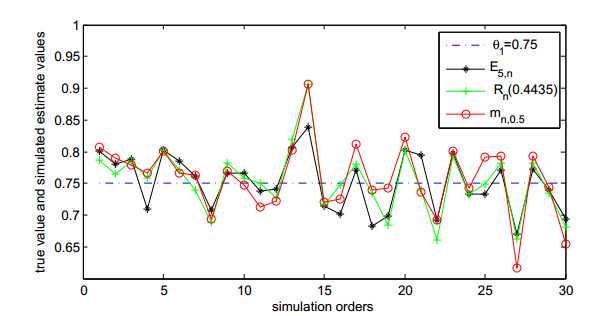
By the relationship between a continuous population X and the uniform distribution U[0,1], we gain for a sample quantile an equivalent expression of its variance and for two different sample quantiles the asymptotic correlation coefficient. As the population of interest can have no expectation, the obtained conclusions are applicable to the location estimating problem of a Cauchy distribution. On that occasion, we finally obtained a quick and effective estimator established by a linear function of some sample quantiles. For similar problems, the presented approach is worthy of reference.
Citation: Jinliang Wang, Fang Wang, Songbo Hu. On asymptotic correlation coefficient for some order statistics[J]. AIMS Mathematics, 2023, 8(3): 6763-6776. doi: 10.3934/math.2023344
[1] | Jin-liang Wang, Chang-shou Deng, Jiang-feng Li . On moment convergence for some order statistics. AIMS Mathematics, 2022, 7(9): 17061-17079. doi: 10.3934/math.2022938 |
[2] | H. M. Barakat, M. A. Alawady, I. A. Husseiny, M. Nagy, A. H. Mansi, M. O. Mohamed . Bivariate Epanechnikov-exponential distribution: statistical properties, reliability measures, and applications to computer science data. AIMS Mathematics, 2024, 9(11): 32299-32327. doi: 10.3934/math.20241550 |
[3] | Danhua He, Liguang Xu . Boundedness analysis of non-autonomous stochastic differential systems with Lévy noise and mixed delays. AIMS Mathematics, 2020, 5(6): 6169-6182. doi: 10.3934/math.2020396 |
[4] | Xiaoyan Zhou, Mingwei Lin, Weiwei Wang . Statistical correlation coefficients for single-valued neutrosophic sets and their applications in medical diagnosis. AIMS Mathematics, 2023, 8(7): 16340-16359. doi: 10.3934/math.2023837 |
[5] | H. M. Barakat, M. H. Dwes . Asymptotic behavior of ordered random variables in mixture of two Gaussian sequences with random index. AIMS Mathematics, 2022, 7(10): 19306-19324. doi: 10.3934/math.20221060 |
[6] | Salim Bouzebda, Amel Nezzal, Issam Elhattab . Limit theorems for nonparametric conditional U-statistics smoothed by asymmetric kernels. AIMS Mathematics, 2024, 9(9): 26195-26282. doi: 10.3934/math.20241280 |
[7] | Salim Bouzebda, Amel Nezzal . Uniform in number of neighbors consistency and weak convergence of kNN empirical conditional processes and kNN conditional U-processes involving functional mixing data. AIMS Mathematics, 2024, 9(2): 4427-4550. doi: 10.3934/math.2024218 |
[8] | Salim Bouzebda . Weak convergence of the conditional single index U-statistics for locally stationary functional time series. AIMS Mathematics, 2024, 9(6): 14807-14898. doi: 10.3934/math.2024720 |
[9] | Mohammad Faisal Khan, Jongsuk Ro, Muhammad Ghaffar Khan . Sharp estimate for starlikeness related to a tangent domain. AIMS Mathematics, 2024, 9(8): 20721-20741. doi: 10.3934/math.20241007 |
[10] | Muhammad Ghaffar Khan, Sheza.M. El-Deeb, Daniel Breaz, Wali Khan Mashwani, Bakhtiar Ahmad . Sufficiency criteria for a class of convex functions connected with tangent function. AIMS Mathematics, 2024, 9(7): 18608-18624. doi: 10.3934/math.2024906 |
By the relationship between a continuous population X and the uniform distribution U[0,1], we gain for a sample quantile an equivalent expression of its variance and for two different sample quantiles the asymptotic correlation coefficient. As the population of interest can have no expectation, the obtained conclusions are applicable to the location estimating problem of a Cauchy distribution. On that occasion, we finally obtained a quick and effective estimator established by a linear function of some sample quantiles. For similar problems, the presented approach is worthy of reference.
Let a random sample X1,...,Xn be drawn from a population X according to a distribution F(x,θ) where θ is unknown. If a statistic ˆθn is unbiased for θ and there are known positive constants bn's such that the normalized random sequence {(ˆθn−θ)/bn,n≥1} converges in both the first and the second moment to a known distribution of a random variable (RV), say ξ, with a known variance σ2, then the effectiveness of the unbiased estimator ˆθn can be assessed by the variance b2nσ2, the less the variance, the more effective the estimator. However, the Cramér-Rao inequality indicates that to estimate the unknown parameter θ of the distribution F(x,θ), the unbiased estimator usually has a variance not less than 1/(nI(θ)) where I(θ) denotes the Fisher information. That indicates that an unbiased estimator with a variance reaching the lower bound 1/(nI(θ)) is sure of minimum variance.
Under a large sample size, the maximum likelihood estimate (m.l.e) method usually (but not always) yields a theoretically desirable estimator, say ^θn, in a sense that ^θn has an asymptotic normal distribution N(θ,1/(nI(θ))) with a variance reaching the lower bound of the well-known Cramér-Rao inequality (see [1] as a reference).
For estimating a parameter of the distribution of a population that has no expectation, the classical moment estimate method is futile. Moreover, the classical method of m.l.e usually becomes invalid too in the sense that it doesn't have a closed solution. Under such a situation, especially in the case of estimating some parameters such as the location of a population, it is worth trying to investigate an unbiased estimator established by a linear function of some sample quantiles. That will be preferable if the efficiency is close to that of the theoretical m.l.e. To approximate the efficiency of the estimator, we need the following conclusions.
Theorem 1.1. For a population X distributed according to a continuous pdf f(x), let p and r be two numbers satisfying 0<p≤r<1 and xp and xr be respectively the p-quantile and r-quantile of X satisfying f(xp)f(xr)>0. Let (X1,...,Xn) be a random sample derived from X. If there are constants ω>0 and ν∈(−∞,∞) such that the cdf F(x) of ωX+ν has an inverse function Q(u) which possesses a continuous third-order derivative function Q‴(u) in the interval (0,1) satisfying
|Q‴(u)|≤Ku−A(1−u)−A | (1.1) |
for some given constants K>0, A≥0 and all u∈(0,1), then:
(1) we have, as n→∞,
E(f(xp)(Xi:n−xp)√p(1−p)/n)2∼E(f(xr)(Xj:n−xr)√r(1−r)/n)2→1 |
provided i/n=p+o(n−1/2) and j/n=r+o(n−1/2) as n→+∞;
(2) the correlation coefficient corr(Xi:n,Xj:n) between Xi:n and Xj:n satisfies
limn→∞corr(Xi:n,Xj:n)=√p(1−r)r(1−p) | (1.2) |
provided i/n=p+o(1) and j/n=r+o(1) as n→∞.
Under the conditions in Theorem 1.1 but without assumption (1.1), it is mentioned (without formal proof) in [2] that the same conclusions hold according to some equations given. We have found a gap there, that is, [2] uses a partial sum of a Taylor expansion of a function to approximate the function itself without rigorous proof. Here we have to apply the assumption (1.1) to fill that gap.
In exploring the measurement of dependence or independence between two order statistics (OSs), many research works based on the Copula function method are instructive. On that subject, Barakat led the research. For references, we can consult Barakat's [3,4,5] and Hürlimann's [6] as well.
Item (2) in Theorem 1.1 can be regarded as a corresponding exploration of the relation between two general OSs, where we measure the asymptotic dependence or independence by providing the limiting correlation coefficient for them. That has two main advantages. First, this preferred measurement has its advantage in that the respective normalization of both OSs has no effect. Second, as was discovered by Bahadur (see [7]) or as summarized by DasGupta in [8], the asymptotic joint distributions of some sample quantiles have a multivariate normal distribution while, for two RVs according to a bivariate normal distribution, being independent is equivalent to being uncorrelated.
Under the conditions of Theorem 1.1, we see that the OSs Xi:n and Xj:n are asymptotically dependent, which supports Barakat's corresponding conclusion in article [3]. Moreover, Theorem 1.1 also supports the exclamation in [9] stating that the dependence between Xi:n and Xj:n decreases as i and j draw apart.
To our studies, conditions in Theorem 1.1 are met for almost all continuous populations including the situation discussed in [10] from which we see that the correlation coefficient between a sample maximum and a sample minimum has a limiting value 0 as the sample size n tends to infinity. Here Theorem 1.1 deals with correlation coefficients for common OSs relevant to some general sample quantiles.
Now we use symbol ⌈z⌉ for the integer part of a positive number z and mn,p for the p-quantile of the random sample (X1,⋯,Xn). Namely mn,p=(Xpn:n+Xpn+1:n)/2 if pn is an integer and mn,p=X⌈pn+1⌉:n otherwise.
Remark 1.1. Assume the condition (1.1) of Theorem 1.1, then:
(1) Corresponding to the central limit theorem for sample quantiles, the following second moment convergence conclusion holds as n→∞,
E(f(xp)(mn,p−xp)√p(1−p)/n)2→1; |
(2) The asymptotic correlation coefficient corr(mn,p,mn,r) for mn,p and mn,r satisfies
limn→∞corr(mn,p,mn,r)=√p(1−r)r(1−p). |
Remark 1.2. For a given sample (X1,...,Xn), it is obvious that the correlation coefficient for two different sample quantiles is relevant to the distribution of the population X from which the sample is drawn. Here Theorem 1 indicates that, as the sample size n tends to infinity, the mentioned correlation coefficient is eventually free from the distribution of X.
Corollary 1.2. Under the condition (1.1) of Theorem 1.1, if we use the sample quantile mn,p as an estimator for the corresponding population quantile xp, then we have the following variance equivalence
Var(mn,p)∼p(1−p)n(f(xp))2. |
Moreover, if we further assume that F(xr)=r and F′(xr)=f(xr)>0 where 0<p≤r<1, then the covariance between mn,p and mn,r is
cov(mn,p,mn,r)=corr(mn,p,mn,r)√Var(mn,p)⋅Var(mn,r)∼p(1−r)nf(xp)f(xr). | (1.3) |
Generally, for real numbers u1,u2,...,uk and p1,p2,...,pk satisfying 0<p1<...<pk<1 and f(xp1)f(xp2)...f(xpk)>0, the following analogous expression holds
Var(k∑i=1uimn,pi)∼k∑i=1u2ipi(1−pi)n(f(xpi))2+∑1≤i<j≤k2uiujpi(1−pj)nf(xpi)f(xpj). | (1.4) |
For a positive integer r, we mean that a random sequence {ξn,n≥1} converges in an r-th order of moment if the number sequence {Eξrn,n≥1} converges. In reference [11], Wang et al. investigated moment convergence conclusions for some OSs connected to a general continuous population. It is found that not only the sequence of sample quantiles but also the corresponding standardized sequence converges in some positive order of moments even when the population of interest has no expectation. Here Theorem 1.1 is a subsequent exploration.
Lemma 2.1. (see [2]). Let Y be a population uniformly distributed over the interval [0,1]; Let Yi:n be the corresponding i-th OS of a random sample (Y1,⋯,Yn) from Y. For nonnegative integers u, v, i and j satisfying 1≤i<j≤n,
E(Yui:nYvj:n)=n!(i−1)!(u+i−1)!(u+j−1)!(v+u+j−1)!(v+u+n)!. |
Remark 2.1. Setting u, v, i and j to be some specified nonnegative integers, we can gain
EYui:n=n!(i−1)!(u+i−1)!(u+n)! | (2.1) |
and
cov(Yi:n,Yj:n)=(j+1)i(2+n)(1+n)−ij(n+1)2=i(n−j+1)(n+2)(n+1)2. | (2.2) |
Lemma 2.2. Now we denote μk:n=EYk:n=k/(n+1). Under the conditions of Lemma 2.1 and by Eq (2.1), we can conclude that for integer k satisfying k/n→ρ∈(0,1), as n→∞,
E(Yk:n−μk:n)2∼ρ(1−ρ)n;E(Yk:n−μk:n)4∼3ρ2(1−ρ)2n2 | (2.3) |
and
E(Yk:n−μk:n)6∼15ρ3(1−ρ)3n3. | (2.4) |
Proof. Denoting Y=F(X) and Yi=F(Xi), we see that Lemmas 2.1 and 2.2 are applicable.
According to the Taylor expansion formula,
Q(t)=Q(t0)+Q′(t0)(t−t0)+12!Q″(t0)(t−t0)2+13!Q‴(Δ)(t−t0)3 |
where Δ∈[min(t,t0),max(t,t0)], there exists some RVs τi:n satisfying
τi:n∈[min(μi:n,Yi:n),max(μi:n,Yi:n)] |
such that
Xi:n=Q(Yi:n)=Q(μi:n)+Q′(μi:n)(Yi:n−μi:n)+Q″(μi:n)(Yi:n−μi:n)22+Q‴(τi:n)(Yi:n−μi:n)36=:Q(μi:n)+part1+part2+part3. | (3.1) |
By the equivalent expressions in Lemma (2.2), we have
E(part21)=(Q′(μi:n))2E(Yi:n−μi:n)2∼(Q′(p))2p(1−p)n=O(n−1) | (3.2) |
and
E(part22)=14(Q″(μi:n))2E(Yi:n−μi:n)4∼(Q″(p))23p2(1−p)24n2=O(n−2). | (3.3) |
Moreover, by the assumption |Q‴(u)|≤Ku−A(1−u)−A, no matter if 0<Yi:n≤τi:n≤μi:n≤1 or 0<μi:n≤τi:n≤Yi:n≤1, we have
|Q‴(τi:n)|≤K[Yi:n−A(1−μi:n)−A⋅μi:n−A(1−Yi:n)−A]. |
Noting that the pdf of Yi:n is n!/((i−1)!(n−i)!)xi−1(1−x)n−iI[0,1](x), we see that
E[Q‴(τi:n)4]≤K4(1−μi:n)−4Aμi:n−4A⋅E[Yi:n−4A(1−Yi:n)−4A].=K4(1−μi:n)−4Aμi:n−4An!(i−1)!(n−i)!∫10xi−4A−1(1−x)n−i−4Adx=K4(1−μi:n)−4Aμi:n−4An!(i−1)!(n−i)!B(i−4A,n−i−4A+1)=K4(1−μi:n)−4Aμi:n−4An!(i−1)!(n−i)!Γ(i−4A)⋅Γ(n−i−4A+1)Γ(n−8A+1). | (3.4) |
Now let M be the nonnegative integer satisfying u=M−4A∈[0,1). By the formula (see [12]) Γ(n+α)∼nα(n−1)! where α>0, we have for i/n→p∈(0,1) as n→∞,
E[Q‴(τi:n)4]≤K4(1−μi:n)−4Aμi:n−4An!(i−1)!(n−i)!Γ(i−M+u)⋅Γ(n+1−i−M+u)Γ(n+1−2M+2u)∼K4(1−μi:n)−4Aμi:n−4An!(i−1)!(n−i)!(i−M)u(i−M−1)!(n+1−i−M)u(n−i−M)!(n+1−2M)2u(n−2M)!∼K4(1−p)−4Ap−4An!(i−1)!(n−i)!(i−M−1)!⋅(n−i−M)!(n−2M)!(i−M)u(n+1−i−M)u(n+1−2M)2u∼K4(1−p)−4Ap−4An!(i−1)!(n−i)!(i−M−1)!⋅(n−i−M)!(n−2M)!pu(1−p)u∼K4(1−p)u−4Apu−4An!(i−1)!(n−i)!(i−M−1)!⋅(n−i−M)!(n−2M)!. | (3.5) |
Furthermore, we can utilize the Stirling formula m!∼(m/e)m√2πm to obtain
K4(1−p)u−4Apu−4An!(i−1)!(n−i)!(i−M−1)!⋅(n−i−M)!(n−2M)!=K4(1−p)u−4Apu−4An(n−1)...(n−(2M−1))(i−1)...(i−M)(n−i)...(n−i−(M−1)) | (3.6) |
∼K4(1−p)u−4Apu−4An2MiM(n−i)M | (3.7) |
→K4(1−p)u−4Apu−4A(p(1−p))−M=K4(1−p)−8Ap−8A. | (3.8) |
According to (3.5) and (3.8) and by using Liapunov's inequality in the form E(ξ2)≤[E(ξ4)]1/2, we see that there exists a positive constant R>0 such that the inequality
E[Q‴(τi:n)4]≤R | (3.9) |
holds uniformly with respect to n≥1. By the Cauchy-Schwarz inequality [E(ξη)]2≤Eξ2⋅Eη2 and Lemma 2.2 as well as the fact |Yi:n−μi:n|≤1, we see that
E(part23)=136E{[Q‴(τi:n)]2(Yi:n−μi:n)6}≤136E{[Q‴(τi:n)]2|Yi:n−μi:n|3}≤136√E[Q‴(τi:n)]4E(Yi:n−μi:n)6=O(n−3/2). | (3.10) |
Similarly
|E(part3)|=16|EQ‴(τi:n)(Yi:n−μi:n)3|≤16√E[Q‴(τi:n)]2⋅E(Yi:n−μi:n)6 | (3.11) |
≤16√√R⋅E(Yi:n−μi:n)6=O(n−3/2). | (3.12) |
Combining the conclusions (3.1) and (3.11), we get
EXi:n=EQ(Yi:n)=Q(μi:n)+Q″(μi:n)2Var(Yi:n)+o(n−1). | (3.13) |
Similarly to (3.1), there exists some RV αj:n∈[min(μj:n,Yj:n),max(μj:n,Yj:n)] such that
Xj:n=Q(Yj:n)=Q(μj:n)+Q′(μj:n)(Yj:n−μj:n)+Q″(μj:n)(Yj:n−μj:n)22+Q‴(αj:n)(Yj:n−μj:n)36=:Q(μj:n)+PART1+PART2+PART3. | (3.14) |
Replacing i with j in (3.2), (3.3), (3.11) and (3.13) yields
E(PART21)∼(Q′(p))2r(1−r)n=O(n−1), | (3.15) |
E(PART22)∼(Q″(p))23r2(1−r)24n2=O(n−2), | (3.16) |
E(PART3)=O(n−3/2),E(PART23)=O(n−3/2) | (3.17) |
and
EXj:n=EQ(Yj:n)=Q(μj:n)+Q″(μj:n)2Var(Yj:n)+o(n−1). | (3.18) |
Moreover, we see that
|cov(part3,PART3)|≤√E(part23)⋅√E(PART23)=O(n−3/2), |
hence
cov(part3,PART3)=o(n−1). | (3.19) |
That results in the following conclusion according to Eqs (3.1) and (3.14):
cov(Xi:n,Xj:n)=∑1≤s≤3;1≤t≤3cov(parts,PARTt)=∑2≤t≤3cov(part1,PARTt)+∑1≤t≤3cov(part2,PARTt)+∑1≤t≤2cov(part3,PARTt)+cov(part1,PART1)+o(n−1). | (3.20) |
Now noting the equations numbered (3.2), (3.3), (3.11) and those from (3.15) to (3.17), we derive
|∑2≤t≤3cov(part1,PARTt)+∑1≤t≤3cov(part2,PARTt)+∑1≤t≤2cov(part3,PARTt)|≤∑2≤t≤3√E(part21)⋅E(PART2t)+∑1≤t≤3√E(part22)⋅E(PART2t)+∑1≤t≤2√E(part23)⋅E(PART2t)=o(n−1), | (3.21) |
from which we conclude that
∑2≤t≤3cov(part1,PARTt)+∑1≤t≤3cov(part2,PARTt)+∑1≤t≤2cov(part3,PARTt)=o(n−1). |
Substituting the corresponding part in (3.20) by the just obtained above result, we have
cov(Xi:n,Xj:n)=cov(part1,PART1)+o(n−1)=Q′(μi:n)Q′(μj:n)i(n−j+1)(n+2)(n+1)2+o(n−1). | (3.22) |
Referring to the procedure in obtaining conclusion (3.22), we can also reach the following conclusions
Var(Xi:n)=Var(part1)+o(n−1)=[Q′(μi:n)]2i(n+1−i)(n+2)(n+1)2+o(n−1) | (3.23) |
and
Var(Xj:n)=Var(PART1)+o(n−1)=[Q′(μj:n)]2j(n−j+1)(n+2)(n+1)2+o(n−1). | (3.24) |
(1) Now we notice that as n→∞,
Q(μi:n)=Q(in+1)=Q(p)+Q′(p)(in+1−p)+o((in+1−p)), |
therefore according to Eq (3.13), we have
limn→∞(f(xp)(EXi:n−xp)√p(1−p)/n)2=limn→∞(f(xp)[Q(μi:n)+Q″(μi:n)2Var(Yi:n)+o(n−1)−xp]√p(1−p)/n)2=limn→∞(f(xp)[Q″(μi:n)2Var(Yi:n)+o(n−1)+Q(μi:n)−Q(p)]√p(1−p)/n)2=limn→∞(f(xp)[Q″(μi:n)2Var(Yi:n)+o(n−1)+Q′(p)(in+1−p)+o((in+1−p))]√p(1−p)/n)2=0 |
provided i/n=p+o(n−1/2) which is equivalent to i/(n+1)=p+o(n−1/2). Consequently we see that
limn→∞E(f(xp)(Xi:n−xp)√p(1−p)/n)2=limn→∞E(f(xp)[(Xi:n−EXi:n)+(EXi:n−xp)]√p(1−p)/n)2=limn→∞[E(f(xp)(Xi:n−EXi:n)√p(1−p)/n)2+(f(xp)(EXi:n−xp)√p(1−p)/n)2]=limn→∞E(f(xp)(Xi:n−EXi:n)√p(1−p)/n)2=limn→∞(f(xp))2⋅Var(Xi:n)p(1−p)/n=limn→∞(f(xp))2[Q′(μi:n)]2i(n+1−i)(n+2)(n+1)2+o(n−1)p(1−p)/n=1 |
according to Eq (3.23). Here the reason for the last equation is that the continuous function Q′(u) is positive according to the deduction Q′(u)=1/F′(x)=1/f(x)>0 at x=xp.
(2) Combining the three conclusions (3.22)–(3.24), we get the asymptotic correlation coefficient corr(Xi:n,Xj:n) by the following procedures:
corr(Xi:n,Xj:n)=cov(Xi:n,Xj:n)√Var(Xi:n)√Var(Xj:n)=Q′(μi:n)Q′(μj:n)i(n−j+1)(n+2)(n+1)2+o(n−1)√[Q′(μi:n)]2i(n−i+1)(n+2)(n+1)2+o(n−1)√[Q′(μj:n)]2j(n−j+1)(n+2)(n+1)2+o(n−1)=Q′(μi:n)Q′(μj:n)i(n−j+1)(n+2)(n+1)2+o(n−1)|Q′(μi:n)Q′(μj:n)|√i(n−i+1)(n+2)(n+1)2+o(n−1)√j(n−j+1)(n+2)(n+1)2+o(n−1)n→∞→Q′(p)Q′(r)p(1−r)|Q′(p)Q′(r)|√p(1−p)√r(1−r)=√p(1−r)r(1−p) | (3.25) |
provided i/n=p+o(1) and j/n=r+o(1).
To continue our discussions, we give the following two propositions beforehand:
Proposition 1. If the inverse function Q(u) of a cdf F(x) has a third-order derivative Q‴(u), then
Q‴(u)=−f″(x)f(x)+3(f′(x))2(f(x))5, | (4.1) |
where x=Q(u) and f(x)=F′(x).
Proposition 2. For a function −ln(uA(1−u)A) (where A≥0 is a constant) and any specified constant ε>0, there exists a corresponding number C(ε)>0 such that the inequality −ln(uA(1−u)A)≤C(ε)(u(1−u))−ε holds for all u∈(0,1).
Although almost all commonly applied continuous types of populations satisfy the conditions in Theorem 1.1, due to length concerns in this section, we will present only one example of Theorem 1.1.
Example. For a population X with a gamma distribution (including special cases such as the Exponential as well as the Chi-square distributions), the pdf is
βαΓ(α)xα−1e−βxI[0,∞)(x),α>0,β>0. |
Corresponding to the case ω=β>0 and ν=0, we now assume x=Q(u) to be the inverse function of the cdf u=F(x) of βX, the pdf of which can be worked out as
f(x)=1Γ(α)xα−1e−xI[0,∞)(x). |
On that occasion, we can easily see that for x>0,
f′(x)=α−1−xxf(x)andf″(x)=1−α+(1−α+x)2x2f(x). | (4.2) |
Noting that it is easy to verify that Theorem 1.1 is applicable in the case α=1, we now assume that α∈(0,1)∪(1,+∞).
As a condition like (1.1) is equivalent to verifying the existence of a positive number q such that
limu→0+uqQ‴(u)=limu→1−(1−u)qQ‴(u)=0 |
and by Proposition 1, it is sufficient to verifying the conditions
limx→0(F(x))qf″(x)f(x)/f5(x)=limx→0(F(x))q(f′(x))2/f5(x)=0 | (4.3) |
and
limx→∞(1−F(x))qf″(x)f(x)/f5(x)=limx→∞(1−F(x))q(f′(x))2/f5(x)=0. | (4.4) |
Now we use the notation g(x)≍h(x) to mean that there are positive constants a<b such that a|g(x)|≤h(x)≤b|g(x)| as x→0+ or x→∞, according to context. Then
● Case 1. x→0+. We only need consider x confined in a sufficiently small interval (0,δ]. Obviously F(x)≍xα, f(x)≍xα−1, f′(x)≍xα−2 and f″(x)≍xα−3. It follows that conditions (4.3) are satisfied if a positive constant q satisfies q>3−1/α.
● Case 2. x→∞. On that occasion, we see according to (4.2) that the following relations hold simultaneously for a positive number q>3:
(1−F(x))qf″(x)f(x)/f5(x)∼(1−F(x))q/f3(x)∼(1−F(x))q−3→0 |
and
(1−F(x))q(f′(x))2/f5(x)∼(1−F(x))q/f3(x)∼(1−F(x))q−3→0. |
Consequently we see the realization of (4.3).
As above analyzed, gamma distributions satisfy condition (1.1).
The Cauchy distribution has a wide range of applications in physics, economics as well as in the medical domain. We may perceive its important application in physics by a simple model depicted as what follows: in a coordinate plane, if we place at a point (θ1,θ2) (where θ2>0) a radioactive material emitting a particle at a random angle U uniformly distributed over an interval [0,2π], then we can show that the particle will reach the abscissa axis at a point X distributed according to a pdf
f(x,θ1,θ2)=θ2π[θ22+(x−θ1)2],−∞<x<+∞ | (4.5) |
which is the pdf of a Cauchy distribution. The relevant kinds of literature are huge. For general introduction we recommend [13] whereas, for some elegant studies on a similar topic to this article, we consult references [14] and [15].
There also is a considerable literature on L-estimation, including determining optimal weights. Some of this is in the robustness literature. See [2] and [16] for more references.
On estimating the location θ1 in (4.5), Sen verified in [17] that the so-called mid-range (mn,0.56+mn,0.44)/2 is more effective than the sample median mn,0.5. By rejecting a fixed number of the largest and the smallest OSs to avoid a large mean squared error of the parameter estimator, Pekasiewicz utilized in [18] a method named the truncated quantile least squares method to estimate the location parameter θ1. Recently, Krykun [19] investigated estimating both θ1 and θ2, by resorting to an arctangent regression function and rejecting some fraction of the largest and the smallest OSs. Some ideal simulated results are obtained in [19]. Comparatively, what we present in the following exploration is a third way using optimal linear combinations of some sample quantiles.
To estimate θ1 in (4.5), we will, without loss of generality, set θ2=1.
Let (X1,...,Xn) be a random sample from a population X according to the pdf
f(x,θ)=1π((x−θ)2+1) | (4.6) |
with an unknown θ. As finding the uniformly minimum variance unbiased estimator (UMVUE) for θ is hopeless, we now think of the estimator
Rn(p):=mn,p+mn,1−p2 | (4.7) |
which is named as sample quasi-midrange (see [20]). It is trivial to see that Rn(r) is unbiased in estimating θ. According to Theorem 1, we see that
Var(Rn(p))=Var(mn,p)+Var(mn,1−p)+2corr(mn,p,mn,1−p)√Var(mn,p)⋅Var(mn,1−p)4=12(1−p)Var(mn,p)∼12(1−p)p(1−p)n(f(xp))2=p2n(f(xp))2=pπ2(1+x2p)22n=pπ2(1+cos2(πp)sin2(πp))22n=pπ22n⋅sin4(πp). | (4.8) |
As we can easily see that the equivalence for the variance of the sample median
Var(mn,0.5)∼π24n≈2.467401016n, |
the result of (4.8) seems to indicate that the unbiased estimator Rn(r) will be more effective than the sample median mn,0.5 if we can diminish the value r/sin4(πr). As the minimum value of r/sin4(πr) exists but can not be obtained as an explicit expression, here we make an approximation of the minimum value of r/sin4(πr) as 0.4724417292 when r=0.4435. By the equivalence (1.3) in Corollary 1.1, the estimator Rn(0.4435) is preferable for θ because the equivalent corresponding variance
Var(mn,0.4435+mn,1−0.44352)∼0.4435π22n⋅sin4(0.4435π)=2.332n |
is a bit smaller than that of the sample median. That is exactly the conclusion drawn in [17]. Moreover, for 0<p<r≤0.5 and t∈(−∞,+∞), we see that the estimator tRn(p)+(1−t)Rn(r) is also unbiased for θ and
Var(tRn(p)+(1−t)Rn(r))=t2Var(Rn(p))+(1−t)2Var(Rn(r))+2t(1−t)cov(Rn(p),Rn(r))∼t2pπ22n⋅sin4(πp)+(1−t)2rπ22n⋅sin4(πr)+t(1−t)2cov(mn,p+mn,1−p,mn,r+mn,1−r); |
According to equivalence (1.4) and by noting that f(xp)=f(x1−p) and f(xr)=f(x1−r), we obtain
cov(mn,p+mn,1−p,mn,r+mn,1−r)∼2pπ2nsin2(πp)sin2(πr) | (4.9) |
and thus for large n,
Var(tRn(p)+(1−t)Rn(r))∼t2pπ22n⋅sin4(πp)+(1−t)2rπ22n⋅sin4(πr)+t(1−t)pπ2nsin2(πp)sin2(πr)=π22n(t2psin4(πp)+(1−t)2rsin4(πr)+2t(1−t)psin2(πp)sin2(πr)). |
Generally, for two sequences of real numbers t1,...,tm and p1,...,pm respectively satisfying tm=1−∑m−1i=1ti and 0≤p1<p2<...<pm≤0.5, the linear combination ∑mi=1tiRn(pi) is an unbiased estimator for θ and the corresponding asymptotic variance is
Var(m∑i=1tiRn(pi))=m∑i=1Var(tiRn(pi))+2∑1≤i<j≤mtitjcov(Rn(pi),Rn(pj))∼π22n[m∑i=1t2ipisin4(πpi)+∑1≤i<j≤m2titjpisin2(πpi)sin2(πpj)]. | (4.10) |
For the unknown θ in the pdf of (4.6), to find an unbiased estimator of the form ˆθm,n=∑mi=1tiRn(pi) with minimum variance, what is left is just a matter of some calculations of finding the ti's and pi's such that the expression (4.10) attains its minimum value. For instance, by putting m=5 in (4.10) and by some numerical calculations, we obtain such an estimator defined by
E5,n=−0.0192Rn(0.0632)−0.0747Rn(0.1347)+0.2953Rn(0.3577)+0.3799Rn(0.4199)+0.4187Rn(0.4739). |
With the aid of Matlab software, the asymptotic variance can be shown to be Var(E5,n)∼2.0314/n. The estimator ˆθ5,n is unbiased and is better than the estimator Rn(0.4435), which was named the optimum mid-range estimator and was admitted in [17] as a superior estimator to the sample median in estimating θ. As p1=0.4435 can be determined numerically for the case m=1, among unbiased estimators Rn(p) in (4.7), Rn(0.4435) is the most efficient one such that (4.10) has a minimum variance when m=1 is specified.
The Fisher information I(θ)=1/2 for the Cauchy pdf (4.6), so we see that even if the UMVUE, say ˆθ∗n for θ exists, the theoretical variance Var(ˆθ∗n) can not be smaller than 2n according to the well-known Cramér-Rao inequality.
Noting that the quotient 22.0314≈0.9845 is close to 1, we see that the quick unbiased estimator E5,n is close to the theoretical ideal unbiased estimator.
To compare the effectiveness of estimating θ1 by the three mentioned estimators, namely, the median mn,0.5, the quasi-midrange Rn(0.4435) in (4.7) and the just discussed estimator E5,n, by the aid of Matlab software, we simulate 30 times a random sample of size n=200 drawn from a specified Cauchy distribution f(x,θ1,θ2)=θ2π[θ22+(x−θ1)2] with respective true values θ1=0.75 and θ2=2. According to the simulated results, Figure 1 shows the effectiveness of the three estimators in estimating θ1. The averaged squared errors for the three estimators are respectively, 0.0030, 0.0025 and 0.0019.
As is indicated by the simulated results, among the three estimators mn,0.5,E5,n and Rn(0.4435), the estimator E5,n is the most effective under the assumption of a large sample size.
This work was supported by the Science and Technology Plan Project of Jiangxi Province Health Commission (Grant NO. 202311165) and the National Natural Science Foundation of China (Grant NO. 81960618).
There exists no conflict of interest between authors.
[1] | J. Shao, Mathematical statistics, New York: Springer, 2010. |
[2] | H. David, H. Nagaraja, Order statistics, New Jersey: Wiley, 2003. http://dx.doi.org/10.1002/0471722162 |
[3] |
H. Barakat, Comments on the rate of convergence to asymptotic independence between order statistics, Stat. Probabil. Lett., 76 (2006), 35–38. http://dx.doi.org/10.1016/j.spl.2005.07.009 doi: 10.1016/j.spl.2005.07.009
![]() |
[4] | H. Barakat, Measuring the asymptotic dependence between generalized order statistics, J. Stat. Theory Appl., 6 (2007), 106–117. |
[5] |
H. Barakat, A nonparametric general criterion of asymptotic dependence between order statistics, Commun. Stat.-Theor. M., 38 (2009), 1960–1968. http://dx.doi.org/10.1080/03610920802689385 doi: 10.1080/03610920802689385
![]() |
[6] |
W. Hürlimann, On the rate of convergence to asymptotic independence between order statistics, Stat. Probabil. Lett., 66 (2004), 355–362. http://dx.doi.org/10.1016/j.spl.2003.10.020 doi: 10.1016/j.spl.2003.10.020
![]() |
[7] |
R. Bahadur, A note on quantiles in large samples, Ann. Math. Statist., 37 (1966), 577–580. http://dx.doi.org/10.1214/aoms/1177699450 doi: 10.1214/aoms/1177699450
![]() |
[8] | A. Dasgupta, Asymptotic theory of statistics and probability, New York: Springer, 2008. http://dx.doi.org/10.1007/978-0-387-75971-5 |
[9] |
J. Averousa, C. Genest, S. Kochar, On the dependence structure of order statistics, J. Multivariate Anal., 94 (2005), 159–171. http://dx.doi.org/10.1016/j.jmva.2004.03.004 doi: 10.1016/j.jmva.2004.03.004
![]() |
[10] |
J. Wang, C. Deng, J. Li, M. Zhou, On variances and covariances of a kind of extreme order statistics, Commun. Stat.-Theor. M., 45 (2016), 3274–3282. http://dx.doi.org/10.1080/03610926.2014.901373 doi: 10.1080/03610926.2014.901373
![]() |
[11] |
J. Wang, C. Deng, J. Li, On moment convergence for some order statistics, AIMS Mathematics, 7 (2022), 17061–17079. http://dx.doi.org/10.3934/math.2022938 doi: 10.3934/math.2022938
![]() |
[12] | V. Zorich, Mathematical analysis II, Berlin: Springer, 2016. http://dx.doi.org/10.1007/978-3-662-48993-2 |
[13] | S. Kotz, N. Balakrishnan, N. Johnson, Continuous multivariate distributions: models and applications, New York: John Wiley & Sons, 2000. http://dx.doi.org/10.1002/0471722065 |
[14] |
Z. Chen, A simple method for estimating parameters of the location-scale distribution family, J. Stat. Comput. Sim., 81 (2011), 49–58. http://dx.doi.org/10.1080/00949650903177497 doi: 10.1080/00949650903177497
![]() |
[15] |
O. Kravchuk, P. Pollett, Hodges-Lehmann scale estimator for Cauchy distribution, Commun. Stat.-Theor. M., 41 (2012), 3621–3632, http://dx.doi.org/10.1080/03610926.2011.563016 doi: 10.1080/03610926.2011.563016
![]() |
[16] | B. Arnold, N. Balakrishnan, H. Nagaraja, A first course in order statistics, New York: SIAM, 1992. http://dx.doi.org/10.1137/1.9780898719062 |
[17] |
P. Sen, On some properties of the asymptotic variance of the sample quantiles and mid-ranges, J. R. Stat. Soc. B, 23 (1961), 453–459. http://dx.doi.org/10.1111/j.2517-6161.1961.tb00428.x doi: 10.1111/j.2517-6161.1961.tb00428.x
![]() |
[18] | D. Pekasiewicz, Application of quantile methods to estimation of Cauchy distribution parameters, Statistics in Transition, 15 (2014), 133–144. |
[19] |
I. Krykun, The arctangent regression and the estimation of parameters of the Cauchy distribution, J Math. Sci., 249 (2020), 739–753. http://dx.doi.org/10.1007/s10958-020-04970-3 doi: 10.1007/s10958-020-04970-3
![]() |
[20] |
K. Raghunandanan, R. Srinivasan, Simplified estimation of parameters in a logistic distribution.Biometrika, 57 (1970), 677–679. http://dx.doi.org/10.1093/biomet/57.3.677 doi: 10.1093/biomet/57.3.677
![]() |