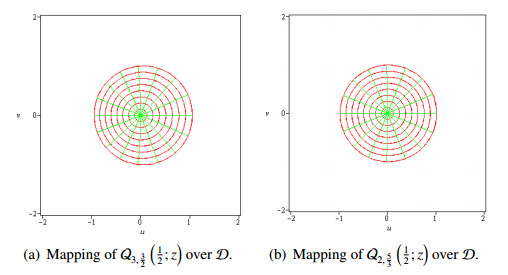
In this paper, we introduced a family of distributions with a very flexible shape named generalized scale mixtures of generalized asymmetric normal distributions (GSMAGN). We investigated the main properties of the new family including moments, skewness, kurtosis coefficients and order statistics. A variant of the expectation maximization (EM)-type algorithm was established by combining the proflie likihood approach (PLA) with the classical expectation conditional maximization (ECM) algorithm for parameter estimation of this model. This approach with analytical expressions in the E-step and tractable M-step can greatly improve the computational speed and efficiency of the algorithm. The performance of the proposed algorithm was assessed by some simulation studies. The feasibility of the proposed methodology was illustrated through two real datasets.
Citation: Ruijie Guan, Aidi Liu, Weihu Cheng. The generalized scale mixtures of asymmetric generalized normal distributions with application to stock data[J]. AIMS Mathematics, 2024, 9(1): 1291-1322. doi: 10.3934/math.2024064
[1] | Kamaraj Dhurai, Nak Eun Cho, Srikandan Sivasubramanian . On a class of analytic functions closely related to starlike functions with respect to a boundary point. AIMS Mathematics, 2023, 8(10): 23146-23163. doi: 10.3934/math.20231177 |
[2] | Lina Ma, Shuhai Li, Huo Tang . Geometric properties of harmonic functions associated with the symmetric conjecture points and exponential function. AIMS Mathematics, 2020, 5(6): 6800-6816. doi: 10.3934/math.2020437 |
[3] | Georgia Irina Oros, Gheorghe Oros, Daniela Andrada Bardac-Vlada . Certain geometric properties of the fractional integral of the Bessel function of the first kind. AIMS Mathematics, 2024, 9(3): 7095-7110. doi: 10.3934/math.2024346 |
[4] | Bo Wang, Rekha Srivastava, Jin-Lin Liu . Certain properties of multivalent analytic functions defined by q-difference operator involving the Janowski function. AIMS Mathematics, 2021, 6(8): 8497-8508. doi: 10.3934/math.2021493 |
[5] | Pinhong Long, Huo Tang, Wenshuai Wang . Functional inequalities for several classes of q-starlike and q-convex type analytic and multivalent functions using a generalized Bernardi integral operator. AIMS Mathematics, 2021, 6(2): 1191-1208. doi: 10.3934/math.2021073 |
[6] | K. Saritha, K. Thilagavathi . Differential subordination, superordination results associated with Pascal distribution. AIMS Mathematics, 2023, 8(4): 7856-7864. doi: 10.3934/math.2023395 |
[7] | Muajebah Hidan, Abbas Kareem Wanas, Faiz Chaseb Khudher, Gangadharan Murugusundaramoorthy, Mohamed Abdalla . Coefficient bounds for certain families of bi-Bazilevič and bi-Ozaki-close-to-convex functions. AIMS Mathematics, 2024, 9(4): 8134-8147. doi: 10.3934/math.2024395 |
[8] | Kholood M. Alsager, Sheza M. El-Deeb, Ala Amourah, Jongsuk Ro . Some results for the family of holomorphic functions associated with the Babalola operator and combination binomial series. AIMS Mathematics, 2024, 9(10): 29370-29385. doi: 10.3934/math.20241423 |
[9] | Zhi-Gang Wang, M. Naeem, S. Hussain, T. Mahmood, A. Rasheed . A class of analytic functions related to convexity and functions with bounded turning. AIMS Mathematics, 2020, 5(3): 1926-1935. doi: 10.3934/math.2020128 |
[10] | İbrahim Aktaş . On some geometric properties and Hardy class of q-Bessel functions. AIMS Mathematics, 2020, 5(4): 3156-3168. doi: 10.3934/math.2020203 |
In this paper, we introduced a family of distributions with a very flexible shape named generalized scale mixtures of generalized asymmetric normal distributions (GSMAGN). We investigated the main properties of the new family including moments, skewness, kurtosis coefficients and order statistics. A variant of the expectation maximization (EM)-type algorithm was established by combining the proflie likihood approach (PLA) with the classical expectation conditional maximization (ECM) algorithm for parameter estimation of this model. This approach with analytical expressions in the E-step and tractable M-step can greatly improve the computational speed and efficiency of the algorithm. The performance of the proposed algorithm was assessed by some simulation studies. The feasibility of the proposed methodology was illustrated through two real datasets.
In the literature, special functions have a great importance in a variety of fields of mathematics, such as mathematical physics, mathematical biology, fluid mechanics, geometry, combinatory and statistics. Due of the essential position of special functions in mathematics, they continue to play an essential role in the subject as well as in the geometric function theory. For geometric behavior of some other special functions, one can refer to [1,2,3,4,5,6,7,8,9,10,11,12]. An interesting way to discuss the geometric properties of special functions is by the means of some criteria due to Ozaki, Fejér and MacGregor. One of the important special functions is the Mathieu series that appeared in the nineteenth century in the monograph [13] defined on R by
S(r)=∑n≥12n(n2+r2)2. | (1.1) |
Surprisingly, the Mathieu series is considered in a variety of fields of mathematical physics, namely, in the elasticity of solid bodies [13]. For more applications regarding the Mathieu series, we refer the interested reader to [14, p. 258, Eq (54)]. The functions bear the name of the mathematician Émile Leonard Mathieu (1835–1890). Recently, a more general family of the Mathieu series was studied by Diananda [15] in the following form:
Sμ(r)=∑n≥12n(n2+r2)μ+1(μ>0,r∈R). | (1.2) |
In 2020, Gerhold et al. [16], considered a new Mathieu type power series, defined by
Sα,β,μ(r;z)=∞∑k=0(k!)αzk((k!)β+r2)μ+1, | (1.3) |
where α,μ≥0,β,r>0 and |z|≤1, such that α<β(μ+1).
In [17], Bansal and Sokól have determined sufficient conditions imposed on the parameters such that the normalized form of the function S(r,z) belong to a certain class of univalent functions, such as starlike and close-to-convex. In [18], the authors presented some generalizations of the results of Bansal and Sokól by using the same technique. In addition, Gerhold et al. [18, Theorems 5 and 6] has established some sufficient conditions imposed on the parameter of the normalized form of the function S1,2,μ(r;z) defined by
Qμ(r;z):=z+∞∑n=2n!(r2+1)μ+1((n!)2+r2)μ+1zn, | (1.4) |
to be starlike and close-to-convex in the open unit disk. The main focus of the present paper is to extend and improve some results from [18] by using a completely different method. More precisely, in this paper we present some sufficient conditions, such as the normalized form of the function S1,β,μ(r;z) defined by
Qμ,β(r;z)=z+∞∑n=2n!(r2+1)μ+1zn((n!)β+r2)μ+1, | (1.5) |
satisfying several geometric properties such as starlikeness, convexity and close-to-convexity.
We denoted by H the class of all analytic functions inside the unit disk
D={z:z∈Cand|z|<1}. |
Assume that A denoted the collection of all functions f∈H, satisfying the normalization f(0)=f′(0)−1=0 such that
f(z)=z+∞∑k=2akzk,(∀z∈D). |
A function f∈A is said to be a starlike function (with respect to the origin zero) in D, if f is univalent in D and f(D) is a starlike domain with respect to zero in C. This class of starlike functions is denoted by S∗. The analytic characterization of S∗ is given [19] below:
ℜ(zf′(z)f(z))>0(∀z∈D). |
If f(z) is a univalent function in D and f(D) is a convex domain in C, then f∈A is said to be a convex function in D. We denote this class of convex functions by K, which can also be described as follows:
ℜ(1+zf′′(z)f′(z))>0(∀z∈D). |
An analytic function f in A is called close-to-convex in the open unit disk D if there exists a function g(z), which is starlike in D such that
ℜ(zf′(z)g(z))>0,∀z∈D. |
It can be noted that every close-to-convex function in D is also univalent in D (see, for details, [19,20]).
In order to show the main results, the following preliminary lemmas will be helpful. The first result is due to Ozaki (see also [21, Lemma 2.1]).
Lemma 1.1. [22] Let
f(z)=z+∞∑n=2anzn, |
be analytic in D. If
1≥2a2≥⋯≥(n+1)an+1≥⋯≥0, |
or if
1≤2a2≤⋯≤(n+1)an+1≤⋯≤2, |
then f is close-to-convex with respect to the function −log(1−z).
Remark 1.2. We note that, as Ponnusamy and Vuorinen pointed out in [21], proceeding exactly as in the proof of Lemma 1.1, one can verify directly that if a function f:D→C satisfies the hypothesis of the above lemma, then it is close-to-convex with respect to the convex function
z1−z. |
The next two lemmas are due to Fejér [23].
Lemma 1.3. Suppose that a function f(z)=1+∑∞k=2akzk−1, with ak≥0(∀k≥2) as analytic in D. If (ak)k≥1 is a convex decreasing sequence, i.e., ak−2ak+1+ak+2≥0 and ak−ak+1≥0 for all k≥1, then
ℜ(f(z))>12(∀z∈D). |
Lemma 1.4. Suppose that a f(z)=z+∑∞k=2akzk, with ak≥0(∀k≥2) as analytic in D. If (kak)k≥1 and (kak−(k+1)ak+1)k≥1 both are decreasing, then f is starlike in D.
Lemma 1.5 ([24]). Assume that f∈A. If the following inequality
|f(z)z−1|<1, |
holds for all z∈D, then f is starlike in
D12:={z∈Cand|z|<12}. |
Lemma 1.6 ([25]). Assume that f∈A and satisfies
|f′(z)−1|<1, |
for each z∈D, then f is convex in D12.
Theorem 2.1. Let μ,β>0 and 0<r≤1 such that β≥1+2μ+1. In addition, if the following condition holds:
H:(2β+12)μ+1≥4, |
then the function Qμ,β(r;z) is close-to-convex in D with respect to the function −log(1−z).
Proof. For the function Qμ,β(r;z), we have
a1=1andak=k!(r2+1)μ+1((k!)β+r2)μ+1(k≥2). |
To prove the result, we need to show that the sequence {kak}k≥1 is decreasing under the given conditions. For k≥2 we have
kak−(k+1)ak+1=(r2+1)μ+1[kk!((k!)β+r2)μ+1−(k+1)(k+1)!(((k+1)!)β+r2)μ+1]=k!(r2+1)μ+1[k((k!)β+r2)μ+1−(k+1)2(((k+1)!)β+r2)μ+1]=k!(r2+1)μ+1Ak(β,μ,r)[((k!)β+r2)(((k+1)!)β+r2)]μ+1, | (2.1) |
where
Ak(β,μ,r)=k(((k+1)!)β+r2)μ+1−(k+1)2((k!)β+r2)μ+1,k≥2. |
However, we have
Ak(β,μ,r)=(k1μ+1((k+1)!)β+k1μ+1r2)μ+1−((k+1)2μ+1(k!)β+(k+1)2μ+1r2)μ+1=exp((μ+1)log[k1μ+1((k+1)!)β+k1μ+1r2])−exp((μ+1)log[(k+1)2μ+1(k!)β+(k+1)2μ+1r2])=∞∑j=0[logj(k1μ+1((k+1)!)β+k1μ+1r2)−logj((k+1)2μ+1(k!)β+(k+1)2μ+1r2)](μ+1)jj!. | (2.2) |
In addition, for all k≥2, we have
k1μ+1((k+1)!)β+k1μ+1r2−(k+1)2μ+1(k!)β+(k+1)2μ+1r2=r2(k1μ+1−(k+1)2μ+1)+k1μ+1((k+1)!)β−(k+1)2μ+1(k!)β≥[k1μ+1−(k+1)2μ+1+k1μ+1((k+1)!)β2]+[k1μ+1((k+1)!)β2−(k+1)2μ+1(k!)β]=k1μ+1(1+((k+1)!)β2−((k+1)2k)1μ+1)+(k!)β(k1μ+1(k+1)β2−(k+1)2μ+1)≥k1μ+1(k+1)2μ+1(1+(k!)β(k+1)2−1k1μ+1)+(k!)β(k+1)2μ+1(k1μ+1(k+1)2−1)≥k1μ+1(k+1)2μ+1(1+(k!)βk1μ+1−1k1μ+1)+(k!)β(k+1)2μ+1(k1μ+1−1), | (2.3) |
which is positive by our assumption. Having (2.1)–(2.3), we conclude that the sequence (kak)k≥2 is decreasing. Finally, we see that the condition (H) implies that a1≥2a2, then the function Qμ,β(r;z) is close-to-convex in D with respect to the function −log(1−z) by Lemma 1.1.
If we set β=32 in Theorem 2.1, we derive the following result as follows:
Corollary 2.2. Let 0<r≤1. If μ≥3, then the function Qμ,32(r;z) is close-to-convex in D with respect to the function −log(1−z).
Upon setting μ=2 in Theorem 2.1, we get the following result:
Corollary 2.3. Let 0<r≤1. If β≥53, then the function Q2,β(r;z) is close-to-convex in D with respect to the function −log(1−z).
Remark 2.4. In [18], it is established that the function Qμ,2(r;z)=:Qμ(r;z) is close-to-convex in D with respect to the function z1−z for all 0<r≤√μ. Moreover, in view of Remark 1.2, we conclude that the function Qμ,2(r;z) is close-to-convex in D with respect to the function −log(1−z) for all 0<r≤√μ. However, in view of Corollaries 2.2 and 2.3, we deduce that Theorem 2.1 improves the corresponding result available in [18, Theorem 5] for 0<r≤1.
Theorem 2.5. Assume that μ,β>0,0<r≤1 such that β≥1+1μ+1. In addition, if the condition (H) holds, then
ℜ(Qμ,β(r;z)z)>12, |
for all z∈D.
Proof. For k≥1, we get
((k!)β+r2)μ+1(((k+1)!)β+r2)μ+1(ak−ak+1)(r2+1)μ+1=k!(((k+1)!)β+r2)μ+1−(k+1)!((k!)β+r2)μ+1=[(k!)1μ+1((k+1)!)β+r2)]μ+1−[((k+1)!)1μ+1((k!)β+r2)]μ+1. | (2.4) |
Further, for all k≥1, we have
(k!)1μ+1((k+1)!)β+r2)−((k+1)!)1μ+1((k!)β+r2)=r2[(k!)1μ+1−((k+1)!)1μ+1]+(k!)1μ+1((k+1)!)β−((k+1)!)1μ+1(k!)β≥(k!)1μ+1−((k+1)!)1μ+1+(k!)1μ+1((k+1)!)β−((k+1)!)1μ+1(k!)β=(k!)1μ+1[1+(k!)β(k+1)β2−(k+1)1μ+1]+(k!)β+1μ+1[(k+1)β2−(k+1)1μ+1]≥(k!)1μ+1[1+(k+1)1+1μ+12−(k+1)1μ+1]+(k!)β+1μ+1[(k+1)1+1μ+12−(k+1)1μ+1]=(k!)1μ+1[1+(k+1)1μ+1((k+1)2−1)]+(k!)β+1μ+1(k+1)1μ+1((k+1)2−1)>0. | (2.5) |
Hence, in view of (2.4) and (2.5), we deduce that the sequence (ak)k≥1 is decreasing. Next, we prove that (ak)k≥1 is a convex decreasing sequence, then, for k≥2 we obtain
((k!)β+r2)μ+1(((k+1)!)β+r2)μ+1(ak−2ak+1)(r2+1)μ+1=k!(((k+1)!)β+r2)μ+1−2(k+1)!((k!)β+r2)μ+1=[(k!)1μ+1((k+1)!)β+r2)]μ+1−[(2(k+1)!)1μ+1((k!)β+r2)]μ+1. | (2.6) |
Moreover, we get
(k!)1μ+1(((k+1)!)β+r2)−(2(k+1)!)1μ+1((k!)β+r2)=r2[(k!)1μ+1−(2(k+1)!)1μ+1]+(k!)1μ+1((k+1)!)β−(2(k+1)!)1μ+1(k!)β>[(k!)1μ+1−(2(k+1)!)1μ+1]+(k!)1μ+1((k+1)!)β3+2(k!)β+1μ+13[(k+1)β−3.2−μμ+1(k+1)1μ+1]≥(k!)1μ+1[1+((k+1)!)1+1μ+13−(2(k+1))1μ+1]+2(k!)β+1μ+13[(k+1)1+1μ+1−3.2−μμ+1(k+1)1μ+1]=(k!)1μ+1[1+(k+1)1μ+1{(k+1)(k!)1+1μ+13−21μ+1}]+2(k!)β+1μ+1(k+1)1μ+13[(k+1)−3.2−μμ+1]>2[1−2−μμ+1](k!)β+1μ+1(k+1)1μ+1>0. | (2.7) |
Keeping (2.6) and (2.7) in mind, we have ak−2ak+1>0 for all k≥2. In addition, the condition (H) implies a1−2a2≥0. This in turn implies that the sequence (ak)k≥1 is convex. Finally, by Lemma 1.3, we obtain the desired result.
Taking β=32 in Theorem 2.5, we derive the following result:
Corollary 2.6. Assume that r∈(0,1]. If μ≥log(4)log(232+1)−log(2)−1∼1.14, then
ℜ(Qμ,32(r;z)z)>12(∀z∈D). |
Setting μ=1 in Theorem 2.5, we established the following result which reads as follows:
Corollary 2.7. Let 0<r≤1. If β≥log(3)log(2), then
ℜ(Q1,β(r;z)z)>12(∀z∈D). |
Remark 2.8. The result obtained in the above theorem has been derived from [18, Theorem 6] for β=2,μ>0 and 0<r<√μ. Hence, in view of Corollaries 2.2 and 2.6, we deduce that Theorem 2.5 improves the corresponding result given in [18, Theorem 6] for 0<r≤1.
Theorem 2.9. Assume that min(μ,β)>0,0<r≤1 such that β≥1+3μ+1, then the function Qμ,β(r;z) is starlike in D.
Proof. We see in the proof of Theorem 2.1 that the sequence (kak)k≥1 is decreasing. Hence, with the aid of Lemma 1.4 to show that the function Qμ,β(r;z) is starlike in D, it suffices to prove that the sequence (kak−(k+1)ak+1)k≥1 is decreasing. We have
kak−2(k+1)ak+1=k!(r2+1)μ+1Bk(β,μ,r)[((k!)β+r2)((k+1)!)β+r2)]μ+1, | (2.8) |
where
Bk(β,μ,r)=k(((k+1)!)β+r2)μ+1−2(k+1)2((k!)β+r2)μ+1,k≥1. |
For k≥2, we have
k1μ+1(((k+1)!)β+r2)−(2(k+1)2)1μ+1((k!)β+r2)≥k1μ+1−(2(k+1)2)1μ+1+k1μ+1((k+1)!)β2+[k1μ+1((k+1)!)β2−(2(k+1)2)1μ+1(k!)β]=k1μ+1+k1μ+1((k+1)!)β2−(2(k+1)2)1μ+1+(k!)β(k1μ+1(k+1)β2−(2(k+1)2)1μ+1)≥k1μ+1+(k+1)2μ+1(k1μ+1(k!)β(k+1)2−21μ+1)+(k!)β(k+1)2μ+1(k1μ+1(k+1)2−21μ+1)≥k1μ+1+(k+1)2μ+1(k1μ+1(k!)β−21μ+1)+(k!)β(k+1)2μ+1(k1μ+1−21μ+1)>0, | (2.9) |
which in turn implies that
Bk(β,μ,r)>0, |
for all k≥2, and consequently, the sequence (kak−(k+1)ak+1)k≥2 is decreasing. Further, a simple computation gives
a1−4a2+3a3(1+r2)μ+1=1(1+r2)μ+1−8(2β+r2)μ+1+18(6β+r2)μ+1≥12μ+1−82β(μ+1)+18(6β+r2)μ+1=2β(μ+1)−2μ+42(β+1)(μ+1)+18(6β+r2)μ+1≥2μ+4−2μ+42(β+1)(μ+1)+18(6β+r2)μ+1>0. |
Therefore, (kak−(k+1)ak+1)k≥1 is decreasing, which leads us to the asserted result.
In the next Theorem we present another set of sufficient conditions to be imposed on the parameters so that the function Qμ,β(r;z) is starlike in D.
Theorem 2.10. Let the parameters be the same as in Theorem 2.1. In addition, if the following conditions
H∗:(2β+12)μ+1≥8(e−2), |
hold true, then the function Qμ,β(r;z) is starlike in D.
Proof. First of all, we need to prove that the sequences (uk)k≥2 and (vk)k≥2 defined by
uk=(k!)2(r2+1)μ+1((k!)β+r2)μ+1andvk=(k−1)(k!)2(r2+1)μ+1((k!)β+r2)μ+1, |
are decreasing. Indeed, we have
((k!)β+r2)μ+1(((k+1)!)β+r2)μ+1(uk−uk+1)(k!)2(r2+1)μ+1=(((k+1)!)β+r2)μ+1−(k+1)2((k!)β+r2)μ+1. | (2.10) |
In addition, for any k≥2, we have
((k+1)!)β+r2−(k+1)2μ+1((k!)β+r2)=r2(1−(k+1)2μ+1)+((k+1)!)β−(k+1)2μ+1(k!)β≥1−(k+1)2μ+1+((k+1)!)β−(k+1)2μ+1(k!)β=1+(((k+1)!)β2−(k+1)2μ+1)+(((k+1)!)β2−(k+1)2μ+1(k!)β)≥1+((k!)β(k+1)1+2μ+12−(k+1)2μ+1)+(k!)β((k+1)1+2μ+12−(k+1)2μ+1)=1+(k+1)2μ+1((k!)β(k+1)2−1)+(k!)β(k+1)2μ+1(k+12−1)>0. | (2.11) |
According to (2.10) and (2.11) we conclude that the sequence (uk)k≥2 is decreasing. Also, for k≥2, we have
((k!)β+r2)μ+1(((k+1)!)β+r2)μ+1(vk−vk+1)(k!)2(r2+1)μ+1=(k−1)(((k+1)!)β+r2)μ+1−k(k+1)2((k!)β+r2)μ+1. | (2.12) |
Moreover, for all k≥2, we find
(k−1)1μ+1(((k+1)!)β+r2)−(k(k+1)2)1μ+1((k!)β+r2)=r2((k−1)1μ+1−(k(k+1)2)1μ+1)+(k−1)1μ+1((k+1)!)β−(k(k+1)2)1μ+1(k!)β≥(k−1)1μ+1−(k(k+1)2)1μ+1+(k−1)1μ+1((k+1)!)β3+2(k−1)1μ+1((k+1)!)β3−(k(k+1)2)1μ+1(k!)β≥(k−1)1μ+1+(k+1)2μ+1((k−1)1μ+1(k!)1+2μ+1(k+1)3−k1μ+1)+(k!)β(k+1)2μ+1(2(k−1)1μ+1(k+1)3−k1μ+1)≥(k−1)1μ+1+(k+1)2μ+1((k−1)1μ+1(k!)1+2μ+1−k1μ+1)+(k!)β(k+1)2μ+1(2(k−1)1μ+1−k1μ+1). | (2.13) |
Since the sequence (k/(k−1))n≥2 is decreasing, we deduce that kk−1≤2 for all k≥2 and consequently,
(kk−1)1μ+1≤21μ+1≤2(k≥2,μ>0). |
Hence, in view of the above inequality combined with (2.13) and (2.12), we conclude that the sequence (vk)k≥2 is decreasing. Now, we set
˜Qμ,β(r;z):=z[Qμ,β(r;z)]′Qμ,β(r;z),z∈D. |
We see that the function ˜Qμ,β(r;z) is analytic in D and satisfies ˜Qμ,β(r;0)=1. Hence, to derive the desired result, it suffices to prove that, for any z∈D, we have
ℜ(˜Qμ,β(r;z))>0. |
For this goal in view, it suffices to show that
|˜Qμ,β(r;z)−1|<1(z∈D). |
For all z∈D, we get
|[Qμ,β(r;z)]′−Qμ,β(r;z)z|<∞∑k=2(k−1)k!(r2+1)μ+1((k!)β+r2)μ+1=∞∑k=2vkk!≤v2(e−2). | (2.14) |
In addition, in view of the inequality:
|a+b|≥||a|−|b||, |
we obtain
|Qμ,β(r;z)z|>1−∞∑k=2(k!)(r2+1)μ+1((k!)β+r2)μ+1=1−∞∑k=2ukk!≥1−u2(e−2). | (2.15) |
By using (2.14) and (2.15), for z∈D, we get
|˜Qμ,β(r;z)−1|=|[Qμ,β(r;z)]′−Qμ,β(r;z)z||Qμ,β(r;z)z|<v2(e−2)1−u2(e−2). | (2.16) |
Furthermore, by using the fact that the function r↦χμ,β(r)=(r2+1r2+2β)μ+1 is strictly increasing on (0,1], and with the aid of condition (H∗), we obtain
(v2+u2)(e−2)=8(e−2)(r2+1)μ+1(2β+r2)μ+1<8(e−2)(22β+1)μ+1≤1. | (2.17) |
Finally, by combining (2.16) and (2.17), we derived the desired results.
By setting β=2 in Theorem 2.10, we obtain the following corollary:
Corollary 2.11. If 0<r≤1 and μ≥1, then the function Qμ(r;z) defined in (1.4) is starlike in D.
Taking β=32 in Theorem 2.10, we obtain:
Corollary 2.12. Under the assumptions of Corollary 2.2, the function Qμ,32(r;z) is starlike in D.
Setting in Theorem 2.10 the values μ=2, we compute the following corollary:
Corollary 2.13. Suppose that all hypotheses of Corollary 2.3 hold, then the function Q2,β(r;z) is starlike in D.
Example 2.14. The functions Q3,32(1/2;z) and Q2,53(1/2;z) are starlike in D.
Figure 1 illustrates the mappings of the above examples in D.
Theorem 2.15. Let μ,β>0 and 0<r≤1 such that β≥1+3μ+1. If the following condition
H∗∗:(2β+12)μ+1≥16(e−2), |
holds true, then the function Qμ,β(r;z) is convex in D.
Proof. We define the sequences (xk)k≥2 and (yk)k≥2 by
xk=k(k!)2(r2+1)μ+1((k!)β+r2)μ+1andyk=k(k−1)(k!)2(r2+1)μ+1((k!)β+r2)μ+1. |
Let k≥2, then
((k!)β+r2)μ+1(((k+1)!)β+r2)μ+1(xk−xk+1)(k!)2(r2+1)μ+1=k(((k+1)!)β+r2)μ+1−(k+1)3((k!)β+r2)μ+1. | (2.18) |
However, we have
k1μ+1(((k+1)!)β+r2)−(k+1)3μ+1((k!)β+r2)≥k1μ+1−(k+1)3μ+1+k1μ+1((k+1)!)β−(k+1)3μ+1(k!)β=k1μ+1+(k1μ+1(k!)β(k+1)β2−(k+1)3μ+1)+(k1μ+1(k!)β(k+1)β2−(k+1)3μ+1(k!)β)≥k1μ+1+(k+1)3μ+1(k1μ+1(k!)β(k+1)2−1)+(k!)β(k+1)3μ+1(k1μ+1(k+1)2−1)>0. | (2.19) |
Hence, in view of (2.18) and (2.19), we get that (xk)k≥2 is decreasing. Also, we have
((k!)β+r2)μ+1(((k+1)!)β+r2)μ+1(yk−yk+1)k(k!)2(r2+1)μ+1=(k−1)(((k+1)!)β+r2)μ+1−(k+1)3((k!)β+r2)μ+1. | (2.20) |
Moreover, for k≥2, we find that
(k−1)1μ+1(((k+1)!)β+r2)−(k+1)3μ+1((k!)β+r2)≥(k−1)1μ+1+((k−1)1μ+1(k!)β(k+1)β2−(k+1)3μ+1)+(k!)β((k−1)1μ+1(n+1)β2−(n+1)3μ+1)≥(k−1)1μ+1+(k+1)3μ+1((k−1)1μ+1(k!)β(k+1)2−1)+(k!)β(k+1)3μ+1((k−1)1μ+1(k+1)2−1)>0. | (2.21) |
Having (2.20) and (2.21) in mind, we deduce that the sequence (yk)k≥2 is decreasing. To show that the function Qμ,β(r;z) is convex in D, it suffices to establish that the function
ˆQμ,β(r;z):=z[Qμ,β(r;z)]′, |
is starlike in D. For this objective in view, it suffices to find that
|z[ˆQμ,β(r;z)]′ˆQμ,β(r;z)−1|<1(∀z∈D). |
For all z∈D and since (yk)k≥2 is decreasing, we get
|[ˆQμ,β(r;z)]′−ˆQμ,β(r;z)z|<∞∑k=2k(k−1)k!(r2+1)μ+1((k!)β+r2)μ+1=∞∑k=2ykk!≤y2(e−2). | (2.22) |
Further, for any z∈D, we obtain
|ˆQμ,β(r;z)z|>1−∞∑k=2k(k!)(r2+1)μ+1((k!)β+r2)μ+1=1−∞∑k=2xkk!≥1−x2(e−2). | (2.23) |
Keeping (2.22) and (2.23) in mind, for z∈D, we get
|z[ˆQμ,β(r;z)]′ˆQμ,β(r;z)−1|=|[ˆQμ,β(r;z)]′−ˆQμ,β(r;z)z||ˆQμ,β(r;z)z|<y2(e−2)1−x2(e−2)=8(e−2)(r2+1)μ+1(2β+r2)μ+1−8(e−2)(r2+1)μ+1. | (2.24) |
Again, by using the fact that the function r↦χμ,β(r) is increasing on (0,1] and with the aid of hypothesis (H∗∗) we obtain that
8(e−2)(r2+1)μ+1(2β+r2)μ+1−8(e−2)(r2+1)μ+1<1. | (2.25) |
Finally, by combining the above inequality and (2.24), we obtain the desired result asserted by Theorem 2.15.
Taking β=2 in Theorem 2.15, in view of (1.4), the following result holds true:
Corollary 2.16. Let 0<r≤1. If μ≥2, then the function Qμ(r;z) is convex in D.
If we set μ=1 in Theorem 2.15, in view of (1.5), we derive the following result:
Corollary 2.17. Let 0<r≤1. If β≥log(8√e−2−1)log(2), then the function Q1,β(r;z) is convex in D.
Example 2.18. The functions Q2(r;z) and Q1,83(r;z) are convex in D.
Figure 2 gives the mappings of the above presented examples in D.
Theorem 2.19. Let the parameters be the same as in Theorem 2.1, then the function Qμ,β(r;z) is starlike in D12.
Proof. For any z∈D we get
|Qμ,β(r;z)z−1|<∞∑k=2k!(r2+1)μ+1(k!)β+r2)μ+1=∞∑k=2ckk!, | (2.26) |
where
ck:=(k!)2(r2+1)μ+1((k!)β+r2)μ+1,k≥2. |
Straightforward calculation gives
[((k!)β+r2)(((k+1)!)β+r2)]μ+1(ck−ck+1)(k!)2(r2+1)μ+1=(((k+1)!)β+r2)μ+1−((k+1)2μ+1((k!)β+r2))μ+1. | (2.27) |
Furthermore, for k≥2, we get
((k+1)!)β+r2−(n+1)2μ+1((k!)β+r2)=r2(1−(k+1)2μ+1)+((k+1)!)β−(k!)β(k+1)2μ+1≥(1+((k+1)!)β2−(k+1)2μ+1)+(k!)β((k+1)β2−(k+1)2μ+1)≥(1+(k!)β(k+1)1+2μ+12−(k+1)2μ+1)+(k!)β(k+1)2μ+1(k−1)2≥(1+(k+1)2μ+1((k!)β(k+1)−2)2)+(k!)β(k+1)2μ+1(k−1)2>0. | (2.28) |
Thus, the sequence (ck)k≥2 is decreasing. However, in view of (2.26), for z∈D we obtain
|Qμ,β(r;z)z−1|<∞∑k=2k!(r2+1)μ+1((k!)β+r2)μ+1=∞∑k=2c2k!=c2(e−2)=4(e−2)(r2+1)μ+1(2β+r2)μ+1. | (2.29) |
According to the monotony property of the function r↦χβ,μ(r) on (0,1) we get
χβ,μ(r)<14. | (2.30) |
Hence, in view (2.29) and (2.30) we find for all z∈D that
|Qμ,β(r;z)z−1|<(e−2)<1. |
With the help of Lemma 1.5, we deduce that the function Qμ,β(r;z) is starlike in D12.
Corollary 2.20. Assume that all conditions of Corollary 2.2 are satisfied, then the function Qμ,32(r;z) is starlike in D12.
Corollary 2.21. Suppose that all hypotheses of Corollary 2.3 hold, then the function Q2,β(r;z) is starlike in D12.
If we set β=2 in the above Theorem, in view of (1.4), the following result is true:
Corollary 2.22. Let 0<r≤1 If μ≥1, then the function Qμ(r;z) is starlike in D12.
Example 2.23. The functions Q3,32(1/2;z),Q1(1;z) and Q2,53(1/2;z) are starlike in D12.
In Figure 3, we give the mappings of the above presented examples in D.
Theorem 2.24. Let β,μ>0 and 0<r<1. If β≥1+3μ+1, then the function Qμ,β(r;z) is convex in D12.
Proof. For all z∈D, it follows that
|Q′μ,β(r;z)−1|<∞∑k=2kk!(r2+1)μ+1((k!)β+r2)μ+1=∞∑k=2dkk(k−1), | (2.31) |
where
dk:=k2(k−1)k!(r2+1)μ+1(k!)β+r2)μ+1,k≥2. |
For all k≥2, we get
((k!)β+r2)μ+1(((k+1)!)β+r2)μ+1(dk−dk+1)kk!(1+r2)μ+1=((k(k−1))1μ+1[((k+1)!)β+r2])μ+1−(((k+1))3μ+1[(k!)β+r2])μ+1. | (2.32) |
However, for all k≥2 and under the conditions imposed on the parameters, we have
(k(k−1))1μ+1[((k+1)!)β+r2]−((k+1))3μ+1[(k!)β+r2]≥(k(k−1))1μ+1−((k+1))3μ+1+(k(k−1))1μ+1((k+1)!)β−(k+1)3μ+1(k!)β=(k(k−1))1μ+1+((k(k−1))1μ+1(k!)β(k+1)β2−(k+1)3μ+1)+(k!)β((k(k−1))1μ+1(k+1)β2−(k+1)3μ+1)≥(k(k−1))1μ+1+(n+1)3μ+1((k(k−1))1μ+1(k!)β(k+1)2−1)+(k!)β(k+1)3μ+1((k(k−1))1μ+1(k+1)2−1)≥(k(k−1))1μ+1+(k+1)3μ+1((k(k−1))1μ+1(k!)β−1)+(k!)β(k+1)3μ+1((k(k−1))1μ+1−1)>0. | (2.33) |
Hence, in view of (2.32) and (2.33) we conclude that the sequence (dk)k≥2 is decreasing. Therefore, by (2.31), we conclude
|Q′μ,β(r;z)−1|<∑k≥2d2k(k−1)=d2. | (2.34) |
Moreover, since β≥1+3μ+1 and r∈(0,1], we get
(r2+1r2+2β)μ+1≤18, |
and consequently, for all z∈D, we obtain
|Q′μ,β(r;z)−1|<1. | (2.35) |
Finally, with the means of Lemma 1.6, we conclude that the function Qμ,β(r;z) is convex in D12.
If we take β=2 in Theorem 2.15, in view of (1.4), the following result holds true:
Corollary 2.25. Let 0<r≤1. If μ≥2, then the function Qμ(r;z) is convex in D12.
If we let μ=1 in Theorem 2.15, in view of (1.5), we derive the following result:
Corollary 2.26. Let 0<r≤1. If β≥52, then the function Q1,β(r;z) is convex in D12.
Example 2.27. The functions Q2(r;z) and Q1,52(r;z) are convex in D12.
In Figure 4, we present the mappings of these examples in D.
Remark 2.28. The geometric properties of the function Qμ(r;z) derived in Corollaries 2.16, 2.22 and 2.25 are new.
In our present paper, we have derived sufficient conditions such that a class of functions associated to the generalized Mathieu type power series are to be starlike, close-to-convex and convex in the unit disk D. The various results, which we have established in this paper, are believed to be new, and their importance is illustrated by several interesting corollaries and examples. Furthermore, we are confident that our paper will inspire further investigation in this field and pave the way for some developments in the study of geometric functions theory involving certain classes of functions related to the Mathieu type powers series.
The authors declare they have not used Artificial Intelligence (AI) tools in the creation of this article.
The authors extend their appreciation to the Deanship of Scientific Research at Northern Border University, Arar, KSA for funding this research work through the project number "NBU-FFR-2023-0093".
The authors declare that they have no conflicts of interest.
[1] |
D. F. Andrews, C. L. Mallows, Scale mixtures of normal distribution, J. R. Stat. Soc. Ser. B Methodol., 36 (1974), 99–102. https://doi.org/10.1111/j.2517-6161.1974.tb00989.x doi: 10.1111/j.2517-6161.1974.tb00989.x
![]() |
[2] |
M. West, On scale mixtures of normal distributions, Biometrika, 74 (1987), 646–648. https://doi.org/10.1093/biomet/74.3.646 doi: 10.1093/biomet/74.3.646
![]() |
[3] |
M. D. Branco, D. K. Dey, A general class of multivariate skew-elliptical distributions, J. Multivar. Anal., 79 (2001), 99–113. https://doi.org/10.1006/jmva.2000.1960 doi: 10.1006/jmva.2000.1960
![]() |
[4] |
C. S. Ferreira, H. Bolfarine, V. H. Lachos, Linear mixed models based on skew scale mixtures of normal distribution, Commun. Stat. Simul. Comput., 51 (2020), 7194–7214. https://doi.org/10.1080/03610918.2020.1827265 doi: 10.1080/03610918.2020.1827265
![]() |
[5] |
R. M. Basso, V. H. Lachos, C. R. B. Cabral, P. Ghosh, Robust mixture modeling based on scale mixtures of skew-normal distributions, Comput. Stat. Data Anal., 54 (2010), 2926–2941. https://doi.org/10.1016/j.csda.2009.09.031 doi: 10.1016/j.csda.2009.09.031
![]() |
[6] |
H. M. Kim, M. G. Genton, Characteristic functions of scale mixtures of multivariate skew-normal distributions, Comput. Stat. Data Anal., 102 (2011), 1105–1117. https://doi.org/10.1016/j.jmva.2011.03.004 doi: 10.1016/j.jmva.2011.03.004
![]() |
[7] |
T. I. Lin, J. C. Lee, W. J. Hsieh, Robust mixture modeling using the skew t distribution, Stat. Comput., 17 (2007), 81–92. https://doi.org/10.1007/s11222-006-9005-8 doi: 10.1007/s11222-006-9005-8
![]() |
[8] |
T. I. Lin, H. J. Ho, C. R. Lee, Flexible mixture modelling using the multivariate skew-t-normal distribution, Stat. Comput., 24 (2014), 531–546. https://doi.org/10.1007/s11222-013-9386-4 doi: 10.1007/s11222-013-9386-4
![]() |
[9] |
I. Lin, J. C. Lee, Y. Y. Shu, Finite mixture modelling using the skew normal distribution, Stat. Sin., 17 (2007), 909–927. https://doi.org/10.2307/24307705 doi: 10.2307/24307705
![]() |
[10] |
A. Mahdavi, V. Amirzadeh, A. Jamalizadeh, T. I. Lin, Maximum likelihood estimation for scale-shape mixtures of flexible generalized skew normal distributions via selection representation, Comput. Stat., 36 (2021), 2201–2230. https://doi.org/10.1007/s00180-021-01079-2 doi: 10.1007/s00180-021-01079-2
![]() |
[11] | A. Azzalini, A class of distributions which includes the normal ones, Scand. J. Stat., 12 (1985), 171–178. |
[12] |
A. Azzalini, A. Dalla Valle, The multivariate skew-normal distribution, Biometrika, 83 (1996), 715–726. https://doi.org/10.1093/biomet/83.4.715 doi: 10.1093/biomet/83.4.715
![]() |
[13] |
A. Azzalini, A. Capitanio, Statistical applications of the multivariate skew normal distribution, J. R. Stat. Soc. Ser. B Methodol., 61 (1999), 579–602. https://doi.org/10.1111/1467-9868.00194 doi: 10.1111/1467-9868.00194
![]() |
[14] |
R. B. Arellano-Valle, A. Azzalini, On the unification of families of skew-normal distributions, Scand. J. Stat., 33 (2006), 561–574. https://doi.org/10.1111/j.1467-9469.2006.00503.x doi: 10.1111/j.1467-9469.2006.00503.x
![]() |
[15] |
R. B. Arellano-Valle, M. G. Genton, On fundamental skew distributions, J. Multivar. Anal., 96 (2005), 93–116. https://doi.org/10.1016/j.jmva.2004.10.002 doi: 10.1016/j.jmva.2004.10.002
![]() |
[16] |
A. Azzalini, The skew-normal distribution and related multivariate families, Scand. J. Stat., 32 (2005), 159–188. https://doi.org/10.1111/j.1467-9469.2005.00426.x doi: 10.1111/j.1467-9469.2005.00426.x
![]() |
[17] |
C. Fernandez, M. F. J. Steel, Reference priors for non-normal two-sample problems, Test, 7 (1988), 179–205. https://doi.org/10.1007/BF02565109 doi: 10.1007/BF02565109
![]() |
[18] |
D. M. Zhu, V. Zinde-Walsh, Properties and estimation of asymmetric exponential power distribution, J. Econom., 148 (2009), 86–99. https://doi.org/10.1016/j.jeconom.2008.09.038 doi: 10.1016/j.jeconom.2008.09.038
![]() |
[19] |
R. J. Guan, X. Zhao, C. H. Cheng, Y. H. Rong, A new generalized t distribution based on a distribution construction method, Mathematics, 9 (2021), 2413. https://doi.org/10.1016/10.3390/math9192413 doi: 10.1016/10.3390/math9192413
![]() |
[20] | H. Exton, Handbook of hypergeometric integrals: Theory, applications, tables, computer programs, New York: Halsted Press, 1978. |
[21] |
A. P. Dempster, N. M. Laird, D. B. Rubin, Maximum likelihood from incomplete data via the em algorithm, J. R. Stat. Soc. Ser. B Methodol., 39 (1977), 1–22. https://doi.org/10.1111/j.2517-6161.1977.tb01600.x doi: 10.1111/j.2517-6161.1977.tb01600.x
![]() |
[22] | K. Lange, The EM algorithm, New York: Springer, 2013. |
[23] |
X. L. Meng, D. B. Rubin, Maximum likelihood estimation via the ecm algorithm: A general framework, Biometrika, 80 (1993), 267–278. https://doi.org/10.2307/2337198 doi: 10.2307/2337198
![]() |
[24] | P. Huber, Robust statistics, New York: Wiley, 1981. |
[25] |
L. L. Wen, Y. J. Qiu, M. H. Wang, J. L. Yin, P. Y. Chen, Numerical characteristics and parameter estimation of finite mixed generalized normal distribution, Commun. Stat. Simul. Comput., 51 (2022), 3596–3620. https://doi.org/10.1080/03610918.2020.1720733 doi: 10.1080/03610918.2020.1720733
![]() |