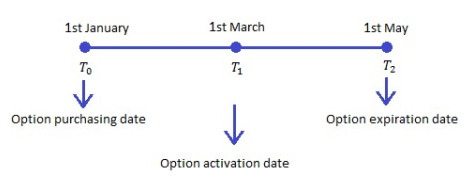
In this study, we derived pricing formulas for various forward-start style exotic options based on an uncertain stock models with periodic dividends. Specifically, we present valuations for forward-start, Cliquet/Ratchet, and spread options. In addition, we conducted numerical simulations of these formulas and compared them to pricing formulas for the same options based on a dividend-paying stock model driven by standard Brownian motion.
Citation: Javed Hussain, Saba Shahid, Tareq Saeed. Pricing forward-start style exotic options under uncertain stock models with periodic dividends[J]. AIMS Mathematics, 2024, 9(9): 24934-24954. doi: 10.3934/math.20241215
[1] | Hua Zhao, Yue Xin, Jinwu Gao, Yin Gao . Power-barrier option pricing formulas in uncertain financial market with floating interest rate. AIMS Mathematics, 2023, 8(9): 20395-20414. doi: 10.3934/math.20231040 |
[2] | Hanjie Liu, Yuanguo Zhu, Yiyu Liu . European option pricing problem based on a class of Caputo-Hadamard uncertain fractional differential equation. AIMS Mathematics, 2023, 8(7): 15633-15650. doi: 10.3934/math.2023798 |
[3] | Guiwen Lv, Ping Xu, Yanxue Zhang . Pricing of vulnerable options based on an uncertain CIR interest rate model. AIMS Mathematics, 2023, 8(5): 11113-11130. doi: 10.3934/math.2023563 |
[4] | Ming Yang, Yin Gao . Pricing formulas of binary options in uncertain financial markets. AIMS Mathematics, 2023, 8(10): 23336-23351. doi: 10.3934/math.20231186 |
[5] | Shaoling Zhou, Huixin Chai, Xiaosheng Wang . Barrier option pricing with floating interest rate based on uncertain exponential Ornstein–Uhlenbeck model. AIMS Mathematics, 2024, 9(9): 25809-25833. doi: 10.3934/math.20241261 |
[6] | Min-Ku Lee, Jeong-Hoon Kim . Closed-form approximate solutions for stop-loss and Russian options with multiscale stochastic volatility. AIMS Mathematics, 2023, 8(10): 25164-25194. doi: 10.3934/math.20231284 |
[7] | Jun Zhao, Peibiao Zhao . Quantile hedging for contingent claims in an uncertain financial environment. AIMS Mathematics, 2023, 8(7): 15651-15669. doi: 10.3934/math.2023799 |
[8] | Yurou Deng, Zhi Li, Liping Xu . Global attracting sets and exponential stability of nonlinear uncertain differential equations. AIMS Mathematics, 2023, 8(11): 26703-26714. doi: 10.3934/math.20231366 |
[9] | Xiaodie Lv, Yi Liu, Yihua Zhong . A novel method to solve the optimization problem of uncertain network system based on uncertainty theory. AIMS Mathematics, 2023, 8(3): 5445-5461. doi: 10.3934/math.2023274 |
[10] | Boling Chen, Guohe Deng . Analytic approximations for European-style Asian spread options. AIMS Mathematics, 2024, 9(5): 11696-11717. doi: 10.3934/math.2024573 |
In this study, we derived pricing formulas for various forward-start style exotic options based on an uncertain stock models with periodic dividends. Specifically, we present valuations for forward-start, Cliquet/Ratchet, and spread options. In addition, we conducted numerical simulations of these formulas and compared them to pricing formulas for the same options based on a dividend-paying stock model driven by standard Brownian motion.
A derivative is a financial instrument whose value is derived from the value of other assets, such as stocks or bonds. Options are a type of derivative that give the holder the right to buy or sell an asset at a specific price (strike price) at a specific time (expiration time). Options play an important role in finance because they can protect financial investors against loss on investments by balancing or compensating for transactions. The study of option pricing has a broad scope in finance because many derivative securities are in the form of options and all derivative securities follow the same pricing principles. Therefore, option pricing methods are useful for determining the pricing of various types of derivative securities [4].
In 1900, Brownian motion was first introduced as a model for the evolution of asset prices over time in a paper by B. Liu [24]. However, since Brownian motion can take on negative values, Geometric Brownian motion was later introduced as a more suitable model for asset pricing. In 1973, Black, Scholes [1], and Merton [26] presented a theory for pricing options using Geometric Brownian motion [24], which has since played a crucial role in finance. These methods, which are based on probability theory, have greatly impacted asset pricing theory.
A forward start option is a financial derivative contract in which the buyer pays for the option upfront, but the option is not activated until a predetermined future date. At the time of purchase t0, the activation date ta, expiration date te, underlying asset, and other terms of the option are agreed upon, but the strike price is not determined until the future activation date. The strike price is the price at which the buyer has the right, but not the obligation, to buy (call option) or sell (put option) the underlying asset. The future price of the underlying asset is also unknown at the time of purchase, and the option is typically structured to be "at the money" or near to the spot price of the underlying asset when it becomes active. The premium, or cost, of the option is usually established and paid in advance of the activation date.
In this paper, we derive pricing formulas for Forward Start, Ratchet/Cliquet, and Spread options, which are exotic options with unique payment structures that can be modified to meet risk tolerance and offer flexibility when exercising the option, although profit is not guaranteed. To derive these pricing formulas, we use the uncertain stock model with periodic dividends developed by Chen and Liu [9]. Numerical results based on this model are also presented.
It is well-known that the pricing problem of a forward starting option, prior to the determination of the strike price, can be transformed into the valuation of a plain vanilla option at the determination time using the Black-Scholes framework, as demonstrated in several publications such as [13,33] and [37]. Ramponi [28] also approached the problem of valuing a forward starting option, obtaining a solution in the form of an integral transform that can be evaluated numerically. A similar technique was used in [20] to price a forward starting option in the Heston stochastic volatility model. Additional literature on this topic, based on probability theory, can be found in [22].
It is understood that a large amount of historical data is needed to accurately determine a probability distribution of asset prices. On the other hand, in situations where no historical data is available or a real-world sample does not exist, it may be necessary to rely on the belief degrees of domain experts to evaluate the likelihood of different events. However, individual beliefs can vary, as noted in [23]. In cases where we are facing new and uncertain situations, it may not be possible to rely on experiences to make decisions. To address this type of uncertainty, the theory of uncertainty was introduced [23].
Cliquet options, also known as ratchet options or interval options, are financial derivative contracts that allow the buyer to receive periodic payments based on the difference between the strike price of the option and the spot price of the underlying asset. The strike price is reset at fixed intervals, and the option holder is entitled to receive a payment whenever the spot price exceeds the current strike price. Cliquet options are often used as a form of protection against potential declines in the value of the underlying asset, as the periodic reset of the strike price can provide some caution against loss. Cliquet options can be structured as either call options or put options, giving the holder the right to buy or sell the underlying asset, respectively (see [27]). Cliquet options can be viewed as a series of forward-starting, at-the-money options that offer a guaranteed minimum return, and a capped maximum payoff over the life of the contract. There is a significant amount of literature available on the pricing of Cliquet options using both probabilistic and partial differential equation (PDE) approaches [17].
Wilmott in [34] and [35] used numerical solutions to a partial differential equation (PDE) model to price Cliquet options. Bernard et al. in [5] obtained closed-form expressions for the prices and "Greeks" (sensitivity measures) of Cliquet options. Kruse and Nogel in [20] and Iseger and Oldenkamp in [20] studied the pricing of Cliquet options under a stochastic volatility model for the underlying asset. Gaudenzi in [12] derived a Cox-Ross-Rubinstein style formula for Cliquet options using a discrete-time framework. Korn, Temoçin, and Wenzel in [19] obtained an analytical approximation formula for Cliquet options based on the central limit theorem. Hess in [16] priced Cliquet options using jump-diffusion Lévy and Meixner processes, respectively. Shi and Zhang in [31] used frame duality projection to price Equity-Indexed Annuities (EIAs) with Cliquet-type guarantees under time-changed Lévy models. Haifeng, Jianqi, and Limin in [14] considered a jump-diffusion model for the underlying asset and priced Cliquet options based on such a stock.
A spread option is a financial derivative contract that gives the holder the right, but not the obligation, to buy or sell a specific basket of financial assets at a predetermined price. The underlying basket comprises two assets, one with long position and the other with short position. The value of the basket is then the spread between two financial assets, and the option payoff is the positive part of the difference between the spread and the strike. There is no additional payment based on the difference between the strike and the underlying assets in the basket. Spread options are often used to hedge against potential losses or to speculate on the relative performance of two different assets or financial instruments. The underlying instruments in a spread option can be stocks, bonds, commodities, currencies, or other financial instruments. Spread options are commonly used in the energy and agricultural sectors to hedge against price fluctuations in commodities. In the case of an EU (European-style) spread option, the difference in the values of the underlying assets at expiration is compared to a fixed or floating strike price. If the strike price is zero, the spread option is called an exchange option. A large amount of work has been done on the analytic and numerical pricing of spread options in a stochastic setting. Girma and Paulson in [11] showed that spread options can be used in a variety of trading strategies in financial markets. Bjerksund and Stensland in [2,3] derived closed-form formulas for spread options when the underlying assets follow long-normal dynamics, and Caldana, Ruggero, and Gianluca in [15] obtained more general closed-form formulas. Hurd and Zhou in [18] used the fast Fourier transform (FFT) to price spread options. Cheang and Garces in [7,10] and Ramponi in [29] assumed that the underlying assets follow a jump-diffusion model and priced spread and exchange options (i.e., zero strike spread options).
The protection of executive option agreements before signing a contract requires both historical data and domain expert belief degrees. However, obtaining sufficient historical data from the complex financial market may not always be possible. In these cases, it is necessary to analyze the belief degrees of domain experts, which indicate the likelihood of an indeterminate event and are heavily influenced by personal awareness and preferences for indeterminate factors. To mathematically represent these personal belief degrees, Liu introduced uncertainty theory in [23], which is based on the principles of normality, duality, subadditivity, and product axioms. Since then, significant progress has been made in the theory and applications of uncertainty theory, including in the areas of uncertain optimal control, uncertain programming, uncertain portfolio selection, and uncertain risk analysis. In decision-making, we often face uncertainty. There are two major approaches to dealing with this uncertainty: probability, which is based on the frequency of outcomes obtained from sampling, and uncertainty, which is based on the degree of belief assigned to an outcome by domain experts. While the probability of an outcome does not change with our knowledge, the degree of belief can be adjusted as our understanding of a situation evolves.
Now, we present some reasons why SDEs are not the good model for financial random processes, while uncertain differential equations could a good possible substitute. The example and explanation is based on[23]. Randomness follows the laws of probability theory, while uncertainty adheres to the laws of uncertainty theory. Probability is based on frequency, whereas uncertainty relies on belief degree. Practically, we distinguish them by examining the distribution function, if it closely matches the frequency, it is randomness; otherwise, it is uncertainty. Although many assume probability distribution can be easily derived from historical data, in reality, it often does not match the frequency closely enough, necessitating the use of uncertainty theory instead. For more details and other examples with the advantage of uncertain differential equations over stochastic differential equations, see the Appendix of [23]. Traditionally, stochastic finance theory assumes that stock prices (including interest rates and currency exchange rates) follow Ito's stochastic differential equation. However, this assumption was challenged by Liu in 2013. Assume the stock price Xt follows
dXt=eXtdt+σXtdWt | (B.5) |
where Wt is a Wiener process. The solution is
Xt=X0exp((e−σ2/2)t+σWt) | (B.6) |
and
Wt=lnXt−lnX0−(e−σ2/2)tσ | (B.7) |
ΔWt=lnXt+Δt−lnXt−(e−σ2/2)Δtσ | (B.8) |
Define
A=−(e−σ2/2)Δtσ | (B.9) |
Stock prices, though appearing continuous, are actually step functions with finite jumps. For example, with 100 jumps in a week divided into 10,000 intervals, ΔWt would include 9,900 values of A and 100 other values:
A,A,⋯,A⏟9900,B,C,⋯,Z⏟100 | (B.10) |
This distribution contradicts the Wiener process property that ΔWt is normally distributed with mean 0 and variance Δt. Hence, stock prices do not follow Ito's stochastic differential equations. Some might believe stock prices follow a geometric Wiener or Ornstein-Uhlenbeck process macroscopically, despite paradoxes microscopically. However, Ito's calculus, central to stochastic finance, is based on the microscopic Wiener process structure, not the macroscopic view.
The Geometric Liu Process (GLP) is known as the exponential of Canonical Process. We refer to a process often used to model the price of a stock as it evolves over time, and hence it is widely used to see the behavior of stock price. According to [30], when used to model the price of a stock over time, the GBM does not possess the flaws of the Liu process. Because it is the logarithm of the share prices that is assumed to be the uncertain normal random variable, the model does not allow for negative share prices. The expected returns of GLP are independent of the value of the process, which agrees with what we would expect in reality.
Since Liu's work, there has been a significant amount of research focused on uncertain finance. Many researchers, including Liu in [23], Liu and Ha in [25], Yao in [36], and Wang in [32], have contributed to the development of uncertainty theory. In 2008, Liu introduced the concept of an uncertain process and the uncertain differential equation [24], and in 2009, he developed the Canonical process and the uncertain stock model, including a pricing formula for European options [24]. In 2011, Chen extended this work by deriving a pricing formula for American options based on the uncertain stock model with periodic dividends [8].
The paper is organized as follows: In Section 2, we provide some supporting definitions. In Section 3, we introduce our uncertain stock model. In Section 4, we derive pricing formulas for the exotic options and present numerical results. Finally, in Section 5, we offer a brief conclusion.
Our main goal of the preliminary section is to provide an introduction to the basic theory of uncertainty, canonical processes, and key concepts in uncertainty theory to familiarize readers with these ideas.
Definition 2.1. Algebra. A family L of subsets of a non-empty set Γ is termed as an algebra on Γ if
a) Γ∈L
b) for any set, ∧∈L its complement ∧c∈L
c) if a sequence ∧j∈L,j=1,2,..., then ∪nj=1∧j∈L
Definition 2.2. [23] Uncertain measure. Uncertain measure M on a σ-algebra L is a number M{∧} assigned to the event ∧ to evaluate the degree of belief with which we believe that the event ∧ will occur. Uncertain measure M is a real-valued set-function on a σ-algebra L over a nonempty set Γ satisfying normality, duality, sub-additivity, and product axioms.
● Normality axiom: M{Γ}=1, for the Universal set Γ.
Normality is a property of a random variable that is distributed according to the normal distribution.
● Duality axiom: M{∧}+M{∧c}=1, for any set ∧∈Γ is an event.
It's a principle whereby one true statement can be obtained from another by interchanging two words.
● Monotonicity axiom: M{∧1}≤M{∧2}, for sets ∧1,∧2∈Γ such that ∧1⊂∧2
● Sub-additivity axiom: For any countable sequence of events, {∧i} we have,
M{⋃∞i=1∧i}≤∑∞i=1M{∧i}
The uncertain measure M{∧} indicates the degree of belief that ∧ will occur.
● Product axiom: let (Γk,Lk,M) be the uncertainty spaces for k∈N.
The product uncertain measure on the product σ-algebra (Lk)k∈N satisfying M{⋂∞k=1∧k}=∏∞k=1M{∧k}
It refers to an independence property which can not be applied to every set but it just holds for sure events (which always happens).
Definition 2.3. [23] Uncertain variable. An uncertain variable is a function from an uncertainty space (Γ,L,M) to the set of real numbers, i.e., for any Borel set B of real numbers, the set
{ξ∈B}={γ∈Γ|ξ(γ)∈B} |
is an event.
Definition 2.4 [23] Expectation. Let ξ be an uncertain variable. Then the expected value of ξ is defined by:
E[ξ]=∫+∞0M{ξ≥v}dv−∫0−∞M{ξ≤v}dv |
It can also be written as:
E[ξ]=∫+∞0(1−Φ(v))dv−∫0−∞Φ(v)dv |
Definition 2.5. [23], Variance. Let e is the finite-expected value of an uncertain variable ξ, then the variance of ξ can be defined as :
Var[ξ]=E[(ξ−e)2] |
Definition 2.6. [23] Canonical process. An uncertain process Ct is said to be a canonical process if:
● C0=0
● Ct has stationary and independent increments.
● Every increment Ct+s−Cs is a normal uncertain variable with expected value 0 and variance t2.
To define uncertain variables, a concept of the normal uncertain distribution \(\Phi: \mathbb{R} \rightarrow [0, 1]\) of an uncertain variable ξ is defined as [23,24]:
Φ(v)=M{ξ≤v}=(1+exp(−πv√3t))−1 | (2.1) |
1−Φ(v)=M{ξ≥v}=(1+exp(πv√3t))−1 | (2.2) |
where v is any real number.
Definition 2.7. [23] Uncertain differential equation. If Ct is a canonical Liu process, f1 and f2 are two real functions, then
dSt=f1(t,St)dt+f2(t,St)dCt | (2.3) |
is called uncertain differential equation with initial value S0.
The uncertain differential equation (2.3) is equivalent to uncertain integral equation,
St=S0+∫t0f1(t,St)dt+∫t0f2(t,St)dCt | (2.4) |
where St is the solution called Liu process that satisfies (2.3).
The ideas in this section are based on [9]. Let St be an uncertain stock model with periodic dividends, it is assumed that the stock follows some uncertain process. Hence, the stock price follows a canonical process. At deterministic times ta,te,T3,....., the equity pays a dividend of a fraction δ of the stock price. An uncertain stock model with periodic dividends can be modeled as:
ST=St(1−δ)n[T]exp(a(T−t)+σ(CT−Ct)),t<T. | (3.1) |
with risk-less bond:
Xt=X0exp(rt). | (3.2) |
where a and σ are supposed to be constants.
n[T] is the number of dividend payments made by time T.
n[T]=max{i;Ti≤T} |
Ct is a canonical process that is counter-part of Brownian motion. We are considering the uncertain stock model (3.1) to price the exotic options in the next sections.
In this section, we price the following exotic options under the uncertain stock model (3.1), using the uncertain approach.
● Forward Start Option
● Ratchet/Cliquet Option
● Spread Option
A forward start option is an exotic option that is purchased and paid today but becomes activated, at some specific time, in the future (see Figure 1). The expiration time and all other related parameters are set at the time when the option is purchased except the strike price. The strike price will be set at the time of activation so that the option is at-the-money (ATM), near-the-money (NTM), in-the-money (ITM), or out-of-the-money (OTM).
● At the money (ATM): The option is ATM when the spot price of the underlying asset is identical to the option's strike price.
● In the money (ITM): The option is ITM when the option's strike price is less than the spot price of the underlying asset.
● Near the money (NTM): The option is NTM when the option's strike price is near to the spot price of the underlying asset.
● Out of the money (OTM): The option is OTM when the option's strike price is greater than the spot price of the underlying asset.
Definition 4.1. A forward start call option gives the holder the right to buy at time ta an ATM European call option with an expiry date of te>ta. Hence, the payoff at te is:
Ψ(Sta,Ste)=max{Ste−Sta,0} |
● Sta=K is the strike price (also stock price) known at time ta.
● The option is bought at the current time t0.
● The option activates at time ta and matures at time te.
Theorem 4.1. Consider a forward start call option for the stock model (3.1) has a strike price K=Sta where Sta is the price of the stock at strike determination time ta. Then the forward start call option price is
Fc=Ste−r(te−t)(1−δ)n[te]∫∞StaSt(1−δ)n[te](1+exp(π√3σ(te−t)lny−πa√3σ))−1dy. |
Proof. At maturity time te, the payoff is:
Ψ(Sta,Ste)=max{Ste−Sta,0}. | (4.1) |
Forward start call option price is the expected present value of payoff:
For ta<t≤te
Fc=e−r(te−t)E[Ψ(Sta,Ste)]=e−r(te−t)E[(Ste−Sta)+] | (4.2) |
As, Ste is uncertain stock price
Ste=St(1−δ)n[te]ea(te−t)+σ(Cte−Ct) |
Applying uncertainty approach to find the expectation in (4.2).
Fc=e−r(te−t)∫∞0M{Ste−Sta≥v}dv=e−r(te−t)∫∞0M{St(1−δ)n[te]ea(te−t)+σ(Cte−Ct)≥v+Sta}dv=e−r(te−t)∫∞0M{ea(te−t)+σ(Cte−Ct)≥v+StaSt(1−δ)n[te]}dv=e−r(te−t)∫∞0M{a(te−t)+σ(Cte−Ct)≥ln(v+StaSt(1−δ)n[te])}dv=e−r(te−t)∫∞0M{(Cte−Ct)≥1σln(v+StaSt(1−δ)n[te])−a(te−t)σ}dv. |
Using substitution y=v+StaSt(1−δ)n[te] it follows that
Fc=Ste−r(te−t)(1−δ)n[te]∫∞StaSt(1−δ)n[te]M{(Cte−Ct)≥1σlny−a(te−t)σ}dy=Ste−r(te−t)(1−δ)n[te]∫∞StaSt(1−δ)n[te](1+exp(π√3σ(te−t)lny−πa√3σ))−1dy. | (4.3) |
Here Sta is strike price and te is expiry time.
Then, by definition (2.4) and (2.6), the theorem is proved
Once the option pricing formula is derived at expiration time te, the formulas for t0 and ta can be derived easily using (4.3).
For t=ta, the option price is:
Fc=Stae−r(te−ta)(1−δ)n[te]∫∞1(1−δ)n[te](1+exp(π√3σ(te−ta)lny−πa√3σ))−1dy. |
For t<ta, the option price is:
Fc=e−r(te−t)(1−δ)n[te]∫∞1(1−δ)n[te](1+exp(π√3σ(te−ta)lny−πa√3σ))−1dyE[Sta,] |
with,
E[Sta]=St(1−δ)n[ta]ea(ta−t)[∫∞0(1+exp(πlnv√3(ta−t)σ))−1dv−∫0−∞(1+exp(−πlnv√3(ta−t)σ))−1dv.] |
It is clear from the result (4.3) that the European call option price decreases as the interest rate r and strike price K increase.
In this section, we present numerical results comparing an uncertain stock model to a stochastic stock model. For the purposes of these simulations, we assume the following parameter values:
● The risk-free interest rate r=0.08
● Dividend δ=0.05
● Total number of periodic payments n=4
● The drift a=0.06
● The current underlying asset price St=40
● The expiry time te=1
The forward start call option prices against the strike prices are shown in Table 1 and Figure 2 for the uncertain stock model and stochastic stock model, with the strike determination time t=0.25 and the volatility σ=0.25.
Strike (Sta) | Stochastic Price | Uncertain Price |
10 | 23.1626 | 23.2487 |
15 | 18.4580 | 18.5404 |
20 | 14.0082 | 13.8412 |
25 | 11.0648 | 9.2296 |
30 | 9.6598 | 5.0858 |
35 | 7.7783 | 2.2123 |
Figure 2 illustrates the forward start call option price as a function of different strike prices based on both our uncertain stock model and the stochastic stock model. As can be seen in the figure, the option prices are a monotonically decreasing function of the strike price in both models. It is worth noting that as the strike price increases, the option price begins to decrease. The diagram also indicates that the cost of an option acquired through an uncertain framework is lower, making it more appealing to investors.
The uncertain stock model follows the uncertainty theory, and the stochastic stock model follows the probability theory. The uncertainty theory provides comparable and sometimes better results than probability theory for option pricing. In order to see difference in price for both models (uncertain and stochastic), figures are provided for all options.
The forward start call option prices against the different volatilities are shown in Table 2 and Figure 3 for the uncertain and stochastic model, with the strike determination time t=0.25 and the strike Sta=30.
Volatility (σ) | Stochastic Price | Uncertain Price |
0.3 | 7.5561 | 2.8194 |
0.42 | 7.6782 | 4.4283 |
0.54 | 8.2470 | 6.2832 |
0.66 | 8.9967 | 8.4366 |
0.78 | 9.8107 | 10.9581 |
0.9 | 10.6296 | 13.9407 |
Based on our uncertain stock model and the stochastic stock model, Figure 3 shows the forward start call option price as a function of different volatilities. It can be seen in the figure that the option price is a monotonically increasing function of volatility in both models. It is worth noting that as volatility increases, the option price also increases.
The forward start call option prices against the strike determination time are shown in Table 3 and Figure 4 for the uncertain stock model and stochastic stock model, with the strike ST1=30 and the volatility σ=0.25.
Strike determination time (t) | Stochastic Price | Uncertain Price |
0.1 | 3.5303 | 2.9451 |
0.26 | 2.5354 | 2.1650 |
0.42 | 1.5579 | 1.4351 |
0.58 | 6.9129 | 0.7747 |
0.72 | 1.2608 | 0.2426 |
0.9 | 1.6313 | 0.0041 |
According to our uncertain stock model and stochastic stock model, Figure 4 shows the forward start call option price with regard to different strike determination times. In Figure 4, it is concluded that the option price is a monotonically decreasing function of different strike determination times in both approaches. It must be noted that when the strike determination time is getting high, the option price starts to fall. The figure additionally suggests that the price of an option obtained through an uncertain framework is reduced, rendering it more enticing for investors.
A ratchet option is a series of ATM forward start options. The term ratchet is used to explain the resetting property. The first option becomes active by setting its strike immediately, and the second option will become active at the expiry time of the first option and so on, and the strike price will be the same as the spot price of the underlying asset at that time. It is also known as the strike reset option because the strike price is adjusted in all successive options to meet the market realities. The total premium will be paid at once [6].
The payoff from time Ti−1 to Ti is:
Ψ(STi)=max{STi−Ki−1,0}=max{STi−STi−1,0} |
It will be paid at expiry time Ti, where the strike price Ki−1 is reset to the strike price STi−1 at time Ti−1.
We consider a 3-leg ratchet call option and will assume that i=0,1,2,3. The overall payoff at expiry time T3 is:
Ψ(ST1,ST2,ST3)=max{ST3−K2,0}+max{ST2−K1,0}+max{ST1−K0,0}=max{ST3−ST2,0}+max{ST2−ST1,0}+max{ST1−K0,0} |
Theorem 4.2. Consider a ratchet call option for the stock model (3.1) has a strike price
● At the time t0 the initial strike is K0.
● At time T1 the strike is reset to K1=ST1.
● At time T2 the strike is reset to K2=ST2.
● At option expiry time, T3 the holder will immediately lock-in the profits with strike K2=ST2.
Then the ratchet call option price is:
Rc=St(1−δ)n[T3]e−r(T3−t)∫∞ST2St(1−δ)n[T3](1+exp(π√3σ(T3−t)lny−πa√3σ))−1dy+e−r(T3−t)max{ST2−ST1,0}+e−r(T3−t)max{ST1−K0,0} |
Proof. The price of an option is the expected present value of the payoff:
Rc=e−r(T3−t)E[Ψ(ST1,ST2,ST3)]. |
For T2<t≤T3. The option price is:
Rc=e−r(T3−t)E[Ψ(ST1,ST2,ST3)]=e−r(T3−t)E[max{ST3−ST2,0}+max{ST2−ST1,0}+max{ST1−K10,0}]=e−r(T3−t)E[max{ST3−ST2,0}]+e−r(T3−t)max{ST2−ST1,0}+e−r(T3−t)max{ST1−K0,0}. | (4.4) |
The information at T1 and T2 is known.
To compute the expectation uncertain measure is applying.
E[max{ST3−ST2,0}]=∫∞0M{ST3−ST2≥v}dv=∫∞0M{St(1−δ)n[T3]ea(T3−t)+σ(CT3−Ct)≥v+ST2}dv=∫∞0M{ea(T3−t)+σ(CT3−Ct)≥v+ST2St(1−δ)n[T3]}dv=∫∞0M{a(T3−t)+σ(CT3−Ct)≥ln(v+ST2St(1−δ)n[T3])}dv=∫∞0M{(CT3−Ct)≥1σln(v+ST2St(1−δ)n[T3])−a(T3−t)σ}dv.c |
Using substitution y=v+ST2St(1−δ)n[T3] it follows that,
E[max{ST3−ST2,0}]=St(1−δ)n[T3]∫∞ST2St(1−δ)n[T3]M{(CT3−Ct)≥1σlny−a(T3−t)σ}dy=St(1−δ)n[T3]∫∞ST2St(1−δ)n[T3](1+exp(π√3σ(T3−t)lny−πa√3σ))−1dy. |
Plugging result in (4.4) provides,
Rc=St(1−δ)n[T3]e−r(T3−t)∫∞ST2St(1−δ)n[T3](1+exp(π√3σ(T3−t)lny−πa√3σ))−1dy+e−r(T3−t)max{ST2−ST1,0}+e−r(T3−t)max{ST1−K0,0}. | (4.5) |
The Ratchet call option price against different strike prices are shown in Table 4 and Figure 5 for the uncertain stock model and stochastic stock model, with the strike determination time t=0.25 and the volatility σ=0.25.
Strike (ST1) | Stochastic Price | Uncertain Price |
10 | 24.8774 | 24.968 |
15 | 20.1703 | 20.259 |
20 | 15.6100 | 15.556 |
25 | 12.2082 | 10.907 |
30 | 10.5748 | 6.563 |
35 | 9.0153 | 3.191 |
Based on our uncertain stock model and the stochastic stock model, Figure 5 illustrates the ratchet call option price as a function of various strike prices. The figure clearly shows that the price of option obtained through uncertain framework is cheaper, and hence more attractive for investors. It can be seen in the figure that the option price is a monotonically decreasing function of the strike price in both models. It is worth noting that as the strike price increases, the option price begins to decrease, because a near-the-money ratchet call option will always be exercised at expiration.
A spread option is a type of option where the profit is determined by the difference, or spread, in spot price between two underlying assets. Spread options are traded over the counter (OTC), which means they are facilitated by a dealer and involve a private transaction between the buyer and seller. These options provide investors with flexibility in terms of the expiration date and strike price.
Let {C(1)t:t≥0} and {C(2)t:t≥0} be Canonical processes on uncertainty space (Γ,L,M), and let asset prices S1t and S2t satisfy the following uncertain differential equations:
S(1)T=S(1)t(1−δ1)n[T]ea1(T−t)+σ1(C(1)T−C(1)t) | (4.6) |
S(2)T=S(2)t(1−δ2)n[T]ea2(T−t)+σ2(C(2)T−C(2)t) | (4.7) |
where σ1,σ2 and a1,a2 are assumed to be constant and n[t] is the number of dividend payments made by time t with periodic dividend δ1 and δ2
n[t]=max{i;Ti≤t}. |
At time t<T the payoff is:
Ψ(S(1)T,S(2)T)=max{S(1)T−S(2)T−K,0}. |
Theorem 4.3. Consider a spread call option for the stock model (4.6) and (4.7) has a strike price K≥0 where S1T and S2T is the price of the stock at strike determination time t<T. Then, the Spread call option price is
Sc=S(1)t(1−δ)n[T]e−r(T−t)∫∞S(2)T+KS(1)t(1−δ)n[T](1+exp(π√3σ1(T−t)lny−πa1√3σ1))−1dy. |
Proof. For a strike price K≥0, the price of a spread call option at time t<T is the expected value of the payoff:
Sc=e−r(T−t)E[Ψ(S(1)T,S(2)T)]=e−r(T−t)E[(S(1)T−S(2)T−K)+]. | (4.8) |
We will derive a formula for the spread call using Kirk's approximation [21]. As there are two underlying assets in the spread option price, Kirk's approximation formula is an option pricing formula applicable to an option to exchange one risky asset for another risky asset at maturity by assuming that Strike (K) is non-zero. This strategy is feasible, but not an optimal exercise strategy. It is assumed that the implicit strategy is to exercise if and only if S(2)T is the scaled power function of S(1)T.
Computing expectation in (4.8) by using an uncertain process provides
Sc=e−r(T−t)E[Ψ(S(1)T,S(2)T)]=e−r(T−t)E[(S(1)T−S(2)T−K)+]=e−r(T−t)∫∞0M{S(1)T−S(2)T−K≥v}dv=e−r(T−t)∫∞0M{S(1)T≥v+S(2)T+K}dv. |
Here
S(1)T=S(1)t(1−δ)n[T]ea1(T−t)+σ1(C(1)T−C(1)t) |
we infer that
Sc=e−r(T−t)∫∞0M{S(1)t(1−δ)n[T]ea1(T−t)+σ1(C(1)T−C(1)t)≥v+S(2)T+K}dv=e−r(T−t)∫∞0M{ea1(T−t)+σ1(C(1)T−C(1)t)≥v+S(2)T+KS(1)t(1−δ)n[T]}dv=e−r(T−t)∫∞0M{(C(1)T−C(1)t)≥1σ1ln(v+S(2)T+KS(1)t(1−δ)n[T])−a1(T−t)σ1}dv. |
Using substitution y=v+S(2)T+KS(1)t(1−δ)n[T] it follows that,
Sc=S(1)t(1−δ)n[T]e−r(T−t)∫∞S(2)T+KS(1)t(1−δ)n[T]M{(C(1)T−C(1)t)≥1σ1lny−a1(T−t)σ1}dy=S(1)t(1−δ)n[T]e−r(T−t)∫∞S(2)T+KS(1)t(1−δ)n[T](1+exp(π√3σ1(T−t)lny−πa1√3σ1))−1dy. |
The spread option prices against different strike prices are shown in Table 5 and Figure 6 for the uncertain stock model and stochastic stock model, with the strike determination time t=0.25 and the volatility σ=0.25.
Strike (ST1) | Stochastic Price | Uncertain Price |
10 | 19.6793 | 19.989 |
15 | 16.0907 | 15.445 |
20 | 14.0879 | 11.112 |
25 | 12.8570 | 7.309 |
30 | 11.1454 | 4.407 |
35 | 8.7249 | 2.518 |
Based on our uncertain stock model and the stochastic stock model, Figure 6 illustrates the spread call option price as a function of various strike prices. It can be seen in the figure that the option price is a monotonically decreasing function of the strike price in both models. It is worth noting that as the strike price increases, the option price begins to decrease because a near-the-money spread call option will always be exercised at expiration.
The spread option prices against different volatilities are shown in Table 6 and Figure 7 for the uncertain stock model and stochastic stock model, with the strike determination time t=0.25 and the strike Sta=30.
Volatility (σ) | Stochastic Price | Uncertain Price |
0.2 | 8.6312 | 21.643 |
0.34 | 8.8960 | 4.358 |
0.48 | 9.6399 | 7.915 |
0.62 | 10.7336 | 12.513 |
0.76 | 11.9363 | 18.516 |
0.9 | 13.1170 | 26.750 |
According to our uncertain stock model and stochastic stock model, Figure 7 shows the spread call option price with regard to a function of different volatilities. It can be seen in the figure that the option price is a monotonically increasing function of volatility in both models. It is worth noting that as volatility increases, the option price also increases. It must be noted that when the volatility is getting high, the option price starts to rise.
In this study, we used uncertainty theory to price exotic options such as forward start, ratchet/cliquet, and spread options. We derived call option formulas for these options using the method of uncertain calculus, assuming that the underlying stock price follows an uncertain stock model with periodic dividends. To verify the accuracy of our newly derived formulas, we conducted numerical simulations and compared the results with those obtained using a stochastic approach. Overall, this research presents a novel method for pricing exotic options using the framework of uncertainty theory.
Javed Hussain: Conceptualization, Methodology, Formal analysis, Writing-original draft and editing; Saba shahid: Writing-review, Polish language; Tareq saeed: Writing-review and editing, supervision. All authors have read and approved the final version of the manuscript for publication.
The authors declare they have not used Artificial Intelligence (AI) tools in the creation of this article.
We would like to thank you for following the instructions above very closely in advance. It will definitely save us lot of time and expedite the process of your paper's publication.
The authors have not disclose any competing interests.
[1] |
F. Black, M. Scholes, The pricing of options and corporate liabilities, J. Polit. Econ., 81 (1973), 637–654. https://doi.org/10.1086/260062 doi: 10.1086/260062
![]() |
[2] | P. Bjerksund, B. Myksvoll, G. Stensland, Managing flexible load contracts: two simple strategies (December 1, 2006), NHH Dept. of Finance & Management Science Discussion Paper No. 2006/20. https://doi.org/10.2139/ssrn.1145206 |
[3] |
P. Bjerksund, G. Stensland, Closed form spread option valuation, Quant. Financ., 14 (2014), 1785–1794. https://doi.org/10.1080/14697688.2011.617775 doi: 10.1080/14697688.2011.617775
![]() |
[4] | Z. Brzezniak, T. Zastawniak, Basic stochastic processes, London: Springer, 1999. http://doi.org/10.1007/978-1-4471-0533-6 |
[5] |
C. Bernard, W. V. Li, Pricing and hedging of cliquet options and locally capped contracts, SIAM J. Financ. Math., 4 (2013), 353–371. http://doi.org/10.1137/100818157 doi: 10.1137/100818157
![]() |
[6] | G. W. Buetow Jr., Ratchet options, Journal of Financial and Strategic Decisions, 12 (1999), 17–30. |
[7] |
G. H. L. Cheang, L. P. D. M. Garces, Representation of exchange option prices under stochastic volatility jump-diffusion dynamics, Quant. Financ., 20 (2020), 291–310. http://doi.org/10.1080/14697688.2019.1655785 doi: 10.1080/14697688.2019.1655785
![]() |
[8] | X. Chen, American option pricing formula for uncertain financial market, International Journal of Operations Research, 8 (2011), 27–32. |
[9] |
X. Chen, Y. Liu, D. A. Ralescu, Uncertain stock model with periodic dividends, Fuzzy Optim. Dcis. Making, 12 (2013), 111–123. http://doi.org/10.1007/s10700-012-9141-x doi: 10.1007/s10700-012-9141-x
![]() |
[10] |
L. P. D. M. Garces, G. H. L. Cheang, A numerical approach to pricing exchange options under stochastic volatility and jump-diffusion dynamics, Quant. Financ., 21 (2021), 2025–2054. http://doi.org/10.1080/14697688.2021.1926534 doi: 10.1080/14697688.2021.1926534
![]() |
[11] |
P. B. Girma, A. S. Paulson, Seasonality in petroleum futures spreads, J. Futures Markets, 18 (1998), 581–598. http://doi.org/10.1002/(sici)1096-9934(199808)18:5<581::aid-fut5>3.0.co;2-1 doi: 10.1002/(sici)1096-9934(199808)18:5<581::aid-fut5>3.0.co;2-1
![]() |
[12] |
M. Gaudenzi, A. Zanette, Pricing cliquet options by tree methods, Comput. Manag. Sci, , 8 (2011), 125–135. http://doi.org/10.1007/s10287-009-0109-4 doi: 10.1007/s10287-009-0109-4
![]() |
[13] |
J.-H. Guo, M.-W. Hung, A generalization of Rubinstein's "pay now, choose later", J. Futures Markets, 28 (2008), 488–515. http://doi.org/10.1002/fut.20311 doi: 10.1002/fut.20311
![]() |
[14] |
Y. Haifeng, Y. Jianqi, L. Limin, Pricing cliquet options in jump-diffusion models, Stoch. Models, 21 (2005), 875–884. http://doi.org/10.1080/15326340500294587 doi: 10.1080/15326340500294587
![]() |
[15] |
J. Helwege, J.-Z. Huang, Y. Wang, Liquidity effects in corporate bond spreads, J. Bank. Financ., 45 (2014), 105–116. http://doi.org/10.1016/j.jbankfin.2013.08.018 doi: 10.1016/j.jbankfin.2013.08.018
![]() |
[16] |
M. Hess, Cliquet option pricing with Meixner processes, Mod. Stoch.-Theory App., 5 (2018), 81–97. http://doi.org/10.15559/18-VMSTA96 doi: 10.15559/18-VMSTA96
![]() |
[17] |
M. Hess, Cliquet option pricing in a jump-diffusion Lévy model, Mod. Stoch.-Theory App., 5 (2018), 317–336. http://doi.org/10.15559/18-vmsta107 doi: 10.15559/18-vmsta107
![]() |
[18] |
T. R. Hurd, Z. Zhou, A Fourier transform method for spread option pricing, SIAM J. Financ. Math., 1 (2010), 142–157. http://doi.org/10.1137/090750421 doi: 10.1137/090750421
![]() |
[19] |
R. Korn, B. Z. Temoçin, J. Wenzel, Applications of the central limit theorem for pricing Cliquet-style options, Eur. Actuar. J., 7 (2017), 465–480. http://doi.org/10.1007/s13385-017-0158-y doi: 10.1007/s13385-017-0158-y
![]() |
[20] |
S. Kruse, U. Nögel, On the pricing of forward starting options in Hestons model on stochastic volatility, Finance Stochast., 9 (2005), 233–250. http://doi.org/10.1007/s00780-004-0146-3 doi: 10.1007/s00780-004-0146-3
![]() |
[21] |
M. Li, S.-J. Deng, J. Zhoc, Closed-form approximations for spread option prices and Greeks, The Journal of Derivatives, 15 (2008), 58–80. http://doi.org/10.3905/jod.2008.702506 doi: 10.3905/jod.2008.702506
![]() |
[22] |
S. Lin, X.-J. He, A closed-form pricing formula for forward start options under a regime-switching stochastic volatility model, Chaos Soliton. Fract., 144 (2021), 110644. http://doi.org/10.1016/j.chaos.2020.110644 doi: 10.1016/j.chaos.2020.110644
![]() |
[23] | B. Liu, Uncertainty theory, In: Uncertainty theory, Berlin, Heidelberg: Springer, 2007,205–234. http://doi.org/10.1007/978-3-540-73165-8_5 |
[24] | B. Liu, Fuzzy process, hybrid process and uncertain process, Journal of Uncertain Systems, 2 (2008), 3–16. |
[25] | Y. H. Liu, M. Ha, Expected value of function of uncertain variables, Journal of Uncertain Systems, 4 (2010), 181–186. |
[26] |
R. C. Merton, Theory of rational option pricing, The Bell Journal of Economics and Management Science, 4 (1973), 141–183. http://doi.org/10.2307/3003143 doi: 10.2307/3003143
![]() |
[27] | N. Patel, The evolving art of pricing cliquets, Risk, 15 (2002), 22–24. |
[28] |
A. Ramponi, Fourier transform methods for regime-switching jump-diffusions and the pricing of forward starting options, Int. J. Theor. Appl. Fin., 15 (2012), 1250037. http://doi.org/10.1142/s0219024912500379 doi: 10.1142/s0219024912500379
![]() |
[29] |
A. Ramponi, Spread option pricing in regime-switching jump diffusion models, Mathamatics, 10 (2022), 1574. http://doi.org/10.3390/math10091574 doi: 10.3390/math10091574
![]() |
[30] | S. M. Ross, An elementary introduction to mathematical finance, Cambridge: Cambridge University Press, 2011. https://doi.org/10.1017/CBO9780511921483 |
[31] |
B. Shi, Z. Zhang, Pricing EIA with cliquet-style guarantees under time-changed Lévy models by frame duality projection, Commun. Nonlinear Sci. Numer. Simulat., 95 (2021), 105651. http://doi.org/10.1016/j.cnsns.2020.105651 doi: 10.1016/j.cnsns.2020.105651
![]() |
[32] |
X. Wang, Z. Gao, H. Guo, Uncertain hypothesis testing for two experts' empirical data, Math. Comput. Model., 55 (2012), 1478–1482. http://doi.org/10.1016/j.mcm.2011.10.039 doi: 10.1016/j.mcm.2011.10.039
![]() |
[33] | P. Wilmott, Derivatives: The theory and practice of financial engineering, Wiley, 1998. |
[34] | P. Wilmott, Cliquet options and volatility models, Wilmott Magazine, 2002, 78–83. |
[35] |
H. A. Windcliff, P. A. Forsyth, K. R. Vetzal, Numerical methods and volatility models for valuing cliquet options, Applied Mathematical Finance, 13 (2006), 353–386. http://doi.org/10.1080/13504860600839964 doi: 10.1080/13504860600839964
![]() |
[36] |
K. Yao, Uncertain calculus with renewal process, Fuzzy Optim. Decis. Making, 11 (2012), 285–297. http://doi.org/10.1007/s10700-012-9132-y doi: 10.1007/s10700-012-9132-y
![]() |
[37] | P. G. Zhang, Exotic options: A guide to second generation options, 2 Eds., World Scientific, 1998. |
Strike (Sta) | Stochastic Price | Uncertain Price |
10 | 23.1626 | 23.2487 |
15 | 18.4580 | 18.5404 |
20 | 14.0082 | 13.8412 |
25 | 11.0648 | 9.2296 |
30 | 9.6598 | 5.0858 |
35 | 7.7783 | 2.2123 |
Volatility (σ) | Stochastic Price | Uncertain Price |
0.3 | 7.5561 | 2.8194 |
0.42 | 7.6782 | 4.4283 |
0.54 | 8.2470 | 6.2832 |
0.66 | 8.9967 | 8.4366 |
0.78 | 9.8107 | 10.9581 |
0.9 | 10.6296 | 13.9407 |
Strike determination time (t) | Stochastic Price | Uncertain Price |
0.1 | 3.5303 | 2.9451 |
0.26 | 2.5354 | 2.1650 |
0.42 | 1.5579 | 1.4351 |
0.58 | 6.9129 | 0.7747 |
0.72 | 1.2608 | 0.2426 |
0.9 | 1.6313 | 0.0041 |
Strike (ST1) | Stochastic Price | Uncertain Price |
10 | 24.8774 | 24.968 |
15 | 20.1703 | 20.259 |
20 | 15.6100 | 15.556 |
25 | 12.2082 | 10.907 |
30 | 10.5748 | 6.563 |
35 | 9.0153 | 3.191 |
Strike (ST1) | Stochastic Price | Uncertain Price |
10 | 19.6793 | 19.989 |
15 | 16.0907 | 15.445 |
20 | 14.0879 | 11.112 |
25 | 12.8570 | 7.309 |
30 | 11.1454 | 4.407 |
35 | 8.7249 | 2.518 |
Volatility (σ) | Stochastic Price | Uncertain Price |
0.2 | 8.6312 | 21.643 |
0.34 | 8.8960 | 4.358 |
0.48 | 9.6399 | 7.915 |
0.62 | 10.7336 | 12.513 |
0.76 | 11.9363 | 18.516 |
0.9 | 13.1170 | 26.750 |
Strike (Sta) | Stochastic Price | Uncertain Price |
10 | 23.1626 | 23.2487 |
15 | 18.4580 | 18.5404 |
20 | 14.0082 | 13.8412 |
25 | 11.0648 | 9.2296 |
30 | 9.6598 | 5.0858 |
35 | 7.7783 | 2.2123 |
Volatility (σ) | Stochastic Price | Uncertain Price |
0.3 | 7.5561 | 2.8194 |
0.42 | 7.6782 | 4.4283 |
0.54 | 8.2470 | 6.2832 |
0.66 | 8.9967 | 8.4366 |
0.78 | 9.8107 | 10.9581 |
0.9 | 10.6296 | 13.9407 |
Strike determination time (t) | Stochastic Price | Uncertain Price |
0.1 | 3.5303 | 2.9451 |
0.26 | 2.5354 | 2.1650 |
0.42 | 1.5579 | 1.4351 |
0.58 | 6.9129 | 0.7747 |
0.72 | 1.2608 | 0.2426 |
0.9 | 1.6313 | 0.0041 |
Strike (ST1) | Stochastic Price | Uncertain Price |
10 | 24.8774 | 24.968 |
15 | 20.1703 | 20.259 |
20 | 15.6100 | 15.556 |
25 | 12.2082 | 10.907 |
30 | 10.5748 | 6.563 |
35 | 9.0153 | 3.191 |
Strike (ST1) | Stochastic Price | Uncertain Price |
10 | 19.6793 | 19.989 |
15 | 16.0907 | 15.445 |
20 | 14.0879 | 11.112 |
25 | 12.8570 | 7.309 |
30 | 11.1454 | 4.407 |
35 | 8.7249 | 2.518 |
Volatility (σ) | Stochastic Price | Uncertain Price |
0.2 | 8.6312 | 21.643 |
0.34 | 8.8960 | 4.358 |
0.48 | 9.6399 | 7.915 |
0.62 | 10.7336 | 12.513 |
0.76 | 11.9363 | 18.516 |
0.9 | 13.1170 | 26.750 |