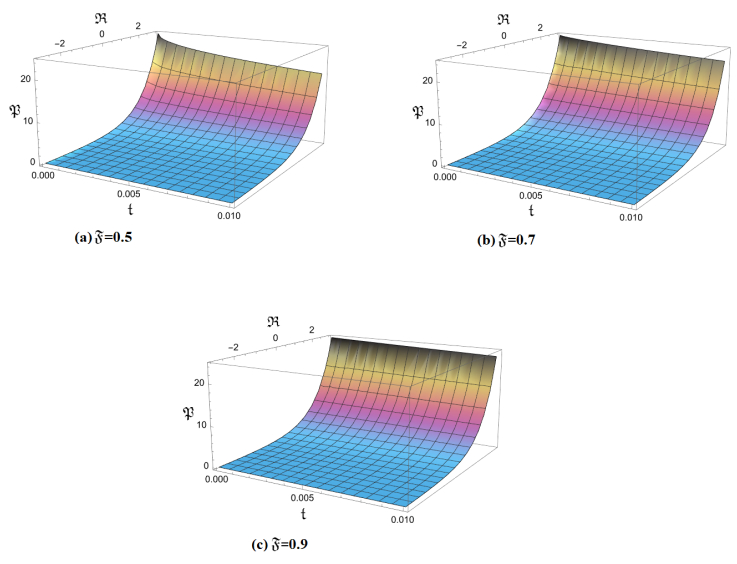
Rumor spreading on social media platforms can significantly impact public opinion and decision-making. In this paper, we proposed an innovative ignorant-spreader-expositor-hibernator-remover (ISEHR) rumor-spreading model with multivariate gatekeepers. Specifically, by analyzing the model's dynamics, we identified the critical threshold that determined the persistence or extinction of rumor spreading. Moreover, we applied the Routh-Hurwitz judgment, Lyapunov theory, and LaSalle's invariance principle to investigate the existence and stability of the rumor-free/rumor equilibrium points. Furthermore, we introduced the optimal control to alleviate rumor spreading with the multivariate gatekeeper mechanism. Finally, extensive numerical simulations validated our theoretical findings, providing insights into the complex dynamics of rumor spreading and the effectiveness of the proposed control measures. Our research contributes to a deeper understanding of rumor spreading on social networks, offering valuable implications for the development of effective strategies to combat rumor.
Citation: Yanchao Liu, Pengzhou Zhang, Deyu Li, Junpeng Gong. Dynamic analysis and optimum control of a rumor spreading model with multivariate gatekeepers[J]. AIMS Mathematics, 2024, 9(11): 31658-31678. doi: 10.3934/math.20241522
[1] | Meshari Alesemi . Innovative approaches of a time-fractional system of Boussinesq equations within a Mohand transform. AIMS Mathematics, 2024, 9(10): 29269-29295. doi: 10.3934/math.20241419 |
[2] | Azzh Saad Alshehry, Humaira Yasmin, Ali M. Mahnashi . Exploring fractional Advection-Dispersion equations with computational methods: Caputo operator and Mohand techniques. AIMS Mathematics, 2025, 10(1): 234-269. doi: 10.3934/math.2025012 |
[3] | Shabir Ahmad, Aman Ullah, Ali Akgül, Fahd Jarad . A hybrid analytical technique for solving nonlinear fractional order PDEs of power law kernel: Application to KdV and Fornberg-Witham equations. AIMS Mathematics, 2022, 7(5): 9389-9404. doi: 10.3934/math.2022521 |
[4] | Abdul Samad, Imran Siddique, Fahd Jarad . Meshfree numerical integration for some challenging multi-term fractional order PDEs. AIMS Mathematics, 2022, 7(8): 14249-14269. doi: 10.3934/math.2022785 |
[5] | Mamta Kapoor, Nehad Ali Shah, Wajaree Weera . Analytical solution of time-fractional Schrödinger equations via Shehu Adomian Decomposition Method. AIMS Mathematics, 2022, 7(10): 19562-19596. doi: 10.3934/math.20221074 |
[6] | Abdul Samad, Imran Siddique, Zareen A. Khan . Meshfree numerical approach for some time-space dependent order partial differential equations in porous media. AIMS Mathematics, 2023, 8(6): 13162-13180. doi: 10.3934/math.2023665 |
[7] | M. S. Alqurashi, Saima Rashid, Bushra Kanwal, Fahd Jarad, S. K. Elagan . A novel formulation of the fuzzy hybrid transform for dealing nonlinear partial differential equations via fuzzy fractional derivative involving general order. AIMS Mathematics, 2022, 7(8): 14946-14974. doi: 10.3934/math.2022819 |
[8] | Asif Khan, Tayyaba Akram, Arshad Khan, Shabir Ahmad, Kamsing Nonlaopon . Investigation of time fractional nonlinear KdV-Burgers equation under fractional operators with nonsingular kernels. AIMS Mathematics, 2023, 8(1): 1251-1268. doi: 10.3934/math.2023063 |
[9] | Muhammad Imran Liaqat, Sina Etemad, Shahram Rezapour, Choonkil Park . A novel analytical Aboodh residual power series method for solving linear and nonlinear time-fractional partial differential equations with variable coefficients. AIMS Mathematics, 2022, 7(9): 16917-16948. doi: 10.3934/math.2022929 |
[10] | Aliaa Burqan, Mohammed Shqair, Ahmad El-Ajou, Sherif M. E. Ismaeel, Zeyad AlZhour . Analytical solutions to the coupled fractional neutron diffusion equations with delayed neutrons system using Laplace transform method. AIMS Mathematics, 2023, 8(8): 19297-19312. doi: 10.3934/math.2023984 |
Rumor spreading on social media platforms can significantly impact public opinion and decision-making. In this paper, we proposed an innovative ignorant-spreader-expositor-hibernator-remover (ISEHR) rumor-spreading model with multivariate gatekeepers. Specifically, by analyzing the model's dynamics, we identified the critical threshold that determined the persistence or extinction of rumor spreading. Moreover, we applied the Routh-Hurwitz judgment, Lyapunov theory, and LaSalle's invariance principle to investigate the existence and stability of the rumor-free/rumor equilibrium points. Furthermore, we introduced the optimal control to alleviate rumor spreading with the multivariate gatekeeper mechanism. Finally, extensive numerical simulations validated our theoretical findings, providing insights into the complex dynamics of rumor spreading and the effectiveness of the proposed control measures. Our research contributes to a deeper understanding of rumor spreading on social networks, offering valuable implications for the development of effective strategies to combat rumor.
Mathematical methods have been used to represent a variety of real-world issues. For example, we can approximate a body's speed for a given distance and time by using the concept of rate of change. Specifically, we use the notion of differential calculus. Many complicated phenomena, such as chaos, solitons, asymptotic properties, singular formation, and others, are either poorly projected or have not yet been discovered [1,2,3]. Furthermore, the notion of calculus with differential operators and integrals is essential for describing physical phenomena and divining natural events connected to variation and changes. However, while researching issues with hereditary characteristics or memory, numerous researchers found numerous shortcomings and restrictions in integer-order calculus [4,5]. Later, new operators defined with the aid of fractional-order were proposed by mathematicians and physicists. Many researchers are drawn to fractional calculus (FC) while looking at different models [6,7,8].
In many branches of physical science and engineering, fractional calculus, which deals with arbitrary order derivatives and integrals, is crucial [9]. Fractional calculus and its applications have developed rapidly in the last few years [10,11]. Significant issues in acoustics, fluid mechanics, electromagnetic, analytical chemistry, signal processing, biology, and many other engineering and physical science branches are modeled by nonlinear and linear partial differential equations (PDEs) [12]. Both nonlinear and linear FDEs have been solved analytically and numerically in recent years using a variety of techniques, including the Yang-Laplace transform [13], the Adomian decomposition method [14], the homotopy analysis method [15], and the Laplace decomposition method [16]. Furthermore, nonlinear and linear FDEs are also subjected to the local fractional variational iteration approach [17,18], the fractional complex transform method [19], the modified Laplace decomposition approach [16,20], and the cylindrical-coordinate method [21].
Mathematical models known as fractional partial differential equations (FPDEs) depict physical processes that exhibit complicated dynamics and non-local effects. FPDEs represent an expansion of the traditional theory of partial differential equations. They enable non-integer orders of differentiation, which more accurately capture the non-local and nonlinear characteristics of an extensive range of physical systems [22,23]. These formulas are being used more and more frequently in several fields, such as biology, engineering, economics, and physics. These formulas are valuable resources for constructing intricate systems and examining the behavior of those operations. This article explores the concept of fractional-order partial differential equations, their applications, and the challenges associated with their analysis and numerical solution [24,25,26].
In 2013, Al-Smadi proposed the Residual Power Series Method (RPSM) [27]. It is generated from the residual error function mixed with the Taylor series. The solution to the problem is an infinite convergence series [28,29,30]. Many DEs have inspired fresh RPSM algorithms [31,32,33]. Among these DEs are several Boussinesq DEs, fuzzy DEs, and KdV Burger's equation, among many others. These systems are built to generate exact and efficient approximations. We provide a technique to investigate the approximation of solutions to fractional PDEs and systems of PDEs using RPSM in the Mohand transform (MT) formulation. The computational series finds the exact solution after a few iterations [34,35,36,37].
The computational complexity and effort necessary to implement the methods that were previously discussed are among the most significant constraints. The Mohand distinguishes our work transform iterative methodology (MTIM), which we developed as an iterative approach to addressing fractional PDEs and systems of PDEs. This technique is highly effective in reducing the amount of computational work and complexity necessary due to integrating the MT with the new iterative process.
In this study, the Mohand residual power series method (MRPSM) and MTIM are used to solve fractional PDEs and systems of PDEs. The numerical values produced by these techniques are more precise when compared to those of other numerical procedures. This study includes a comparison study of the numerical data. A strong indicator of the efficacy and reliability of these methods is the fact that the results of the many approaches presented are compatible with one another. The attractiveness of fractional-order derivatives grows in direct correlation with their worth. Because of this, the algorithms can withstand spikes in computational error, are easy to use, and are quick and accurate. Discovering this will make solving many partial differential equations much easier for mathematicians.
The portions that follow will cover the basic elements and ideas of the MT, providing the foundation for this operation.
Definition 2.1. The MT of the function P(t) is defined as [38]
M[P(t)]=R(s)=s2∫t0P(t)e−stdt, k1≤s≤k2. |
The inverse Mohand transform (IMT) is defined as
M−1[R(s)]=P(t). |
Definition 2.2. ([39]). The derivative of fractional-order in the framework of MT is defined as
M[PF(t)]=sFR(s)−n−1∑k=0Pk(0)sk−(F+1), 0<F≤n. |
Definition 2.3. The properties of MT are given as follows:
(1) M[P′(t)]=sR(s)−s2R(0).
(2) M[P″(t)]=s2R(s)−s3R(0)−s2R′(0).
(3) M[Pn(t)]=snR(s)−sn+1R(0)−snR′(0)−⋯−snRn−1(0).
Lemma 2.4. Suppose there exists a function represented by P(R,t), having exponential order. M[R(s)]=P(R,t) denotes the MT in this case:
M[DrFtP(R,t)]=srFR(s)−r−1∑j=0sF(r−j)−1DjFtP(R,0),0<F≤1, | (2.1) |
where R=(R1,R2,⋯,RF)∈RF, F∈N, and DrFt=DFt.DFt.⋯.DFt(r−times).
Proof. To validate Eq (2.4), we use the induction method. Taking r=1 in Eq (2.4):
M[D2FtP(R,t)]=s2FR(s)−s2F−1P(R,0)−sF−1DFtP(R,0). |
Equation (2.4) is true for r=1 on the basis of Definition 2.2. Now, put r=2 in Eq (2.4) to obtain the following outcome:
M[D2FrP(R,t)]=s2FR(s)−s2F−1P(R,0)−sF−1DFtP(R,0). | (2.2) |
We obtain the next result from the LHS of Eq (2.2):
L.H.S=M[D2FtP(R,t)]. | (2.3) |
We may also write Eq (2.3) as
L.H.S=M[DFtDFtP(R,t)]. | (2.4) |
Assume
z(R,t)=DFtP(R,t). | (2.5) |
Putting Eq (2.5) in Eq (2.4),
L.H.S=M[DFtz(R,t)]. | (2.6) |
Using the derivative of Caputo, Eq (2.6) becomes
L.H.S=M[J1−Fz′(R,t)]. | (2.7) |
Applying the RL integral on Eq (2.7),
L.H.S=M[z′(R,t)]s1−F. | (2.8) |
The derivative property of MT is applied on Eq (2.8) to obtain the following result:
L.H.S=sFZ(R,s)−z(R,0)s1−F. | (2.9) |
Using Eq (2.5), we obtain
Z(R,s)=sFR(s)−P(R,0)s1−F. |
As M[z(t,R)]=Z(R,s), we can write Eq (2.9) as
L.H.S=s2FR(s)−P(R,0)s1−2F−DFtP(R,0)s1−F. | (2.10) |
Assume that Eq (2.4) is true for r=K. Taking r=K in Eq (2.4),
M[DKFtP(R,t)]=sKFR(s)−K−1∑j=0sF(K−j)−1DjFtDjFtP(R,0), 0<F≤1. | (2.11) |
Next, we will have to show that Eq (2.4) for r=K+1 holds. Taking r=K+1 in Eq (2.4),
M[D(K+1)FtP(R,t)]=s(K+1)FR(s)−K∑j=0sF((K+1)−j)−1DjFtP(R,0). | (2.12) |
From the left-hand side of Eq (2.12), we derive
L.H.S=M[DKFt(DKFt)]. | (2.13) |
Letting DKFt=g(R,t), Eq (2.13) gives us
L.H.S=M[DFtg(R,t)]. | (2.14) |
Applying Caputo's derivative and the RL integral on Eq (2.14),
L.H.S=sFM[DKFtP(R,t)]−g(R,0)s1−F. | (2.15) |
On the basis of Eq (2.11), we can write Eq (2.15) as
L.H.S=srFR(s)−r−1∑j=0sF(r−j)−1DjFtP(R,0). | (2.16) |
Equation (2.16) can also be written as
L.H.S=M[DrFtP(R,0)]. |
Using mathematical induction, Eq (2.4) is true for r=K+1. Hence, it is proved that for all positive integers, Eq (2.4) holds.
Lemma 2.5. Let assume that there exists an exponential-order function P(R,t). M[P(R,t)]=R(s) denotes the MT of P(R,t). The multiple fractional power series (MFPS) in MT is given as
R(s)=∞∑r=0ℏr(R)srF+1,s>0, | (2.17) |
where, R=(s1,R2,⋯,RF)∈RF, F∈N.
Proof. Let us consider the Taylor series
P(R,t)=ℏ0(R)+ℏ1(R)tFΓ[F+1]++ℏ2(R)t2FΓ[2F+1]+⋯. | (2.18) |
MT is subjected to Eq (2.18) to obtain
M[P(R,t)]=M[ℏ0(R)]+M[ℏ1(R)tFΓ[F+1]]+M[ℏ1(R)t2FΓ[2F+1]]+⋯. |
Utilizing the features of MT, we derive
M[P(R,t)]=ℏ0(R)1s+ℏ1(R)Γ[F+1]Γ[F+1]1sF+1+ℏ2(R)Γ[2F+1]Γ[2F+1]1s2F+1⋯. |
Thus, a new Taylor series form is obtained.
Lemma 2.6. If M[P(R,t)]=R(s) denotes MT, then the new Taylor series form in MFPS is given as
ℏ0(R)=lims→∞sR(s)=P(R,0). | (2.19) |
Proof. Assume the Taylor's series
ℏ0(R)=sR(s)−ℏ1(R)sF−ℏ2(R)s2F−⋯. | (2.20) |
When the limit in Eq (2.19) is calculated and simplified, we get Eq (2.20).
In this part, we construct the framework of the proposed method for the solution of fractional PDEs.
Step 1. Let us assume the fractional PDE
DFtP(R,t)+K(R)N(P)−δ(R,P)=0. | (3.1) |
Step 2. Applying the MT on both sides of Eq (3.1),
M[DFtP(R,t)+K(R)N(P)−δ(R,P)]=0. | (3.2) |
On the basis of Lemma 2.4, we derive
R(s)=q−1∑j=0DjtP(R,0)sjF+1−K(R)Y(s)sjF+F(R,s)sjF, | (3.3) |
where, M[δ(R,P)]=F(R,s),M[N(P)]=Y(s).
Step 3. The subsequent result is derived from Eq (3.3):
R(s)=∞∑r=0ℏr(R)srF+1, s>0. |
Step 4. To obtain series form solution use the following procedure step by step:
ℏ0(R)=lims→∞sR(s)=P(R,0). |
Subsequently, we obtain
ℏ1(R)=DFtP(R,0),ℏ2(R)=D2FtP(R,0),⋮ℏw(R)=DwFtP(R,0). |
Step 5. To obtain R(s) as a Kth truncated series, we use
RK(s)=K∑r=0ℏr(R)srF+1, s>0, |
RK(s)=ℏ0(R)s+ℏ1(R)sF+1+⋯+ℏw(R)swF+1+K∑r=w+1ℏr(R)srF+1. |
Step 6. The Mohand residual function (MRF) from (3.3) is solved separately from the Kth-truncated Mohand residual function
MRes(R,s)=R(s)−q−1∑j=0DjtP(R,0)sjF+1+K(R)Y(s)sjF−F(R,s)sjF, |
and
MResK(R,s)=RK(s)−q−1∑j=0DjtP(R,0)sjF+1+K(R)Y(s)sjF−F(R,s)sjF. | (3.4) |
Step 7. In Eq (3.4), use RK(s) in place of its expansion form:
MResK(R,s)=(ℏ0(R)s+ℏ1(R)sF+1+⋯+ℏw(R)swF+1+K∑r=w+1ℏr(R)srF+1)−q−1∑j=0DjtP(R,0)sjF+1+K(R)Y(s)sjF−F(R,s)sjF. | (3.5) |
Step 8. Multiplying sKF+1 with Eq (3.5),
sKF+1MResK(R,s)=sKF+1(ℏ0(R)s+ℏ1(R)sF+1+⋯+ℏw(R)swF+1+K∑r=w+1ℏr(R)srF+1−q−1∑j=0DjtP(R,0)sjF+1+K(R)Y(s)sjF−F(R,s)sjF). | (3.6) |
Step 9. Taking the limit s→∞ of Eq (3.6),
lims→∞sKF+1MResK(R,s)=lims→∞sKF+1(ℏ0(R)s+ℏ1(R)sF+1+⋯+ℏw(R)swF+1+K∑r=w+1ℏr(R)srF+1−q−1∑j=0DjtP(R,0)sjF+1+K(R)Y(s)sjF−F(R,s)sjF). |
Step 10. The values of ℏK(R) are obtained by solving the following expression:
lims→∞(sKF+1MResK(R,s))=0, |
where K=1+w,2+w,⋯.
Step 11. Put ℏK(R) in Eq (3.3).
Step 12. To determine the required solution, take IMT to obtain RK(s) as PK(R,t).
Mohand transform iterative method
Suppose the PDE
DFtP(R,t)=Υ(P(R,t),DηRP(R,t),D2ηRP(R,t),D3ηRP(R,t)), 0<F,η≤1, | (3.7) |
with IC's
P(k)(R,0)=hk, k=0,1,2,⋯,m−1, | (3.8) |
where P(R,t) is a function to be determined and Υ(P(R,t),DηRP(R,t),D2ηRP(R,t),D3ηRP(R,t)) is operator of P(R,t),DηRP(R,t),D2ηRP(R,t) and D3ηRP(R,t). Equation (3.7) is subjected to MT to obtain
M[P(R,t)]=1sF(m−1∑k=0P(k)(R,0)s1−F+k+M[Υ(P(R,t),DηRP(R,t),D2ηRP(R,t),D3ηRP(R,t))]). | (3.9) |
The IMT gives us
P(R,t)=M−1[1sF(m−1∑k=0P(k)(R,0)s1−F+k+M[Υ(P(R,t),DηRP(R,t),D2ηRP(R,t),D3ηRP(R,t))])]. | (3.10) |
The solution via the MTIM technique is represented as
P(R,t)=∞∑i=0Pi. | (3.11) |
The decomposition of the operator Υ(P,DηRP,D2ηRP,D3ηRP) is
Υ(P,DηRP,D2ηRP,D3ηRP)=Υ(P0,DηRP0,D2ηRP0,D3ηRP0)+∞∑i=0(Υ(i∑k=0(Pk,DηRPk,D2ηRPk,D3ηRPk))−Υ(i−1∑k=1(Pk,DηRPk,D2ηRPk,D3ηRPk))). | (3.12) |
Putting Eqs (3.11) and (3.12) into Eq (3.10), we obtain
∞∑i=0Pi(R,t)=M−1[1sF(m−1∑k=0P(k)(R,0)s2−F+k+M[Υ(P0,DηRP0,D2ηRP0,D3ηRP0)])]+M−1[1sF(M[∞∑i=0(Υi∑k=0(Pk,DηRPk,D2ηRPk,D3ηRPk))])]−M−1[1sF(M[(Υi−1∑k=1(Pk,DηRPk,D2ηRPk,D3ηRPk))])] | (3.13) |
P0(R,t)=M−1[1sF(m−1∑k=0P(k)(R,0)s2−F+k)],P1(R,t)=M−1[1sF(M[Υ(P0,DηRP0,D2ηRP0,D3ηRP0)])],⋮Pm+1(R,t)=M−1[1sF(M[∞∑i=0(Υi∑k=0(Pk,DηRPk,D2ηRPk,D3ηRPk))])]−M−1[1sF(M[(Υi−1∑k=1(Pk,DηRPk,D2ηRPk,D3ηRPk))])], m=1,2,⋯. | (3.14) |
The general solution of Eq (3.7) is given as
P(R,t)=m−1∑i=0Pi. | (3.15) |
Example 4.1. ∙ Implementation of MRPSM
Let us consider the fractional PDE
DFtP(R,K,Z,t)+∂2P(R,K,Z,t)∂R2+∂2P(R,K,Z,t)∂K2+∂2P(R,K,Z,t)∂Z2=0, where 0<F≤1. | (4.1) |
The initial condition is
P(R,K,Z,0)=eR+K+Z, | (4.2) |
with exact solution
P(R,K,t)=eR+K+Z−3t. | (4.3) |
Equation (4.1) is subjected to MT, and using Eq (4.2) we get the following result:
P(R,K,Z,s)−eR+K+Zs+1sF[∂2P(R,K,Z,s)∂R2]+1sF[∂2P(R,K,Z,s)∂K2]+1sF[∂2P(R,K,Z,s)∂Z2]=0. | (4.4) |
The kth term's series is represented as
P(R,K,Z,s)=eR+K+Zs+k∑r=1fr(R,K,Z,s)srF+1, r=1,2,3,4⋯. | (4.5) |
The residual function of Mohand is given by
MtRes(R,K,Z,s)=P(R,K,Z,s)−eR+K+Zs+1sF[∂2P(R,K,Z,s)∂R2]+1sF[∂2P(R,K,Z,s)∂K2]+1sF[∂2P(R,K,Z,s)∂Z2]=0, | (4.6) |
and the kth-MRFs as
MtResk(R,K,Z,s)=Pk(R,K,Z,s)−eR+K+Zs+1sF[∂2Pk(R,K,Z,s)∂R2]+1sF[∂2Pk(R,K,Z,s)∂K2]+1sF[∂2Pk(R,K,Z,s)∂Z2]=0. | (4.7) |
Now, we use these steps to find the values of fr(R,K,Z,s) for r=1,2,3,...: Take the rth-Mohand residual function Eq (4.7) for the rth-truncated series Eq (4.5), and then multiply the equation by srF+1 and solve lims→∞(srF+1)MtResP,r(R,K,Z,s))=0 for r=1,2,3,⋯. Using this procedure, we obtain the following terms:
f1(R,K,Z,s)=−3eR+K+Z, | (4.8) |
f2(R,K,Z,s)=9eR+K+Z, | (4.9) |
f3(R,K,Z,s)=−27eR+K+Z, | (4.10) |
and so on.
The values of Eqs (4.9) and (4.10) are inserted in Eq (4.5) to obtain the following result:
P(R,K,Z,s)=eR+K+Zs−3eR+K+ZsF+1+9eR+K+Zs2F+1−27eR+K+Zs3F+1+⋯. | (4.11) |
Using IMT, we obtain the final solution
P1(R,K,Z,t)=eR+K+Z−3tFeR+K+ZΓ(F+1)+9t2FeR+K+ZΓ(2F+1)−27t3FeR+K+ZΓ(3F+1). | (4.12) |
∙ Implementation of MTIM
Consider the fractional PDE
DFtP(R,K,Z,t)=−∂2P(R,K,Z,t)∂R2−∂2P(R,K,Z,t)∂K2−∂2P(R,K,Z,t)∂Z2, where 0<F≤1. | (4.13) |
The initial condition is
P(R,K,Z,0)=eR+K+Z. | (4.14) |
MT is used on Eq (4.13), giving
M[DFtP(R,K,Z,t)]=1sF(m−1∑k=0P(k)(R,K,Z,0)s2−F+k+M[−∂2P(R,K,Z,t)∂R2−∂2P(R,K,Z,t)∂K2−∂2P(R,K,Z,t)∂Z2)]). | (4.15) |
Applying IMT on Eq (4.15), we obtain
P(R,K,Z,t)=M−1[1sF(m−1∑k=0P(k)(R,K,Z,0)s2−F+k+M[−∂2P(R,K,Z,t)∂R2−∂2P(R,K,Z,t)∂K2−∂2P(R,K,Z,t)∂Z2])]. | (4.16) |
Recursively applying the MT, we obtain
P0(R,K,Z,t)=M−1[1sF(m−1∑k=0P(k)(R,K,Z,0)s2−F+k)]=M−1[P(R,K,Z,0)s2]=eR+K+Z. |
The RL integral is implemented on Eq (4.13), giving
P(R,K,Z,t)=eR+K+Z+M[−∂2P(R,K,Z,t)∂R2−∂2P(R,K,Z,t)∂K2−∂2P(R,K,Z,t)∂Z2]. | (4.17) |
By using MTIM approach, we obtain the following terms:
P0(R,K,Z,t)=eR+K+Z, | (4.18) |
P1(R,K,Z,t)=−3tFeR+K+ZΓ(F+1), | (4.19) |
P2(R,K,Z,t)=9t2FeR+K+ZΓ(2F+1), | (4.20) |
P3(R,K,Z,t)=−27t3FeR+K+ZΓ(3F+1). | (4.21) |
The final solution is represented as follows:
P(R,K,Z,t)=P0(R,K,Z,t)+P1(R,Z,K,t)+P2(R,K,Z,t)+P3(R,K,Z,t)+⋯, | (4.22) |
P(R,K,Z,t)=eR+K+Z−3tFeR+K+ZΓ(F+1)+9t2FeR+K+ZΓ(2F+1)−27t3FeR+K+ZΓ(3F+1)+⋯. | (4.23) |
Example 4.2. ∙ Implementation of MRPSM
Consider the system of nonlinear PDEs
DFtP1(R,t)+∂3P1(R,t)∂R3−3P2(R,t)∂P2(R,t)∂R+6P1(R,t)∂P1(R,t)∂R=0,DFtP2(R,t)+∂3P2(R,t)∂R3+3P1(R,t)∂P2(R,t)∂R=0 where 0<F≤1. | (4.24) |
The IC's are given as
P1(R,0)=4c2ecR(ecR+1)2,P2(R,0)=4c2ecR(ecR+1)2. | (4.25) |
Equation (4.24) is subjected to MT, and using Eq (4.25), we get the following result:
P1(R,s)+4c2ecR(ecR+1)2s+1sF[∂3P1(R,s)∂R3]−3sFMt[M−1tP2(R,s)×∂M−1tP2(R,s)∂R]+6sFMt[M−1tP1(R,s)×∂M−1tP1(R,s)∂R]=0,P2(R,s)−4c2ecR(ecR+1)2s+1sF[∂3P2(R,s)∂R3]+3sFMt[M−1tP1(R,s)×∂M−1tP2(R,s)∂R]=0. | (4.26) |
The kth term's series is represented as
P1(R,s)=4c2ecR(ecR+1)2s+k∑r=1fr(R,s)srF+1,P2(R,s)=4c2ecR(ecR+1)2s+k∑r=1gr(R,s)srF+1, r=1,2,3,4⋯. | (4.27) |
The residual function of Mohand is given by
AtRes(R,s)=P1(R,s)+4c2ecR(ecR+1)2s+1sF[∂3P1(R,s)∂R3]−3sFMt[M−1tP2(R,s)×∂M−1tP2(R,s)∂R]+6sFMt[M−1tP1(R,s)×∂M−1tP1(R,s)∂R]=0,AtRes(R,s)=P2(R,s)−4c2ecR(ecR+1)2s+1sF[∂3P2(R,s)∂R3]+3sFMt[M−1tP1(R,s)×∂M−1tP2(R,s)∂R]=0, | (4.28) |
and the kth-MRFs as
AtResk(R,s)=P1k(R,s)+4c2ecR(ecR+1)2s+1sF[∂3P1k(R,s)∂R3]−3sFMt[M−1tP2k(R,s)×∂M−1tP2k(R,s)∂R]+6sFMt[M−1tP1k(R,s)×∂M−1tP1k(R,s)∂R]=0,AtResk(R,s)=P2k(R,s)−4c2ecR(ecR+1)2s+1sF[∂3P2k(R,s)∂R3]+3sFMt[M−1tP1k(R,s)×∂M−1tP2k(R,s)∂R]=0. | (4.29) |
Now, we use these steps to find the values of fr(R,K,Z,s) and gr(R,K,Z,s) for r=1,2,3,...: Put the rth-Mohand residual function Eq (4.29) for the rth-truncated series Eq (4.27), and then multiply the equation by srF+1, and solve MtResP1,r(R,s)=0 and MtResP2,r(R,s)=0 for r=1,2,3,⋯. Using this procedure, we obtain
f1(R,s)=4c5ecR(ecR−1)(ecR+1)3,g1(R,s)=4c5ecR(ecR−1)(ecR+1)3, | (4.30) |
f2(R,s)=4c8ecR(−4ecR+e2cR+1)(ecR+1)4,g2(R,s)=4c8ecR(−4ecR+e2cR+1)(ecR+1)4, | (4.31) |
and so on.
The values of Eqs (4.30) and (4.31) are inserted into Eq (4.27) to obtain the following result:
P1(R,s)=4c2ecRs(ecR+1)2+4c5ecR(ecR−1)sF+1(ecR+1)3+4c8ecR(−4ecR+e2cR+1)s2F+1(ecR+1)4+⋯,P2(R,s)=4c2ecRs(ecR+1)2+4c5ecR(ecR−1)sF+1(ecR+1)3+4c8ecR(−4ecR+e2cR+1)s2F+1(ecR+1)4+⋯. | (4.32) |
Using IMT, we obtain the final solution:
P1(R,t)=4c2ecR(ecR+1)2+4c5tFecR(ecR−1)Γ(F+1)(ecR+1)3+4c8t2FecR(−4ecR+e2cR+1)Γ(2F+1)(ecR+1)4+⋯,P2(R,t)=4c2ecR(ecR+1)2+4c5tFecR(ecR−1)Γ(F+1)(ecR+1)3+4c8t2FecR(−4ecR+e2cR+1)Γ(2F+1)(ecR+1)4+⋯. | (4.33) |
∙ Implementation of MTIM
Consider the system of nonlinear PDEs
DFtP1(R,t)=−∂3P1(R,t)∂R3+3P2(R,t)∂P2(R,t)∂R−6P1(R,t)∂P1(R,t)∂R,DFtP2(R,t)=−∂3P2(R,t)∂R3−3P1(R,t)∂P2(R,t)∂R, where 0<F≤1. | (4.34) |
The IC's are given as
P1(R,0)=4c2ecR(ecR+1)2,P2(R,0)=4c2ecR(ecR+1)2. | (4.35) |
MT is used on Eq (4.34), giving
M[DFtP1(R,t)]=1sF(m−1∑k=0P(k)1(R,0)s2−F+k+M[−∂3P1(R,t)∂R3+3P2(R,t)∂P2(R,t)∂R−6P1(R,t)∂P1(R,t)∂R]),M[DFtP2(R,t)]=1sF(m−1∑k=0P(k)2(R,0)s2−F+k+M[−∂3P2(R,t)∂R3−3P1(R,t)∂P2(R,t)∂R]). | (4.36) |
Applying IMT on Eq (4.36), we obtain
P1(R,t)=M−1[1sF(m−1∑k=0P(k)1(R,0)s2−F+k+M[−∂3P1(R,t)∂R3+3P2(R,t)∂P2(R,t)∂R−6P1(R,t)∂P1(R,t)∂R])],P2(R,t)=M−1[1sF(m−1∑k=0P(k)2(R,0)s2−F+k+M[−∂3P2(R,t)∂R3−3P1(R,t)∂P2(R,t)∂R])]. | (4.37) |
Recursively applying the MT, we obtain
P10(R,t)=M−1[1sF(m−1∑k=0P(k)1(R,0)s2−F+k)]=M−1[P1(R,0)s2]=4c2ecR(ecR+1)2, |
P20(R,t)=M−1[1sF(m−1∑k=0P(k)2(R,0)s2−F+k)]=M−1[P2(R,0)s2]=4c2ecR(ecR+1)2. |
The RL integral is implemented on Eq (4.34), giving
P1(R,t)=4c2ecR(ecR+1)2−M[−∂3P1(R,t)∂R3+3P2(R,t)∂P2(R,t)∂R−6P1(R,t)∂P1(R,t)∂R],P2(R,t)=4c2ecR(ecR+1)2−M[−∂3P2(R,t)∂R3−3P1(R,t)∂P2(R,t)∂R]. | (4.38) |
By using the MTIM approach, we obtain the following terms:
P10(R,t)=4c2ecR(ecR+1)2,P11(R,t)=c5tFtanh(cR2)sech2(cR2)Γ(F+1),P12(R,t)=−c8t2F(3sech2(cR2)−2)(3c3tFΓ(2F+1)2tanh(cR2)sech2(cR2)+Γ(F+1)2Γ(3F+1))Γ(F+1)2Γ(2F+1)Γ(3F+1)(cosh(cR)+1). | (4.39) |
P20(R,t)=4c2ecR(ecR+1)2,P21(R,t)=c5tFtanh(cR2)sech2(cR2)Γ(F+1),P22(R,t)=−c8t2F(3sech2(cR2)−2)(3c3tFΓ(2F+1)2tanh(cR2)sech2(cR2)+Γ(F+1)2Γ(3F+1))Γ(F+1)2Γ(2F+1)Γ(3F+1)(cosh(cR)+1). | (4.40) |
The final solution is represented as follows:
P1(R,t)=P10(R,t)+P11(R,t)+P12(R,t)+⋯, | (4.41) |
P2(R,t)=P20(R,t)+P21(R,t)+P22(R,t)+⋯. | (4.42) |
P1(R,t)=4c2ecR(ecR+1)2+c5tFtanh(cR2)sech2(cR2)Γ(F+1)−c8t2F(3sech2(cR2)−2)(3c3tFΓ(2F+1)2tanh(cR2)sech2(cR2)+Γ(F+1)2Γ(3F+1))Γ(F+1)2Γ(2F+1)Γ(3F+1)(cosh(cR)+1)+⋯, | (4.43) |
P2(R,t)=4c2ecR(ecR+1)2+c5tFtanh(cR2)sech2(cR2)Γ(F+1)−c8t2F(3sech2(cR2)−2)(3c3tFΓ(2F+1)2tanh(cR2)sech2(cR2)+Γ(F+1)2Γ(3F+1))Γ(F+1)2Γ(2F+1)Γ(3F+1)(cosh(cR)+1)+⋯. | (4.44) |
The graphical and tabular results offer a comprehensive evaluation of the effectiveness and accuracy of the MTIM and MRPSM for solving FPDEs.
Figures 1 and 2 demonstrate the influence of fractional order on the solutions of the equation P(R,K,Z,t) for specific values of the parameters K, Z, and t. Both 3D and 2D plots highlight the effectiveness of MTIM and MRPSM in accurately capturing the behavior of the solution for varying fractional orders. Figures 3 and 4 provide a comparison between the exact solution and the solutions obtained via MTIM and MRPSM. The close agreement between these solutions demonstrates the reliability of the proposed methods for fractional PDEs. Figures 5 and 6 offer further insights into the effect of fractional order on MRPSM solutions for two distinct cases, P1 and P2. These plots indicate that MRPSM is highly sensitive to fractional-order variations, which enhances its adaptability for different problems. Figures 7 and 8 depict the results for MTIM in comparison with MRPSM for P1 and P2 under different conditions. The strong correlation between the solutions obtained by the two methods further validates the applicability of both techniques. Figures 9 and 10 show a direct comparison of MRPSM and MTIM for the same fractional order, demonstrating their consistency across both 2D and 3D perspectives. The graphical results affirm that the methods provide nearly identical results, emphasizing their robustness.
Table 1 presents an absolute error comparison for the solutions of example 1 using MTIM and MRPSM. The minimal error values indicate that both methods provide highly accurate solutions, making them practical for solving complex fractional PDEs. Table 2 extends this error comparison to example 2, where both P1 and P2 are evaluated. Once again, the absolute errors are minimal, supporting the precision and reliability of MTIM and MRPSM for different cases. In conclusion, the graphical and tabular results strongly support the efficacy of the proposed methods. MTIM and MRPSM not only simplify the solution process for fractional PDEs, but also provide highly accurate and adaptable solutions. The methods demonstrate strong potential for application in various scientific and engineering fields requiring the analysis of fractional-order systems.
t | R | MRPSMF=1 | MTIMF=1 | Exact | MRPSM ErrorF=1 | MTIM ErrorF=1 |
0.01 | 0.10 | 1.30996440544749 | 1.30996440544749 | 1.30996445073324 | 4.528574915063×10−8 | 4.528574915063×10−8 |
0.35 | 1.68202759155083 | 1.68202759155083 | 1.68202764969888 | 5.814805303927×10−8 | 5.814805303927×10−8 | |
0.60 | 2.15976617912133 | 2.15976617912133 | 2.15976625378491 | 7.466357798691×10−8 | 7.466357798691×10−8 | |
0.85 | 2.77319466809436 | 2.77319466809436 | 2.77319476396429 | 9.586993199306×10−8 | 9.586993199306×10−8 | |
0.03 | 0.10 | 1.23367443521972 | 1.23367443521972 | 1.23367805995674 | 3.624737017871×10−6 | 3.624737017871×10−6 |
0.35 | 1.58406933074002 | 1.58406933074002 | 1.58407398499448 | 4.654254460056×10−6 | 4.654254460056×10−6 | |
0.60 | 2.03398528246572 | 2.03398528246572 | 2.03399125864675 | 5.976181022049×10−6 | 5.976181022049×10−6 | |
0.85 | 2.61168879985479 | 2.61168879985479 | 2.61169647342311 | 7.673568327426×10−6 | 7.673568327426×10−6 | |
0.05 | 0.10 | 1.16180660244557 | 1.16180660244557 | 1.16183424272828 | 2.764028271173×10−5 | 2.764028271173×10−5 |
0.35 | 1.49178920681574 | 1.49178920681574 | 1.49182469764127 | 3.549082552645×10−5 | 3.549082552645×10−5 | |
0.60 | 1.91549525789186 | 1.91549525789186 | 1.91554082901389 | 4.557112203484×10−5 | 4.557112203484×10−5 | |
0.85 | 2.45954459667798 | 2.45954459667798 | 2.45960311115694 | 5.851447895999×10−5 | 5.851447895999×10−5 | |
0.07 | 0.10 | 1.09406933762259 | 1.09406933762259 | 1.09417428370521 | 1.049460826105×10−4 | 1.049460826105×10−4 |
0.35 | 1.40481283712613 | 1.40481283712613 | 1.40494759056359 | 1.347534374536×10−4 | 1.347534374536×10−4 | |
0.60 | 1.80381538855917 | 1.80381538855917 | 1.80398841539785 | 1.730268386763×10−4 | 1.730268386763×10−4 | |
0.85 | 2.31614480592246 | 2.31614480592246 | 2.31636697678109 | 2.221708586298×10−4 | 2.221708586298×10−4 | |
0.10 | 0.10 | 0.99957044701003 | 0.99957044701003 | 1.00000000000000 | 4.295529899697×10−4 | 4.295529899697×10−4 |
0.35 | 1.28347385973080 | 1.28347385973080 | 1.28402541668774 | 5.515569569352×10−4 | 5.515569569352×10−4 | |
0.60 | 1.64801305754867 | 1.64801305754867 | 1.64872127070012 | 7.082131514557×10−4 | 7.082131514557×10−4 | |
0.85 | 2.11609065292577 | 2.11609065292577 | 2.11700001661267 | 9.093636869019×10−4 | 9.093636869019×10−4 |
t | R | MRPSMF=0.7 | MTIMF=0.7 | MRPSMF=1.0 | MTIMF=1.0 | |MRPSM−MTIM|F=0.7 | |MRPSM−MTIM|F=1.0 |
0.1 | 0.10 | 0.2499527853 | 0.2499526861 | 0.2499121337 | 0.2499121276 | 9.9242792095×10−8 | 6.0831994996×10−8 |
0.35 | 0.2486293113 | 0.2486289768 | 0.2483568594 | 0.2483568389 | 3.3452402567×10−7 | 2.0505029479×10−8 | |
0.60 | 0.2454001942 | 0.2453996649 | 0.2449043498 | 0.2449043174 | 5.2929990035×10−7 | 3.2444037623×10−8 | |
0.85 | 0.2403639798 | 0.2403633177 | 0.2396597476 | 0.2396597070 | 6.6212486968×10−7 | 4.0585694638×10−8 | |
0.3 | 0.10 | 0.2499229699 | 0.2499219730 | 0.2499903237 | 0.2499901595 | 9.9690462096×10−7 | 1.6424638585×10−7 |
0.35 | 0.2490954767 | 0.2490921164 | 0.2488224297 | 0.2488218760 | 3.3603301558×10−6 | 5.5363579581×10−7 | |
0.60 | 0.2463478586 | 0.2463425417 | 0.2457429414 | 0.2457420654 | 5.3168749624×10−6 | 8.7598901432×10−7 | |
0.85 | 0.2417642150 | 0.2417575638 | 0.2408460059 | 0.2408449101 | 6.6511162011×10−6 | 1.0958137564×10−6 | |
0.5 | 0.10 | 0.2497783238 | 0.2497754095 | 0.2499905839 | 0.2499898235 | 2.9143115888×10−6 | 7.6039993426×10−7 |
0.35 | 0.2493515651 | 0.2493417416 | 0.2492122418 | 0.2492096786 | 9.8234564366×10−6 | 2.5631286842×10−6 | |
0.60 | 0.2469966017 | 0.2469810585 | 0.2465102198 | 0.2465061643 | 1.5543142236×10−5 | 4.0555046959×10−6 | |
0.85 | 0.2427855197 | 0.2427660760 | 0.2419673860 | 0.2419623128 | 1.9443610367×10−5 | 5.0732118353×10−6 | |
0.7 | 0.10 | 0.2495611717 | 0.2495552642 | 0.2499129141 | 0.2499108276 | 5.9075153423×10−6 | 2.0865374195×10−6 |
0.35 | 0.2494909149 | 0.2494710021 | 0.2495262957 | 0.2495192624 | 1.9912839737×10−5 | 7.0332251095×10−6 | |
0.60 | 0.2474879230 | 0.2474564159 | 0.2472061853 | 0.2471950570 | 3.1507046666×10−5 | 1.1128304885×10−5 | |
0.85 | 0.2436133438 | 0.2435739303 | 0.2430238880 | 0.2430099671 | 3.9413570944×10−5 | 1.3920893276×10−5 | |
1.0 | 0.10 | 0.2491344789 | 0.2491219850 | 0.2496502911 | 0.2496442079 | 1.2493927289×10−5 | 6.0831994737×10−6 |
0.35 | 0.2495497378 | 0.2495076238 | 0.2498553300 | 0.2498348249 | 4.2114079676×10−5 | 2.0505029473×10−5 | |
0.60 | 0.2480300251 | 0.2479633902 | 0.2481164215 | 0.2480839774 | 6.6634909492×10−5 | 3.2444037567×10−5 | |
0.85 | 0.2446212213 | 0.2445378648 | 0.2444869946 | 0.2444464089 | 8.3356582432×10−5 | 4.0585694683×10−5 |
In conclusion, this study has demonstrated the efficacy of MTIM and MRPSM in solving fractional-order PDEs and systems of PDEs. By employing the Caputo operator to define fractional derivatives, we have shown that these methods provide a powerful and flexible framework for tackling complex problems in applied mathematics. The results obtained through our examples confirm that MTIM and MRPSM not only simplify the solution process, but also improve the accuracy and reliability of the solutions. These methods hold significant potential for application in various scientific and engineering disciplines, particularly in situations where traditional approaches may fall short. Future work can extend these techniques to more complex and higher-dimensional problems, further establishing their utility in the field of fractional calculus and beyond.
A.S.A and H.Y Conceptualization, A.M.M; formal analysis, A.S.A. investigation, A.S.A. project administration, A.S.A. validation, visualization, H.Y. writing-review & editing; A.M.M; Data curation, H.Y. resources, A.M.M; validation, H.Y. software, H.Y. visualization, A.M.M; resources, H.Y. project administration, H.Y. writing-review & editing, H.Y. funding. All authors have read and agreed to the published version of the manuscript.
This work was supported by Princess Nourah bint Abdulrahman University Researchers Supporting Project number (PNURSP2024R183), Princess Nourah bint Abdulrahman University, Riyadh, Saudi Arabia. This work was supported by the Deanship of Scientific Research, Vice Presidency for Graduate Studies and Scientific Research, King Faisal University, Saudi Arabia (KFU242276).
This work was supported by Princess Nourah bint Abdulrahman University Researchers Supporting Project number (PNURSP2024R183), Princess Nourah bint Abdulrahman University, Riyadh, Saudi Arabia. This work was supported by the Deanship of Scientific Research, Vice Presidency for Graduate Studies and Scientific Research, King Faisal University, Saudi Arabia (KFU242276).
The authors declare that they have no conflicts of interest.
[1] |
L. H. Zhu, B. X. Wang, Stability analysis of a SAIR rumor spreading model with control strategies in online social networks, Inform. Sciences, 526 (2020), 1–19. https://doi.org/10.1016/j.ins.2020.03.076 doi: 10.1016/j.ins.2020.03.076
![]() |
[2] |
Y. C. Liu, P. Z. Zhang, L. Shi, J. P. Gong, A survey of information dissemination model, datasets, and insight, Mathematics, 11 (2023), 3707. https://doi.org/10.3390/math11173707 doi: 10.3390/math11173707
![]() |
[3] |
S. Z. Yu, Z. Y. Yu, H. J. Jiang, S. Yang, The dynamics and control of 2i2sr rumor spreading models in multilingual online social networks, Inform. Sciences, 581 (2021), 18–41. https://doi.org/10.1016/j.ins.2021.08.096 doi: 10.1016/j.ins.2021.08.096
![]() |
[4] |
G. Y. Jiang, S. P. Li, M. L. Li, Dynamic rumor spreading of public opinion reversal on weibo based on a two-stage SPNR model, Physica A, 558 (2020), 125005. https://doi.org/10.1016/j.physa.2020.125005 doi: 10.1016/j.physa.2020.125005
![]() |
[5] |
M. Ghosh, P. Das, P. Das, A comparative study of deterministic and stochastic dynamics of rumor propagation model with counter-rumor spreader, Nonlinear Dyn., 111 (2023), 16875–16894. https://doi.org/10.1007/s11071-023-08768-1 doi: 10.1007/s11071-023-08768-1
![]() |
[6] |
J. Ding, N. Gul, G. Liu, T. Saeed, Dynamical aspects of a delayed computer viruses model with horizontal and vertical dissemination over internet, Fractals, 31 (2023), 2340092. https://doi.org/10.1142/S0218348X23400923 doi: 10.1142/S0218348X23400923
![]() |
[7] |
M. U. Rahman, G. Alhawael, Y. Karaca, Multicompartmental analysis of middle eastern respiratory syndrome coronavirus model under fractional operator with next-generation matrix methods, Fractals, 31 (2023), 2340093. https://doi.org/10.1142/S0218348X23400935 doi: 10.1142/S0218348X23400935
![]() |
[8] | D. Bernoulli, Essai d'une nouvelle analyse de la mortalité causée par la petite vérole, et des avantages de l'inoculation pour la prévenir, Méin. de I'Acad. Roy. des Sciences de I'Année, 98 (1760), 11145. |
[9] |
A. Raza, A. Ahmadian, M. Rafiq, M. C. Ang, S. Salahshour, M. Pakdaman, The impact of delay strategies on the dynamics of coronavirus pandemic model with nonlinear incidence rate, Fractals, 30 (2022), 2240121. https://doi.org/10.1142/S0218348X22401211 doi: 10.1142/S0218348X22401211
![]() |
[10] |
H. Alqahtani, Q. Badshah, G. U. Rahman, D. Baleanu, S. Sakhi, Threshold dynamics bifurcation analysis of the epidemic model of mers-CoV, Fractals, 31 (2023), 2340167. https://doi.org/10.1142/S0218348X23401679 doi: 10.1142/S0218348X23401679
![]() |
[11] |
Y. M. Guo, T. T. Li, Modeling the competitive transmission of the Omicron strain and Delta strain of COVID-19, J. Math. Anal. Appl., 526 (2023), 127283. https://doi.org/10.1016/j.jmaa.2023.127283 doi: 10.1016/j.jmaa.2023.127283
![]() |
[12] |
Y. M. Guo, T. T. Li, Modeling and dynamic analysis of novel coronavirus pneumonia (COVID-19) in China, J. Appl. Math. Comput., 68 (2022), 2641–2666. https://doi.org/10.1007/s12190-021-01611-z doi: 10.1007/s12190-021-01611-z
![]() |
[13] |
L. H. Zhu, W. X. Zheng, S. L. Shen, Dynamical analysis of a si epidemic-like propagation model with non-smooth control, Chaos Soliton. Fract., 169 (2023), 113273. https://doi.org/10.1016/j.chaos.2023.113273 doi: 10.1016/j.chaos.2023.113273
![]() |
[14] |
L. H. Zhu, F. Yang, G. Guan, Z. D. Zhang, Modeling the dynamics of rumor diffusion over complex networks, Inform. Sciences, 562 (2021), 240–258. https://doi.org/10.1016/j.ins.2020.12.071 doi: 10.1016/j.ins.2020.12.071
![]() |
[15] |
X. R. Ma, S. L. Shen, L. H. Zhu, Complex dynamic analysis of a reaction-diffusion network information propagation model with non-smooth control, Inform. Sciences, 622 (2023), 1141–1161. https://doi.org/10.1016/j.ins.2022.12.013 doi: 10.1016/j.ins.2022.12.013
![]() |
[16] |
D. J. Daley, D. G. Kendall, Epidemics and rumours, Nature, 204 (1964), 1118. https://doi.org/10.1038/2041118a0 doi: 10.1038/2041118a0
![]() |
[17] | D. P. Maki, Mathematical models and applications: with emphasis on the social, life, and management sciences, New Haven: Pearson College Div, 1973. |
[18] |
A. Sudbury, The proportion of the population never hearing a rumour, J. Appl. Probab., 22 (1985), 443–446. https://doi.org/10.2307/3213787 doi: 10.2307/3213787
![]() |
[19] |
X. W. Wang, Y. Q. Li, J. X. Li, Y. T. Liu, C. C. Qiu, A rumor reversal model of online health information during the Covid-19 epidemic, Inform. Process. Manag., 58 (2021), 102731. https://doi.org/10.1016/j.ipm.2021.102731 doi: 10.1016/j.ipm.2021.102731
![]() |
[20] |
H. X. Ding, L. Xie, Simulating rumor spreading and rebuttal strategy with rebuttal forgetting: An agent-based modeling approach, Physica A, 612 (2023), 128488. https://doi.org/10.1016/j.physa.2023.128488 doi: 10.1016/j.physa.2023.128488
![]() |
[21] |
G. F. de Arruda, L. G. S. Jeub, A. S. Mata, F. A. Rodrigues, Y. Moreno, From subcritical behavior to a correlation-induced transition in rumor models, Nat. Commun., 13 (2022), 3049. https://doi.org/10.1038/s41467-022-30683-z doi: 10.1038/s41467-022-30683-z
![]() |
[22] |
L. A. Huo, S. J. Chen, L. J. Zhao, Dynamic analysis of the rumor propagation model with consideration of the wise man and social reinforcement, Physica A, 571 (2021), 125828. https://doi.org/10.1016/j.physa.2021.125828 doi: 10.1016/j.physa.2021.125828
![]() |
[23] |
F. L. Yin, X. Y. Jiang, X. Q. Qian, X. Y. Xia, Y. Y. Pan, J. H. Wu, Modeling and quantifying the influence of rumor and counter-rumor on information propagation dynamics, Chaos Soliton. Fract., 162 (2022), 112392. https://doi.org/10.1016/j.chaos.2022.112392 doi: 10.1016/j.chaos.2022.112392
![]() |
[24] |
S. S. Chen, H. J. Jiang, L. Li, J. R. Li, Dynamical behaviors and optimal control of rumor propagation model with saturation incidence on heterogeneous networks, Chaos Soliton. Fract., 140 (2020), 110206. https://doi.org/10.1016/j.chaos.2020.110206 doi: 10.1016/j.chaos.2020.110206
![]() |
[25] |
Y. Y. Cheng, L. A. Huo, L. J. Zhao, Dynamical behaviors and control measures of rumor-spreading model in consideration of the infected media and time delay, Inform. Sciences, 564 (2021), 237–253. https://doi.org/10.1016/j.ins.2021.02.047 doi: 10.1016/j.ins.2021.02.047
![]() |
[26] |
Y. Y. Cheng, L. A. Huo, L. J. Zhao, Stability analysis and optimal control of rumor spreading model under media coverage considering time delay and pulse vaccination, Chaos Soliton. Fract., 157 (2022), 111931. https://doi.org/10.1016/j.chaos.2022.111931 doi: 10.1016/j.chaos.2022.111931
![]() |
[27] |
Y. M. Guo, T. T. Li, Dynamics and optimal control of an online game addiction model with considering family education, AIMS Mathematics, 7 (2022), 3745–3770. https://doi.org/10.3934/math.2022208 doi: 10.3934/math.2022208
![]() |
[28] |
Y. F. Dong, L. A. Huo, L. Zhao, An improved two-layer model for rumor propagation considering time delay and event-triggered impulsive control strategy, Chaos Soliton. Fract., 164 (2022), 112711. https://doi.org/10.1016/j.chaos.2022.112711 doi: 10.1016/j.chaos.2022.112711
![]() |
[29] |
W. Q. Pan, W. J. Yan, Y. H. Hu, R. M. He, L. B. Wu, Dynamic analysis of a sidrw rumor propagation model considering the effect of media reports and rumor refuters, Nonlinear Dyn., 111 (2023), 3925–3936. https://doi.org/10.1007/s11071-022-07947-w doi: 10.1007/s11071-022-07947-w
![]() |
[30] |
L. H. Zhu, L. He, Pattern formation in a reaction-diffusion rumor propagation system with allee effect and time delay, Nonlinear Dyn., 107 (2022), 3041–3063. https://doi.org/10.1007/s11071-021-07106-7 doi: 10.1007/s11071-021-07106-7
![]() |
[31] |
L. H. Zhu, X. W. Wang, Z. D. Zhang, C. X. Lei, Spatial dynamics and optimization method for a rumor propagation model in both homogeneous and heterogeneous environment, Nonlinear Dyn., 105 (2021), 3791–3817. https://doi.org/10.1007/s11071-021-06782-9 doi: 10.1007/s11071-021-06782-9
![]() |
[32] |
L. H. Zhu, W. S. Liu, Z. D. Zhang, Delay differential equations modeling of rumor propagation in both homogeneous and heterogeneous networks with a forced silence function, Appl. Math. Comput., 370 (2020), 124925. https://doi.org/10.1016/j.amc.2019.124925 doi: 10.1016/j.amc.2019.124925
![]() |
1. | Yousef Jawarneh, Humaira Yasmin, Ali M. Mahnashi, A new solitary wave solution of the fractional phenomena Bogoyavlenskii equation via Bäcklund transformation, 2024, 9, 2473-6988, 35308, 10.3934/math.20241678 |
t | R | MRPSMF=1 | MTIMF=1 | Exact | MRPSM ErrorF=1 | MTIM ErrorF=1 |
0.01 | 0.10 | 1.30996440544749 | 1.30996440544749 | 1.30996445073324 | 4.528574915063×10−8 | 4.528574915063×10−8 |
0.35 | 1.68202759155083 | 1.68202759155083 | 1.68202764969888 | 5.814805303927×10−8 | 5.814805303927×10−8 | |
0.60 | 2.15976617912133 | 2.15976617912133 | 2.15976625378491 | 7.466357798691×10−8 | 7.466357798691×10−8 | |
0.85 | 2.77319466809436 | 2.77319466809436 | 2.77319476396429 | 9.586993199306×10−8 | 9.586993199306×10−8 | |
0.03 | 0.10 | 1.23367443521972 | 1.23367443521972 | 1.23367805995674 | 3.624737017871×10−6 | 3.624737017871×10−6 |
0.35 | 1.58406933074002 | 1.58406933074002 | 1.58407398499448 | 4.654254460056×10−6 | 4.654254460056×10−6 | |
0.60 | 2.03398528246572 | 2.03398528246572 | 2.03399125864675 | 5.976181022049×10−6 | 5.976181022049×10−6 | |
0.85 | 2.61168879985479 | 2.61168879985479 | 2.61169647342311 | 7.673568327426×10−6 | 7.673568327426×10−6 | |
0.05 | 0.10 | 1.16180660244557 | 1.16180660244557 | 1.16183424272828 | 2.764028271173×10−5 | 2.764028271173×10−5 |
0.35 | 1.49178920681574 | 1.49178920681574 | 1.49182469764127 | 3.549082552645×10−5 | 3.549082552645×10−5 | |
0.60 | 1.91549525789186 | 1.91549525789186 | 1.91554082901389 | 4.557112203484×10−5 | 4.557112203484×10−5 | |
0.85 | 2.45954459667798 | 2.45954459667798 | 2.45960311115694 | 5.851447895999×10−5 | 5.851447895999×10−5 | |
0.07 | 0.10 | 1.09406933762259 | 1.09406933762259 | 1.09417428370521 | 1.049460826105×10−4 | 1.049460826105×10−4 |
0.35 | 1.40481283712613 | 1.40481283712613 | 1.40494759056359 | 1.347534374536×10−4 | 1.347534374536×10−4 | |
0.60 | 1.80381538855917 | 1.80381538855917 | 1.80398841539785 | 1.730268386763×10−4 | 1.730268386763×10−4 | |
0.85 | 2.31614480592246 | 2.31614480592246 | 2.31636697678109 | 2.221708586298×10−4 | 2.221708586298×10−4 | |
0.10 | 0.10 | 0.99957044701003 | 0.99957044701003 | 1.00000000000000 | 4.295529899697×10−4 | 4.295529899697×10−4 |
0.35 | 1.28347385973080 | 1.28347385973080 | 1.28402541668774 | 5.515569569352×10−4 | 5.515569569352×10−4 | |
0.60 | 1.64801305754867 | 1.64801305754867 | 1.64872127070012 | 7.082131514557×10−4 | 7.082131514557×10−4 | |
0.85 | 2.11609065292577 | 2.11609065292577 | 2.11700001661267 | 9.093636869019×10−4 | 9.093636869019×10−4 |
t | R | MRPSMF=0.7 | MTIMF=0.7 | MRPSMF=1.0 | MTIMF=1.0 | |MRPSM−MTIM|F=0.7 | |MRPSM−MTIM|F=1.0 |
0.1 | 0.10 | 0.2499527853 | 0.2499526861 | 0.2499121337 | 0.2499121276 | 9.9242792095×10−8 | 6.0831994996×10−8 |
0.35 | 0.2486293113 | 0.2486289768 | 0.2483568594 | 0.2483568389 | 3.3452402567×10−7 | 2.0505029479×10−8 | |
0.60 | 0.2454001942 | 0.2453996649 | 0.2449043498 | 0.2449043174 | 5.2929990035×10−7 | 3.2444037623×10−8 | |
0.85 | 0.2403639798 | 0.2403633177 | 0.2396597476 | 0.2396597070 | 6.6212486968×10−7 | 4.0585694638×10−8 | |
0.3 | 0.10 | 0.2499229699 | 0.2499219730 | 0.2499903237 | 0.2499901595 | 9.9690462096×10−7 | 1.6424638585×10−7 |
0.35 | 0.2490954767 | 0.2490921164 | 0.2488224297 | 0.2488218760 | 3.3603301558×10−6 | 5.5363579581×10−7 | |
0.60 | 0.2463478586 | 0.2463425417 | 0.2457429414 | 0.2457420654 | 5.3168749624×10−6 | 8.7598901432×10−7 | |
0.85 | 0.2417642150 | 0.2417575638 | 0.2408460059 | 0.2408449101 | 6.6511162011×10−6 | 1.0958137564×10−6 | |
0.5 | 0.10 | 0.2497783238 | 0.2497754095 | 0.2499905839 | 0.2499898235 | 2.9143115888×10−6 | 7.6039993426×10−7 |
0.35 | 0.2493515651 | 0.2493417416 | 0.2492122418 | 0.2492096786 | 9.8234564366×10−6 | 2.5631286842×10−6 | |
0.60 | 0.2469966017 | 0.2469810585 | 0.2465102198 | 0.2465061643 | 1.5543142236×10−5 | 4.0555046959×10−6 | |
0.85 | 0.2427855197 | 0.2427660760 | 0.2419673860 | 0.2419623128 | 1.9443610367×10−5 | 5.0732118353×10−6 | |
0.7 | 0.10 | 0.2495611717 | 0.2495552642 | 0.2499129141 | 0.2499108276 | 5.9075153423×10−6 | 2.0865374195×10−6 |
0.35 | 0.2494909149 | 0.2494710021 | 0.2495262957 | 0.2495192624 | 1.9912839737×10−5 | 7.0332251095×10−6 | |
0.60 | 0.2474879230 | 0.2474564159 | 0.2472061853 | 0.2471950570 | 3.1507046666×10−5 | 1.1128304885×10−5 | |
0.85 | 0.2436133438 | 0.2435739303 | 0.2430238880 | 0.2430099671 | 3.9413570944×10−5 | 1.3920893276×10−5 | |
1.0 | 0.10 | 0.2491344789 | 0.2491219850 | 0.2496502911 | 0.2496442079 | 1.2493927289×10−5 | 6.0831994737×10−6 |
0.35 | 0.2495497378 | 0.2495076238 | 0.2498553300 | 0.2498348249 | 4.2114079676×10−5 | 2.0505029473×10−5 | |
0.60 | 0.2480300251 | 0.2479633902 | 0.2481164215 | 0.2480839774 | 6.6634909492×10−5 | 3.2444037567×10−5 | |
0.85 | 0.2446212213 | 0.2445378648 | 0.2444869946 | 0.2444464089 | 8.3356582432×10−5 | 4.0585694683×10−5 |
t | R | MRPSMF=1 | MTIMF=1 | Exact | MRPSM ErrorF=1 | MTIM ErrorF=1 |
0.01 | 0.10 | 1.30996440544749 | 1.30996440544749 | 1.30996445073324 | 4.528574915063×10−8 | 4.528574915063×10−8 |
0.35 | 1.68202759155083 | 1.68202759155083 | 1.68202764969888 | 5.814805303927×10−8 | 5.814805303927×10−8 | |
0.60 | 2.15976617912133 | 2.15976617912133 | 2.15976625378491 | 7.466357798691×10−8 | 7.466357798691×10−8 | |
0.85 | 2.77319466809436 | 2.77319466809436 | 2.77319476396429 | 9.586993199306×10−8 | 9.586993199306×10−8 | |
0.03 | 0.10 | 1.23367443521972 | 1.23367443521972 | 1.23367805995674 | 3.624737017871×10−6 | 3.624737017871×10−6 |
0.35 | 1.58406933074002 | 1.58406933074002 | 1.58407398499448 | 4.654254460056×10−6 | 4.654254460056×10−6 | |
0.60 | 2.03398528246572 | 2.03398528246572 | 2.03399125864675 | 5.976181022049×10−6 | 5.976181022049×10−6 | |
0.85 | 2.61168879985479 | 2.61168879985479 | 2.61169647342311 | 7.673568327426×10−6 | 7.673568327426×10−6 | |
0.05 | 0.10 | 1.16180660244557 | 1.16180660244557 | 1.16183424272828 | 2.764028271173×10−5 | 2.764028271173×10−5 |
0.35 | 1.49178920681574 | 1.49178920681574 | 1.49182469764127 | 3.549082552645×10−5 | 3.549082552645×10−5 | |
0.60 | 1.91549525789186 | 1.91549525789186 | 1.91554082901389 | 4.557112203484×10−5 | 4.557112203484×10−5 | |
0.85 | 2.45954459667798 | 2.45954459667798 | 2.45960311115694 | 5.851447895999×10−5 | 5.851447895999×10−5 | |
0.07 | 0.10 | 1.09406933762259 | 1.09406933762259 | 1.09417428370521 | 1.049460826105×10−4 | 1.049460826105×10−4 |
0.35 | 1.40481283712613 | 1.40481283712613 | 1.40494759056359 | 1.347534374536×10−4 | 1.347534374536×10−4 | |
0.60 | 1.80381538855917 | 1.80381538855917 | 1.80398841539785 | 1.730268386763×10−4 | 1.730268386763×10−4 | |
0.85 | 2.31614480592246 | 2.31614480592246 | 2.31636697678109 | 2.221708586298×10−4 | 2.221708586298×10−4 | |
0.10 | 0.10 | 0.99957044701003 | 0.99957044701003 | 1.00000000000000 | 4.295529899697×10−4 | 4.295529899697×10−4 |
0.35 | 1.28347385973080 | 1.28347385973080 | 1.28402541668774 | 5.515569569352×10−4 | 5.515569569352×10−4 | |
0.60 | 1.64801305754867 | 1.64801305754867 | 1.64872127070012 | 7.082131514557×10−4 | 7.082131514557×10−4 | |
0.85 | 2.11609065292577 | 2.11609065292577 | 2.11700001661267 | 9.093636869019×10−4 | 9.093636869019×10−4 |
t | R | MRPSMF=0.7 | MTIMF=0.7 | MRPSMF=1.0 | MTIMF=1.0 | |MRPSM−MTIM|F=0.7 | |MRPSM−MTIM|F=1.0 |
0.1 | 0.10 | 0.2499527853 | 0.2499526861 | 0.2499121337 | 0.2499121276 | 9.9242792095×10−8 | 6.0831994996×10−8 |
0.35 | 0.2486293113 | 0.2486289768 | 0.2483568594 | 0.2483568389 | 3.3452402567×10−7 | 2.0505029479×10−8 | |
0.60 | 0.2454001942 | 0.2453996649 | 0.2449043498 | 0.2449043174 | 5.2929990035×10−7 | 3.2444037623×10−8 | |
0.85 | 0.2403639798 | 0.2403633177 | 0.2396597476 | 0.2396597070 | 6.6212486968×10−7 | 4.0585694638×10−8 | |
0.3 | 0.10 | 0.2499229699 | 0.2499219730 | 0.2499903237 | 0.2499901595 | 9.9690462096×10−7 | 1.6424638585×10−7 |
0.35 | 0.2490954767 | 0.2490921164 | 0.2488224297 | 0.2488218760 | 3.3603301558×10−6 | 5.5363579581×10−7 | |
0.60 | 0.2463478586 | 0.2463425417 | 0.2457429414 | 0.2457420654 | 5.3168749624×10−6 | 8.7598901432×10−7 | |
0.85 | 0.2417642150 | 0.2417575638 | 0.2408460059 | 0.2408449101 | 6.6511162011×10−6 | 1.0958137564×10−6 | |
0.5 | 0.10 | 0.2497783238 | 0.2497754095 | 0.2499905839 | 0.2499898235 | 2.9143115888×10−6 | 7.6039993426×10−7 |
0.35 | 0.2493515651 | 0.2493417416 | 0.2492122418 | 0.2492096786 | 9.8234564366×10−6 | 2.5631286842×10−6 | |
0.60 | 0.2469966017 | 0.2469810585 | 0.2465102198 | 0.2465061643 | 1.5543142236×10−5 | 4.0555046959×10−6 | |
0.85 | 0.2427855197 | 0.2427660760 | 0.2419673860 | 0.2419623128 | 1.9443610367×10−5 | 5.0732118353×10−6 | |
0.7 | 0.10 | 0.2495611717 | 0.2495552642 | 0.2499129141 | 0.2499108276 | 5.9075153423×10−6 | 2.0865374195×10−6 |
0.35 | 0.2494909149 | 0.2494710021 | 0.2495262957 | 0.2495192624 | 1.9912839737×10−5 | 7.0332251095×10−6 | |
0.60 | 0.2474879230 | 0.2474564159 | 0.2472061853 | 0.2471950570 | 3.1507046666×10−5 | 1.1128304885×10−5 | |
0.85 | 0.2436133438 | 0.2435739303 | 0.2430238880 | 0.2430099671 | 3.9413570944×10−5 | 1.3920893276×10−5 | |
1.0 | 0.10 | 0.2491344789 | 0.2491219850 | 0.2496502911 | 0.2496442079 | 1.2493927289×10−5 | 6.0831994737×10−6 |
0.35 | 0.2495497378 | 0.2495076238 | 0.2498553300 | 0.2498348249 | 4.2114079676×10−5 | 2.0505029473×10−5 | |
0.60 | 0.2480300251 | 0.2479633902 | 0.2481164215 | 0.2480839774 | 6.6634909492×10−5 | 3.2444037567×10−5 | |
0.85 | 0.2446212213 | 0.2445378648 | 0.2444869946 | 0.2444464089 | 8.3356582432×10−5 | 4.0585694683×10−5 |