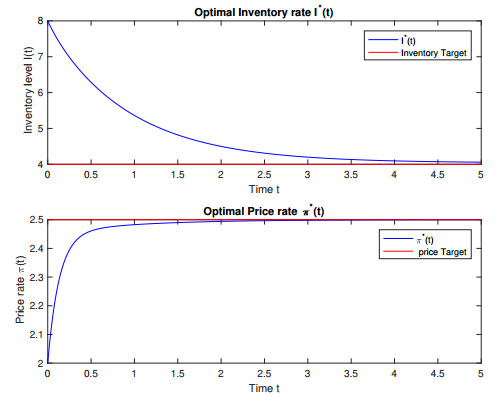
We witness an increased emphasis on the integration of different areas of manufacturing firms with the intention of avoiding suboptimal solutions. In particular, due to new technologies which make it easy to change the price of a product in real time, the integration of pricing and production planning may be garnering the most interest. We are proposing in this paper a way to model the dynamics of the price. Thus, the price and the inventory level are considered as state variables whereas the supply (production) rate is the control variable. The demand rate is dynamic and state-dependent. Using a model predictive control approach, the optimal supply rate, and thus the optimal price and inventory level, are obtained. Different examples are provided under different scenarios for the supply rate and for the demand rate.
Citation: Messaoud Bounkhel, Lotfi Tadj. Model predictive control based integration of pricing and production planning[J]. AIMS Mathematics, 2024, 9(1): 2282-2307. doi: 10.3934/math.2024113
[1] | Avijit Duary, Md. Al-Amin Khan, Sayan Pani, Ali Akbar Shaikh, Ibrahim M. Hezam, Adel Fahad Alrasheedi, Jeonghwan Gwak . Inventory model with nonlinear price-dependent demand for non-instantaneous decaying items via advance payment and installment facility. AIMS Mathematics, 2022, 7(11): 19794-19821. doi: 10.3934/math.20221085 |
[2] | Spyridon D. Mourtas, Emmanouil Drakonakis, Zacharias Bragoudakis . Forecasting the gross domestic product using a weight direct determination neural network. AIMS Mathematics, 2023, 8(10): 24254-24273. doi: 10.3934/math.20231237 |
[3] | Xiaoyi Tang, Wei Liu, Wanyin Wu, Yijun Hu . An optimal investment strategy for DC pension plans with costs and the return of premium clauses under the CEV model. AIMS Mathematics, 2024, 9(8): 21731-21754. doi: 10.3934/math.20241057 |
[4] | Jun Wang, Dan Wang, Yuan Yuan . Research on low-carbon closed-loop supply chain strategy based on differential games-dynamic optimization analysis of new and remanufactured products. AIMS Mathematics, 2024, 9(11): 32076-32101. doi: 10.3934/math.20241540 |
[5] | Yao Fu, Sisi Zhou, Xin Li, Feng Rao . Multi-assets Asian rainbow options pricing with stochastic interest rates obeying the Vasicek model. AIMS Mathematics, 2023, 8(5): 10685-10710. doi: 10.3934/math.2023542 |
[6] | Shaoling Zhou, Huixin Chai, Xiaosheng Wang . Barrier option pricing with floating interest rate based on uncertain exponential Ornstein–Uhlenbeck model. AIMS Mathematics, 2024, 9(9): 25809-25833. doi: 10.3934/math.20241261 |
[7] | Guiwen Lv, Ping Xu, Yanxue Zhang . Pricing of vulnerable options based on an uncertain CIR interest rate model. AIMS Mathematics, 2023, 8(5): 11113-11130. doi: 10.3934/math.2023563 |
[8] | Salvatore Mancha Gonzales, Hasnain Iftikhar, Javier Linkolk López-Gonzales . Analysis and forecasting of electricity prices using an improved time series ensemble approach: an application to the Peruvian electricity market. AIMS Mathematics, 2024, 9(8): 21952-21971. doi: 10.3934/math.20241067 |
[9] | Yuejiao Wang, Chenguang Cai . Equilibrium strategies of customers and optimal inventory levels in a make-to-stock retrial queueing system. AIMS Mathematics, 2024, 9(5): 12211-12224. doi: 10.3934/math.2024596 |
[10] | Nisa Özge Önal Tuğrul, Kamil Karaçuha, Esra Ergün, Vasil Tabatadze, Ertuğrul Karaçuha . A novel modeling and prediction approach using Caputo derivative: An economical review via multi-deep assessment methodology. AIMS Mathematics, 2024, 9(9): 23512-23543. doi: 10.3934/math.20241143 |
We witness an increased emphasis on the integration of different areas of manufacturing firms with the intention of avoiding suboptimal solutions. In particular, due to new technologies which make it easy to change the price of a product in real time, the integration of pricing and production planning may be garnering the most interest. We are proposing in this paper a way to model the dynamics of the price. Thus, the price and the inventory level are considered as state variables whereas the supply (production) rate is the control variable. The demand rate is dynamic and state-dependent. Using a model predictive control approach, the optimal supply rate, and thus the optimal price and inventory level, are obtained. Different examples are provided under different scenarios for the supply rate and for the demand rate.
One of the critical drivers in the management of value chains is supply and demand matching. Demand swings can occur monthly, daily or even hourly. Firms have to even out the demand to avoid lost sales and to avoid increasing capacity cushions. The marketing department can influence the demand through means such as offering complementary services and products, offering promotional pricing, using pre-scheduled appointments and reservations, allowing backlogs, backorders and stockouts and/or implementing revenue management.
Revenue management, also called yield management, appeared first in the service sector. It is the process of adjusting price at the right time to maximize revenue. It has been used successfully by such industries as airlines, hotels, cruise lines, car rental agencies, etc. In revenue management, computer software can make updates in real time, using decision rules for opening or closing price categories depending on parameters such as the difference between capacity and demand, production schedules or inventory levels.
A number of industries, such as Dell, Alibaba Group, and Amazon.com have adopted dynamic pricing strategies and sell products directly to customers through their websites. Among the factors that contributed to this phenomenon are the availability of demand data, the ease of adjusting prices thanks to new technologies and the availability of decision-support systems for analysis of the demand data and dynamic pricing.
It has been observed that research on pricing and research on production planning differ in the way they look at the demand rate. Research on production planning assumes that the demand rate is determined exogenously and therefore is uncontrollable. However, the demand rate can often be controlled by varying the price structure. For this reason, pricing research often focuses on demand function properties. The artificial separation of production planning and pricing can only lead to suboptimal solutions.
These considerations have led to an increased interest in the development of models integrating pricing and production decisions to improve the profitability of companies. Among the early references is the work of Whitin [1] who analyzed the newsvendor problem with price-dependent demand. Since then there has been a considerable amount of literature that deals with the interface between marketing and manufacturing decisions, i.e., the simultaneous determination of pricing and production. Also, many review papers have appeared. Among them are those written by Eliashberg and Steinberg [2], Elmaghraby and Keskinocak [3], Chan et al. [4], Simchi-Levi et al. [5], Yano and Gilbert [6], Niu et al. [7], Chen and Simchi-Levi [8], Zhang [9], and den Boer [10].
Optimal control techniques provide powerful tools to understand the behavior of dynamic systems. They have been applied naturally to pricing and production where the system is dynamic. Among the early research using optimal control theory, there is the work by Feichtinger and Hartl [11] who deal with the problem of simultaneously determining the optimal price policy and production rate over a given planning horizon. They use a nonlinear demand function f(π(t),t) where the control variable is π(t), i.e., the price at time t.
Lin and Shue [12] investigated the optimal policies for price and warranty length determination when defective items are replaced free of charge. The demand f(π,w,Q) is dependent on the two control variables, i.e., the price π(t) and the warranty length w(t), while Q(t) is the accumulated sales up to time t. A similar model has been studied by Lin [13] who incorporated a dynamic product quality into the model of Lin and Shue [12].
Feng et al. [14] studied the optimal control of an assembly system that produces one final product with multiple components and sells it at variable price. In their paper, the product order arrivals are modeled as a nonhomogeneous Poisson process with a rate that is dependent on the selling price at the time. There are two choices of price levels to sell the product: high p1 and low p2, with corresponding demand rates λ1 and λ2. The control variable is π(t)=p1 or p2. Feng et al. [14] assumed that backorder costs are linear in the length of time that a backorder remains on the books. Keblis and Feng [15] extended the work of Feng et al. by allowing a more general stockout cost function that includes both fixed and variable cost elements.
Cai et al. [16] studied the optimal selling price of a deteriorating product in a finite time horizon where the time horizon is either known or unknown. They assumed that the demand rate depends linearly on the selling price π(t) at time t. As such, they describe the demand d(π(t))=a−bπ(t), where a>0,b>0 and π(t) is the control variable.
Chenavaz [17] analyzed the conditions under which better product quality implies a higher or lower product price. In an optimal control framework, the firm sets the dynamic pricing and product innovation policies. The demand D=D(π,q) depends on the price π and the quality q. In particular, he considers the multiplicative separable demand function D=h(π)l(q) and the additive separable demand function D=h(π)+l(q). Similar models were analyzed by Chenavaz [18] and Vörös [19]; however, they ignored the relationship between price and quality.
Adida and Perakisy [20] studied the same model as Cai et al. [16] for multiple products with a shared production capacity rate. The demand rate for product i is di(t)=αi(t)−βi(t)πi(t) and the control variable is the price πi(t) of one unit of product i.
In a paper by Weber [21], a retailer is allowed to choose a dynamic price, a dynamic advertising rate and the inventory capacity for a sales period of fixed length. The inventory deteriorates at an exponential rate. The time- and price-dependent deterministic demand rate λR(π,t) is assumed to be a nonincreasing separable function of price and time.
Herbon and Khmelnitsky [22] have developed a dynamic pricing model of storable perishable items to determine the optimal replenishment schedule of a product. In their work, customer demand is assumed to be a pseudo-additive function of price and time since replenishment: λ(π(t),t)=λ1(π(t))+λ2(t),t≤Tmax,π(t)≤pmax(t).
Yang and Cai [23] previously focused on an emission-dependent supply chain consisting of one emission-dependent manufacturer and one emission permit supplier under the carbon-and-trade scheme. In their work, the demand not only depends on the current price, it is also sensitive to the historical price. They introduced a reference price r(t), expressed by the differential equation
dr(t)dt=δ[π(t)−r(t)], |
where δ>0 and π(t) is the price at time t, while the demand rate is D(t)=α−β[π(t)−r(t)], with α,β>0. The control variables are the price π(t) for the manufacturer and the carbon pricing policy We(t) for the supplier.
We consider two models in this paper, and both are of the tracking type and aim to coordinate the pricing and production strategies. A single product is produced by a firm. All of the models surveyed above assume that the price is a control variable. We take a different approach where the dynamic price is a state variable. We provide a rule for the dynamics of the price. The model predictive approach we use here provides the optimal production policies as well as the resulting optimal inventory and price paths.
Our models incorporate several economic and management characteristics that are crucial for obtaining an understanding of the pricing dynamics in a market. The economic and management characteristics of this model are centered around understanding and leveraging the dynamics of demand, supply, inventory; and pricing. We explain briefly the economic and management characteristics (in short E.C. and M.C. respectively) of each feature of our model. The key features are as follows: 1- Price Changes in Response to Demand and Supply (E.C.: This reflects a fundamental principle in economics in which prices are determined by the interaction of demand and supply. In a competitive market, prices tend to adjust to balance the quantity demanded with the quantity supplied; M.C.: Managers need to be aware of market dynamics and factors influencing both demand and supply); 2- Incorporation of Inventory Levels (E.C.: Inventory levels are a key economic consideration. The model recognizes that the quantity of unsold products in inventory can impact pricing decisions; M.C.: Managers must balance the costs associated with holding inventory against potential revenue gains from adjusting prices based on inventory levels); 3- Price-Demand Relationship (E.C.: The model acknowledges that price is not fixed but can change in response to shifts in demand; M.C.: Understanding the price-demand relationship is essential for managers to optimize revenue and market share); 4- Dynamic Pricing Strategies (E.C.: The model suggests a dynamic pricing approach whereby prices change over time based on market conditions; M.C.: Managers employing dynamic pricing strategies need to be adaptive and responsive to market changes); 5- Market Equilibrium Considerations (E.C.: The model is implicitly based on the concept of market equilibrium, where the quantity demanded equals the quantity supplied, leading to a stable price; M.C.: Managers must be aware of the market equilibrium point and the factors that can shift it. Pricing decisions should aim to achieve equilibrium to avoid persistent surpluses or shortages).
The next section describes the two integrated production planning-pricing models and solves them by using a model predictive control approach. Analytical solutions are obtained whenever possible, while numerical solutions, along with examples, are given whenever an explicit solution cannot be derived. In Section 3, the paper is summarized and future research directions are given.
Consider a manufacturer that can control its inventory level by focusing on production and pricing jointly. To state the considered models we use the following notation:
I(t): The inventory level at time t,
π(t): The price at time t,
S(t): The supply rate at time t,
D(t,I(t),π(t)): The demand rate at time t, inventory level I(t); and price π(t),
H: The length of the planning horizon,
T: The length of the prediction horizon,
I0: The initial inventory level,
π0: The initial price value,
ˆS(t): The goal supply rate at time t,
ˆπ(t): The goal price at time t,
ˆI(t): The goal inventory level at time t,
qi,p,ri: The positive unit costs.
The control problem is formulated in continuous time over a planning horizon [0,H]. The firm manufactures a product that can be sold during [0,H]. The selling price of each unit is set as π(t) at time t. Let I(t) denote the inventory level at time t. To model the variations of the price, we are going to consider in this section two models. In the first one, the supply rate is dynamic. A more general model is considered next, where we assume that the supply rate depends on time and on both state variables namely, the price and the inventory level. In both models, the demand rate depends on time and on both states.
The system is controlled by using S(t), i.e., the supply (production) rate at time t, while I(t) and π(t) are the state variables. It is assumed that, at time t, the demand rate D(t,I(t),π(t)) depends on both the inventory level and the price. To describe the variations of the inventory level, we use the usual state equation
˙I(t)=S(t)−D(t,I(t),π(t)), | (2.1) |
with the known initial inventory level I(0)=I0. Let us now model the variations of the price. According to the Walrasian assumption, price tends to increase (decrease) if the demand is greater than (less) than the supply. The general dynamic formalization of the Walrasian assumption is as follows:
˙π=f(D−S), |
where it is assumed that xf(x)>0 for x≠0. We shall study the properties of this model by using the linear approximation f(x)=k1x,k1>0.
With this linearization, the dynamics of price adjustments in a model of a competitive market reflects the difference between demand and supply as follows
˙π=k1(D−S). |
However, this model neglects the inventory of unsold merchandise. To study how the dynamics of price adjustments are affected if we take into account this inventory, it is natural to assume that inventory has a negative effect on the price. This consideration leads to the following integro-differential formulation
˙π(t)=k1(D(t,I(t),π(t))−S(t))−k2∫t0[S(τ)−D(τ,I(τ),π(τ))]dτ, |
with k1>0, k2>0. The second term expresses the accumulated stock as the integral of past differences. With k2>0, this term causes the price to adjust downward when the inventory is positive. Taking into account that the price increases when the demand increases, we write the dynamics of the price on the planning horizon as follows:
˙π(t)=k1[D(t,I(t),π(t))−S(t)]−k2∫t0[S(τ)−D(τ,I(τ),π(τ))]dτ+k3D(t,I(t),π(t)), | (2.2) |
with k1>0, k2>0, and k3>0 and the known initial price π(0)=π0. Finally, substituting (2.1) into (2.2) yields
˙π(t)=−k1˙I(t)−k2[I(t)−I(0)]+k3D(t,I(t),π(t)). | (2.3) |
The system under study is of the tracking type, and the firm has set a goal inventory level ˆI, a goal supply rate ˆS, and a goal price ˆπ as its targets. Penalties are incurred if a variable deviates from its target. Letting t0∈[0,H] and 0<T<H, the objective is to minimize the sum of the deviations over the prediction horizon [t0,t0+T]:
J=∫t0+Tt0{q12[I(t)−ˆI(t)]2+q22[π(t)−ˆπ(t)]2dt+p2[S(t)−ˆS]2}dt+r12[I(t0+T)−ˆI(t0+T)]2+r22[π(t0+T)−ˆπ(t0+T)]2. | (2.4) |
First, we have to point out that the targets have to satisfy the state equations, that is,
ddtˆI(t)=ˆS(t)−D(t,ˆI(t),ˆπ(t)),ddtˆπ(t)=−k1ddtˆI(t)−k2[ˆI(t)−ˆI(0)]+k3D(t,ˆI(t),ˆπ(t)). |
We introduce the shifting variables, as follows:
ΔI(t)=I(t)−ˆI(t),Δπ(t)=π(t)−ˆπ(t),ΔS(t)=S(t)−ˆS(t). |
We rewrite both (2.1) and (2.3) in terms of shifting variables as follows:
ddtΔI(t)=ΔS(t)+˜D(t,I(t),π(t)), | (2.5) |
ddtΔπ(t)=−k1ΔS(t)+ˉD(t,ˆI(t),ˆπ(t)), | (2.6) |
with
˜D(t,I(t),π(t)):=−D(t,I(t),π(t))+D(t,ˆI(t),ˆπ(t)) |
and
ˉD(t,I(t),π(t)):=−(k1+k3)˜D(t,I(t),π(t))−k2[ΔI(t)−ΔI(0)]. |
Using the shifting operator Δ, the problem is to minimize
J=∫t0+Tt0F(t)dt+R(t0+T), | (2.7) |
where
F(t)=q12ΔI(t)2+q22Δπ(t)2+p2ΔS(t)2 | (2.8) |
and
R(t0+T)=r12ΔI(t0+T)2+r22Δπ(t0+T)2. | (2.9) |
Calculation of the integral (2.7) is done by using the trapezoid formula. Divide the time interval [t0,t0+T] into m subintervals of equal length h=Tm. Then,
J≃h2[F(t0)+2m−1∑i=1F(t0+ih)+F(t0+mh)]+r12ΔI(t0+mh)2+r22Δπ(t0+mh)2. | (2.10) |
The first-order Taylor approximation, combined with (2.5) and (2.6), yields
ΔI(t+ih)≃c1(t,i)+ihΔS(t), | (2.11) |
Δπ(t+ih)≃c2(t,i)−k1ihΔS(t), | (2.12) |
with
c1(t,i)=ΔI(t)+ih˜D(t,I(t),π(t)),c2(t,i)=Δπ(t)+ihˉD(t,I(t),π(t)). |
Taking the squares of (2.11) and (2.12) and substituting the result into (2.8) yields
F(t+ih)≃12[q1c1(t,i)2+q2c2(t,i)2]+ih[q1c1(t,i)−q2c2(t,i)k1]ΔS(t)+12ˉqi2ΔS(t)2+p2ΔS(t+ih)2, |
where ˉq=(q1+k21q2)h2. This equation can be written in the following simpler form:
F(t+ih)≃A(t,i)+B(t,i)ΔS(t)+E(t,i)ΔS(t)2+p2ΔS(t+ih)2, |
where
A(t,i):=12[q1c1(t,i)2+q2c2(t,i)2],B(t,i):=ih[q1c1(t,i)−q2c2(t,i)k1],E(i):=12ˉqi2. |
Then, we can write the objective function (2.10) in terms of the control variables:
J≃A(t0)+B(t0)ΔS(t0)+EΔS(t0)2+hp4ΔS(t0+mh)2+hp2m−1∑i=1ΔS(t0+ih)2, |
where A(t0) is independent of the control variables. The explicit forms of A(t0), B(t0) and E will be needed to compute the optimal value J∗ of J, and they are given as follows:
A(t0):=hq14ΔI(t0)2+hq24Δπ(t0)2+hm−1∑i=1A(t0,i)+h2A(t0,m)+12[r1c1(t0,m)2+r2c2(t0,m)2],B(t0):=a11ΔI(t0)−a12Δπ(t0)+a13˜D(t0,I(t0),π(t0))−a14ˉD(t0,I(t0),π(t0)),E:=hp14+hˉq2(β+m22)+ˉrm22, |
where
a11=h2q1α+mh(hq12+r1),a12=h2k1q2α+mhk1(hq22+r2),a13=h3q1β+m2h2(hq12+r1),a14=h2k1q2βh+m2h2k1(hq22+r2), | (2.13) |
with α:=∑m−1i=1i=m(m−1)2, β:=∑m−1i=1i2=m(m−1)(2m−1)6, and ˉr:=(r1+k21r2)h2. Let us now introduce a matrix notation and set
ΔS(t0):=[ΔS(t0),ΔS(t0+h),⋯,ΔS(t0+mh)]T(m+1)×1;B(t0):=B(t0)e1 with e1=[1,0,⋯,0]T(m+1)×1;E:=(Eij)(m+1)×(m+1). |
Here, E is an (m+1)×(m+1) diagonal matrix whose elements are E00=E, Eii=hp2,i=1,⋯,m−1, and Emm=hp4. In order to derive the optimality condition, we rewrite the objective function in the following vectorial form:
J(ΔS(t0))≃A(t0)+B(t0)TΔS(t0)+ΔS(t0)TEΔS(t0). | (2.14) |
The unique global minimum of the objective function J is reached at ΔS∗(t0), which is the solution of the vectorial equation
∂J∂ΔS(t0)=0, |
i.e.,
ΔS∗(t0)=−12E−1B(t0). |
This implies that
ΔS∗(t0)=−B(t0)2E. | (2.15) |
Now we can readily find the explicit form of the optimal objective function value. By substituting the optimal control (2.15) in (2.14), we get:
J(ΔS∗(t0))≃A(t0)−hp4B2(t0). |
However, we still have to find the optimal price and the optimal inventory level. Since our previous analysis is valid for any t0∈[0,H], we substitute the expressions of ΔS∗(t) in (2.5) and (2.6) to obtain a system of linear differential equations:
{ddtΔI(t)=ΔS∗(t)+˜D(t,I(t),π(t)),ddtΔπ(t)=−k1ΔS∗(t)+ˉD(t,I(t),π(t)). | (2.16) |
While (2.15) provides the optimal supply rate, the solution of the system of differential equations given by (2.16) provides the optimal inventory level and the optimal price. Of course, the optimal trajectories depend on the shape of the demand rate function. To illustrate how the solution of (2.16) can be obtained, let us assume the following explicit form of the function D in terms of I and π:
D(t,I(t),π(t))=d1(t)−d2I(t)+d3π(t). |
Then,
˜D(t,I(t),π(t))=d2ΔI(t)−d3Δπ(t),ˉD(t,I(t),π(t))=−[k2+(k1+k3)d2]ΔI(t)+d3(k1+k3)Δπ(t)+k2ΔI(0)]. |
Upon substitution, the system (2.16) becomes
{ddtI(t)=l11I(t)+l12π(t)+ˉl1(t),ddtπ(t)=l21I(t)+l22π(t)+ˉl2(t), | (2.17) |
i.e.,
ddtX(t)=AX(t)+B(t), |
where
X(t):=(I(t)π(t)),B(t):=(ˉl1(t)ˉl2(t)),A:=(l11l12l21l22), |
and with x1:=hˉq2(β+m22)+ˉrm22,
l11:=−a11+a14k22(hp14+x1)+[1−a13+a14(k1+k3)2(hp14+x1)]d2,l12:=a122(hp14+x1)−[1−a13+a14(k1+k3)2(hp14+x1)]d3,l21:=−k2+k1(a11+a14k2)2(hp14+x1)+[k1[a13+a14(k1+k3)]2(hp14+x1)−(k1+k3)]d2,l22:=−k1a122(hp14+x1)+[−k1[a13+a14(k1+k3)]2(hp14+x1)+k1+k3]d3,ˉl1(t):=a14k2ΔI(0)2(hp14+x1)−l11ˆI(t)−l12ˆπ(t)+ddtˆI(t),ˉl2(t):=k2(1−a14k12(hp14+x1))ΔI(0)−l21ˆI(t)−l22ˆπ(t)+ddtˆπ(t). |
This is a nonhomogeneous system of linear equations with constant coefficients, and the explicit form of its solution X(t) can be computed as
X(t)=M(t)⋅M(0)−1X(0)+M(t)∫t0M−1(s)B(s)ds, |
where λ1 and λ2 are the eigenvalues of A and M(t) is the fundamental matrix
M(t)=(eλ1tl12eλ2tl12eλ1t(λ1−l11)eλ2t(λ2−l11)). |
In order to go further we need to compute the integral term in the general solution, which is not possible without the explicit forms of ˉl1(t) and ˉl2(t), that is, the explicit forms of the target rates ˆI(t) and ˆπ(t). For illustration purposes, let us consider the following two cases:
Case 1: ˆI(t) and ˆπ(t) are constant.
In this case, ˉl1(t) and ˉl2(t) are both constant and we put ˉl1(t)≡ˉl1 and ˉl2(t)≡ˉl2. Then, the integral term can be easily computed and we have
∫t0M−1(s)B(s)ds=(−[(λ2−l11)ˉl1−l12ˉl2]λ1l12(λ2−λ1)[e−λ1t−1][(λ1−l11)ˉl1−l12ˉl2]λ2l12(λ2−λ1)[e−λ2t−1]). |
We also need to compute M−1(0)X(0):
M−1(0)X(0)=((λ2−l11)l12(λ2−λ1)I0+1(λ2−λ1)π0(λ1−l11)l12(λ2−λ1)I0+1(λ2−λ1)π0). |
Therefore, the optimal inventory level and the optimal price are respectively given by
I∗(t)=1(λ2−λ1)(eλ1t[(λ2−l11)I0−l12π0]+eλ2t[(l11−λ1)I0+l12π0])+l12C11λ1(eλ1t−1)+l12C21λ2(eλ2t−1), | (2.18) |
π∗(t)=(λ1−l11)eλ1tl12(λ2−λ1)[(λ2−l11)I0−l12π0]+(λ2−l11)eλ2tl12(λ2−λ1)[(l11−λ1)I0+l12π0]+(λ1−l11)C11λ1(eλ1t−1)+(λ2−l11)C21λ2(eλ2t−1), | (2.19) |
where
C11=(λ2−l11)l12(λ2−λ1)(a14k2ΔI(0)2(hp14+x1)−l12ˆπ−l11ˆI)−1(λ2−λ1)(k2(1−a14k12(hp14+x1))ΔI(0)−l21ˆI−l22ˆπ),C21=1(λ2−λ1)(a14k2ΔI(0)2(hp14+x1)−l12ˆπ−l11ˆI)−(λ1−l11)l12(λ2−λ1)(k2(1−a14k12(hp14+x1))ΔI(0)−l21ˆI−l22ˆπ). |
Example 2.1. To illustrate this case, we take the target rates ˆl(t) and ˆπ(t) as constant and ˆI(t)=4 and ˆπ(t)=2.5. We take the goal supply rate ˆS(t)=3sin(t)+10, and we take d1(t)=3cos(t)+t2+4. The constants used in this example are as follows: T=5;m=100;h=0.05;q1=0.01;q2=0.1;r1=0.01;r2=0.1; p1=0.01;k1=0.9;k2=0.01;k3=1;d2=1;d3=2;I0=8;π0=2. Figure 1 depicts the variations of the optimal state variables. As can be seen, the inventory level tends to the goal inventory level, and the price tends to the goal price. Figure 2 depicts the variations of the optimal supply and demand rates. As can be seen, both tend to the goal supply rate.
Case 2: ˆI(t) and ˆπ(t) are not necessarily constant.
We consider the following explicit forms of the target rates: ˆI(t)=d5sin(t)+d6 and ˆπ(t)=d7cos(t)+d8, with di∈R,i=5,6,7,8. In this case, we have
ˉl1(t):=L11sin(t)+L12cos(t)+L13,ˉl2(t):=L21sin(t)+L22cos(t)+L23, |
with
L11:=−l11d5;L12:=(d5−l12d7);L13:=a14k2ΔI(0)2(hp14+x1)−l12d8−l11d6;L21:=−(l21d5+d7);L22:=−l22d7;L23:=k2(1−a14k12(hp14+x1))ΔI(0)−l21d6−l22d8, |
and
M−1(t)B(t)=(C11e−λ1t+C12e−λ1tsin(t)+C13e−λ1tcos(t)C21e−λ2t+C22e−λ2tsin(t)+C23e−λ2tcos(t)), |
with
C11=1l12(λ2−λ1)[(λ2−l11)L13−l12L23];C12=1l12(λ2−λ1)[(λ2−l11)L11−l12L21];C13=1l12(λ2−λ1)[(λ2−l11)L12−l12L22];C21=1l12(λ2−λ1)[l12L23−(λ1−l11)L13];C22=1l12(λ2−λ1)[l12L21−(λ1−l11)L11];C23=1l12(λ2−λ1)[l12L22−(λ1−l11)L12]. |
Then, the integral term can be easily computed and we have
I∗(t)=1(λ2−λ1)(eλ1t[(λ2−l11)I0−l12π0]+eλ2t[(l11−λ1)I0+l12π0])+C12l121+λ21[eλ1t−λ1sint−cost)]+C22l121+λ22[eλ2t−λ2sint−cost)]+C13l121+λ21[λ1eλ1t−λ1cost+sint)]+C23l121+λ22[λ2eλ2t−λ2cost+sint)]+l12C11λ1(eλ1t−1)+l12C21λ2(eλ2t−1), | (2.20) |
π∗(t)=(λ1−l11)eλ1tl12(λ2−λ1)[(λ2−l11)I0−l12π0]+(λ2−l11)eλ2tl12(λ2−λ1)[(l11−λ1)I0+l12π0]+C12(λ1−l11)1+λ21[eλ1t−λ1sint−cost)]+C22(λ2−l11)1+λ22[eλ2t−λ2sint−cost)]+C13(λ1−l11)1+λ21[λ1eλ1t−λ1cost+sint)]+C23(λ2−l11)1+λ22[λ2eλ2t−λ2cost+sint)]+(λ1−l11)C11λ1(eλ1t−1)+(λ2−l11)C21λ2(eλ2t−1). | (2.21) |
Example 2.2. To illustrate this case, we take the goal rates ˆl(t) and ˆπ(t), where both are of the form ˆI(t)=sin(t)+4 and ˆπ(t)=0.2cos(t)+2. We assume that the goal supply rate ˆS(t)=3sin(t)+10. We take d1(t)=3cos(t)+t2+4. The constants used in this example are as follows: T=5;m=100;h=0.05;q1=0.01;d2=1;d3=1;q2=0.1;r1=0.01; r2=0.1;p1=0.01;k1=0.9;k2=0.01;k3=1;I0=8;π0=2. Figure 3 depicts the variations of the optimal state variables. As can be seen, the inventory level tends to the goal inventory level, and the price tends to the goal price. Figure 4 depicts the variations of the optimal supply and demand rates. As can be seen, both tend to the goal supply rate.
Assume now that the supply rate depends on the two state variables, i.e., I(t), the inventory level at time t, and π(t), the price at time t. In order to go further in our analysis, we take the following explicit form for S:
S(t,I(t),π(t))=s1(t)−s2(t)I(t)+s3(t)π(t). |
Thus, si(t),i=1,2,3 now denotes the new control variables and ˆsi,i=1,2,3 denotes the corresponding goal controls. We notice that, for simplicity, we assume that the goal controls ˆsi,i=1,2,3 are constants. Since all targets have to satisfy the state equations, we write
ddtˆI(t)=ˆs1−ˆs2ˆI(t)+ˆs3ˆπ(t)−D(t,ˆI(t),ˆπ(t)),ddtˆπ(t)=−k1[ˆs1−ˆs2ˆI(t)+ˆs3ˆπ(t)−D(t,ˆI(t),ˆπ(t)]−k2[ˆI(t)−ˆI(0)]+k3D(t,ˆI(t),ˆπ(t)). |
Combining these two differential equations with the state differential equations, we can write the following differential system in terms of the shifting operator Δ:
ddtΔI(t)=Δs1(t)−I(t)Δs2(t)+π(t)Δs3(t)+˜D(t,I(t),π(t)), | (2.22) |
ddtΔπ(t)=−k1Δs1(t)+k1I(t)Δs2(t)−k1π(t)Δs3(t)+ˉD(t,I(t),π(t)), | (2.23) |
with
˜D(t,I(t),π(t)):=−ˆs2ΔI(t)+ˆs3Δπ(t)−[D(t,I(t),π(t))−D(t,ˆI(t),ˆπ(t))],ˉD(t,I(t),π(t)):=k1ˆs2ΔI(t)+k1ˆs3Δπ(t)−k2[ΔI(t)−ΔI(0)]+(k1+k3)[D(t,I(t),π(t))−D(t,ˆI(t),ˆπ(t))]. |
The new objective function to minimize is
J=∫t0+Tt0F(t)dt+R(t0+T), | (2.24) |
where
F(t)=q12ΔI(t)2+q22Δπ(t)2+p12Δs1(t)2+p22Δs2(t)2+p32Δs3(t)2 | (2.25) |
and
R(t0+T)=r12ΔI(t0+T)2+r22Δπ(t0+T)2. | (2.26) |
Proceeding as in the previous section, we employ the trapezoid formula to calculate the integral in (2.24); the first-order Taylor approximation, combined with (2.22) and (2.23), yields
ΔI(t+ih)≃c1(t,i)+u1(t,i),Δπ(t+ih)≃c2(t,i)+u2(t,i), |
with
c1(t,i)=ΔI(t)+ih˜D(t,I(t),π(t)),u1(t,i)=ihΔs1(t)−ihI(t)Δs2(t)+ihπ(t)Δs3(t), |
and
c2(t,i)=Δπ(t)+ihˉD(t,I(t),π(t)),u2(t,i)=−k1ih[Δs1(t)−I(t)Δs2(t)+π(t)Δs3(t)]. |
Some lengthy calculations allow to write F(t+ih) as follows:
F(t+ih)≃A(t,i)+3∑k=1[Bk(t,i)Δsk(t)+Ek(t,i)Δsk(t)2]+L1(t,i)Δs1(t)Δs2(t)+L2(t,i)Δs1(t)Δs3(t)+L3(t,i)Δs2(t)Δs3(t)+p12Δs1(t+ih)2+p22Δs2(t+ih)2+p32Δs3(t+ih)2, |
where A(t,i):=12[q1c1(t,i)2+q2c2(t,i)2],
B1(t,i):=ih[q1c1(t,i)−q2c2(t,i)k1];E1(t,i):=12ˉqi2;L1(t,i):=−ˉqi2I(t);B2(t,i):=−B1(t,i)I(t);E2(t,i):=E1(t,i)I(t)2;L2(t,i):=ˉqi2π(t);B3(t,i):=B1(t,i)π(t);E3(t,i):=E1(t,i)π(t)2;L3(t,i):=−ˉqi2I(t)π(t). |
Therefore, we can write the objective function (2.24) in terms of the control variables, as follows:
J≃A(t0)+3∑k=1Bk(t0)Δsk(t0)+3∑k=1Ek(t0)Δsk(t0)2+L1(t0)Δs1(t0)Δs2(t0)+L2(t0)Δs1(t0)Δs3(t0)+L3(t0)Δs2(t0)Δs3(t0)+hp14Δs1(t0+mh)2+hp24Δs2(t0+mh)2+hp32Δs3(t0+mh)2+m−1∑i=1hp12Δs1(t0+ih)2+m−1∑i=1hp22Δs2(t0+ih)2+m−1∑i=1hp32Δs3(t0+ih)2, |
where A(t0) is independent of the control variables; also,
B1(t0):=hm−1∑i=1B1(t0,i)+h2B1(t0,m)+mh[r1c1(t0,m)−r2c2(t0,m)k1];B2(t0):=hm−1∑i=1B2(t0,i)+h2B2(t0,m)+mh[r2c2(t0,m)k1−r1c1(t0,m)]I(t0);B3(t0):=hm−1∑i=1B3(t0,i)+h2B3(t0,m)+mh[r1c1(t0,m)−r2c2(t,m)k1]π(t0);E1(t0):=hp14+hm−1∑i=1E1(t0,i)+h2E1(t0,m)+12ˉrm2;E2(t0):=hp24+hm−1∑i=1E2(t0,i)+h2E2(t0,m)+12ˉrm2I(t0)2;E3(t0):=hp34+hm−1∑i=1E3(t0,i)+h2E3(t0,m)+12ˉrm2π(t0)2;L1(t0):=hm−1∑i=1L1(t0,i)+h2L1(t0,m)−ˉrm2I(t0);L2(t0):=hm−1∑i=1L2(t0,i)+h2L2(t0,m)+ˉrm2π(t0);L3(t0):=hm−1∑i=1L3(t0,i)+h2L3(t0,m)−ˉrm2I(t0)π(t0). |
We introduce a vector-matrix notation by setting
ΔSk(t0):=[Δsk(t0),Δsk(t0+h),⋯,Δsk(t0+mh)]T(m+1)×1,k=1,2,3;Bk(t0):=Bk(t0)e1 with e1=[1,0,⋯,0]T(m+1)×1,k=1,2,3;Ek(t0):=Diag[Ek(t0),hpk2,hpk2,hpk2,…,hpk2,hpk4];k=1,2,3;Lk(t0):=Diag[Lk(t0),0,0,0,…,0,0];k=1,2,3. |
In order to derive the optimality conditions, we rewrite the objective function in the following vectorial form:
J(ΔS1(t0),ΔS2(t0),ΔS3(t0))≃A(t0)+3∑k=1Bk(t0)TΔSk(t0)+3∑k=1ΔSk(t0)TEk(t0)ΔSk(t0)+ΔS1(t0)TL1(t0)ΔS2(t0)+ΔS1(t0)TL2(t0)ΔS3(t0)+ΔS2(t0)TL3(t0)ΔS3(t0). |
The unique global minimum of the objective function J is reached at the point (ΔS∗1(t0),ΔS∗2(t0),ΔS∗3(t0)), which is the solution of the linear system, i.e., ∂J∂ΔSk(t0)=0,k=1,2,3, which can be written in the following vectorial form:
{2E1(t0)ΔS1(t0)+L1(t0)ΔS2(t0)+L2(t0)ΔS3(t0)=−B1(t0);L1(t0)ΔS1(t0)+2E2(t0)ΔS2(t0)+L3(t0)ΔS3(t0)=−B2(t0);L2(t0)ΔS1(t0)+L3(t0)ΔS2(t0)+2E3(t0)ΔS3(t0)=−B3(t0), |
which implies that
{2E1(t0)Δs1(t0)+L1(t0)Δs2(t0)+L2(t0)Δs3(t0)=−B1(t0);L1(t0)Δs1(t0)+2E2(t0)Δs2(t0)+L3(t0)Δs3(t0)=−B2(t0);L2(t0)Δs1(t0)+L3(t0)Δs2(t0)+2E3(t0)Δs3(t0)=−B3(t0). |
Set
A(t0):=[2E1(t0)L1(t0)L2(t0)L1(t0)2E2(t0)L3(t0)L2(t0)L3(t0)2E3(t0)];A1(t0):=[−B1(t0)L1(t0)L2(t0)−B2(t0)2E2(t0)L3(t0)−B3(t0)L3(t0)2E3(t0)]; |
A2(t0):=[2E1(t0)−B1(t0)L2(t0)L1(t0)−B2(t0)L3(t0)L2(t0)−B3(t0)2E3(t0)];A3(t0):=[2E1(t0)L1(t0)−B1(t0)L1(t0)2E2(t0)−B2(t0)L2(t0)L3(t0)−B3(t0)]. |
Since
E1(t0)=hp14+x1,E2(t0)=hp24+x1I(t0)2,E3(t0)=hp34+x1π(t0)2,L1(t0)=−2x1I(t0),L2(t0)=2x1π(t0),L3(t0)=−2x1I(t0)π(t0), |
it follows that
Det(A(t0))=h3p2p3p18+h2p2p3x12+h2p1p3x12I(t0)2+h2p2p1x12π(t0)2>0, |
and, thus,
Δs∗1(t0)=det(A1(t0))det(A(t0)),Δs∗2(t0)=det(A2(t0))det(A(t0)),Δs∗3(t0)=det(A3(t0))det(A(t0)). |
Since our previous analysis is valid for any t0∈[0,H], we substitute the expressions of Δs∗k(t),k=1,2,3 in (2.22) and (2.23) to obtain a system of linear differential equations:
ddtΔI(t)=Δs∗1(t)−I(t)Δs∗2(t)+π(t)Δs∗3(t)+˜D(t,I(t),π(t)),ddtΔπ(t)=−k1Δs∗1(t)+k1I(t)Δs∗2(t)−k1π(t)Δs∗3(t)+ˉD(t,I(t),π(t)) |
with
˜D(t,I(t),π(t)):=−ˆs2ΔI(t)+ˆs3Δπ(t)−[D(t,I(t),π(t))−D(t,ˆI(t),ˆπ(t))],ˉD(t,I(t),π(t)):=k1ˆs2ΔI(t)+k1ˆs3Δπ(t)−k2[ΔI(t)−ΔI(0)]+(k1+k3)[D(t,I(t),π(t))−D(t,ˆI(t),ˆπ(t))]. |
To solve this system of equations, we need to calculate the above determinants. Using the notation (2.13), we have
B1(t)=a11ΔI(t)−a12Δπ(t)+a13˜D(t,I(t),π(t))−a14ˉD(t,I(t),π(t)),B2(t)=−B1(t)I(t),B3(t)=B1(t)π(t). |
In order to go further in our analysis and solve this differential system, we need an explicit form of the function D in terms of I and π. To do that, we assume that D has the form
D(t,I(t),π(t))=d1(t)−d2(t)I(t)+d3(t)π(t). |
Then,
˜D(t,I(t),π(t))=[ˆs2−d2(t)]ˆI(t)−[ˆs3−d3(t)]ˆπ(t)−[ˆs2−d2(t)]I(t)+[ˆs3−d3(t)]π(t),ˉD(t,I(t),π(t))=k2ΔI(0)−k1[ˆs1−d1(t)]+k3d1(t)−[k1ˆs2−k2−(k1+k3)d2(t)]ˆI(t)−[k1ˆs3+(k1+k3)d3(t)]ˆπ(t)+[k1ˆs2−k2−(k1+k3)d2(t)]I(t)+[k1ˆs3+(k1+k3)d3(t)]π(t). |
Substituting ˉD(t,I(t),π(t)) and ˜D(t,I(t),π(t)) in B1(t),B2(t), and B3(t), we obtain
B1(t)=b1(t)I(t)+b2(t)π(t)+b3(t),B2(t)=−[b1(t)I(t)+b2(t)π(t)+b3(t)]I(t),B3(t)=[b1(t)I(t)+b2(t)π(t)+b3(t))π(t), |
where
b1(t)=α1+β1d2(t),b2(t)=α2−β1d3(t),b3(t)=α3(t)−β1ˆI(t)d2(t)+β1ˆπ(t)d3(t), |
with
α1=a11−a13ˆs2−a14(k1ˆs2−k2),β1=a13+a14(k1+k3),α2=−a12+a13ˆs3−a14k1ˆs3,α3(t)=−a14k2ΔI(0)+(a12−a13ˆs3+a14k1ˆs3)ˆπ(t)+(a13ˆs2+a14(k1ˆs2+k2)−a11)ˆI(t). |
Now, we can compute the determinants:
Det(A1(t))=−[h2p3p2b3(t)4+h2p3p2b1(t)4I(t)+h2p3p2b2(t)4π(t)], |
Det(A2(t))=h2p1p3b3(t)4I(t)+h2p1p3b2(t)4I(t)π(t)+h2p1p3b1(t)4I(t)2, |
Det(A3(t))=−[h2p1p2b3(t)4π(t)+h2p1p2b1(t)4I(t)π(t)+h2p1p2b2(t)4π(t)2]. |
Consequently, we obtain the optimal solution of the vectorial minimization problem as follows:
Δs∗1(t)=−p3p2b3(t)2+p3p2b1(t)2I(t)+p3p2b2(t)2π(t)hp2p3p14+p2p3x1+p1p3x1I(t)2+p2p1x1π(t)2,Δs∗2(t)=−p1p3b3(t)2I(t)+p1p3b2(t)2I(t)π(t)+p1p3b1(t)2I(t)2hp2p3p14+p2p3x1+p1p3x1I(t)2+p2p1x1π(t)2,Δs∗3(t)=−p1p2b3(t)2π(t)+p1p2b1(t)2I(t)π(t)+p1p2b2(t)2π(t)2hp2p3p14+p2p3x1+p1p3x1I(t)2+p2p1x1π(t)2. |
By substituting these expressions in the system of differential equations given by (2.22) and (2.23), we get a system of differential equations with a nonlinear right-hand side which cannot be solved explicitly, and, in order to go further, we take some particular cases:
Case 1: s2 and s3 are constant.
Assume that the supply rate, which is our control, is given in the following form:
S(t,I(t),π(t))=s1(t)−s2I(t)+s3π(t), |
where the control is reduced to one function s1 and the two other coefficients s2 and s3 are given. In this case, the parameters p2 and p3 in the objective function will be taken to be zero and the optimal solution reduces to a single value Δs∗1, which is given by
Δs∗1(t)=−b3(t)2(hp14+x1)−b1(t)2(hp14+x1)I(t)−b2(t)2(hp14+x1)π(t). |
Our differential system will take the following simple form:
{ddtΔI(t)=Δs∗1(t)+˜D(t,I(t),π(t)),ddtΔπ(t)=−k1Δs∗1(t)+ˉD(t,I(t),π(t)), | (2.27) |
with
˜D(t,I(t),π(t))=d2(t)I(t)−d3(t)π(t)+d3(t)ˆπ(t)−d2(t)ˆI(t),ˉD(t,I(t),π(t))=k2ΔI(0)−k1[ˆs1−d1(t)]+k3d1(t)+[k2+(k1+k3)d2(t)]ˆI(t)−(k1+k3)d3(t)ˆπ(t)−[k2+(k1+k3)d2(t)]I(t)+(k1+k3)d3(t)π(t). |
After rearrangement, we can write the above system in the following form:
{ddtI(t)=l11(t)I(t)+l12(t)π(t)+ˉl1(t),ddtπ(t)=l21(t)I(t)+l22(t)π(t)+ˉl2(t), | (2.28) |
i.e.,
ddtX(t)=A(t)X(t)+B(t), |
where
X(t):=(I(t)π(t)),B(t):=(ˉl1(t)ˉl2(t)),A(t):=(l11(t)l12(t)l21(t)l22(t)), |
and
l11(t):=−α12(hp14+x1)+[1−β12(hp14+x1)]d2(t),l12(t):=−α22(hp14+x1)−[1−β12(hp14+x1)]d3(t),l21(t):=−k2+k1α12(hp14+x1)+[k1β12(hp14+x1)−(k1+k3)]d2(t),l22(t):=k1α22(hp14+x1)+[−k1β12(hp14+x1)+k1+k3]d3(t),ˉl1(t):=ddtˆI(t)−α3(t)2(hp14+x1)+[1+β12(hp14+x1)]ˆπ(t)d3(t)+[β12(hp14+x1)−1]ˆI(t)d2(t),ˉl2(t):=ddtˆπ(t)+k1α3(t)2(hp14+x1)+k2ΔI(0)−k1ˆs1+k2ˆI(t)+(k1+k3)d1(t)+[(k1+k3)−k1β12(hp14+x1)]ˆI(t)d2(t)+[k1β12(hp14+x1)−(k1+k3)]ˆπ(t)d3(t). |
This is a nonhomogeneous system of linear equations with variable coefficients, and the explicit form of its solution X(t) can be computed analytically in some cases.
Case 1.1: d2 and d3 are constant.
Assume that the demand rate D(t,I(t),π(t)) is given by the following form:
D(t,I(t),π(t))=d1(t)−d2I(t)+d3π(t). |
In this case, A(t) becomes a constant matrix:
A(t)≡A:=(l11l12l21l22); |
hence, the explicit form of the solution X(t) of the system of differential equations can be computed analytically. Proceeding as in Subsection 2.1, the general solution of the nonhomogenous differential system is given by the following formula:
X(t)=M(t)⋅M(0)−1X(0)+M(t)∫t0M−1(s)B(s)ds, |
where M(t) is the fundamental matrix. In order to go further, we need to compute the integral term in the general solution, which is not possible without the explicit form of the targets ˆI(t) and ˆπ(t). Note that, when ˆI(t) and ˆπ(t) are constant, we fall back on a system similar to the one studied in case 1 of Subsection 2.1, and the solution can be computed as in (2.18) and (2.19). Also, when ˆI(t) and ˆπ(t) are not necessarily constant, then we fall back on a system similar to the one studied in case 2 of Subsection 2.1, and the solution can be found as in (2.20) and (2.21).
Case 1.2: Not both d2 and d3 are constant.
In this case, the matrix A(t) is dependent on time and the solution of the linear differential system cannot be found analytically. However, using packages (such as ode45 solver in MATLAB), the solution can be found numerically, as the following example shows:
Example 2.3. To illustrate, we take the following functions d1,d2, and d3:
d1(t):=4+4sin(t),d2(t):=0.1+t2,d3(t):=t23−2sin(t)3−0.63. |
The constants used in this example are as follows: m=20;h=0.01;q1=1;q2=1;r1=0.1;r2=0.1;p1=0.1;k1=0.7;k2=0.1;k3=0.1;I0=2;ˆI=7;π0=10;ˆπ=6.6,ˆs1=1.47. Figure 5 depicts the variations of the optimal state variables. As can be seen, the inventory level tends to the goal inventory level, and the price tends to the goal price. Figure 6 depicts the variations of the optimal supply and demand rates. As can be seen, both tend to the goal supply rate.
Case 2: Not both s2 and s3 are constant.
In this case, the differential system becomes nonlinear even when the functions d1,d2, and d3 are constants. The analytic solution of the differential system cannot be found and we need software to solve it numerically. We take the following example as a demonstration of the numerical solvability of the differential system.
Example 2.4. Take the functions d1,d2, and d3 to be given respectively by
d1(t):=2+4sin(t),d2(t):=0.1+t2,d3(t):=0.274t2−0.548sin(t)+0.7534. |
The constants used in this example are as follows: m=200;h=0.01;q1=1;q2=1;r1=0.01;r2=0.1;p1=0.01;p2=0.001;p3=0.01,k1=0.9;k2=0.01;k3=0.1;I0=8;ˆI=2;π0=2;ˆπ=7.3;ˆs1=1.47;ˆs2=0.1;ˆs3=0.1. Figure 7 shows the variations of the optimal inventory level and the optimal price rate. Figure 8 shows the variations of the optimal supply and demand rates. All variables converge to their respective goals.
Since firms may not be able to control the price, we have proposed, in this paper, a model for the variations of a dynamic price. The model is based on the Walrasian assumption that the price changes in a manner that is reflected in the difference between the demand and the supply. The model also takes into account the inventory of unsold product and the fact that the price changes with the demand. Using the model predictive control technique, we have obtained the optimal supply rate (control variable) and the optimal price and inventory level (state variables). These solutions are obtained analytically when possible and numerically otherwise. Numerical examples illustrate the results obtained. Although we did not conduct any sensitivity analysis, we note that this could have been easily done either on the explicit results of the paper, or numerically.
As future work, we suggest to consider the case in which the product is subject to deterioration. A dynamic pricing policy is particularly well-suited in this case, and the price can be adjusted as the product deteriorates.
The authors declare they have not used Artificial Intelligence (AI) tools in the creation of this article.
The first author extends his appreciations to Researchers Supporting Project, number (RSPD2023R1001), King Saud University, Riyadh Saudi Arabia. The authors would like to thank the referees for their careful and thorough reading of the paper.
The authors declare no conflicts of interest.
[1] |
T. M. Whitin, Inventory control and price theory, Manag. Sci., 2 (1955), 61–80. https://doi.org/10.1287/mnsc.2.1.61 doi: 10.1287/mnsc.2.1.61
![]() |
[2] | J. Eliashberg, R. Steinberg, Marketing-production joint decision-making, In: Handbooks in operations research and management science, 5 (1993), 828–880. https://doi.org/10.1016/S0927-0507(05)80041-6 |
[3] |
W. Elmaghraby, P. Keskinocak, Dynamic pricing in the presence of inventory considerations: research overview, current practices, and future directions, Manag. Sci., 49 (2003), 1287–1309. https://doi.org/10.1287/mnsc.49.10.1287.17315 doi: 10.1287/mnsc.49.10.1287.17315
![]() |
[4] | L. M. A. Chan, Z. J. M. Shen, D. Simchi-Levi, J. L. Swann, Coordination of pricing and inventory decisions: a survey and classification, In: D. Simchi-Levi, S. D. Wu, Z. J. M. Shen, Handbook of quantitative supply chain analysis, International Series in Operations Research & Management Science, Boston: Springer, 74 (2004), 335–392. https://doi.org/10.1007/978-1-4020-7953-5_9 |
[5] | D. Simchi-Levi, X. Chen, J. Bramel, The logic of logistics: theory, algorithms, and applications for logistics and supply chain management, 2 Eds, New York: Springer, 2005 https://doi.org/10.1007/b97669 |
[6] | C. A. Yano, S. M. Gilbert, Coordinated pricing and production/procurement decision: a review, In: A. K. Chakravarty, J. Eliashberg, Managing business interfaces, International Series in Quantitative Marketing, Boston: Springer, 16 (2005), 65–103. https://doi.org/10.1007/0-387-25002-6_3 |
[7] | R. H. Niu, I. Castillo, T. Joro, Joint pricing and inventory/production decisions in a supply chain: a state-of-the-art survey, Proceedings of the Western Decision Sciences Institute, WDSI 2001 Fortieth Annual Meeting, Portland Oregon, 2011. |
[8] | X. Chen, D. Simchi-Levi, Pricing and inventory management, In: R. Philips, O. Özalp, The Oxford handbook of pricing management, Oxford University Press, 2012,784–824. https://doi.org/10.1093/oxfordhb/9780199543175.013.0030 |
[9] | R. Zhang, An introduction to joint pricing and inventory management under stochastic demand, 2013. |
[10] |
A. V. Den Boer, Dynamic pricing and learning: Historical origins, current research, and new directions, Surv. Oper. Res. Manage. Sci., 20 (2015), 1–18. https://doi.org/10.1016/j.sorms.2015.03.001 doi: 10.1016/j.sorms.2015.03.001
![]() |
[11] |
G. Feichtinger, R. Hartl, Optimal pricing and production in an inventory model, Eur. J. Oper. Res., 19 (1985), 45–56. https://doi.org/10.1016/0377-2217(85)90307-8 doi: 10.1016/0377-2217(85)90307-8
![]() |
[12] |
P. C. Lin, L. Y. Shue, Application of optimal control theory to product pricing and warranty with free replacement under the influence of basic lifetime distributions, Comput. Ind. Eng., 48 (2005), 69–82. https://doi.org/10.1016/j.cie.2004.07.009 doi: 10.1016/j.cie.2004.07.009
![]() |
[13] |
P. C. Lin, Optimal pricing, production rate, and quality under learning effects, J. Bus. Res., 61 (2008), 1152–1159. https://doi.org/10.1016/j.jbusres.2007.11.008 doi: 10.1016/j.jbusres.2007.11.008
![]() |
[14] |
Y. Feng, J. Ou, Z. Pang, Optimal control of price and production in an assemble-to-order system, Oper. Res. Lett., 36 (2008), 506–512. https://doi.org/10.1016/j.orl.2008.01.012 doi: 10.1016/j.orl.2008.01.012
![]() |
[15] |
M. F. Keblis, Y. Feng, Optimal pricing and production control in an assembly system with a general stockout cost, IEEE Trans. Automat. Contr., 57 (2012), 1821–1826. https://doi.org/10.1109/TAC.2011.2178335 doi: 10.1109/TAC.2011.2178335
![]() |
[16] |
X. Cai, Y. Feng, Y. Li, D. Shi, Optimal pricing policy for a deteriorating product by dynamic tracking control, Int. J. Prod. Res., 51 (2013), 2491–2504. https://doi.org/10.1080/00207543.2012.743688 doi: 10.1080/00207543.2012.743688
![]() |
[17] |
R. Chenavaz, Better product quality may lead to lower product price, B.E. J. Theor. Econ., 17 (2016), 1–22. https://doi.org/10.1515/bejte-2015-0062 doi: 10.1515/bejte-2015-0062
![]() |
[18] | R. Chenavaz, Dynamic pricing rule and R & D, Econ. Bull., 31 (2011), 2229–2236. |
[19] |
J. Vörös, Multi-period models for analyzing the dynamics of process improvement activities, Eur. J. Oper. Res., 230 (2013), 615–623. https://doi.org/10.1016/j.ejor.2013.04.036 doi: 10.1016/j.ejor.2013.04.036
![]() |
[20] |
E. Adida, G. Perakisy, A nonlinear continuous time optimal control model of dynamic pricing and inventory control with no backorders, Nav. Res. Log., 54 (2007), 767–795. https://doi.org/10.1002/nav.20250 doi: 10.1002/nav.20250
![]() |
[21] | M. Weber, Optimal inventory control in the presence of dynamic pricing and dynamic advertising, Ph.D. Dissertation, Humboldt-Universität of Berlin, Germany, 2015. https://doi.org/10.18452/17339 |
[22] |
A. Herbon, E. Khmelnitsky, Optimal dynamic pricing and ordering of a perishable product under additive effects of price and time on demand, Eur. J. Oper. Res., 260 (2017), 546–556. https://doi.org/10.1016/j.ejor.2016.12.033 doi: 10.1016/j.ejor.2016.12.033
![]() |
[23] | L. Yang, Y. Cai, Optimal dynamic pricing for fresh products under the cap-and-trade scheme, 2017 International Conference on Service Systems and Service Management, Dalian, IEEE, 2017. https://doi.org/10.1109/ICSSSM.2017.7996183 |