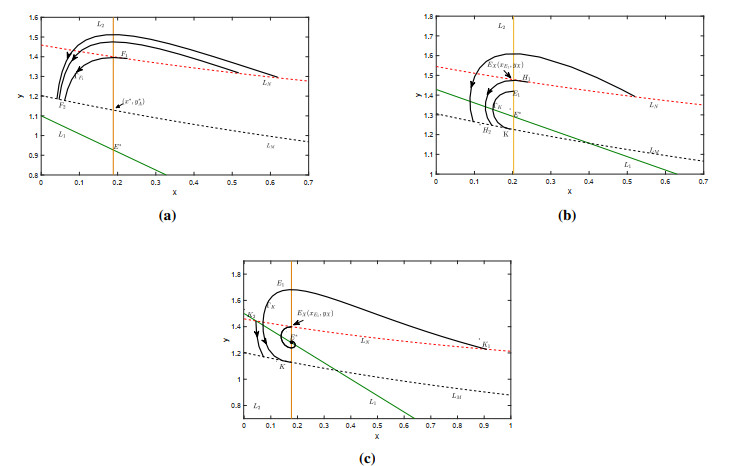
The picture fuzzy soft set (PiFSS) is a new hybrid model to address complex and uncertain information in Industry 4.0. Topological structure on PiFSS develops an innovative approach for topological data analysis to seek an optimal and unanimous decision in decision-making processes. This conception combines the advantages of a picture fuzzy set (PiFS) and a soft set (SS), allowing for a more comprehensive representation of the ambiguity in the supplier selection. Moreover, the criteria importance through intercriteria correlation (CRITIC) and the combined compromise solution (CoCoSo) technique is applied to the proposed framework to determine the relative importance of the evaluation parameter and to select the most suitable supplier in the context of sustainable development. The suggested technique was implemented and evaluated by applying it to a manufacturing company as a case study. The outcomes reveal that the approach is practical, efficient and produces favorable results when used for decision-making purposes. Evaluating and ranking of efficient suppliers based on their sustainability performance can be effectively accomplished through the use of PiFS-topology, thus facilitating the decision-making process in the CE and Industry 4.0 era.
Citation: Ayesha Razzaq, Muhammad Riaz, Muhammad Aslam. Efficient picture fuzzy soft CRITIC-CoCoSo framework for supplier selection under uncertainties in Industry 4.0[J]. AIMS Mathematics, 2024, 9(1): 665-701. doi: 10.3934/math.2024035
[1] | Yan-Fei Yang, Chun-Lei Tang . Positive and sign-changing solutions for Kirchhoff equations with indefinite potential. Communications in Analysis and Mechanics, 2025, 17(1): 159-187. doi: 10.3934/cam.2025008 |
[2] | Chen Yang, Chun-Lei Tang . Sign-changing solutions for the Schrödinger-Poisson system with concave-convex nonlinearities in R3. Communications in Analysis and Mechanics, 2023, 15(4): 638-657. doi: 10.3934/cam.2023032 |
[3] | Rui Sun, Weihua Deng . A generalized time fractional Schrödinger equation with signed potential. Communications in Analysis and Mechanics, 2024, 16(2): 262-277. doi: 10.3934/cam.2024012 |
[4] | Yonghang Chang, Menglan Liao . Nonexistence of asymptotically free solutions for nonlinear Schrödinger system. Communications in Analysis and Mechanics, 2024, 16(2): 293-306. doi: 10.3934/cam.2024014 |
[5] | Fangyuan Dong . Multiple positive solutions for the logarithmic Schrödinger equation with a Coulomb potential. Communications in Analysis and Mechanics, 2024, 16(3): 487-508. doi: 10.3934/cam.2024023 |
[6] | Shengbing Deng, Qiaoran Wu . Existence of normalized solutions for the Schrödinger equation. Communications in Analysis and Mechanics, 2023, 15(3): 575-585. doi: 10.3934/cam.2023028 |
[7] | Wenqian Lv . Ground states of a Kirchhoff equation with the potential on the lattice graphs. Communications in Analysis and Mechanics, 2023, 15(4): 792-810. doi: 10.3934/cam.2023038 |
[8] | Maomao Wu, Haidong Liu . Multiple solutions for quasi-linear elliptic equations with Berestycki-Lions type nonlinearity. Communications in Analysis and Mechanics, 2024, 16(2): 334-344. doi: 10.3934/cam.2024016 |
[9] | Xueqi Sun, Yongqiang Fu, Sihua Liang . Normalized solutions for pseudo-relativistic Schrödinger equations. Communications in Analysis and Mechanics, 2024, 16(1): 217-236. doi: 10.3934/cam.2024010 |
[10] | Huiyang Xu . Existence and blow-up of solutions for finitely degenerate semilinear parabolic equations with singular potentials. Communications in Analysis and Mechanics, 2023, 15(2): 132-161. doi: 10.3934/cam.2023008 |
The picture fuzzy soft set (PiFSS) is a new hybrid model to address complex and uncertain information in Industry 4.0. Topological structure on PiFSS develops an innovative approach for topological data analysis to seek an optimal and unanimous decision in decision-making processes. This conception combines the advantages of a picture fuzzy set (PiFS) and a soft set (SS), allowing for a more comprehensive representation of the ambiguity in the supplier selection. Moreover, the criteria importance through intercriteria correlation (CRITIC) and the combined compromise solution (CoCoSo) technique is applied to the proposed framework to determine the relative importance of the evaluation parameter and to select the most suitable supplier in the context of sustainable development. The suggested technique was implemented and evaluated by applying it to a manufacturing company as a case study. The outcomes reveal that the approach is practical, efficient and produces favorable results when used for decision-making purposes. Evaluating and ranking of efficient suppliers based on their sustainability performance can be effectively accomplished through the use of PiFS-topology, thus facilitating the decision-making process in the CE and Industry 4.0 era.
The relationship between predator and prey is the most common biological system in nature. Its basic mathematical model is the Lotka-Volterra system, which is an ordinary differential equation system proposed by mathematicians Lotka and Volterra in the 1920s. By building on its basic idea, Lotka-Volterra systems have been extensively studied [1,2,3,4,5]. It has been applied to model various phenomena, including predator harvesting, impulse control strategies, anti-predatory behavior, spatiotemporal patterns, the fear effect [6,7,8,9,10,11,12].
Predator-prey systems in nature can be associated with a variety of different factors that can cause dramatic predator or prey populations change to undergo changes. In this study, we investigate the effect of impulsive control strategies on predator-prey relationships by employing the Lotka-Volterra model. Two types of impulsive control, i.e., periodic impulse and state-dependent impulse, have been widely studied in literature [13,14,15]. The periodic impulsive control strategy is to harvest or capture the predators or prey periodically, which can be modeled by using fixed-time impulsive differential equations [16,17,18]. A disadvantage of the periodic pulse control strategy is that the timing of the pulses does not take into account the actual changing population density. Therefore, the state-dependent impulsive control strategy has been introduced, which can be modeled by using state-dependent differential equations [19,20,21]. In nature, there exists the phenomenon of a high population density but slow growth and low population density but fast growth. Therefore, scholars are now paying more attention to state-dependent impulsive differential equations with nonlinear threshold control [20,22,23,24].
State-dependent impulsive differential equations with nonlinear threshold control have been widely applied to analyze real-life problems, including modeling the population dynamics [25,26], tumor control [27,28], and viral dynamics [29,30]. Meanwhile, the theoretical development of the state-dependent impulsive differential equations has continued to advance, particularly in the realms of the invariant and limit sets [31,32] and the Poincaré-Bendixson theorem [31]. Tang and Cheke[14] obtained the maximum invariant set of the system by using a Lyapunov function, and they studied the properties of the order-1 periodic solution and sufficient conditions for stability. Bonotto and Federson [31] studied the biological phenomena of predator-prey systems by using the Poincaré-Bendixson theorem.
In 2005, Tang and Cheke[14] studied the following impulse differential equation with a fixed threshold:
{dx(t)dt=rx(t)−βx(t)y(t),dy(t)dt=ηβx(t)y(t)−δy(t),}x(t)<ET,x(t+)=(1−p)x(t),y(t+)=y(t)+τ,}x(t)=ET, | (1.1) |
x(t) and y(t) denote the prey (pest) and predator (natural enemy) densities, respectively. r represents the inherent growth rate of the prey, β represents the capturing rate, η denotes the effective conversion rate of natural enemies, δ indicates the mortality rate of natural enemies, 1−p denotes the survival rate of pests, and τ denotes the total number of predators released at regular intervals. ET is a positive number and the threshold for impulse control. In [14], the scholars discuss the existence of periodic solutions of order 1 or 2 for the system (1.1) and show that with the exception of a special case, there are no periodic solutions of order greater than order 2. Zhang and Tang [19] in 2021 considered more realistic factors by replacing ET in system (1.1) with the action threshold AT and investigated the stability and existence of the order-1 periodic solution. Further, Tian and Tang[21] studied a predator-prey system with nonlinear impulse functions and nonlinear threshold control and discussed the existence and stability of the order-1 periodic solution. It is worth noting that the order 1 periodic solution is very significant for studies on controlling policy decisions. It ensures that, when the control measures are implemented, they are able to maintain the population of the prey or predators below a given AT level.
In a number of studies, scholars have focused on modeling the anti-predatory behavior in nature, where prey can attack predators, i.e., the prey population indirectly reduces the predator population [33,34]. Anti-predatory behavior is typical; for example, the adult prey can kill juvenile predators or eat them; examples include crustaceans and sea snails, predatory and herbivorous mites, and 'predatory' mites and thrips 'prey' [35,36]. Mathematically, a predator-prey model with anti-predatory behavior was proposed by Tang and Xiao [37], in which adult prey can attack immature predators and cause harm to the predators. Tian and Tang [21] also studied the effect of anti-predatory behavior on the changes in the system.
Kent et al. [38] and van Voorn et al. [39] obtained the effect of predators on the predator-prey system. The results showed that the invasion of predators into a prey network can fail and lead to the collapse of the system or maintain the coexistence of the prey and predator populations. In other words, predator invasion can alter the dynamic behavior of the system. Threshold control for the number of predators was considered in the predator-prey system proposed by Wei and Chen [40]. The authors considered the situation whereby the number of predators could be detected, and the population in the system was controlled through harvesting when the predator density reached a fixed threshold. The unique order-1 periodic solution was derived.
According to the existing literature, few scholars have studied state-dependent models from the perspective of the predators. However, from the above discussion, we can get that the influence of predators on population relations is also crucial. Therefore, in this paper, we apply the action threshold, which is closely related to the size of the predator population and the rate of continuous predator development. The influence of the threshold change on the system and the resulting complex biodynamics are studied.
This study applied the assumption that the predator population size is large and detectable. The decrease in predator density is assumed to be caused by anti-predatory behavior. The control strategy is implemented by releasing a certain number of predators that capture the corresponding prey when the predator density and rate of change reach given values. Therefore, a predator-prey system that integrates anti-predatory behavior and the nonlinear threshold is proposed; the threshold control of the predator-prey system is modeled by a nonlinear function of the predator density and rate of change. We explore the effects of predator density and rate of change threshold control on the system and discuss how to achieve economic benefits on the basis of the proposed predator-prey system.
The paper is organized as follows. In Section 2, a predator-prey model with anti-predator behavior is proposed, and the nonlinear threshold control depends on the number and growth rate of predators. The situation without impulsive control is discussed. In Section 3, the exact impulse set and the exact phase set of the model are obtained and the expressions of the Poincaré map are defined. The monotonicity of the Poincaré map and the extreme value points are analyzed in Section 4. In Section 5, we work on analyzing the nature of the periodic solutions to the model. The parameters are given values in Section 6, and the results show that the predator-prey system can demonstrate several interesting phenomena. Finally, we conclude the article with a summary of the content.
According to the above discussion, we present a nonlinear state-dependent feedback control model with an action threshold based on [21]:
{dx(t)dt=rx(t)(1−x(t)K)−βx(t)y(t),dy(t)dt=(ηβ−ε)x(t)y(t)−δy(t),}α1y(t)+α2dy(t)dt>ET,x(t+)=x(t)−σx2(t)x(t)+μ,y(t+)=y(t)+τ1+ωy(t),}α1y(t)+α2dy(t)dt=ET. | (2.1) |
Let us represent by x(t) and y(t) the densities of prey (pest) and predator (natural enemy), respectively. r denotes the intrinsic velocity of the growing prey, the carrying capacity of the environment is denoted by K, β represents the efficiency of prey capture by the predators, η denotes the effective conversion rate of the predators, εx(t)y(t) represents anti-predatory behavior (satisfying the restriction ηβ−ε>0), and δ represents the mortality rate of the predators. The parameters α1, α2 and ET are all positive numbers satisfying that α1+α2=1. Let ET be a threshold value such that if the density of the predator population and its speed of growth are equal to or below this value, impulse control is applied to the system immediately. In system (2.1) the carrying capacity of the environment is involved, so the condition x∈[0,K],y∈[0,K] holds.
In the early studies of state-dependent impulse differential equations, the control threshold was assumed to be a fixed number of organisms in a given population. However, in terms of the actual growth of living species, the control of fixed threshold does not accurately reflect the real changes in a population. In recent years, scholars have considered an action threshold that incorporates population size and rate of change. Therefore, we consider the action threshold based on the number of predator populations and their rates of change, i.e., impulse control measures for both populations in the system when the number of predators and the rate of change reach our given threshold (α1y(t)+α2dy(t)dt=ET).
We still employ the nonlinear impulse functions associated with population density and the capture rate as follows.
ϕ=−σx2(t)x(t)+μ,φ=τ1+ωy(t). |
Here, σ and μ represent the highest prey acquisition rate and the semi-saturation parameter, respectively, τ shows the largest number of released predators, and ω is the morphological coefficient. In this study, we suppose that the density of the predator population and its rate of change satisfy the condition (α1y(t)+α2dy(t)dt>ET). When the predator population density decreases to the action threshold α1y(t)+α2dy(t)dt=ET, the prey is captured via control measures to reach x(t)−σx2(t)x(t)+μ (i.e., x(t+)) and the predator will be released to bring the density to y(t)+τ1+ωy(t) (i.e., y(t+)).
Without the feedback control, the ordinary differential equation is as follows:
{dx(t)dt=x(t)r(1−x(t)K)−βx(t)y(t),dy(t)dt=y(t)(ηβ−ε)x(t)−δy(t). | (2.2) |
The two nullclines of system (2.2) are denoted by L1 and L2, where
L1:y=rβ−rKβx,L2:x=δηβ−ε. |
Obviously, we can get three equilibrium points, which are the zero equilibrium point (0,0), boundary equilibrium point (K,0), and positive equilibrium spot E∗(x∗,y∗)=(δηβ−ε,r(K(ηβ−ε)−δ)βK(ηβ−ε)) when K>δηβ−ε. For the system (2.2) the equilibrium point is studied as shown below, which helps us to analyze the nature of the system (2.1).
Lemma 2.1. For the system (2.2), the trivial equilibrium point (0,0) is the saddle point. There is no positive equilibrium point if K<δηβ−ε and (K,0) is a globally stable node. If K>δ+√δ(δ+r)2(ηβ−ε), the point E∗(x∗,y∗) is a globally stable focus. If δηβ−ε<K<δ+√δ(δ+r)2(ηβ−ε), the equilibrium point E∗(x∗,y∗) is a globally stable node.
Proof. The Jacobian matrix of system (2.2) can be obtained as follows:
Jam(x,y)=(r(1−2xK)−βy−βx(ηβ−ε)y(ηβ−ε)x−δ). |
Substituting (0,0), (K,0) and E∗(x∗,y∗) into Jam(x,y) respectively, we get
Jam(0,0)=(r00−δ),Jam(K,0)=(−r−βK0(ηβ−ε)K−δ),Jam(x∗,y∗)=(−δrK(ηβ−ε)−δβηβ−εr(ηβK−εK−δ)Kβ0). |
From this, (0,0) is a saddle point, and the equilibrium point (K,0) is a globally stable node when K<δηβ−ε and a saddle point when K>δ+√δ(δ+r)2(ηβ−ε). The characteristic polynomial of Jam(x∗,y∗) is given by
det(λI−Jam(x∗,y∗))=λ2+(δrK(ηβ−ε))λ+(δr(ηβK−εK−δ)(ηβ−ε)K)=0, |
thus yielding the eigenvalues: λ1,2=−δrK(ηβ−δ)±√Δ02, where
Δ0=(δrK(ηβ−ε))2−4(δr(ηβK−εK−δ)(ηβ−ε)K). |
Since the real part of the eigenvalue λ1,2 is below zero, the equilibrium point E∗(x∗,y∗) is locally stable. In addition, the inequality Δ0<0 can be expressed as
K2−δKηβ−ε−δr4(ηβ−ε)2=(K−δ−√δ(δ+r)2(ηβ−ε))(K−δ+√δ(δ+r)2(ηβ−ε))>0. |
Therefore, if K>δ+√δ(δ+r)2(ηβ−ε) then Δ0<0 and K>δηβ−ε=x∗, which signifies that the equilibrium point E∗(x∗,y∗) is a focal spot. If δηβ−ε<K<δ+√δ(δ+r)2(ηβ−ε) and Δ0>0, this implies that the equilibrium point E∗(x∗,y∗) is a node. Furthermore, the equilibrium spot E∗(x∗,y∗) is also globally stable on the interval in which the system is located, as proven below:
Let
P(x,y)=xr(1−xK)−βxy,Q(x,y)=y(ηβ−ε)x−δy. |
Constructing a Dulac function B(x,y)=1xy, we have that ∂(PB)∂x+∂(QB)∂y=−ryK<0. By the Bendixson-Dulac discriminant, there is no limit cycle in the positive xy-plane, i.e., the point E∗(x∗,y∗) is globally stable. The proof is complete.
As a result, we can know that E∗ is a stable focus or node when δηβ−ε<K. When δηβ−ε>K, there is no internal equilibrium point. In our following study, we consider the case in which the equilibrium point E∗ is stable, i.e., δηβ−ε<K.
Regarding the biological significance, our study is limited to the range R2+={(x,y):x≥0,y≥0}. From α1y+α2dydt=ET and dy(t)dt=(ηβ−ε)x(t)y(t)−δy(t), we can get
y=ETα1−δα2+α2(ηβ−ε)x, |
represented by LM. By calculating the x+=x−σx2x+μ, we can get
x=x+−μ+√(μ−x+)2−4x+(1−σ)2(1−σ). |
Substituting the above equation into the equation for LM, we obtain
y=ETα1−δα2+α2(ηβ−ε)x+−μ+√(μ−x+)2−4x+(1−σ)2(1−σ). |
Let
y+=y+τ1+ωy=ψ(x+), |
which is denoted by LN. We assume that the curves LM and LN intersect the vertical coordinates in the right-angle coordinate system at the points Q1(0,Y1) and Q2(0,Y2), respectively. In the following discussion, we suppose that the starting point p+0(x+0,y+0) are on LN, unless there are special cases. Because the initial values taken are different, the trajectories from the LN may not reach the curve LM. Therefore, from the phase diagram of the system (2.1) and the relationship between the locations of the equilibrium points, we can obtain the concrete ranges of the curve LM and the curve LN (see Figure 1).
Case (A). y∗≤y∗A.
The curve L2 with the vertical coordinate of the equilibrium point E∗ intersects the impulse curve LM at the point (x∗,y∗A). Because y∗≤y∗A, there is a curve ΓF1 that is tangential to the curve LN at the point F1(xF1,yF1) and intersecting the curve LM at F2(xF2,yF2) (see Figure 1(a)). From Figure 1(a), we can get that the trace from the phase set will definitely arrive at the arrival pulse set after a certain period of time. Thus, considering this circumstance, we can obtain the concrete ranges of the impulse set and phase set as expressed below:
M1={(x,y)|0≤x≤xF2,yF2≤y≤Y1},N1={(x+,y+)|0≤x+≤xF2−σx2F2xF2+μ,yF2+τ1+ωyF2≤y+≤Y2}. |
Here (x,y) is the point in the impulse curve LM and (x+,y+) is the spot in the phase curve LN.
Case (B). y∗>y∗A.
In this case, because y∗>y∗A, there must exist a curve ΓK that is tangential to the impulse curve LM at point K(xK,yK) and intersecting the curve L2 at point E1(xE1,yE1). The curve L2, where the longitudinal coordinates of point E1(xE1,yE1) are located, intersects the phase curve LN at point EX(xE1,yX). Thus, we have the following two cases:
(B1) yE1≤yX. Since yE1≤yX, this circumstance is similar to Case (A). Under this condition, the curve ΓK has no intersection with the phase curve LN. From the phase set, all points can arrive at the pulse set. There exists a curve ΓH1 that lies tangent to the phase curve LN with the point H1(xH1,yH1) and intersects the impulse curve at the point H2(xH2,yH2) (see Figure 1(b)). Therefore, the corresponding impulse set and phase set are expressed as follows:
M2={(x,y)|0≤x≤xH2,yH2≤y≤Y1},N2={(x+,y+)|0≤x+≤xH2−σx2H2xH2+μ,yH2+τ1+ωyH2≤y+≤Y2}, |
respectively.
(B2) yE1>yX. In this case, as yE1>yX, which cause the curve ΓK to intersect the phase set at points K1(xK1,yK1) and K2(xK2,yK2) for xK2<xK1. Hence, none of the initial points on the phase curve LN from between points K1 and K2 can reach the impulse curve LM (see Figure 1(c)). From this, we can determine the scope of the impulse set and phase set as below:
M3={(x,y)|0≤x≤xK,yK≤y≤Y1},N3={(x+,y+)|x+∈[0,xK2]∪[xK1,xK−σx2KxK+μ],y+∈[yK2,Y2]∪[yK+τ1+ωyK,yK1]}, |
respectively.
From the above discussion, we can construct the following Poincaré map. Provided an initial point P+0(x+0,y+0) belonging to the phase curve LN, we can determine the path that starts from point P+0 as follows:
Γ(t,t0,P+0)=Γ(x+0(t,t0,(x+0,y+0)),y+0(t,t0,(x+0,y+0))). |
As for P+i(x+i,y+i) belonging to the phase set, point P+i arrives at the impulse set becoming point Pi+1(xi+1,yi+1) after time ˜t. The corresponding formulation is as follows:
Γ(˜t,x+i,y+i)=Γ(˜t,xi+1,yi+1)=Γ(x+(˜t,x+i,y+i),y+(˜t,x+i,y+i))=Γ(xi+1,yi+1), |
where xi+1=x+(˜t,x+i,y+i). By the Cauchy-Lipschitz theorem, we learn that xi+1 is uniquely denoted by x+. By the definition xi+1=ξ(x+i), we have
x+i+1=xi+1−σx2i+1xi+1+μ=ξ(x+i)−σξ(x+i)2ξ(x+i)+μ=EM(x+i). | (3.1) |
The following scalar differential equation for the system (2.1) is considered:
{dxdy=rx(1−xK)−βxy(ηβ−ε)xy−δyΔ=e(y,x),x(ET)=x+0. | (3.2) |
From the system (2.1) we know that the function e(y,x) is continuously differentiable. In addition, we set y+0=U,x+0=V and P+0(x+0,y+0)∈N. Let
x(y)=x(y;U,V)=x(y,V). | (3.3) |
The data variants of y are between the curve LN and the curve LM. Solving (3.2) we obtain
x(y,V)=V+∫Uye(v,x(v,V))dv. | (3.4) |
Therefore, from (3.1) and (3.4), we can get the expression for the Poincaré map:
E(V)=x(U,V)−σx2(U,V)x(U,V)+μ. | (3.5) |
In this section, we will discuss the properties of E(V).
Theorem 4.1. Assume that y∗≤y∗A. We obtain the following properties of E(V) (see Figure 2):
(i) The domain of definition of E(V) is (0,+∞). Moreover, E(V) is decreasing monotonically in the interval (0,xF1] and increasing monotonically in the interval [xF1,+∞).
(ii) E(V) is continuously differentiable in the interval (0,+∞), and it follows that there is a unique fixed point.
Proof. (ⅰ) When y∗≤y∗A, there exists a point F1(xF1,yF1)∈LN such that the curve ΓF1 is tangent to LN and ΓF1 intersects LM at the point F2(xF2,yF2). It follows that any trajectory starting from LN can reach LM after some time. Therefore, the Poincaré map is defined within the range (0,+∞). This means that E(V) is significant within (0,+∞).
Assume that there exists P+a1(x+a1,y+a1),P+a2(x+a2,y+a2)∈LN. By the dynamical behaviour of the system (2.1), at the elapsed time ˜t, we can obtain that Γ(˜t,x+i,y+i)=Γ(˜t,xi+1,yi+1). If x+a1,x+a2∈(0,xF1] and x+a1<x+a2, because of the uniqueness of the solution, we obtain that xa1+1>xa2+1. From the formulation of E(V), we get that E(xa1+1)>E(xa2+1). Therefore, E(V) is monotonically decreasing within the range (0,xF1].
When x+a1,x+a2∈[xF1,+∞) and x+a1<x+a2, the initial points P+a1 and P+a2 return to the curve LN at points P′a1(x′a1,y′a1) and P′a2(x′a2,y′a2) after a period of impulses. We easily get that x′a1<x′a2. By the uniqueness of the solution, we get that E(x′a1)<E(x′a2). Therefore, E(V) is monotonically increasing within the range [xF1,+∞).
(ⅱ) From (3.2), it is obvious that the function e(y,x) is continuously differentiable. By the theorems on continuity and differentiability for differential equations, we gain that E(V) is continuously differentiable within the range (0,+∞).
The curve ΓF1 from the point F1 reaches LM at the point F2 and is impulsed to LN at the spot F+2(x+F2,y+F2) after some more time. If E(xF1)<xF1, then x+F2=E(xF1)∈(0,xF1] and x+F2<xF1. And since E(V) is monotonically decreasing within the range (0,xF1], we have that E(x+F2)>E(xF1)=x+F2. By the existence theorem for zeros of the function E(V) and continuous differentiability, we can obtain that there exists ˉx∈(x+F2,xF1) such that E(ˉx)=ˉx. Therefore, there exists a unique immobile point of E(V) in the zone (0,+∞), as shown in Figure 2(a). The proof for the case in Figure 2(b) is obvious.
When y∗>y∗A and yE1≤yX, the characteristics of E(V) are similar to those in Case (A). For this reason, we discuss the nature of E(V) when y∗>y∗A and yE1>yX.
Theorem 4.2. When y∗>y∗A and yE1>yX, the Poincaré map E(V) possesses the following nature (see Figure 3):
(i) The domain of the definition of E(V) is (0,xK2]∪[xK1,+∞), with E(V) decreasing monotonically in the interval (0,xK2] and increasing monotonically in the interval [xK1,+∞).
(ii) E(V) is continuously differentiable in the interval (0,xK2]∪[xK1,+∞).
(iii) If E(V) satisfies that E(xK2)<xK2 at point xK2, then there is only one immobile point of E(V) in the interval (0,xK2]. However, when E(V) is at point xK1 at E(xK1)>xK1, there is no fixed point over the entire domain of the definition.
Proof. (ⅰ) When the condition yE1>yX is satisfied, the curve ΓK which is tangent to the impulse curve LM intersects with the phase curve LN at the points K1 and K2, respectively. Considering the following: ∀Pi(xi,yi)∈N, if x+i∈(xK2,xK1), none of the trajectories from the phase curve LN can arrive at the curve LM. Therefore, we denote the domain of the definition of E(V) as (0,xK2]∪[xK1,+∞).
Assume that there exists P+h1(x+h1,y+h1),P+h2(x+h2,y+h2)∈LN; if x+h1,x+h2∈[xK1,+∞), let x+h1<x+h2. From the previous discussion, we can know that the trajectory from point x+i to point xi+1 after time ˜t can be denoted as Γ(˜t,x+i,y+i)=Γ(˜t,xi+1,yi+1). By the sole nature of the solution, we obtain that xh1+1<xh2+1. Under the equation of the Poincaré map E(V), we then obtain that E(xh1+1)<E(xh2+1). As a consequence, we obtain the Poincaré map E(V) that is monotonically increasing in the interval [xK1,+∞).
When x+h1,x+h2∈(0,xK2] and x+h1<x+h2, we obtain the paths of points P+h1,P+h2 passing through the curve L2 and respectively intersecting the phase curve LN at points P′h1(x′h1,y′h1),P′h2(x′h2,y′h2) where x′h1>x′h2. Under the equation of the Poincaré map E(V), we then obtain that EM(x′h1)>EM(x′h2). As a consequence, we obtain the Poincaré map E(V) that is monotonically decreasing in the interval (0,xK2].
(ⅱ) Based on (3.2) given earlier, we can know that the function e(y,x) is continuously differentiable. By the theorems of continuity and differentiability for the differential equations, we therefore easily obtain that E(V) is continuously differentiable in the interval (0,xK2]∪[xK1,+∞).
(ⅲ) The trajectory ΓK moves from the point K2 to the impulse curve LM at the point K, and then to the phase curve LN at the point K+(x+K,y+K), according to which we have that x+K=E(xK2)∈(0,xK2] holds. Since the Poincaré map E(V) is monotonically decreasing in the interval (0,xK2], we have that E(x+K)>E(xK2)=x+K holds. If E(xK2)<xK2, then by the zero theorem there is ˉx∈(x+K,xK2) such that E(ˉx)=ˉx. Accordingly, the Poincaré map E(V) holds a uniquely fixed point in the interval (0,xK2].
If E(xK1)>xK1 holds when x=xK1, for any xi belonging to the interval [xK1,+∞), the orbit from the spot Pi(xi,yi) arrives at the impulse curve LM at the point Pi+1(xi+1,yi+1) and is then pulsed to the phase curve LN at the spot P+i+1(x+i+1,y+i+1). By the dynamical behavior of the system (2.1), we can work out that xi<xi+1<x+i+1. As a result, there is no ˉx∈[xK1,+∞) such that E(ˉx)=ˉx holds, i.e., there is no indefinite point of E(V) in the interval [xK1,+∞). The proof is completed.
From the previous discussion, we know that the system (2.1) has an order-1 periodic solution. In the following theorem, we will consider its stability and other possibilities.
Theorem 5.1. In Case (A), i.e., y∗≤y∗A, the system (2.1) has an order-1 periodic solution, and in addition, we know that
(i) if E(xF1)>xF1, the order-1 periodic solution of the system (2.1) is globally stable;
(ii) if E(xF1)<xF1, the order-1 periodic solution of the system (2.1) is globally stable if and only if E2(x+0)<x+0 for ∀x+0∈[ˉx,xF1].
Proof. From (3.1), we get that x+i+1=ξ(x+i)−σξ(x+i)2ξ(x+i)+μ=EM(x+i). Thus, the starting point P+0(x+0,y+0) from the phase curve LN arrives at the impulse curve LM at the point P1(x1,y1), and after a period of action pulses in the impulse curve LM arrives at the phase curve LN at the point P+1(x+1,y+1). This process can be expressed as E(x+0)=ξ(x+0)−σξ(x+0)2ξ(x+0)+μ=x+i. By a similar process, we obtain that E[E(x+0)]=E(x+1)=x+2=E2(x+0). In more depth, we obtain that Ek(x+0)=x+k.
(ⅰ) For Case (A), if E(xF1)>xF1 is satisfied at x=xF1, the Poincaré map has a single immovable point ˉx within the zone [xF1,+∞). Two cases are discussed according to different positional relations of x+0:
a. If x+0∈(xF1,ˉx], from the previous discussion, we know that the Poincaré map E(V) is monotonically increasing within this interval. For xF1<x+0<ˉx, we have that xF1<E(xF1)<E(x+0)<E(ˉx)=ˉx. By the monotonicity of the Poincaré map E(V), we have that E(x+0)<E(x+1)<E(ˉx)=ˉx. Repeating the above process, it can be deduced that
xF1<E(x+0)<⋯<Ek(x+0)<Ek+1(x+0)<⋯<ˉx, |
which shows that the formula Ek(x+0) is a monotonically increasing function in the interval (xF1,ˉx] and limk→∞Ek(x+0)=ˉx.
b. If x+0∈(ˉx,+∞), analogous to the discussion above, we have that ˉx=E(ˉx)<E(x+0)<x+0 and ˉx=E(ˉx)<E(x+1)<E(x+0)<x+0 is established. After that, we can get
ˉx<⋯<Ek+1(x+0)<Ek(x+0)<Ek−1(x+0)<⋯<E(x+0)<x+0. |
Therefore, we get to know that the equation for Ek(x+0) is a decreasing monotonic function in the zone (ˉx,+∞), so we have that limk→∞Ek(x+0)=ˉx. It can be seen that it is globally stable when E(xF1)>xF1.
(ⅱ) Sufficient condition: If we have that E(xF1)<xF1 at point x=xF1; we learn from Theorem 4.1 that the Poincaré map E(V) has a single immovable point ˉx within the zone (0,xF1] and is decreasing monotonically in this zone. It follows that when E2(x+0)<x+0 for any x+0∈[ˉx,xF1], there exist ˉx=E(ˉx)>E(x+0)>E(xF1) and ˉx=E(ˉx)<E2(x+0)<x+0, i.e., E(xF1)<E(x+0)<ˉx=E(ˉx)<E2(x+0)<x+0<xF1. Repeating the above process, we get
E(xF1)<⋯<E2k−1(x+0)<E2k+1(x+0)<ˉx<E2k+2(x+0)<E2k(x+0)<⋯<xF1. |
According to the monotone convergence theorem for the function, we obtain that limk→∞E2k+2(x+0)=limk→∞E2k+1(x+0)=ˉx, i.e., our theorem holds.
Necessary condition: When E(xF1)<xF1 and the order-1 periodic solution ˉx of the system (2.1) is globally stable, suppose that the existence of a point ˜x+1∈(ˉx,xF1] yields E(˜x+1)≥˜x+1. From the stability of a point ˉx, we know that there exists a point ˜x+2∈(ˉx−ε,ˉx+ε) that yields E2(˜x+2)<˜x+2 for ε sufficiently small. From the continuity of the Poincaré map E(V), we learn that there exists at least one ˉx′∈(˜x+1,˜x+2) such that E(ˉx′)=ˉx′ holds, which contradicts our previous assumptions. Consequently, if Theorem 5.1 holds, E2(x+0)<x+0 holds for ∀x+0(ˉx,xF1].
Theorem 5.2. In Case (B), the order-1 periodic solution of the system (2.1) is globally stable when E(xK2)<xK2 and E2(x+0)<x+0 for ∀x+0∈(ˉx,xK2].
Proof. In accordance with Theorem 4.2, we get that when E(xK1)>xK1, the Poincaré map E(V) has no fixed point in the domain of the definition, and when E(xK2)<xK2, there is a unique fixed point, so the fixed point ˉx∈(0,xK2]. By the previous discussion, the Poincaré map E(V) is monotonically decreasing in the interval (0,xK2] and Ek(x+0)=x+k. For any x+0∈(ˉx,xK2], i.e., xK2≥x+0>ˉx, it follows that E(xK2)≤E(x+0)=x+1<E(ˉx)=ˉx holds. If E2(x+0)<x+0, then we have that xK2>x+0>x+2>ˉx. By further computation, we have
xK2>x+0>x+2>⋯>x+2k−2>x+2k>ˉx>x+2k+1>x+2k−1>⋯>x+3>x+1≥E(xK2). |
Thus, we get that limk→∞x+2k=limk→∞x+2k+1=ˉx. This means that Theorem 5.2 holds if E(xK2)<xK2 and E2(x+0)<x+0 for ∀x+0∈(ˉx,xK2].
Theorem 5.3. In Case (A), if E(xF1)<xF1 and E2(xF1)<xF1, the system (2.1) has a stable order-1 or order-2 periodic solution.
Proof. For any one point P+0(x+0,y+0)∈LN, where x+0>0 and y+0>0, from the previous discussion, we have that Ek(x+0)=x+k. According to Theorem 4.1, we obtain the Poincaré map E(V) that is increasing monotonically in the region [xF1,+∞) and without fixed points. So, there is a point q such that x+q<xF1 and x+q−1>xF1; then, x+q=E(x+q−1)>E(xF1), i.e., E(xF1)<x+q<xF1. By the monotonicity of the Poincaré mapping and condition E2(xF1)<xF1, we have that E[E(xF1),xF1]=[E(xF1),E2(xF1)]⊂[E(xF1),xF1]. So we just focus on the periodic solutions for the interval [E(xF1),xF1]. Let E(x+0)=x+1≠x+0 and E2(x+0)=x+2≠x+0, which can be used to obtain the system (2.1) with order-1 or order-2 periodic solution. Depending on the placement of the initial point, we discuss the next four cases:
(ⅰ) xF1≥x+2>x+0>x+1≥E(xF1), i.e., xF1≥E2(x+0)>x+0>E(x+0)≥E(xF1). By the monotonicity of the Poincaré map E(V), we have that x+3=E3(x+0)<E(x+0)=x+1 and x+4=E4(x+0)>E2(x+0)=x+2, i.e., xF1≥x+4>x+2>x+0>x+1>x+3>E(xF1). Applying mathematical induction and repeating the above process, we can obtain
xF1≥x+2k+2>x+2k>⋯>x+4>x+2>x+0>x+1>x+3>⋯>x+2k−1>x+2k+1≥E(xF1). |
(ⅱ) xF1≥x+0>x+2>x+1≥E(xF1), then xF1≥x+0>E2(x+0)>E(x+0)≥E(xF1). By the monotonicity of the Poincaré map E(V), we have that x+1=E(x+0)<E3(x+0)=x+3 and x+2=E2(x+0)>E4(x+0)=x+4, i.e., xF1≥x+0>x+2>x+4>x+3>x+1≥E(xF1). Furthermore, we get
xF1≥x+0>x+2>x+4>⋯>x+2k>x+2k+2>⋯>x+2k+1>x+2k−1>⋯>x+3>x+1≥E(xF1). |
(ⅲ) xF1≥x+1>x+2>x+0≥E(xF1). Analogous to the previous discussion, we have
xF1≥x+1>x+3>⋯>x+2k−1>x+2k+1>⋯>x+2k+2>x+2k>⋯>x+4>x+2>x+0≥E(xF1). |
(ⅳ) xF1≥x+1>x+0>x+2≥E(xF1). By a similar discussion we have
xF1≥x+2k+1>x+2k−1>⋯>x+3>x+1>x+0>x+2>x+4>⋯>x+2k>x+2k+2>⋯≥E(xF1). |
Accordingly, in Cases (ⅱ) and (ⅲ), we have that ˉx∈(E(xF1),xF1); thus, limk→∞x+2k+1=limk→∞x+2k+2=ˉx, i.e., there is a stable order-1 periodic solution for the system (2.1).
For Cases (ⅰ) and (ⅳ), there exists ˉx1,ˉx2∈(E(xF1),xF1) such that limk→∞x+2k+1=ˉx1 and limk→∞x+2k+2=ˉx2, where ˉx1≠ˉx2. This implies that in both cases, the predator-prey system has a stable order-2 periodic solution. There is no order-k (k>2) periodic solution for the system (2.1) [14].
In this paragraph, we assign some values to the parameters to verify the theoretical consequences given earlier and analyze some dynamic behavior of the system (2.1).
We set r=1.1,β=1.2,η=0.63, ε=0.1,δ=0.1, K=1.2,σ=0.2,μ=3, τ=1.8,ω=5. When the parameters α1=1,α2=0, the impulse line and the phase line become two direct lines that run parallel to the horizontal coordinate of the coordinate axis, which is comparable to the results on many of our prior studies (Figure 4(a)). For this situation, the threshold is controlled only by dependence on the size of the predator population density. When we modify the weighting factor for the action threshold, we can observe that the slope of the impulse and phase lines changes (Figure 4(b), (c)). On the other hand, under the control of an action threshold with predator population density and its rate of change, the system (2.1) can still be obtained with a stable order-1 periodic solution.
For Case (A), we set r=1.1,β=1.23,η=0.72,ε=0.1,δ=0.1,K=1.2,AT=0.55,σ=0.2,μ=3,τ=1.8,ω=5. By Theorem 5.1, the system (2.1) has a stable order-1 periodic solution when y∗≤y∗A. We can look at Figure 5, where Figure 5(a) indicates that the system (2.1) has an order-1 stable periodic solution, and Figure 5(b), (c) indicate the time series plots for the prey and predator, respectively. For Case (B)(B2), let r=1.8,β=1.26,η=0.63,ε=0.3,δ=0.1,K=1.2,ET=0.7,σ=0.2,μ=3,τ=1.8,ω=5. When E(xK2)<xK2, we can know that the system (2.1) has a stable order-1 periodic solution, as shown in Figure 6. When E(xK1)>xK1, i.e., r=1.1,β=1,η=0.72,σ=0.5,μ=0.8, we can observe Figure 7 to obtain that after impulse control, the system (2.1) has no periodic solution, but the system solution stabilizes at a fixed value.
The trajectories of the system (2.1) obtained for various starting points in Cases (A) and (B)(B2) are shown in Figures 8 and 9, respectively. We can ascertain from Figures 8 and 9 that the trajectories from various starting spots converge to a stable order-1 periodic solution. In Figure 10, we let r=1.7,β=1.2,η=0.63,ε=0.3,δ=0.1,K=8,ET=0.7,σ=0.2,μ=3,τ=1.8,ω=5. At this point, we can ascertain from Figure 10 that system (2.1) has no periodic solution, but the trajectories from various starting points eventually converge to the same point and stabilize at that point.
The state dependent impulsive semi-dynamical system, among non-smooth systems is one of the most discontinuous type of system; it has been studied in many fields, such as cancer and diabetes treatment, neuronal dynamics, HIV and infectious disease control, integrated pest management, neuronal dynamics, etc. In recent years, research on such systems has made substantial progress in terms of application, qualitative analysis, and analytical techniques. In this study, we have modeled predator-prey state impulse feedback control based on predator density and the rate of change, taking into account the capture of prey and release of predators, where the control point depends on whether the weights of the predator number and its growth rate reach ET. We have studied the dynamical behavior of the model comprehensively and analyzed the biological significance of the system (2.1).
First, the model was introduced and the significance of each parameter in the model was analyzed. The existence condition and stability of the equilibrium point of the model without impulse have been proved by applying the Jacobian matrix and Bendixson-Dulac Discriminative theorem. Second, the model of E(V) was defined and the main properties of E(V) were studied. Moreover, we discussed the existence and stability conditions of the periodic solutions. Finally, the theoretical results were verified through the use of numerical examples.
In this study, the monotonicity, continuity, immobility points, and polar values of system (2.1) were studied by using a Poincaré map. We have demonstrated the existence and stability of an order-1 periodic solution in the system (2.1). According to Figures 5–9, we find that the prey population density and predator population density remain in periodic oscillation. Although there is no periodic solution in Figure 10, we can see that the points originating from different initial points eventually converge to the same value. Therefore, under the control of the action threshold in this study, using the method of Poincaré map, and after implementing effective impulse control, we can get that the prey and predator populations maintain periodic oscillations, i.e., they are stable within a small interval, which means that the biological populations have reached a state of coexistence. This is of ecological and economic significance for those who study control strategies for predator-prey systems.
The novelty of this study is the use of an action threshold, which is a function of the predator population number and the current rate of predator development. The fixed threshold is a special case of the dynamic threshold, where the dynamic threshold is an extension of the fixed threshold. Under this action threshold, we use the state feedback control strategy of increasing the predator and capturing the prey when the predator density and rate of change decrease to a certain amount. The control methods and control models used in this study are more general, which allows the models to demonstrate more complex dynamical behaviors that are more consistent with the development of biological populations.
In the future, we will focus on the effects of parameter changes on the predator and prey system and on studying the mutual restrictions between populations.
The authors declare they have not used Artificial Intelligence (AI) tools in the creation of this article.
The authors would like to thank the editor and anonymous referees for their valuable comments and suggestions which have led to improvement of the paper.
This study was partially supported by the National Natural Science Foundation of China (Grant Nos. 12261033).
The authors declare that they have no conflict of interest.
[1] |
L. A. Zadeh, The concept of a linguistic variable and its application to approximate reasoning-I, Inf. Sci., 8 (1975), 199–249. https://doi.org/10.1016/0020-0255(75)90036-5 doi: 10.1016/0020-0255(75)90036-5
![]() |
[2] |
K. T. Atanassov, Intuitionistic fuzzy sets, Fuzzy Set. Syst., 20 (1986), 87–96. https://doi.org/10.1016/S0165-0114(86)80034-3 doi: 10.1016/S0165-0114(86)80034-3
![]() |
[3] | R. R. Yager, Pythagorean fuzzy subsets, 2013 joint IFSA world congress and NAFIPS annual meeting (IFSA/NAFIPS), 2013, 57–61. https://doi.org/10.1109/IFSA-NAFIPS.2013.6608375 |
[4] |
R. R. Yager, Generalized orthopair fuzzy sets, IEEE T. Fuzzy Syst., 25 (2017), 1222–1230. https://doi.org/10.1109/TFUZZ.2016.2604005 doi: 10.1109/TFUZZ.2016.2604005
![]() |
[5] |
I. Deli, N. Cagman, Intuitionistic fuzzy parameterized soft set theory and its decision making, Appl. Soft Comput., 28 (2015), 109–113. https://doi.org/10.1016/j.asoc.2014.11.053 doi: 10.1016/j.asoc.2014.11.053
![]() |
[6] |
T. M. Al-shami, A. Mhemdi, Generalized frame for orthopair fuzzy sets: (m, n)-Fuzzy sets and their applications to multi-criteria decision-making methods, Information, 14 (2023), 56. https://doi.org/10.3390/info14010056 doi: 10.3390/info14010056
![]() |
[7] |
T. M. Al-shami, J. C. R. Alcantud, A. Mhemdi, New generalization of fuzzy soft sets: (a, b)-Fuzzy soft sets, AIMS Mathematics, 8 (2023), 2995–3025. https://doi.org/10.3934/math.2023155 doi: 10.3934/math.2023155
![]() |
[8] |
B. C. Cuong, V. Kreinovich, Picture fuzzy sets, J. Comput. Sci. Cybern., 30 (2014), 409–420. https://doi.org/10.15625/1813-9663/30/4/5032 doi: 10.15625/1813-9663/30/4/5032
![]() |
[9] |
Y. Yang, C. Liang, S. Ji, T. Liu, Adjustable soft discernibility matrix based on picture fuzzy soft sets and its applications in decision making, J. Intell. Fuzzy Syst., 29 (2015), 1711–1722. https://doi.org/10.3233/IFS-151648 doi: 10.3233/IFS-151648
![]() |
[10] | B. C. Cuong, V. H. Pham, Some fuzzy logic operators for picture fuzzy sets, 2015 seventh international conference on knowledge and systems engineering (KSE), 2015,132–137. https://doi.org/10.1109/KSE.2015.20 |
[11] |
P. H. Thong, S. L. Hoang, A novel automatic picture fuzzy clustering method based on particle swarm optimization and picture composite cardinality, Knowl.-Based Syst., 109 (2016), 48–60. https://doi.org/10.1016/j.knosys.2016.06.023 doi: 10.1016/j.knosys.2016.06.023
![]() |
[12] | F. Smarandache, Neutrosophy: Neutrosophic Probability, Set, and Logic: Analytic Synthesis & Synthetic Analysis, American Research Press: Champaign, 1998. |
[13] |
T. Mahmood, K. Ullah, Q. Khan, N. Jan, An Approach towards decision making and medical diagnosis problems using the concept of spherical fuzzy sets, Neural Comput. Appl., 31 (2019), 7041–7053. https://doi.org/10.1007/s00521-018-3521-2 doi: 10.1007/s00521-018-3521-2
![]() |
[14] |
S. Ashraf, S. Abdullah, T. Mahmood, F. Ghani, T. Mahmood, Spherical fuzzy sets and their applications in multi-attribute decision making problems, J. Intell. Fuzzy Syst., 36 (2019), 2829–2844. https://doi.org/10.3233/JIFS-172009 doi: 10.3233/JIFS-172009
![]() |
[15] |
F. K. Gündogdu, C. Kahraman, Spherical fuzzy sets and spherical fuzzy TOPSIS method, J. Intell. Fuzzy Syst, 36 (2018), 337–352. https://doi.org/10.3233/JIFS-181401 doi: 10.3233/JIFS-181401
![]() |
[16] |
M. Akram, U. Ahmad, Rukhsar, Threshold graphs under picture Dombi fuzzy information, Granular Comput., 7 (2022), 691–707. https://doi.org/10.1007/s41066-021-00291-1 doi: 10.1007/s41066-021-00291-1
![]() |
[17] |
M. Akram, A. Khan, U. Ahmad, Extended MULTIMOORA method based on 2-tuple linguistic Pythagorean fuzzy sets for multi-attribute group decision-making, Granular Comput., 8 (2023), 311–332. https://doi.org/10.1007/s41066-022-00330-5 doi: 10.1007/s41066-022-00330-5
![]() |
[18] |
C. L. Chang, Fuzzy topological spaces, J. Math. Anal. Appl., 24 (1968), 182–190. https://doi.org/10.1016/0022-247X(68)90057-7 doi: 10.1016/0022-247X(68)90057-7
![]() |
[19] |
D. Çoker, An introduction to intuitionistic fuzzy topological spaces, Fuzzy Sets Syst., 88 (1997), 81–89. https://doi.org/10.1016/S0165-0114(96)00076-0 doi: 10.1016/S0165-0114(96)00076-0
![]() |
[20] |
M. Shabir, M. Naz, On soft topological spaces, Comput. Math. Appl., 61 (2011), 1786–1799. https://doi.org/10.1016/j.camwa.2011.02.006 doi: 10.1016/j.camwa.2011.02.006
![]() |
[21] |
B. Tanay, M. B. Kandemir, Topological structure of fuzzy soft sets, Comput. Math. Appl., 61 (2011), 2952–2957. https://doi.org/10.1016/j.camwa.2011.03.056 doi: 10.1016/j.camwa.2011.03.056
![]() |
[22] |
A. Razzaq, M. Riaz, M-parameterized N-soft set-based aggregation operators for multi-attribute decision making, Soft Comput., 27 (2023), 13701–13717. https://doi.org/10.1007/s00500-023-08853-y doi: 10.1007/s00500-023-08853-y
![]() |
[23] |
M. Riaz, A. Razzaq, M. Aslam, D. Pamucar, M-parameterized N-soft topology-based TOPSIS approach for multi-attribute decision making, Symmetry, 13 (2021), 748. https://doi.org/10.3390/sym13050748 doi: 10.3390/sym13050748
![]() |
[24] |
R. Lowen, Fuzzy topological spaces and fuzzy compactness, J. Math. Anal. Appl., 56 (1976), 621–633. https://doi.org/10.1016/0022-247X(76)90029-9 doi: 10.1016/0022-247X(76)90029-9
![]() |
[25] |
R. Lowen, Convergence in fuzzy topological spaces, General Topol. Appl., 10 (1979), 147–160. https://doi.org/10.1016/0016-660X(79)90004-7 doi: 10.1016/0016-660X(79)90004-7
![]() |
[26] |
A. K. Chaudhuri, P. Das, Fuzzy connected sets in fuzzy topological spaces, Fuzzy Sets Syst., 49 (1992), 223–229. https://doi.org/10.1016/0165-0114(92)90327-Z doi: 10.1016/0165-0114(92)90327-Z
![]() |
[27] |
S. Ozçag, D. Coker, On connectedness in intuitionistic fuzzy special topological spaces, Int. J. Math. Math. Sci., 21 (1998), 33–40. https://doi.org/10.1155/S0161171298000040 doi: 10.1155/S0161171298000040
![]() |
[28] |
N. Çagman, S. Karatas, S. Enginoglu, Soft topology, Comput. Math. Appl., 62 (2011), 351–358. https://doi.org/10.1016/j.camwa.2011.05.016 doi: 10.1016/j.camwa.2011.05.016
![]() |
[29] |
M. Shabir, M. Naz, On soft topological spaces, Comput. Math. Appl., 61 (2011), 1786–1799. https://doi.org/10.1016/j.camwa.2011.02.006 doi: 10.1016/j.camwa.2011.02.006
![]() |
[30] |
M. Riaz, N. Çağman, I. Zareef, M. Aslam, N-soft topology and its applications to multi-criteria group decision making, J. Intell. Fuzzy Syst., 36 (2019), 6521–6536. https://doi.org/10.3233/JIFS-182919 doi: 10.3233/JIFS-182919
![]() |
[31] |
M. Riaz, M. R. Hashmi, Fuzzy parameterized fuzzy soft topology with applications, Ann. Fuzzy Math. Inf., 13 (2017), 593–613. https://doi.org/10.30948/afmi.2017.13.5.593 doi: 10.30948/afmi.2017.13.5.593
![]() |
[32] |
M. Riaz, B. Davvaz, A. Fakhar, A. Firdous, Hesitant fuzzy soft topology and its applications to multi-attribute group decision-making, Soft Comput., 24 (2020), 16269–16289. https://doi.org/10.1007/s00500-020-04938-0 doi: 10.1007/s00500-020-04938-0
![]() |
[33] |
A. H. Es, Connectedness in Pythagorean fuzzy topological spaces, Int. J. Math. Trends Tech., 65 (2019), 110–116. https://doi.org/10.14445/22315373/IJMTT-V65I7P517 doi: 10.14445/22315373/IJMTT-V65I7P517
![]() |
[34] |
A. Razaq, I. Masmali, H. Garg, U. Shuaib, Picture fuzzy topological spaces and associated continuous functions, AIMS Mathematics, 7 (2022), 14840–14861. https://doi.org/10.3934/math.2022814 doi: 10.3934/math.2022814
![]() |
[35] |
S. Kolla, M. Minufekr, P. Plapper, Deriving essential components of lean and Industry 4.0 assessment model for manufacturing SMEs, Proc. CIRP, 81 (2019), 753–758. https://doi.org/10.1016/j.procir.2019.03.189 doi: 10.1016/j.procir.2019.03.189
![]() |
[36] |
P. Soderholm, A. K. Bergquist, K. Soderholm, Environmental regulation in the pulp and paper industry: Impacts and challenges, Curr. For. Rep., 5 (2019), 185–198. https://doi.org/10.1007/s40725-019-00097-0 doi: 10.1007/s40725-019-00097-0
![]() |
[37] |
L. S. Dalenogare, G. B. Benitez, N. F. Ayala, A. G. Frank, The expected contribution of Industry 4.0 technologies for industrial performance, Int. J. Prod. Econ., 204 (2018), 383–394. https://doi.org/10.1016/j.ijpe.2018.08.019 doi: 10.1016/j.ijpe.2018.08.019
![]() |
[38] |
M. Lezoche, J. E. Hernandez, M. D. M. E. A. Díaz, H. Panetto, J. Kacprzyk, Agri-food 4.0: A survey of the supply chains and technologies for the future agriculture, Comput. Ind., 117 (2020), 103187. https://doi.org/10.1016/j.compind.2020.103187 doi: 10.1016/j.compind.2020.103187
![]() |
[39] |
U. Ullah, F. A. Bhatti, A. R. Maud, M. I. Asim, K. Khurshid, M. Maqsood, IoT-enabled computer vision-based parts inspection system for SME 4.0, Microprocessors MicroSyst., 87 (2021), 104354. https://doi.org/10.1016/j.micpro.2021.104354 doi: 10.1016/j.micpro.2021.104354
![]() |
[40] |
T. Stock, M. Obenaus, S. Kunz, H. Kohl, Industry 4.0 as enabler for a sustainable development: A qualitative assessment of its ecological and social potential, Process Saf. Environ. Prot., 118 (2018), 254–267. https://doi.org/10.1016/j.psep.2018.06.026 doi: 10.1016/j.psep.2018.06.026
![]() |
[41] |
A. Belhadi, S. S. Kamble, C. J. C. Jabbour, V. Mani, S. A. R. Khan, F. E. Touriki, A self-assessment tool for evaluating the integration of circular economy and Industry 4.0 principles in closed-loop supply chains, Int. J. Prod. Econ., 245 (2022), 108372. https://doi.org/10.1016/j.ijpe.2021.108372 doi: 10.1016/j.ijpe.2021.108372
![]() |
[42] |
P. Schmitt, J. Schmitt, B. Engelmann, Evaluation of proceedings for SMEs to conduct I4.0 projects, Proc. CIRP, 86 (2019), 257–263. https://doi.org/10.1016/j.procir.2020.01.007 doi: 10.1016/j.procir.2020.01.007
![]() |
[43] |
S. Kumar, R. D. Raut, K. Nayal, S. Kraus, V. S. Yadav, B. E. Narkhede, To identify Industry 4.0 and circular economy adoption barriers in the agriculture supply chain by using ISM-ANP, J. Cleaner Prod., 293 (2021), 126023. https://doi.org/10.1016/j.jclepro.2021.126023 doi: 10.1016/j.jclepro.2021.126023
![]() |
[44] |
P. Centobelli, R. Cerchione, E. Esposito, Pursuing supply chain sustainable development goals through the adoption of green practices and enabling technologies: A cross-country analysis of LSPs, Technol. Forecast. Soc. Change, 153 (2020), 119920. https://doi.org/10.1016/j.techfore.2020.119920 doi: 10.1016/j.techfore.2020.119920
![]() |
[45] |
M. Pagell, A. Shevchenko, Why research in sustainable supply chain management should have no future, J. Supply Chain Manag., 50 (2014), 44–55. https://doi.org/10.1111/jscm.12037 doi: 10.1111/jscm.12037
![]() |
[46] |
Z. Wu, F. Jia, Toward a theory of supply chain fields–understanding the institutional process of supply chain localization, J. Oper. Manag., 58 (2018), 27–41. https://doi.org/10.1016/j.jom.2018.03.002 doi: 10.1016/j.jom.2018.03.002
![]() |
[47] |
S. Seuring, M. Müller, From a literature review to a conceptual framework for sustainable supply chain management, J. Cleaner Prod., 16 (2008), 1699–1710. https://doi.org/10.1016/j.jclepro.2008.04.020 doi: 10.1016/j.jclepro.2008.04.020
![]() |
[48] |
E. Koberg, A. Longoni, A systematic review of sustainable supply chain management in global supply chains, J. Cleaner Prod., 207 (2019), 1084–1098. https://doi.org/10.1016/j.jclepro.2018.10.033 doi: 10.1016/j.jclepro.2018.10.033
![]() |
[49] |
S. Das, R. Das, B. C. Tripathy, Multi-criteria group decision making model using single-valued neutrosophic set, Infinite Stud., 16 (2020), 421–429. https://doi.org/10.17270/J.LOG.2020.446 doi: 10.17270/J.LOG.2020.446
![]() |
[50] |
M. Gul, M. F. Ak, A comparative outline for quantifying risk ratings in occupational health and safety risk assessment, J. Cleaner Prod., 196 (2018), 653–664. https://doi.org/10.1016/j.jclepro.2018.06.106 doi: 10.1016/j.jclepro.2018.06.106
![]() |
[51] |
M. Gul, Application of Pythagorean fuzzy AHP and VIKOR methods in occupational health and safety risk assessment: The case of a gun and rifle barrel external surface oxidation and colouring unit, JOSE, 26 (2018), 705–718. https://doi.org/10.1080/10803548.2018.1492251 doi: 10.1080/10803548.2018.1492251
![]() |
[52] |
H. Gupta, A. Kumar, P. Wasan, Industry 4.0, cleaner production and circular economy: An integrative framework for evaluating ethical and sustainable business performance of manufacturing organizations, J. Cleaner Prod., 295 (2021), 126253. https://doi.org/10.1016/j.jclepro.2021.126253 doi: 10.1016/j.jclepro.2021.126253
![]() |
[53] |
P. Ghadimi, C. Wang, M. K. Lim, C. Heavey, Intelligent sustainable supplier selection using multi-agent technology: Theory and application for Industry 4.0 supply chains, Comput. Ind. Eng., 127 (2019), 588–600. https://doi.org/10.1016/j.cie.2018.10.050 doi: 10.1016/j.cie.2018.10.050
![]() |
[54] |
N. Banaeian, H. Mobli, B. Fahimnia, I. E. Nielsen, M. Omid, Green supplier selection using fuzzy group decision making methods: A case study from the agri-food industry, Comput. Oper. Res., 89 (2018), 337–347. https://doi.org/10.1016/j.cor.2016.02.015 doi: 10.1016/j.cor.2016.02.015
![]() |
[55] |
Z. Chen, X. Ming, T. Zhou, Y. Chang, Sustainable supplier selection for smart supply chain considering internal and external uncertainty: An integrated rough-fuzzy approach, Appl. Soft Comput., 87 (2020), 106004. https://doi.org/10.1016/j.asoc.2019.106004 doi: 10.1016/j.asoc.2019.106004
![]() |
[56] |
M. Riaz, S. Tanveer, D. Pamucar, D. S. Qin, Topological data analysis with spherical fuzzy soft AHP-TOPSIS for environmental mitigation system, Mathematics, 10 (2022), 1826. https://doi.org/10.3390/math10111826 doi: 10.3390/math10111826
![]() |
[57] |
H. Garg, Some picture fuzzy aggregation operators and their applications to multicriteria decision-making, Arab. J. Sci. Eng., 42 (2017), 5275–5290. https://doi.org/10.1007/s13369-017-2625-9 doi: 10.1007/s13369-017-2625-9
![]() |
[58] |
G. Wei, Picture fuzzy aggregation operators and their application to multiple attribute decision making, J. Intell. Fuzzy Syst., 33 (2017), 713–724. https://doi.org/10.3233/JIFS-161798 doi: 10.3233/JIFS-161798
![]() |
[59] |
G. Wei, Picture fuzzy Hamacher aggregation operators and their application to multiple attribute decision making, Fund. Inform., 157 (2018), 271–320. https://doi.org/10.3233/FI-2018-1628 doi: 10.3233/FI-2018-1628
![]() |
[60] |
M. Riaz, M. R. Hashmi, D. Pamucar, Y. M. Chu, Spherical linear Diophantine fuzzy sets with modeling uncertainties in MCDM, CMES, 126 (2021), 1125–1164. https://doi.org/10.32604/cmes.2021.013699 doi: 10.32604/cmes.2021.013699
![]() |
[61] |
D. Molodtsov, Soft set theory-first results, Comput. Math. Appl., 37 (1999), 19–31. https://doi.org/10.1016/S0898-1221(99)00056-5 doi: 10.1016/S0898-1221(99)00056-5
![]() |
[62] |
D. Diakoulaki, G. Mavrotas, L. Papayannakis, Determining objective weights in multiple criteria problems: The critic method, Comput. Oper. Res., 22 (1995), 763–770. https://doi.org/10.1016/0305-0548(94)00059-H doi: 10.1016/0305-0548(94)00059-H
![]() |
[63] |
I. M. Cavalcante, E. M. Frazzon, F. A. Forcellini, D. Ivanov, A supervised machine learning approach to data-driven simulation of resilient supplier selection in digital manufacturing, Int. J. Inf. Manag., 49 (2019), 86–97. https://doi.org/10.1016/j.ijinfomgt.2019.03.004 doi: 10.1016/j.ijinfomgt.2019.03.004
![]() |
[64] |
S. V. Parkouhi, A. S. Ghadikolaei, A resilience approach for supplier selection: Using Fuzzy Analytic Network Process and grey VIKOR techniques, J. Cleaner Prod., 161 (2017), 431–451. https://doi.org/10.1016/j.jclepro.2017.04.175 doi: 10.1016/j.jclepro.2017.04.175
![]() |
[65] |
J. Feng, Z. Gong, Integrated linguistic entropy weight method and multi-objective programming model for supplier selection and order allocation in a circular economy: A case study, J. Cleaner Prod., 277 (2020), 122597. https://doi.org/10.1016/j.jclepro.2020.122597 doi: 10.1016/j.jclepro.2020.122597
![]() |
[66] |
Q. Hou, L. Xie, Research on supplier evaluation in a green supply chain, Discrete Dyn. Nature Soc., 2019 (2019), 2601301. https://doi.org/10.1155/2019/2601301 doi: 10.1155/2019/2601301
![]() |
[67] |
H. Mina, D. Kannan, S. M. Gholami-Zanjani, M. Biuki, Transition towards circular supplier selection in petrochemical industry: A hybrid approach to achieve sustainable development goals, J. Cleaner Prod., 286 (2021), 125273. https://doi.org/10.1016/j.jclepro.2020.125273 doi: 10.1016/j.jclepro.2020.125273
![]() |
[68] |
K. Govindan, S. Rajendran, J. Sarkis, P. Murugesan, Multi criteria decision making approaches for green supplier evaluation and selection: A literature review, J. Cleaner Prod., 98 (2015), 66–83. https://doi.org/10.1016/j.jclepro.2013.06.046 doi: 10.1016/j.jclepro.2013.06.046
![]() |
[69] |
A. H. Lee, H. Y. Kang, C. F. Hsu, H. C. Hung, A green supplier selection model for high-tech industry, Expert Syst. Appl., 36 (2009), 7917–7927. https://doi.org/10.1016/j.eswa.2008.11.052 doi: 10.1016/j.eswa.2008.11.052
![]() |
[70] |
D. Kannan, Role of multiple stakeholders and the critical success factor theory for the sustainable supplier selection process, Int. J. Prod. Econ., 195 (2018), 391–418. https://doi.org/10.1016/j.ijpe.2017.02.020 doi: 10.1016/j.ijpe.2017.02.020
![]() |
[71] |
S. A. S. Haeri, J. Rezaei, A grey-based green supplier selection model for uncertain environments, J. Cleaner Prod., 221 (2019), 768–784. https://doi.org/10.1016/j.jclepro.2019.02.193 doi: 10.1016/j.jclepro.2019.02.193
![]() |
[72] |
M. Yazdani, P. Chatterjee, E. K. Zavadskas, S. H. Zolfani, Integrated QFD-MCDM framework for green supplier selection, J. Cleaner Prod., 142 (2017), 3728–3740. https://doi.org/10.1016/j.jclepro.2016.10.095 doi: 10.1016/j.jclepro.2016.10.095
![]() |
[73] |
H. G. Goren, A decision framework for sustainable supplier selection and order allocation with lost sales, J. Cleaner Prod., 183 (2018), 1156–1169. https://doi.org/10.1016/j.jclepro.2018.02.211 doi: 10.1016/j.jclepro.2018.02.211
![]() |
[74] |
J. Feng, Z. Gong, Integrated linguistic entropy weight method and multi-objective programming model for supplier selection and order allocation in a circular economy: A case study, J. Cleaner Prod., 277 (2020), 122597. https://doi.org/10.1016/j.jclepro.2020.122597 doi: 10.1016/j.jclepro.2020.122597
![]() |
[75] |
E. Haktanir, C. Kahraman, A novel picture fuzzy CRITIC & REGIME methodology: Wearable health technology application, Eng. Appl. Artif. Intell., 113 (2022), 104942. https://doi.org/10.1016/j.engappai.2022.104942 doi: 10.1016/j.engappai.2022.104942
![]() |
[76] |
H. Zhang, G. Wei, Location selection of electric vehicles charging stations by using the spherical fuzzy CPT–CoCoSo and D-CRITIC method, Comput. Appl. Math., 42 (2023), 60. https://doi.org/10.1007/s40314-022-02183-9 doi: 10.1007/s40314-022-02183-9
![]() |
[77] |
X. Peng, Z. Luo, Decision-making model for China's stock market bubble warning: The CoCoSo with picture fuzzy information, Artif. Intell. Rev., 54 (2021), 5675–5697. https://doi.org/10.1007/s10462-021-09954-6 doi: 10.1007/s10462-021-09954-6
![]() |
[78] |
S. Korucuk, A. Aytekin, F. Ecer, D. S. S. Pamucar, Ç. Karamasa, Assessment of ideal smart network strategies for logistics companies using an integrated picture fuzzy LBWA–CoCoSo framework, Manag. Decis., 61 (2023), 1434–1462. https://doi.org/10.1108/MD-12-2021-1621 doi: 10.1108/MD-12-2021-1621
![]() |
[79] |
M. Qiyas, M. Naeem, S. Khan, S. Abdullah, T. Botmart, T. Shah, Decision support system based on CoCoSo method with the picture fuzzy information, J. Math., 2022 (2022), 1476233. https://doi.org/10.1155/2022/1476233 doi: 10.1155/2022/1476233
![]() |
[80] | A. Mohata, N. Mukhopadhyay, V. Kumar, CRITIC-COPRAS-Based Selection of Commercially Viable Alternative Fuel Passenger Vehicle, In: Advances in Modelling and Optimization of Manufacturing and Industrial Systems, Singapore: Springer, 2023, 51–69. https://doi.org/10.1007/978-981-19-6107-6_5 |
[81] |
M. Kamali Saraji, D. Streimikiene, G. L. Kyriakopoulos, Fermatean fuzzy CRITIC-COPRAS method for evaluating the challenges to Industry 4.0 adoption for a sustainable digital transformation, Sustainability, 13 (2021), 9577. https://doi.org/10.3390/su13179577 doi: 10.3390/su13179577
![]() |
[82] | M. K. Saraji, D. Streimikiene, A. Lauzadyte-Tutliene, A novel pythogorean fuzzy-SWARA-CRITIC-COPRAS method for evaluating the barriers to developing business model innovation for sustainability, In: Handbook of research on novel practices and current successes in achieving the sustainable development goals, Hershey, PA: IGI Global, 2021, 1–31. https://doi.org/10.4018/978-1-7998-8426-2.ch001 |
[83] | N. YILMAZ, Economic and financial performance of the balkans: Integrated BWM based CoCoSo Method, In: International Academic Research and Reviews in Social, Human and Administrative Sciences, ANKARA: Serüven Publishing, 2023,121–140. |