.89 | 155.7 | 1.00 | 118.4 | 0.73 | 157.0 | 0.99 | 24.6 | 4.5 | 0.8 |
GKD-b_48_n150_b02_m45.txt | 99.8 | 70.1 | 0.70 | 94.3 | 0.97 | 72.8 | 0.73 | 96.1 | 1.00 | 63.9 | 0.64 | 93.6 | 1.00 | 31.8 | -0.7 | -2.7 |
GKD-b_49_n150_b02_m45.txt | 166.3 | 113.6 | 0.68 | 165.4 | 1.00 | 139.0 | 0.84 | 161.8 | 1.00 | 123.4 | 0.74 | 163.4 | 1.00 | 24.5 | -1.2 | 1.0 |
GKD-b_50_n150_b02_m45.txt | 110.1 | 65.7 | 0.60 | 105.4 | 0.99 | 68.1 | 0.97 | 106.2 | 1.00 | 69.0 | 0.63 | 106.5 | 1.00 | 35.2 | 1.0 | 0.3 |
MDG-b_01_n500_b02_m50.txt | 52.0 | 31.0 | 0.60 | 34.0 | 0.99 | 12.5 | 0.24 | 33.2 | 0.95 | 23.9 | 0.46 | 39.4 | 0.95 | 39.3 | 13.9 | 15.9 |
MDG-b_02_n500_b02_m50.txt | 43.6 | 25.4 | 0.58 | 25.9 | 1.00 | 15.8 | 0.36 | 32.5 | 0.99 | 23.2 | 0.53 | 26.2 | 0.98 | 11.2 | 1.0 | -24.1 |
MDG-b_03_n500_b02_m50.txt | 43.6 | 24.5 | 0.56 | 29.7 | 0.96 | 9.5 | 0.22 | 25.5 | 0.96 | 21.8 | 0.50 | 31.9 | 1.00 | 31.7 | 7.0 | 20.0 |
MDG-b_04_n500_b02_m50.txt | 44.5 | 33.2 | 0.73 | 35.3 | 1.00 | 24.0 | 0.54 | 40.4 | 1.00 | 22.4 | 0.76 | 24.9 | 1.00 | 10.0 | -41.8 | -62.3 |
MDG-b_05_n500_b02_m50.txt | 46.5 | 32.0 | 0.69 | 33.1 | 1.00 | 31.6 | 0.68 | 31.7 | 1.00 | 32.4 | 0.70 | 32.6 | 1.00 | 0.6 | -1.5 | 2.8 |
MDG-b_06_n500_b02_m50.txt | 45.3 | 32.3 | 0.71 | 35.0 | 0.99 | 27.2 | 0.60 | 29.8 | 0.99 | 27.1 | 0.71 | 27.4 | 0.99 | 1.2 | -27.7 | -8.6 |
MDG-b_07_n500_b02_m50.txt | 42.6 | 24.8 | 0.58 | 34.6 | 1.00 | 19.4 | 0.46 | 26.0 | 1.00 | 23.8 | 0.53 | 28.0 | 0.99 | 15.0 | -23.8 | 7.0 |
MDG-b_08_n500_b02_m50.txt | 48.0 | 38.4 | 0.80 | 44.5 | 1.00 | 38.0 | 0.95 | 44.5 | 1.00 | 39.0 | 0.81 | 40.3 | 1.00 | 3.3 | -10.4 | -10.4 |
MDG-b_09_n500_b02_m50.txt | 49.4 | 37.6 | 0.76 | 38.3 | 1.00 | 30.1 | 0.91 | 32.7 | 1.00 | 27.2 | 0.75 | 29.5 | 0.99 | 7.8 | -29.8 | -10.8 |
MDG-b_10_n500_b02_m50.txt | 50.9 | 34.5 | 0.68 | 37.5 | 0.96 | 17.3 | 0.34 | 34.3 | 1.00 | 31.7 | 0.62 | 38.9 | 0.98 | 18.5 | 3.5 | 11.8 |
GKD-c_01_n500_b02_m50.txt | 9.1 | 6.9 | 0.76 | 8.5 | 0.97 | 6.6 | 0.73 | 8.5 | 0.99 | 6.8 | 0.74 | 8.8 | 0.99 | 23.3 | 4.0 | 3.1 |
GKD-c_02_n500_b02_m50.txt | 9.3 | 5.6 | 0.61 | 9.0 | 0.99 | 6.1 | 0.66 | 8.7 | 0.99 | 5.6 | 0.60 | 9.0 | 1.00 | 38.1 | -0.1 | 3.2 |
GKD-c_03_n500_b02_m50.txt | 9.2 | 7.0 | 0.76 | 8.6 | 0.99 | 8.5 | 0.92 | 8.7 | 0.99 | 7.1 | 0.77 | 8.5 | 0.97 | 16.0 | -1.8 | -2.9 |
GKD-c_04_n500_b02_m50.txt | 9.0 | 5.1 | 0.57 | 8.6 | 0.98 | 4.0 | 0.44 | 8.8 | 1.00 | 4.4 | 0.48 | 8.7 | 0.99 | 50.2 | 1.4 | -0.6 |
GKD-c_05_n500_b02_m50.txt | 9.0 | 7.3 | 0.81 | 8.8 | 0.99 | 7.6 | 0.96 | 8.5 | 1.00 | 7.8 | 0.87 | 8.6 | 0.99 | 9.1 | -2.3 | 1.3 |
GKD-c_06_n500_b02_m50.txt | 9.0 | 6.0 | 0.67 | 8.7 | 1.00 | 5.8 | 0.65 | 8.7 | 0.99 | 5.8 | 0.65 | 8.6 | 1.00 | 32.4 | -1.0 | -1.3 |
GKD-c_07_n500_b02_m50.txt | 9.1 | 5.9 | 0.66 | 8.7 | 0.99 | 8.0 | 0.95 | 8.6 | 1.00 | 8.1 | 0.89 | 8.4 | 0.99 | 4.1 | -2.6 | -1.8 |
GKD-c_08_n500_b02_m50.txt | 9.3 | 6.7 | 0.72 | 8.9 | 1.00 | 8.7 | 0.94 | 9.0 | 1.00 | 6.8 | 0.73 | 8.8 | 1.00 | 22.5 | -1.3 | -2.5 |
GKD-c_09_n500_b02_m50.txt | 9.0 | 4.7 | 0.52 | 8.7 | 1.00 | 7.8 | 0.87 | 8.7 | 1.00 | 7.6 | 0.84 | 8.7 | 1.00 | 12.4 | -0.2 | -0.3 |
GKD-c_10_n500_b02_m50.txt | 9.1 | 6.8 | 0.75 | 8.8 | 1.00 | 7.3 | 0.81 | 8.6 | 0.98 | 6.5 | 0.72 | 8.7 | 1.00 | 24.9 | -1.2 | 0.9 |
Average | 71.5 | 47.7 | 0.67 | 65.5 | 0.99 | 53.5 | 0.77 | 65.7 | 0.99 | 48.0 | 0.68 | 64.7 | 0.99 | 23.2 | -2.9 | -2.4 |
Figure 6 presents a comparison of solutions across various scenarios for all instances. Figure 6(a) illustrates the behavior of the methodologies with high-level coefficients, while Figure 6(b) shows the results for low-level coefficients. As expected, the deterministic values serve as upper bounds for all solutions. Notably, across solution methodologies—MSBR-sim, MSBR-ml, and MSBR-sim-ml—the objective function values consistently surpass those of deterministic solutions in stochastic, non-static, and combined stochastic-non-static environments for both levels of coefficients. The results underscore the robustness of the sim-learnheuristic algorithm, demonstrating its efficacy alongside MSBR-sim and MSBR-ml approaches in addressing combined uncertainties within the stochastic-non-static scenario.
Figure 7 compares the percentage gaps of the MSBR-sim-ml with other methodologies. The data is provided in the last three columns of Tables 3 and 4. These figures highlight the significant positive gaps between the solutions provided by the MSBR-sim-ml and the deterministic solutions in a stochastic-non-static environment for both levels of coefficients. Figure 7(a) demonstrates minimal gaps between MSBR-sim-ml and other methodologies, such as MSBR-sim and MSBR-ml. Additionally, Figure 7(b) confirms the similar behavior of MSBR-sim-ml to MSBR-sim and MSBR-ml in low-level scenarios. Besides, it indicates that even in low-level scenarios, the performance of the sim-learnheuristic is superior to the deterministic solution in a stochastic-non-static environment.
In this study, novel variants of the CDP have been explored by considering the storage capacity parameter of the facilities as stochastic, non-static, and a combination of stochastic and non-static values. To address the proposed combination problem, a sim-learnheuristic solution approach is developed in order to use the power of machine learning, metaheuristic, and simulation to respond to the stochastic and non-static characteristics of the problem. In this approach, the facilities are classified into two distinct categories based on their unique characteristics. In particular, stochastic facilities are characterized by capacities produced by a log-normal distribution function, while non-static facilities are modeled using a machine learning framework to predict their non-static behavior. Defining both a black box and a white box allows for the creation of a procedure designed to receive real data and generate an approximation thereof. Notably, a multivariate logistic regression model has been applied to perform a classification prediction. The predictions are multiplied by the deterministic capacities. Thus, the non-static capacities can be either zero or equal to the corresponding deterministic capacity value. The logistic regression model incorporates independent factors, each contributing unique coefficients to the modeling process. A constructive multi-start biased-randomized (MSBR) technique is proposed as the metaheuristic component to resolve the problem's deterministic version by replacing the random capacities' expected values. Additionally, a Monte Carlo simulation technique is incorporated to provide the solution for the combination model by a maximum iteration number.
To provide a comprehensive assessment of the results, four different scenarios for the CDP are considered: deterministic, solo-stochastic, solo- non-static, and stochastic- non-static. The deterministic scenario has been answered by using the proposed metaheuristic algorithm. However, the second scenario is resolved by MSBR and the simulation component. Besides, the solo- non-static variant has been answered by a combination of MSBR and our machine-learning component. Finally, the stochastic- non-static scenario has been addressed by our sim-learnheuristic method, which is a combination of the metaheuristic, simulation, and machine-learning components. Two types of comparisons have been conducted. First, we generate deterministic solutions within stochastic, non-static, or stochastic- non-static environments, accompanied by their respective reliability values, to facilitate comparisons with our methodologies. Second, we evaluate the efficiency of our proposed sim-learnheuristic (MSBR-sim-ml) algorithm by comparing it with other solution approaches like MSBR-sim and MSBR-ml. This comparison aims to demonstrate the effectiveness of our methodology in addressing two types of uncertainties.
As expected, the results demonstrated that the deterministic scenario produced the most favorable outcomes. However, the results for the deterministic scenario are not reliable, since it underestimates the real-world constraints. In the first comparison, the sim-learnheuristic outperforms deterministic solutions within the stochastic- non-static environment, as well as the other two solution approaches (MSBR-sim and MSBR-ml). The second comparison highlights the strong performance of the sim-learnheuristic, with only minor gaps observed in its behavior compared with the two other methodologies.
In the future, prospective research should explore deeper the complexities associated with non-static storage capacities. For instance, exploring more intricate models, such as neural networks or other advanced methods, can enhance the predictions. Moreover, employing sophisticated modeling techniques like time-series forecasting has the potential to significantly enhance the predictions of facility capacity. Besides, in future works, a comprehensive analysis can be conducted to discover other factors influencing changes in facility capacity and identify additional variables that may impact the dynamic values. Finally, future studies can improve our understanding of this field by proposing other methodologies to compare the results achieved in this paper using different solution approaches.
A. A. Juan and J. Panadero developed the main idea of the study; E. Ghorbani carried out the research, testing the instances; J. F. Gomez and E. Ghorbani planned the algorithms used in the research and implemented them in software; E. Ghorbani wrote the first draft of the paper; J. Panadero checked the results to make sure they were accurate; J. Panadero and A. A. Juan supervised the project, reviewed the paper, and provided guidance throughout the process. All authors have read and approved the final version of the manuscript for publication.
The authors declare they have not used Artificial Intelligence (AI) tools in the creation of this article.
This work has been partially funded by the Spanish Ministry of Science and Innovation (PID2022-138860NB-I00, RED2022-134703-T) as well as by the Generalitat Valenciana (PROMETEO/2021/065).
The authors declare no conflict of interest.
[1] | M. Chipot, Elements of Nonlinear Analysis, Birkhäuser, Basel, 2000. |
[2] |
M. Chipot, B. Lovat, Some remarks on nonlocal elliptic and parabolic problems, Nonlinear Anal., 30 (1997), 4619–4627. https://doi.org/10.1016/S0362-546X(97)00169-7 doi: 10.1016/S0362-546X(97)00169-7
![]() |
[3] |
M. Chipot, J. F. Rodrigues, On a class of nonlocal nonlinear elliptic problems, RAIRO Modél. Math. Anal. Numér., 26 (1992), 447–467. https://doi.org/10.1051/M2AN/1992260304471 doi: 10.1051/M2AN/1992260304471
![]() |
[4] | B. Lovat, Etudes de quelques problèmes paraboliques non locaux, PhD Thesis, Uniersité de Metz, 1995. |
[5] |
Y. Shi, X. H. Yang, Pointwise error estimate of conservative difference scheme for supergeneralized viscous Burgers' equation, Electron. Res. Arch., 32 (2024), 1471–1497. https://doi.org/10.3934/era.2024068 doi: 10.3934/era.2024068
![]() |
[6] |
J. W. Wang, X. X. J, H. X. Zhang, A BDF3 and new nonlinear fourth-order difference scheme for the generalized viscous Burgers' equation, Appl. Math. Lett., 151 (2024), 109002. https://doi.org/10.1016/j.aml.2024.109002 doi: 10.1016/j.aml.2024.109002
![]() |
[7] |
J. W. Wang, X. Xiao, X. H. Yang, H. X. Zhang, A nonlinear compact method based on double reduction order scheme for the nonlocal fourth-order PDEs with Burgers' type nonlinearity, J. Appl. Math. Comput., 70 (2024), 489–511. https://doi.org/10.1007/s12190-023-01975-4 doi: 10.1007/s12190-023-01975-4
![]() |
[8] |
X. Wang, X. H. Yang, Z. Y. Zhou, X. Wang, X. J. Yang, Z. Y. Zhou, Pointwise-in-time-robust error estimate of the ADI difference scheme for three-dimensional fractional subdiffusion equations with variable coefficients, Commun. Anal. Mech., 16 (2024), 53–70. https://doi.org/10.3934/cam.2024003 doi: 10.3934/cam.2024003
![]() |
[9] |
C. J. Li, H. X. Zhang, X. H. Yang, A new-robust nonlinear numerical algorithm for the time fractional nonlinear KdV equation, Commun. Anal. Mech., 16 (2024), 147–168. https://doi.org/10.3934/cam.2024007 doi: 10.3934/cam.2024007
![]() |
[10] |
J. H. Xu, Z. C. Zhang, T. Caraballo, Mild solutions to time fractional stochastic 2D-Stokes equations with bounded and unbounded delay, J. Dynam. Differential Equations, 34 (2022), 583–603. https://doi.org/10.1007/s10884-019-09809-3 doi: 10.1007/s10884-019-09809-3
![]() |
[11] |
H. X. Zhang, X. X. Jiang, F. R. Wang, X. H. Yang, The time two-grid algorithm combined with difference scheme for 2D nonlocal nonlinear wave equation. J. Appl. Math. Comput., (2024). https://doi.org/10.1007/s12190-024-02000-y doi: 10.1007/s12190-024-02000-y
![]() |
[12] |
R. Servadei, E. Valdinoci, Variational methods for non-local operators of elliptic type, Discrete Contin. Dyn. Syst., 33 (2013), 2105–2137. https://doi.org/10.3934/dcds.2013.33.2105 doi: 10.3934/dcds.2013.33.2105
![]() |
[13] |
R. Servadei, E. Valdinoci, On the spectrum of two different fractional operators, Proc. Roy. Soc. Edinburgh Sect. A, 144 (2014), 831–855. https://doi.org/10.1017/S0308210512001783 doi: 10.1017/S0308210512001783
![]() |
[14] |
J. H. Xu, Z. C. Zhang, T. Caraballo, Non-autonomous nonlocal partial differential equations with delay and memory, J. Differential Equations, 270 (2021), 505–546. https://doi.org/10.1016/j.jde.2020.07.037 doi: 10.1016/j.jde.2020.07.037
![]() |
[15] |
M. Fardi, M. A. Zaky, A. S. Hendy, Nonuniform difference schemes for multi-term and distributed-order fractional parabolic equations with fractional Laplacian, Math. Comput. Simulation, 206 (2023), 614–635. https://doi.org/10.1016/j.matcom.2022.12.009 doi: 10.1016/j.matcom.2022.12.009
![]() |
[16] | M. Fardi, S. K. Q. Al-Omari, S. Araci, A pseudo-spectral method based on reproducing kernel for solving the time-fractional diffusion-wave equation, Adv. Contin. Discrete Models, (2022), Paper No. 54, 14. https://doi.org/10.1186/s13662-022-03726-4 |
[17] |
S. Mohammadi, M. Fardi, M. Ghasemi, A numerical investigation with energy-preservation for nonlinear space-fractional Klein-Gordon-Schrödinger system, Comput. Appl. Math., 42 (2023), Paper No. 356, 27. https://doi.org/10.1007/s40314-023-02495-4 doi: 10.1007/s40314-023-02495-4
![]() |
[18] |
S. Mohammadi, M. Ghasemi, M. Fardi, A fast Fourier spectral exponential time-differencing method for solving the time-fractional mobile-immobile advection-dispersion equation, Comput. Appl. Math., 41 (2022), Paper No. 264, 26. https://doi.org/10.1007/s40314-022-01970-8 doi: 10.1007/s40314-022-01970-8
![]() |
[19] |
H. L, J. G. Qi, B. X. Wang, M. J. Zhang, Random attractors for non-autonomous fractional stochastic parabolic equations on unbounded domains, Discrete Contin. Dyn. Syst., 39 (2019), 683–706. https://doi.org/10.3934/dcds.2019028 doi: 10.3934/dcds.2019028
![]() |
[20] |
B. X. Wang, Sufficient and necessary criteria for existence of pullback attractors for non-compact random dynamical systems, J. Differential Equations, 253 (2012), 1544–1583. https://doi.org/10.1016/j.jde.2012.05.015 doi: 10.1016/j.jde.2012.05.015
![]() |
[21] |
B. X. Wang, Random attractors for non-autonomous stochastic wave equations with multiplicative noise, Discrete Contin. Dyn. Syst., 34 (2014), 269–300. https://doi.org/10.3934/dcds.2014.34.269 doi: 10.3934/dcds.2014.34.269
![]() |
[22] |
H. Crauel, A. Debussche, F. Flandoli, Random attractors, J. Dynam. Differential Equations, 9 (1997), 307–341. https://doi.org/10.1007/BF02219225 doi: 10.1007/BF02219225
![]() |
[23] |
H. Crauel, F. Flandoli, Attractors for random dynamical systems, Probab. Th. Rel. Fields, 100 (1994), 365–393. https://doi.org/10.1007/BF01193705 doi: 10.1007/BF01193705
![]() |
[24] | B. Schmalfuß, Backward cocycles and attractors of stochastic differential equations, International Seminar on Applied Mathematics-Nonlinear Dynamics: Attractor Approximation and Global Behavior, 185–192, Dresden, 1992. |
[25] |
E. Di Nezza, G. Palatucci, E. Valdinoci, Hitchhiker's guide to the fractional Sobolev spaces, Bull. Sci. Math., 136 (2012), 521–573. https://doi.org/10.1016/j.bulsci.2011.12.004 doi: 10.1016/j.bulsci.2011.12.004
![]() |
[26] |
B. X. Wang, Asymptotic behavior of non-autonomous fractional stochastic reaction-diffusion equations, Nonlinear Anal., 158 (2017), 60–82. https://doi.org/10.1016/j.na.2017.04.006 doi: 10.1016/j.na.2017.04.006
![]() |
[27] |
J. H. Xu, T. Caraballo, Dynamics of stochastic nonlocal reaction-diffusion equations driven by multiplicative noise, Anal. Appl., 21 (2023), 597–633. https://doi.org/10.1142/S0219530522500075 doi: 10.1142/S0219530522500075
![]() |
[28] | J. L. Lions, Quelques Methodes de Resolution des Problemes aux Limites Non Lineaires, Dunod, Paris, 1969. |
[29] |
J. H. Xu, T. Caraballo, Long time behavior of stochastic nonlocal partial differential equations and Wong-Zakai approximations, SIAM J. Math. Anal., 54 (2022), 2792–2844. https://doi.org/10.1137/21M1412645 doi: 10.1137/21M1412645
![]() |
Variable | Description |
fail | Counter for fails |
capacity | List storing the capacities of all nodes after each simulation |
max_simulations | Maximum number of simulations to run |
all_nodes_capacity | Sum of capacities for all nodes in the current simulation |
solution | Set of nodes selected to be established |
dict_type | Dictionary indicating the type of each node |
StochasticValue | Pre-determined function to generate stochastic using log-normal function |
simulate_blackbox | Function to calculate probability value based on inputs |
operation_disruption | Input parameter for the fit_blackbox function |
seasonal_demand | Input parameter for the fit_blackbox function |
probValue | Probability value obtained from fit_blackbox |
white_box | Data structure to store intermediate results for nodes not of type 1 |
node_capacity | Capacity of the current node |
max_demand | Maximum demand that all nodes' capacity must meet |
reliability | Reliability metric for the solution |
non-static component | stochastic component | ||||
β0 | β1 | β2 | β3 | σ | |
High | -1.5 | 0.005 | 2.0 | 1.0 | 10.0 |
Low | -0.7 | 0.010 | 0.8 | 1.0 | 5.0 |
Instance | Deterministic Sol. | Solution to Stochastic Problem | Solution to Non-Static Problem | Solution to Stochastic- Non-Static Problem | Gaps% | |||||||||||
Deterministic Sol. in Stochastic Env. | Reliability | MSBR-Sim Sol. (a) | Reliability | Deterministic Sol. in Non-Static Env. | Reliability | MSBR-ML Sol. (b) | Reliability | Deterministic Sol. in Stochastic- Non-Static Env. (c) | Reliability | MSBR-Sim-ML Sol. (d) | Reliability | (d)-(c) | (d)-(a) | (d)-(b) | ||
GKD-b_11_n50_b02_m5.txt | 147.2 | 83.5 | 0.56 | 142.5 | 1.00 | 76.5 | 0.90 | 111.3 | 0.79 | 86.3 | 0.59 | 143.4 | 0.99 | 39.8 | 0.6 | 22.4 |
GKD-b_12_n50_b02_m5.txt | 178.1 | 144.1 | 0.81 | 170.4 | 1.00 | 45.1 | 0.25 | 168.8 | 1.00 | 84.4 | 0.47 | 166.8 | 0.99 | 49.4 | -2.2 | -1.2 |
GKD-b_13_n50_b02_m5.txt | 96.1 | 58.4 | 0.61 | 91.7 | 1.00 | 84.6 | 0.96 | 92.4 | 0.96 | 61.8 | 0.64 | 89.2 | 0.97 | 30.7 | -2.9 | -3.7 |
GKD-b_14_n50_b02_m5.txt | 84.6 | 64.3 | 0.76 | 81.2 | 1.00 | 28.3 | 0.34 | 69.3 | 0.94 | 59.3 | 0.70 | 79.6 | 0.99 | 25.5 | -2.0 | 13.0 |
GKD-b_15_n50_b02_m5.txt | 154.9 | 111.4 | 0.72 | 147.3 | 1.00 | 57.2 | 0.37 | 121.7 | 0.83 | 58.1 | 0.38 | 143.7 | 0.98 | 59.6 | -2.5 | 15.3 |
GKD-b_16_n50_b02_m15.txt | 77.7 | 55.2 | 0.71 | 76.1 | 0.98 | 40.8 | 0.53 | 67.0 | 0.95 | 46.1 | 0.59 | 60.8 | 0.87 | 24.2 | -25.3 | -10.2 |
GKD-b_17_n50_b02_m15.txt | 41.8 | 21.6 | 0.52 | 35.8 | 0.98 | 23.2 | 0.56 | 33.8 | 0.98 | 13.5 | 0.32 | 26.9 | 0.76 | 50.0 | -33.0 | -25.5 |
GKD-b_18_n50_b02_m15.txt | 108.5 | 69.0 | 0.64 | 104.0 | 1.00 | 99.8 | 0.92 | 103.4 | 1.00 | 69.1 | 0.64 | 96.7 | 0.99 | 28.5 | -7.5 | -6.9 |
GKD-b_19_n50_b02_m15.txt | 119.1 | 112.0 | 0.96 | 114.6 | 1.00 | 64.3 | 0.54 | 108.6 | 0.97 | 87.4 | 0.73 | 110.6 | 0.99 | 21.0 | -3.6 | 1.8 |
GKD-b_20_n50_b02_m15.txt | 115.3 | 58.1 | 0.50 | 107.4 | 1.00 | 105.8 | 0.92 | 106.0 | 1.00 | 59.6 | 0.52 | 104.9 | 0.97 | 43.2 | -2.4 | -1.0 |
GKD-b_41_n150_b02_m15.txt | 163.9 | 92.1 | 0.56 | 156.2 | 1.00 | 87.9 | 0.54 | 152.8 | 1.00 | 93.6 | 0.58 | 152.8 | 1.00 | 38.8 | -2.2 | 0.0 |
GKD-b_42_n150_b02_m15.txt | 84.0 | 66.3 | 0.79 | 74.0 | 0.97 | 7.1 | 0.08 | 75.8 | 1.00 | 19.7 | 0.24 | 79.9 | 1.00 | 75.3 | 7.4 | 5.1 |
GKD-b_43_n150_b02_m15.txt | 62.5 | 39.5 | 0.63 | 57.2 | 0.99 | 6.4 | 0.10 | 58.2 | 1.00 | 12.6 | 0.20 | 54.7 | 1.00 | 76.9 | -4.6 | -6.5 |
GKD-b_44_n150_b02_m15.txt | 100.6 | 63.8 | 0.63 | 95.4 | 0.99 | 0.1 | 0.00 | 91.7 | 1.00 | 5.0 | 0.10 | 95.9 | 1.00 | 94.8 | 0.5 | 4.4 |
GKD-b_45_n150_b02_m15.txt | 104.4 | 59.0 | 0.57 | 99.4 | 1.00 | 1.3 | 0.01 | 89.6 | 0.91 | 17.6 | 0.17 | 94.4 | 0.95 | 81.3 | -5.3 | 5.1 |
GKD-b_46_n150_b02_m45.txt | 123.3 | 69.3 | 0.56 | 116.6 | 0.99 | 62.6 | 0.51 | 111.7 | 1.00 | 55.9 | 0.45 | 115.7 | 1.00 | 51.7 | -1.0 | 3.5 |
GKD-b_47_n150_b02_m45.txt | 162.8 | 112.3 | 0.69 | 150.0 | 0.99 | 100.3 | 0.62 | 148.5 | 1.00 | 95.1 | 0.58 | 153.8 | 1.00 | 38.2 | 2.5 | 3.4 |
GKD-b_48_n150_b02_m45.txt | 99.8 | 70.1 | 0.70 | 94.3 | 0.97 | 4.0 | 0.04 | 86.1 | 0.99 | 17.7 | 0.18 | 93.1 | 1.00 | 81.0 | -1.2 | 7.5 |
GKD-b_49_n150_b02_m45.txt | 166.3 | 113.6 | 0.68 | 165.4 | 1.00 | 85.0 | 0.51 | 158.7 | 0.99 | 107.1 | 0.64 | 152.1 | 0.94 | 29.6 | -8.7 | -4.3 |
GKD-b_50_n150_b02_m45.txt | 110.1 | 65.7 | 0.60 | 105.4 | 0.99 | 90.8 | 0.83 | 100.9 | 0.96 | 65.0 | 0.59 | 98.8 | 0.94 | 34.3 | -6.7 | -2.1 |
MDG-b_01_n500_b02_m50.txt | 52.0 | 31.0 | 0.60 | 34.0 | 0.99 | 0.0 | 0.00 | 23.0 | 0.96 | 1.2 | 0.02 | 23.1 | 0.97 | 94.8 | -47.1 | 0.2 |
MDG-b_02_n500_b02_m50.txt | 43.6 | 25.4 | 0.58 | 25.9 | 1.00 | 4.1 | 0.09 | 29.2 | 0.97 | 14.7 | 0.34 | 26.4 | 1.00 | 44.2 | 1.9 | -10.6 |
MDG-b_03_n500_b02_m50.txt | 43.6 | 24.5 | 0.56 | 29.7 | 0.96 | 0.0 | 0.00 | 26.4 | 0.98 | 1.1 | 0.03 | 29.7 | 0.99 | 96.2 | 0.1 | 11.3 |
MDG-b_04_n500_b02_m50.txt | 44.5 | 29.3 | 0.71 | 31.6 | 1.00 | 0.0 | 0.00 | 24.0 | 1.00 | 3.6 | 0.08 | 21.4 | 0.63 | 83.3 | -47.7 | -12.4 |
MDG-b_05_n500_b02_m50.txt | 46.5 | 25.1 | 0.69 | 32.0 | 0.96 | 0.1 | 0.00 | 23.4 | 1.00 | 6.2 | 0.13 | 26.5 | 1.00 | 76.5 | -20.7 | 11.7 |
MDG-b_06_n500_b02_m50.txt | 45.3 | 28.7 | 0.63 | 35.0 | 1.00 | 7.5 | 0.17 | 33.2 | 0.98 | 20.6 | 0.46 | 30.3 | 0.99 | 32.0 | -15.7 | -9.7 |
MDG-b_07_n500_b02_m50.txt | 42.6 | 24.8 | 0.58 | 34.6 | 0.99 | 0.0 | 0.00 | 21.0 | 0.86 | 13.7 | 0.32 | 24.5 | 1.00 | 44.0 | -41.2 | 14.4 |
MDG-b_08_n500_b02_m50.txt | 48.0 | 29.6 | 0.80 | 38.4 | 0.99 | 23.9 | 0.50 | 41.3 | 1.00 | 23.9 | 0.70 | 33.6 | 0.93 | 28.8 | -14.6 | -23.1 |
MDG-b_09_n500_b02_m50.txt | 49.4 | 37.6 | 0.76 | 38.3 | 1.00 | 15.4 | 0.31 | 32.9 | 0.98 | 26.3 | 0.53 | 26.5 | 1.00 | 0.8 | -44.5 | -24.3 |
MDG-b_10_n500_b02_m50.txt | 50.9 | 34.5 | 0.68 | 37.5 | 1.00 | 0.0 | 0.00 | 28.7 | 1.00 | 2.2 | 0.04 | 27.1 | 0.98 | 91.9 | -38.3 | -5.7 |
GKD-c_01_n500_b02_m50.txt | 9.1 | 6.9 | 0.76 | 8.4 | 1.00 | 1.9 | 0.21 | 8.5 | 0.98 | 4.9 | 0.54 | 8.6 | 1.00 | 43.4 | 2.4 | 0.9 |
GKD-c_02_n500_b02_m50.txt | 9.3 | 6.0 | 0.64 | 9.0 | 0.97 | 1.9 | 0.20 | 4.6 | 0.52 | 3.9 | 0.42 | 8.3 | 0.97 | 52.5 | -8.8 | 44.1 |
GKD-c_03_n500_b02_m50.txt | 9.2 | 7.0 | 0.76 | 8.6 | 0.99 | 3.4 | 0.37 | 8.5 | 1.00 | 5.6 | 0.61 | 8.3 | 0.99 | 32.4 | -3.4 | -2.1 |
GKD-c_04_n500_b02_m50.txt | 9.0 | 5.1 | 0.57 | 8.6 | 0.99 | 1.4 | 0.15 | 8.4 | 1.00 | 2.8 | 0.31 | 8.4 | 0.98 | 66.8 | -2.3 | 0.3 |
GKD-c_05_n500_b02_m50.txt | 9.0 | 7.3 | 0.81 | 8.8 | 0.98 | 4.0 | 0.44 | 8.4 | 1.00 | 6.2 | 0.69 | 8.5 | 1.00 | 27.3 | -3.7 | 1.2 |
GKD-c_06_n500_b02_m50.txt | 9.0 | 6.1 | 0.67 | 8.7 | 0.99 | 1.8 | 0.20 | 7.5 | 0.90 | 4.2 | 0.46 | 8.5 | 0.99 | 51.0 | -2.1 | 11.9 |
GKD-c_07_n500_b02_m50.txt | 9.1 | 8.0 | 0.88 | 8.7 | 1.00 | 4.1 | 0.46 | 8.3 | 0.97 | 6.3 | 0.70 | 8.6 | 0.99 | 26.4 | -0.8 | 3.5 |
GKD-c_08_n500_b02_m50.txt | 9.3 | 6.7 | 0.72 | 8.9 | 1.00 | 4.6 | 0.49 | 8.6 | 1.00 | 5.8 | 0.62 | 8.9 | 1.00 | 35.0 | 0.0 | 3.4 |
GKD-c_09_n500_b02_m50.txt | 9.0 | 7.0 | 0.78 | 8.7 | 1.00 | 2.8 | 0.31 | 0.6 | 0.35 | 5.9 | 0.66 | 8.7 | 0.99 | 31.9 | 0.2 | 92.9 |
GKD-c_10_n500_b02_m50.txt | 9.1 | 6.8 | 0.75 | 8.8 | 1.00 | 0.0 | 0.00 | 8.4 | 0.97 | 1.2 | 0.13 | 8.7 | 1.00 | 86.6 | -1.2 | 3.0 |
Average | 71.5 | 47.2 | 0.68 | 65.2 | 0.99 | 28.7 | 0.34 | 60.3 | 0.94 | 31.9 | 0.43 | 61.7 | 0.97 | 50.5 | -9.7 | 3.3 |
Instance | Deterministic Sol. | Solution to Stochastic Problem | Solution to Non-Static Problem | Solution to Stochastic- Non-Static Problem | Gaps% | |||||||||||
Deterministic Sol. in Stochastic Env. | Reliability | MSBR-Sim Sol. (a) | Reliability | Deterministic Sol. in Non-Static Env. | Reliability | MSBR-ML Sol. (b) | Reliability | Deterministic Sol. in Stochastic- Non-Static Env. (c) | Reliability | MSBR-Sim-ML Sol. (d) | Reliability | (d)-(c) | (d)-(a) | (d)-(b) | ||
GKD-b_11_n50_b02_m5.txt | 147.2 | 83.5 | 0.57 | 142.8 | 1.00 | 140.0 | 0.95 | 142.9 | 1.00 | 89.4 | 0.61 | 137.3 | 1.00 | 34.9 | -4.0 | -4.1 |
GKD-b_12_n50_b02_m5.txt | 178.1 | 144.1 | 0.81 | 170.4 | 1.00 | 153.2 | 0.86 | 171.4 | 1.00 | 146.9 | 0.83 | 166.1 | 1.00 | 11.6 | -2.6 | -3.2 |
GKD-b_13_n50_b02_m5.txt | 96.1 | 58.4 | 0.61 | 91.9 | 1.00 | 65.2 | 1.00 | 92.2 | 1.00 | 62.1 | 0.65 | 92.2 | 1.00 | 32.7 | 0.3 | 0.0 |
GKD-b_14_n50_b02_m5.txt | 84.6 | 64.3 | 0.76 | 81.2 | 1.00 | 75.2 | 0.89 | 80.6 | 1.00 | 70.4 | 0.83 | 81.2 | 1.00 | 13.3 | 0.0 | 0.7 |
GKD-b_15_n50_b02_m5.txt | 154.9 | 111.4 | 0.72 | 147.3 | 1.00 | 143.6 | 0.93 | 150.0 | 0.98 | 114.0 | 0.74 | 148.7 | 0.99 | 23.3 | 0.9 | -0.9 |
GKD-b_16_n50_b02_m15.txt | 77.7 | 55.2 | 0.71 | 76.1 | 0.98 | 66.6 | 0.97 | 74.9 | 1.00 | 65.5 | 0.84 | 71.3 | 0.94 | 8.1 | -6.9 | -5.1 |
GKD-b_17_n50_b02_m15.txt | 41.8 | 21.6 | 0.52 | 35.8 | 0.98 | 26.2 | 0.97 | 39.8 | 0.98 | 22.3 | 0.53 | 34.6 | 1.00 | 35.5 | -3.4 | -15.0 |
GKD-b_18_n50_b02_m15.txt | 108.5 | 69.0 | 0.64 | 103.9 | 1.00 | 75.1 | 0.99 | 104.3 | 1.00 | 71.3 | 0.66 | 103.7 | 0.99 | 31.2 | -0.2 | -0.6 |
GKD-b_19_n50_b02_m15.txt | 119.1 | 114.6 | 0.96 | 115.3 | 1.00 | 115.2 | 0.97 | 115.3 | 0.96 | 110.2 | 0.98 | 111.6 | 1.00 | 1.2 | -3.3 | -3.3 |
GKD-b_20_n50_b02_m15.txt | 115.3 | 58.1 | 0.50 | 107.3 | 1.00 | 60.1 | 1.00 | 111.3 | 1.00 | 61.3 | 0.53 | 111.8 | 1.00 | 45.1 | 4.0 | 0.5 |
GKD-b_41_n150_b02_m15.txt | 163.9 | 92.1 | 0.56 | 156.2 | 1.00 | 154.0 | 0.95 | 156.0 | 0.99 | 106.1 | 0.65 | 154.4 | 0.99 | 31.3 | -1.1 | -1.0 |
GKD-b_42_n150_b02_m15.txt | 84.0 | 66.3 | 0.79 | 74.0 | 0.97 | 75.5 | 0.90 | 76.3 | 1.00 | 71.7 | 0.85 | 79.0 | 1.00 | 9.2 | 6.3 | 3.4 |
GKD-b_43_n150_b02_m15.txt | 62.5 | 39.5 | 0.63 | 57.2 | 0.99 | 57.4 | 0.92 | 59.8 | 0.97 | 40.9 | 0.66 | 58.5 | 0.99 | 30.0 | 2.2 | -2.3 |
GKD-b_44_n150_b02_m15.txt | 100.6 | 63.8 | 0.63 | 95.4 | 0.99 | 55.3 | 0.55 | 98.9 | 1.00 | 51.7 | 0.51 | 95.6 | 0.99 | 45.9 | 0.2 | -3.4 |
GKD-b_45_n150_b02_m15.txt | 104.4 | 59.0 | 0.57 | 99.4 | 1.00 | 66.3 | 0.64 | 102.4 | 1.00 | 56.2 | 0.54 | 101.5 | 0.98 | 44.7 | 2.1 | -0.9 |
GKD-b_46_n150_b02_m45.txt | 123.3 | 69.3 | 0.56 | 116.6 | 0.99 | 90.1 | 0.73 | 114.3 | 0.97 | 65.1 | 0.53 | 115.4 | 1.00 | 43.6 | -1.1 | 0.9 |
GKD-b_47_n150_b02_m45.txt | 162.8 | 112.3 | 0.69 | 150.0 | 0.99 | 144.1 | 0.89 | 155.7 | 1.00 | 118.4 | 0.73 | 157.0 | 0.99 | 24.6 | 4.5 | 0.8 |
GKD-b_48_n150_b02_m45.txt | 99.8 | 70.1 | 0.70 | 94.3 | 0.97 | 72.8 | 0.73 | 96.1 | 1.00 | 63.9 | 0.64 | 93.6 | 1.00 | 31.8 | -0.7 | -2.7 |
GKD-b_49_n150_b02_m45.txt | 166.3 | 113.6 | 0.68 | 165.4 | 1.00 | 139.0 | 0.84 | 161.8 | 1.00 | 123.4 | 0.74 | 163.4 | 1.00 | 24.5 | -1.2 | 1.0 |
GKD-b_50_n150_b02_m45.txt | 110.1 | 65.7 | 0.60 | 105.4 | 0.99 | 68.1 | 0.97 | 106.2 | 1.00 | 69.0 | 0.63 | 106.5 | 1.00 | 35.2 | 1.0 | 0.3 |
MDG-b_01_n500_b02_m50.txt | 52.0 | 31.0 | 0.60 | 34.0 | 0.99 | 12.5 | 0.24 | 33.2 | 0.95 | 23.9 | 0.46 | 39.4 | 0.95 | 39.3 | 13.9 | 15.9 |
MDG-b_02_n500_b02_m50.txt | 43.6 | 25.4 | 0.58 | 25.9 | 1.00 | 15.8 | 0.36 | 32.5 | 0.99 | 23.2 | 0.53 | 26.2 | 0.98 | 11.2 | 1.0 | -24.1 |
MDG-b_03_n500_b02_m50.txt | 43.6 | 24.5 | 0.56 | 29.7 | 0.96 | 9.5 | 0.22 | 25.5 | 0.96 | 21.8 | 0.50 | 31.9 | 1.00 | 31.7 | 7.0 | 20.0 |
MDG-b_04_n500_b02_m50.txt | 44.5 | 33.2 | 0.73 | 35.3 | 1.00 | 24.0 | 0.54 | 40.4 | 1.00 | 22.4 | 0.76 | 24.9 | 1.00 | 10.0 | -41.8 | -62.3 |
MDG-b_05_n500_b02_m50.txt | 46.5 | 32.0 | 0.69 | 33.1 | 1.00 | 31.6 | 0.68 | 31.7 | 1.00 | 32.4 | 0.70 | 32.6 | 1.00 | 0.6 | -1.5 | 2.8 |
MDG-b_06_n500_b02_m50.txt | 45.3 | 32.3 | 0.71 | 35.0 | 0.99 | 27.2 | 0.60 | 29.8 | 0.99 | 27.1 | 0.71 | 27.4 | 0.99 | 1.2 | -27.7 | -8.6 |
MDG-b_07_n500_b02_m50.txt | 42.6 | 24.8 | 0.58 | 34.6 | 1.00 | 19.4 | 0.46 | 26.0 | 1.00 | 23.8 | 0.53 | 28.0 | 0.99 | 15.0 | -23.8 | 7.0 |
MDG-b_08_n500_b02_m50.txt | 48.0 | 38.4 | 0.80 | 44.5 | 1.00 | 38.0 | 0.95 | 44.5 | 1.00 | 39.0 | 0.81 | 40.3 | 1.00 | 3.3 | -10.4 | -10.4 |
MDG-b_09_n500_b02_m50.txt | 49.4 | 37.6 | 0.76 | 38.3 | 1.00 | 30.1 | 0.91 | 32.7 | 1.00 | 27.2 | 0.75 | 29.5 | 0.99 | 7.8 | -29.8 | -10.8 |
MDG-b_10_n500_b02_m50.txt | 50.9 | 34.5 | 0.68 | 37.5 | 0.96 | 17.3 | 0.34 | 34.3 | 1.00 | 31.7 | 0.62 | 38.9 | 0.98 | 18.5 | 3.5 | 11.8 |
GKD-c_01_n500_b02_m50.txt | 9.1 | 6.9 | 0.76 | 8.5 | 0.97 | 6.6 | 0.73 | 8.5 | 0.99 | 6.8 | 0.74 | 8.8 | 0.99 | 23.3 | 4.0 | 3.1 |
GKD-c_02_n500_b02_m50.txt | 9.3 | 5.6 | 0.61 | 9.0 | 0.99 | 6.1 | 0.66 | 8.7 | 0.99 | 5.6 | 0.60 | 9.0 | 1.00 | 38.1 | -0.1 | 3.2 |
GKD-c_03_n500_b02_m50.txt | 9.2 | 7.0 | 0.76 | 8.6 | 0.99 | 8.5 | 0.92 | 8.7 | 0.99 | 7.1 | 0.77 | 8.5 | 0.97 | 16.0 | -1.8 | -2.9 |
GKD-c_04_n500_b02_m50.txt | 9.0 | 5.1 | 0.57 | 8.6 | 0.98 | 4.0 | 0.44 | 8.8 | 1.00 | 4.4 | 0.48 | 8.7 | 0.99 | 50.2 | 1.4 | -0.6 |
GKD-c_05_n500_b02_m50.txt | 9.0 | 7.3 | 0.81 | 8.8 | 0.99 | 7.6 | 0.96 | 8.5 | 1.00 | 7.8 | 0.87 | 8.6 | 0.99 | 9.1 | -2.3 | 1.3 |
GKD-c_06_n500_b02_m50.txt | 9.0 | 6.0 | 0.67 | 8.7 | 1.00 | 5.8 | 0.65 | 8.7 | 0.99 | 5.8 | 0.65 | 8.6 | 1.00 | 32.4 | -1.0 | -1.3 |
GKD-c_07_n500_b02_m50.txt | 9.1 | 5.9 | 0.66 | 8.7 | 0.99 | 8.0 | 0.95 | 8.6 | 1.00 | 8.1 | 0.89 | 8.4 | 0.99 | 4.1 | -2.6 | -1.8 |
GKD-c_08_n500_b02_m50.txt | 9.3 | 6.7 | 0.72 | 8.9 | 1.00 | 8.7 | 0.94 | 9.0 | 1.00 | 6.8 | 0.73 | 8.8 | 1.00 | 22.5 | -1.3 | -2.5 |
GKD-c_09_n500_b02_m50.txt | 9.0 | 4.7 | 0.52 | 8.7 | 1.00 | 7.8 | 0.87 | 8.7 | 1.00 | 7.6 | 0.84 | 8.7 | 1.00 | 12.4 | -0.2 | -0.3 |
GKD-c_10_n500_b02_m50.txt | 9.1 | 6.8 | 0.75 | 8.8 | 1.00 | 7.3 | 0.81 | 8.6 | 0.98 | 6.5 | 0.72 | 8.7 | 1.00 | 24.9 | -1.2 | 0.9 |
Average | 71.5 | 47.7 | 0.67 | 65.5 | 0.99 | 53.5 | 0.77 | 65.7 | 0.99 | 48.0 | 0.68 | 64.7 | 0.99 | 23.2 | -2.9 | -2.4 |
Variable | Description |
fail | Counter for fails |
capacity | List storing the capacities of all nodes after each simulation |
max_simulations | Maximum number of simulations to run |
all_nodes_capacity | Sum of capacities for all nodes in the current simulation |
solution | Set of nodes selected to be established |
dict_type | Dictionary indicating the type of each node |
StochasticValue | Pre-determined function to generate stochastic using log-normal function |
simulate_blackbox | Function to calculate probability value based on inputs |
operation_disruption | Input parameter for the fit_blackbox function |
seasonal_demand | Input parameter for the fit_blackbox function |
probValue | Probability value obtained from fit_blackbox |
white_box | Data structure to store intermediate results for nodes not of type 1 |
node_capacity | Capacity of the current node |
max_demand | Maximum demand that all nodes' capacity must meet |
reliability | Reliability metric for the solution |
non-static component | stochastic component | ||||
β0 | β1 | β2 | β3 | σ | |
High | -1.5 | 0.005 | 2.0 | 1.0 | 10.0 |
Low | -0.7 | 0.010 | 0.8 | 1.0 | 5.0 |
Instance | Deterministic Sol. | Solution to Stochastic Problem | Solution to Non-Static Problem | Solution to Stochastic- Non-Static Problem | Gaps% | |||||||||||
Deterministic Sol. in Stochastic Env. | Reliability | MSBR-Sim Sol. (a) | Reliability | Deterministic Sol. in Non-Static Env. | Reliability | MSBR-ML Sol. (b) | Reliability | Deterministic Sol. in Stochastic- Non-Static Env. (c) | Reliability | MSBR-Sim-ML Sol. (d) | Reliability | (d)-(c) | (d)-(a) | (d)-(b) | ||
GKD-b_11_n50_b02_m5.txt | 147.2 | 83.5 | 0.56 | 142.5 | 1.00 | 76.5 | 0.90 | 111.3 | 0.79 | 86.3 | 0.59 | 143.4 | 0.99 | 39.8 | 0.6 | 22.4 |
GKD-b_12_n50_b02_m5.txt | 178.1 | 144.1 | 0.81 | 170.4 | 1.00 | 45.1 | 0.25 | 168.8 | 1.00 | 84.4 | 0.47 | 166.8 | 0.99 | 49.4 | -2.2 | -1.2 |
GKD-b_13_n50_b02_m5.txt | 96.1 | 58.4 | 0.61 | 91.7 | 1.00 | 84.6 | 0.96 | 92.4 | 0.96 | 61.8 | 0.64 | 89.2 | 0.97 | 30.7 | -2.9 | -3.7 |
GKD-b_14_n50_b02_m5.txt | 84.6 | 64.3 | 0.76 | 81.2 | 1.00 | 28.3 | 0.34 | 69.3 | 0.94 | 59.3 | 0.70 | 79.6 | 0.99 | 25.5 | -2.0 | 13.0 |
GKD-b_15_n50_b02_m5.txt | 154.9 | 111.4 | 0.72 | 147.3 | 1.00 | 57.2 | 0.37 | 121.7 | 0.83 | 58.1 | 0.38 | 143.7 | 0.98 | 59.6 | -2.5 | 15.3 |
GKD-b_16_n50_b02_m15.txt | 77.7 | 55.2 | 0.71 | 76.1 | 0.98 | 40.8 | 0.53 | 67.0 | 0.95 | 46.1 | 0.59 | 60.8 | 0.87 | 24.2 | -25.3 | -10.2 |
GKD-b_17_n50_b02_m15.txt | 41.8 | 21.6 | 0.52 | 35.8 | 0.98 | 23.2 | 0.56 | 33.8 | 0.98 | 13.5 | 0.32 | 26.9 | 0.76 | 50.0 | -33.0 | -25.5 |
GKD-b_18_n50_b02_m15.txt | 108.5 | 69.0 | 0.64 | 104.0 | 1.00 | 99.8 | 0.92 | 103.4 | 1.00 | 69.1 | 0.64 | 96.7 | 0.99 | 28.5 | -7.5 | -6.9 |
GKD-b_19_n50_b02_m15.txt | 119.1 | 112.0 | 0.96 | 114.6 | 1.00 | 64.3 | 0.54 | 108.6 | 0.97 | 87.4 | 0.73 | 110.6 | 0.99 | 21.0 | -3.6 | 1.8 |
GKD-b_20_n50_b02_m15.txt | 115.3 | 58.1 | 0.50 | 107.4 | 1.00 | 105.8 | 0.92 | 106.0 | 1.00 | 59.6 | 0.52 | 104.9 | 0.97 | 43.2 | -2.4 | -1.0 |
GKD-b_41_n150_b02_m15.txt | 163.9 | 92.1 | 0.56 | 156.2 | 1.00 | 87.9 | 0.54 | 152.8 | 1.00 | 93.6 | 0.58 | 152.8 | 1.00 | 38.8 | -2.2 | 0.0 |
GKD-b_42_n150_b02_m15.txt | 84.0 | 66.3 | 0.79 | 74.0 | 0.97 | 7.1 | 0.08 | 75.8 | 1.00 | 19.7 | 0.24 | 79.9 | 1.00 | 75.3 | 7.4 | 5.1 |
GKD-b_43_n150_b02_m15.txt | 62.5 | 39.5 | 0.63 | 57.2 | 0.99 | 6.4 | 0.10 | 58.2 | 1.00 | 12.6 | 0.20 | 54.7 | 1.00 | 76.9 | -4.6 | -6.5 |
GKD-b_44_n150_b02_m15.txt | 100.6 | 63.8 | 0.63 | 95.4 | 0.99 | 0.1 | 0.00 | 91.7 | 1.00 | 5.0 | 0.10 | 95.9 | 1.00 | 94.8 | 0.5 | 4.4 |
GKD-b_45_n150_b02_m15.txt | 104.4 | 59.0 | 0.57 | 99.4 | 1.00 | 1.3 | 0.01 | 89.6 | 0.91 | 17.6 | 0.17 | 94.4 | 0.95 | 81.3 | -5.3 | 5.1 |
GKD-b_46_n150_b02_m45.txt | 123.3 | 69.3 | 0.56 | 116.6 | 0.99 | 62.6 | 0.51 | 111.7 | 1.00 | 55.9 | 0.45 | 115.7 | 1.00 | 51.7 | -1.0 | 3.5 |
GKD-b_47_n150_b02_m45.txt | 162.8 | 112.3 | 0.69 | 150.0 | 0.99 | 100.3 | 0.62 | 148.5 | 1.00 | 95.1 | 0.58 | 153.8 | 1.00 | 38.2 | 2.5 | 3.4 |
GKD-b_48_n150_b02_m45.txt | 99.8 | 70.1 | 0.70 | 94.3 | 0.97 | 4.0 | 0.04 | 86.1 | 0.99 | 17.7 | 0.18 | 93.1 | 1.00 | 81.0 | -1.2 | 7.5 |
GKD-b_49_n150_b02_m45.txt | 166.3 | 113.6 | 0.68 | 165.4 | 1.00 | 85.0 | 0.51 | 158.7 | 0.99 | 107.1 | 0.64 | 152.1 | 0.94 | 29.6 | -8.7 | -4.3 |
GKD-b_50_n150_b02_m45.txt | 110.1 | 65.7 | 0.60 | 105.4 | 0.99 | 90.8 | 0.83 | 100.9 | 0.96 | 65.0 | 0.59 | 98.8 | 0.94 | 34.3 | -6.7 | -2.1 |
MDG-b_01_n500_b02_m50.txt | 52.0 | 31.0 | 0.60 | 34.0 | 0.99 | 0.0 | 0.00 | 23.0 | 0.96 | 1.2 | 0.02 | 23.1 | 0.97 | 94.8 | -47.1 | 0.2 |
MDG-b_02_n500_b02_m50.txt | 43.6 | 25.4 | 0.58 | 25.9 | 1.00 | 4.1 | 0.09 | 29.2 | 0.97 | 14.7 | 0.34 | 26.4 | 1.00 | 44.2 | 1.9 | -10.6 |
MDG-b_03_n500_b02_m50.txt | 43.6 | 24.5 | 0.56 | 29.7 | 0.96 | 0.0 | 0.00 | 26.4 | 0.98 | 1.1 | 0.03 | 29.7 | 0.99 | 96.2 | 0.1 | 11.3 |
MDG-b_04_n500_b02_m50.txt | 44.5 | 29.3 | 0.71 | 31.6 | 1.00 | 0.0 | 0.00 | 24.0 | 1.00 | 3.6 | 0.08 | 21.4 | 0.63 | 83.3 | -47.7 | -12.4 |
MDG-b_05_n500_b02_m50.txt | 46.5 | 25.1 | 0.69 | 32.0 | 0.96 | 0.1 | 0.00 | 23.4 | 1.00 | 6.2 | 0.13 | 26.5 | 1.00 | 76.5 | -20.7 | 11.7 |
MDG-b_06_n500_b02_m50.txt | 45.3 | 28.7 | 0.63 | 35.0 | 1.00 | 7.5 | 0.17 | 33.2 | 0.98 | 20.6 | 0.46 | 30.3 | 0.99 | 32.0 | -15.7 | -9.7 |
MDG-b_07_n500_b02_m50.txt | 42.6 | 24.8 | 0.58 | 34.6 | 0.99 | 0.0 | 0.00 | 21.0 | 0.86 | 13.7 | 0.32 | 24.5 | 1.00 | 44.0 | -41.2 | 14.4 |
MDG-b_08_n500_b02_m50.txt | 48.0 | 29.6 | 0.80 | 38.4 | 0.99 | 23.9 | 0.50 | 41.3 | 1.00 | 23.9 | 0.70 | 33.6 | 0.93 | 28.8 | -14.6 | -23.1 |
MDG-b_09_n500_b02_m50.txt | 49.4 | 37.6 | 0.76 | 38.3 | 1.00 | 15.4 | 0.31 | 32.9 | 0.98 | 26.3 | 0.53 | 26.5 | 1.00 | 0.8 | -44.5 | -24.3 |
MDG-b_10_n500_b02_m50.txt | 50.9 | 34.5 | 0.68 | 37.5 | 1.00 | 0.0 | 0.00 | 28.7 | 1.00 | 2.2 | 0.04 | 27.1 | 0.98 | 91.9 | -38.3 | -5.7 |
GKD-c_01_n500_b02_m50.txt | 9.1 | 6.9 | 0.76 | 8.4 | 1.00 | 1.9 | 0.21 | 8.5 | 0.98 | 4.9 | 0.54 | 8.6 | 1.00 | 43.4 | 2.4 | 0.9 |
GKD-c_02_n500_b02_m50.txt | 9.3 | 6.0 | 0.64 | 9.0 | 0.97 | 1.9 | 0.20 | 4.6 | 0.52 | 3.9 | 0.42 | 8.3 | 0.97 | 52.5 | -8.8 | 44.1 |
GKD-c_03_n500_b02_m50.txt | 9.2 | 7.0 | 0.76 | 8.6 | 0.99 | 3.4 | 0.37 | 8.5 | 1.00 | 5.6 | 0.61 | 8.3 | 0.99 | 32.4 | -3.4 | -2.1 |
GKD-c_04_n500_b02_m50.txt | 9.0 | 5.1 | 0.57 | 8.6 | 0.99 | 1.4 | 0.15 | 8.4 | 1.00 | 2.8 | 0.31 | 8.4 | 0.98 | 66.8 | -2.3 | 0.3 |
GKD-c_05_n500_b02_m50.txt | 9.0 | 7.3 | 0.81 | 8.8 | 0.98 | 4.0 | 0.44 | 8.4 | 1.00 | 6.2 | 0.69 | 8.5 | 1.00 | 27.3 | -3.7 | 1.2 |
GKD-c_06_n500_b02_m50.txt | 9.0 | 6.1 | 0.67 | 8.7 | 0.99 | 1.8 | 0.20 | 7.5 | 0.90 | 4.2 | 0.46 | 8.5 | 0.99 | 51.0 | -2.1 | 11.9 |
GKD-c_07_n500_b02_m50.txt | 9.1 | 8.0 | 0.88 | 8.7 | 1.00 | 4.1 | 0.46 | 8.3 | 0.97 | 6.3 | 0.70 | 8.6 | 0.99 | 26.4 | -0.8 | 3.5 |
GKD-c_08_n500_b02_m50.txt | 9.3 | 6.7 | 0.72 | 8.9 | 1.00 | 4.6 | 0.49 | 8.6 | 1.00 | 5.8 | 0.62 | 8.9 | 1.00 | 35.0 | 0.0 | 3.4 |
GKD-c_09_n500_b02_m50.txt | 9.0 | 7.0 | 0.78 | 8.7 | 1.00 | 2.8 | 0.31 | 0.6 | 0.35 | 5.9 | 0.66 | 8.7 | 0.99 | 31.9 | 0.2 | 92.9 |
GKD-c_10_n500_b02_m50.txt | 9.1 | 6.8 | 0.75 | 8.8 | 1.00 | 0.0 | 0.00 | 8.4 | 0.97 | 1.2 | 0.13 | 8.7 | 1.00 | 86.6 | -1.2 | 3.0 |
Average | 71.5 | 47.2 | 0.68 | 65.2 | 0.99 | 28.7 | 0.34 | 60.3 | 0.94 | 31.9 | 0.43 | 61.7 | 0.97 | 50.5 | -9.7 | 3.3 |
Instance | Deterministic Sol. | Solution to Stochastic Problem | Solution to Non-Static Problem | Solution to Stochastic- Non-Static Problem | Gaps% | |||||||||||
Deterministic Sol. in Stochastic Env. | Reliability | MSBR-Sim Sol. (a) | Reliability | Deterministic Sol. in Non-Static Env. | Reliability | MSBR-ML Sol. (b) | Reliability | Deterministic Sol. in Stochastic- Non-Static Env. (c) | Reliability | MSBR-Sim-ML Sol. (d) | Reliability | (d)-(c) | (d)-(a) | (d)-(b) | ||
GKD-b_11_n50_b02_m5.txt | 147.2 | 83.5 | 0.57 | 142.8 | 1.00 | 140.0 | 0.95 | 142.9 | 1.00 | 89.4 | 0.61 | 137.3 | 1.00 | 34.9 | -4.0 | -4.1 |
GKD-b_12_n50_b02_m5.txt | 178.1 | 144.1 | 0.81 | 170.4 | 1.00 | 153.2 | 0.86 | 171.4 | 1.00 | 146.9 | 0.83 | 166.1 | 1.00 | 11.6 | -2.6 | -3.2 |
GKD-b_13_n50_b02_m5.txt | 96.1 | 58.4 | 0.61 | 91.9 | 1.00 | 65.2 | 1.00 | 92.2 | 1.00 | 62.1 | 0.65 | 92.2 | 1.00 | 32.7 | 0.3 | 0.0 |
GKD-b_14_n50_b02_m5.txt | 84.6 | 64.3 | 0.76 | 81.2 | 1.00 | 75.2 | 0.89 | 80.6 | 1.00 | 70.4 | 0.83 | 81.2 | 1.00 | 13.3 | 0.0 | 0.7 |
GKD-b_15_n50_b02_m5.txt | 154.9 | 111.4 | 0.72 | 147.3 | 1.00 | 143.6 | 0.93 | 150.0 | 0.98 | 114.0 | 0.74 | 148.7 | 0.99 | 23.3 | 0.9 | -0.9 |
GKD-b_16_n50_b02_m15.txt | 77.7 | 55.2 | 0.71 | 76.1 | 0.98 | 66.6 | 0.97 | 74.9 | 1.00 | 65.5 | 0.84 | 71.3 | 0.94 | 8.1 | -6.9 | -5.1 |
GKD-b_17_n50_b02_m15.txt | 41.8 | 21.6 | 0.52 | 35.8 | 0.98 | 26.2 | 0.97 | 39.8 | 0.98 | 22.3 | 0.53 | 34.6 | 1.00 | 35.5 | -3.4 | -15.0 |
GKD-b_18_n50_b02_m15.txt | 108.5 | 69.0 | 0.64 | 103.9 | 1.00 | 75.1 | 0.99 | 104.3 | 1.00 | 71.3 | 0.66 | 103.7 | 0.99 | 31.2 | -0.2 | -0.6 |
GKD-b_19_n50_b02_m15.txt | 119.1 | 114.6 | 0.96 | 115.3 | 1.00 | 115.2 | 0.97 | 115.3 | 0.96 | 110.2 | 0.98 | 111.6 | 1.00 | 1.2 | -3.3 | -3.3 |
GKD-b_20_n50_b02_m15.txt | 115.3 | 58.1 | 0.50 | 107.3 | 1.00 | 60.1 | 1.00 | 111.3 | 1.00 | 61.3 | 0.53 | 111.8 | 1.00 | 45.1 | 4.0 | 0.5 |
GKD-b_41_n150_b02_m15.txt | 163.9 | 92.1 | 0.56 | 156.2 | 1.00 | 154.0 | 0.95 | 156.0 | 0.99 | 106.1 | 0.65 | 154.4 | 0.99 | 31.3 | -1.1 | -1.0 |
GKD-b_42_n150_b02_m15.txt | 84.0 | 66.3 | 0.79 | 74.0 | 0.97 | 75.5 | 0.90 | 76.3 | 1.00 | 71.7 | 0.85 | 79.0 | 1.00 | 9.2 | 6.3 | 3.4 |
GKD-b_43_n150_b02_m15.txt | 62.5 | 39.5 | 0.63 | 57.2 | 0.99 | 57.4 | 0.92 | 59.8 | 0.97 | 40.9 | 0.66 | 58.5 | 0.99 | 30.0 | 2.2 | -2.3 |
GKD-b_44_n150_b02_m15.txt | 100.6 | 63.8 | 0.63 | 95.4 | 0.99 | 55.3 | 0.55 | 98.9 | 1.00 | 51.7 | 0.51 | 95.6 | 0.99 | 45.9 | 0.2 | -3.4 |
GKD-b_45_n150_b02_m15.txt | 104.4 | 59.0 | 0.57 | 99.4 | 1.00 | 66.3 | 0.64 | 102.4 | 1.00 | 56.2 | 0.54 | 101.5 | 0.98 | 44.7 | 2.1 | -0.9 |
GKD-b_46_n150_b02_m45.txt | 123.3 | 69.3 | 0.56 | 116.6 | 0.99 | 90.1 | 0.73 | 114.3 | 0.97 | 65.1 | 0.53 | 115.4 | 1.00 | 43.6 | -1.1 | 0.9 |
GKD-b_47_n150_b02_m45.txt | 162.8 | 112.3 | 0.69 | 150.0 | 0.99 | 144.1 | 0.89 | 155.7 | 1.00 | 118.4 | 0.73 | 157.0 | 0.99 | 24.6 | 4.5 | 0.8 |
GKD-b_48_n150_b02_m45.txt | 99.8 | 70.1 | 0.70 | 94.3 | 0.97 | 72.8 | 0.73 | 96.1 | 1.00 | 63.9 | 0.64 | 93.6 | 1.00 | 31.8 | -0.7 | -2.7 |
GKD-b_49_n150_b02_m45.txt | 166.3 | 113.6 | 0.68 | 165.4 | 1.00 | 139.0 | 0.84 | 161.8 | 1.00 | 123.4 | 0.74 | 163.4 | 1.00 | 24.5 | -1.2 | 1.0 |
GKD-b_50_n150_b02_m45.txt | 110.1 | 65.7 | 0.60 | 105.4 | 0.99 | 68.1 | 0.97 | 106.2 | 1.00 | 69.0 | 0.63 | 106.5 | 1.00 | 35.2 | 1.0 | 0.3 |
MDG-b_01_n500_b02_m50.txt | 52.0 | 31.0 | 0.60 | 34.0 | 0.99 | 12.5 | 0.24 | 33.2 | 0.95 | 23.9 | 0.46 | 39.4 | 0.95 | 39.3 | 13.9 | 15.9 |
MDG-b_02_n500_b02_m50.txt | 43.6 | 25.4 | 0.58 | 25.9 | 1.00 | 15.8 | 0.36 | 32.5 | 0.99 | 23.2 | 0.53 | 26.2 | 0.98 | 11.2 | 1.0 | -24.1 |
MDG-b_03_n500_b02_m50.txt | 43.6 | 24.5 | 0.56 | 29.7 | 0.96 | 9.5 | 0.22 | 25.5 | 0.96 | 21.8 | 0.50 | 31.9 | 1.00 | 31.7 | 7.0 | 20.0 |
MDG-b_04_n500_b02_m50.txt | 44.5 | 33.2 | 0.73 | 35.3 | 1.00 | 24.0 | 0.54 | 40.4 | 1.00 | 22.4 | 0.76 | 24.9 | 1.00 | 10.0 | -41.8 | -62.3 |
MDG-b_05_n500_b02_m50.txt | 46.5 | 32.0 | 0.69 | 33.1 | 1.00 | 31.6 | 0.68 | 31.7 | 1.00 | 32.4 | 0.70 | 32.6 | 1.00 | 0.6 | -1.5 | 2.8 |
MDG-b_06_n500_b02_m50.txt | 45.3 | 32.3 | 0.71 | 35.0 | 0.99 | 27.2 | 0.60 | 29.8 | 0.99 | 27.1 | 0.71 | 27.4 | 0.99 | 1.2 | -27.7 | -8.6 |
MDG-b_07_n500_b02_m50.txt | 42.6 | 24.8 | 0.58 | 34.6 | 1.00 | 19.4 | 0.46 | 26.0 | 1.00 | 23.8 | 0.53 | 28.0 | 0.99 | 15.0 | -23.8 | 7.0 |
MDG-b_08_n500_b02_m50.txt | 48.0 | 38.4 | 0.80 | 44.5 | 1.00 | 38.0 | 0.95 | 44.5 | 1.00 | 39.0 | 0.81 | 40.3 | 1.00 | 3.3 | -10.4 | -10.4 |
MDG-b_09_n500_b02_m50.txt | 49.4 | 37.6 | 0.76 | 38.3 | 1.00 | 30.1 | 0.91 | 32.7 | 1.00 | 27.2 | 0.75 | 29.5 | 0.99 | 7.8 | -29.8 | -10.8 |
MDG-b_10_n500_b02_m50.txt | 50.9 | 34.5 | 0.68 | 37.5 | 0.96 | 17.3 | 0.34 | 34.3 | 1.00 | 31.7 | 0.62 | 38.9 | 0.98 | 18.5 | 3.5 | 11.8 |
GKD-c_01_n500_b02_m50.txt | 9.1 | 6.9 | 0.76 | 8.5 | 0.97 | 6.6 | 0.73 | 8.5 | 0.99 | 6.8 | 0.74 | 8.8 | 0.99 | 23.3 | 4.0 | 3.1 |
GKD-c_02_n500_b02_m50.txt | 9.3 | 5.6 | 0.61 | 9.0 | 0.99 | 6.1 | 0.66 | 8.7 | 0.99 | 5.6 | 0.60 | 9.0 | 1.00 | 38.1 | -0.1 | 3.2 |
GKD-c_03_n500_b02_m50.txt | 9.2 | 7.0 | 0.76 | 8.6 | 0.99 | 8.5 | 0.92 | 8.7 | 0.99 | 7.1 | 0.77 | 8.5 | 0.97 | 16.0 | -1.8 | -2.9 |
GKD-c_04_n500_b02_m50.txt | 9.0 | 5.1 | 0.57 | 8.6 | 0.98 | 4.0 | 0.44 | 8.8 | 1.00 | 4.4 | 0.48 | 8.7 | 0.99 | 50.2 | 1.4 | -0.6 |
GKD-c_05_n500_b02_m50.txt | 9.0 | 7.3 | 0.81 | 8.8 | 0.99 | 7.6 | 0.96 | 8.5 | 1.00 | 7.8 | 0.87 | 8.6 | 0.99 | 9.1 | -2.3 | 1.3 |
GKD-c_06_n500_b02_m50.txt | 9.0 | 6.0 | 0.67 | 8.7 | 1.00 | 5.8 | 0.65 | 8.7 | 0.99 | 5.8 | 0.65 | 8.6 | 1.00 | 32.4 | -1.0 | -1.3 |
GKD-c_07_n500_b02_m50.txt | 9.1 | 5.9 | 0.66 | 8.7 | 0.99 | 8.0 | 0.95 | 8.6 | 1.00 | 8.1 | 0.89 | 8.4 | 0.99 | 4.1 | -2.6 | -1.8 |
GKD-c_08_n500_b02_m50.txt | 9.3 | 6.7 | 0.72 | 8.9 | 1.00 | 8.7 | 0.94 | 9.0 | 1.00 | 6.8 | 0.73 | 8.8 | 1.00 | 22.5 | -1.3 | -2.5 |
GKD-c_09_n500_b02_m50.txt | 9.0 | 4.7 | 0.52 | 8.7 | 1.00 | 7.8 | 0.87 | 8.7 | 1.00 | 7.6 | 0.84 | 8.7 | 1.00 | 12.4 | -0.2 | -0.3 |
GKD-c_10_n500_b02_m50.txt | 9.1 | 6.8 | 0.75 | 8.8 | 1.00 | 7.3 | 0.81 | 8.6 | 0.98 | 6.5 | 0.72 | 8.7 | 1.00 | 24.9 | -1.2 | 0.9 |
Average | 71.5 | 47.7 | 0.67 | 65.5 | 0.99 | 53.5 | 0.77 | 65.7 | 0.99 | 48.0 | 0.68 | 64.7 | 0.99 | 23.2 | -2.9 | -2.4 |