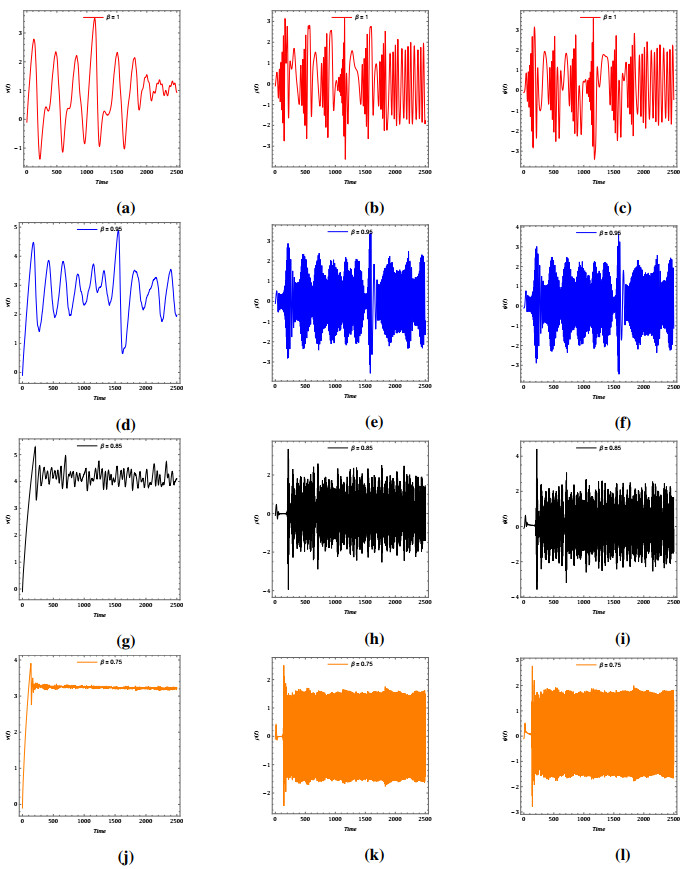
In this study, changes in westerly waves and their connections to increased global warming under the influence of greenhouse gases were investigated via a Caputo fractional four-dimensional atmospheric system. The idea of the existence of chaotic behavior in the westerly wind's motion was depicted. It has been noted that westerlies are becoming stronger due to rising air temperatures. An analysis of the existence, uniqueness, boundedness, stability of equilibrium points, and conservative behavior of the solutions was conducted. To prove the existence of chaos in the modified model, the Lyapunov exponents, Poincaré map, and bifurcation were computed. A sliding mode controller to control the chaos in this novel fractional-order system was designed, and conditions for the global stability of the controlled system with and without external disturbances and uncertainties were derived. The finite-time interval for the system to reach the sliding surface was computed. The developed controller's performance was evaluated with respect to both commensurate and non-commensurate fractional derivatives. In each scenario, the impact of fractional orders was investigated. Numerical simulations were used to support theoretical statements about how the controller affects the system.
Citation: Asharani J. Rangappa, Chandrali Baishya, Reny George, Sina Etemad, Zaher Mundher Yaseen. On the existence, stability and chaos analysis of a novel 4D atmospheric dynamical system in the context of the Caputo fractional derivatives[J]. AIMS Mathematics, 2024, 9(10): 28560-28588. doi: 10.3934/math.20241386
[1] | Junhong Li, Ning Cui . A hyperchaos generated from Rabinovich system. AIMS Mathematics, 2023, 8(1): 1410-1426. doi: 10.3934/math.2023071 |
[2] | A. E. Matouk . Chaos and hidden chaos in a 4D dynamical system using the fractal-fractional operators. AIMS Mathematics, 2025, 10(3): 6233-6257. doi: 10.3934/math.2025284 |
[3] | Hany A. Hosham, Thoraya N. Alharthi . Bifurcation and chaos in simple discontinuous systems separated by a hypersurface. AIMS Mathematics, 2024, 9(7): 17025-17038. doi: 10.3934/math.2024826 |
[4] | Ning Cui, Junhong Li . A new 4D hyperchaotic system and its control. AIMS Mathematics, 2023, 8(1): 905-923. doi: 10.3934/math.2023044 |
[5] | Michael Precious Ineh, Umar Ishtiaq, Jackson Efiong Ante, Mubariz Garayev, Ioan-Lucian Popa . A robust uniform practical stability approach for Caputo fractional hybrid systems. AIMS Mathematics, 2025, 10(3): 7001-7021. doi: 10.3934/math.2025320 |
[6] | Rahat Zarin, Abdur Raouf, Amir Khan, Aeshah A. Raezah, Usa Wannasingha Humphries . Computational modeling of financial crime population dynamics under different fractional operators. AIMS Mathematics, 2023, 8(9): 20755-20789. doi: 10.3934/math.20231058 |
[7] | Kareem Alanazi, Omar Naifar, Raouf Fakhfakh, Abdellatif Ben Makhlouf . Innovative observer design for nonlinear systems using Caputo fractional derivative with respect to another function. AIMS Mathematics, 2024, 9(12): 35533-35550. doi: 10.3934/math.20241686 |
[8] | Michael Precious Ineh, Edet Peter Akpan, Hossam A. Nabwey . A novel approach to Lyapunov stability of Caputo fractional dynamic equations on time scale using a new generalized derivative. AIMS Mathematics, 2024, 9(12): 34406-34434. doi: 10.3934/math.20241639 |
[9] | A. M. Alqahtani, Shivani Sharma, Arun Chaudhary, Aditya Sharma . Application of Caputo-Fabrizio derivative in circuit realization. AIMS Mathematics, 2025, 10(2): 2415-2443. doi: 10.3934/math.2025113 |
[10] | Naimi Abdellouahab, Keltum Bouhali, Loay Alkhalifa, Khaled Zennir . Existence and stability analysis of a problem of the Caputo fractional derivative with mixed conditions. AIMS Mathematics, 2025, 10(3): 6805-6826. doi: 10.3934/math.2025312 |
In this study, changes in westerly waves and their connections to increased global warming under the influence of greenhouse gases were investigated via a Caputo fractional four-dimensional atmospheric system. The idea of the existence of chaotic behavior in the westerly wind's motion was depicted. It has been noted that westerlies are becoming stronger due to rising air temperatures. An analysis of the existence, uniqueness, boundedness, stability of equilibrium points, and conservative behavior of the solutions was conducted. To prove the existence of chaos in the modified model, the Lyapunov exponents, Poincaré map, and bifurcation were computed. A sliding mode controller to control the chaos in this novel fractional-order system was designed, and conditions for the global stability of the controlled system with and without external disturbances and uncertainties were derived. The finite-time interval for the system to reach the sliding surface was computed. The developed controller's performance was evaluated with respect to both commensurate and non-commensurate fractional derivatives. In each scenario, the impact of fractional orders was investigated. Numerical simulations were used to support theoretical statements about how the controller affects the system.
Over the last three decades, a great deal of research has been done on the fascinating nonlinear phenomenon known as chaos. Mathematical modeling, which promotes deeper insights and well-informed decision-making, is regarded as a very effective method of formulating and addressing real-world problems. It has great potential in many fields, including biomedical engineering applications to the human brain and heart [1], comprehensive liquid mixing with low power consumption, circuit systems [2], collapse prevention of power systems, chemistry, physics [2,3], engineering, and climate science. Within the field of climate research, mathematical models facilitate the simulation and comprehension of intricate climate systems, empowering scientists to forecast and examine climate trends. For example, the Lorenz-84 system illustrates the irregularity of the atmosphere [4], and Yong-Guang's study examined a low-order model for Hadley circulation [5]. The complexity and intrinsic unpredictability of weather and climate systems have been acknowledged by chaotic models, which have revolutionized our understanding of atmospheric dynamics. Although challenges remain advancements in computer methods and multidisciplinary studies have the potential to improve our capacity to simulate and forecast atmospheric events. Future atmospheric research will be guided by the insights provided by chaos theory, which provides a more profound understanding of the complex and frequently unpredictable nature of the Earth's atmosphere. The surface of the Earth and the lower atmosphere are warmed by the absorption and reradiation of heat by atmospheric gases such as carbon dioxide (CO2), methane (CH4), and water vapor (H2O). The term "greenhouse effect" refers to this mechanism of heat absorption and radiation. The modeling and control of greenhouse gases (GHGs) have attracted the attention of numerous scholars in recent years, leading to the development of various control strategies.
The concept of fractional differentiation [6] is 300 years old; however, in the last 50 years, it has gained a lot of attention and discussion. One of the most popular trends in applied mathematics has been the investigation of fractional calculus [7,8]. Due to its more accurate explanation of physical occurrences. It has been recently shown that approaches such as integer-order differential operators are not necessarily appropriate for describing complicated and nonlinear phenomena. Fractional calculus has increasingly been applied to describe a wide range of real-world events in fields like economics, biology [9], chemistry, control theory, mechanics, signal and image processing, and electricity. Due to the limitations of existing methods, scientists and mathematicians are aiming to create complex and rigorous mathematical operators that can accurately mimic and capture these natural phenomena. In this sense, the power law and the definition of local differential operators have been employed.
In recent years, researchers have shown interest in the synchronization and control of chaotic systems [10] with fractional-order dynamics. This led to numerous notable contributions to the Chua and Liu's circuit of chaos synchronization [11,12,13]. Many fractional-order chaotic systems have been effectively controlled using a variety of techniques such as adaptive controller [14,15], optimal controller [16,17], or sliding mode controller (SMC) [18,19,20], etc. The SMC is one of the most popular methods to control the chaos. In [21], authors applied adaptive SMC for uncertain fractional reaction-diffusion systems. In [22,23], SMC was used for fractional reaction-diffusion neural networks.
Understanding and projecting Earth's climate system requires the use of climate modeling, which also offers important insights for adaptation and mitigation strategies related to climate change. Scientists can estimate future climate scenarios and evaluate the possible implications of human activity using these models, which mimic and analyze the intricate interactions between numerous atmospheric, marine, and land surface systems [5,24]. Inspired by meteorological models and other forms of abstract models such as a chaotic atmosphere propagation model [25], atmospheric circulation systems [26], a model of atmospheric dynamics of carbon dioxide gas [27], post-quantum model of Navier problem [28], a fractal-fractional model of polluted lakes [29], financial systems in the sliding modes [30], memristor-based chaotic model of circuits [31], quantum-based model on integro-differential system [32], hybrid comformable initial value problems [33], and fractal-fractional model of HIV-1 infection [34], we have developed an atmospheric model describing the interaction between westerly currents, temperature, and GHGs in the Caputo fractional domain. To conduct the study, we analyze chaos using fractional-order commensurate and non-commensurate derivatives through analytical techniques and numerical simulations. A fractional derivative is introduced to obtain more details about the system's chaotic behavior. In the presence or absence of uncertainties and outside disturbances, we formulate sliding mode control (SMC) laws to manage chaos in the proposed atmospheric fractional-order system. We evaluate the controllers' efficiency by integrating them into the westerly current dynamics.
The rest of the paper is organized as follows: In Section 2, we give some basic definitions, lemmas, theories, and notations related to fractional calculus. Section 3 provides an extensive overview of the proposed fractional-order atmospheric model. In Section 4, we discuss the existence and boundedness of solutions, equilibrium points and their stability, and the conservative behavior of the system. Section 5 presents the detection of the chaos behavior of the system with the help of the Lyapunov exponent with graphical representations of the Poincaré maps and bifurcation analysis. Section 6 focuses on designing the SMC for chaotic systems and analyzing their stability. In Section 7, we provide a numerical example of the suggested work. Section 8 draws a conclusion for the current work.
Definition 2.1 ([6]). The Caputo derivative of the continuous function s(t) of fractional order m−1<γ<m is defined as
CDγt0s(t)=1Γ(m−γ)∫tt0s(m)(v)(t−v)γ+1−mdv, |
where Γ(⋅) is the Gamma function.
Definition 2.2 ([6]). For the integrable function s(t), the Riemann-Liouville fractional-order integral is defined as
Iγt0s(t)=1Γ(γ)∫tt0s(v)(t−v)1−γdv,γ>0,I0t0s(t)=s(t). |
Lemma 2.3 ([35]). Let s(t) be a continuous function on [t0,∞] that satisfies
CDγt0s(t)≤−αs(t)+ζ,s(t0)=gt0, |
here, 0<γ≤1, (α, ζ) ∈R2, and α≠0, then
s(t)≤(s(t0)−ζα)Eγ[−α(t−t0)γ]+ζα. |
Lemma 2.4 ([6]). Consider the system
CDγt0h(t)=s(t,h(t)), h(t0)=h0, t>t0, |
with γ∈(0,1], s:[t0,∞)×Γ→Rn. When s(t,h) holds the local Lipschitz criteria regarding h on v, the system has a unique solution.
A mathematical representation of atmospheric circulation that incorporates a condensed version of Earth's topography and climatic patterns was proposed by Edward N. Lorenz in 1984, becoming popularized as the Lorenz-84 climate model. The globe-encircling wind, also known as the Hadley cell circulation [5], is an important atmospheric circulation pattern that transfers heat from the equator toward the poles, being propelled by their temperature differential. This circulation can be observed in the Lorenz-84 model using a global wind component. In this model, the globe-encircling wind interacts with the thermal differential between the equator and the poles to provide poleward heat movement. As heat gets distributed with the wind, the westerlies get stronger due to greenhouse effect-induced global warming. In [25], authors demonstrated chaos for the fractional order model (3.1).
Dβtν=−ρ2−ψ2−aν+aM+F,Dβtρ=νρ−bνψ−ρ+P,Dβtψ=bνρ+νψ−ψ. | (3.1) |
Figure 1 demonstrates the dynamics of the model (3.1) for β=1,β=0.95,β=0.85, and β=0.75 for the parameter values a=0.24,b=11, P=3, M=8, and the constant CO2 input F=1.66.
In the present work, instead of using a constant input of CO2, we have incorporated the radiative forcing of GHGs as a new equation to investigate their continuous impact on the Lorenz-84 climate circulation system [36]. We aim to examine the change in the strength of the westerly current with the continuous change in growth rate of GHGs in the atmosphere. Therefore, we have installed an additional equation representing the radiative force of GHGs; its effect on westerly current is represented by the incorporation of a term representing the continuous impact of GHGs in the first equation. The Caputo fractional derivative framework is utilized in this study to further examine the atmospheric system. The proposed fractional differential equation (FDE) is:
CDβt0ν=−ρ2−ψ2−aν+aM+nμ,CDβt0ρ=νρ−bνψ−ρ+P,CDβt0ψ=bνρ+νψ−ψ,CDβt0μ=lμ−rμ2, | (3.2) |
where ν is the force of the symmetrically circling westerly wind current around the Earth. Heat is transported poleward by a series of superposed large-scale currents, the cosine and sine phases, that are represented by ρ and ψ. μ represents the GHGs, M is the symmetric thermal forcing brought on by the temperature differential across latitudes, and P is the difference in temperature between the ocean and the continent. The wave advection force that results from the westerly current is expressed as b. n is the rate of GHGs added to the westerly current, and r is the natural depletion rate of GHGs that may happen due to chemical reactions.
Our investigation aims to establish whether the solutions to the fractional four-dimensional chaotic atmospheric model exist and are unique under the Caputo fractional derivative framework. By careful exploitation of mathematical details, we have confirmed that the solutions not only exist but are unique, thus ensuring the validity of model (3.2) for further analysis and applications.
Theorem 4.1. In the region B×[0,T], where B={(ν,ρ,ψ,μ)∈R4:max{|ν|,|ρ|,|ψ|,|μ|}≤F}, and T<+∞, there exists a unique solution of the system (3.2).
Proof. Let us consider
U(t)=(ν(t),ρ(t),ψ(t),μ(t)), ˉU(t)=(ˉν(t),ˉρ(t),ˉψ(t),ˉμ(t)), | (4.1) |
and a function
V(t,U)=(V1(t,U),V2(t,U),V3(t,U),V4(t,U)), |
where
V1(t,U)=−ρ2−ψ2−aν+aM+nμ,V2(t,U)=νρ−bνψ−ρ+P,V3(t,U)=bνρ+νψ−ψ,V4(t,U)=lμ−rμ2. | (4.2) |
Here, V(t,U) is defined on B×[0,T] and let N=supB||V(t,U)||.
We interpret the norm as ||U(t)||=supt∈[0,T]|U(t)|. We shall demonstrate the existence of some Φ such that
‖V(U)−V(ˉU)‖≤Φ‖U−ˉU‖. |
Consider that
‖V(U)−VˉU‖=||−ρ2−ψ2−aν+aM+nμ+νρ−bνψ−ρ+P+bνρ+νψ−ψ+lμ−rμ2+ˉρ2+ˉψ2+aˉν−aM−nˉμ−ˉνˉρ+bˉνˉψ+ˉρ−P−bˉνˉρ−ˉνˉψ+ˉψ−lˉμ+rˉμ2||≤||ρ2−ˉρ2||+||ψ2−ˉψ2||+a||(ν−ˉν)||+||ρ−ˉρ||+||ψ−ˉψ||+(1+b)||νρ−ˉνˉρ||+(1+b)||νψ−ˉνˉψ||+(n+l+2rF)||μ−ˉμ||≤{a+2F(1+b)}||ν−ˉν||+{1+(3+b)F}||ρ−ˉρ||+{1+(3+b)F}||ψ−ˉψ||+(n+l+2rF)||μ−ˉμ||. |
This implies
‖V(U)−VˉU‖≤Φ1||ν−ˉν||+Φ2||ρ−ˉρ||+Φ3||ψ−ˉψ||+Φ4||μ−ˉμ||, | (4.3) |
where
Φ1=a+2F(1+b),Φ2=1+(3+b)F,Φ3=1+(3+b)F,Φ4=n+l+2rF. | (4.4) |
Consider
Φ=max{Φ1,Φ2,Φ3,Φ4}. |
This gives
‖V(U)−VˉU‖≤Φ||U−ˉU||. |
Let Δ represent the Picard's operator that is being constructed through the utilization of the fractional integral and function V. Consequently, we get the following equation
ΔU=U(0)+IβV(t,U). | (4.5) |
It is necessary to demonstrate that this operator is a contraction mapping and that it maps a whole non-empty metric space into itself.
Consider
||U−U(0)||≤m, |
where m is a constant. Using (4.5) as the norm, we obtain
||ΔU−U(0)||≤||V(t,U)||Iβ(1)≤nTβΓ(β+1)<m. | (4.6) |
The above inequality in (4.6) holds if
TβΓ(β+1)<mN. |
We now isolate a condition in which the operator Δ is contraction. We take the following actions to fulfill this requirement:
||ΔU−ΔˉU||=||Iβ(V(t,U)−V(t,ˉU))||≤Iβ||(V(t,U)−V(t,ˉU))||≤||(V(t,U)−V(t,ˉU))||Iβ(1)≤TβΓ(β+1)Φ||U−ˉU||. | (4.7) |
The preceding formula demonstrates that when the relationship is defined by the Picard's operator Δ, it turns into a contraction, and
TβΓ(β+1)≤1Φ. |
In this way, it is proven that the Picard's operator Δ is a contraction. We determine that the operator Δ has a unique fixed point by applying the Banach fixed-point theorem. As a result, there is only one solution for the FDE (3.2).
Here, note that
TβΓ(β+1)<min{mn , 1Φ}. |
Based on the model's parameters, we can interpret Φi, where i = 1, 2, 3, 4, as follows:
Φ1 = Strength of the westerly current due to the impact of sinusoidal waves;
Φ2 = Strength of the sine waves;
Φ3 = Strength of the cosine waves;
Φ4 = Total amount of greenhouse gas present in the armosphere;
All these Φi, i = 2(1)4, indicate the strength of the westerly current.
(1) The combined effect of the sine and cosine waves maximizes the strength of the westerly current if Φ1 is at its maximum.
(2) Suppose that Φ4 represents the maximum. If the depletion rate increases in the atmosphere, then the rate of GHGs reduces, and due to this effect, the strength of the westerly waves decreases.
In this section, we establish that the solutions of system (3.2) are bounded.
Theorem 4.2. The solutions of system (3.2) are bounded uniformly.
Proof. Define a function,
Q(t)=ν(t)+ρ(t)+ψ(t)+μ(t). |
Utilizing the Caputo fractional derivative, we obtain
CDβt0Q(t)+Q(t)=CDβt0ν(t)+CDβt0ρ(t)+CDβt0ψ(t)+CDβt0μ(t)+[ν(t)+ρ(t)+ψ(t)+μ(t)],=(−ρ2−ψ2−aν+aM+nμ)+(νρ−bνψ−ρ+P)+(bνρ+νψ−ψ)+(lμ−rμ2)+(ν(t)+ρ(t)+ψ(t)+μ(t))≤aM+nμ+νρ+P+bνρ+νψ+lμ+ν+ρ+ψ+μ≤aM+P+(n+l+1)μ+(1+b)νρ+(1+ν)ψ+ν+ρ. |
There is a unique and existing solution in
B={(ν,ρ,ψ,μ):max{|ν|,|ρ|,|ψ|,|μ|}≤F}. |
The inequality stated above gives that
CDβt0Q(t)+Q(t)≤(n+l+4)F+(2+b)F2+aM+P=(√2+bF+(n+l+4)2√2+b)2−((n+l+4)2√2+b)2+aM+P≤(√2+bF+(n+l+4)2√2+b)2+aM+P. |
By Lemma (2.3), we have
CDβt0Q(t)≤(Q(t0)−(√2+bF+(n+l+4)2√2+b)2+aM+P)Eβ[−(t−t0)β]+(√2+bF+(n+l+4)2√2+b)2+aM+P. |
Clearly,
Q(t)→(√2+bF+(n+l+4)2√2+b)2+aM+P |
as t→ ∞.
Thus, the system (3.2) that initiates in ℧ and its whole solution remained bounded in
Θ={(ν,ρ,ψ,μ)∈℧+|Q(t)≤(√2+bF+(n+l+4)2√2+b)2+aM+P+ϵ,ϵ>0}. |
The stability of the system (3.2) is examined here at each equilibrium point. The following system of equations must be solved in order to determine the equilibrium points:
−ρ2−ψ2−aν+aM+nμ=0, | (4.8) |
νρ−bνψ−ρ+P=0, | (4.9) |
νρ+νψ−ψ=0, | (4.10) |
lμ−rμ2=0. | (4.11) |
Solving the aforementioned system, we obtain more than one equilibrium point. Let (ν∗,ρ∗,ψ∗,μ∗) be one such equilibrium point. From Eq (4.11), we get
lμ∗−rμ∗2=0,μ∗=0, and μ∗=lr. |
Solving (4.10) for ψ∗, we get
ψ∗=−bν∗ρ∗−1+ν. | (4.12) |
Solving (4.9) for ρ∗ and then substituting the value of ψ∗, it gives
ρ∗=−(−1+ν∗)P1−2ν∗+ν∗2+b2ν∗2. | (4.13) |
From Eqs (4.12) and (4.14),
ψ∗=bν∗P1−2ν∗+(1+b2)ν∗2. | (4.14) |
Substituting the values of ρ∗,ψ∗,μ∗ in (4.8), we obtain an equation in terms of ν∗.
B1ν∗3+B2ν∗2+B3ν∗+B4=0, | (4.15) |
where,
B1=−a(1+b2), B2=2a+a(1+b2)M, B3=−a−2aM−2nμ∗, B4=aM+nμ∗−P2. |
Here, two cases arise, because μ has two values:
ⅰ) If μ∗ = 0, then
B1=−a(1+b2), B2=2a+a(1+b2)M, B3=−a−2aM, B4=aM−P2. | (4.16) |
Since B1<0, B2>0, B3<0, if aM<P2, by Descartes' rule of signs, Eq (4.16) has at most two real roots. If aM>P2, then Eq (4.16) has at most three real roots.
ⅱ) If μ∗=lr, then
B1=−a(1+b2), B2=2a+a(1+b2)M, B3=−a−2aM−2lμr, B4=aM+lr−P2. | (4.17) |
Since B1<0, B2>0, B3<0, if (aM+lr)<P2 then by Descartes' rule of signs, Eq (4.15) has at most two real roots. If (aM+lr)>P2, then Eq (4.15) has at most three real roots. Consequently, there is a coexistence point of equilibrium.
The Jacobian matrix of system (3.2) is
J=(−a−2ρ−2ψnρ−bψ−1+ν−bν0bρ+ψbν−1+ν0000l−2rμ). |
Theorem 4.3. The axial equilibrium point, E0=(0,0,0), is always present, but it is unstable.
Proof. The eigenvalues of the Jacobian matrix J at E0 are
λ11=−a,λ12=−1,λ13=−1,λ14=l. |
It is clear that E0 is unstable.
Theorem 4.4. When P≠0 and b=0, there are two unstable equilibrium points E1=(ν1,ρ1,0,0) = E1(ν1,−Pν1−1,0,0) and E2=(ν1,ρ1,0,μ1) = E2(ν1,−Pν1−1,0,lr).
Proof. (ⅰ) At E1, the Jacobian matrix is
J=(−a−2ρ10nρ1−1+ν10000−1+ν10000l). |
Eigenvalues of J at E1 are
λ11=ν1−1,λ12=l,λ13=12(−(1−ν1−a)+√(ν1−1−a)2−8P2(ν1−1)2),λ14=12(−(1−ν1−a)−√(ν1−1−a)2−8P2(ν1−1)2). |
λ12>0 indicates that the equilibrium point E1 is unstable.
(ⅱ) At E2, the Jacobian matrix is
J=(−a−2ρ10nρ1−1+ν10000−1+ν10000−l). |
The corresponding eigenvalues are
λ21=ν1−1,λ22=−l,λ23=12(−(1−ν1+a)+√(ν1−1+a)2−8P2(ν1−1)2),λ24=12(−(1−ν1+a)−√(ν1−1+a)2−8P2(ν1−1)2). |
When b = 0, system (3.2) yields
a(M−ν1)(1−ν1)2+nlr(1−ν1)2=P2. | (4.18) |
If a>0, then Eq (4.18) has a solution ν1<1, then λ21<0. Since 8P2(ν1−1)2>0 and (ν1−1−a)>0, ν1>1+a and it clearly shows that Re(λ23)<0 and Re(λ24)<0. Hence, E2 is a stable equilibrium point.
The system (3.2) is expressed in vector form as CDβt0U=V(U) such that
V(U)=(V1(U)V2(U)V3(U)V4(U)), |
where V1–V4 are defined in Eq (4.2). In this paper, we consider the values of the parameters as:
a=0.24,b=11,P=3,n=0.25,l=0.3,r=0.0001,M=8. |
The vector region f on R4 has a divergence [37] that can be expressed as
divV=δV1(U)δν+δV2(U)δρ+δV3(U)δψ+δV4(U)δμ,=−a+ν−1+ν−1+l−2rμ,<−1.94+2F<0. |
Consider any smooth-boundary region in R4 denoted by B. Let B(t)=Pt(B), where Pt is the path of the vector field f. Let V(t) represent B hyper-volume. According to Liouville's theorem, it implies that
dV(t)dt=∫B(t)(divV)dνdρdψdμ. | (4.19) |
The (divV) value can be substituted in (4.19) to get
dV(t)dt≤(−1.94+2F)∫B(t)dνdρdψdμ≤(−1.94+2F)V(t). | (4.20) |
When the linear differential inequation (4.20) is integrated, we get
V(t)≤V0e(−1.94+2F)t, where,(−1.94+2F)<0. | (4.21) |
We can conclude that −1.94+2F<0 that is F<0.97 is essential for the dissipativeness of the system (3.2). The volume V(t) decreases exponentially to zero as t→∞, according to Eq (4.21). As a result, the novel chaotic system (3.2) is dissipative.
In this section, we explain the chaos that results from different fractional-order derivatives concerning time. β=1 and β=0.95 are chosen for this purpose. Values of the Lyapunov exponent for β=1 and β=0.95 are presented in Tables 1 and 2. Since at least two Lyapunov exponents are positive, for β=1, β=0.95, the system exhibits hyperchaotic behavior as shown in Figure 2. Hence, system (3.2) is a hyper-chaotic model. Authors of [7,38,39] have uncovered certain fractional-order hyperchaotic systems with a one-positive Lyapunov exponent.
T | LE1 | LE2 | LE3 | LE4 |
100 | 0.6808 | 0.7047 | -0.5422 | 0.2999 |
200 | 0.8787 | -0.4683 | -1.2725 | 0.2999 |
300 | 0.9245 | -0.2770 | -1.0604 | 0.2999 |
400 | 0.3552 | -0.1723 | -0.9711 | 0.2998 |
500 | 0.4961 | -0.0017 | -1.2033 | 0.2998 |
600 | 0.6279 | -0.0093 | -1.1763 | 0.2997 |
700 | 0.6552 | 0.0670 | -1.1259 | 0.2995 |
800 | 0.8235 | 0.1597 | -1.2415 | 0.2993 |
900 | 0.8645 | 0.0018 | -1.1701 | 0.2989 |
1000 | 0.7542 | 0.0581 | -1.1636 | 0.2982 |
T | LE1 | LE2 | LE3 | LE4 |
100 | 1.9752 | -0.3531 | -1.5458 | 0.3722 |
200 | 1.5186 | -0.7385 | -1.8590 | 0.3722 |
300 | 1.0170 | -0.153 | -1.7393 | 0.3721 |
400 | 0.8684 | 0.0294 | -1.5641 | 0.3720 |
500 | 0.7705 | -0.0592 | -1.6395 | 0.3719 |
600 | 0.6112 | 0.0205 | -1.5097 | 0.3716 |
700 | 0.9984 | 0.0283 | -1.6295 | 0.3710 |
800 | 1.0916 | 0.0289 | -1.6073 | 0.3699 |
900 | 1.2012 | 0.0938 | -1.6858 | 0.3680 |
1000 | 1.3357 | 0.0448 | -1.7237 | 0.3643 |
An additional technique to describe the chaotic reaction is a Poincaré map or a return map. It forecasts the point on the trajectory where the current Poincaré section [40] crosses over. This mapping aims to achieve a minimum of two objectives:
(1) It helps to reveal any sequential order from the recurrent visit of paths to a phase space cross-section in graphical form.
(2) It aids in the prediction of the system's subsequent states in that Poincaré section.
In this section, we have considered two Poincaré sections: U={ρ(t),ψ(t)∈R2|ν(t)=−0.1} and V={ν(t),ψ(t)∈R2|ρ(t)=1}.
While the Poincaré sections across chaotic attractors of the system (3.2) are relatively complex, they typically exhibit some form of structure. We aim to observe the Poincaré section for changes concerning the growth rate l and depletion rate r of GHGs in the atmosphere. r=0.001,l=0.3 leads to Figure 3a, while r=0.0001,l=0.0037 leads to Figure 3b. Both figures represent the Poincaré sections parallel to the ρ−ψ plane sectioned by ν=−0.1. On the other hand, r=0.001,l=0.0037 lead to the Poincaré section of Figure 3c parallel to ν−ψ plane sectioned by ρ=1. Moreover, a Poincaré section traversing a chaotic attractor typically consists of an infinite number of points. It is significant to remember that each surface in the phase plane's Poincaré section refers to a chaotic attractor. Thus, for β=1, it is expected that system (3.2) will produce chaotic attractors. The outcomes of the modeling shown in Figure 4a are validated in this section via the Poincaré section parallel to ρ−ψ and ν−ψ plane.
Quantitative bifurcation analysis is a tool to observe the existence of chaos in a system. Here, we examine the dynamics of the system (3.2) for the change of the values of the parameter a. The presence of chaos is shown in Figure 5 for b=11,P=3,n=0.25,l=0.3,r=0.0001, and M=8. The a−ν,a−ρ,a−ψ phase portraits for a range of parameter a values can be seen in Figure 5. These figures indicate the existence of chaos.
The fundamental principle of the SMC rule is to drive the system state trajectories to certain, predefined sliding surfaces where stability and other desirable system properties are present by use of a discontinuous control. This is accomplished by establishing the sliding surface, which facilitates the assessment of the fractional-order system's efficacy in sliding mode. The sliding mode controller is created after the sliding surface is determined. It is composed of two parts:
● We need to construct a sliding surface that depicts the intended system dynamics prior to creating a sliding mode controller.
● We need to create a switching control rule such that, at each point on the sliding surface, it will result in a sliding mode and drive any states that are outside of it to the surface in a finite amount of time.
A useful control method that guarantees both the occurrence of sliding motion and chaotic system control is the SMC law. Among its many fascinating characteristics are the resistance to parameters such as uncertainty and the desensitization to outside disruptions.
With the aid of an initial numerical analysis, we found that controlling the first equation is crucial to control the chaos within the system. The SMC law is a practical control strategy that ensures both chaotic system control and sliding motion. Resistance to constraints like uncertainty and desensitization to outside disruptions are only two of its many intriguing traits. Let us assume that A(t) represents a control input that has to be applied in the following manner to the first state equation of the fractional-order system (3.2)
CDβ1t0ν=−ρ2−ψ2−aν+aM+nμ+A(t),CDβ2t0ρ=νρ−bνψ−ρ+P,CDβ3t0ψ=bνρ+νψ−ψ,CDβ4t0μ=lμ−rμ2. | (6.1) |
We propose a sliding surface λ(U(t)) as a function of the system states U(t)=(ν(t),ρ(t),ψ(t),μ(t)) as follows:
λ(U(t))=CDβ1−1ν(t)+CD−1(δ(U(t))=CDβ1−1ν(t)+∫t0δ(U(τ))dτ, | (6.2) |
in which the function δ(U(t)) is described as
δ(U(t))=ρ2+ψ2−mμ+sν, | (6.3) |
such that it involves all the state variables. Here, the constant s>0 is arbitrary.
The sliding surface and its derivative in a sliding mode must satisfy
λ(U(t))=0,λ′(U(t))=0. | (6.4) |
From (6.2) and (6.4), we get λ′(U(t))=CDβ1ν(t)+δ(U(t))=0. Hence,
CDβ1ν(t)=−δ(U(t))=−(ρ2(t)+ψ2(t)−mμ+sν(t)). | (6.5) |
Hence, from the SMC theory, the first equation of systems (6.1) and (6.5) is applied to determine the corresponding control law
Aeq(t)=Dβ1ν(t)+ρ2+ψ2+aν−aM−nμ=−ρ2−ψ2+mμ−sν+ρ2+ψ2+aν−aM−nμ=(a−s)ν−aM+(m−n)μ. | (6.6) |
With respect to the sliding criterion, the reaching law can be selected as follows:
Ar=Wrsign(λ), |
where
sign(λ)={+1,λ>0,0,λ=0,−1,λ<0, |
and the controller gain is represented as Wr.
Here, the total control law can be defined as follows:
A(t)=Aeq+Ar=(a−s)ν−aM+(m−n)μ+Wrsgn(λ). | (6.7) |
Theorem 6.1. The fractional system (6.1) with the influence of the control law (6.7) is globally asymptotically stable if the controller gains Wr<0.
Proof. Let us choose the Lyapunov candidate
X=12(λ(U(t)))2. | (6.8) |
Its derivative is computed by
˙X(U(t))=λ(U(t))˙λ(U(t))=λ(U(t))[Dβ1ν+ρ2+ψ2−mμ+sν]=λ(U(t))[−ρ2−ψ2−aν+aM+nμ+A+ρ2+ψ2−mμ+sν]=λ(U(t))[−aν+aM+nμ+aν−sν−aM+mμ−nμ+Wrsgn(λ(U(t)))−mμ+sν]=Wr|λ(U(t))|<0. |
Thus, the Lyapunov function X meets the conditions of the Lyapunov theorem, that is X>0 and ˙X<0. Hence, the controller system (6.1) with the impact of the control law (6.7) is globally asymptotically stable when Wr<0.
Theorem 6.2. The finite-time interval for fractional system (6.1) to reach the sliding surface is [0,τr] with τr=−12Wr(λ(U(0)))2.
Proof. For stability and finite-time convergence, the control gain Wr must be chosen such that ˙X(U(t)) is negative definite. From Theorem 6.1, we have
˙X(U(t))=Wr|λ(U(t))|, | (6.9) |
where Wr<0. We consider the time to reach the sliding surface to be τr. Integrating both sides of Eq (6.9), from the initial time t=0 to the time τr, when λ(U(t)) tends to zero, we get
∫τr0d(X(U(t)))|λ(U(t))|=∫τr0Wrdt. | (6.10) |
We have X(U(τr))=0 and X(U(0))=12(λ(U(0)))2. This yields that
τr=−12Wr(λ(U(0)))2, |
where λ(U(0)) is the value of the sliding surface at the initial state. By decreasing Wr, one can reduce the finite-time interval, ensuring the system reaches the sliding surface faster.
Theorem 6.3. When there are uncertainities and an external disturbance, the fractional system (6.5) takes on the following form:
CDβ1t0ν=−ρ2−ψ2−aν+aM+nμ+A(t)+Δh(ν,ρ,ψ,μ)+f,CDβ2t0ρ=νρ−bνψ−ρ+P,CDβ3t0ψ=bνρ+νψ−ψ,CDβ4t0μ=lμ−rμ2. | (6.11) |
Where Δh(ν,ρ,ψ,μ) and f(t) are considered to be bounded such that Δh(ν,ρ,ψ,μ)<g1, and f(t)<g2, where g1,g2>0. Then, the above system (6.11) with the impact of SMC law (6.7) is globally asymptotically stable if Wr<−(g1+g2).
Proof. We consider here the Lyapunov function as
X=12λ2. |
We have
˙X=λ(t)˙λ(t)=λ[Dβ1ν+ρ2+ψ2−mμ+sν]=λ[−ρ2−ψ2−aν+aM+nμ+A+Δh(ν,ρ,ψ,μ)+f+ρ2+ψ2−mμ+sν]=λ[−aν+aM+nμ+aν−aM−nμ+Wrsgn(λ)+Δh(ν,ρ,ψ,μ)+f]≤(Wr+g1+g2)|λ|. | (6.12) |
Hence, ˙X<0 and Wr<−(g1+g2).
Using the Adams-Bashforth-Moulton approach, which is compatible with FDEs, numerical simulations are performed [41]. We consider the parameter values at which the projected atmospheric model (3.2) becomes chaotic, namely a=0.24,b=11,P=3,n=0.25,l=0.3,r=0.0001, and M=8. (ν0,ρ0,ψ0,μ0)=(−0.1,−0.1,−0.1,0.1) represents the initial approximation of state variable of the atmospheric model (3.2).
The equilibrium points corresponding to the model (3.2) are E1(7.995,0.002,0.033,0), E2(0.184,0.509,1.271,0), E3(−0.163,0.759,−1.175,0), E4(3133,0,0.00008,3000), E5(0.008−0.089i,248.584+22.469i,22.605−247.08i,3000), and E6(0.008+0.089i,248.584−22.469i,22.605+247.08i,3000).
Here, equilibrium points E1–E4 are real, whereas E5, and E6 are complex conjugate. As atmospheric events are real phenomena, we shall consider only real equilibrium points.
(1) Eigenvalues of the Jacobian matrix at E1 are
λ1,1=6.995+87.947i,λ1,2=6.995−87.947i,λ1,3=0.3,λ1,4=−0.239. |
From the Matignon criterion provided by [42],
|arg(λ1,1)|=1.491<π2,|arg(λ1,2)|=1.491<π2, |
|arg(λ1,3)|=0<π2,|arg(λ1,4)|=3.142>π2. |
As |arg(λ1,3)|<βπ2 holds for any values of fractional derivative β, the equilibrium point E1 is unstable.
(2) Eigenvalues of the Jacobian matrix at E2 are
λ2,1=−2.56+4.318i,λ2,2=−2.56−4.318i,λ2,3=3.183,λ2,4=0.3. |
|arg(λ2,1)|=2.105>π2,|arg(λ2,2)|=2.105>π2,|arg(λ2,3)|=0<π2,|arg(λ2,4)|=0<π2. |
Since |arg(λ2,j)|<βπ2,3≤j≤4, for 0<β≤1, the equilibrium point E2 is unstable.
(3) At E3, the eigenvalues of the Jacobian matrix are
λ3,1=−4.569,λ3,2=1.001+4.149i,λ3,3=1.001−4.149i,λ3,4=0.3. |
|arg(λ3,1)|=π>π2,|arg(λ3,2)|=1.33<π2,|arg(λ3,3)|=1.33<π2,|arg(λ3,4)|=0<π2. |
Since |arg(λ3,4)|≤βπ2, for 0<β≤1, the equilibrium point E3 is always unstable.
(4) Eigenvalues of the Jacobian matrix at E4 are
λ4,1=3132+34463i,λ4,2=3132−34463i,λ4,3=−0.3,λ4,4=−0.24. |
|arg(λ4,1)|=1.48<π2,|arg(λ4,2)|=1.48<π2,|arg(λ4,3)|=π>π2,|arg(λ4,4)|=π>π2. |
Since |arg(λ4,i)|<βπ2,1≤i≤2, 0.945<β≤1, the system (3.2) is unstable at E4 for 0.945<β≤1.
We consider β1=β2=β3=β4=β. Figures 1 and 6 display the system trajectories of Eq (3.2) for commensurate orders β=1,β=0.95,β=0.85, and β=0.75. Figure 1 represents the dynamics of the system (3.1) illustrating the impact of a fixed input of CO2. On the other hand, Figure 6 depicts the dynamics of the system (3.2) illustrating a continuous input of GHGs.
The Southern Hemisphere westerlies (SHWs) are crucial for regulating global climate and ocean circulation, but their future changes under varying levels of greenhouse gases (GHG) remain uncertain. According to this study, GHG-induced surface warming is unevenly distributed in the Southern Hemisphere under moderate to high GHG forcing, with the greatest warming occurring in sub-Antarctic coastal regions. Additionally, land areas on the continent are expected to warm more rapidly than the surrounding oceans. As a result, the equator-to-pole temperature gradient is likely to increase south of 600S latitude and decrease north of 600S latitude. This could lead to a strengthening of westerly wind speeds and a poleward shift of the SHWs [43]. The fourth equation of system (3.2) represents the changes in GHGs' intake in the atmosphere, highlighting the fact that, along with the growth of GHGs, there are depletions of GHGs due to their internal reactions. Chaotic behaviors are strongly observed in both Figures 1 and 6. However, in Figure 1, for various values of β, we observe the identical pattern of the strength of the westerly current, cosine, and sine waves. Whereas in Figure 6, an increasing strength of sine and cosine waves concerning time is noticeable. Also, the presence of continuous input of GHGs delays the amplitude of westerlies. This suggests that stronger westerlies result in more intense westerly currents compared to the new 4D atmospheric model (3.2) for the fractional order shown in Figure 6. 3D and 2D portfolios are shown in Figure 4. The increase in the strength of the westerlies can influence sea surface temperatures and marine ecosystems by affecting ocean circulation. Therefore, controlling these westerlies is critical.
A preliminary numerical investigation reveals that the installation of the control variable in the first equation of (3.2) will induce control in the underlying chaos. As a result, we obtained the resulting control system (6.1). The dynamics of the system's solution under the impact of SMC are projected in Figure 7, demonstrating that the resultant solution shows that chaotic behavior becomes controlled. The strength of the westerlies in the new 4D model increases as the fractional differential order decreases from β=1 to β=0.75. Moreover, we observe that when the fractional order decreases, the corresponding GHGs also decrease.
Figure 8a–d shows the dynamics of the non-commensurate fractional-order system (3.2) without controller, and Figure 8e–h shows the dynamics of the non-commensurate fractional-order system (6.1) with controller, for β1=0.85,β2=0.95,β3=0.9, and β4=1.
We observed the dynamics of the controlled system (6.1) under the influence of uncertainties and external disturbances, namely Δh(ν,ρ,ψ,μ)=0.11sin(ν3+ρ3) and f(t)=0.04sin(πt), respectively. Here, |Δh(ν,ρ,ψ,μ)|≤g1=0.11 and |f(t)|≤g2=0.04. By incorporating these parameters, we obtain the system (6.11). Figure 9 represent the dynamics of the non-commensurate ordered system (6.11) in the presence of assigned uncertainties and external disturbances. Westerlies, sine, and cosine waves remain in control even when external disturbances and uncertainties are applied. The system converges toward stability as pressure over the poles increases and the strong westerlies weaken. Figure 10 illustrates that even in the face of uncertainty, the controlled system (6.11) is efficient in controlling the chaos. In Figure 10, we show the power of the controller in system (6.11) for non-commensurate fractional derivatives β1=0.85,β2=0.95,β3=0.9, and β4=1. The controller has forced the states of the system onto the sliding plane, where they remain for all following times. The simulation results demonstrate that in all three scenarios, the system responds to the derived control law (6.7). Put differently, the findings of the simulation demonstrate that the theoretical conclusions drawn are workable and effective for regulating the fractional-order atmospheric system.
Using the following set of parameter values, we validated the existence of the solution for the projected model: a=0.24,b=11,P=3,n=0.25,l=0.3,r=0.0001,M=8. Furthermore, taking the guidance from the bounds shown in Figure 6, we considered η1=4, η2=6.5, η3=6.5, and η4=170.
(1) For a maximum Φ1, we examined the values of fixed parameters. Thus, we obtained Φ1=156.24,Φ2=57.2,Φ3=57.2,Φ4=0.584. In this case, the strength of the westerly current is maximized due to the combined impact of sine and cosine waves; these observations are shown in Figures 4a, 6a, 6e, and 6i.
(2) To get the Φ4 maximum in comparison to others, we increased the depletion rate to r=0.5, maintaining the same values for the other parameters. Consequently, we obtained Φ1=156.24,Φ2=57.2,Φ3=57.2,Φ4=170.55. When the depletion rate of GHGs increases, the exponential growth of GHGs (Figure 6m–p) converts to steady growth (Figure 11d), and this leads to depleting the amplitude of the strength of the westerly current. These observations are shown in Figure 11.
In summary, our research on the fractional four-dimensional chaotic atmospheric model has yielded significant insights into the existence and uniqueness of solutions, the system's dissipative nature, and methods for controlling chaos. We calculated the Lyapunov exponents, which are critical for understanding the system's stability and chaotic behavior. Poincaré map and bifurcation analysis strengthened the existence of chaos in the projected model. To address the chaos in the atmospheric system, we derived a sliding-mode controller. This control mechanism is designed to globally stabilize the system, ensuring it can operate under the influence of external disturbances. The numerical simulations illustrate that the sliding-mode controller successfully stabilizes the chaotic system, thereby proving its practical applicability. The control law we proposed effectively mitigates chaos for both commensurate and non-commensurate orders of the system. All theoretical findings are well-supported by numerical simulations and graphical representations, enhancing our understanding of complex atmospheric models.
Conceptualization: A.J.R., C.B., R.G.; Methodology: A.J.R., R.G., Z.M.Y.; Software: C.B., S.E.; Validation: C.B., S.E., Z.M.Y.; Formal analysis: C.B., S.E., Z.M.Y.; Investigation: R.G., S.E., Z.M.Y.; Writing-original draft preparation: A.J.R., C.B., S.E.; Writing-review and editing: C.B., R.G., Z.M.Y.; Supervision: C.B., R.G.; Project administration: R.G. All authors have read and approved the final version of the manuscript for publication.
The authors extend their appreciation to Prince Sattam bin Abdulaziz University, Saudi Arabia for funding this research work through the project number (2024/01/922572).
The authors declare no conflicts of interest.
[1] |
J. E. Skinner, M. Molnar, T. Vybiral, M. Mitra, Application of chaos theory to biology and medicine, Integr. Physiol. Behav. Sci., 27 (1992), 39–53. https://doi.org/10.1007/bf02691091 doi: 10.1007/bf02691091
![]() |
[2] |
Y. Liu, Circuit implementation and finite-time synchronization of the 4D Rabinovich hyperchaotic system, Nonlinear Dyn., 67 (2012), 89–96. https://doi.org/10.1007/s11071-011-9960-2 doi: 10.1007/s11071-011-9960-2
![]() |
[3] |
M. E. Sahin, Z. G. Cam Taskiran, H. Guler, S. E. Hamamci, Application and modeling of a novel 4D memristive chaotic system for communication systems, Circuits Syst. Signal Process., 39 (2020), 3320–3349. https://doi.org/10.1007/s00034-019-01332-6 doi: 10.1007/s00034-019-01332-6
![]() |
[4] |
E. N. Lorenz, Irregularity: a fundamental property of the atmosphere, Tellus A, 36A (1984), 98–110. https://doi.org/10.3402/tellusa.v36i2.11473 doi: 10.3402/tellusa.v36i2.11473
![]() |
[5] | Y. Yu, Dynamical analysis of a low-order model representing Hadley circulation, J. Beijing Jiaotong Univ., 30 (2006), 50–53. |
[6] | I. Podlubny, Fractional differential equations: an introduction to fractional derivatives, fractional differential equations, to methods of their solution and some of their applications, Academic Press, 1998. |
[7] |
N. Sene, Analysis of a four-dimensional hyperchaotic system described by the Caputo-Liouville fractional derivative, Complexity, 2020 (2020), 8889831. https://doi.org/10.1155/2020/8889831 doi: 10.1155/2020/8889831
![]() |
[8] |
Y. Cao, Y. Kao, J. H. Park, H. Bao, Global Mittag-Leffler stability of the delayed fractional-coupled reaction-diffusion system on networks without strong connectedness, IEEE Trans. Neural Networks Learn. Syst., 33 (2022), 6473–6483. https://doi.org/10.1109/TNNLS.2021.3080830 doi: 10.1109/TNNLS.2021.3080830
![]() |
[9] |
R. N. Premakumari, C. Baishya, M. K. A. Kaabar, Dynamics of a fractional plankton-fish model under the influence of toxicity, refuge, and combine-harvesting efforts, J. Inequal. Appl., 2022 (2022), 137. https://doi.org/10.1186/s13660-022-02876-z doi: 10.1186/s13660-022-02876-z
![]() |
[10] | S. Vaidyanathan, A. T. Azar, Analysis and control of a 4-D novel hyperchaotic system, In: A. Azar, S. Vaidyanathan, Chaos modeling and control systems design: studies in computational intelligence, Springer, Cham, 581 (2015), 3–17. https://doi.org/10.1007/978-3-319-13132-0_1 |
[11] |
L. O. Chua, M. Itoh, L. Kocarev, K. Eckert, Chaos synchronization in Chua's Circuit, J. Circuits Syst. Comput., 3 (1993), 93–108. https://doi.org/10.1142/S0218126693000071 doi: 10.1142/S0218126693000071
![]() |
[12] |
D. Baleanu, S. S. Sajjadi, J. H. Asad, A. Jajarmi, E. Estiri, Hyperchaotic behaviors, optimal control, and synchronization of a nonautonomous cardiac conduction system, Adv. Differ. Equ., 2021 (2021), 157. https://doi.org/10.1186/s13662-021-03320-0 doi: 10.1186/s13662-021-03320-0
![]() |
[13] |
U. E. Kocamaz, B. Cevher, Y. Uyaroǧlu, Control and synchronization of chaos with sliding mode control based on cubic reaching rule, Chaos Soliton. Fract., 105 (2017), 92–98. https://doi.org/10.1016/j.chaos.2017.10.008 doi: 10.1016/j.chaos.2017.10.008
![]() |
[14] |
Y. Kao, Y. Li, J. H. Park, X. Chen, Mittag-Leffler synchronization of delayed fractional memristor neural networks via adaptive control, IEEE Trans. Neural Networks Learn. Syst., 32 (2021), 2279–2284. https://doi.org/10.1109/TNNLS.2020.2995718 doi: 10.1109/TNNLS.2020.2995718
![]() |
[15] |
C. Baishya, M. K. Naik, R. N. Premakumari, Design and implementation of a sliding mode controller and adaptive sliding mode controller for a novel fractional chaotic class of equations, Results Control Optim., 14 (2024), 100338. https://doi.org/10.1016/j.rico.2023.100338 doi: 10.1016/j.rico.2023.100338
![]() |
[16] | A. Moufid, N. Bennis, A multi-modelling approach and optimal control of greenhouse climate, In: S. El Hani, M. Essaaidi, Recent advances in electrical and information technologies for sustainable development, Advances in Science, Technology & Innovation, Cham: Springer, 2019,201–208. https://doi.org/10.1007/978-3-030-05276-8_22 |
[17] |
R. N. Premakumari, C. Baishya, M. E. Samei, M. K. Naik, A novel optimal control strategy for nutrient-phytoplankton-zooplankton model with viral infection in plankton, Commun. Nonlinear Sci. Numer. Simul., 137 (2024), 108157. https://doi.org/10.1016/j.cnsns.2024.108157 doi: 10.1016/j.cnsns.2024.108157
![]() |
[18] |
S. Vaidyanathan, Global stabilisation of control systems using a new sliding mode control method and its application to a general type of synchronisation for chaotic systems, Int. J. Model. Identif. Control, 40 (2022), 1–10. https://doi.org/10.1504/IJMIC.2022.124067 doi: 10.1504/IJMIC.2022.124067
![]() |
[19] |
M. K. Naik, C. Baishya, R. N. Premakumari, M. E. Samei, Navigating climate complexity and its control via hyperchaotic dynamics in a 4D Caputo fractional model, Sci. Rep., 14 (2024), 18015. https://doi.org/10.1038/s41598-024-68769-x doi: 10.1038/s41598-024-68769-x
![]() |
[20] |
M. Roohi, S. Mirzajani, A. Basse-O'Connor, A no-chatter single-input finite-time PID sliding mode control technique for stabilization of a class of 4D chaotic fractional order laser systems, Mathematics, 11 (2023), 21. https://doi.org/10.3390/math11214463 doi: 10.3390/math11214463
![]() |
[21] | Y. Kao, C. Wang, H. Xia, Y. Cao, Projective synchronization for uncertain fractional reaction-diffusion systems via adaptive sliding mode control based on finite-time scheme, In: Analysis and control for fractional-order systems, Singapore: Springer, 2024,141–163. https://doi.org/10.1007/978-981-99-6054-5_8 |
[22] |
Y. Cao, Y. Kao, Z. Wang, X. Yang, J. H. Park, W. Xie, Sliding mode control for uncertain fractional order reaction-diffusion memristor neural networks with time delays, Neural Networks, 178 (2024), 106402. https://doi.org/10.1016/j.neunet.2024.106402 doi: 10.1016/j.neunet.2024.106402
![]() |
[23] |
Y. Kao, Y. Cao, X. Chen, Global Mittag-Leffler synchronization of coupled delayed fractional reaction-diffusion Cohen-Grossberg neural networks via sliding mode control, Chaos, 32 (2022), 113123. https://doi.org/10.1063/5.0102787 doi: 10.1063/5.0102787
![]() |
[24] |
P. J. Roebber, Climate variability in a low-order coupled atmosphere-ocean model, Tellus A, 47 (1995), 473–494. https://doi.org/10.3402/tellusa.v47i4.11534 doi: 10.3402/tellusa.v47i4.11534
![]() |
[25] |
A. Chakraborty, P. Veeresha, Effects of global warming, time delay and chaos control on the dynamics of a chaotic atmospheric propagation model within the frame of Caputo fractional operator, Commun. Nonlinear Sci. Numer. Simul., 128 (2024), 107657. https://doi.org/10.1016/j.cnsns.2023.107657 doi: 10.1016/j.cnsns.2023.107657
![]() |
[26] |
R. N. Premakumari, C. Baishya, P. Veeresha, L. Akinyemi, A fractional atmospheric circulation system under the influence of a sliding mode controller, Symmetry, 14 (2022), 2618. https://doi.org/10.3390/sym14122618 doi: 10.3390/sym14122618
![]() |
[27] |
J. Singh, R. Agrawal, K. S. Nisar, A new forecasting behavior of fractional model of atmospheric dynamics of carbon dioxide gas, Partial Differ. Equ. Appl. Math., 9 (2024), 100595. https://doi.org/10.1016/j.padiff.2023.100595 doi: 10.1016/j.padiff.2023.100595
![]() |
[28] |
S. Etemad, S. K. Ntouyas, I. Stamova, J. Tariboon, On solutions of two post-quantum fractional generalized sequential Navier problems: an application on the elastic beam, Fractal Fract., 8 (2024), 236. https://doi.org/10.3390/fractalfract8040236 doi: 10.3390/fractalfract8040236
![]() |
[29] |
T. Kanwal, A. Hussain, İ. Avcı, S. Etemad, S. Rezapour, D. F. M. Torres, Dynamics of a model of polluted lakes via fractal-fractional operators with two different numerical algorithms, Chaos Soliton. Fract., 181 (2024), 114653. https://doi.org/10.1016/j.chaos.2024.114653 doi: 10.1016/j.chaos.2024.114653
![]() |
[30] |
P. Y. Dousseh, C. Ainamon, C. H. Miwadinou, A. V. Monwanou, J. B. C. Orou, Chaos in a financial system with fractional order and its control via sliding mode, Complexity, 2021 (2021), 4636658. https://doi.org/10.1155/2021/4636658 doi: 10.1155/2021/4636658
![]() |
[31] |
C. T. Deressa, S. Etemad, S. Rezapour, On a new four-dimensional model of memristor-based chaotic circuit in the context of nonsingular Atangana-Baleanu-Caputo operators, Adv. Differ. Equ., 2021 (2021), 444. https://doi.org/10.1186/s13662-021-03600-9 doi: 10.1186/s13662-021-03600-9
![]() |
[32] |
N. D. Phuong, F. M. Sakar, S. Etemad, S. Rezapour, A novel fractional structure of a multi-order quantum multi-integro-differential problem, Adv. Differ. Equ., 2020 (2020), 633. https://doi.org/10.1186/s13662-020-03092-z doi: 10.1186/s13662-020-03092-z
![]() |
[33] |
A. Amara, S. Etemad, S. Rezapour, Approximate solutions for a fractional hybrid initial value problem via the Caputo conformable derivative, Adv. Differ. Equ., 2020 (2020), 608. https://doi.org/10.1186/s13662-020-03072-3 doi: 10.1186/s13662-020-03072-3
![]() |
[34] |
H. Najafi, S. Etemad, N. Patanarapeelert, J. K. K. Asamoah, S. Rezapour, T. Sitthiwirattham, A study on dynamics of CD4+ T-cells under the effect of HIV-1 infection based on a mathematical fractal-fractional model via the Adams-Bashforth scheme and Newton polynomials, Mathematics, 10 (2022), 1366. https://doi.org/10.3390/math10091366 doi: 10.3390/math10091366
![]() |
[35] |
H. L. Li, L. Zhang, C. Hu, Y. L. Jiang, Z. Teng, Dynamical analysis of a fractional-order predator-prey model incorporating a prey refuge, J. Appl. Math. Comput., 54 (2017), 435–449. https://doi.org/10.1007/s12190-016-1017-8 doi: 10.1007/s12190-016-1017-8
![]() |
[36] |
H. Wang, Y. Yu, G. Wen, Dynamical analysis of the Lorenz-84 atmospheric circulation model, J. Appl. Math., 2014 (2014), 296279. https://doi.org/10.1155/2014/296279 doi: 10.1155/2014/296279
![]() |
[37] | A. T. Azar, S. Vaidyanathan, Chaos modeling and control systems design, Vol. 581, Springer International Publishing, Springer Cham, 2015. https://doi.org/10.1007/978-3-319-13132-0 |
[38] |
M. F. Danca, Lyapunov exponents of a discontinuous 4D hyperchaotic system of integer or fractional order, Entropy, 20 (2018), 337. https://doi.org/10.3390/e20050337 doi: 10.3390/e20050337
![]() |
[39] |
Z. Liu, J. Li, X. Di, A new hyperchaotic 4D-FDHNN system with four positive lyapunov exponents and its application in image encryption, Entropy, 24 (2022), 900. https://doi.org/10.3390/e24070900 doi: 10.3390/e24070900
![]() |
[40] | G. P. Williams, Chaos theory tamed, 1 Ed., CRC Press, 1997. https://doi.org/10.1201/9781482295412 |
[41] | K. Diethelm, An algorithm for the numerical solution of differential equations of fractional order systems, Electron. Trans. Numer. Anal., 5 (1997), 1–6. |
[42] |
N. Sene, Introduction to the fractional-order chaotic system under fractional operator in Caputo sense, Alexandria Eng. J., 60 (2021), 3997–4014. https://doi.org/10.1016/j.aej.2021.02.056 doi: 10.1016/j.aej.2021.02.056
![]() |
[43] |
K. Deng, C. Azorin-Molina, S. Yang, C. Hu, G. Zhang, L. Minola, et al., Changes of southern hemisphere westerlies in the future warming climate, Atmos. Res., 270 (2022), 106040. https://doi.org/10.1016/j.atmosres.2022.106040 doi: 10.1016/j.atmosres.2022.106040
![]() |
T | LE1 | LE2 | LE3 | LE4 |
100 | 0.6808 | 0.7047 | -0.5422 | 0.2999 |
200 | 0.8787 | -0.4683 | -1.2725 | 0.2999 |
300 | 0.9245 | -0.2770 | -1.0604 | 0.2999 |
400 | 0.3552 | -0.1723 | -0.9711 | 0.2998 |
500 | 0.4961 | -0.0017 | -1.2033 | 0.2998 |
600 | 0.6279 | -0.0093 | -1.1763 | 0.2997 |
700 | 0.6552 | 0.0670 | -1.1259 | 0.2995 |
800 | 0.8235 | 0.1597 | -1.2415 | 0.2993 |
900 | 0.8645 | 0.0018 | -1.1701 | 0.2989 |
1000 | 0.7542 | 0.0581 | -1.1636 | 0.2982 |
T | LE1 | LE2 | LE3 | LE4 |
100 | 1.9752 | -0.3531 | -1.5458 | 0.3722 |
200 | 1.5186 | -0.7385 | -1.8590 | 0.3722 |
300 | 1.0170 | -0.153 | -1.7393 | 0.3721 |
400 | 0.8684 | 0.0294 | -1.5641 | 0.3720 |
500 | 0.7705 | -0.0592 | -1.6395 | 0.3719 |
600 | 0.6112 | 0.0205 | -1.5097 | 0.3716 |
700 | 0.9984 | 0.0283 | -1.6295 | 0.3710 |
800 | 1.0916 | 0.0289 | -1.6073 | 0.3699 |
900 | 1.2012 | 0.0938 | -1.6858 | 0.3680 |
1000 | 1.3357 | 0.0448 | -1.7237 | 0.3643 |
T | LE1 | LE2 | LE3 | LE4 |
100 | 0.6808 | 0.7047 | -0.5422 | 0.2999 |
200 | 0.8787 | -0.4683 | -1.2725 | 0.2999 |
300 | 0.9245 | -0.2770 | -1.0604 | 0.2999 |
400 | 0.3552 | -0.1723 | -0.9711 | 0.2998 |
500 | 0.4961 | -0.0017 | -1.2033 | 0.2998 |
600 | 0.6279 | -0.0093 | -1.1763 | 0.2997 |
700 | 0.6552 | 0.0670 | -1.1259 | 0.2995 |
800 | 0.8235 | 0.1597 | -1.2415 | 0.2993 |
900 | 0.8645 | 0.0018 | -1.1701 | 0.2989 |
1000 | 0.7542 | 0.0581 | -1.1636 | 0.2982 |
T | LE1 | LE2 | LE3 | LE4 |
100 | 1.9752 | -0.3531 | -1.5458 | 0.3722 |
200 | 1.5186 | -0.7385 | -1.8590 | 0.3722 |
300 | 1.0170 | -0.153 | -1.7393 | 0.3721 |
400 | 0.8684 | 0.0294 | -1.5641 | 0.3720 |
500 | 0.7705 | -0.0592 | -1.6395 | 0.3719 |
600 | 0.6112 | 0.0205 | -1.5097 | 0.3716 |
700 | 0.9984 | 0.0283 | -1.6295 | 0.3710 |
800 | 1.0916 | 0.0289 | -1.6073 | 0.3699 |
900 | 1.2012 | 0.0938 | -1.6858 | 0.3680 |
1000 | 1.3357 | 0.0448 | -1.7237 | 0.3643 |