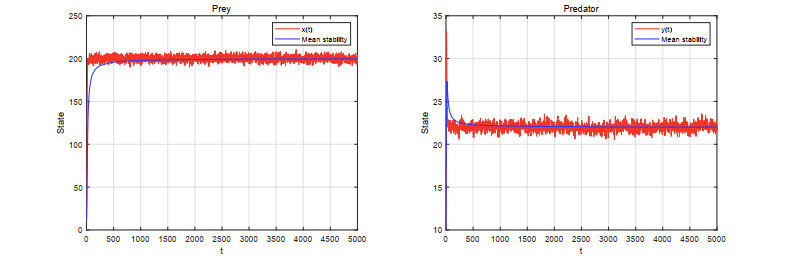
In this paper, we investigate a stochastic prey-predator model with Holling-type IV functional responses, anti-predatory behavior (referring to prey resistance to predator), gestation time delay of prey and Lévy noise. We investigate the existence and uniqueness of global positive solutions through Itô's formulation and Lyapunov's method. We also provide sufficient conditions for the persistence and extinction of prey-predator populations. Additionally, we examine the stability of the system distribution and validate our analytical findings through detailed numerical simulations. Our paper concludes with the implications of our results.
Citation: Chuanfu Chai, Yuanfu Shao, Yaping Wang. Analysis of a Holling-type IV stochastic prey-predator system with anti-predatory behavior and Lévy noise[J]. AIMS Mathematics, 2023, 8(9): 21033-21054. doi: 10.3934/math.20231071
[1] | Yuanfu Shao . Dynamics and optimal harvesting of a stochastic predator-prey system with regime switching, S-type distributed time delays and Lévy jumps. AIMS Mathematics, 2022, 7(3): 4068-4093. doi: 10.3934/math.2022225 |
[2] | Binfeng Xie, Na Zhang . Influence of fear effect on a Holling type III prey-predator system with the prey refuge. AIMS Mathematics, 2022, 7(2): 1811-1830. doi: 10.3934/math.2022104 |
[3] | Chuangliang Qin, Jinji Du, Yuanxian Hui . Dynamical behavior of a stochastic predator-prey model with Holling-type III functional response and infectious predator. AIMS Mathematics, 2022, 7(5): 7403-7418. doi: 10.3934/math.2022413 |
[4] | Hong Qiu, Yanzhang Huo, Tianhui Ma . Dynamical analysis of a stochastic hybrid predator-prey model with Beddington-DeAngelis functional response and Lévy jumps. AIMS Mathematics, 2022, 7(8): 14492-14512. doi: 10.3934/math.2022799 |
[5] | Kexin Zhang, Caihui Yu, Hongbin Wang, Xianghong Li . Multi-scale dynamics of predator-prey systems with Holling-IV functional response. AIMS Mathematics, 2024, 9(2): 3559-3575. doi: 10.3934/math.2024174 |
[6] | Heping Jiang . Complex dynamics induced by harvesting rate and delay in a diffusive Leslie-Gower predator-prey model. AIMS Mathematics, 2023, 8(9): 20718-20730. doi: 10.3934/math.20231056 |
[7] | Yubo Liu, Daipeng Kuang, Jianli Li . Threshold behaviour of a triple-delay SIQR stochastic epidemic model with Lévy noise perturbation. AIMS Mathematics, 2022, 7(9): 16498-16518. doi: 10.3934/math.2022903 |
[8] | Xuegui Zhang, Yuanfu Shao . Analysis of a stochastic predator-prey system with mixed functional responses and Lévy jumps. AIMS Mathematics, 2021, 6(5): 4404-4427. doi: 10.3934/math.2021261 |
[9] | Jagdev Singh, Behzad Ghanbari, Ved Prakash Dubey, Devendra Kumar, Kottakkaran Sooppy Nisar . Fractional dynamics and computational analysis of food chain model with disease in intermediate predator. AIMS Mathematics, 2024, 9(7): 17089-17121. doi: 10.3934/math.2024830 |
[10] | Naret Ruttanaprommarin, Zulqurnain Sabir, Salem Ben Said, Muhammad Asif Zahoor Raja, Saira Bhatti, Wajaree Weera, Thongchai Botmart . Supervised neural learning for the predator-prey delay differential system of Holling form-III. AIMS Mathematics, 2022, 7(11): 20126-20142. doi: 10.3934/math.20221101 |
In this paper, we investigate a stochastic prey-predator model with Holling-type IV functional responses, anti-predatory behavior (referring to prey resistance to predator), gestation time delay of prey and Lévy noise. We investigate the existence and uniqueness of global positive solutions through Itô's formulation and Lyapunov's method. We also provide sufficient conditions for the persistence and extinction of prey-predator populations. Additionally, we examine the stability of the system distribution and validate our analytical findings through detailed numerical simulations. Our paper concludes with the implications of our results.
In recent years, there has been increasing interest in population dynamics models of prey-predator interactions within both the fields of biology and mathematics [1]. The Volterra prey-predator model has received criticism for its unrealistic assumptions. However, theoretical results have shown that the prey-predator interaction is a crucial relationship in an ecosystem. To model this interaction, the functional response function is used. This mathematical model describes the predation rates of predators at varying prey densities and their response to changes in prey density. C. S. Holling [2] proposed Holling-type functional response functions, which have received significant attention in the academic community. Holling classified functional response functions into three types: Holling-type I, Holling-type II, and Holling-type III. Holling-type I (m(x)=bx, linear) is commonly used to describe the rate at which predators feed on a population of unresisting prey without a saturation point, such as herbivores feeding on grass. [3,4] has conducted further studies on this topic. On the other hand, Holling-type II (m(x)=bxa+x, concave increasing) is used to describe low-level predators that are sensitive to prey selection and have saturation limits. [5,6,7,8] has explored this type of predator. Finally, Holling-type III (m(x)=bx2a+x2, sigmoid increasing) is an extension of Holling-type II and is used to describe more complex predatory predators like predatory birds. This type of predator has been studied by [9,10,11,12].
An improved Holling-type function, known as the Holling-type IV functional response function, has been proposed [13]. This function m(x)=bxa+x2 suggests a non-monotonic response at higher levels of nutrient concentration, meaning that there may be an inhibitory effect on specific growth rates, as noted in previous research [14]. Specifically, when prey densities are low, predation rates increase with prey abundance. However, when prey concentrations reach a threshold, prey collectively defend themselves, causing predator intake rates to decrease [15]. The Holling-type IV functional response function has been studied by many scholars, see [16,17,18,19]. A differential equation model with Holling-type IV is obtained:
{dx(t)=x(t)(r1(1−x(t)K)−by(t)a+x2(t))dt,dy(t)=y(t)(r2+μbx(t)a+x2(t)−c)dt, | (1.1) |
where x(t) and y(t) represent the population density of prey and predator at time t, respectively. r1 denotes the intrinsic growth rate of prey. r2 represents the natural birth rate of the predator. K denotes the environmental holding capacity of the population, b denotes the capture rate of the predator. μ denotes the prey-to-predator conversion rate. c denotes the natural mortality rate of the predator population.
The following model, which take into account the predation resistance, intraspecific competition for predators, and prey gestation time delays, were developed as follows:
{dx(t)=x(t)(r1(1−x(t)K)−by(t)a+x2(t))dt,dy(t)=y(t)(r2+μbx(t−τ)a+x2(t−τ)−c−dy(t)−ηx(t))dt. | (1.2) |
The size of the population is significantly impacted by the existence of intraspecific competition. The specifics are in [20,21,22,23]. Let d stand for the intra-species competition rate. [24] added the knowledge that adult prey can kill young predators in addition to intraspecific rivalry. It actually affects the ecosystem. Reports from Africa [25] suggest that elephants may exhibit anti-predatory behavior by turning on lion cubs and killing them, especially if the lions pose a threat to young elephants. The impact of such behavior on population size remains uncertain and requires further research. [26] said that although African hunting dogs will defend themselves when confronted by powerful predators, the effect on their population size is negligible. On the other hand, [27] indicated that seals' anti-predatory behavior can aid in boosting their population growth when faced with challenges. We propose the parameter η, which represents the rate of adult prey resistance to predation on juvenile predators, based on the reasoning from above.
Furthermore, the authors [28] emphasized that time delays must be taken into account because a model's evolution frequently involves the past till present states in addition to the current one. In [29], a model with gestation time delay was investigated, where the delay exceeding a predetermined threshold and the significant effects on population growth were discovered. The authors of [30] examined how harvest rates and time delays affected generalized Gaussian-type prey-predator models and discovered that time delays could change stability or perhaps make it more unstable. C. J. Xu et al. [31] conducted a study on the impact of time delay on the stability of integer-order and fractional-order delayed BAM neural networks exhibiting Hopf bifurcation. The study found that by adjusting the value of the time delay, the stability region of fractional-order BAM neural networks can be expanded and the onset of Hopf bifurcation can be delayed. The significance of time delays is also illustrated in [32]. Based on the aforementioned research, we consider the prey's delayed gestational time in this study. τ indicates the delayed gestation of the prey.
On the other hand, populations are disturbed by various kinds of random noise as shown in [33]. Among them, let us first consider white noise. Assuming that ri (i=1,2) is affected by white noise, we have ri→ri+σidWi(t), where Wi(t) is a standard Wiener process defined on the complete probability space (Ω,{Ft}t≥0,P); σ2i is the intensity of white noise, i=1,2. The authors [33] mathematically demonstrated that white noise increased the risk of species extinction. In [34], the problem of optimal harvesting of delayed logic models with white noise was investigated by Liu et al. It once again validated this view. [35] studied the effects of white noise on the persistence, extinction and stability of biological populations. It revealed that the effects of white noise on different species are not uniform for all populations. In summary, there is a need to study the effect of white noise on the system dynamics. Thus, the following stochastic biomathematical model with white noise is given:
{dx(t)=x(t)(r1(1−x(t)K)−by(t)a+x2(t))dt+σ1x(t)dW1(t),dy(t)=y(t)(r2+μbx(t−τ)a+x2(t−τ)−c−dy(t)−ηx(t))dt+σ2y(t)dW2(t). | (1.3) |
In addition, the growth of populations affected by environmental fluctuations is usually a stochastic process. In reality, the process of population growth is inevitably exposed to natural disasters such as floods, earthquakes and tsunamis. However, these phenomena can not be explained by white noise [36,37]. Some scholars (e.g., [38,39,40,41]) have suggested that such sudden random perturbations can be represented by Lévy noise which plays a key role in the persistence and extinction of populations. Zhao et al. [21] studied a two-species Lotka-Volterra model in a stochastic environment and found that Lévy noise can have the opposite effect on the population growth. Zhou et al. [42] studied models of infectious diseases with Lévy noise and found that Lévy noise can be effective in controlling outbreaks of infectious diseases. It is necessary to consider the effect of Lévy noise on the population size of organisms, so we include Lévy noise as a stochastic environmental factor in the system for our study:
{dx(t)=x(t)(r1(1−x(t)K)−by(t)a+x2(t))dt+σ1x(t)dW1(t)+∫Yγ1(u)x(t−)˜N(dt,du),dy(t)=y(t)(r2+μbx(t−τ)a+x2(t−τ)−c−dy(t)−ηx(t))dt+σ2y(t)dW2(t)+∫Yγ2(u)y(t−)˜N(dt,du). | (1.4) |
Here xi(t−)=lims↑txi(s),Y⊆(0,+∞),˜N(dt,du)=N(dt,du)−λ(du)dt,N(dt,du) represents a Poisson counting measure with characteristic measure λ and λ(Y)<∞,γi(u)>−1,u∈Y,i=1,2. Further details can be found in [21].
Combining the above analysis, this paper investigates a stochastic prey-predator model with Holling-type IV functional responses, anti-predatory behavior, gestation time delay of prey and Lévy noise. We discuss the dynamical properties of model (1.4) in subsequent sections. The theoretical knowledge and model assumptions used in the proofs are presented in Section 2. Section 3 covers the existence and limitations of a global positive solution to system (1.4) and provides sufficient conditions for the persistence and extinction of both species. The stable distribution is discussed in Section 4. In Section 5, we use MATLAB R2021b to carry out simulations of the results. The conclusions of this paper are given in Section 6.
In this section, we give the theoretical knowledge needed for the subsequent proofs and make reasonable assumptions about the model (1.4).
First, we introduce the form of Itô's formula with Lévy noise. The following stochastic differential equation with Lévy noise is given:
dx(t)=F1(x(t−),t−)dt+F2(x(t−),t−)dW(t)+∫YF3(x(t−),t−,u)˜N(dt,du), | (2.1) |
where F1,F2 and F3 denote measurable functions mapped onto Rn. Let x(t)∈Rn be the solution of Eq (2.1) and set V∈C2,1(Rn×R+;R). We define the operator function by:
LV(x,t)=Vt(x,t)+Vx(x,t)F1(x,t)+12Vxx(x,t)F22(x,t)+∫Y{V(x+F3(x,t,u),t)−V(x,t)−Vx(x,t)F3(x,t,u)}λ(du). | (2.2) |
We obtain the following Itô's formula with Lévy noise:
dV(x,t)=LV(x,t)dt+Vx(x,t)F2(x,t)dW(t)+∫Y{V(x+F3(x,t,u),t)−V(x,t)}˜N(dt,du), | (2.3) |
where Vt(x,t)=∂V(x,t)∂t,Vx(x,t)=∂V(x,t)∂x,Vxx(x,t)=∂2V(x,t)∂xi∂xj. See [43] for more details on stochastic differential equations with Lévy noise.
Next, for further calculations, it is assumed that the model (1.4) satisfies the following assumptions.
(A1) We let the initial values (x(ϱ),y(ϱ)) be positive and belong to the Banach space Cg. The definition is as follows
Cg={ξ∈C((−∞,0];R2+):‖ξ‖g=sup−∞<θ≤0erθ|ξ(θ)|<+∞}, |
where g>0, there exists a probability measure π on (−∞,0] such that
πr=∫0−∞e−2rθdπi(θ)<+∞,i=1,2. |
If θ≤0 in the above equation, it is obvious that the assumption holds when πi(θ)=emrθ(m>2). Then, there are many such probability measures.
(A2) In this paper, we assume that there exist constants ci>0,i=1,2 such that
∫Y{|γi(u)|2∨[ln(1+γi(u))]2}λ(du)≤c1<+∞,∫Y{γi(u)−ln(1+γi(u))}λ(du)≤c2<+∞. |
For each h>0, there exists ωh such that
∫Y‖Mi(x,u)−Mi(y,u)‖2λ(du)≤ωh‖x−y‖2,i=1,2, |
M1(x,u)=γ1(u)x(t−),M2(y,u)=γ2(u)y(t−),‖x‖∨‖y‖≤h. |
(A3) All parameters are positive values. The stochastic perturbations N, W1 and W2 are all independent of each other.
In this section, we begin with the existence and uniqueness of a global positive solution to model (1.4), then give some sufficient conditions for the extinction and persistence of the solution for population x(t) and y(t).
For the sake of subsequent proofs and calculations, we define the following notations:
δ1=r1−12σ21+∫Y[ln(1+γ1(u))−γ1(u)]λ(du),δ2=r2−c−12σ22+∫Y[ln(1+γ2(u))−γ2(u)]λ(du),⟨f(t)⟩=1t∫t0f(s)ds,⟨f(t)⟩∗=limt→∞sup⟨f(t)⟩,⟨f(t)⟩∗=limt→∞inf⟨f(t)⟩. |
Theorem 3.1. Supposing (A1) and (A2) hold. For any given positive initial value (x(ϱ),y(ϱ))∈Cg, the system (1.4) has a unique global solution (x(t),y(t))∈R2+ for all t≥−τ and the solution will remain in R2+ with probability 1, that is
P{(x(t),y(t))∈R2+:∀t≥0}=1. |
Proof. All coefficients of the model (1.4) are locally Lipschitz continuous, so for initial values (x(ϱ),y(ϱ)) in the space R2+, there exists a unique local solution (x(t),y(t)) for all t∈[−τ,τe], where the concept τe represents the duration of the explosion. To prove that this solution is also global, it suffices to show that τe=∞ almost surely. To prove this, we consider a sufficiently large positive integer k0 such that (x(ϱ),y(ϱ)) belong to interval [1k0,k0]. Furthermore, for any k≥k0, we define the stopping time as
τk=inf{t∈[0,τe):x(t)∉(1k,k) or y(t)∉(1k,k)}. |
In this paper, we set inf∅=∞(∅ denotes the empty set). Let τ∞=limk→∞τk. Since τk is non-decreasing, then τ∞≤τe. We only need to verify that τ∞=∞. Otherwise, there exists T>0 and ε∈(0,1) such that P{τ∞≤T}>ε. Thus, by indicating Ψk={τk≤T}, there exists k1≥k0 such that
P(Ψk)≥ε∀k≥k1. | (3.1) |
To further prove this, we define a C2-function V from the space R2+ to R2+:
V(x,y)=x−1−lnx+y−1−lny. |
When (x(t),y(t))∈R2+, by using Itô's formula
dV(x,y)=LV(x,y)dt+σ1(x−1)dW1(t)+σ2(y−1)dW2(t)+∫Y{[γ1(u)x−ln(1+γ1(u))]+[γ2(u)y−ln(1+γ2(u))]}˜N(dt,du), | (3.2) |
where
LV(x,y)=(x−1)(r1−r1xK−bya+x2)+σ212+(y−1)(r2+μbx(t−τ)a+x2(t−τ)−c−dy−ηx)+σ222+∫Y[γ1(u)−ln(1+γ1(u))]λ(du)+∫Y[γ2(u)−ln(1+γ2(u))]λ(du)≤x(r1−r1xK−bya+x2)−(r1−r1xK−bya+x2)+μbyx(t−τ)+σ21+σ222−y(c+dy+ηx)+c+dy+ηx+r2y−r2+∫Y[γ1(u)−ln(1+γ1(u))]λ(du)+∫Y[γ2(u)−ln(1+γ2(u))]λ(du)≤(r1+η+r1K)x+c−r1x2K−cy−ηxy−dy2+dy+byx2+μbyx(t−τ)+σ21+σ222+r2y+2Γ≤(r1+η+r1K)x+r2y+c+dy+byx2+μbyx(t−τ)+σ21+σ222+2Γ:=Ξ>0, |
where Ξ is a positive constant. The following proof is similar to Theorem 3.1 in Xue and Shao [44], so we omit it here. So far, Theorem 3.1 is proved.
Lemma 3.1. (See Lemma 2.2 in [45]) Let A(t)∈C(Ω×[0,∞),R+) and assumption (A2) holds.
(1) If there exist positive values δ,δ0,˜δi(i=1,2) and some positive moment T, for any t≥T, there exists the following equation
lnA(t)≤δt−δ0∫t0A(s)ds+σiWi(t)+˜δi∫t0∫Yln(1+γi(u))˜N(ds,du), |
then we have
{⟨A(t)⟩∗≤δδ0a.s., ifδ≥0,limt→+∞A(t)=0a.s., ifδ<0. |
(2) If there exist positive values δ,δ0,˜δi(i=1,2) and some positive moment T, for any t≥T, there exists the following equation
lnA(t)≥δt−δ0∫t0A(s)ds+σiWi(t)+˜δi∫t0∫Yln(1+γi(u))˜N(ds,du), |
then we have
⟨A(t)⟩∗≥δδ0a.s. |
Theorem 3.2. Supposing (A1) and (A2) hold. For the model (1.4), we have
(1) if δ1<0, δ2<0, then both x(t) and y(t) tend to extinction:
limt→+∞x(t)=limt→+∞y(t)=0a.s.; |
(2) if δ1<0, δ2>0, x(t) tends to extinction, but y(t) is persistent in time average:
limt→+∞x(t)=0,limt→+∞⟨y(t)⟩=δ2da.s.; |
(3) if δ1>0, δ2<0, y(t) tends to extinction, but x(t) is persistent in time average:
limt→+∞⟨x(t)⟩=Kδ1r1,limt→+∞y(t)=0a.s. |
Proof. By Itô's formula, from the model (1.4), we obtain the following equation
{dlnx(t)=[(r1−r1x(t)K)−bya+x2(t)]dt+σ1dW1(t)−12σ21dt+∫Yln(1+γ1(u))˜N(dt,du),dlny(t)=[r2+μbx(t−τ)a+x2(t−τ)−c−dy(t)−ηx(t)]dt+σ2dW2(t)−12σ22dt+∫Yln(1+γ2(u))˜N(dt,du). | (3.3) |
Integrating both sides of the above Eq (3.3) simultaneously yields
lnx(t)−lnx(0)=r1t−r1K∫t0x(s)ds−b∫t0y(s)a+x2(s)ds+σ1W1(t)−12σ21t+∫t0∫Y[ln(x(s−)+x(s−)γ1(u))−ln(x(s−))−γ1(u)]λ(du)ds+∫t0∫Y[ln(x(s−)+x(s−)γ1(u))−ln(x(s−))]˜N(ds,du)=r1t−12σ21t+t∫Y[ln(1+γ1(u))−γ1(u)]λ(du)−r1K∫t0x(s)ds−b∫t0y(s)a+x2(s)ds+σ1W1(t)+∫t0∫Y[ln(1+γ1(u))]˜N(ds,du)=[r1−12σ21+∫Y[ln(1+γ1(u))−γ1(u)]λ(du)]t−r1K∫t0x(s)ds−b∫t0y(s)a+x2(s)ds+σ1W1(t)+∫t0∫Y[ln(1+γ1(u))]˜N(ds,du), | (3.4) |
and
lny(t)−lny(0)=r2t−ct−12σ22t+σ2W2(t)−d∫t0y(s)ds−η∫t0x(s)ds+μb∫t0x(s−τ)a+x2(s−τ)ds+∫t0∫Y[ln(y(s−)+y(s−)γ2(u))−ln(y(s−))−γ2(u)]λ(du)ds+∫t0∫Y[ln(y(s−)+y(s−)γ2(u))−ln(y(s−))]˜N(ds,du)=r2t−ct−12σ22t+t∫Y[ln(1+γ2(u))−γ2(u)]λ(du)+σ2W2(t)−d∫t0y(s)ds+∫t0∫Yln(1+γ2(u))˜N(ds,du)−η∫t0x(s)ds+μb∫t0x(s−τ)a+x2(s−τ)ds=[r2−c−12σ22+∫Y[ln(1+γ2(u))−γ2(u)]λ(du)]t−η∫t0x(s)ds−d∫t0y(s)ds+σ2W2(t)+∫t0∫Yln(1+γ2(u))˜N(ds,du)+μb∫t−τ−τx(s)a+x2(s)ds. | (3.5) |
First, we prove (1). From Eqs (3.4) and (3.5), we have
lnx(t)−lnx(0)t=[r1−12σ21+∫Y[ln(1+γ1(u))−γ1(u)]λ(du)]−r1Kt∫t0x(s)ds−bt∫t0y(s)a+x2(s)ds+σ1W1(t)+∫t0∫Y[ln(1+γ1(u))]˜N(ds,du)t≤δ1−r1K⟨x(t)⟩+σ1W1(t)+∫t0∫Y[ln(1+γ1(u))]˜N(ds,du)t, | (3.6) |
and
lny(t)−lny(0)t=[r2−c−12σ22+∫Y[ln(1+γ2(u))−γ2(u)]λ(du)]−ηt∫t0x(s)ds−dt∫t0y(s)ds+μbt∫t−τ−τx(s)a+x2(s)ds+σ2W2(t)+∫t0∫Yln(1+γ2(u))˜N(ds,du)t≤δ2−d⟨y(t)⟩+σ2W2(t)+∫t0∫Yln(1+γ2(u))˜N(ds,du)+μb∫t−τ−τx(s)a+x2(s)dst. | (3.7) |
According to the law of large numbers, it yields that
limt→+∞σiWi(t)+∫t0∫Yln(1+γi(u))˜N(ds,du)t=0,i=1,2,limt→+∞∫t−τ−τx(s)a+x2(s)dst=0. |
According to Lemma 3.1, we have limt→+∞x(t)=limt→+∞y(t)=0a.s.
Second, we prove (2). The following formula can be obtained from Eq (3.5)
lny(t)−lny(0)t=δ2−dt∫t0y(s)ds−ηt∫t0x(s)ds+μbt∫t−τ−τx(s)a+x2(s)ds+σ2W2(t)+∫t0∫Yln(1+γ2(u))˜N(ds,du)t. | (3.8) |
If δ1<0, then it follows from (1) that limt→+∞x(t)=0,a.s. Further computations show that
limt→+∞x(t)a+x2(t)=0a.s. |
(i) It is clear that
lny(t)t≤δ2−d⟨y(t)⟩+μb∫t−τ−τx(s)a+x2(s)dst+σ2W2(t)+∫t0∫Yln(1+γ2(u))˜N(ds,du)t≤(δ2+ε)−d⟨y(t)⟩+σ2W2(t)+∫t0∫Yln(1+γ2(u))˜N(ds,du)t. | (3.9) |
When assumption (A2) holds, we can easily obtain limt→+∞σ2W2(t)+∫t0∫Yln(1+γ2(u))˜N(ds,du)t=0a.s. By utilizing Lemma 3.1, it derives that
⟨y(t)⟩∗≤δ2+εda.s. |
Letting ε→0, by further calculation, we can obtain
⟨y(t)⟩∗≤δ2da.s. |
(ii) Similarly,
lny(t)t=δ2−d⟨y(t)⟩−η⟨x(t)⟩+μbt[∫t−τ−τx(s)a+x2(t)ds]+σ2W2(t)+∫t0∫Yln(1+γ2(u))˜N(ds,du)t≥δ2−ε−d⟨y(t)⟩+σ2W2(t)+∫t0∫Yln(1+γ2(u))˜N(ds,du)t. | (3.10) |
By the same token, we can obtain
⟨y(t)⟩∗≥δ2−εda.s. |
Letting ε→0, by further calculation, we can obtain
⟨y(t)⟩∗≥δ2da.s. |
Summarizing (i) and (ii), when δ1<0,δ2>0, it can be concluded that
limt→+∞x(t)=0,limt→+∞⟨y(t)⟩=δ2da.s. |
Third, we prove (3). The proof of (3) is very similar to the proof of (2). From synthesis of the above analysis, when δ1>0,δ2<0, it can be concluded that
limt→+∞⟨x(t)⟩=Kδ1r1,limt→+∞y(t)=0a.s. |
Definition 3.1. (See in [46]) The system (1.4) is permanent in time average if there exist positive constants Li and Ci, for any solution (x(t),y(t)) under initial conditions (x(ϱ),y(ϱ))∈Cg, satisfies the following equation
Li≤limt→+∞inf∫t0g(s)dst≤limt→+∞sup∫t0g(s)dst≤Cia.s.g=x,y. |
Theorem 3.3. Under the conditions δ1−bδ2ad>0,δ2−kηδ1r1>0 and assumptions (A1) and (A2) hold, for any initial data (x(ϱ),y(ϱ))∈Cg, the solutions (x(t),y(t)) of the system have the following properties
limt→+∞sup⟨x(t)⟩≤C1a.s.,limt→+∞sup⟨y(t)⟩≤C2a.s.,limt→+∞inf⟨x(t)⟩≥L1a.s.,limt→+∞inf⟨y(t)⟩≥L2a.s., |
where
C1=Kδ1r1,C2=δ2d+Kμbδ1adr1,L1=Kδ1r1−Kbδ2r1ad,L2=δ2d−Kηδ1r1d. |
That is, the model (1.4) will be permanent in time average.
Proof. Due to (3.4), it yields that
lnx(t)−lnx(0)t=δ1−r1Kt∫t0x(s)ds−bt∫t0y(s)a+x2(s)ds+σ1W1(t)+∫t0∫Y[ln(1+γ1(u))]˜N(ds,du)t≤δ1+ε−r1K⟨x(t)⟩+σ1W1(t)+∫t0∫Y[ln(1+γ1(u))]˜N(ds,du)t. | (3.11) |
Letting ε→0, i.e.,
limt→+∞sup⟨x(t)⟩=Kδ1r1:=C1. |
Further calculations are performed to get
lnx(t)−lnx(0)t=δ1−r1Kt∫t0x(s)ds−bt∫t0y(s)a+x2(s)ds+σ1W1(t)+∫t0∫Y[ln(1+γ1(u))]˜N(ds,du)t≥δ1−r1K⟨x(t)⟩−bat∫t0y(s)ds+σ1W1(t)+∫t0∫Y[ln(1+γ1(u))]˜N(ds,du)t. | (3.12) |
From Theorem 3.2, it follows that
lnx(t)−lnx(0)t≥δ1−r1K⟨x(t)⟩−baδ2d+σ1W1(t)+∫t0∫Y[ln(1+γ1(u))]˜N(ds,du)t. | (3.13) |
Making use of the condition δ1−bδ2ad>0, we obtain
limt→+∞inf⟨x(t)⟩≥δ1−bδ2adr1K=Kδ1r1−Kbδ2r1ad:=L1. |
Similarly,
lny(t)−lny(0)t=δ2−dt∫t0y(s)ds−ηt∫t0x(s)ds+μbt∫t−τ−τx(s)a+x2(s)ds+σ2W2(t)+∫t0∫Yln(1+γ2(u))˜N(ds,du)t≤δ2−dt∫t0y(s)ds−ηt∫t0x(s)ds+μbat∫t−τ−τx(s)ds+σ2W2(t)+∫t0∫Yln(1+γ2(u))˜N(ds,du)t=δ2−dt∫t0y(s)ds−ηt∫t0x(s)ds+μbat∫0−τx(s)ds+μbat∫t0x(s)ds−μbat∫tt−τx(s)ds+σ2W2(t)+∫t0∫Yln(1+γ2(u))˜N(ds,du)t≤δ2−dt∫t0y(s)ds+μbat∫0−τx(s)ds+μbat∫t0x(s)ds+σ2W2(t)+∫t0∫Yln(1+γ2(u))˜N(ds,du)t≤δ2−dt∫t0y(s)ds+μbKδ1r1a+μbat∫0−τx(s)ds+σ2W2(t)+∫t0∫Yln(1+γ2(u))˜N(ds,du)t. | (3.14) |
Making use of the condition δ2−kηδ1r1>0, we obtain
limt→+∞sup⟨y(t)⟩≤δ2+Kbμδ1ar1d=δ2d+Kμbδ1adr1:=C2. |
Meanwhile,
lny(t)−lny(0)t=δ2−dt∫t0y(s)ds−ηt∫t0x(s)ds+μbt∫t−τ−τx(s)a+x2(s)ds+σ2W2(t)+∫t0∫Yln(1+γ2(u))˜N(ds,du)t≥δ2−dt∫t0y(s)ds−ηt∫t0x(s)ds+σ2W2(t)+∫t0∫Yln(1+γ2(u))˜N(ds,du)t≥δ2−Kηδ1r1−dt∫t0y(s)ds+σ2W2(t)+∫t0∫Yln(1+γ2(u))˜N(ds,du)t. | (3.15) |
Making use of the condition δ2−kηδ1r1>0, we obtain
limt→+∞inf⟨y(t)⟩≥δ2−Kηδ1r1d=δ2d−Kηδ1r1d:=L2. |
The proof is complete.
In this section, we establish the sufficient criteria for stability in distribution of the system (1.4). First, we give the following important lemma.
Lemma 4.1. Assuming (x(t),y(t)) is a component of the solution of the system (1.4) with any initial value, then there exists a positive constant K(ρ)>0 for any ρ>0 such that
limt→+∞supE|xρ(t)|≤K(ρ),limt→+∞supE|yρ(t)|≤K(ρ). |
Proof. Since the proof procedure for this lemma is very similar to the method of [38,47], we omit it here.
Definition 4.1. Let (x(t;ϕ),y(t;ϕ)) and (x(t;ψ),y(t;ψ)) be two solutions of the model (1.4) and the initial values satisfy ϕ(θ),ψ(θ)∈Cg. We call a system globally attractive or globally asymptotically stable if the following equation is satisfied.
{limt→+∞E|x(t;ϕ)−x(t;ψ)|=0a.s.limt→+∞E|y(t;ϕ)−y(t;ψ)|=0a.s. |
Theorem 4.1. If assumption (A3) holds, then system (1.4) is asymptotically stable in distribution. That is, as t→+∞, there exists a unique probability measure μ(⋅) such that, for any given initial value (x(ϱ),y(ϱ))∈R2+, the transition probability density p(t,ϕ,⋅) of solution (x(t),y(t)) converges weakly to μ(⋅).
Proof. Let (x(t;ϕ),y(t;ϕ)) and (x(t;ψ),y(t;ψ)) be two solutions of the model (1.4) and the initial values satisfy ϕ(θ),ψ(θ)∈Cg. Define
V(t)=|lnx(t;ϕ)−lnx(t;ψ)|+|lny(t;ϕ)−lny(t;ψ)|+μb∫tt−τ|x(s;ϕ)a+x2(s;ϕ)−x(s;ψ)a+x2(s;ψ)|ds. | (4.1) |
Using Itô's formula to find the right differentiation of the above Eq (4.1) yields
d+V(t)=sgn[x(t;ϕ)−x(t;ψ)][−r1K(x(t;ϕ)−x(t;ψ))−b(y(t;ϕ)a+x2(t;ϕ)−y(t;ψ)a+x2(t;ψ))]dt+sgn[y(t;ϕ)−y(t;ψ)][μb(x(t−τ;ϕ)a+x2(t−τ;ϕ)−x(t−τ;ψ)a+x2(t−τ;ψ))−d(y(t;ϕ)−y(t;ψ))−η(x(t;ϕ)−x(t;ψ))]dt+μb{|x(t;ϕ)a+x2(t;ϕ)−x(t;ψ)a+x2(t;ψ)|−|x(t−τ;ϕ)a+x2(t−τ;ϕ)−x(t−τ;ψ)a+x2(t−τ;ψ)|}dt≤sgn[x(t;ϕ)−x(t;ψ)][−r1K(x(t;ϕ)−x(t;ψ))−ba(y(t;ϕ)−y(t;ψ))]dt+sgn[y(t;ϕ)−y(t;ψ)][μba(x(t−τ;ϕ)−x(t−τ;ψ))−d(y(t;ϕ)−y(t;ψ))−η(x(t;ϕ)−x(t;ψ))]dt+μba{|x(t;ϕ)−x(t;ψ)|−|x(t−τ;ϕ)−x(t−τ;ψ)|}dt≤−r1K|x(t;ϕ)−x(t;ψ)|dt−ba|y(t;ϕ)−y(t;ψ)|dt−d|y(t;ϕ)−y(t;ψ)|dt−η|x(t;ϕ)−x(t;ψ)|dt+μba|(x(t−τ;ϕ)−x(t−τ;ψ))|dt+μba|x(t;ϕ)−x(t;ψ)|dt−μba|(x(t−τ;ϕ)−x(t−τ;ψ))|dt=−(r1K+η−μba)|x(t;ϕ)−x(t;ψ)|dt−(ba+d)|y(t;ϕ)−y(t;ψ)|dt. |
Thus,
E(V(t))≤V(0)−(r1K+η−μba)∫t0E|x(s;ϕ)−x(s;ψ)|ds−(ba+d)∫t0E|y(s;ϕ)−y(s;ψ)|ds. | (4.2) |
Obviously V(0)≥0, and we can obtain
E(V(t))+(r1K+η−μba)∫t0E|x(s;ϕ)−x(s;ψ)|ds+(ba+d)∫t0E|y(s;ϕ)−y(s;ψ)|ds≤V(0)<+∞. |
Under assumption (A3), as well as r1K+η−μba>0 and ba+d>0, we can obtain
E|x(t;ϕ)−x(t;ψ)|∈L1[0,+∞),E|y(t;ϕ)−y(t;ψ)|∈L1[0,+∞). | (4.3) |
In addition, the following equation can be obtained from the model (1.4)
{E(x(t))≤x(0)+r1∫t0E(x(s))ds−r1K∫t0E(x(s))2ds−ba∫t0E(x(s))E(y(s))ds,E(y(t))≤y(0)+r2∫t0E(y(s))ds+μba∫t0E(y(s))E(x(s−τ))ds−c∫t0E(y(s))ds−d∫t0E(y(s))2ds−η∫t0E(x(s))E(y(s))ds. | (4.4) |
From Lemma 4.1, we have
{dE(x(t))dt≤r1E(x(t))≤G∗x,dE(y(t))dt≤r2E(y(t))+μbaE(y(t))E(x(t−τ))≤G∗y, | (4.5) |
where G∗x and G∗y are both positive constants. By using Barbalat's Lemma [33], we yield that
{limt→+∞E|x(t;ϕ)−x(t;ψ)|=0a.s.limt→+∞E|y(t;ϕ)−y(t;ψ)|=0a.s. | (4.6) |
Next, let p(t,(ϕ,ψ),dς) denote the transfer probability density of the process (x(t),y(t))∈B. B is a Borel measurable set on R2+. Let P(R2+) denote the probability measures on R2+. For any given measures P1,P2∈P(R2+), the metric dBL is defined as follows,
dBL(P1,P2)=supu∈BL|∫R2+u(s)P1ds−∫R2+u(s)P2ds|, |
where
BL={u:R2+→R:|u(s1)−u(s2)|≤‖s1−s2‖,|u(⋅)|≤1}. |
We first prove p(t,(ϕ,ψ),dς) is Cauchy in the space P(R2+) with dBL. According to Chebyshev's inequality [48] and Lemma 4.1, we can get P(R2+) to be tight. For arbitrary u∈BL,t>0,s>0, we can obtain
|Eu(x(t+s;(ϕ,ψ)))−Eu(x(t;(ϕ,ψ)))|=|E[E(u(x(t+s;(ϕ,ψ)))|Fs)]−Eu(x(t;(ϕ,ψ)))|=|∫R2+Eu(x(t;(ς1,ς2)))p(s,(ϕ,ψ),dς)−Eu(x(t;(ϕ,ψ)))|≤∫R2+|Eu(x(t;(ς1,ς2)))−Eu(x(t;(ϕ,ψ)))|p(s,(ϕ,ψ),dς). |
From equation above, there exists a constant T>0 such that
supu∈BL|Eu(x(t;(ς1,ς2)))−Eu(x(t;(ϕ,ψ)))|<ε,∀t≥T. |
Similarly,
supu∈BL|Eu(y(t+s;(ς1,ς2)))−Eu(y(t;(ϕ,ψ)))|<ε,∀t≥T. | (4.7) |
Combining the above analyses, we can obtain
dBL(p(t+s,(ϕ,ψ),⋅),p(t,(ϕ,ψ),⋅))≤ε. |
We have proved p(t,(ϕ,ψ),dς) is Cauchy in the space P(R2+) with dBL, so there is a unique probability measure μ(⋅)∈R2+ such that
limt→+∞dBL(p(t,0,⋅),μ(⋅))=0. | (4.8) |
By further calculation, combining with (4.6) and (4.8), then
limt→+∞dBL(p(t,(ϕ,ψ),μ(⋅)))≤limt→+∞dBL(p(t,(ϕ,ψ),⋅),p(t,0,⋅))+limt→+∞dBL(p(t,0,⋅),μ(⋅)). |
That is
limt→+∞dBL(p(t,(ϕ,ψ),μ(⋅)))=0. |
This completes the proof.
In this section, we perform simulations using MATLAB R2021b to confirm the accuracy of our previous conclusions. Therefore, we set each parameter in the model (1.4) as follows.
r1=0.3,a=0.02,K=200,b=0.02,d=0.01,r2=0.5,c=0.08,μ=0.01,η=0.001. |
In addition, Y=(0,+∞),λ(Y)=1, and we set the initial value to (x(ϱ),y(ϱ))=(10,10).
First, we set σ1=σ2=0.01. In the above setup, we calculate that δ1=0.29995>0,δ2=0.41995>0. Both conditions are consistent with the setting of the stable persistence condition for prey and predators in Theorem 3.2. It follows that both prey and predator numbers fluctuate up and down along the mean. The results of this simulation are shown in Figure 1.
Second, we analyze the anti-predation behavior rate η for adult prey attacking young predators, taking η=0.002 and η=0.01, respectively, and the simulation results can be seen in Figure 2. Compared to Figure 1, the anti-predation behavior rate has a greater effect on the population size of predators. It indicates that smaller anti-predation rate can easily cause fluctuations in predator populations, while the anti-predation rate is larger, and the predator populations face extinction.
Third, by contrast to Figure 1, we now consider adjusting the strength of the random term to investigate changes in prey and predator population size for different white noise intensities. We set the random term parameter to σ1=σ2=0.05, which satisfy δ1=0.29875>0,δ2=0.295>0 at this point, and the population is persistent. We set σ1=σ2=1, then by calculation, δ1=−0.2<0,δ2=−0.08<0. Through the simulation results shown in Figure 3, we find that the population size fluctuates significantly as the random term parameter increases, and the population size tends to become extinct when the random term parameter is large enough. This is consistent with our conclusion.
Fourth, we investigate how the delay in gestation time of prey affects the population size. We keep the model parameters unchanged but set parameters σ1=0.01,σ2=0.07, and τ1=1,τ2=20. It is clear that both populations are consistently stable. The comparison of two plots in Figure 4 shows that different sizes of gestational delay have minimal effects on either population, which is consistent with our findings in this paper.
Finally, we investigate the effect of Lévy noise on the prey and predator populations in the model (1.4). We use the same parameter settings as before, but adjust the parameters γ1=0,γ2=0 to γ1=0.01,γ2=0.06. We conclude that, without Lévy noise, the number of predators remains low and fluctuates less, and that Lévy noise with appropriate intensity can increase the number of prey and predators to some extent. This is consistent with our research, as seen in Figure 5.
This paper examines the existence and stability of a delayed prey-predator system with anti-predatory behavior and Lévy noise. Building on previous work, we involve the Holling-type IV functional response into the prey-predator model and establish sufficient conditions that ensure the stability of the solutions. The previous conclusions are verified through numerical simulations, and the effects of anti-predatory behavior, time lag, and stochastic parameters are analyzed.
The simulation results indicate that anti-predation behavior brings a significant effect on the population stability. Increasing the rate of anti-predation behavior leads to the acceleration of population extinction. While the intensity of white noise has a minimal impact on the global stability of the model (1.4), but when the intensity of white noise is increased, the system stability is lost and both populations become extinct. According to numerical simulations, anti-predation behavior and white noise play crucial roles in maintaining population stability, while time delay has almost no effect. If the effect of Lévy noise is absent, then both prey and predators remain at a low fluctuation level. Increasing the intensity of Lévy noise leads to a moderate increase in the population of both prey and predators.
This paper focuses on a Holling-type IV functional response in the model setting, but there are other functional responses related to ratios that require further study. Additionally, only the prey's gestation delay is examined in the time delay setting, leaving room for future research on different types of time delays. C. J. Xu et al. [49,50] investigated a category of fractional-order predator models that incorporated both distributed and discrete delays. They were able to establish a stability and bifurcation criterion that was independent of delay, and used time delay as a bifurcation parameter. This research offers valuable insights that can inform our future investigations. We leave these to future investigations.
The authors declare they have not used Artificial Intelligence (AI) tools in the creation of this article.
The authors express their gratitude towards the reviewers for their insightful suggestions and valuable comments. These inputs have significantly contributed to improving the quality of this paper's presentation.
This article is financially supported by NSF of China (11861027).
The authors declare that they have no competing interests in this article.
[1] | W. Thomas, Stability and complexity in model ecosystems, Princeton University Press, 1973. https://doi.org/10.1515/9780691206912 |
[2] |
C. S. Holling, The functional response of predator to prey density and its role in mimicry and population regulation, Mem. Entomol. Soc. Can., 45 (1965), 1–60. https://doi.org/10.4039/entm9745fv doi: 10.4039/entm9745fv
![]() |
[3] |
W. W. Murdoch, A. Oaten, Predation and population stability, Adv. Ecol. Res., 9 (1975), 1–131. https://doi.org/10.1016/S0065-2504(08)60288-3 doi: 10.1016/S0065-2504(08)60288-3
![]() |
[4] |
L. A. Real, The kinetics of functional response, Am. Nat., 111 (1977), 289–300. https://doi.org/10.1086/283161 doi: 10.1086/283161
![]() |
[5] | N. V. Kampen, A. Heertjes, Statistical aspects of the predator-prey problem, J. Theor. Biol., 7 (1959), 1–36. |
[6] |
Y. F. Shao, Fear and delay effects on a food chain system with two kinds of different functional responses, Int. J. Biomath., 34 (2023), 2350025. https://doi.org/10.1142/S1793524523500250 doi: 10.1142/S1793524523500250
![]() |
[7] |
S. Baba, A comprehensive cost-effectiveness analysis of control of maize streak virusdisease with Holling's Type II predation form and standard incidence, Results Phys., 40 (2022), 105862. https://doi.org/10.1016/j.rinp.2022.105862 doi: 10.1016/j.rinp.2022.105862
![]() |
[8] |
A. Singh, V. S. Sharma, Bifurcations and chaos control in a discrete-time prey-predator model with Holling type-II functional response and prey refuge, J. Comput. Appl. Math., 418 (2023), 114666. https://doi.org/10.1016/j.cam.2022.114666 doi: 10.1016/j.cam.2022.114666
![]() |
[9] |
C. S. Holling, Some characteristics of simple types of predation and parasitism, Can. Entomol., 91 (1959), 385–398. https://doi.org/10.4039/Ent91385-7 doi: 10.4039/Ent91385-7
![]() |
[10] |
F. Y. Wei, Uniform persistence of asymptotically periodic multispecies competition predator-prey systems with Holling III type functional response, Appl. Math. Comput., 170 (2005), 994–998. https://doi.org/10.1016/j.amc.2004.12.040 doi: 10.1016/j.amc.2004.12.040
![]() |
[11] |
Y. J. Huang, Stability analysis of a prey-predator model with holling type III response function incorporating a prey refuge, Appl. Math. Comput., 182 (2006), 672–683. https://doi.org/10.1016/j.amc.2006.04.030 doi: 10.1016/j.amc.2006.04.030
![]() |
[12] |
V. Madhusudanana, HOPF-bifurcation analysis of delayed computer virus modelwith holling type iii incidence function and treatment, Sci. Afr., 15 (2022), e01125. https://doi.org/10.1016/j.sciaf.2022.e01125 doi: 10.1016/j.sciaf.2022.e01125
![]() |
[13] |
W. Sokol, J. A. Howell, Kinetics of phenol exidation by washed cells, Biotechnol. Bioeng., 23 (1981), 2039–2049. https://doi.org/10.1002/bit.260230909 doi: 10.1002/bit.260230909
![]() |
[14] |
V. H. Edwards, The influence of high substrate concentrations on microbial kinetics, Biotechnol. Bioeng., 12 (1970), 679–712. https://doi.org/10.1002/bit.260120504 doi: 10.1002/bit.260120504
![]() |
[15] |
H. H. C. Alvino, M. Marvá, Group defense promotes coexistence in interference competition: The Holling type IV competitive response, Math. Comput. Simulat., 198 (2022), 426–445. https://doi.org/10.1016/j.matcom.2022.02.031 doi: 10.1016/j.matcom.2022.02.031
![]() |
[16] |
S. W. Zhang, A food chain model with impulsive perturbations and Holling IV functional response, Chaos Soliton. Fract., 26 (2005), 855–866. https://doi.org/10.1016/j.chaos.2005.01.053 doi: 10.1016/j.chaos.2005.01.053
![]() |
[17] |
S. W. Zhang, Chaos in periodically forced Holling type IV predator-prey system with impulsive perturbations, Chaos Soliton. Fract., 27 (2006), 980–990. https://doi.org/10.1016/j.chaos.2005.04.065 doi: 10.1016/j.chaos.2005.04.065
![]() |
[18] |
C. X. Shen, Permanence and global attractivity of the food-chain system with Holling IV type functional response, Appl. Math. Comput., 194 (2007), 179–185. https://doi.org/10.1016/j.amc.2007.04.019 doi: 10.1016/j.amc.2007.04.019
![]() |
[19] |
X. X. Liu, Q. D. Huang, The dynamics of a harvested predator-prey system with Holling type IV functional response, BioSystems, 169–170 (2018), 26–39. https://doi.org/10.1016/j.biosystems.2018.05.005 doi: 10.1016/j.biosystems.2018.05.005
![]() |
[20] |
K. Gopalsamy, Time lags and global stability in two species competition, Bull. Math. Biol., 42 (1980), 729–737. https://doi.org/10.1016/S0092-8240(80)80069-3 doi: 10.1016/S0092-8240(80)80069-3
![]() |
[21] |
Y. Zhao, S. L. Yuan, Q. M. Zhang, The effect of Lévy noise on the survival of a stochastic competitive model in an impulsive polluted environment, Appl. Math. Model., 40 (2016), 7583–7600. https://doi.org/10.1016/j.apm.2016.01.056 doi: 10.1016/j.apm.2016.01.056
![]() |
[22] |
Y. Zhao, S. L. Yuan, Stability in distribution of a stochastic hybrid competitive Lotka-Volterra model with Lévy jumps, Chaos Soliton. Fract., 85 (2016), 98–109. https://doi.org/10.1016/j.chaos.2016.01.015 doi: 10.1016/j.chaos.2016.01.015
![]() |
[23] |
C. Lu, Dynamical behavior of stochastic delay Lotka-Volterra competitive model with general Lévy jumps, Physica A, 531 (2019), 121730. https://doi.org/10.1016/j.physa.2019.121730 doi: 10.1016/j.physa.2019.121730
![]() |
[24] |
B. Tang, Y. Xiao, Bifurcation analysis of a predator-prey model with anti-predator behaviour, Chaos Soliton. Fract., 70 (2015), 58–68. https://doi.org/10.1016/j.chaos.2014.11.008 doi: 10.1016/j.chaos.2014.11.008
![]() |
[25] |
K. M. Comb, G. Shannon, S. M. Durant, Leadership in elephants: The adaptive value of age, P. Roy. Soc. B-Biol. Sci., 278 (2011), 3270–3276. https://doi.org/10.1098/rspb.2011.0168 doi: 10.1098/rspb.2011.0168
![]() |
[26] |
S. Creel, N. M. Creel, Limitation of African wild dogs by competition with larger carnivores, Conserv. Biol., 10 (1996), 526–538. https://doi.org/10.1046/j.1523-1739.1996.10020526.x doi: 10.1046/j.1523-1739.1996.10020526.x
![]() |
[27] | J. V. Craig, Effects of predation risk on reproductive behavior of northern fur seals, J. Mammal., 86 (2005), 1059–1067. |
[28] | K. Gopalsamy, Stability and oscillations in delay differential equations of population dynamics, Springer Science+Business Media Dordrecht, 1992. https://doi.org/10.1007/978-94-015-7920-9 |
[29] |
J. P. Tripathi, S. Abbas, M. Thakur, A density dependent delayed predator-prey model with Beddington-DeAngelis type function response incorporating a prey refuge, Commun. Nonlinear Sci. Numer. Simul., 22 (2015), 427–450. https://doi.org/10.1016/j.cnsns.2014.08.018 doi: 10.1016/j.cnsns.2014.08.018
![]() |
[30] |
A. Martin, S. Ruan, Predator-prey models with delay and prey harvesting, J. Math. Biol., 43 (2001), 247–267. https://doi.org/10.1007/s002850100095 doi: 10.1007/s002850100095
![]() |
[31] |
C. J. Xu, W. Zhang, C. Aouiti, Z. X. Liu, L. Y. Yao, Comparative exploration on bifurcation behavior for integer-order and fractional-order delayed BAM neural networks, Nonlinear Anal. Model. Control, 27 (2022), 1030–1053. https://doi.org/10.15388/namc.2022.27.28491 doi: 10.15388/namc.2022.27.28491
![]() |
[32] |
S. Ruan, Delay differential equations in single species dynamics, Delay Differ. Equat. Appl., 205 (2006), 477–517. https://dx.doi.org/10.1007/1-4020-3647-7_11 doi: 10.1007/1-4020-3647-7_11
![]() |
[33] | J. Barbalat, Systems d'equations differentielles d'osci d'oscillations nonlinéaires, Romanian J. Pure Appl. Math., 4 (1959), 267–270. |
[34] |
M. Liu, C. Z. Bai, Optimal harvesting of a stochastic logistic model with time delay, J. Nonlinear Sci., 25 (2015), 277–289. https://doi.org/10.1007/s00332-014-9229-2 doi: 10.1007/s00332-014-9229-2
![]() |
[35] |
M. Liu, P. S. Mandal, Dynamical behavior of a one-prey two-predator model with random perturbations, Commun. Nonlinear Sci. Numer. Simulat., 28 (2015), 123–137. https://doi.org/10.1016/j.cnsns.2015.04.010 doi: 10.1016/j.cnsns.2015.04.010
![]() |
[36] |
Y. Guo, Stochastic regime switching SIR model driven by Lévy noise, Physica A, 497 (2017), 1–11. https://doi.org/10.1016/j.physa.2017.02.053 doi: 10.1016/j.physa.2017.02.053
![]() |
[37] |
H. Qiu, Optimal harvesting of a stochastic delay tri-trophic food-chain model with Lévy jumps, Physica A, 492 (2018), 1715–1728. https://doi.org/10.1016/j.physa.2017.11.092 doi: 10.1016/j.physa.2017.11.092
![]() |
[38] |
J. H. Bao, X. R. Mao, G. Yin, C. G. Yuan, Competitive Lotka-Volterra population dynamics with jumps, Nonlinear Anal., 74 (2011), 6601–6616. https://doi.org/10.1016/j.na.2011.06.043 doi: 10.1016/j.na.2011.06.043
![]() |
[39] |
J. Yu, Stationary distribution and ergodicity of a stochastic food-chain model with Lévy jumps, Physica A, 482 (2017), 14–28. https://doi.org/10.1016/j.physa.2017.04.067 doi: 10.1016/j.physa.2017.04.067
![]() |
[40] |
M. Deng, Stability of a stochastic delay commensalism model with Lévy jumps, Physica A, 527 (2019), 121061. https://doi.org/10.1016/j.physa.2019.121061 doi: 10.1016/j.physa.2019.121061
![]() |
[41] |
C. Liu, Q. L. Zhang, Y. K. Li, Dynamical behavior in a hybrid stochastic triple delayed prey predator bioeconomic system with Lévy jumps, J. Franklin I., 356 (2019), 592–628. https://doi.org/10.1016/j.jfranklin.2018.11.015 doi: 10.1016/j.jfranklin.2018.11.015
![]() |
[42] |
Y. L. Zhou, S. L. Yuan, D. L. Zhao, Threshold behavior of a stochastic SIS model with Lévy jumps, Appl. Math. Comput., 275 (2016), 255–267. https://doi.org/10.1016/j.amc.2015.11.077 doi: 10.1016/j.amc.2015.11.077
![]() |
[43] |
H. Kunita, Itô's stochastic calculus: Its surprising power for applications, Stoch. Process. Appl., 120 (2010), 622–652. https://doi.org/10.1016/j.spa.2010.01.013 doi: 10.1016/j.spa.2010.01.013
![]() |
[44] | R. X. Xue, Y. F. Shao, Analysis of a stochastic predator-prey system with fear effect and Lévy noise, Adv. Cont. Discr. Mod., 72 (2022). https://doi.org/10.1186/s13662-022-03749-x |
[45] |
C. Liu, X. Y. Xun, Q. L. Zhang, Y. K. Li, Dynamical analysis and optimal control in a hybrid stochastic double delayed bioeconomic system with impulsive contaminants emission and Lévy jumps, Appl. Math. Comput., 352 (2019), 99–118. https://doi.org/10.1016/j.amc.2019.01.045 doi: 10.1016/j.amc.2019.01.045
![]() |
[46] |
Z. Ma, G. Cui, W. Wang, Persistence and extinction of a population in a polluted environment, Math. Biosci., 101 (1990), 75–97. https://doi.org/10.1016/0025-5564(90)90103-6 doi: 10.1016/0025-5564(90)90103-6
![]() |
[47] |
X. Y. Li, X. R. Mao, Population dynamical behavior of non-autonomous Lotka-Volterra competitive system with random perturbation, Discrete Cont. Dyn-S., 24 (2009), 523–545. https://doi.org/10.3934/dcds.2009.24.523 doi: 10.3934/dcds.2009.24.523
![]() |
[48] | M. Kot, Elements of mathematical biology, Cambridge University Press, 2001. https://doi.org/10.1017/CBO9780511608520 |
[49] |
C. J. Xu, D. Mu, Y. L. Pan, C. Aouiti, L. Y. Yao, Exploring bifurcation in a fractional-order predator-prey system with mixed delays, J. Appl. Anal. Comput., 13 (2023), 1119–1136. https://doi.org/10.11948/20210313 doi: 10.11948/20210313
![]() |
[50] |
C. J. Xu, W. Zhang, C. Aouiti, Z. X. Liu, L. Y. Yao, Bifurcation insight for a fractional-order stage-structured predator-prey system incorporating mixed time delays, Math. Method. Appl. Sci., 46 (2023), 7489–7513. https://doi.org/10.1002/mma.9041 doi: 10.1002/mma.9041
![]() |
1. | Xiao-jing Zhuo, Yong-feng Guo, Jing-yan Qi, Qian-qian Wang, Stationary distribution and mean extinction time in a generalist prey–predator model driven by Lévy noises, 2024, 187, 09600779, 115432, 10.1016/j.chaos.2024.115432 | |
2. | Weili Kong, Yuanfu Shao, Bifurcations of a Leslie-Gower predator-prey model with fear, strong Allee effect and hunting cooperation, 2024, 9, 2473-6988, 31607, 10.3934/math.20241520 | |
3. | Kexin Zhang, Caihui Yu, Hongbin Wang, Xianghong Li, Multi-scale dynamics of predator-prey systems with Holling-IV functional response, 2024, 9, 2473-6988, 3559, 10.3934/math.2024174 |