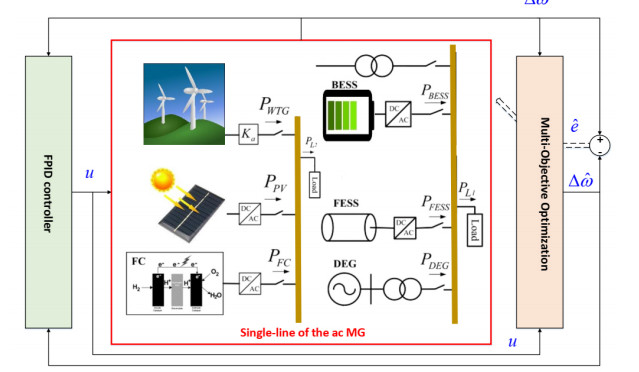
The PID controller is a popular controller that is widely used in various industrial applications. On the other hand, the control problems in microgrids (MGs) are so challenging, because of natural disturbances such as wind speed changes, load variation, and changes in other sources. This paper proposes an input-output scaling factor tuning of interval type-2 fuzzy (IT2F) PID controller using a multi-objective optimization technique. The suggested controller is applied to an MG frequency regulation problem. In the introduced controller the effect of variations of renewable energies (REs) and other disturbances are taken into account, and the robustness is investigated. In the multi-objective scheme, some factors such as least overshoot, and minimum settling/rising time are considered. The simulations show that by considering the suitable adjustment the desired regulation accuracy is achieved, such that the frequency trajectory shows the desired overshoot, and settling/rising time.
Citation: Kamran Sabahi, Chunwei Zhang, Nasreen Kausar, Ardashir Mohammadzadeh, Dragan Pamucar, Amir H. Mosavi. Input-output scaling factors tuning of type-2 fuzzy PID controller using multi-objective optimization technique[J]. AIMS Mathematics, 2023, 8(4): 7917-7932. doi: 10.3934/math.2023399
[1] | Kunting Yu, Yongming Li . Adaptive fuzzy control for nonlinear systems with sampled data and time-varying input delay. AIMS Mathematics, 2020, 5(3): 2307-2325. doi: 10.3934/math.2020153 |
[2] | Liping Yin, Yangyu Zhu, Yangbo Xu, Tao Li . Dynamic optimal operational control for complex systems with nonlinear external loop disturbances. AIMS Mathematics, 2022, 7(9): 16673-16691. doi: 10.3934/math.2022914 |
[3] | Nasser Aedh Alreshidi, Muhammad Rahim, Fazli Amin, Abdulaziz Alenazi . Trapezoidal type-2 Pythagorean fuzzy TODIM approach for sensible decision-making with unknown weights in the presence of hesitancy. AIMS Mathematics, 2023, 8(12): 30462-30486. doi: 10.3934/math.20231556 |
[4] | Aceng Sambas, Ardashir Mohammadzadeh, Sundarapandian Vaidyanathan, Ahmad Faisal Mohamad Ayob, Amiral Aziz, Mohamad Afendee Mohamed, Ibrahim Mohammed Sulaiman, Mohamad Arif Awang Nawi . Investigation of chaotic behavior and adaptive type-2 fuzzy controller approach for Permanent Magnet Synchronous Generator (PMSG) wind turbine system. AIMS Mathematics, 2023, 8(3): 5670-5686. doi: 10.3934/math.2023285 |
[5] | Sarah A. Alzakari, Davut Izci, Serdar Ekinci, Amel Ali Alhussan, Fatma A. Hashim . A new control scheme for temperature adjustment of electric furnaces using a novel modified electric eel foraging optimizer. AIMS Mathematics, 2024, 9(5): 13410-13438. doi: 10.3934/math.2024654 |
[6] | Liandi Fang, Li Ma, Shihong Ding . Finite-time fuzzy output-feedback control for $ p $-norm stochastic nonlinear systems with output constraints. AIMS Mathematics, 2021, 6(3): 2244-2267. doi: 10.3934/math.2021136 |
[7] | Muhammad Akram, Naila Ramzan, Anam Luqman, Gustavo Santos-García . An integrated MULTIMOORA method with 2-tuple linguistic Fermatean fuzzy sets: Urban quality of life selection application. AIMS Mathematics, 2023, 8(2): 2798-2828. doi: 10.3934/math.2023147 |
[8] | Chuang Liu, Jinxia Wu, Weidong Yang . Robust $ {H}_{\infty} $ output feedback finite-time control for interval type-2 fuzzy systems with actuator saturation. AIMS Mathematics, 2022, 7(3): 4614-4635. doi: 10.3934/math.2022257 |
[9] | Saravanan Shanmugam, Mohamed Rhaima, Hamza Ghoudi . Exponential synchronization analysis for complex dynamical networks with hybrid delays and uncertainties under given control parameters. AIMS Mathematics, 2023, 8(12): 28976-29007. doi: 10.3934/math.20231484 |
[10] | Yifan Liu, Guozeng Cui, Ze Li . Fixed-time consensus control of stochastic nonlinear multi-agent systems with input saturation using command-filtered backstepping. AIMS Mathematics, 2024, 9(6): 14765-14785. doi: 10.3934/math.2024718 |
The PID controller is a popular controller that is widely used in various industrial applications. On the other hand, the control problems in microgrids (MGs) are so challenging, because of natural disturbances such as wind speed changes, load variation, and changes in other sources. This paper proposes an input-output scaling factor tuning of interval type-2 fuzzy (IT2F) PID controller using a multi-objective optimization technique. The suggested controller is applied to an MG frequency regulation problem. In the introduced controller the effect of variations of renewable energies (REs) and other disturbances are taken into account, and the robustness is investigated. In the multi-objective scheme, some factors such as least overshoot, and minimum settling/rising time are considered. The simulations show that by considering the suitable adjustment the desired regulation accuracy is achieved, such that the frequency trajectory shows the desired overshoot, and settling/rising time.
The increasing use of fossil fuels has caused major problems such as global warming, climate change, and environmental pollution. Using renewable energy as a viable alternative for reducing fossil fuel consumption is of particular importance. Nowadays, the integration of renewable energies has created microgrid (MG) power systems. The existing perturbations due to changes in wind speed over time and solar radiation as well as load power changes challenge the proper utilization of renewable energy. Designing a suitable controller that can balance energy generation and consumption and eliminate frequency fluctuations is of particular importance for the proper use of renewable energy [6,12].
PIDs are extensively utilized in the industrial process because of their simplicity and capability [27]. However, uncertainties, nonlinearities, and the control parameters' variation deteriorate their efficiency of them. So, fuzzy logic systems (FLSs) and artificial neural networks (ANNs) are developed. To tackle the problem of the tuning procedures, some evolutionary optimization methods like GA and PSO have been utilized to design PID [1]. In these optimization algorithms, the overshoot and settling time are usually defined as the objective function (OB) to obtain suitable values for the PID gains. Although these tuning methods can improve the efficiency of these linear and fixed structure controllers, they are susceptible to the uncertainties and nonlinearities existing in the plant. As FLSs provides the ability to model uncertainty and imprecision which exists in most of the controlled systems, they were exploited to tune the PID parameters in the online mode and according to the defined rules by the experts [20,32].
In these works, which are famous for fuzzy gain scheduling PID (FGSPID) techniques, the tracking error and derivative of error are considered as the input variables of FLS, and the PID gains are extracted from FLSs. In FGSPIDs, the control command is produced by the PID controller in which its gains change adaptively. Another combination of FLS and PID controller to design a nonlinear fuzzy PID (FPID) is to use the rules to realize parameters. The scheme of the FPID, a nonlinear PID, is similar to the structure of the classic PID from the viewpoint of input/output (I/O) dynamics. Unlike the FGSPID approach, in the FPID controller, the required command to the plant is deduced directly from the fuzzy inference. The FPID controller can be constructed using different types of FLSs. Among them, type-1 (T1) FLSs (T1FLSs) are a kind of linguistic variable that only can characterize a specific degree of imprecision or ambiguity. Interval type-2 (IT2) FLSs (IT2FLSs) are developed from of T1FLSs that introduce the concepts of uncertainty and imprecision as intervals and more robust to uncertainties such as measurement noise [8,30,31].
The other important forms of representing FLSs, generalized type-2 (GT2) FLS (GT2FLSs), which are similar to IT2FLSs, also inherently deal with uncertainty. In a situation where instead of representing uncertainty through an area, it is represented by a volume. Compared to the IT2FLSs, this form of uncertainty representation, fundamentally, is much more resilient to noise. In the FPID control design approaches, the important matter is how to set the input scaling coefficients (ISC)s and output-scaling factor (OSF), and parameters of the fuzzy controller (FC). In [10], PSO scheme was used to optimize the T1FPID controller for frequency in a power plant and it was shown that the controller provided more desirable dynamic responses with regard to settling time and undershoots/overshoots. To better handle the nonlinearities, the big bang-big crunc and GA optimization approaches have been applied to adjust the interval T2FPID controllers' parametres in [16,25], respectively. Using the combination of gradient-descent algorithm and Lyapunov theory, the OSFs and consequent parameters of an interval T2FPID controller were tuned for frequency control of micro-grid system [21]. The interval T2FPID controller in [21] was extended to regulate the frequency deviation of a time-delayed power system using a Lyapunov-Krasovskii functional. A fractional order GT2FPID controller was proposed in [23] in which its parameters were tuned based on the trial-and-error method. In many control problems, the control designing is a multi-objective (MO) problem in which several objectives, including its overshoot-undershoot and rising-settling time, must be optimized simultaneously. In this design method, the OBs are defined separately but optimized simultaneously, and the obtained results can be discussed based on the values of these OBs. It is worth pointing out that in the above methods, weighted values of different OBs were aggregated that resulting in a single OB which should be optimized by achieving optimal parameters for the FPID controller [33].
FPIDs are widely used for frequency control problems [15,34]. For example, a hybrid version of bat and fuzzy algorithms is employed in [15] to tune the PI for the load control (LFC) application. The controller is employed in the four-area power plant. It is shown that the presented controller is robust enough in case of facing uncertainties related to external disturbances. An optimal interval T2F-PID is employed by Yesil [25] for an LFC problem. Big Bang Big Crunch optimization algorithm is used to adjust the scaling factors of the designed control. The efficiency of the designed algorithm is represented by comparing its performance with that of FPID. An FPID is proposed by Sahu et al., for automatic control. A teaching-learning approach is suggested in order to optimize the FPID in [22]. A hybrid scheme of harmony and cuckoo search methods is suggested in [9] for balancing the FPID parameters for an LFC problem. A hybrid model of PID-fuzzy-PID is designed by Debnath et al. [4] for the LFC problem. The parameters of the presented model are optimized using a modified grey wolf optimization algorithm. The sensitivity is analyzed to study the robustness of the presented controller. A novel hybrid fuzzy PD-PI controller based on an improved version of the moth swarm algorithm is suggested in [14].
● Unlike the other LFC systems, the suggested controller does not depend on the MG models.
● The suggested approach considers both transient and steady-state response.
● The multi-optimization scheme is faster, and it results in better LFC accuracy.
● The suggested type-2 fuzzy-based approach has better robustness against uncertainties.
The remainder of this study is as follows: In Section 2, MG is explained. The FPID and associated tuning approach is illustrated in Sections 3–5. Simulations are given in Section 6. Finally, the conclusion is given in Section 7.
MG systems consist of locally distributed loads connected to the distributed energy source. As can be seen in Figure 1, the studied MG included a PV system (with Ppv output), photocell (with Ppc output), WT generator (with Pwtg output), battery, flywheel energy storage system (ESS) and loads. The power changes of the studied system in the jth area are described as follows [13]:
ΔPj,tie=N∑i=1,i≠jΔPi,tie=2πs[N∑i=1,i≠jTjiΔωj−N∑i=1,i≠jTjiΔωi]. | (2.1) |
In (2.1), ΔPj,tie denotes power changes. Δω and Tji are frequency deviation and torque coefficient respectively. First assume that we have just 2 areas. So, by applying disturbance to the first area, and considering N=2, the power changes between the tie line and first area are calculated as follows:
ΔP1,tie=2πs[T12Δω1−T12Δω2]. | (2.2) |
Considering a first-order model, Δω2 is obtained as follows:
Δω2=(∑nj=1ΔPm2i−ΔP2,tie−ΔP2L)D2+2H2s. | (2.3) |
From the fact thatΔPm=0 under no speed governing, and ΔP2L=0, we have:
Δω2=−ΔP2,tieD2+2H2s. | (2.4) |
Also, by ignoring Δω1, we can rewrite (2.2) as:
ΔP1,tie=2πsT12Δω1. | (2.5) |
Now consider the general scheme, and apply disturbance to the ith area, then ΔPj,tie is obtained, similarly, as follows:
ΔPj,tie=2πsN∑i=1,i≠jTjiΔωj, | (2.6) |
Δωj=(−ΔPj,tie−ΔPjL)Dj+2Hjs. | (2.7) |
By substituting (2.7) into (2.6) and considering step perturbation in load as ΔPjL(s)=ΔPjLs, ΔPj,tie is expressed as follows:
ΔPj,tie=−2πΔPjL∑Ni=1,i≠j Tji2Hjs(s2+Dj/2Hj+2π∑Ni=1,i≠j Tji2Hj). | (2.8) |
Moreover, RDR is defined as follows:
RDPj=δ−d2ΔPj,tiedt2Hjπ∑Ni=1,i≠j Tji, | (2.9) |
where d is between 0 and 1. Figure 2 indicates diagram of the studied system and dynamics of various units.
PID controllers are used in a wide range of industrial plants regarding the good capability. Nevertheless, the performance of these linear controllers is worsened due to nonlinearities, uncertainties, and parameter variation in the plant. To obtain a better system efficiency over the conventional PID controller, FLSs have been attempted to combine with PID controllers in two ways: (ⅰ) FLS has been employed to adjust the PID coefficients in an online way. In this approach which is known as gain adjusting PID by the use of fuzz logic (FGSPID), the inputs for FLS are considered to be the closed-loop error and its derivative, and according to the defined rules, the FLS determines the PID gains instantaneously. In this approach, the linear PID produces the control command to the controlled system (ⅱ) in the second approach, the fuzzy rules are used for establishing a nonlinear PID. This approach is known as the FPID controller. Considering I/O relations, an FPID is analogous to linear PID, except that the FPID is an appropriate method for nonlinear systems. The common block of the FPID is provided in Figure 3 [26]. The membership functions (MFs) for inputs are depicted in Figure 4. For each input, 3 MFs are considered. To design the MFs just the upper and lower bounds of signals are taken into account. The parameters of MFs can also be optimized [35].
In the diagram, u represents the controller applied to the controlled system, e is the regulation/tracking error, uf is the output signal of FC, α/β are the OSFs, and K1 and K2 are the ISCs. The FPID output can be regarded as follows:
u(t)=αuf(t)+β∫t0uf(t)dt. | (3.1) |
In [26] it is shown that the I/O relation of the FPID controller, like the classical PID controller, has the integral, derivative and proportional terms considering that this controller has a nonlinear characteristic and, therefore, it has been successfully utilized in controlling nonlinear and uncertain systems [5]. As mentioned earlier, the FPID controller can be realized using T1FLSs, IT2FLSs, and GT2FLSs. Since GT2FLSs are much more resilient dealing with noise and uncertainties, in this study, the designed FPID controller has been realized using this type FC.
Since in this problem, we have a high level of uncertainties the T2FLSs are used. The T2FLSs can handle more levels of uncertainty. In this paper, a T2FLS determines the parameters of a PI as shown in Figure 3.
As mentioned earlier, the proper adjustment of the FPID controller i.e., α, β, K1, and K2, can enhance the efficiency [36]. Evolutionary optimization methods such as PSO and GA are one of the common offline methods in adjusting these parameters. In these scenarios, the FPID parameters are evaluated in a feasible area of response until the defined lost function is reduced. For a step reference input, the value of peak overshoot/undershoot, rise time, and settling-time are some important specifications to describe the transient performance. Disturbance rejection can be identified by presenting the peak overshoot/undershoot and settling-time. In order to establish the mentioned steady-state and transient performances using the evolutionary optimization methods, the performance indices based on various functions of the error are desired. Common performance indices including an error function in [0,∞) have been presented as the integral
I=∫∞0fk(e(t))dt | (4.1) |
where fk(.) can takes various forms of error such as error. For example, using absolute error and square error as performance index penalize large errors more heavily than small ones which provide a longer settling time. By considering (2) as an OB, it is not possible to optimize the closed-loop performance characteristics i.e., the value of maximum overshoot/undershoot, rising-time, and settling time, at the same time. The reason for this is that some of these objectives are in conflict with each other and improving one of them leads to making the other worthwhile. Therefore, considering the objectives to be optimized, the tuning procedure of the FPID controller parameters can be regarded as a MO optimization problem. In this optimization method, several conflicting goals must be optimized simultaneously. Reducing the amount of the rise time (or settling time) will certainly raise the value of the peak overshoot of an optimized design. So, for MO problems, there is not a single solution, but with one run, we achieve a group of solutions, which is a kind of trade-off between the goals that must be optimized. It should be noted that in this paper α, β, K1 and K2 are the decision variables (it can be represented by vector x) and the settling time f1(x) and the value of peak overshoot/undershoot (f2(x)) are the two conflicting objectives (see Figure 5).
Based on the relative importance of each objective (f1 and f2), each point of this curve is a solution for the decision variables, i.e., α, β, K1 and K2. For example, if point A with the decision variables (solution) xA is chosen, it means that, from the solutions obtained in the Pareto front, the closed-loop system with the lowest amount of overshoot/undershoot and the maximum amount of settling time has been preferred. If point B with the decision variables xB is chosen for the controller parameters, an output system with the lowest values for both objectives can be achieved. According to the Pareto front and unlike the single objective optimization methods, it is clear that in the MO methods a set of responses (located in the Pareto front) is obtained in which the controller designer can select the appropriate parameters on the basis of the relative importance of the objectives.
To solve the MO problem, some approaches that essentially depend on PSO and GA techniques were introduced [17,19]. For example, Non-dominated sorting GA (NSGA-Ⅱ) [3] and Niched Pareto GA (NPGA) [24] algorithms are based on GA technique. Also, strength Pareto evolutionary algorithm (SPEA) [11], adaptive weighted PSO (AWPSO) [18] algorithms are based on the PSO technique.
The PSO is based on population search and it depends on the simulation of bird behavior. Some methods have been suggested to increase the accuracy of the basic PSO. One of these methods is the adaptive weighted PSO (AWPSO) algorithm; the velocity equation of the basic PSO algorithm in it is changed as follows (see the main general flowchart in Figure 6):
→vi(t)=w→vi(t−1)+ρ[c1r1(→Pbi−→xi(t−1))+c2r2(→Pg−→xi(t−1))] | (5.1) |
where, →xi shows the position of Pi at t. In this algorithm, ρ is the acceleration variable as:
ρ=ρ0+tT. | (5.2) |
Defining an aggregate OB is examined as one of the straightforward methods to tackle the MO problems. To get optimum solutions, single objective optimization algorithms, without any revision to these algorithms, can then be utilized. An aggregation method is used to introduce the evaluation function, Eval, for MO problem as follows [7]:
Eval(k)=n∑i=1θifi(k),n∑i=1θi=1 | (5.3) |
for k-th particle, n denotes number of OBs and θi denotes the related parameters for each OB. This Eval function can be normalized as follows:
θi=μi∑ni=1μj | (5.4) |
where μi and μj are uniform random numbers in the interval [0, 1].
Remark 1. To apply the AWPSO algorithm to our control problem, the various values for the parameters of suggested FPID are considered as the possible solution (population) of the AWPSO algorithm. Then, at each sample time, the best solution of AWPSO is considered as the coefficient of our controller.
The IEEE 39-bus test system (see Figure 7 [28]) is used to evaluate the efficiency of the presented controller for the fuzzy logic controller(FLC) under three different scenarios. The parameters are given in Table 1. The first column of Table 1 indicates the restrictions on the performance of output signals. For example, to have a minimum overshoot and maximum settling time, the values of parameters are α=1.63, β=0.186, K1=2.98, and K2=3.4. The demand response (DR) was obtained as 98.77, 22.21, and 54.6 MW in the first, second and third areas, respectively. Two main scenarios have been discussed in this paper. In the first scenario, the effect of load changes is investigated, and in the second one, the effect of wind speed is examined.
α | β | K1 | K2 | |
Maximum settling time, Minimum overshoot | 1.63 | 0.186 | 2.98 | 3.4 |
Mediocre | 2.9 | 0.12 | 1.186 | 3.86 |
Minimum settling time, Maximum overshoot | 2.66 | 0.109 | 0.86 | 4.28 |
The load changes are investigated as a disturbance. Figure 8 shows the load change considered in the study. We see that load is changed the time t = 30 s, from 0 to 8 MV. It can be considered as a high disturbance. Figure 9 compares frequency regulation in the first, second and third areas with other methods. Figure 9 shows that frequency changes tended to 0 in all three areas using the proposed method. The comparison condition for the suggested method, PI controller based on type-1 FLC (T1-FLC), and PI controller based on type-2 FLC (T2-FLC) [29] are the same. It means that the rules are the same for all three controllers.
The results revealed performance of the suggested method was superior to other approaches. Furthermore, the effect of wind speed, which varies over time, on the performance of the controller in frequency regulation was investigated in this scenario. Figure 10 indicates the speed change diagram. We see that the wind speed is randomly changed, and it can be considered as a high perturbation. Figure 11 examines the proposed controller performance in frequency regulation. The proposed controller had good performance in resisting wind speed changes over time and was superior to other methods.
The results show that the suggested LFC adequately resists the uncertainties, such as wind speed changes, DR changes, and uncertain dynamics. The main reason is that the parameters of the PI are not fixed, but they are adaptively changed. Also, the suggested optimization scheme finds suitable rules for T2FLS.
Remark 2. The primary focus of this study is on the LFC problem. But, the designed controller does not use dynamics and can be easily extended for other problems in MGs. Also, the suggested controller can be improved using new PIDs and fuzzy controllers [37,38,39,40,41,42].
The PIDs are widely used for load frequency control problems. But the main problem is their accuracy in the verses of high uncertainties. Also, in most of the existing studies, just the tracking error is considered, and the transient response is neglected. In this study, a novel technique to frequency control in MGs is proposed. A multi-objective optimization approach is proposed, as well as a novel PID controller. The developed controller is implemented in a case-study multi-area MG, and its performance is assessed under perturbations. In the first case, a rapid change in output load is examined, in addition to unknown dynamics. It is demonstrated that the proposed PID controller effectively manages perturbations and stabilizes the plant. The wind turbines are also supposed time-varying in the second assessment, in addition to dynamic uncertainty and load fluctuations. The results, as well as comparisons with other FPID approachers, reveal that the introduced PID has satisfactory regulatory results. The main disadvantage of the suggested approach is the computation process of the optimization, which can be simplified for future studies.
This research is financially supported by the Ministry of Science and Technology of China (Grant No. 2019YFE0112400), the Department of Science and Technology of Shandong Province (Grant No. 2021CXGC011204)
The authors declare no conflict of interest.
[1] | N. Cherrat, H. Boubertakh, H. Arioui, Adaptive fuzzy PID control for a class of uncertain mimo nonlinear systems with dead-zone inputs' nonlinearities, IJST-T. Electr. Eng., 42 (2018), 21–39. |
[2] |
Y. Arya, Agc of two-area electric power systems using optimized fuzzy pid with filter plus double integral controller, J. Franklin I., 355 (2018), 4583–4617. https://doi.org/10.1016/j.jfranklin.2018.05.001 doi: 10.1016/j.jfranklin.2018.05.001
![]() |
[3] |
K. Deb, A. Pratap, S. Agarwal, T. Meyarivan, A fast and elitist multiobjective genetic algorithm: Nsga-Ⅱ, IEEE T. Evolut. Comput., 6 (2002), 182–197. https://doi.org/10.1109/4235.996017 doi: 10.1109/4235.996017
![]() |
[4] |
M. K. Debnath, T. Jena, S. K. Sanyal, Frequency control analysis with PID-fuzzy-PID hybrid controller tuned by modified gwo technique, Int. T. Electr. Energy, 29 (2019), e12074. https://doi.org/10.1002/2050-7038.12074 doi: 10.1002/2050-7038.12074
![]() |
[5] |
M. El-Bardini, A. M. El-Nagar, Interval type-2 fuzzy PID controller for uncertain nonlinear inverted pendulum system, ISA T., 53 (2014), 732–743. https://doi.org/10.1016/j.isatra.2014.02.007 doi: 10.1016/j.isatra.2014.02.007
![]() |
[6] |
R. M. Elavarasan, The motivation for renewable energy and its comparison with other energy sources: A review, Eur. J. Sustain. Dev., 3 (2019), em0076. https://doi.org/10.20897/ejosdr/4005 doi: 10.20897/ejosdr/4005
![]() |
[7] | A. P. Engelbrecht, Computational intelligence: An introduction, John Wiley & Sons, 2007. |
[8] |
B. Firouzi, K. A. Alattas, M. Bakouri, A. K. Alanazi, A. Mohammadzadeh, S. Mobayen, et al., A type-2 fuzzy controller for floating tension-leg platforms in wind turbines, Energies, 15 (2022), 1705. https://doi.org/10.3390/en15051705 doi: 10.3390/en15051705
![]() |
[9] |
M. Gheisarnejad, An effective hybrid harmony search and cuckoo optimization algorithm based fuzzy PID controller for load frequency control, Appl. Soft Comput., 65 (2018), 121–138. https://doi.org/10.1016/j.asoc.2018.01.007 doi: 10.1016/j.asoc.2018.01.007
![]() |
[10] |
A. H. G. Haroun, Y. Li, A novel optimized hybrid fuzzy logic intelligent PID controller for an interconnected multi-area power system with physical constraints and boiler dynamics, ISA T., 71 (2017), 364–379. https://doi.org/10.1016/j.isatra.2017.09.003 doi: 10.1016/j.isatra.2017.09.003
![]() |
[11] |
S. Jiang, S. Yang, A strength pareto evolutionary algorithm based on reference direction for multiobjective and many-objective optimization, IEEE T. Evolut. Comput., 21 (2017), 329–346. https://doi.org/10.1109/TEVC.2016.2592479 doi: 10.1109/TEVC.2016.2592479
![]() |
[12] |
B. Jimada-Ojuolape, J. Teh, Surveys on the reliability impacts of power system cyber-physical layers, Sustain. Cities Soc., 62 (2020), 102384. https://doi.org/10.1016/j.scs.2020.102384 doi: 10.1016/j.scs.2020.102384
![]() |
[13] | H. Bevrani, Robust power system frequency control, New York, Springer, 4 (2014). |
[14] |
D. Khamari, R. K. Sahu, S. Panda, A modified moth swarm algorithm-based hybrid fuzzy PD-PI controller for frequency regulation of distributed power generation system with electric vehicle, J. Control Autom. Elec., 31 (2020), 675–692. https://doi.org/10.1007/s40313-020-00565-0 doi: 10.1007/s40313-020-00565-0
![]() |
[15] |
M. H. Khooban, T. Niknam, A new intelligent online fuzzy tuning approach for multi-area load frequency control: Self adaptive modified bat algorithm, Int. J. Elec. Power, 71 (2015), 254–261. https://doi.org/10.1016/j.ijepes.2015.03.017 doi: 10.1016/j.ijepes.2015.03.017
![]() |
[16] |
A. Kumar, V. Kumar, Evolving an interval type-2 fuzzy pid controller for the redundant robotic manipulator, Expert Syst. Appl., 73 (2017), 161–177. https://doi.org/10.1016/j.eswa.2016.12.029 doi: 10.1016/j.eswa.2016.12.029
![]() |
[17] | M. Mahfouf, M. Y. Chen, D. A. Linkens, Adaptive weighted particle swarm optimisation for multi-objective optimal design of alloy steels, In: International conference on parallel problem solving from nature, Springer, 2004,762–771. |
[18] |
A. Nickabadi, M. M. Ebadzadeh, R. Safabakhsh, A novel particle swarm optimization algorithm with adaptive inertia weight, Appl. Soft Comput., 11 (2011), 3658–3670. https://doi.org/10.1016/j.asoc.2011.01.037 doi: 10.1016/j.asoc.2011.01.037
![]() |
[19] | J. Horn, N. Nafpliotis, D. E. Goldberg, Multiobjective optimization using the niched pareto genetic algorithm, IlliGAL Report, 93005 (1993), 61801–2296. |
[20] |
K. Sabahi, S. Ghaemi, S. Pezeshki, Gain scheduling technique using mimo type-2 fuzzy logic system for lfc in restructure power system, Int. J. Fuzzy Syst., 19 (2017), 1464–1478. https://doi.org/10.1007/s40815-016-0240-7 doi: 10.1007/s40815-016-0240-7
![]() |
[21] |
K. Sabahi, M. Tavan, A. Hajizadeh, Adaptive type-2 fuzzy pid controller for lfc in ac microgrid, Soft Comput., 25 (2021), 7423–7434. https://doi.org/10.1007/s00500-021-05704-6 doi: 10.1007/s00500-021-05704-6
![]() |
[22] |
B. K. Sahu, S. Pati, P. K. Mohanty, S. Panda, Teaching-learning based optimization algorithm based fuzzy-pid controller for automatic generation control of multi-area power system, Appl. Soft Comput., 27 (2015), 240–249. https://doi.org/10.1016/j.asoc.2014.11.027 doi: 10.1016/j.asoc.2014.11.027
![]() |
[23] |
J. Z. Shi, A fractional order general type-2 fuzzy pid controller design algorithm, IEEE Access, 8 (2020), 52151–52172. https://doi.org/10.1109/ACCESS.2020.2980686 doi: 10.1109/ACCESS.2020.2980686
![]() |
[24] | V. Tongur, E. Üker, B-spline curve knot estimation by using niched pareto genetic algorithm (npga), Intelligent and evolutionary systems, Springer, 2016,305–316. |
[25] |
E. Yesil, Interval type-2 fuzzy pid load frequency controller using big bang-big crunch optimization, Appl. Soft Comput., 15 (2014), 100–112. https://doi.org/10.1016/j.asoc.2013.10.031 doi: 10.1016/j.asoc.2013.10.031
![]() |
[26] | E. Yeşil, M. Güzelkaya, İ. Eksin, Self tuning fuzzy pid type load and frequency controller, Energ. Convers. Manage., 45 (2004), 377–390. |
[27] |
J. Ziegler, N. Nichols, Optimum settings for automatic controllers, J. Dyn. Syst. Meas. Control, 115 (1993). https://doi.org/10.1115/1.2899060 doi: 10.1115/1.2899060
![]() |
[28] |
A. D. Shakibjoo, M. Moradzadeh, S. U. Din, A. Mohammadzadeh, A. H. Mosavi, L. Vandevelde, Optimized type-2 fuzzy frequency control for multi-area power systems, IEEE Access, 10 (2021), 6989–7002. https://doi.org/10.1109/ACCESS.2021.3139259 doi: 10.1109/ACCESS.2021.3139259
![]() |
[29] |
A. Mohammadzadeh, M. H. Sabzalian, A. Ahmadian, N. Nabipour, A dynamic general type-2 fuzzy system with optimized secondary membership for online frequency regulation, ISA T., 112 (2021), 150–160. https://doi.org/10.1016/j.isatra.2020.12.008 doi: 10.1016/j.isatra.2020.12.008
![]() |
[30] |
D. Li, S. S. Ge, T. H. Lee, Fixed-time-synchronized consensus control of multiagent systems, IEEE T. Control Netw., 8 (2021), 89–98. https://doi.org/10.1109/TCNS.2020.3034523 doi: 10.1109/TCNS.2020.3034523
![]() |
[31] |
R. Ye, P. Liu, K. Shi, B. Yan, State damping control: A novel simple method of rotor UAV with high performance, IEEE Access, 8 (2020), 214346–214357. https://doi.org/10.1109/ACCESS.2020.3040779 doi: 10.1109/ACCESS.2020.3040779
![]() |
[32] |
K. Liu, Z. Yang, W. Wei, B. Gao, D. Xin, C. Sun, et al., Novel detection approach for thermal defects: Study on its feasibility and application to vehicle cables, High Volt., 2022, 1–10. https://doi.org/10.1049/hve2.12258 doi: 10.1049/hve2.12258
![]() |
[33] |
J. Wang, J. Tian, X. Zhang, B. Yang, S. Liu, L. Yin, et al., Control of time delay force feedback teleoperation system with finite time convergence, Front. Neurorobotics, 2022. https://doi.org/10.3389/fnbot.2022.877069 doi: 10.3389/fnbot.2022.877069
![]() |
[34] |
S. Lu, Y. Ban, X. Zhang, B. Yang, S. Liu, L. Yin, et al., Adaptive control of time delay teleoperation system with uncertain dynamics, Front. Neurorobotics, 2022. https://doi.org/10.3389/fnbot.2022.928863 doi: 10.3389/fnbot.2022.928863
![]() |
[35] |
X. Qin, Z. Liu, Y. Liu, S. Liu, B. Yang, L. Yin, et al., User OCEAN personality model construction method using a BP neural network, Electronics, 11 (2022), 3022. https://doi.org/10.3390/electronics11193022 doi: 10.3390/electronics11193022
![]() |
[36] |
H. Zheng, S. Jin, A multi-source fluid queue based stochastic model of the probabilistic offloading strategy in a MEC system with multiple mobile devices and a single MEC server, Int. J. Appl. Math. Comput. Sci., 32 (2022), 125–138. https://doi.org/10.34768/amcs-2022-0010 doi: 10.34768/amcs-2022-0010
![]() |
[37] |
M. Sharma, S. Dhundhara, Y. Arya, S. Prakash, Frequency excursion mitigation strategy using a novel COA optimised fuzzy controller in wind integrated power systems, IET Renew. Power Gen., 14 (2020), 4071–4085. https://doi.org/10.1049/iet-rpg.2020.0882 doi: 10.1049/iet-rpg.2020.0882
![]() |
[38] |
Y. Arya, N. Kumar, Fuzzy gain scheduling controllers for automatic generation control of two-area interconnected electrical power systems, Electr. Pow. Compo. Sys., 44 (2016), 737–751. https://doi.org/10.1080/15325008.2015.1131765 doi: 10.1080/15325008.2015.1131765
![]() |
[39] |
E. Çelik, N. Öztürk, Y. Arya, C. Ocak, (1+PD)-PID cascade controller design for performance betterment of load frequency control in diverse electric power systems, Neural Comput. Appl., 33 (2021), 15433–15456. https://doi.org/10.1080/15325008.2015.1131765 doi: 10.1080/15325008.2015.1131765
![]() |
[40] |
A. Yogendra, N. Kumar, AGC of a two-area multi-source power system interconnected via AC/DC parallel links under restructured power environment, Optim. Contr. Appl. Met., 37 (2016), 590–607. https://doi.org/10.1002/oca.2181 doi: 10.1002/oca.2181
![]() |
[41] |
S. Mustafa, A. A. Bajwa, S. Iqbal, A new fuzzy grach model to forecast stock market technical analysis, Oper. Res. Eng. Sci., 5 (2022), 185–204. https://doi.org/10.31181/oresta040422196m doi: 10.31181/oresta040422196m
![]() |
[42] |
M. Riaz, H. M. A. Farid, Picture fuzzy aggregation approach with application to third-party logistic provider selection process, Rep. Mech. Eng., 3 (2022), 318–327. https://doi.org/10.31181/rme20023062022r doi: 10.31181/rme20023062022r
![]() |
1. | Jing Zhang, Feifei Peng, Lulu Wang, Yang Yang, Yingna Li, A load frequency control strategy based on double deep Q-network and upper confidence bound algorithm of multi-area interconnected power systems, 2024, 120, 00457906, 109778, 10.1016/j.compeleceng.2024.109778 | |
2. | Krzysztof Wiktorowicz, T2RFIS: type-2 regression-based fuzzy inference system, 2023, 35, 0941-0643, 20299, 10.1007/s00521-023-08811-7 | |
3. | Ghadeer Ghazi Shayea, Mohd Hazli Mohammed Zabil, A. S. Albahri, Shahad Sabbar Joudar, Rula A. Hamid, O. S. Albahri, A. H. Alamoodi, Idrees A. Zahid, Iman Mohamad Sharaf, Fuzzy Evaluation and Benchmarking Framework for Robust Machine Learning Model in Real-Time Autism Triage Applications, 2024, 17, 1875-6883, 10.1007/s44196-024-00543-3 | |
4. | Gerardo Maximiliano Méndez, Ismael López-Juárez, María Aracelia Alcorta García, Dulce Citlalli Martinez-Peon, Pascual Noradino Montes-Dorantes, The Enhanced Wagner–Hagras OLS–BP Hybrid Algorithm for Training IT3 NSFLS-1 for Temperature Prediction in HSM Processes, 2023, 11, 2227-7390, 4933, 10.3390/math11244933 |
α | β | K1 | K2 | |
Maximum settling time, Minimum overshoot | 1.63 | 0.186 | 2.98 | 3.4 |
Mediocre | 2.9 | 0.12 | 1.186 | 3.86 |
Minimum settling time, Maximum overshoot | 2.66 | 0.109 | 0.86 | 4.28 |