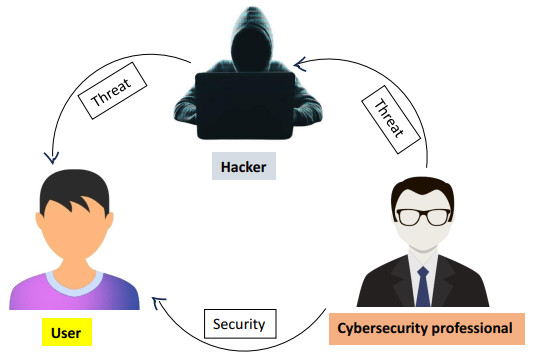
The widespread use of computer hardware and software in society has led to the emergence of a type of criminal conduct known as cybercrime, which has become a major worldwide concern in the 21st century spanning multiple domains. As a result, in the present setting, academics and practitioners are showing a great deal of interest in conducting research on cybercrime. In this work, a fractional-order model was replaced by involving three sorts of human populations: online computer users, hackers, and cyber security professionals, in order to examine the online computer user-hacker system. The existence, uniqueness and boundedness were studied. To support our theoretical conclusions, a numerical analysis of the influence of the various logical parameters was conducted and we derived the necessary conditions for the different equilibrium points to be locally stable. We examined the effects of the fear level and refuge factor on the equilibrium densities of prey and predators in order to explore and understand the dynamics of the system in a better way. Using some special circumstances, the model was examined. Our theoretical findings and logical parameters were validated through a numerical analysis utilizing the generalized Adams-Bashforth-Moulton technique.
Citation: José F. Gómez-Aguilar, Manisha Krishna Naik, Reny George, Chandrali Baishya, İbrahim Avcı, Eduardo Pérez-Careta. Chaos and stability of a fractional model of the cyber ecosystem[J]. AIMS Mathematics, 2024, 9(8): 22146-22173. doi: 10.3934/math.20241077
[1] | Xiaoming Su, Jiahui Wang, Adiya Bao . Stability analysis and chaos control in a discrete predator-prey system with Allee effect, fear effect, and refuge. AIMS Mathematics, 2024, 9(5): 13462-13491. doi: 10.3934/math.2024656 |
[2] | Kottakkaran Sooppy Nisar, G Ranjith Kumar, K Ramesh . The study on the complex nature of a predator-prey model with fractional-order derivatives incorporating refuge and nonlinear prey harvesting. AIMS Mathematics, 2024, 9(5): 13492-13507. doi: 10.3934/math.2024657 |
[3] | Nehad Ali Shah, Iftikhar Ahmed, Kanayo K. Asogwa, Azhar Ali Zafar, Wajaree Weera, Ali Akgül . Numerical study of a nonlinear fractional chaotic Chua's circuit. AIMS Mathematics, 2023, 8(1): 1636-1655. doi: 10.3934/math.2023083 |
[4] | A. Q. Khan, Ibraheem M. Alsulami . Complicate dynamical analysis of a discrete predator-prey model with a prey refuge. AIMS Mathematics, 2023, 8(7): 15035-15057. doi: 10.3934/math.2023768 |
[5] | Xiao-Long Gao, Hao-Lu Zhang, Xiao-Yu Li . Research on pattern dynamics of a class of predator-prey model with interval biological coefficients for capture. AIMS Mathematics, 2024, 9(7): 18506-18527. doi: 10.3934/math.2024901 |
[6] | Weili Kong, Yuanfu Shao . The effects of fear and delay on a predator-prey model with Crowley-Martin functional response and stage structure for predator. AIMS Mathematics, 2023, 8(12): 29260-29289. doi: 10.3934/math.20231498 |
[7] | Asharani J. Rangappa, Chandrali Baishya, Reny George, Sina Etemad, Zaher Mundher Yaseen . On the existence, stability and chaos analysis of a novel 4D atmospheric dynamical system in the context of the Caputo fractional derivatives. AIMS Mathematics, 2024, 9(10): 28560-28588. doi: 10.3934/math.20241386 |
[8] | Yao Shi, Zhenyu Wang . Bifurcation analysis and chaos control of a discrete fractional-order Leslie-Gower model with fear factor. AIMS Mathematics, 2024, 9(11): 30298-30319. doi: 10.3934/math.20241462 |
[9] | Guilin Tang, Ning Li . Chaotic behavior and controlling chaos in a fast-slow plankton-fish model. AIMS Mathematics, 2024, 9(6): 14376-14404. doi: 10.3934/math.2024699 |
[10] | Xuyang Cao, Qinglong Wang, Jie Liu . Hopf bifurcation in a predator-prey model under fuzzy parameters involving prey refuge and fear effects. AIMS Mathematics, 2024, 9(9): 23945-23970. doi: 10.3934/math.20241164 |
The widespread use of computer hardware and software in society has led to the emergence of a type of criminal conduct known as cybercrime, which has become a major worldwide concern in the 21st century spanning multiple domains. As a result, in the present setting, academics and practitioners are showing a great deal of interest in conducting research on cybercrime. In this work, a fractional-order model was replaced by involving three sorts of human populations: online computer users, hackers, and cyber security professionals, in order to examine the online computer user-hacker system. The existence, uniqueness and boundedness were studied. To support our theoretical conclusions, a numerical analysis of the influence of the various logical parameters was conducted and we derived the necessary conditions for the different equilibrium points to be locally stable. We examined the effects of the fear level and refuge factor on the equilibrium densities of prey and predators in order to explore and understand the dynamics of the system in a better way. Using some special circumstances, the model was examined. Our theoretical findings and logical parameters were validated through a numerical analysis utilizing the generalized Adams-Bashforth-Moulton technique.
Crime has become a ubiquitous sociological plague in our society, showing up both explicitly and implicitly in recent decades. Although traditional criminal behavior has long been a worry, a new era of cybercrime has emerged due to the internet's rapid spread, posing unique challenges to individuals, organizations, and governments globally [1]. Online criminal activity has surged in parallel with the internet's rapid ascent to prominence as a tool for communication and business. With more than 3 billion users worldwide, cyberspace has emerged as the main platform for governmental, cultural, and economic exchanges. It also contains sensitive data and essential infrastructures for modern living. But because of its interconnection, cyberspace is now more vulnerable to being exploited by cybercriminals, who may operate internationally with a certain degree of anonymity and impunity. Strong cybersecurity measures are becoming more and more necessary as cybercrimes increase in number. Global spending on cybersecurity is projected to exceed 1 trillion dollars between 2017 and 2021. Even with the advances in detection technologies, it is still difficult to identify offenders and determine their motivations, especially in the case of hackers who operate with sophisticated strategies beyond traditional jurisdictions [2]. To address these challenges, a deeper comprehension of hacker motivations and tactics is essential. Historically perceived as a monolithic entity, hackers comprise a diverse spectrum of actors with varying intentions and techniques. Over time, different typologies of hackers and their motivations provide cybersecurity experts insights into the constellation of cybercrimes. However, the dynamic nature of cyber threats necessitates continual refinement and updating of these typologies to keep pace with evolving tactics and behaviors. Recognizing the urgency, this article scrutinizes the impacts of hackers and the tactics that they use to accomplish their goals. Through the clarification of the complex interactions among users, hackers, and cybersecurity professionals in a wider cyber ecosystem, this research seeks to improve the ability to identify, address, and avert cybercrimes. By considering the human aspects of users, Grobler et al. [3] illustrated the paradigm change from functional and usage-centered cyber security to user-centered cyber security. In addition to demonstrating how different cognitive capacities of computer users affect their capacity to fend off information security threats, Moustafa et al. [4] offered potential psychological strategies to support computer users in adhering to security guidelines, thereby enhancing network and information security. People are increasingly expressing concern regarding the consequences of hacking, prompting them to take proactive measures to protect their documents and the system they use based on prior experience [5]. Users may adopt several measures to protect their system and data from cyber threats, such as antivirus software, firewall protection, strong passwords, updated software, or avoiding suspicious links. This shift underscores a heightened awareness of personal responsibility in mitigating vulnerability to hackers.
In this study, we have looked at how users, hackers, and cybersecurity experts interact as three major parts of the cyber ecosystem. We have explored the complex interrelationships among these objects and clarified their behavior. Additionally, we have included a thorough analysis with graphical representations to show how refuge elements and fear levels affect the survival and growth trajectories of hackers as well as users. Traditionally, predators were believed to only impact prey populations via direct killing of prey, decreasing their chances of surviving. However, new research reveals a more profound ecological function, as predators change the behavioral and mental state of their victims. Prey feel there is a chance of being eaten when they come across predators, which causes them to react with fear or tension. Beyond just simple mortality impacts, this perceived danger influences prey behaviors and ecological dynamics. Zanette et al. [6] conducted a large-scale field experiment in which direct killing was purposefully avoided in order to measure the effect of predator fear on the population of prey. The strongest argument in favor of the idea that fear will have an impact on prey populations everywhere is the fact that afraid prey consumes less because they are unable to keep their heads up to avoid predators and down to forage [6,7,8]. This fear of the prey may exhibit some anti-predator behaviors which causes them to avoid predators. Refuge is one of them: a strategy where prey conceals themselves in inaccessible areas, protecting oneself from predation. This tactic ensures that a portion of the prey population remains hidden from predators, thereby lowering the risk of predation. Nevertheless, extended refuge leads to diminished opportunities for feeding and mating, highlighting a trade-off between predator avoidance and other essential activities. In addition to taking cover, the dread of approaching predators causes prey to engage in a variety of anti-predator behaviors, such as releasing toxins, forming groups, playing dead, mimicking, herd behavior, camouflage, and apostatic selection [9,10,11].
When we employ differential equations, the utilization of fractional operators has been recognized as a more rational and organized tool. Numerous ecologists have started incorporating different types of fractional- order derivatives (FOD) into integer-order ecological models. Various theories of fractional operators, including the Caputo-Liouville, Gronwald-Letnikov, Caputo-Fabrizio, and Atangana-Baleanu, can be explored in references such as [12,13,14,15]. Noteworthy outcomes from analyzing various population models in relation to the fractional operators are documented in some works like [16,17,18]. Gao et al. [19] focused on enhancing the complexity of chaotic systems by introducing the memristor and a nonlinear component and also proposed an encryption method for face images: EFR-CSTP [20]. The EFR-CSTP is very efficient because it only encrypts the facial component in the face image. Inspired by the research of [21,22,23,24,25,26,27], this article introduces a 3D chaotic cybernetic model that delineates the relationships among user, hacker, and cybersecurity professionals within the Caputo fractional domain. The model is designed using fractional-order derivatives which, unlike integer-order derivatives, can capture memory and hereditary properties of various processes. This makes them particularly suitable for modeling systems with such characteristics. The current study highlights the significance of employing a mathematical model to address real-world issues and evaluates the performance of the fractional operator in this context. Additionally, the proposed solution procedure for solving the system of fractional differential equations is both systematic and effective.
A key novelty in this work is the consideration of Lyapunov exponents in the fractional context, addressing an open problem and discerning the chaotic nature of the projected chaos system. The primary innovation in this paper lies in connecting the 3D chaotic model to three significant components, users, hackers, and cybersecurity professionals, in the context of a Caputo-type fractional differential equation (FDE) in a cyber ecosystem. We analyze their dynamics and induce the antipredator factors, exploring their impact. Furthermore, we investigate the stability of the chaotic FO theoretically as well as graphically to enhance its validity; this is the key novel exploration from the existing literature. In order to solve this, we numerically simulate the projected model using the Adams-Bashforth-Moulton predictor-corrector method, which Diethelm generalized for FDEs [28].
The subsequent sections of the article are as follows. In Section 2, we provide definitions related to FO systems. A comprehensive explanation of the FO 3D chaotic system is presented in Section 3. The analysis of the existence and uniqueness of the solution is conducted in Section 4. Section 5 describes the boundedness of the system. The detection of the equilibrium point, existence, and its stability analysis are discussed in Section 6. Section 7 determines the chaotic nature with the Lyapunov exponent. Numerical illustrations of the proposed work are provided in Section 8. Finally, the comprehensive conclusion of the present work is drawn in Section 9.
In this study, the Caputo fractional derivative has been utilized to accommodate the integer-order initial condition. In support, we have reviewed some definitions and lemma here.
Definition 2.1. [29] If 0<α<1, the Caputo derivative of the continuous function z(t) is defined by:
Dαtz(t)=1Γ(1−α)∫t0(t−τ)−αz′(τ)dτ, |
where Γ(⋅) is the standard Gamma function.
Definition 2.2. [29] The Riemann-Liouville fractional integral Iαtz(t) of order α>0 is defined as
Iαtz(t)=1Γ(α)∫t0z(τ)(t−τ)1−αdτ. |
Lemma 2.3. [30] Consider the nonlinear equation
Dαt0x(t)=p(t,x),t>t0, |
so that 0<α≤1 and p:[t0,∞)×Rn→Rn. The initial condition is chosen as x(t0). p(t,x) admits the local Lipschitz conditions w.r.t. x on [t0,∞)×Rn guaranteeing the existence of the unique solution for the given IVP. (The corresponding Lipschitz constant should be the less than one.)
Lemma 2.4. [31] Let g(t) be a continuous function on [t0,+∞) satisfying:
Dαt0g(t)≤−λg(t)+ξ,g(t0)=ft0. |
Note that 0<α≤1, (λ,ξ)∈R2 and λ≠0. Also, t0≥0 is the initial time. Then
g(t)≤(g(t0)−ξλ)Eα[−λ(t−t0)α]+ξλ. |
In this study, we employed the 3D chaotic attractor [18] to propose a cybernetic model illustrating the interplay among the user (U), hacker (V), and cybersecurity Professional (W). A user is a person or an entity that uses digital devices, networks, or computer systems to perform tasks or retrieve data. A hacker is a skilled person who uses technical expertise to gain unauthorized access to computer systems or networks for a variety of reasons, such as disruption, exploitation, or data theft. A cybersecurity professional is an expert who focuses on implementing security measures, performing risk assessments, and responding to security incidents in order to safeguard computer systems, networks, and data from cyber threats. To encapsulate this interaction, we have characterized the subsequent set of nonlinear differential equations:
DαtU=U(1−U)b1+dV−βUVU+σ,DαtV=−δV+θρUVU+χ−λVWV+ϕW+ξ,DαtW=−γW+μVWV+ϕW+ξ. | (3.1) |
where σ=σ1K,χ=χ1K,ϕ=bϕ1,ξ=ξ1bK, (see Figure 1 and Table 1).
Parameters | Description |
b | Growth rate of users in online platform |
β | Reduction rate of users due to hackers |
σ1 | Attack threshold constant of user at which loss rate is half of β |
χ1 | Attack threshold constant of the user at which gain to hackers is half of ρ |
K | Carrying capacity of the users |
ϕ1 | Escape rate from cybersecurity professionals |
δ | Caught rate of hackers |
λ | Security in support of user |
ρ | Maximum profit gain for hackers due to hacking of user |
θ | Growth rate of hackers |
γ | Retirement of cybersecurity professionals |
ξ1 | Normalizing coefficient to the environment for interaction of hacker and cybersecurity professionals |
μ | Gain for cybersecurity professionals when hackers got caught |
d | Fear factor |
In this section, we provide some theorems in relation to the existence and uniqueness of the solution for the fractional given model described by Eq (3.1).
Theorem 4.1. In the region K×[0,T] with
K={(U,V,W)∈R3:||U||≤M1,||V||≤M2,||W||≤M3,}, |
and T<+∞, there is a solution of the given fractional system of Eq (3.1) and it is unique.
Proof. Here, let B(t) = (U(t),V(t),W(t)), ˉB(t)=(ˉU(t), ˉV(t),ˉW(t)) and a function
A(t,B)=(A1(t,B),A2(t,B),A3(t,B)), |
so that
A1(t,B)=U(1−U)b1+dV−βUVU+σ,A2(t,B)=−δV+θρUVU+χ−λVWV+ϕw+ξ,A3(t,B)=−γW+μVWV+ϕW+ξ. |
A(t,Y) is formulated on K×[0,T], m=supK||A(t,Y)||, and ||B(t)||=supt∈[0,T]|B(t)|. We aim here to show the existence of some Φ such that
||A(B)−A(ˉB)||≤Φ|||B−ˉB||. |
Consider,
||A(B)−A(ˉB)||=||U(1−U)b1+dV−βUVU+σ−ˉU(1−ˉU)b1+dˉV+βˉUˉVˉU+σ−δV+θρUVU+χ−λVWV+ϕW+ξ+δˉV−θρˉUˉVˉU+χ+λˉVˉWˉV+ϕˉW+ξ−γW+μVWV+ϕW+ξ+γˉW−μˉVˉWˉV+ϕˉW+ξ||≤(2(b+bdM)(1+M)+M+θρ)||U−ˉU||+(bdM2+bdM+M2+σM+δ+θρχM+M2θρ+ϕM2+ξM+ϕμM2+ξμM)||V−ˉV||+(M2+ξM+γ+μM2+μξM)||W−ˉW||. |
This implies,
||A(B)−A(ˉB)||≤Φ1||U−ˉU||+Φ2||V−ˉV||+Φ3||W−ˉW||, | (4.1) |
where,
Φ1=2(b+bdM)(1+M)+M+θρ,Φ2=bdM2+bdM+M2+σM+δ+θρχM+M2θρ+ϕM2+ξM+ϕμM2+ξμM,Φ3=M2+ξM+γ+μM2+μξM. | (4.2) |
Consider Φ=max{Φ1,Φ2,Φ3}. This yields
||A(B)−A(ˉB)||≤Φ||B−ˉB||. |
On contructing a Picard's operator Δ, using the function K and applying the fractional R-L integral,
ΔB=B(0)+IαA(t,B). | (4.3) |
We should investigate two things about this operator: It maps a complete non-empty metric space into itself and also it is a contraction. Let us take
||B−B(0)||≤m. |
By the norm on (4.3), we get
||ΔB−B(0)||≤||A(t,B)||Iα(1)≤mTαΓ(α+1)<b. | (4.4) |
The above inequality in (4.4) is to be held if TαΓ(α+1)<bm. Next, we establish a condition under which the operator Δ shows the contractive property. To establish this condition, we follow the following steps:
||ΔB−ΔˉB||=||Iα(A(t,B)−A(t,ˉB))||≤Iα||A(t,B)−A(t,ˉB)||≤||A(t,B)−A(t,ˉB)||Iα(1)≤TαΓ(α+1)Φ||B−ˉB||. | (4.5) |
The equation above illustrates that the Picard's operator Δ transforms into a contraction whenever
TαΓ(α+1)≤1Φ. |
This confirms that the Picard's operator Δ functions as a contraction. According to the Banach principle, we can infer that the operator Δ possesses a unique fixed point, thereby indicating that the initial value system of FDEs (3.1) admits a unique solution so that TαΓ(α+1)<min{bm,1Φ}.
Now, we try to find out that the solutions of the system (3.1) are bounded.
Theorem 5.1. The solutions of the initial value system of FDEs (3.1) are bounded uniformly.
Proof. Let us consider the function L(t)=U(t)+V(t)+W(t).
We have
Dαt0L(t)+γL(t)=Dαt0[U(t)+V(t)+W(t)]+γ[U(t)+V(t)+W(t)]=U(1−U)b1+dV−βUVU+σ−δV+θρUVU+χ−λVWV+ϕW+ξ−γW+μVWV+ϕW+ξ+γ(U+V+W)≤Ub1+dV+θρUVU+χ+μVWV+ϕW+ξ+γ(U+V),≤Ub1+dK+θρK2K+χ+μK2K+ϕK+ξ+γ(2K), |
on the ball
B={(U,V,W):max{|U|,|V|,|W|}≤K}, |
which guarantees the existence of the unique solution. So, the above inequality gives that
Dαt0L(t)+γL(t)≤Ub1+dK+θρK2K+χ+μK2K+ϕK+ξ+γ(2K). |
By Lemma 2.4, we get
Dαt0L(t)≤(L(t0)−1γ(Ub1+dK+θρK2K+χ+μK2K+ϕK+ξ+γ(2K))Eα[−γ(t−t0)α]+1γ(Ub1+dK+θρK2K+χ+μK2K+ϕK+ξ+γ(2K))→Ub1+dK+θρK2K+χ+μK2K+ϕK+ξ+γ(2K),t→∞. |
Therefore, all solutions of the initial value system of FDEs (3.1) that initiate in B are bounded on
Θ={(U,V,W)∈B+|L(t)≤Ub1+dK+θρK2K+χ+μK2K+ϕK+ξ+γ(2K)+ϵ,ϵ>0}. |
The proof is complete.
Theorem 6.1. The coexistence equilibrium point E1=(ˆU,ˆV,ˆW) exists.
Proof. The coexistence equilibrium point is obtained by solving the following equations:
ˆU(1−ˆU)b1+dˆV−βˆUˆVˆU+σ=0, | (6.1) |
−δˆV+θρˆUˆVˆU+χ−λˆVˆWˆV+ϕˆW+ξ=0, | (6.2) |
−γˆW+μˆVˆWˆV+ϕˆW+ξ=0. | (6.3) |
Solving Eq (6.1), we get
ˆU=1−σ2+√(1+σ)2b−4ˆV(1+dˆV)2√ˆV |
and ˆU exists if (1+σ)2>4ˆV(1+dˆV).
Here, σ is the attack threshold constant of the user, d is the fear induced by hackers.
If the fear factor instilled by hackers to users within the cyber ecosystem surpasses the threshold attack rate, then users persist due to the efficacy of cybersecurity tactics. Through effective cybersecurity tactics, users surpass this fear, ensuring their continued presence in the virtual sphere.
Solving Eq (6.3) yields
ˆW=ˆV(μ−γ)−ξγϕ+γ |
and ˆW exists if
ˆV(μ−γ)>ξγ. | (6.4) |
The rate of profit for cybersecurity professionals should be more than the rate of retirement for ˆW to exist.
Now, substituting the value of ˆU and ˆW in (6.2), we get,
A1ˆV4+A2ˆV3+A3ˆV2+A4ˆV+A5=0, | (6.5) |
where
A1=dμ(1+ϕ(δ−θρ))(−2γ+μ+ϕμ(δ−θρ),A2=−μ(θρ−δ)ϕ−1)(−2(1+dξ)γ+μ+ϕμ(δ−θρ),A3=−μ(2ξγ(1+ϕ(δ−ρθ))+bχ(−μ(1+ϕδ)(1+χ(1+ϕδ)+ϕ(δ−θρ))+γ(2+2ϕδ+2χ(1+ϕδ)−ϕθρσ(μ(1+ϕ(δ−θρ)))(1+χ(1+ϕδ)+ϕ(δ−θρ))+γ(−2−2ϕδ+2ϕθρ+χ(−2−2ϕδ+ϕθρ)))),A4=bξγμ(−χ(2+2ϕδ+2χ(1+ϕδ−ϕθρ)))),A5=γ2(ξ+V2)(b+χ(1+χ)+ˆV(1+dˆV)−σb(1+χ). |
Clearly A5>0. Hence, Equation (6.5) has a change of sign between the fourth and fifth terms if A4<0. Therefore, it has exactly one positive root according to Descarte's rule of sign. Then, ˆV equilibrium point exists.
Hence, coexistence equilibrium point ~E1 exists.
Stability analysis of equilibrium point
Here, we analyze the user, cybersecurity professional free and coexistence equilibrium of the system (3.1). If all eigenvalues Θj(j=1,2,…,β) related to the Jacobian matrix J(ˉE) (note that ˉE is the equilibrium point) satisfy
|arg(Eigenvalue(J(ˉE)))|=|arg(Θj)|>απ2, | (6.6) |
then, ˉE will be a stable equilibrium point.
We determine these eigenvalues by solving the characteristic equation
|J(ˉE)−ΘjI|=0. |
Lemma 6.2. [32] Consider the following characteristic equation
P(Θ)=Θβ+A1Θβ−1+A2Θβ−2+...Aβ=0. | (6.7) |
The conditions stated below make all the roots of the characteristic equation (6.7) to satisfy the inequality (6.6):
(I) If β=1, then the necessary condition for (6.7) is A1>0.
(II) If β=2, then the necessary conditions for (6.7) are either the Routh-Hurwitz conditions to be held or A1>0,4A2>A21, and |tan−1√4A2−A21A1|>απ2.
(III) Let β=3. If the discriminant of the polynomial P(Θ) is positive, in this case, the necessary and sufficient conditions for satisfying (6.7) are
A1>0,A2>0,A1A2>A3. |
If this discriminant is negative, then the necessary and sufficient conditions for satisfying (6.7) are
A1>0,A2>0,A1A2=A3. |
(IV) For every values of β, Aβ>0 is the necessary condition for (6.7) to be fulfilled.
Theorem 6.3. The user equilibrium point of the projected FO model (3.1) E2(U,0,0)=(1,0,0) exixts and is unstable.
Proof. First of all, the Jacobian matrix at E2 is
J(E2)=(−b−11+σ00−δ+θρ1+χ000−γ). |
Simplifying and solving for equilibrium point, we get these eigenvalues
Λ1=−b, |
Λ2=−γ, |
Λ3=−δ+χδ−θρ1+χ, |
which complete the proof.
Here, θρ represents the overall impact of hackers' growth on their profitability, indicating the rate at which hackers are expanding their operations and the resulting financial gains from their activities. 1+χ means the tipping point where hackers can sustain their activities effectively and achieve a level of profitability that outweighs the costs and risks associated with cybercrime. When θρ exceeds (1+χ), then hackers are operating at a level where their growth and profitability outweigh the defensive capabilities of users, leading to a positive impact for hackers. As a result, cyberattacks may be more successful, hackers may make more money, and potentially expand their operations. Hence, for the above reasons, we have considered Λ3>0 if θρ>(1+χ), which leads to E3 unstable.
This can be further verified by the Routh-Hurwitz stability criterion. The characteristic polynomial of J(E3) is
B3+B2K1+BK2+K3=0, | (6.8) |
where K1=b+γ+δ−θρ1+χ and
K2=bγ+δ(b+γ)−θρ1+χ(b+γ), |
K3=bγδ−bηθρ1+χ. |
The necessary and sufficient condition for the stability of the linear time-invariant dynamical system is the Routh-Hurwitz stability criterion. According to this, in third-order polynomial, Equation (6.8) must satisfy the following conditions:
● the coefficients K1,K2, and K3 must be positive.
● K1K2>K3
Remark: The values K1=2.64, K2=−1.30, and K3=0.73, for the given values of parameters in this paper, fail to satisfy the first condition. Hence, by Lemma 6.2, the hacker and cybersecurity professional free equilibrium point is unstable.
Theorem 6.4. The cybersecurity professional free equilibrium E3(U,V,0) of the projected FO model (3.1) is unstable.
Proof. The Jacobian matrix at E3 is
J(E3)=(UV(σ+U)2−Vσ+V+b−2bU1+dV−Uσ+U+bd(U−1)U(1+dV)20χVθρ(χ+U)2−δ+Uθρχ+U−Vξ+V00−h+μVξ+V). |
The characteristic polynomial is
Θ(ξ+V)+ξγ+γV−μV(σ+U)2(χ+U)2(ξ+V)2(1+dV)2(B1Θ2+B2Θ+B3)=0,(ξ+V)(Θ−Θ1)(σ+U)2(χ+U)2(ξ+V)2(1+dV)2(B1Θ2+B2Θ+B3)=0 |
where Θ1=V(μ−γ)−ξγξ+V
Θ1>0 with reference to condition (6.4). Hence, one of the eigenvalues is positive. This implies that the cybersecurity professional free equilibrium point is unstable.
The user equilibrium point and cybersecurity professional free equilibrium point is unstable due to the following reasons:
● Cybersecurity professionals assist in strengthening systems against possible future threats by putting strong security measures in place and making sure that organizations are safe even in the absence of direct attacks.
● Accidental breaches, internal mistakes, and system malfunctions can happen even in the absence of external hackers. Professionals in cybersecurity are qualified to handle security problems and respond to them in a way that minimizes or sometimes completely protects from damage that impacts users and organizations.
● Cybersecurity professionals are responsible for ensuring that organisations follow pertinent laws, guidelines, information security, and best practices in data protection. Compliance controls aid in preserving the reliability and integrity of data and systems.
Hence, the presence of the hackers and cybersecurity professionals keep the cyber ecosystem stable.
Lyapunov characteristic exponent (LCE) or the Lyapunov exponent (LE) of a system, as referenced in [33,34], quantifies the rate at which closely situated trajectories diverge. The sign of the LE determines the state of a given dynamical system.
The conditions for a 3D-dimensional chaotic system with LE are as follows:
● Let all exponents be negative, i.e., (−,−,−). In this case, the attractor reduces to a stable fixed point.
● Let one of the LEs be zero and the rest of them be negative, i.e., (0,−,−). In this case, the attractor exhibits a limit cycle.
● Let at least one of the LEs be positive, i.e., ((+,0,−),(+,−,−),(+,0,0)). In this case, the given system displays a chaotic behavior and instability.
● Let two ones of the LEs be zero and the other one be negative, i.e., (0,0,−). In this case, the given system exhibits a two-torus.
● When at least two ones of the LE are positive, i.e., ((+,+,−),(+,+,0),(+,+,+)), the given system demonstrates a hyper-chaotic behavior.
After computing the numerical values of the Lyapunov exponents (LE) [35] and investigating the chaotic behavior of the 4D hyper-chaotic climatic model, we have written the obtained values of the LEs in Tables 2, 3, and 4 for different values of the order α.
Times | LE1 | LE2 | LE3 |
10 | -0.117 | -0.019 | 0.1032 |
15 | -0.125 | -0.014 | 0.095 |
18 | -0.085 | -0.035 | 0.079 |
20 | -0.913 | 0.417 | -0.092 |
22 | -0.080 | -0.012 | 0.025 |
Times | LE1 | LE2 | LE3 |
10 | -0.078 | -0.099 | 0.118 |
15 | -0.073 | -0.059 | 0.048 |
18 | -0.062 | -0.066 | 0.049 |
20 | -0.056 | -0.070 | 0.050 |
22 | -0.048 | -0.139 | 0.094 |
Times | LE1 | LE2 | LE3 |
10 | -0.337 | -0.276 | 0.376 |
15 | -0.305 | -0.312 | 0.381 |
18 | -0.254 | -0.357 | 0.331 |
20 | -0.882 | -0.272 | 0.137 |
22 | -0.771 | -0.019 | 0.018 |
● By considering α=1 in Table 2, the negative sum of the LE indicates the dissipativeness of the FO chaotic system. The presence of one positive LE at each timeline confirms its chaotic behavior.
● Tables 3 and 4 show the LEs versus the time series for the orders α = 0.89 and α = 0.7, respectively. In both tables, only one positive LE is observed at each timeline, signifying a reduction of chaotic behavior with varying the values for the fractional order. The same is visible in Figure 2.
It is notable that the chosen initial conditions are U(0)=0.3251,V(0)=0.2093,and W(0)=0.0264.
Here, we present the numerical method used to solve the cyber ecosystem 3.1. We use the generalized Adams-Bashforth Moulton technique [28] to solve the system 3.1. The solution to the system 3.1, by using the Adams-Bashforth Moulton technique, can be represented as:
Un+1=U0+hα1Γ(α1+2)(Upn+1(1−Upn+1)b1+dVpn+1−βUpn+1Vpn+1Upn+1+σ)+hα1Γ(α1+2)n∑i=0ai,n+1(Ui(1−Ui)b1+dVi−βUiViUi+σ),Vn+1=V0+hα2Γ(α2+2)(−δVpn+1+θρUpn+1Vpn+1Upn+1+χ−λVpn+1Wpn+1Vpn+1+ϕWpn+1+ξ)+hα2Γ(α2+2)n∑i=0ai,n+1(−δVi+θρUiViUi+χ−λViWiVi+ϕWi+ξ),Wn+1=W0+hα3Γ(α3+2)(−γWpn+1+μVpn+1Wpn+1Vpn+1+ϕWpn+1+ξ)+hα3Γ(α3+2)n∑i=0ai,n+1(−γWi+μViWiVi+ϕWi+ξ). |
where
Upn+1=U0+hα1Γ(α1+1)n∑i=0bi,n+1(Ui(1−Ui)b1+dVi−βUiViUi+σ),Vpn+1=V0+hα2Γ(α2+1)n∑i=0bi,n+1(−δVi+θρUiViUi+χ−λViWiVi+ϕWi+ξ),Wpn+1=W0+hα3Γ(α3+1)n∑i=0bi,n+1(−γWi+μViWiVi+ϕWi+ξ). |
In which
ai,n+1={nα+1−(n−α)(n+1)α,i=0,(n−i+2)α+1+(n−i)α+1−2(n−i+1)α+1,1≤i≤n,1,i=n+1,
and
bi,n+1=((n−i+1)α−(n−i)α),0≤i≤n. |
Here, discuss the five scenarios and analyze it graphically. The values of the parameter we used are as follows:
b=1,d=0.02,β=1,σ=0.5,δ=1.15,θ=0.8,ρ=1,λ=1,ϕ=0.21,ξ=0.08,γ=0.35μ=0.55,κ1=0.02,κ2=0.3
Case 1: Dynamics of system without fear and refuge:
DαtU=U(1−U)−βUVU+σ,DαtV=−δV+θρUVU+χ−λVWV+ϕW+ξ,DαtW=−γW+μVWV+ϕW+ξ. | (8.1) |
This system indicates the dynamic interrelation between users, hackers, and cybersecurity professionals. The chaotic behavior, which is obtained in Figure 3, is a reflection of the intrinsic complexity and unpredictability of the cyber ecosystem, where various multiple factors and interactions cause nonlinear dynamical behavior. Gaining an understanding of these conditions, it is crucial to create strategies that effectively manage and reduce cyber risks in a society that is becoming more digitally linked and networked. Case 2: Dynamics of system (8.1) with fear factor to user:
DαtU=b1+dVU(1−U)−βUVU+σ,DαtV=−δV+θρUVU+χ−λVWV+ϕW+ξ,DαtW=−γW+μVWV+ϕW+ξ. | (8.2) |
Here, 11+dV is the fear factor induced to users by hackers. b is the growth rate of users and d is the fear factor. Figure 4 represents the case when the fear factor is induced to users by hackers. Users become uncertain and anxious due to hacker's fear, which causes irregular and unpredictable behavior within the cyber environment. In this scenario, users become more vulnerable to social engineering techniques used by hackers, such as phishing emails or fraudulent websites, which would increase the chaos inside the system. This was further explained in Case Ⅳ with the refuge factor.
Case 3: System (8.1) with fear factor induced to hackers:
DαtU=bU(1−U)−βUVU+σ,DαtV=−δV+θρUVU+χ11+dV−λVWV+ϕW+ξ,DαtW=−γW+μVWV+ϕW+ξ. | (8.3) |
A closed trajectory within the phase space is denoted by a limit cycle. This trajectory has a unique property: At least one other trajectory converges toward it, either as time extends infinitely or as time reaches towards negative infinity. Limit cycles serve as effective models for understanding the behavior of numerous real-world oscillatory systems especially in nonlinear systems. Figures 5 and 6 represent the case when the fear factor (d) is induced to hackers from cybersecurity professionals. We have been introduced the two different values of the fear. In both cases, we can see space phase trajectories are reduced from chaotic behavior to a limit cycle. The imposition of fear by cybersecurity professionals may act as a form of deterrence, discouraging hackers from engaging in high-risk or aggressive tactics that could reduce the chaotic behavior in the cyber ecosystem. Here, some trajectories spiral in and some out, which rotates the boundary of the closed curve, and the maximum nearby neighborhood of the orbits moves outside the neighborhood, which leads to unstable limit cycle [36,37]. The coexistence equilibrium point is (0.76882′,0.293251,0.417009). The eigenvalues at this point are: −0.494661,0.187604+i0.435966, and 0.187604−i0.435966.
Since two of the eigenvalues have positive real parts, the system exhibits unstable behavior.
Case 4: System (8.1) with refuge factor induced to users:
DαtU=bU(1−U)−βUV(1−κ1)U(1−κ1)+σ,DαtV=−δV+θρUV(1−κ1)U(1−κ1)+χ−λVWV+ϕW+ξ,DαtW=−γW+μVWV+ϕW+ξ. | (8.4) |
In real life, not all members of the users group are exposed to hackers, since they often have refuges where they can hide from the predators. Therefore, here we suppose that only a fraction (1−κ1) of prey species is accessible to hackers and that a portion κ1U of users is fully protected from hackers. Here, κ1 is the refuge factor induced to users. When the refuge factor is induced to users, the chaos of the system has been reduced, which is seen in Figure 7. Cybersecurity solutions help users strengthen their safety measures against possible threats by erecting a barrier that discourages hackers and lessens the chance of security breaches. Implementing security controls such as firewalls and antivirus software, threat intelligence analysis, user awareness and training, and many other security measures shelter users from direct exposure to criminal activity, which reduces the probability of instability that would arise inside the cyber environment. When users believe that they are sufficiently safeguarded by cybersecurity experts, they are less likely fear hackers, who try to instill it in them. Furthermore, the existence of strong cybersecurity defenses helps to stop the methods and plans of hackers, thereby limiting the chaos within the system.
Case 5: System of FDEs (8.1) with refuge factor induced to hackers:
DαtU=bU(1−U)−βUVU+σ,DαtV=−δV+θρUVU+χ−λVW(1−κ2)V(1−κ2)+ϕW+ξ,DαtW=−γW+μVW(1−κ2)V(1−κ2)+ϕW+ξ. | (8.5) |
Figure 8 shows the behavior of the system when the hackers are hidden or undetected by cybersecurity professionals. Here, κ2 is refuge the factor related to hackers. When hackers successfully remain hidden from cybersecurity professionals, it leads to instability within the cyber ecosystem. This instability occurs because cybersecurity professionals are unable to identify the presence of the threats caused by hackers, which hinders their ability to implement effective mitigation strategies and respond to cyber attacks. As a result, the undetected activities of the hackers can proliferate unchecked, leading to potential vulnerabilities, data breaches, and compromised system integrity. This highlights the critical importance of advanced detection mechanisms and proactive security measures to maintain stability and security in the cyber ecosystem.
The practical implications of the findings of this study:
1. By understanding the stability conditions and how various parameters influence the dynamics of the system, cybersecurity professionals can develop more adaptive and robust defense mechanisms. This includes adjusting their strategies based on the predicted behavior of hackers and online users, potentially preventing or mitigating attacks more effectively.
2. Policymakers can use the study's findings to design regulations and policies that create an environment less conducive to cybercrime. Understanding the impact of fear and refuge factors, for instance, can lead to policies that promote safer online behavior and environments, thus reducing the overall threat level.
3. This model raises the awareness about safe online practices and the importance of cybersecurity measures by behavioral dynamics of online users.
Remark 8.1 All the figures are directly propotional to FO derivative. As the FO derivative decreases, the chaotic behavior also reduces, which underscores the direct proportionality between fractional derivatives and system stability. This suggests that FO derivatives provide more accurate control over chaotic dynamics than do integer derivatives, which is why FOD are invaluable in a variety of industries, such as engineering and finance, where managing and comprehending complicated systems is crucial.
Figure 9 represents a geometric figure of a trajectory's behavior at a specific cross section of the attractor. This cross section is essentially a slice through the attractor, perpendicular to the flow or bundle of trajectories [38]. The intricate branches and twigs visible in these depictions unveil the chaotic and complex foldings within the atmospheric attractor described by equation (8.1). We have depicted the Poincaré maps of the system (8.1) for α=1. Projection on U−V plane while cutting through the plane W=0.15 is projected in Figure 9(a). If the section is made by the plane U=0.7 the dynamics of the system in V−W plane is as shown in Figure 9(b). On the other hand, projection on U−W plane making a section by V=0.15 plane can be seen in Figure 9(c).
From Figure 9 (a)–(c), the 3D representation of the Figure 3 (a) is cut and projected into two dimensions. This projection uncovers the internal structure of the attractor, offering an alternative perspective on it. Since we have focused on a single cross-section through the attractor, these figures provide precise information about the attractor's shape, general position in phase space, and other characteristics. Additionally, they highlight the anatomy of the attractor with much greater detail and clarity compared to a standard phase space plot. Poincaré section is a vast reduction in the size of the dataset, because it allows us to ignore most points along the trajectory and focus only on the points on or near the Poincaré section.
Using a 3D chaotic model with Caputo FOD, we recently studied the relationships between users, hackers, and cybersecurity experts. In our model, we investigated the existence, uniqueness, and boundedness of the solution. Our examination of stability, existence, and equilibrium points highlighted how important cybersecurity is. In addition, we looked at particular different scenarios involving fear-induced responses in users and hackers, as well as refuge-seeking behaviors. From this, we have shown the indispensable role of cybersecurity professionals in ensuring that users are adequately protected and can navigate the online world with confidence. We used phase portraits to analyse the effects of FOD on the dynamics of chaotic systems. We found evidence for chaotic behavior in Lyapunov exponents. Furthermore, the analysis of Poincaré sections reflects the complex chaotic movement in the system. In our predicted cybernetic model, our results imply that FOD can successfully manage chaos, contributing to our understanding of intricate dynamics in the cyber ecosystem. This holistic approach in modeling analysis enhances our comprehension of intricate cybernetic dynamics and aids decision-making in the face of persistent hacking threats by employing protective measures such as firewalls, antivirus software, robust passwords, software updates, and prudent handling of suspicious links. For future research, it is important to address the sensitivity of the model's outcomes to various logical parameters. Small changes in these parameters can lead to significantly different results, potentially limiting the model's robustness and its ability to generalize across different scenarios. Furthermore, this study primarily relies on theoretical and numerical analysis. To enhance the practical applicability and validation of the model's predictions, one can incorporate empirical validation using real-world data.
The authors declare they have not used Artificial Intelligence (AI) tools in the creation of this article.
J.F.G.A., M.K.N., R.G.: Conceptualization; M.K.N., C.B., İ.A.: Methodology; C.B., İ.A.: Software; C.B., İ.A., E.P.C.: Validation; J.F.G.A., R.G., E.P.C.: Formal analysis; J.F.G.A., C.B., E.P.C.: Investigation; M.K.N., C.B., İ.A.: Writing-original draft preparation; J.F.G.A., R.G., C.B.: Writing-review and editing; J.F.G.A., C.B.: Supervision; R.G.: Project administration. All authors have read and agreed to the published version of the manuscript.
The authors extend their appreciation to Prince Sattam bin Abdulaziz University, Al-Kharj, Saudi Arabia for funding this research work through the project number (2024/01/922572).
The authors declare no conflict of interest.
[1] |
Y. Li, Q. Liu, A comprehensive review study of cyber-attacks and cyber security, Emerging trends and recent developments, Energy Rep., 7 (2021), 8176–8186. https://doi.org/10.1016/j.egyr.2021.08.126 doi: 10.1016/j.egyr.2021.08.126
![]() |
[2] |
S. Chng, H. Y. Lu, A. Kumar, D. Yau, Hacker types, motivations and strategies: A comprehensive framework, Comput. Hum. Behav. Rep., 5 (2022), 100167. https://doi.org/10.1016/j.chbr.2022.100167 doi: 10.1016/j.chbr.2022.100167
![]() |
[3] | M. Grobler, R. Gaire, S. Nepal, User, usage and usability: Redefining human centric cyber security, Front. Big Data, 4 (2021). https://doi.org/10.3389/fdata.2021.583723 |
[4] | A. A. Moustafa, A. Bello, The role of user behaviour in improving cyber security management, Front. Psychol., 12 (2021). https://doi.org/10.3389/fpsyg.2021.561011 |
[5] |
J. Wang, H. Li, Surpassing the fractional derivative: Concept of the memory dependent derivative, Comput. Math. Appl., 62 (2011), 1562–1567. https://doi.org/10.1016/j.camwa.2011.04.028 doi: 10.1016/j.camwa.2011.04.028
![]() |
[6] |
L. Zanette, A. White, A. C. Allen, M. Clinchy, Perceived predation risk reduces the number of offspring songbirds produce per year, Science, 334 (2011), 1398–1401. https://dx.doi.org/10.1126/science.1210908 doi: 10.1126/science.1210908
![]() |
[7] | L. Y. Zanette, M. Clinchy, Ecology of fear, Curr. Biol., 29 (2019), 309–313. https://doi.org/10.1016/j.cub.2019.02.042 |
[8] |
J. P. Tripathi, P. S. Mandal, A. Poonia, V. P. Bajiya, A widespread interaction between generalist and specialist enemies: The role of intraguild predation and allee effect, Appl. Math. Model., 89 (2021), 105–135. https://doi.org/10.1016/j.apm.2020.06.074 doi: 10.1016/j.apm.2020.06.074
![]() |
[9] |
R. K. Upadhyay, Chaotic dynamics in a three species aquatic population model with holling type Ⅱ functional response, Nonlinear Anal-Model., 13 (2008), 103–115. https://doi.org/10.15388/NA.2008.13.1.14592 doi: 10.15388/NA.2008.13.1.14592
![]() |
[10] |
R. K. Upadhyay, R. D. Parshad, K. Antwi-Fordjour, E. Quansah, S. Kumari, Global dynamics of stochastic predator–prey model with mutual interference and prey defense, J. Appl. Math. Comput., 60 (2019), 169–190. https://doi.org/10.1007/s12190-018-1207-7 doi: 10.1007/s12190-018-1207-7
![]() |
[11] |
S. Kim, K. Antwi-Fordjour, Prey group defense to predator aggregated induced fear, Eur. Phys. J. Plus, 137 (2022), 1–17. https://doi.org/10.1140/epjp/s13360-022-02926-x doi: 10.1140/epjp/s13360-022-02926-x
![]() |
[12] | A. A. Kilbas, H. M. Srivastava, J. J. Trujillo, Theory and Applications of Fractional Differential Equations, Elsevier, 2006. |
[13] | B. Ross, Fractional Calculus and Its Applications, Proceedings of the International Conference held at the University of New Haven, Springer, 2014. |
[14] |
A. Atangana, New fractional derivatives with nonlocal and non-singular kernel: Theory and application to heat transfer model, Therm. Sci., 20 (2016), 763–769. https://doi.org/10.2298/TSCI160111018A doi: 10.2298/TSCI160111018A
![]() |
[15] |
M. Caputo, M. Fabrizio, A new definition of fractional derivative without singular Kernel, Progr. Fract. Differ. Appl., 1 (2015), 73–85. https://doi.org/10.12785/pfda/010201 doi: 10.12785/pfda/010201
![]() |
[16] | M. K. Naik, C. Baishya, P. Veeresha, D. Baleanu, Design of a fractional-order atmospheric model via a class of ACT-like chaotic system and its sliding mode chaos control, Chaos, 33 (2023). https://doi.org/10.1063/5.0130403 |
[17] | R. N. Premakumari, C. Baishya, M. Sajid, M. K. Naik, Modeling the dynamics of a marine system using the fractional order approach to assess its susceptibility to global warming, Results Nonlinear Anal., 7 (2024), 89–109. |
[18] | S. N Raw, P. Mishra, B. P. Sarangi, B. Tiwari, Appearance of temporal and spatial chaos in an ecological system: A mathematical modeling study, Iranian Journal of Science and Technology, Transactions A: Science, 45 (2021) 1417–1436. https://doi.org/10.1007/s40995-021-01139-8 |
[19] | S. Gao, H. Lu, M. Wang, D. Jiang, A. A. Abd El-Latif, R. Wu, et al., Design, hardware implementation, and application in video encryption of the 2D memristive cubic map, IEEE Int. Things, 11 (2024). https://doi.org/10.1109/JIOT.2024.3376572 |
[20] | S. Gao, R. Wu, X. Wang, J. iu, Q. Li, X. Tang, EFR-CSTP: Encryption for face recognition based on the chaos and semi-tensor product theory, Inf. Sci., 621 (2023) 766–781. https://doi.org/10.1016/j.ins.2022.11.121 |
[21] | N. Malleson, A. Evans, Agent-based models to predict crime at places, Encyclopedia of Criminology Criminal Justice, 12 (2013) 41–48. https://doi.org/10.1007/978-1-4614-5690-2_208 |
[22] |
M. K. Naik, C. Baishya, P. Veeresha, A chaos control strategy for the fractional 3D Lotka- Volterra like attractor, Math. Comput. Simul., 211 (2023), 1–22. https://doi.org/10.1016/j.matcom.2023.04.001 doi: 10.1016/j.matcom.2023.04.001
![]() |
[23] |
T. Bosse, C. Gerritsen, M. Hoogendoorn, S. W. Jaffry, J. Treur, Agent-based vs. population-based simulation of displacement of crime: A comparative study, Web Intelligence and Agent Systems: An International Journal, 9 (2011), 147–160. https://dx.doi.org/10.3233/WIA-2011-0212 doi: 10.3233/WIA-2011-0212
![]() |
[24] |
P. A. Jones, P. J. Brantingham, L. R. Chayes, Statistical models of criminal behavior: The effects of law enforcement actions, Math. Mod. Meth. Appl. S., 20 (2010), 1397–1423. https://doi.org/10.1142/S0218202510004647 doi: 10.1142/S0218202510004647
![]() |
[25] | S. Gao, R. Wu, X. Wang, J. Liu, Q. Li, X. Tang, Asynchronous updating Boolean network encryption algorithm, IEEE T. Circ. Syst. Vid., (2023). https://dx.doi.org/10.1109/TCSVT.2023.3237136 |
[26] |
S. Gao, H. H. Iu, J. Mou, U. Erkan, J. Liu, R Wu, et al., Temporal action segmentation for video encryption, Chaos, Soliton. Fract., 183 (2024), 114958. https://doi.org/10.1016/j.chaos.2024.114958 doi: 10.1016/j.chaos.2024.114958
![]() |
[27] |
S. Raw, B. Mishra, B. Tiwari, Mathematical study about a predator–prey model with antipredator behavior, Int. J. Appl. Comput. Math., 6 (2020), 1–22. https://doi.org/10.1007/s40819-020-00822-5 doi: 10.1007/s40819-020-00822-5
![]() |
[28] | K. Diethelm, N. J. Ford, A. D. Freed, A Predictor-Corrector approach for the numerical solution of fractional differential equations, Nonlinear Dynam., 29 (2002) 3–22. https://doi.org/10.1023/A: 1016592219341 |
[29] | I. Podlubny, Fractional Differential Equations, Elsevier, 1999. |
[30] |
X. Wang, Y. j. He, M. j. Wang, Chaos control of a fractional order modified coupled dynamos system, Nonlinear Anal-Theory, 71 (2009), 6126–6134. https://doi.org/10.1016/j.na.2009.06.065 doi: 10.1016/j.na.2009.06.065
![]() |
[31] |
H. Li, L. Zhang, C. Hu, Y. Jiang, Z. Teng, Dynamical analysis of a fractional-order predatorprey model incorporating a prey refuge, J. Appl. Math. Comput., 54 (2017), 435–449. https://doi.org/10.1007/s12190-016-1017-8 doi: 10.1007/s12190-016-1017-8
![]() |
[32] |
E. Ahmed, A. S. Elgazzar, On fractional order differential equations model for nonlocal epidemics, Physica A., 379 (2007), 607–614. https://doi.org/10.1016/j.physa.2007.01.010 doi: 10.1016/j.physa.2007.01.010
![]() |
[33] |
N. Sene, Introduction to the fractional-order chaotic system under fractional operator in Caputo sense, Alex. Eng. J., 60 (2021), 3997–4014. https://doi.org/10.1016/j.aej.2021.02.056 doi: 10.1016/j.aej.2021.02.056
![]() |
[34] | C. Baishya, M. K. Naik, R. N. Premakumari, Design and implementation of a sliding mode controller and adaptive sliding mode controller for a novel fractional chaotic class of equations, Results Control Optim., 14 (2024). https://doi.org/10.1016/j.rico.2023.100338 |
[35] | M. Sandri, Numerical calculation of Lyapunov exponents, Math. J., 6 (1996), 78–84. |
[36] |
A. Sharp, J. Pastor, Stable limit cycles and the paradox of enrichment in a model of chronic wasting disease, Ecolog. Appl., 21 (2011), 1024–1030. https://doi.org/10.1890/10-1449.1 doi: 10.1890/10-1449.1
![]() |
[37] |
E. Gonzalez-Olivares, H. Meneses-Alcay, B. Gonzalez-Yanez, J. Mena-Lorca, A. Rojas-Palma, R. Ramos-Jiliberto, Multiple stability and uniqueness of the limit cycle in a Gause-type predator-prey model considering the Allee effect on prey, Nonlinear Anal-Real, 12 (2011), 2931–2942. https://doi.org/10.1016/j.nonrwa.2011.04.003 doi: 10.1016/j.nonrwa.2011.04.003
![]() |
[38] | G. Williams, Chaos Theory Tamed, CRC press, 1997. |
1. | Anqi Wang, Dandan Xue, Zhanyu Wang, Jian Zhao, Feng Rao, Dynamics of a stochastic tumor–immune interaction system, 2024, 139, 2190-5444, 10.1140/epjp/s13360-024-05898-2 | |
2. | Samir Biswas, Lakpa Thendup Bhutia, Tapan Kumar Kar, Impact of the herd harvesting on a temporal and spatiotemporal prey–predator model with certain prey herd behaviors and density-dependent cross-diffusion, 2024, 139, 2190-5444, 10.1140/epjp/s13360-024-05862-0 | |
3. | Mudasir Younis, Haroon Ahmad, Mahpeyker Ozturk, Fahim Ud Din, Muhammad Qasim, Unveiling fractional-order dynamics: A new method for analyzing Rössler Chaos, 2025, 468, 03770427, 116639, 10.1016/j.cam.2025.116639 |
Parameters | Description |
b | Growth rate of users in online platform |
β | Reduction rate of users due to hackers |
σ1 | Attack threshold constant of user at which loss rate is half of β |
χ1 | Attack threshold constant of the user at which gain to hackers is half of ρ |
K | Carrying capacity of the users |
ϕ1 | Escape rate from cybersecurity professionals |
δ | Caught rate of hackers |
λ | Security in support of user |
ρ | Maximum profit gain for hackers due to hacking of user |
θ | Growth rate of hackers |
γ | Retirement of cybersecurity professionals |
ξ1 | Normalizing coefficient to the environment for interaction of hacker and cybersecurity professionals |
μ | Gain for cybersecurity professionals when hackers got caught |
d | Fear factor |
Times | LE1 | LE2 | LE3 |
10 | -0.117 | -0.019 | 0.1032 |
15 | -0.125 | -0.014 | 0.095 |
18 | -0.085 | -0.035 | 0.079 |
20 | -0.913 | 0.417 | -0.092 |
22 | -0.080 | -0.012 | 0.025 |
Times | LE1 | LE2 | LE3 |
10 | -0.078 | -0.099 | 0.118 |
15 | -0.073 | -0.059 | 0.048 |
18 | -0.062 | -0.066 | 0.049 |
20 | -0.056 | -0.070 | 0.050 |
22 | -0.048 | -0.139 | 0.094 |
Times | LE1 | LE2 | LE3 |
10 | -0.337 | -0.276 | 0.376 |
15 | -0.305 | -0.312 | 0.381 |
18 | -0.254 | -0.357 | 0.331 |
20 | -0.882 | -0.272 | 0.137 |
22 | -0.771 | -0.019 | 0.018 |
Parameters | Description |
b | Growth rate of users in online platform |
β | Reduction rate of users due to hackers |
σ1 | Attack threshold constant of user at which loss rate is half of β |
χ1 | Attack threshold constant of the user at which gain to hackers is half of ρ |
K | Carrying capacity of the users |
ϕ1 | Escape rate from cybersecurity professionals |
δ | Caught rate of hackers |
λ | Security in support of user |
ρ | Maximum profit gain for hackers due to hacking of user |
θ | Growth rate of hackers |
γ | Retirement of cybersecurity professionals |
ξ1 | Normalizing coefficient to the environment for interaction of hacker and cybersecurity professionals |
μ | Gain for cybersecurity professionals when hackers got caught |
d | Fear factor |
Times | LE1 | LE2 | LE3 |
10 | -0.117 | -0.019 | 0.1032 |
15 | -0.125 | -0.014 | 0.095 |
18 | -0.085 | -0.035 | 0.079 |
20 | -0.913 | 0.417 | -0.092 |
22 | -0.080 | -0.012 | 0.025 |
Times | LE1 | LE2 | LE3 |
10 | -0.078 | -0.099 | 0.118 |
15 | -0.073 | -0.059 | 0.048 |
18 | -0.062 | -0.066 | 0.049 |
20 | -0.056 | -0.070 | 0.050 |
22 | -0.048 | -0.139 | 0.094 |
Times | LE1 | LE2 | LE3 |
10 | -0.337 | -0.276 | 0.376 |
15 | -0.305 | -0.312 | 0.381 |
18 | -0.254 | -0.357 | 0.331 |
20 | -0.882 | -0.272 | 0.137 |
22 | -0.771 | -0.019 | 0.018 |