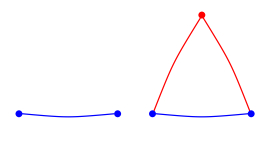
We have introduced a new continuous-time network evolution model. We have described cooperation, so we have considered the cliques of nodes. The evolution of the network was based on cliques of nodes of the network and was governed by a branching process. The basic properties of the evolution process were described. Asymptotic theorems were proved for the number of cliques having a fixed size and the degree of a fixed node. The generating function was calculated, and then the probability of extinction was obtained. For the proof, advanced results of multi-type branching processes were used. Besides precise mathematical proofs, simulation examples also supported our results.
Citation: István Fazekas, Attila Barta, László Fórián, Bettina Porvázsnyik. A continuous-time network evolution model describing N-interactions[J]. AIMS Mathematics, 2024, 9(12): 35721-35742. doi: 10.3934/math.20241695
[1] | Xiangqi Zheng . On the extinction of continuous-state branching processes in random environments. AIMS Mathematics, 2021, 6(1): 156-167. doi: 10.3934/math.2021011 |
[2] | Boubaker Smii . Markov random fields model and applications to image processing. AIMS Mathematics, 2022, 7(3): 4459-4471. doi: 10.3934/math.2022248 |
[3] | Andrey Borisov . Filtering of hidden Markov renewal processes by continuous and counting observations. AIMS Mathematics, 2024, 9(11): 30073-30099. doi: 10.3934/math.20241453 |
[4] | Min Han, Bin Pei . An averaging principle for stochastic evolution equations with jumps and random time delays. AIMS Mathematics, 2021, 6(1): 39-51. doi: 10.3934/math.2021003 |
[5] | Chahn Yong Jung, Muhammad Shoaib Saleem, Shamas Bilal, Waqas Nazeer, Mamoona Ghafoor . Some properties of η-convex stochastic processes. AIMS Mathematics, 2021, 6(1): 726-736. doi: 10.3934/math.2021044 |
[6] | Meiqin Wei, Jun Yue, Xiaoyu zhu . On the edge metric dimension of graphs. AIMS Mathematics, 2020, 5(5): 4459-4465. doi: 10.3934/math.2020286 |
[7] | Sufyan Asif, Muhammad Khalid Mahmood, Amal S. Alali, Abdullah A. Zaagan . Structures and applications of graphs arising from congruences over moduli. AIMS Mathematics, 2024, 9(8): 21786-21798. doi: 10.3934/math.20241059 |
[8] | Fan Yang, Xiaohui Ai . Exponential stability of stochastic complex networks with multi-weights driven by second-order process based on graph theory. AIMS Mathematics, 2024, 9(4): 9847-9866. doi: 10.3934/math.2024482 |
[9] | Hyun Geun Lee, Youngjin Hwang, Yunjae Nam, Sangkwon Kim, Junseok Kim . Benchmark problems for physics-informed neural networks: The Allen–Cahn equation. AIMS Mathematics, 2025, 10(3): 7319-7338. doi: 10.3934/math.2025335 |
[10] | Sakander Hayat, Hafiz Muhammad Afzal Siddiqui, Muhammad Imran, Hafiz Muhammad Ikhlaq, Jinde Cao . On the zero forcing number and propagation time of oriented graphs. AIMS Mathematics, 2021, 6(2): 1833-1850. doi: 10.3934/math.2021111 |
We have introduced a new continuous-time network evolution model. We have described cooperation, so we have considered the cliques of nodes. The evolution of the network was based on cliques of nodes of the network and was governed by a branching process. The basic properties of the evolution process were described. Asymptotic theorems were proved for the number of cliques having a fixed size and the degree of a fixed node. The generating function was calculated, and then the probability of extinction was obtained. For the proof, advanced results of multi-type branching processes were used. Besides precise mathematical proofs, simulation examples also supported our results.
Nowadays, network theory is a popular and important research field. The book by Barabási [1] contains several general facts on network theory. A mathematical tool to describe an evolving network is the theory of random graphs. The graph's vertices are the nodes of the network and the edges of the graph are the connections among the nodes. In real life, a connection can be a collaboration or an interaction.
In this paper, we do not want to overview the vast literature on network theory. As we intend to apply branching processes to describe a network evolution, we focus on papers using branching processes in network theory. Bollobás and Riordan in [2] considered several problems of random graphs and applied Galton-Watson branching processes to prove some of the theorems. Rudas et al. [3] as well as Rudas and Tóth [4] applied continuous-time branching processes to study random tree growth models. In [5], a preferential attachment random graph is embedded in a continuous-time branching process to find the asymptotic behavior of the graph. In [6], continuous-time branching processes were applied to study an estimator in sublinear preferential attachment trees. In their survey paper [7], Holmgren and Janson studied the asymptotics of certain trees using Crump-Mode-Jagers branching processes. In [8], multi-type preferential attachment trees were studied using the theory of multi-type continuous-time branching processes. Banerjee and Huang [9] studied growing networks via embedding into a continuous-time branching process. In [10], Iksanov et al. underlined that one of the main applications of the theory of branching processes is the study of evolving networks. Usually, the advanced theory of branching processes is applied to prove new results for growing networks. However, sometimes network theory inspires new research in the field of branching processes, e.g., [11].
Most studies in network theory concentrate on connections of two nodes. However, cooperation of more than two units is also important. There are models describing such cooperation. Backhausz and Móri studied three-interactions in [12], while Fazekas and Porvázsnyik in [13] considered N-interactions.
In this paper, we shall study a continuous-time network evolution model based on the cooperation of several units. Our model was motivated by the activities of teams of people. We focused on cooperation among friends, recruitment of party members, recruitment of volunteers, collaboration among scientists, and teams in social networks. In the above cases, a person can be a member of several teams at the same time, new teams can emerge and disappear, and newcomers can join an existing team.
In this paper, we apply similar mathematical tools as in [14,15], where continuous-time branching processes were used (see also [16]). In [14], the starting network is a single edge. Any edge produces several new vertices during evolution, and then the edge disappears. Any new vertex produced by an edge is joined to the endpoints of the reproducing edge with one or two new edges. In [14], the reproduction process of the edges is a continuous-time branching process. In [15], 2- and 3-cliques are considered and some results of [14] are extended.
In this paper, we shall study networks containing cliques (i.e., groups) of sizes 1,2,…,N, where N is fixed. We shall apply multi-type branching processes, where the type is the size of a clique. The researches [8,17] also applied multi-type branching processes, but there the vertices had different types. We also mention, that the evolution process considered in this paper is similar to the one studied in [15], but we emphasize that the process in [15] is not a particular case of the process in the present paper.
During the evolution of the network, when a newcomer joins the network, it joins directly with certain members of an existing clique in the network. Then they will form a new clique which we shall consider as a child of the old clique. Any clique produces an offspring clique each time its driving Poisson process jumps. Then these offspring start their reproduction processes. The length of life of a clique depends on the number of offspring it has.
This paper is divided into eight sections. In Section 3, we define our model precisely. In Section 4, we present our general results. We calculate the survival function of a clique (Theorem 4.1), the mean offspring number of a clique (Corollary 4.1), the Laplace transforms (Proposition 4.1), and then we turn to the Perron root and the Malthusian parameter. We do not obtain an explicit expression for the Malthusian parameter, so we can numerically compute it. In Section 5, we prove asymptotic theorems for the number of cliques of a given size (Theorem 5.1). Each has magnitude eαt in the event of non-extinction, where α is the Malthusian parameter. The proof of Theorem 5.1 is based on the asymptotic theorems of [18]. A most interesting question in the theory of evolving networks is the degree process of a fixed vertex. Therefore, in Section 6, we prove asymptotic theorems for the degree of a fixed vertex. For the proof, we introduce a new branching process, called the "good children" process, and then we can apply the asymptotic theorems of [18]. We obtain that the magnitude of the degree of a fixed vertex is eˆαt, where ˆα is the Malthusian parameter of the "good children" process. In Section 7, we obtain the generating functions. We apply the generating functions to find the probability of extinction of the network, see Theorem 7.1. In Section 8, we show simulation results. We shall see that the simulation results support our theorems.
We mention that basic results on branching processes are contained e.g., in [19,20,21,22,23].
In this paper, we shall consider the following model to describe certain evolving networks. The main ingredients of the network are teams. Any team is represented as a clique, i.e., a graph having n vertices so that any two vertices are connected with one edge. The size of a clique is the number of its vertices. In our model, there are cliques of sizes 1,2,…,N, where N is an arbitrarily large but fixed number. We shall describe the evolution of the network by a multi-type branching process. In terms of multi-type branching processes, see, e.g., [21], an n-clique would be a type-n individual.
At the time t=0 there is one team, and the size of this team can be 1,2,…,N. In terms of branching processes, we call this team the ancestor. This ancestor team produces offspring teams which can be cliques of sizes 1,2,…,N. Then these children teams also produce their own children teams, and so on. Any team has its rate of 1 Poisson process. The jumping times of this Poisson process are the reproduction times of the team. We shall suppose that the reproduction processes of different teams are independent. The reproduction processes of the teams of size n are independent copies of the reproduction process of the generic team of size n.
Now, we identify any team with the clique representing it. The mathematical description of the evolution of the generic n-clique is the following. Let Πn(t) denote the Poisson process with parameter 1 corresponding to the generic n-clique. The jumping times of Πn(t) are the reproduction times. When Πn(t) jumps, then a new vertex appears and we connect it to certain vertices of the generic n-clique. The new vertex will be connected to j vertices of the generic n-clique with probability qn,j, where 0≤qn,j≤1, j=0,1,…,n, and ∑nj=0qn,j=1. (We assume that qN,N=0 because the largest team is of size N.) When j is chosen, then together with the new vertex, j new edges appear. One endpoint of any new edge is the new vertex. The other j endpoints of the j new edges are chosen randomly from the vertices of the generic n-clique. The number of subsets having j elements is (nj). We choose one of these (nj) subsets uniformly at random. The vertices of this subset are already connected, and now we connect each of them to the new vertex with one edge. So the j old connected vertices chosen, the new vertex, and the j new edges form a (j+1)-clique. This new (j+1)-clique is a child of the generic n-clique and it is the only child at this step. We should emphasize that the result of the above step is precisely one child because we shall count only this one child. The reason for this point of view is that we are interested in the number of teams, and the new sub-cliques of the new (j+1)-clique are not considered as teams.
We see that the generic n-clique produces precisely one child clique at any birth time. If j=0, this child is just one vertex, i.e., the new vertex joining the network with 0 edges. If j=1, then this child is an edge, which consists of the new vertex and the old vertex connected to the new one. If j=2, this child is a triangle consisting of the new vertex and two vertices of the generic n-clique. If j=n<N, this child is an (n+1)-clique consisting of the whole n-clique ancestor and the new vertex. We emphasize that when the parent is the largest possible clique, i.e., an N-clique, we do not allow the birth of an (N+1)-clique. We also see that the probability that an i-type ancestor produces a j-type child is pi,j=qi,j−1, j=1,2,…,i+1. The ancestor clique, the children cliques of the ancestor, and the grandchildren cliques, etc., will form an evolving network.
Example 3.1. We visualize four simple reproduction steps in Figures 1–4.
In our model, cliques can die. When a clique dies, it will be an inactive clique not producing children. But we do not delete its vertices and edges, because any vertex or edge can belong to several cliques. So we shall study both the number of all j-cliques born during the evolution process and the number of active j-cliques. We underline again that we are interested in the number of teams. The teams (i.e., cliques) are born during the above evolution process, i.e., the offspring of the ancestor. So we will not count those cliques that are not offspring of the ancestor clique.
Let us denote by ξi,j(t) the number of type-j children cliques of the type-i generic clique up to time t (i,j=1,2,…,N). The processes ξi,j are point processes. Then
ξi(t)=i+1∑j=1ξi,j(t) | (3.1) |
is the number of all children of the generic i-clique up to time t.
Let τi(1),τi(2),… be the birth times of the generic i-clique and denote by εi(1),εi(2),… the corresponding total litter sizes. In our model, εi(k)≡1.
Let λi be the life length of the generic i-clique. λi is a random variable with P(0≤λi<∞)=1. After its death, a clique does not produce offspring, so ξi(t)=ξi(λi), when t>λi. So
ξi(t)=∑τi(k)≤t∧λiεi(k)=Πi(t∧λi), | (3.2) |
where Πi(t) is the Poisson process, and x∧y is the minimum of {x,y}.
Now we turn to the survival function of the life length of an i-clique. Li(t) will denote the distribution function of λi. Then the survival function of λi is
1−Li(t)=P(λi>t)=exp(−∫t0li(u)du). | (3.3) |
Here li(t) denotes the hazard rate function of λi.
A major assumption of this paper is that the hazard rate is determined by the total number of offspring in the following way:
li(t)=b+cξi(t), | (3.4) |
where b≥0 and c>0 are fixed constants.
We emphasize that the reproduction processes of the i-cliques are independent copies of the reproduction process of the generic i-clique.
First, we calculate the survival function of an i-clique.
Theorem 4.1. For any i, the survival function of an i-clique is
1−Li(t)=P(λi>t)=e−(b+1)te1−e−ctc. | (4.1) |
Proof. Using the general calculation of [15, Theorem 1], we have
P(λi>t)=e−(b+1)te∑∞j=1sj1−e−ctjcj, |
where sj denotes the distribution of εi. As now εi≡1, so s1=1, and therefore the survival function for an i-clique is
P(λi>t)=e−(b+1)te1−e−ctc. |
Now, we turn to the mean offspring number. It is mi,j(t)=Eξi,j(t), which is the expectation of the number of type-j offspring of a type-i parent up to time t.
Corollary 4.1. For any t≥0, we have
mi,j(t)=pi,jF(t), | (4.2) |
where
F(t)=∫t0(1−Li(s))ds=∫t0e−(b+1)se1−e−cscds=1c∫1−e−ct0(1−u)b+1c−1eucdu, |
0<F(∞)<∞.
Eλi=1c∫10(1−u)b+1c−1eucdu. | (4.3) |
Proof. Similar to the proof of [15, Corollary 1], we have
mi,j(t)=Eξi,j(t)=E(εi,j(1)+εi,j(2)+⋯+εi,j(Π(t∧λi))), |
where εi,j(k) is the number of type-j children of a type-i mother at her kth birth event. Applying Wald's identity, we get
mi,j(t)=E(εi,j(1))E(Π(t∧λi)). | (4.4) |
Π is a rate-1 Poisson process, and t∧λ is bounded, so (4.4) implies that
mi,j(t)=E(εi,j(1))E(t∧λi)=E(εi,j(1))∫t0(1−Li(x))dx. | (4.5) |
In our case P(εi,j(k)=1)=pi,j, so from (4.1) and inserting u=1−e−cs, we get
mi,j(t)=pi,j∫t0e−(b+1)se1−e−cscds=pi,jc∫1−e−ct0(1−u)b+1c−1eucdu. | (4.6) |
We need the Laplace transform of mi,j,
m∗i,j(κ)=∫∞0e−κsmi,j(ds),i,j=1,2,…,N. |
Proposition 4.1. We have
m∗i,j(κ)=pi,jA(κ),κ≥0, | (4.7) |
where
A(κ)=∫∞0e−κte−(b+1)te1−e−ctcdt=1c∫10(1−u)κ+b+1c−1eucdu. | (4.8) |
mi,j(∞)=pi,jA(0). If A(0)>1, then A(α)=1 for some α>0.
Proof. For the proof, one can use Corollary 4.1.
Now, we shall consider the Perron root. The Laplace transform matrix is
M(κ)=(m∗i,j(κ))Ni,j=1. | (4.9) |
The characteristic roots of M(κ) are denoted by ϱl(κ), l=1,…,N. The Perron root is the greatest of the values ϱl(κ). We denote it by ϱ(κ).
We suppose that
ϱ(0)>1, | (4.10) |
that is, we assume that our process is supercritical.
We shall need the Malthusian parameter. α is called the Malthusian parameter if ϱ(α)=1.
If α is the Malthusian parameter, then we denote by v=(v1,…,vN)⊤ the right eigenvector of M(α) corresponding to eigenvalue 1 and normalized as v1+⋯+vN=1. Let u=(u1,…,uN)⊤ be the left eigenvector of M(α) satisfying u1v1+⋯+uNvN=1.
We shall suppose that the stochastic matrix (pi,j)Ni,j=1 is irreducible and acyclic. Then its Perron root is 1, so ϱ(κ)=A(κ). Therefore, our branching process is supercritical if and only if A(0)>1. If A(0)>1, then there exists a Malthusian parameter, that is, an α>0 such that A(α)=1. We also see that v=(1/N,…,1/N)⊤.
For any evolving network, a basic question is the growth of the number of ingredients of the network. So, for our network, we should find the number of cliques. For the proof, we shall use powerful results on multi-type branching processes, see [18, Theorem 2.4,and Proposition 4.1]. In [15, Section 8], we summarized those results in one proposition, see [15, Proposition 4]. So here in the proof, we shall check conditions (a), (b1), (b2), (c), (d), and (i)−(iii) of [15, Section 8].
We shall assume that the matrix (pi,j) is irreducible and acyclic. Therefore, [24, Theorem 1.4] gives that there exists a positive integer K, such that each element of the Kth power of the matrix (pi,j) is positive. As mi,j(∞)=pi,jA(0) and A(0)>0, condition (d) will be satisfied.
For condition (a), we have to show that not all measures mi,j are concentrated on a lattice. By Corollary 4.1, these measures are absolutely continuous, so condition (a) is fulfilled.
For (b2), we shall assume that A(0)>1. As mi,j(∞)=pi,jA(0), it will imply (b2). Concerning condition (b1), we mention that A(0)>1 implies that there exists a Malthusian parameter, that is, an α>0 such that A(α)=1. We can numerically calculate the value of α.
We shall check condition (c) during the proof of Theorem 5.1.
Now, we shall find the denominator in the limit theorem. We can see that the denominator of mΦ∞ in the asymptotic expression does not depend on Φ and it has the form
D(α)=N∑l,j=1ulvj∫∞0se−αsml,j(ds). |
It is the same as
D(α)=N∑l,j=1ulvj(−m∗l,j(α))′. | (5.1) |
Here ui and vi are the coordinates of the eigenvectors and we know that v=(1/N,…,1/N)⊤. Moreover, by Proposition 4.1, we can see that
(−m∗l,j(α))′=pl,j(−A′(α)). | (5.2) |
So
D(α)=−A′(α)N∑l,j=1ulvjpl,j=−A′(α). | (5.3) |
Here
−A′(α)=∫∞0te−αte−(b+1)te1−e−ctcdt=−1c2∫10ln(1−x)(1−x)α+b+1c−1excdx. | (5.4) |
Now, we shall consider the number of the n-cliques.
Theorem 5.1. Assume that the matrix (pi,j)Ni,j=1 is irreducible and acyclic. Assume that A(0)>1. Let α be the Malthusian parameter, i.e., a finite positive solution of equation A(α)=1. Let n be fixed, 1≤n≤N. We denote by kT(t) the number of all n-cliques being born up to time t if the ancestor of the network was a k-clique, k=1,2,…,N. Then
limt→∞e−αtkT(t)=kWvkunα(−A′(α)) | (5.5) |
almost surely for k=1,2,…,N.
We denote by kˆT(t) the number of all n-cliques alive at time t if the ancestor of the network was a k-clique, k=1,2,…,N. Then
limt→∞e−αtkˆT(t)=kWvkunA(α)(−A′(α)) | (5.6) |
almost surely for k=1,2,…,N.
The quantity kW is a.s. non-negative, E(kW)=1, and kW is a.s. positive on the event of survival.
Proof. We shall use [15, Proposition 4] as in the proof of [15, Theorem 2]. To prove condition (vi), it is enough to show that
E[αξi(∞)log+αξi(∞)]<∞,i=1,2,…,N, | (5.7) |
where
αξi(∞)=∫∞0e−αtξi(dt),i=1,2,…,N, | (5.8) |
and
ξi(t)=ξi,1(t)+ξi,2(t)+⋯+ξi,i+1(t),i=1,2,…,N. | (5.9) |
At any birth, there is precisely 1 child, so
αξi(∞)=∫∞0e−αtξi(dt)=∑τ(j)≤λi1e−ατ(j)≤∞∑j=11e−ατ(j)=M, |
where τ(1),τ(2),… are the jumping times of the Poisson process Πi. The interarrival time (τ(j)−τ(j−1)) is exponentially distributed with rate 1, so τ(j) has Γ-distribution Γ(j,1). It implies that
E(M)=∞∑j=1E(e−ατ(j))=∞∑j=11(1+α)j=1α. | (5.10) |
Let ηj=τ(j)−τ(j−1). Let η0 be a random variable having an exponential distribution with parameter 1 and assume that η0 and M are independent. Then
e−αη0(1+M)=e−αη0+e−αη0∞∑j=1e−α(η1+⋯+ηj)=∞∑j=0e−α(η0+η1+⋯+ηj). |
So e−αη0(1+M) has the same distribution as that of M. From this and Eq (5.10), we get
EM2=E(e−αη0(1+M))2=11+2α(1+2α+EM2). |
So we obtain
EM2=α+22α2<∞. |
Therefore (5.7) is true for any i.
To prove conditions (c) and (iv), it is enough to show that ∫∞0t2e−αtmi,j(dt)<∞, for i,j=1,2,…,N. Now, from Corollary 4.1, we get that
∫∞0s2e−αsmi,j(ds)≤∫∞0s2e−αse−s(b+1)e1−e−cscds≤∫∞0s2e−s(α+b+1−1)ds<∞, |
as α+b is positive. Therefore conditions (c) and (iv) are true.
Now, consider the number of n-cliques. To show (5.5), let Φx(t)=1 if x is an n-clique, and Φx(t)=0 otherwise. Therefore EΦn(t)=1 and EΦj(t)=0 for j≠n. So assumptions (i)−(iii) and (v) are true. Therefore, [15, Proposition 4] implies (5.5).
To prove (5.6), let Φx(t)=1 if x is an n-clique and it is alive at time t, and let Φx(t)=0 otherwise. Therefore EΦn(t)=1−Ln(t) and EΦj(t)=0 for j≠n. So (i)−(iii) and (v) are fulfilled. We see that
∫∞0e−αsEΦn(s)ds=∫∞0e−αs(1−Ln(s))ds=A(α). |
Using [15, Proposition 4], we get (5.6).
Remark 5.1. The process is supercritical if
1<ϱ(0)=A(0)=1c∫10(1−u)b+1c−1eucdu. |
If the process is supercritical, then there exists a Malthusian parameter.
For any pair b, c, one can show whether the process is supercritical. Here we just list a few cases.
If 1≤c<1/ln2 and 0≤b≤c−1, then the process corresponding to b, c is supercritical. Moreover the case b=0, c=1/2 is also supercritical.
If b≥1, c=1, then the process is not supercritical. The case b=1, c=2 is also not supercritical.
To consider the other values, we have made a numerical investigation on a rectangle: We have considered the cases when 0≤b≤2 and 0.01≤c≤2, because the parameter c is in the denominator. Figure 5 shows the set of parameters (b,c) for which the process is supercritical.
Example 5.1. Consider the Leslie model. For n=1,2,…,N−1, when a new vertex joins to an n-clique, then either it joins to all vertices of the n-clique, or the new vertex alone creates a new clique. So pn,n+1=pn, pn,1=1−pn for n=1,2,…,N−1. But for n=N, when a new vertex appears, then either it creates alone a new clique or the new vertex and N−1 old vertices create an N-clique. So pN,N=pN and pN,1=1−pN.
Now, the matrix of the Laplace transforms is
M(κ)=A(κ)(1−p1p10…01−p20p2…0⋮⋮⋮⋱1−pN−100…pN−11−pN00…pN). |
We assume that p1>0,…,pN−1>0, and 1−pN>0. We also assume that the greatest common divisor of the set {i=1,2,…,N:1−pi>0} is equal to 1. So the matrix (pi,j) is irreducible and acyclic. Therefore its Perron root is 1. So the Perron root of M(κ) is A(κ), and the corresponding right eigenvector is
v=(1N,1N,…,1N)⊤. |
Direct calculations show that the coordinates of the left eigenvector are
uN=Np1p2⋯pN−1(1−pN)(1+p1+p1p2+⋯+p1p2⋯pN−2)+p1p2⋯pN−1, |
and
uN−1=1−pNpN−1uN, uN−2=1−pNpN−1pN−2uN, …, u1=1−pNpN−1pN−2⋯p1uN. |
Now, Theorem 5.1 gives the asymptotic number of cliques.
Consider the particular case of the Leslie model when pi=a for any i, where 0<a<1. Then uN=NaN−1, and un=N(1−a)an−1, n=1,2,…,N−1. Inserting these values into the formulae (5.5) and (5.6), we obtain the asymptotic number of cliques. For n-cliques with n=1,2,…,N−1,
limt→∞e−αtkT(t)=kW(1−a)an−1⋅1α(−A′(α)), | (5.11) |
but for the N-cliques,
limt→∞e−αtkT(t)=kWaN−1⋅1α(−A′(α)) | (5.12) |
almost surely for k=1,2,…,N.
Similarly, for n-cliques with n=1,2,…,N−1,
limt→∞e−αtkˆT(t)=kW(1−a)an−1⋅A(α)(−A′(α)), | (5.13) |
and for the N-cliques,
limt→∞e−αtkˆT(t)=kWaN−1⋅A(α)(−A′(α)). | (5.14) |
We see that as a→1, then N-cliques dominate. It is a plausible consequence of the definition of the model.
Another particular case of the Leslie model is p1=pN=1/2 and pi=1 for i=2,…,N−1. Then u1=uN=(2N)/(N+2), and ui=uN/2 for i=2,…,N−1.
A basic question for any evolving network is the degree process of a fixed vertex. We study the topics of the connected network and the degree of a fixed vertex in the same chapter because both of them are studied by multi-type branching processes having N−1 types.
Remark 6.1. When the network is connected then it is not possible that a separated vertex is born. In that case, we can consider just the 2,3,…,N cliques. So the possible types of our branching process will be 2,3,…,N, where the type is 2 if the clique is an edge, it is 3 if the clique is a triangle, …, and it is N in the case of an N-clique. The probability that the new vertex will be connected to j vertices of the generic n-clique is again qn,j, where 0≤qn,j≤1, but now j=1,…,n and ∑nj=1qn,j=1. So we should consider a multi-type branching process having N−1 types. Now, the probability that a type-i parent gives birth to a type-j child is pi,j=qi,j−1 for i,j=2,3,…,N.
The results of the previous two sections remain valid with obvious modifications. So mi,j, m∗i,j are the same, but i,j=2,3,…,N.
We shall suppose that the stochastic matrix (pi,j)Ni,j=2 is irreducible and acyclic. Then its Perron root is 1, so ϱ(κ)=A(κ). Therefore, our branching process is supercritical if and only if A(0)>1. If A(0)>1, then there exists a Malthusian parameter, that is, an α>0 such that A(α)=1. v=(1/(N−1),…,1/(N−1))⊤ is the right eigenvector of (pi,j)Ni,j=2 corresponding to eigenvalue 1. u=(u2,…,uN)⊤ is the left eigenvector of (pi,j)Ni,j=2 satisfying the condition u2v2+⋯+uNvN=1.
We can modify Theorem 5.1 to find the number of the n-cliques in the case of the connected network. Assume that the matrix (pi,j)Ni,j=2 is irreducible and acyclic. Assume that A(0)>1. Let α be the Malthusian parameter. Let n be fixed, 2≤n≤N. Denote by kT(t) the number of all n-cliques being born up to time t if the ancestor of the population was a k-clique, k=2,3,…,N. Then
limt→∞e−αtkT(t)=kWvkunα(−A′(α)) | (6.1) |
almost surely for k=2,3,…,N.
This is similar for the number of n-cliques alive at time t.
Now, we turn to the degree process of a fixed vertex. Let V be a fixed vertex. Assume that at the beginning, V is a member of an i-clique. We assume that i≥2, so the initial degree of V is i−1≥1. We call a child a "good child" if it contains V. So the degree of V increases by 1, when a good child is born. More precisely, the degree of V increases when good children of the initial i-clique are born, and then good children of the good children are born, etc. Then the degree of V at time t is the total number of good children at that time.
Let ˆpi,j be the probability that a type-i parent gives birth to a type-j good child. Then
ˆpi,j=pi,jj−1i. |
We see that the good children process is a branching process having types 2,3,…,N. The length of the life of an i-clique is the same λi as in the case of our original process.
Let ˆξi,j be the number of type-j good children of a type-i good child. Then the average number of good children is
ˆmi,j(t)=Eˆξi,j(t)=j−1imi,j(t)=j−1ipi,jF(t). |
The Laplace transform of ˆmi,j(t) is
ˆm∗i,j(κ)=j−1im∗i,j(κ)=j−1ipi,jA(κ). |
Let
ˆM(κ)=(ˆm∗i,j(κ))Ni,j=2 |
be the matrix of the Laplace transforms. Denote by ˆϱ(κ) the Perron root of ˆM(κ). We shall suppose that the good children process is supercritical, i.e., ˆϱ(0)>1. If the process is supercritical, then in our case there exists a positive Malthusian parameter ˆα such that ˆϱ(ˆα)=1. Let ˆv=(ˆv2,…,ˆvN)⊤ be the right eigenvector of ˆM(ˆα) corresponding to eigenvalue 1 and normed according to ˆv2+⋯+ˆvN=1. Let ˆu=(ˆu2,…,ˆuN)⊤ be the left eigenvector of ˆM(ˆα) corresponding to eigenvalue 1 and normed according to ˆv2ˆu2+⋯+ˆvNˆuN=1.
Now, we can present our result on the asymptotic behavior of the degree of a fixed vertex.
Theorem 6.1. Assume that the matrix (pi,j)Ni,j=2 is irreducible and acyclic. Assume that the good children process is supercritical, i.e., ˆϱ(0)>1. Let ˆα be the positive Malthusian parameter, so ˆϱ(ˆα)=1. Let V be a fixed vertex which is initially a member of an i-clique, 2≤i≤N.
Let iV(t) denote the degree of V, more precisely, the number of all edges being connected to V up to time t. Then
limt→∞e−ˆαtiV(t)=iˆWˆvi∑Nj=2ˆuj−ˆαA′(ˆα) | (6.2) |
almost surely.
Let iˉV(t) denote the number of those edges, whose one endpoint is V and belonging to a clique alive at time t. Then
lim inft→∞e−ˆαtiˉV(t)≥iˆWA(ˆα)ˆvi∑Nj=2ˆuj−A′(ˆα) | (6.3) |
almost surely.
The quantity iˆW is a.s. non-negative, E(iˆW)=1, and iˆW is a.s. positive in the event of survival of the good children process.
Proof. Here we shall use some results obtained during the proof of Theorem 5.1. We have
iV(t)=(i−1)+N∑j=2Vi,j(t), |
where Vi,j(t) denotes the number of type-j good offspring of a type-i mother. We shall find the limit of Vi,j(t).
As in the proof of Theorem 5.1, we shall check the conditions of [15, Proposition 4]. Conditions (a), (c), (iv), and (vi) are true because they are true for the original process (see Theorem 5.1). (b1) and (b2) are true because we supposed that ˆϱ(0)>1. Condition (d) is true because we supposed that the matrix (pi,j)Ni,j=2 is irreducible and acyclic.
Now, let Φx(t)=1, if the clique x is a good j-clique, and Φx(t)=0 otherwise. Then conditions (i)−(iii), and (v) are satisfied. Moreover, EΦj(t)=1 and EΦl(t)=0 for l≠j.
So [15, Proposition 4] implies
limt→∞e−ˆαtVi,j(t)=iˆWˆviˆuj−ˆαA′(ˆα) |
almost surely. So we obtain (6.2).
To obtain (6.3), let Φx(t)=1 if x is a good j-clique and it is alive at time t, and let Φx(t)=0 otherwise. Therefore EΦj(t)=1−Lj(t) and EΦl(t)=0 for l≠j. Conditions (i)−(iii) and (v) are true. Now
∫∞0e−αsEΦj(s)ds=∫∞0e−αs(1−Lj(s))ds=A(α). |
Now, we can apply [15, Proposition 4]. However, in (6.3) we cannot offer equality, because an edge can belong to a clique being alive and at the same time it can belong to a clique being dead.
To find the probability of the extinction of our network, we need the generating function. We calculate the joint generating function of the variables Πn(λn) and ξn,j(λn), j=1,…,(n+1)∧N, n=1,…,N. Consider the generic n-clique, where n is fixed with 1≤n≤N. Consider the sequence
wi,{kj}(n+1)∧Nj=1=P(Πn(λn)=i,ξn,j(λn)=kj,j=1,…,(n+1)∧N), | (7.1) |
where i=0,1,2,…, kj=0,1,2,….
Then (7.1) describes the joint distribution of the last reproduction time and the offspring size of the generic n-clique during its whole lifetime. Here Πn is the Poisson process that describes the birth times of the generic n-clique and λn is its life length. In other words, we can say that wi,{kj}(n+1)∧Nj=1 gives the probability of the event that the last birth time of the generic n-clique before its death is τi and the total number of type-j offspring up to its death is kj. That is, we can write wi,{kj}(n+1)∧Nj=1 in the following form:
wi,{kj}(n+1)∧Nj=1=P(τi≤λn<τi+1,ξn,j(τi)=kj,j=1,…,(n+1)∧N). | (7.2) |
To determine the desired joint generating function, first we consider the following sequence:
ui,{kj}(n+1)∧Nj=1=P(τi≤λn,ξn,j(τi)=kj,j=1,…,(n+1)∧N). | (7.3) |
We use the notation τ0=0, so u0,{kj}(n+1)∧Nj=1=1 if each kj is zero, but it is 0 if any kj is positive. Moreover, ui,{kj}(n+1)∧Nj=1=0, if any subscript is negative. Now, for a while, assume that τi and τi−1 are fixed and ξn,j(τi−1)=m is known. Then using the definition of the survival function of the life length given in (3.3) and by Assumption (3.4), we have
P(λn≥τi|λn≥τi−1)=exp(−(τi−τi−1)(b+cm)). | (7.4) |
But τi and τi−1 are random. So, a simple calculation that uses the fact that the increments of a Poisson process with intensity 1 are exponential with parameter 1 can lead us to obtain that
P(λn≥τi|λn≥τi−1)=11+b+cm. | (7.5) |
That is, (7.5) is the probability that the object will not die before the ith birth event. Using the above calculations and the law of total probability, we can give the following recursion for the sequence ui,{kj}(n+1)∧Nj=1, i=1,2,…
ui,{kj}(n+1)∧Nj=1=(n+1)∧N∑l=1P(τi−1≤λn,ξn,l(τi−1)=kl−1,ξn,j(τi−1)=kj,j=1,…,(n+1)∧N,j≠l)×pn,l−111+b+c((∑(n+1)∧Nj=1kj)−1)=(n+1)∧N∑l=1ui−1,kl−1,{kj}(n+1)∧Nj=1,j≠lpn,l−111+b+c((∑(n+1)∧Nj=1kj)−1). | (7.6) |
Here pn,j is the probability that the new vertex is born with j new edges. To obtain the above recursion, we also used the following. Considering the generic n-clique, the definition of the evolution process implies that at each birth step, exactly 1 offspring is born, the smallest possible offspring size is 1, and the maximal offspring size is (n+1)∧N. Moreover, the offspring sizes of the generic n-clique at any two consecutive birth steps are independent. Using (7.3) and (7.5), we can also see that
wi,{kj}(n+1)∧Nj=1P(τi≤λn<τi+1,ξn,j(τi)=kj,j=1,…,(n+1)∧N)=P(λn<τi+1|τi≤λn,ξn,j(τi)=kj,j=1,…,(n+1)∧N)×P(τi≤λn, ξn,j(τi)=kj,j=1,…,(n+1)∧N)=b+c∑(n+1)∧Nj=1kj1+b+c∑(n+1)∧Nj=1kjui,{kj}(n+1)∧Nj=1. | (7.7) |
Let us consider the following sequence vi,{kj}(n+1)∧Nj=1, where wi,{kj}(n+1)∧Nj=1 is defined in (7.1):
vi,{kj}(n+1)∧Nj=1=wi,{kj}(n+1)∧Nj=1b+c∑(n+1)∧Nj=1kj=ui,{kj}(n+1)∧Nj=11+b+c∑(n+1)∧Nj=1kj. | (7.8) |
Moreover, using the recursion (7.6), we see that the sequence vi,{kj}(n+1)∧Nj=1 satisfies the following recurrence relation:
(1+b+c(n+1)∧N∑j=1kj)vi,{kj}(n+1)∧Nj=1=(n+1)∧N∑l=1pn,l−1vi−1,kl−1,{kj}(n+1)∧Nj=1,j≠l, | (7.9) |
where the initial values are
v0,{0}(n+1)∧Nj=1=11+b and v0,{kj}(n+1)∧Nj=1=0 if ∃j:kj≠0. | (7.10) |
Let us denote by G(x,{xj}(n+1)∧Nj=1) the generating function of the sequence vi,{kj}(n+1)∧Nj=1. We have
G(x,{xj}(n+1)∧Nj=1)=∞∑i=0(n+1)∧N∑j=1∞∑kj=0vi,{kj}(n+1)∧Nj=1xi(n+1)∧N∏j=1xkjj. | (7.11) |
To determine the generating function G(x,{xj}(n+1)∧Nj=1), we multiply with xi∏(n+1)∧Nj=1xkjj and then take the sum of both sides of (7.9). In this way, we obtain that
∞∑i=0(n+1)∧N∑j=1∞∑kj=0(1+b+c(n+1)∧N∑j=1kj)vi,{kj}(n+1)∧Nj=1xi(n+1)∧N∏j=1xkjj | (7.12) |
=(n+1)∧N∑l=1pn,l−1∞∑i=0(n+1)∧N∑j=1∞∑kj=0vi−1,kl−1,{kj}(n+1)∧Nj=1,j≠lxi(n+1)∧N∏j=1xkjj. | (7.13) |
From this equation and using the definition of the generating function given by (7.11), we can obtain that
(1+b)(G(x,{xj}(n+1)∧Nj=1)−11+b)+c(n+1)∧N∑j=1xjG′xj(x,{xj}(n+1)∧Nj=1) | (7.14) |
=(n+1)∧N∑l=1pn,l−1xxlG(x,{xj}(n+1)∧Nj=1). | (7.15) |
Let h(t)=G(x,{txj}(n+1)∧Nj=1). By (7.10), we have the initial condition
h(0)=G(x,{0}(n+1)∧Nj=1)=∞∑i=0vi,{0}(n+1)∧Nj=1xi=11+b. |
Now, substituting xj with txj in (7.14), we get the following first-order differential equation:
h′(t)+h(t)1ct((1+b)−t(n+1)∧N∑l=1pn,l−1xxl)=1ct, | (7.16) |
where the initial value is
h(0)=11+b. | (7.17) |
The solution of the above initial value problem (7.16) and (7.17) is
h(t)=t−(1+b)ce∑(n+1)∧Nl=1pn,l−1xxlct1c∫t0s1+bc−1e−∑(n+1)∧Nl=1pn,l−1xxlcsds. | (7.18) |
Substituting t=1 in (7.18), we obtain that the generating function of the sequence vi,{kj}(n+1)∧Nj=1 is
G(x,{xj}(n+1)∧Nj=1)=h(1)=1c∫10s1+bc−1e∑(n+1)∧Nl=1pn,l−1xxlc(1−s)ds. | (7.19) |
Now, let us denote by gΠn,{ξn,j}(n+1)∧Nj=1 the joint generating function of the variables Πn(λn) and ξn,j(λn), j=1,…,(n+1)∧N. Using the definitions of the sequences wi,{kj}(n+1)∧Nj=1 and vi,{kj}(n+1)∧Nj=1, by (7.14) we have
gΠn,{ξn,j}(n+1)∧Nj=1(x,{xj}(n+1)∧Nj=1)=E(xΠn(λn)(n+1)∧N∏j=1xξn,j(λn)j)=∞∑i=0(n+1)∧N∑j=1∞∑kj=0P(Πn(λn)=i,ξn,j(λn)=kj,j=1,…,(n+1)∧N)xi(n+1)∧N∏j=1xkjj=bG(x,{xj}(n+1)∧Nj=1)+c(n+1)∧N∑j=1xjG′xj(x,{xj}(n+1)∧Nj=1)=e∑(n+1)∧Nl=1pn,l−1xlc1c∫10s1+bc−1e−∑(n+1)∧Nl=1pn,l−1xxlcs(b+(1−s)(n+1)∧N∑l=1pn,l−1xxl)ds. | (7.20) |
With x=1 in (7.20), we obtain that the generating function of the total offspring distribution of the generic n-clique is
fn({xj}(n+1)∧Nj=1)=e∑(n+1)∧Nl=1pn,l−1xlc1c∫10s1+bc−1e−(n+1)∧N∑l=1pn,l−1xlcs(b+(1−s)(n+1)∧N∑l=1pn,l−1xl)ds. | (7.21) |
Consider the embedded multi-type Galton-Watson process which can be constructed in the following way. At the initial time, the ancestor alone constitutes the starting generation of the Galton-Watson process. During its life, the ancestor produces a random number of offspring. All of the offspring of this ancestor form the 1st generation. Generally, the nth generation is formed by the offspring of the members of the (n−1)th generation.
Under some reasonable conditions, the probability of extinction of our process is the same as the probability of extinction of the embedded multi-type Galton-Watson process, see [21, Theorem 7.1 in Chapter 3]. In our case those conditions are satisfied. Let M be the matrix of the expected total offspring number of our process. Then
M=(mi,j(∞))Ni,j=1=A(0)(pi,j(∞))Ni,j=1. |
We see that the matrix M contains the expected offspring numbers of the embedded Galton-Watson process.
From the theory of multi-type branching processes, we know that the probability of extinction may depend on the type of the ancestor. Moreover, the vector of extinction probabilities is the solution of a vector equation.
Theorem 7.1. Let us denote by si the probability of extinction when the ancestor of the network is an i-type object. Let s=(s1,…,sN). Assume that (pi,j(∞))Ni,j=1 is an irreducible acyclic Markov transition matrix. Denote by ϱ the Perron–Frobenius root of M. If ϱ≤1, then s1=s2=⋯=sN=1. If ϱ>1, then s1<1,s2<1,…,sN<1. In any case, s is the smallest non-negative solution of the vector equation
s=f(s), | (7.22) |
where f=(f1,…,fN) and the functions fk are defined in (7.21).
Proof. Our conditions ensure that the matrix M is positively regular. Now, applying [21, Theorem 7.1 in Chapter 1], we can obtain the desired result.
In this section, we present some numerical results for our previously presented asymptotic theorems. We used the Julia environment because of the possibility of fast numerical computing allowed by the well-written dynamic structures. The code can be downloaded from GitHub, see [25].
According to Theorem 5.1, the numbers of n-cliques, when the process moves forward in time, are asymptotically close to a straight line on the logarithmic scale. To support numerically our Theorem 5.1, we studied the slope of the sequence of the simulated number of n-cliques being born up to time t on the logarithmic scale.
Example 8.1. Now, we present an example of the Leslie model with N=5, the transition matrix
P1=(0.10.90000.200.8000.3000.700.40000.60.50000.5), |
and with parameters of the hazard rate b=0.2 and c=0.2. In Figure 6, we illustrate the first 219 birth steps of two different simulated processes. The five solid lines represent the number of n-cliques being born, for n=1,…,5 on a logarithmic scale, while the dotted line's slope ˆα = 0.6462 equals the numerical approximation of the Malthusian parameter. We can see that the plotted lines are parallel straight lines for large values of t, which in fact gives nice feedback to our asymptotic results.
Then, to obtain statistically significant evidence, we used 100 simulated processes to construct a 99% confidence stripe for the trajectory of the number of n-cliques. For demonstration, in Figure 7, the 99% confidence stripes are presented for 4- and 5-cliques. The red lines are the borders of the stripes.
In Table 1, the boundaries of the 99% confidence intervals for α are presented. Each fixed clique size gives a confidence interval. The columns labeled with 0.5% and 99.5% show the lower and the upper bounds calculated from simulations. As the results show, the numerical approximation ˆα1=0.6462 is contained by all confidence intervals.
Type | 0.5% | 99.5% |
1 | 0.6435 | 0.6769 |
2 | 0.6454 | 0.7205 |
3 | 0.6437 | 0.8244 |
4 | 0.6167 | 0.6420 |
5 | 0.6392 | 0.7276 |
Example 8.2. Now, we present another example with a transition matrix
P2=(0.10.90000.10.10.8000.10.10.10.700.10.10.10.10.60.10.10.10.10.6), |
and parameters b=0.4 and c=0.4. In this model, a newcomer joining an n-clique can contact any other group members, when n=1,…,4. For n=5, it is not possible that the newcomer joins all former clique members. In Figure 8, we show a simulated example of the process. The five solid lines represent the number of n-cliques being born, for n=1,…,5 on a logarithmic scale, while the dotted line's slope equals ˆα2=0.3391. Table 2 contains the confidence intervals for the slope, using 100 simulated processes.
Type | 0.5% | 99.5% |
1 | 0.3265 | 0.3623 |
2 | 0.3361 | 0.3547 |
3 | 0.3208 | 0.3490 |
4 | 0.3279 | 0.3411 |
5 | 0.3350 | 0.3548 |
We have introduced a new continuous-time network evolution model. We have proven asymptotic theorems for the number of cliques having a fixed size and the degree of a fixed node. We have obtained the probability of extinction. Besides precise mathematical proofs, we have presented simulation examples supporting our results.
I. Fazekas: Conceptualization, methodology for the whole paper, formal analysis, investigation, project administration, supervision, validation, writing-original draft, writing-review and editing; A. Barta: Formal analysis, investigation of Section 8, software, visualization, writing-original draft; L. Fórián: Formal analysis and investigation of Section 5, software, visualization, writing-original draft; B. Porvázsnyik: Formal analysis and investigation of Section 7, writing-original draft. All authors have read and approved the final version of the manuscript for publication.
The authors declare they have not used Artificial Intelligence (AI) tools in the creation of this article.
The authors would like to thank the referees and the editor for their helpful remarks.
The authors declare no conflict of interest.
[1] | A. L. Barabási, Network science, Cambridge University Press: Cambridge, UK, 2018. |
[2] | B. Bollobás, O. Riordan, Random graphs and branching processes, In: B. Bollobás, R. Kozma, D. Miklós (Eds.), Handbook of Large-Scale Random Networks, Bolyai Society Mathematical Studies, Springer: Berlin, Heidelberg, 18 (2008). https://doi.org/10.1007/978-3-540-69395-6_1 |
[3] |
A. Rudas, B. Tóth, B. Valkó, Random trees and general branching processes, Random Struct. Algor., 31 (2007), 186–202. https://doi.org/10.1002/rsa.20137 doi: 10.1002/rsa.20137
![]() |
[4] | A. Rudas, B. Tóth, Random tree growth with branching processes — A survey, In: B. Bollobás, R. Kozma, D. Miklós (Eds.), Handbook of Large-Scale Random Networks, Bolyai Society Mathematical Studies, Springer: Berlin, Heidelberg, 18, (2008). https://doi.org/10.1007/978-3-540-69395-6_4 |
[5] |
K. B. Athreya, A. P. Ghosh, S. Sethuraman, Growth of preferential attachment random graphs via continuous-time branching processes, P. Indian AS-Math. Sci., 118 (2008), 473–494. https://doi.org/10.1007/s12044-008-0036-2 doi: 10.1007/s12044-008-0036-2
![]() |
[6] |
F. Gao, A. van der Vaart, R. Castro, R. van der Hofstad, Consistent estimation in general sublinear preferential attachment trees, Electron. J. Stat., 11 (2017), 3979–3999. https://doi.org/10.1214/17-EJS1356 doi: 10.1214/17-EJS1356
![]() |
[7] |
C. Holmgren, S. Janson, Fringe trees, Crump-Mode-Jagers branching processes and m-ary search trees, Probab. Surv., 14 (2017), 53–154. https://doi.org/10.1214/16-PS272 doi: 10.1214/16-PS272
![]() |
[8] | S. Rosengren, A multi-type preferential attachment tree, Internet Math., 1 (2018). https://doi.org/10.24166/im.05.2018 |
[9] |
S. Banerjee, X. Huang, Degree centrality and root finding in growing random networks, Electron. J. Probab., 28 (2023), 1–39. https://doi.org/10.1214/23-EJP930 doi: 10.1214/23-EJP930
![]() |
[10] |
A. Iksanov, K. Kolesko, M. Meiners, Asymptotic fluctuations in supercritical Crump-Mode-Jagers processes, Ann. Probab., 52 (2024), 1538–1606. https://doi.org/10.1214/24-AOP1697 doi: 10.1214/24-AOP1697
![]() |
[11] |
T. F. Móri, S. Rokob, Moments of general time dependent branching processes with applications, Acta Math. Hung., 159 (2019), 131–149. https://doi.org/10.1007/s10474-019-00976-9 doi: 10.1007/s10474-019-00976-9
![]() |
[12] | Á. Backhausz, T. F. Móri, A random graph model based on 3-interactions, Ann. Univ. Sci. Budapest. Sect. Comput., 36 (2012), 41–52. |
[13] |
I. Fazekas, B. Porvázsnyik, Scale-free property for degrees and weights in an N-interactions random graph model, J. Math. Sci., 214 (2016), 69–82. https://doi.org/10.1007/s10958-016-2758-5 doi: 10.1007/s10958-016-2758-5
![]() |
[14] | T. F. Móri, S. Rokob, A random graph model driven by time-dependent branching dynamics, Ann. Univ. Sci. Budapest. Sect. Comp., 46 (2017), 191–213. |
[15] |
I. Fazekas, A. Barta, A continuous-time network evolution model describing 2- and 3-interactions, Mathematics, 9 (2021), 3143. https://doi.org/10.3390/math9233143 doi: 10.3390/math9233143
![]() |
[16] |
Q. Feng, X. Li, Z. Hu, Asymptotic degree distribution in a homogeneous evolving network model, Stat. Probabil. Lett., 193 (2023), 109740. https://doi.org/10.1016/j.spl.2022.109740 doi: 10.1016/j.spl.2022.109740
![]() |
[17] | M. Deijfen, R. Fitzner, Birds of a feather or opposites attract-effects in network modelling, arXiv Preprint, 2017. https://doi.org/10.48550/arXiv.1612.03127 |
[18] |
A. Iksanov, M. Meiners, Rate of convergence in the law of large numbers for supercritical general multi-type branching processes, Stoch. Proc. Appl., 125 (2015), 708–738. https://doi.org/10.1016/j.spa.2014.10.004 doi: 10.1016/j.spa.2014.10.004
![]() |
[19] | P. Jagers, Branching processes with biological applications, Wiley: London, 1975. |
[20] | P. Haccou, P. Jagers, V. A. Vatunin, Branching processes: Variation, growth, and extinction of populations, Cambridge University Press: Cambridge, UK, 2005. |
[21] | C. J. Mode, Multitype branching processes: Theory and applications, American Elsevier: New York, USA, 1971. |
[22] | O. Nerman, On the convergence of supercritical general branching processes, PhD Theses, University of Göteborg, 1979. |
[23] |
O. Nerman, On the convergence of supercritical general (C-M-J) branching processes, Z. Wahrsch. Verw. Gebiete, 57 (1981), 365–395. https://doi.org/10.1007/BF00534830 doi: 10.1007/BF00534830
![]() |
[24] | E. Seneta, Non-negative matrices and Markov chains, Springer: New York, USA, 1981. https://doi.org/10.1007/0-387-32792-4 |
[25] | Simulation codes for the article 'A continuous-time network evolution model describing N-interactions', 2024. Available from: https://github.com/bartaa89/n_interact. |
Type | 0.5% | 99.5% |
1 | 0.6435 | 0.6769 |
2 | 0.6454 | 0.7205 |
3 | 0.6437 | 0.8244 |
4 | 0.6167 | 0.6420 |
5 | 0.6392 | 0.7276 |
Type | 0.5% | 99.5% |
1 | 0.3265 | 0.3623 |
2 | 0.3361 | 0.3547 |
3 | 0.3208 | 0.3490 |
4 | 0.3279 | 0.3411 |
5 | 0.3350 | 0.3548 |