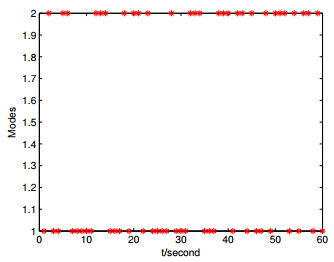
This paper is dedicated to the following Choquard system:
{−Δu+u=2pp+q(Iα∗|v|q)|u|p−2u,−Δv+v=2qp+q(Iα∗|u|p)|v|q−2v,u(x)→0, v(x)→0 as |x|→∞,
where N≥1, α∈(0,N) and N+αN<p, q<2α∗, in which 2α∗ denotes N+αN−2 if N≥3 and 2α∗:=∞ if N=1, 2. Iα is a Riesz potential. We obtain the odd symmetry of ground state solutions via a variant of Nehari constraint. Our results can be looked on as a partial generalization to results by Ghimenti and Schaftingen (Nodal solutions for the Choquard equation, J. Funct. Anal. 271 (2016), 107).
Citation: Jianqing Chen, Qihua Ruan, Qian Zhang. Odd symmetry of ground state solutions for the Choquard system[J]. AIMS Mathematics, 2023, 8(8): 17603-17619. doi: 10.3934/math.2023898
[1] | Yanting Xiao, Yifan Shi . Robust estimation for varying-coefficient partially nonlinear model with nonignorable missing response. AIMS Mathematics, 2023, 8(12): 29849-29871. doi: 10.3934/math.20231526 |
[2] | Heng Liu, Xia Cui . Adaptive estimation for spatially varying coefficient models. AIMS Mathematics, 2023, 8(6): 13923-13942. doi: 10.3934/math.2023713 |
[3] | Yanping Liu, Juliang Yin . B-spline estimation in varying coefficient models with correlated errors. AIMS Mathematics, 2022, 7(3): 3509-3523. doi: 10.3934/math.2022195 |
[4] | Anum Iftikhar, Hongbo Shi, Saddam Hussain, Ather Qayyum, M. El-Morshedy, Sanaa Al-Marzouki . Estimation of finite population mean in presence of maximum and minimum values under systematic sampling scheme. AIMS Mathematics, 2022, 7(6): 9825-9834. doi: 10.3934/math.2022547 |
[5] | Sanaa Al-Marzouki, Christophe Chesneau, Sohail Akhtar, Jamal Abdul Nasir, Sohaib Ahmad, Sardar Hussain, Farrukh Jamal, Mohammed Elgarhy, M. El-Morshedy . Estimation of finite population mean under PPS in presence of maximum and minimum values. AIMS Mathematics, 2021, 6(5): 5397-5409. doi: 10.3934/math.2021318 |
[6] | Jieqiong Lu, Peixin Zhao, Xiaoshuang Zhou . Orthogonality based modal empirical likelihood inferences for partially nonlinear models. AIMS Mathematics, 2024, 9(7): 18117-18133. doi: 10.3934/math.2024884 |
[7] | Zawar Hussain, Atif Akbar, Mohammed M. A. Almazah, A. Y. Al-Rezami, Fuad S. Al-Duais . Diagnostic power of some graphical methods in geometric regression model addressing cervical cancer data. AIMS Mathematics, 2024, 9(2): 4057-4075. doi: 10.3934/math.2024198 |
[8] | Peng Lai, Wenxin Tian, Yanqiu Zhou . Semi-supervised estimation for the varying coefficient regression model. AIMS Mathematics, 2024, 9(1): 55-72. doi: 10.3934/math.2024004 |
[9] | Dayang Dai, Dabuxilatu Wang . A generalized Liu-type estimator for logistic partial linear regression model with multicollinearity. AIMS Mathematics, 2023, 8(5): 11851-11874. doi: 10.3934/math.2023600 |
[10] | Abdullah Mohammed Alomair, Weineng Zhu, Usman Shahzad, Fawaz Khaled Alarfaj . Non-parametric calibration estimation of distribution function under stratified random sampling. AIMS Mathematics, 2025, 10(2): 4457-4472. doi: 10.3934/math.2025205 |
This paper is dedicated to the following Choquard system:
{−Δu+u=2pp+q(Iα∗|v|q)|u|p−2u,−Δv+v=2qp+q(Iα∗|u|p)|v|q−2v,u(x)→0, v(x)→0 as |x|→∞,
where N≥1, α∈(0,N) and N+αN<p, q<2α∗, in which 2α∗ denotes N+αN−2 if N≥3 and 2α∗:=∞ if N=1, 2. Iα is a Riesz potential. We obtain the odd symmetry of ground state solutions via a variant of Nehari constraint. Our results can be looked on as a partial generalization to results by Ghimenti and Schaftingen (Nodal solutions for the Choquard equation, J. Funct. Anal. 271 (2016), 107).
Recently, neural networks such as the Hopfield neural network, cellular neural network, Cohen-Grossberg neural network and bidirectional associative neural network have attracted much attention due to their significant promise for many practical applications[1,2,3,4,5,6]. For example, in the field of signal processing, based on the theoretical results of discrete-time high-order switched neural networks synchronization, the encryption and decryption scheme of multi-channel audio signal design has good security [3]. In the field of fault diagnosis, by using deep parameter-free reconstruction-classification networks with parameter-free adaptively rectified linear units, the fault characteristics of vibration signals under the same fault state under different operating conditions can be better captured [5]. In the field of image processing, the theoretical results of random synchronization control framework based on semi-Markov switching quaternion-valued neural networks can be effectively applied to image encryption [6]. In particular, stability properties of the neural networks play a significant role in their design for solving practical problems. There are some different factors to influence the stability of neural networks. In particular, time delay is often unavoidable. Moreover, for the neural networks with time delay, there exists many techniques to reduce the conservatism of stability conditions, such as the improved bounding technique [7], free-weighting matrix theory [8], integral inequality technique [9] and so on.
It is well known that many dynamical systems may switch in different unpredictable modes, such as random failures [10]. As an effective tool, the Markovian jump process can be used to model these switching systems. Until now, there existed many literatures to investigate the stability, stabilization and observation for Markovian jump systems. For example, the authors investigated the adaptive sliding mode control problem of nonlinear Markovian jump systems with partly unknown transition probabilities, and obtained some conditions to guarantee the stochastic stability of the closed-loop system in [11]. The authors in [12] discussed the realization of H∞ finite-time control for a class of uncertain stochastic time-delay systems with unmeasured states through sliding mode control, and obtained some conditions to guarantee the system state was stabilized within a limited time interval. In [13], the authors established some passivity analysis criteria for Markovian jump singularly perturbed systems with partially unknown probabilities by using the hidden Markov model, and presented a unified controller design method to ensure the passivity of the system. Much more literatures can be found in [14,15,16].
In recent years, sliding mode control has become an effective robust control method because it is insensitive to model uncertainties, parameter variations and external disturbances. So, the sliding mode control has been used for lots of physical systems, such as robot manipulators, automotive engines and power systems. There are some existing results for linear or nonlinear systems[17,18,19,20,21,22]. For example, the authors in [17] investigated the adaptive sliding mode control issue for switched nonlinear systems with matched and mismatched uncertainties, designed the switched adaptive sliding mode control law and estimated the upper bound parameters of the matched uncertainty. The authors in [18] considered the synchronization of delayed chaotic neural networks with unknown disturbance via the observer-based sliding mode control, where the sliding surface involves an integral structure and a discontinuous controller.
In addition, synchronization has received considerable attention from various research fields, such as secure communication [3], engineering [23] and chemistry [24]. Synchronization phenomenons can be observed in many real systems such as neural systems, lasers and electronic circuits [25,26,27,28]. For example, the authors in [25] dealt with chaos synchronization for master-slave piecewise linear systems and provided some new sufficient conditions by using a Lyapunov approach and the so-called S-procedure. In [26], the authors designed a proportional-derivative (PD) controller for the master-slave synchronization of chaotic Lurie systems and presented a new synchronization criterion based on Lyapunov functions with a quadratic form of states and nonlinear functions of the systems. In [27], the authors studied the finite-time lag synchronization issue of master-slave complex networks with unknown signal propagation delays by the linear and adaptive error state feedback approaches and discovered that the setting time was related to initial values.
Motivated by the above discussion, we will consider the master-slave synchronization for uncertain neural networks with time-delay by using the sliding mode control method. The main contributions of our paper are as follows: (1) The master-slave synchronization for uncertain Markov jump neural networks with time-delay is considered by using the sliding mode control method, (2) the effect of time-delay and uncertainty for the neural networks stability are considered, and the uncertain parts in the neural networks only need be bounded other than any structure condition and (3) the provided sliding mode controller is very general.
The rest of this letter is organized as follows. In Section 2, the considered neural network model and some preliminaries are given. In Section 3, some sufficient conditions are proposed by the sliding mode control. In Section 4, a numerical example is provided to illustrate the effectiveness of the method proposed. In the last section, conclusions are presented.
Notation:
Rn: the n-dimensional Euclidean space; Rn×m: the set of all n×m real matrices; ||⋅||: the two-norm of a vector; ||⋅||1: its one-norm; In: the n order unit matrix; λmin(H): the minimum eigenvalues of matrix H; the notation X>Y, where X,Y are symmetric matrices, meaning that X−Y is a positive definite symmetric matrix. For a given matrix A∈Rn×n, AT denotes its transpose. sign(⋅) is the sign function. ∗ in a symmetric matrix denotes the symmetric terms.
Let (Ω,F,Ft,P) be a probability space related to an increasing family {Ft}t≥0 of the σ-algebras Ft⊂F, where Ω is the sample space. F is the σ-algebras of the sample space and P is the probability measure defined on F.
Consider the uncertain time delay neural networks with the Markovian jump defined on the probability space (Ω,F,Ft,P) as follows:
M:{˙xm(t)=−A(r(t))xm(t)+[B(r(t))+ΔB(r(t))]f(xm(t))+[C(r(t))+ΔC(r(t))]g(xm(t−d(t)))+J,xm(t)=φ(t),t∈[−d,0], | (2.1) |
where xm(t)=(xm1(t),xm2(t),...,xmn(t))T∈Rn is the state of neuron networks and A(r(t))∈Rn×n and B(r(t))∈Rn×m and C(r(t))∈Rn×m are coefficient matrices. ΔB(r(t)) and ΔC(r(t))∈Rn×m denote the system's uncertain parts and satisfy
[ΔB(r(t))ΔC(r(t))]=M(r(t))W(t)[N1(r(t))N2(r(t))], |
where matrix W(t) satisfies WT(t)W(t)≤I, M(r(t)),N1(r(t)),N2(r(t)) are some known matrices with appropriate dimensions. d(t) represents time delay and satisfies 0≤d(t)≤d and ˙d(t)≤μ≤1. f(xm(t)) and g(xm(t−d(t))) are the neuron activation functions, and J∈Rn is a constant vector. {r(t),t≥0} is a finite state Markov jumping process and represents the switching process among different modes, which takes values in a state space L={1,2,...,l}, and l is the number of modes. Let ∏=[πij]l×l denote the transition rate matrix, where the mode transition probabilities are
Pr{r(t+Δt)=j|r(t)=i}={πijΔt+o(Δt),i≠j,1+πiiΔt+o(Δt),i=j, | (2.2) |
where Δt>0 and limΔt→0o(Δt)Δt=0, πij satisfies πij>0 with i≠j and πii=−∑lj=1,j≠iπij for each mode i.
In order to be notional convenience, for the i-th mode, system (2.1) can be rewritten as
{˙xm(t)=−Aixm(t)+(Bi+ΔBi)f(xm(t))+(Ci+ΔCi)g(xm(t−d(t)))+J,xm(t)=φ(t),t∈[−d,0]. | (2.3) |
Let (2.3) be the master system, then the slave system is
S:{˙xs(t)=−A(r(t))xs(t)+B(r(t))f(xs(t))+C(r(t))g(xs(t−d(t)))+J+u(t),xs(t)=ψ(t),t∈[−d,0]. | (2.4) |
Definition 1. [29] Master system (2.3) and slave system (2.4) are said to be asymptotic synchronization if
limt→∞||xm(t)−xs(t)||=0 |
for any initial conditions.
In this paper, our objective is to design a suit controller u(t) such that the master system (2.3) and slave system (2.4) are in synchronization by using the sliding mode control method.
To the end, writing the state error e(t)=xs(t)−xm(t), and the corresponding state error system can be described by
{˙e(t)=−Aie(t)+BiF(e(t))+CiG(e(t−d(t)))−ΔBif(xm(t))−ΔCig(xm(t−d(t)))+u(t),e(t)=ψ(t)−φ(t),t∈[−d,0], | (2.5) |
where F(e(t))=f(xs(t))−f(xm(t)) and G(e(t−d(t)))=g(xs(t−d(t)))−g(xm(t−d(t))).
First, we take the sliding mode surface as
σi(t)=e(t)+∫t0[(Ai+Ki)e(θ)−BiF(e(θ))−CiG(e(θ−d(θ)))]dθ. | (2.6) |
Thus, the derivative of σi(t) is
˙σi(t)=˙e(t)+(Ai+Ki)e(t)−BiF(e(t))−CiG(e(t−d(t)))=Kie(t)−ΔBif(xm(t))−ΔCig(xm(t−d(t)))+u(t), | (2.7) |
where Ki(1≤i≤l) are some unknown matrices to be determined later. When the state trajectories reach the sliding mode surface, then ˙σi(t)=0 and σi(t)=0. So, we obtain the equivalent controller
ueq(t)=−Kie(t)+ΔBif(xm(t))+ΔCig(xm(t−d(t))). | (2.8) |
Substituting (2.8) into (2.5), we have
{˙e(t)=−(Ai+Ki)e(t)+BiF(e(t))+CiG(e(t−d(t))),e(t)=ψ(t)−φ(t),t∈[−d,0]. | (2.9) |
In this paper, the following assumptions for the neuron activation functions are needed.
Assumption 1. [30] Assume that each component of the nonlinear function f(⋅) and g(⋅) are continuous and bounded and satisfy
η−k≤fk(z1)−fk(z2)z1−z2≤η+k, |
θ−k≤gk(z1)−gk(z2)z1−z2≤θ+k,∀k=1,2,...,n, |
for any z1,z2∈R, where η−k>0,η+k>0,θ−k>0,θ+k>0 are some known positive constants.
From Assumption 1, it is easy to get the following inequalities
[eT(t)FT(e(t))][Θ1HΘ2H∗H][e(t)F(e(t))]≤0 | (2.10) |
and
[eT(t)GT(e(t−d(t)))][Θ3HΘ4H∗H][e(t)G(e(t−d(t)))]≤0, | (2.11) |
where H=diag{h1,h2,...,hn} is a positive definite diagonal matrix,
Θ1=diag{η−1η+1,η−2η+2,...,η−nη+n},Θ2=diag{−η−1+η+12,−η−2+η+22,...,−η−n+η+n2}, |
Θ3=diag{θ−1θ+1,θ−2θ+2,...,θ−nθ+n},Θ4=diag{−θ−1+θ+12,−θ−2+θ+22,...,−θ−n+θ+n2}. |
Assumption 2.[31] Assume that each component of the nonlinear functions f(⋅) and g(⋅) are bounded, which means that there exists positive scalars Bf and Bg such that
||fk(⋅)||≤Bf,||gk(⋅)||≤Bg |
for k=1,2,...,n.
Remark 1. In fact, the activation functions of neural networks are usually bounded. For example, the Logistic Sigmoid function h1(x)={1+e−ax}−1 and the threshold value function
h2(x)={1,x≥0,−1,x<0, |
and so on.
Throughout the paper, we need the following lemmas.
Lemma 1.[32] For any positive definite symmetric matrix W∈Rn×n and scalar τ>0, there is
∫tt−τxT(s)dsW∫tt−τx(s)ds≤τ∫tt−τxT(s)Wx(s)ds. |
Lemma 2.[33] The linear matrix inequality
[S11S12ST12S22]<0 |
is equivalent to the following condition
S22<0,S11−S12S−122ST12<0, |
where S11 and S22 are symmetric matrices.
Now, we will analyze the synchronization condition and construct the sliding mode controller.
Theorem 1. Under Assumption 1, if there exists positive definite symmetric matrices Pi,Wi,Ri∈Rn×n such that
Φ1i=[Φ1i,1101dRiPiBi−Θ2HPiCi−Θ4H−ATiRi−KTiRi∗−(1−μ)Wi0000∗∗−1dRi000∗∗∗−H0BTiRi∗∗∗∗−HCTiRi∗∗∗∗∗−1dRi]<0 | (3.1) |
for i=1,2,...,l, then the master system (2.1) and slave system (2.4) are in synchronization, where
Φ1i,11=−PiAi−ATiPi−PiKi−KTiPi+l∑j=1,j≠iπij(Pj−Pi)+Wi−1dRi−Θ1H−Θ3H. |
Proof. Constructing the following Lyapunov function
V(t)=eT(t)Pie(t)+∫tt−d(t)eT(θ)Wie(θ)dθ+∫0−d∫tt+s˙eT(θ)Ri˙e(θ)dθds. |
The derivative of V(t) along with the trajectories of system (2.9) is
˙V(t)=2eT(t)Pi˙e(t)+eT(t)∑lj=1πijPje(t)+eT(t)Wie(t)−(1−˙d(t))eT(t−d(t))Wie(t−d(t))+d˙eT(t)Ri˙e(t)−∫tt−d˙eT(θ)Ri˙e(θ)dθ≤2eT(t)Pi[−(Ai+Ki)e(t)+BiF(e(t))+CiG(e(t−d(t)))]+eT(t)∑lj=1,j≠iπij(Pj−Pi)e(t)+eT(t)Wie(t)−(1−μ)eT(t−d(t))Wie(t−d(t))+d[−(Ai+Ki)e(t)+BiF(e(t))+CiG(e(t−d(t)))]TRi⋅[−(Ai+Ki)e(t)+BiF(e(t))+CiG(e(t−d(t)))]−1d[e(t)−e(t−d)]TRi[e(t)−e(t−d)]. |
Letting ξ(t)=(eT(t),eT(t−d(t)),eT(t−d),FT(e(t))andGT(e(t−d(t)))T, one yields
˙V(t)≤ξT(t)[Φ2i+dΨTRiΨ]ξ(t), | (3.2) |
where
Φ2i=[Φ2i,1101dRiPiBiPiCi∗−(1−μ)Wi000∗∗−1dRi00∗∗∗00∗∗∗∗0], |
Ψ=[−Ai−Ki00BiCi], |
Φ2i,11=−PiAi−ATiPi−PiKi−KTiPi+l∑j=1,j≠iπij(Pj−Pi)+Wi−1dRi. |
It is noted that
ξT(t)[Φ2i+dΨTRiΨ]ξ(t)−[eT(t)FT(e(t))][Θ1HΘ2H∗H][e(t)F(e(t))]−[eT(t)GT(e(t−d(t)))][Θ3HΘ4H∗H][e(t)G(e(t−d(t)))]=ξT(t)[Φ3i+dΨTRiΨ]ξ(t), | (3.3) |
where
Φ3i=[Φ1i,1101dRiPiBi−Θ2HPiCi−Θ4H∗−(1−μ)Wi000∗∗−1dRi00∗∗∗−H0∗∗∗∗−H]. |
By using Lemma 2, we know that Φ3i+dΨTRiΨ<0 is equivalent to Φ1i<0. Thus, one obtains ˙V(t)<0 and system (2.9) is asymptotically stable, which shows that the master system (2.1) and slave system (2.4) are in synchronization. The proof is completed.
Theorem 2. Under Assumption 2 and the action of controller
u(t)=−Kie(t)−ζ(t)sign(σi(t)), | (3.4) |
master system (2.1) and slave system (2.4) are in synchronization, where
ζ(t)=Bf⋅||Mi||⋅||N1i||+Bg⋅||Mi||⋅||N2i||+α, |
and α>0 is a positive scalar.
Proof. Constructing the following Lyapunov function
U(t)=12σTi(t)σi(t), |
then
˙U(t)=σTi(t)˙σi(t)=σTi(t)[Kie(t)−ΔBif(xm(t))−ΔCig(xm(t−d(t)))−Kie(t)−ζ(t)sign(σi(t))]≤||σi(t)||[||ΔBi||⋅||f(xm(t))||+||ΔCi||⋅||g(xm(t−d(t)))||]−ζ(t)||σi(t)||. | (3.5) |
Because of
||ΔBi||⋅||f(xm(t))||+||ΔCi||⋅||g(xm(t−d(t)))||≤||Mi||[Bf⋅||N1i||+Bg⋅||N2i||], |
then
˙U(t)≤−α||σi(t)||=−α√2U(t). |
Thus, the state trajectories can attain the sliding mode surface in the finite time interval [0,T∗], where T∗≤√2U(0)2α. The proof is completed.
Remark 2. Compared with the uncertain system in [15], we especially considered the effect of time delay. From Theorems 1 and 2, we see that the designed sliding mode controller can realize the master-slave synchronization of the Markov jump neural networks.
Remark 3. The control gain Ki can be obtained from matrix inequality (3.1). However, (3.1) is not a linear matrix inequality. In order to solve it, we can take Pi=Ri.
From Theorem 1, we can obtain the following useful corollary as r(t)=1, which means that system (2.1) only has one mode.
Corollary 1. Under Assumption 1, if there exist positive definite symmetric matrices P,W,R∈Rn×n such that
Φ1=[Φ1,1101dRPB−Θ2HPC−Θ4H−ATR−KTR∗−(1−μ)W0000∗∗−1dR000∗∗∗−H0BTR∗∗∗∗−HCTR∗∗∗∗∗−1dR]<0, | (3.6) |
then the master system
{˙xm(t)=−Axm(t)+(B+ΔB)f(xm(t))+(C+ΔC)g(xm(t−d(t)))+J,xm(t)=φ(t),t∈[−d,0] | (3.7) |
and slave system
{˙xs(t)=−Axs(t)+Bf(xs(t))+Cg(xs(t−d(t)))+J+u(t),xs(t)=ψ(t),t∈[−d,0] | (3.8) |
are in synchronization, where
Φ1,11=−PA−ATP−PK−KTP+W−1dR−Θ1H−Θ3H. |
Consider master system (2.1) and slave system (2.4) with the following parameters
A1=[42105−20−26],A2=[5120700−18],B1=[0.20.60.20.2−0.200.2−0.1−0.1], |
B2=[0.20.50.30.2−0.400.3−0.1−0.2],C1=[0.50.4−0.30.50.10.10.50.10.5],C2=[0.8−0.30.30.10.20.2−0.60.30.2], |
Π=[−221−1],M1=[−0.20.50.7],M2=[0.30.8−0.4], |
N11=[0.1−0.2−0.1],N12=[0.05−0.1−0.03], |
N21=[−0.40.10.3],N22=[−0.10.020.07],μ=0.5,d=2, |
f(x(t))=[tanh(0.5x1(t)),tanh(0.4x2(t)),tanh(0.6x3(t))]T, |
g(x(t))=[tanh(0.3x1(t)),tanh(0.4x2(t)),tanh(0.2x3(t))]T, |
J1=0.5I3,J2=0.3I3,Θ1=Θ3=I3,Θ2=Θ4=−I3,w(t)=sin(t). |
By using the linear matrix inequality (LMI) toolbox in the MATLAB, we obtain the following solutions of inequality (3.1):
P1=[23.4405−7.37377.0525−7.373765.8658−20.94927.0525−20.949240.9154],P2=[21.2128−6.29397.4587−6.293961.5282−19.50707.4587−19.507038.4827], |
W1=[10.4452−3.21871.4795−3.218724.3005−7.12561.4795−7.125615.5240],W2=[5.7913−1.22002.4543−1.220016.4749−4.94762.4543−4.947610.7346], |
K1=[0.13660.0093−0.01880.00930.05500.0266−0.01880.02660.0902],K2=[0.13660.0093−0.01880.00930.05500.0266−0.01880.02660.0902], |
H=[76.637400059.824500045.2654]. |
For the initial values xm(0)=(−25,5,25)T and xs(0)=(30,−5,−25)T, Figure 1 is the Markovian jump process in different modes. Figure 2 is the state trajectories of error system (2.5), which shows that the error system is convergent and the master system synchronizes with the slave system. Figure 3 is the curve of the sliding mode surface, which shows that the state trajectories can arrive at the surface in a finite time interval.
In this paper, the master-slave synchronization for uncertain neural networks with time delay by using the sliding mode control method has been studied. An integral sliding mode surface and sliding mode controller was designed. Moreover, the state trajectories of the neural networks can reach the sliding mode surface in finite time under the action of the controller. Sufficient conditions in terms of linear matrix inequalities were presented to guarantee the neural networks asymptotical stability. Finally, an example was provided to illustrate the validity of the proposed design method. In the future, we will consider how to solve some physical problems by applying the obtained theoretical results.
The authors declare they have not used Artificial Intelligence (AI) tools in the creation of this article.
This work is supported by the National Natural Science Foundation (NNSF) of China under Grant 11602134.
The authors declare that they have no conflicts of interest.
[1] |
N. Ackermann, On a periodic Schrödinger equation with nonlocal superlinear part, Math. Z., 248 (2004), 423–443. https://doi.org/10.1007/s00209-004-0663-y doi: 10.1007/s00209-004-0663-y
![]() |
[2] |
J. Chen, B. Guo, Blow up solutions for one class of system of Pekar-Choquard type nonlinear Schrödinger equation, Appl. Math. Comput., 186 (2007), 83–92. https://doi.org/10.1016/j.amc.2006.07.089 doi: 10.1016/j.amc.2006.07.089
![]() |
[3] |
P. Chen, X. Liu, Ground states of linearly coupled systems of Choquard type, Appl. Math. Lett., 84 (2018), 70–75. https://doi.org/10.1016/j.aml.2018.04.016 doi: 10.1016/j.aml.2018.04.016
![]() |
[4] |
M. Ghimenti, J. V. Schaftingen, Nodal solutions for the Choquard equation, J. Funct. Anal., 271 (2016), 107–135. https://doi.org/10.1016/j.jfa.2016.04.019 doi: 10.1016/j.jfa.2016.04.019
![]() |
[5] |
M. Clapp, D. Salazar, Positive and sign changing solutions to a nonlinear Choquard equation, J. Math. Anal. Appl., 407 (2013), 1–15. https://doi.org/10.1016/j.jmaa.2013.04.081 doi: 10.1016/j.jmaa.2013.04.081
![]() |
[6] |
C. Gui, H. Guo, On nodal solutions of the nonlinear Choquard equation, Adv. Nonlinear Stud., 19 (2019), 677–691. https://doi.org/10.1515/ans-2019-2061 doi: 10.1515/ans-2019-2061
![]() |
[7] |
C. Gui, H. Guo, Nodal solutions of a nonlocal Choquard equation in a bounded domain, Commun. Contemp. Math., 23 (2019), 1950067. https://doi.org/10.1142/S0219199719500676 doi: 10.1142/S0219199719500676
![]() |
[8] | Z. Huang, J. Yang, W. Yu, Multiple nodal solutions of nonlinear Choquard equations, Electron. J. Differ. Equations, 2017 (2017), 1–18. |
[9] |
X. Li, S. Ma, G. Zhang, Existence and qualitative properties of solutions for Choquard equations with a local term, Nonlinear Anal., 45 (2019), 1–25. https://doi.org/10.1016/j.nonrwa.2018.06.007 doi: 10.1016/j.nonrwa.2018.06.007
![]() |
[10] |
E. H. Lieb, Existence and uniqueness of the minimizing solution of Choquard's nonlinear equation, Stud. Appl. Math., 57 (1977), 93–105. https://doi.org/10.1002/sapm197757293 doi: 10.1002/sapm197757293
![]() |
[11] | E. Lieb, M. Loss, Graduate studies in mathematics, American Mathematical Society, 2001. |
[12] |
P. L. Lions, The Choquard equation and related questions, Nonlinear Anal., 4 (1980), 1063–1072. https://doi.org/10.1016/0362-546X(80)90016-4 doi: 10.1016/0362-546X(80)90016-4
![]() |
[13] |
P. L. Lions, The concentration-compactness principle in the calculus of variations. The locally compact case, part 2, Ann. Inst. H. Poincaré Anal. Non Linéaire., 1 (1984), 223–283. https://doi.org/10.1016/S0294-1449(16)30422-X doi: 10.1016/S0294-1449(16)30422-X
![]() |
[14] |
L. Ma, L. Zhao, Classification of positive solitary solutions of the nonlinear Choquard equation, Arch. Ration. Mech. Anal., 195 (2010), 455–467. https://doi.org/10.1007/s00205-008-0208-3 doi: 10.1007/s00205-008-0208-3
![]() |
[15] |
I. M. Moroz, R. Penrose, P. Tod, Spherically-symmetric solutions of the Schrödinger-Newton equations, Class. Quantum Grav., 15 (1998), 2733–2742. https://doi.org/10.1088/0264-9381/15/9/019 doi: 10.1088/0264-9381/15/9/019
![]() |
[16] |
V. Moroz, J. Schaftingen, Nonexistence and optimal decay of supersolutions to Choquard equations in exterior domains, J. Differ Equations, 254 (2013), 3089–3145. https://doi.org/10.1016/j.jde.2012.12.019 doi: 10.1016/j.jde.2012.12.019
![]() |
[17] |
V. Moroz, J. Schaftingen, Ground states of nonlinear Choquard equations: existence, qualitative properties and decay asymptotics, J. Funct. Anal., 265 (2013), 153–184. https://doi.org/10.1016/j.jfa.2013.04.007 doi: 10.1016/j.jfa.2013.04.007
![]() |
[18] | V. Moroz, J. Schaftingen, Existence of groundstates for a class of nonlinear Choquard equations, Trans. Amer. Math. Soc., 367 (2015), 6557–6579. |
[19] |
V. Moroz, J. Schaftingen, Groundstates of nonlinear Choquard equations: Hardy-Littlewood-Sobolev critical exponent, Commun. Contemp. Math., 17 (2015), 1550005. https://doi.org/10.1142/S0219199715500054 doi: 10.1142/S0219199715500054
![]() |
[20] |
V. Moroz, J. Schaftingen, A guide to the Choquard equation, J. Fixed Point Theory Appl., 19 (2017), 773–813. https://doi.org/10.1007/s11784-016-0373-1 doi: 10.1007/s11784-016-0373-1
![]() |
[21] | S. I. Pekar, Untersuchung über die elektronentheorie der kristalle, Akademie Verlag, 1954. https://doi.org/10.1515/9783112649305 |
[22] |
J. V. Schaftingen, Interpolation inequalities between Sobolev and Morrey-Campanato spaces: a common gateway to concentration-compactness and Gagliardo-Nirenberg interpolation inequalities, Port. Math., 71 (2014), 159–175. https://doi.org/10.4171/PM/1947 doi: 10.4171/PM/1947
![]() |
[23] |
J. V. Schaftingen, J. Xia, Groundstates for a local nonlinear perturbation of the Choquard equations with lower critical exponent, J. Math. Anal. Appl., 464 (2018), 1184–1202. https://doi.org/10.1016/j.jmaa.2018.04.047 doi: 10.1016/j.jmaa.2018.04.047
![]() |
[24] |
G. Vaira, Ground states for Schrödinger-Poisson type systems, Ric. Mat., 60 (2011), 263–297. https://doi.org/10.1007/s11587-011-0109-x doi: 10.1007/s11587-011-0109-x
![]() |
[25] |
G. Vaira, Existence of bound states for Schrödinger-Newton type systems, Adv. Nonlinear Stud., 13 (2013), 495–516. https://doi.org/10.1515/ans-2013-0214 doi: 10.1515/ans-2013-0214
![]() |
[26] |
T. Wang, H. Guo, Existence and nonexistence of nodal solutions for Choquard type equations with perturbation, J. Math. Anal. Appl., 480 (2019), 123438. https://doi.org/10.1016/j.jmaa.2019.123438 doi: 10.1016/j.jmaa.2019.123438
![]() |
[27] | M. Willem, Minimax theorems, Birkhäuser, 1996. https://doi.org/10.1007/978-1-4612-4146-1 |
[28] |
N. Xu, S. Ma, R. Xing, Existence and asymptotic behavior of vector solutions for linearly coupled Choquard-type systems, Appl. Math. Lett., 104 (2020), 106249. https://doi.org/10.1016/j.aml.2020.106249 doi: 10.1016/j.aml.2020.106249
![]() |
[29] |
M. Yang, J. C. D. Albuquerque, E. D. Silva, M. L. Silva, On the critical cases of linearly coupled Choquard systems, Appl. Math. Lett., 91 (2018), 1–8. https://doi.org/10.1016/j.aml.2018.11.005 doi: 10.1016/j.aml.2018.11.005
![]() |
[30] |
X. Zhong, C. Tang, Ground state sign-changing solutions for a class of subcritical Choquard equations with a critical pure power nonlinearity in RN, Comput. Math. Appl., 76 (2018), 23–34. https://doi.org/10.1016/j.camwa.2018.04.001 doi: 10.1016/j.camwa.2018.04.001
![]() |