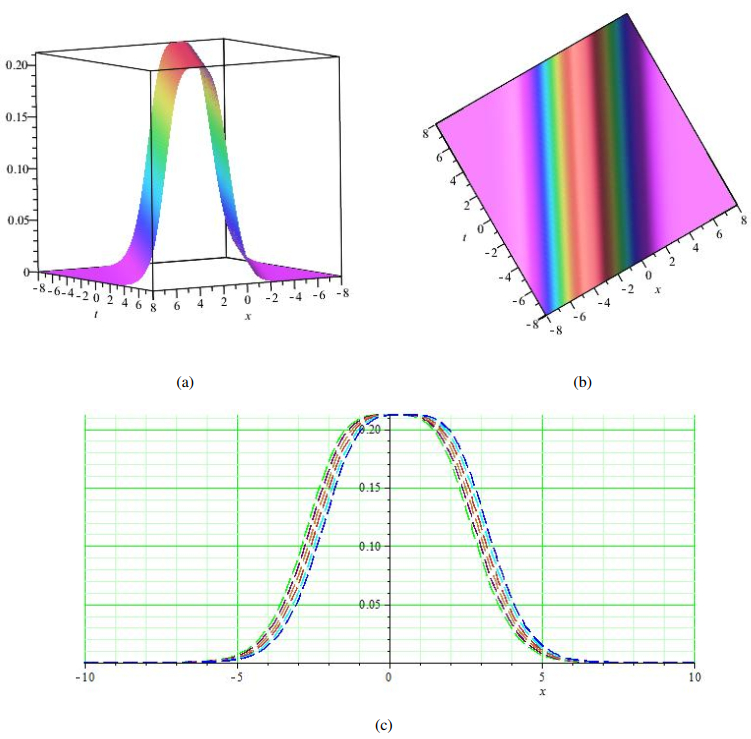
The purpose of this work is to present the stochastic computing study based on the artificial neural networks (ANNs) along with the scaled conjugate gradient (SCG), ANNs-SCG for solving the predator-prey delay differential system of Holling form-III. The mathematical form of the predator-prey delay differential system of Holling form-III is categorized into prey class, predator category and the recent past effects. Three variations of the predator-prey delay differential system of Holling form-III have been numerical stimulated by using the stochastic ANNs-SCG procedure. The selection of the data to solve the predator-prey delay differential system of Holling form-III is provided as 13%, 12% and 75% for testing, training, and substantiation together with 15 neurons. The correctness and exactness of the stochastic ANNs-SCG method is provided by using the comparison of the obtained and data-based reference solutions. The constancy, authentication, soundness, competence, and precision of the stochastic ANNs-SCG technique is performed through the analysis of the correlation measures, state transitions (STs), regression analysis, correlation, error histograms (EHs) and MSE.
Citation: Naret Ruttanaprommarin, Zulqurnain Sabir, Salem Ben Said, Muhammad Asif Zahoor Raja, Saira Bhatti, Wajaree Weera, Thongchai Botmart. Supervised neural learning for the predator-prey delay differential system of Holling form-III[J]. AIMS Mathematics, 2022, 7(11): 20126-20142. doi: 10.3934/math.20221101
[1] | Haitham Qawaqneh, Ali Altalbe, Ahmet Bekir, Kalim U. Tariq . Investigation of soliton solutions to the truncated M-fractional (3+1)-dimensional Gross-Pitaevskii equation with periodic potential. AIMS Mathematics, 2024, 9(9): 23410-23433. doi: 10.3934/math.20241138 |
[2] | Zhe Ji, Yifan Nie, Lingfei Li, Yingying Xie, Mancang Wang . Rational solutions of an extended (2+1)-dimensional Camassa-Holm- Kadomtsev-Petviashvili equation in liquid drop. AIMS Mathematics, 2023, 8(2): 3163-3184. doi: 10.3934/math.2023162 |
[3] | Muhammad Bilal Riaz, Syeda Sarwat Kazmi, Adil Jhangeer, Jan Martinovic . Unveiling solitons and dynamic patterns for a (3+1)-dimensional model describing nonlinear wave motion. AIMS Mathematics, 2024, 9(8): 20390-20412. doi: 10.3934/math.2024992 |
[4] | Haci Mehmet Baskonus, Md Nurul Raihen, Mehmet Kayalar . On the extraction of complex behavior of generalized higher-order nonlinear Boussinesq dynamical wave equation and (1+1)-dimensional Van der Waals gas system. AIMS Mathematics, 2024, 9(10): 28379-28399. doi: 10.3934/math.20241377 |
[5] | Naeem Ullah, Muhammad Imran Asjad, Jan Awrejcewicz, Taseer Muhammad, Dumitru Baleanu . On soliton solutions of fractional-order nonlinear model appears in physical sciences. AIMS Mathematics, 2022, 7(5): 7421-7440. doi: 10.3934/math.2022415 |
[6] | Jin Hyuk Choi, Hyunsoo Kim . Exact traveling wave solutions of the stochastic Wick-type fractional Caudrey-Dodd-Gibbon-Sawada-Kotera equation. AIMS Mathematics, 2021, 6(4): 4053-4072. doi: 10.3934/math.2021240 |
[7] | Shami A. M. Alsallami . Investigating exact solutions for the (3+1)-dimensional KdV-CBS equation: A non-traveling wave approach. AIMS Mathematics, 2025, 10(3): 6853-6872. doi: 10.3934/math.2025314 |
[8] | Huitzilin Yépez-Martínez, Mir Sajjad Hashemi, Ali Saleh Alshomrani, Mustafa Inc . Analytical solutions for nonlinear systems using Nucci's reduction approach and generalized projective Riccati equations. AIMS Mathematics, 2023, 8(7): 16655-16690. doi: 10.3934/math.2023852 |
[9] | Mohammed Aly Abdou, Loubna Ouahid, Saud Owyed, A. M. Abdel-Baset, Mustafa Inc, Mehmet Ali Akinlar, Yu-Ming Chu . Explicit solutions to the Sharma-Tasso-Olver equation. AIMS Mathematics, 2020, 5(6): 7272-7284. doi: 10.3934/math.2020465 |
[10] | Yanxia Hu, Qian Liu . On traveling wave solutions of a class of KdV-Burgers-Kuramoto type equations. AIMS Mathematics, 2019, 4(5): 1450-1465. doi: 10.3934/math.2019.5.1450 |
The purpose of this work is to present the stochastic computing study based on the artificial neural networks (ANNs) along with the scaled conjugate gradient (SCG), ANNs-SCG for solving the predator-prey delay differential system of Holling form-III. The mathematical form of the predator-prey delay differential system of Holling form-III is categorized into prey class, predator category and the recent past effects. Three variations of the predator-prey delay differential system of Holling form-III have been numerical stimulated by using the stochastic ANNs-SCG procedure. The selection of the data to solve the predator-prey delay differential system of Holling form-III is provided as 13%, 12% and 75% for testing, training, and substantiation together with 15 neurons. The correctness and exactness of the stochastic ANNs-SCG method is provided by using the comparison of the obtained and data-based reference solutions. The constancy, authentication, soundness, competence, and precision of the stochastic ANNs-SCG technique is performed through the analysis of the correlation measures, state transitions (STs), regression analysis, correlation, error histograms (EHs) and MSE.
In recent decades, researchers have paid considerable attention to nonlinear waves at the ocean surface. Phenomena of nonlinear waves play a key role in many fields of engineering and science, such as ocean engineering, plasma physics, Control theory, tsunami waves, communications industry, fluid dynamics, and coastal engineering, etc.
Non-linear PDEs have great potential for application in various fields; therefore, researchers pay special attention to their analytical and numerical solutions [1,2,3,4,5,6,7,8]. In the literature, many researchers in mathematics and physics have developed various methods to analyze the nonlinear evolution equations (NLEEs), such as the exp-function approach [9], the modified simple equation technique [10,11,12,13,14], the generalized Kudryashov technique [15,16], the G′G-expansion approach [17,18,19,20,21,22,23], the extended rational function expansion approach [23], the Hirota bilinear method [24,25,26,27,28,29,30], the extended homoclinic approach [31], the traveling wave scheme [32], the Darboux transformation method [32,33,34,35], the sine-cosine approach [36], the semi-inverse variational principle [37], He's variational iteration technique [38], the sine-Gordon method [39,40,41], A special kind of distributive product [42], the Lie symmetric method [43], the extended homogeneous principal method [44], the power series method [45] and some other methods [46,47].
The Boussinesq equation represents a long-wavelength and weakly nonlinear approximation used in numerical models, water waves, and coastal engineering for simulating water waves in shallow seas and harbors. A Scottish engineer named John Scott Russell closely observed solitary waves (also called translational waves and solitons). Joseph Boussinesq based his approximation on the obervation of John Scott Russell observation. In 1872, the simulation of one-dimensional water waves was determined by Boussinesq which states that the horizontal velocity is constant and the vertical velocity is linear in addition to the water depth, referred to as the Boussinesq equation [48]. Previously, the Boussinesq equation was investigated using various mathematical approaches [49,50,51,52,53].
Consider, the Boussinesq equation in the following form
Qtt−Qxx=(Q2)xx+λQxxxx, | (1.1) |
where Q=Q(x,t) represents the wave envelope containing x as a spatial variable and t as a temporal variable. Here λ is an arbitrary constant. This is called frequency dispersion phenomenon when water waves of different wavelengths are related, and in the case of an infinitesimal wave amplitude it is also called a linear frequency dispersion. For this reason, this is a valid approximation. The Boussinesq equation allows for waves to propagate in different directions as well, but it is advantageous to consider waves that propagate in the same direction. To form strong and reliable solitons of the Boussinesq equation using (SSET) [54,55,56], the following traveling wave transformation is used.
X(x,t)=X(β), β=ηx+χt. | (1.2) |
Here η and χ are real constants. Applying Eq (1.2) in Eq (1.1), the following ordinary differential equation (ODE) is constructed.
χ2Q″−η2Q″−η2(Q2)″−λη4Q(iv)=0. | (1.3) |
Integrating Eq (1.3) twice with respect to β and neglecting the integration constants, we obtain the following equation.
(χ2−η2)Q−η2Q2−λη4Q″=0. | (1.4) |
Where χ and η are the velocity and frequency of the propagating wave.
We proceed with these main steps in this work:
Step 1:
H(X,Xx,Xt,Xxx,Xtt,...)=0, | (2.1) |
where H is a polynomial of X and X(β)=X(x,t) is a unknown function. Consider the wave transformation
β=ηx+χt, |
where χ≠0 is a constant to be determined later.
Using the transformation, Eq (2.1) is converted to the following ODE.
G=(Q,Q′,Q″,...,)=0. | (2.2) |
Step 2: G is a function of Q(β) and prime express the derivatives in regard to β.
Solution of Eq (2.2) can be formulated as
Q(β)=N∑l=0clMl(β), cl≠0, | (2.3) |
where cl(0≤l≤N) are real constants and M(β) satisfying the ODE in the form
M′(β)=√ξ+uM(β)2+M(β)4. | (2.4) |
Here ξ and u are real constants and Eq (2.4) presents the solutions as
Case I: If u>0 and ξ=0, then
M±1(β)=±√−pqusechpq(√uβ),M±2(β)=±√pqucschpq(√uβ), |
where
sechpq(β)=2peβ+qe−β,cschpq(β)=2peβ−qe−β. |
Case II: If u<0 and ξ=0, then
M±3(β)=±√−pqusecpq(√−uβ),M±4(β)=±√−pqucscpq(√−uβ), |
where
secpq(β)=2peιβ+qe−ιβ,cscpq(β)=2ιpeιβ−qe−ιβ. |
Case III: If u<0 and ξ=u24, then
M±5(β)=±√−u2tanhpq(√−u2β),M±6(β)=±√−u2cothpq(√−u2β),M±7(β)=±√−u2(tanhpq(√−2uβ)±ι√pqsechpq(√−2uβ)),M±8(β)=±√−u2(cothpq(√−2uβ)±√pqcschpq(√−2uβ)),M±9(β)=±√−u8(tanhpq(√−u8β)+cothpq(√−u8β)), |
where
tanhpq(β)=peβ−qe−βpeβ+qe−β,cothpq(β)=peβ+qe−βpeβ−qe−β. |
Case IV: If u>0 and ξ=u24, then
M±10(β)=±√u2tanpq(√u2β),M±11(β)=±√u2cotpq(√u2β),M±12(β)=±√u2(tanpq(√2uβ)±√pqsecpq(√2uβ)),M±13(β)=±√u2(cotpq(√2uβ)±√pqcscpq(√2uβ)),M±14(β)=±√u8(tanpq(√u8β)+cotpq(√u8β)), |
where
tanpq(β)=−ιpeιβ−qe−ιβpeιβ+qe−ιβ,cotpq(β)=ιpeιβ+qe−ιβpeιβ−qe−ιβ. |
These are generalized trigonometric and hyperbolic functions with parameters p and q. If we take p=q=1, they become known trigonometric and hyperbolic functions.
Step 3: We calculate the integer N by balancing the capital. Substituting Eq (2.3) into Eq (2.2) we obtain an algebraic equation in the form of Ml(β), which we balance by equating the powers of Ml(β) l = (0, 1, 2, ...) to zero thus obtaining a set of algebraic equations.
Step 4: This set of equations leads to the required parameters and the exact solution of the given equation.
In this section, SSET is applied to the Boussinesq equation to construct the traveling wave solution. By the equilibrium rule Eq (2.3) reduces into
Q(β)=c0+c1M(β)+c2M(β)2, | (3.1) |
where c0, c1, c2 are constants. Substitute Eq (3.1), Eq (1.4) into Eq (2.4), we obtain a polynomial in the form of Ml(β) and equate powers of Ml(β) to zero resulting in algebraic equations in c0, c1, c2, χ, η and λ.
Set of algebraic equations are as:
−2c2η4λξ−c20η2−c0η2+c0χ2=0, |
−2c0c1η2−c1η2+c1η4λ(−u)+c1χ2=0, |
−2c0c2η2−c2η2−c21η2−4c2η4λu+c2χ2=0, |
−2c1η4λ−2c1c2η2=0, |
−6c2η4λ−c22η2=0. | (3.2) |
With the help of Mathematica software, the following parameters are determined.
c0=13(c2u−√c22u2−3c22ξ),c1=0,c2=c2, |
χ=−√3η2−2η2√c22(u2−3ξ)√3,λ=−c26η2. | (3.3) |
Using Eqs (2.4), (3.1) and (3.3) with Eq (1.2), following solutions are constructed.
Case I: If u>0 and ξ=0, then
Q±1(x,t)=13(c2u−√c22u2−3c22ξ)+c2(±√−pqu)2sechpq(√u(tχ+ηx))2, | (3.4) |
Q±2(x,t)=13(c2u−√c22u2−3c22ξ)+c2(±√pqu)2cschpq(√u(tχ+ηx))2. | (3.5) |
Case II: If u<0 and ξ=0, then
Q±3(x,t)=13(c2u−√c22u2−3c22ξ)+c2(±√−pqu)2secpq(√−u(tχ+ηx))2, | (3.6) |
Q±4(x,t)=13(c2u−√c22u2−3c22ξ)+c2(±√−pqu)2cscpq(√−u(tχ+ηx))2. | (3.7) |
Case III: If u<0 and ξ=u24, then
Q±5(x,t)=13(c2u−√c22u2−3c22ξ)+c2(±√−u√2)2tanhpq(√−u(tχ+ηx)√2)2, | (3.8) |
Q±6(x,t)=13(c2u−√c22u2−3c22ξ)+c2(±√−u√2)2cothpq(√−u(tχ+ηx)√2)2, | (3.9) |
Q±7(x,t)=13(c2u−√c22u2−3c22ξ)+c2(±√−u√2(tanhpq(√2√−u(tχ+ηx))±i√pqsechpq(√2√−u(tχ+ηx))))2, | (3.10) |
Q±8(x,t)=13(c2u−√c22u2−3c22ξ)+c2(±√−u√2(cothpq(√2√−u(tχ+ηx))±√pqcschpq(√2√−u(tχ+ηx))))2, | (3.11) |
Q±9(x,t)=13(c2u−√c22u2−3c22ξ)+c2(±√−u2√2(tanhpq(√−u(tχ+ηx)2√2)+cothpq(√−u(tχ+ηx)2√2)))2. | (3.12) |
Case IV: If u>0 and ξ=u24, then
Q±10(x,t)=13(c2u−√c22u2−3c22ξ)+c2(±√u√2)2tanpq(√u(tχ+ηx)√2)2, | (3.13) |
Q±11(x,t)=13(c2u−√c22u2−3c22ξ)+c2(±√u√2)2cotpq(√u(tχ+ηx)√2)2, | (3.14) |
Q±12(x,t)=13(c2u−√c22u2−3c22ξ)+c2(±√u√2(tanpq(√2√u(tχ+ηx))±√pqsecpq(√2√u(tχ+ηx))))2, | (3.15) |
Q±13(x,t)=13(c2u−√c22u2−3c22ξ)+c2(±√u√2(cotpq(√2√u(tχ+ηx))±√pqcscpq(√2√u(tχ+ηx))))2, | (3.16) |
Q±14(x,t)=13(c2u−√c22u2−3c22ξ)+c2(±√u2√2(tanpq(√u(tχ+ηx)2√2)+cotpq(√u(tχ+ηx)2√2)))2. | (3.17) |
Mathematical calculations of achieved exact solutions are more proficient and advantageous in analyzing the dynamical behavior of non-linear wave phenomena based upon their graphical depiction. These obtained solutions elaborates different types of soliton solutions. Some of which are presented in 3D, 2D and contour plots with the help of maple. Figure 1 represents bright soliton solution, Figure 2 represents singular soliton solution for Eqs (3.4) and (3.5) respectively. Figures 3 and 5 demonstrate periodic singular soliton solutions for Eqs (3.7) and (3.17). Solution (3.10) represents dark-bright soliton and is plotted in Figure 4.
SSET is a realistic, effective, and expressive tool that has been successfully implemented in the Boussinesq equation to extract exact traveling wave solutions that are highly beneficial. The obtained results are in the form of rational, hyperbolic and trigonometric functions. As we can see, this method is powerful, efficient and simple tool for solving various types of nonlinear PDEs found in different models of engineering and natural sciences. The obtained results may be practical, beneficial and can explain the water waves in marine engineering, shallow water with long wavelength, optics, nonlinear grids, coupled circuits, elastic rods and so on. At the end 3D, 2D and contour plots of these solutions are sketched using maple.
The authors extend their appreciation to the Deanship of Scientific Research at King Khalid University, Abha, Saudi Arabia for funding this work through research groups program under grant number RGP.2/20/43.
The authors declare no conflict of interest.
[1] |
J. D. Ferreira, C. A. T. Salazar, P. C. C. Tabares, Weak Allee effect in a predator-prey model involving memory with a hump, Nonlinear Anal.: Real World Appl., 14 (2013), 536–548. https://doi.org/10.1016/j.nonrwa.2012.07.014 doi: 10.1016/j.nonrwa.2012.07.014
![]() |
[2] |
M. Cavani, M. Farkas, Bifurcations in a predator-prey model with memory and diffusion. I: Andronov-Hopf bifurcation, Acta Math. Hung., 63 (1994), 213–229. https://doi.org/10.1007/bf01874129 doi: 10.1007/bf01874129
![]() |
[3] |
M. Umar, Z. Sabir, M. A. Z. Raja, Intelligent computing for numerical treatment of nonlinear prey-predator models, Appl. Soft Comput., 80 (2019), 506–524. https://doi.org/10.1016/j.asoc.2019.04.022 doi: 10.1016/j.asoc.2019.04.022
![]() |
[4] |
Z. Sabir, T. Botmart, M. A. Z. Raja, W. Weera, An advanced computing scheme for the numerical investigations of an infection-based fractional-order nonlinear prey-predator system, Plos One, 17 (2022), 1–13. https://doi.org/10.1371/journal.pone.0265064 doi: 10.1371/journal.pone.0265064
![]() |
[5] |
U. Ghosh, S. Pal, M. Banerjee, Memory effect on Bazykin's prey-predator model: Stability and bifurcation analysis, Chaos Solitons Fract., 143 (2021), 1–10. https://doi.org/10.1016/j.chaos.2020.110531 doi: 10.1016/j.chaos.2020.110531
![]() |
[6] |
A. Gökçe, The influence of past in a population system involving intraspecific competition and Allee effect, Eur. Phys. J. Plus, 137 (2022), 1–11. https://doi.org/10.1140/epjp/s13360-022-02425-z doi: 10.1140/epjp/s13360-022-02425-z
![]() |
[7] |
B. Sahoo, S. Poria, Dynamics of predator-prey system with fading memory, Appl. Math. Comput., 347 (2019), 319–333. https://doi.org/10.1016/j.amc.2018.11.013 doi: 10.1016/j.amc.2018.11.013
![]() |
[8] |
L. Berec, E. Angulo, F. Courchamp, Multiple Allee effects and population management, Trends Ecol. Evol., 22 (2007), 185–191. https://doi.org/10.1016/j.tree.2006.12.002 doi: 10.1016/j.tree.2006.12.002
![]() |
[9] |
B. Souayeh, Z. Sabir, M. Umar, M. W. Alam, Supervised neural network procedures for the novel fractional food supply model, Fractal Fract., 6 (2022), 1–15. https://doi.org/10.3390/fractalfract6060333 doi: 10.3390/fractalfract6060333
![]() |
[10] | E. Angulo, G. M. Luque, S. D. Gregory, J. W. Wenzel, C. Bessa‐Gomes, L. Berec, et al., Allee effects in social species, J. Anim. Ecol., 87 (2018), 47–58. https://doi.org/10.1111/1365-2656.12759 |
[11] |
T. Perälä, J. A. Hutchings, A. Kuparinen, Allee effects and the Allee-effect zone in northwest Atlantic cod, Biol. Lett., 18 (2022), 1–6. https://doi.org/10.1098/rsbl.2021.0439 doi: 10.1098/rsbl.2021.0439
![]() |
[12] |
B. Dennis, Allee effects: Population growth, critical density, and the chance of extinction, Nat. Resour. Model., 3 (1989), 481–538. https://doi.org/10.1111/j.1939-7445.1989.tb00119.x doi: 10.1111/j.1939-7445.1989.tb00119.x
![]() |
[13] | T. Botmart, Z. Sabir, M. A. Z. Raja, M. R. Ali, R. Sadat, A. A. Aly, et al., A hybrid swarming computing approach to solve the biological nonlinear Leptospirosis system, Biomed. Signal Process. Control, 77 (2022), 103789. https://doi.org/10.1016/j.bspc.2022.103789 |
[14] | F. Courchamp, L. Berec, J. Gascoigne, Allee effects in ecology and conservation, 1 Ed., New York: Oxford University Press Inc., 2008. https://doi.org/10.1093/acprof:oso/9780198570301.001.0001 |
[15] | C. Çelik, H. Merdan, O. Duman, Ö. Akın, Allee effects on population dynamics with delay, Chaos Solitons Fract., 37 (2008), 65–74. https://doi.org/10.1016/j.chaos.2006.08.019 |
[16] |
J. P. Tripathi, P. S. Mandal, A. Poonia, V. P. Bajiya, A widespread interaction between generalist and specialist enemies: The role of intraguild predation and Allee effect, Appl. Math. Model., 89 (2021), 105–135. https://doi.org/10.1016/j.apm.2020.06.074 doi: 10.1016/j.apm.2020.06.074
![]() |
[17] |
P. C. Tabares, J. D. Ferreira, V. Rao, Weak Allee effect in a predator-prey system involving distributed delays, Comput. Appl. Math., 30 (2011), 675–699. https://doi.org/10.1590/S1807-03022011000300011 doi: 10.1590/S1807-03022011000300011
![]() |
[18] |
T. Botmart, W. Weera, Guaranteed cost control for exponential synchronization of cellular neural networks with mixed time-varying delays via hybrid feedback control, Abstr. Appl. Anal., 2013 (2013), 175796. https://doi.org/10.1155/2013/175796 doi: 10.1155/2013/175796
![]() |
[19] |
M. JovanoviĆ, M. KrstiĆ, Extinction in stochastic predator-prey population model with Allee effect on prey, Discrete Cont. Dyn. Syst. Ser. B, 22 (2017), 2651–2667. https://doi.org/10.3934/dcdsb.2017129 doi: 10.3934/dcdsb.2017129
![]() |
[20] |
P. J. Pal, T. Saha, M. Sen, M. Banerjee, A delayed predator–prey model with strong Allee effect in prey population growth, Nonlinear Dyn., 68 (2012), 23–42. https://doi.org/10.1007/s11071-011-0201-5 doi: 10.1007/s11071-011-0201-5
![]() |
[21] |
A. Surendran, M. J. Plank, M. J. Simpson, Population dynamics with spatial structure and an Allee effect, Proc. Math. Phys. Eng. Sci., 476 (2020), 20200501. https://doi.org/10.1098/rspa.2020.0501 doi: 10.1098/rspa.2020.0501
![]() |
[22] |
M. Jankovic, S. Petrovskii, Are time delays always destabilizing? Revisiting the role of time delays and the Allee effect, Theor. Ecol., 7 (2014), 335–349. https://doi.org/10.1007/s12080-014-0222-z doi: 10.1007/s12080-014-0222-z
![]() |
[23] | A. W. Stoner, M. Ray-Culp, Evidence for Allee effects in an over-harvested marine gastropod: Density-dependent mating and egg production, Mar. Ecol. Prog. Ser., 202 (2000), 297–302. http://dx.doi.org/10.3354/meps202297 |
[24] | F. Courchamp, B. T. Grenfell, T. H. Clutton‐Brock, Impact of natural enemies on obligately cooperative breeders, Oikos, 91 (2000), 311–322. https://doi.org/10.1034/j.1600-0706.2000.910212.x |
[25] |
M. Kuussaari, I. Saccheri, M. Camara, I. Hanski, Allee effect and population dynamics in the Glanville fritillary butterfly, Oikos, 82 (1998), 384–392. https://doi.org/10.2307/3546980 doi: 10.2307/3546980
![]() |
[26] |
Z. Ma, Hopf bifurcation of a generalized delay-induced predator-prey system with habitat complexity, Int. J. Bifurcat. Chaos, 30 (2020), 2050082. https://doi.org/10.1142/S0218127420500820 doi: 10.1142/S0218127420500820
![]() |
[27] |
H. Yu, M. Zhao, R. P. Agarwal, Stability and dynamics analysis of time delayed eutrophication ecological model based upon the Zeya reservoir, Math. Comput. Simul., 97 (2014), 53–67. https://doi.org/10.1016/j.matcom.2013.06.008 doi: 10.1016/j.matcom.2013.06.008
![]() |
[28] |
Y. Tang, L. Zhou, Stability switch and Hopf bifurcation for a diffusive prey–predator system with delay, J. Math. Anal. Appl., 334 (2007), 1290–1307. https://doi.org/10.1016/j.jmaa.2007.01.041 doi: 10.1016/j.jmaa.2007.01.041
![]() |
[29] |
A. Gökçe, A mathematical study for chaotic dynamics of dissolved oxygen-phytoplankton interactions under environmental driving factors and time lag, Chaos Solitons Fract., 151 (2021), 1–13. https://doi.org/10.1016/j.chaos.2021.111268 doi: 10.1016/j.chaos.2021.111268
![]() |
[30] |
K. Chakraborty, M. Chakraborty, T. K. Kar, Bifurcation and control of a bioeconomic model of a prey–predator system with a time delay, Nonlinear Anal.: Hybrid Syst., 5 (2011), 613–625. https://doi.org/10.1016/j.nahs.2011.05.004 doi: 10.1016/j.nahs.2011.05.004
![]() |
[31] |
H. Zhao, X. Huang, X. Zhang, Hopf bifurcation and harvesting control of a bioeconomic plankton model with delay and diffusion terms, Phys. A: Stat. Mech. Appl., 421 (2015), 300–315. https://doi.org/10.1016/j.physa.2014.11.042 doi: 10.1016/j.physa.2014.11.042
![]() |
[32] |
A. Gökçe, Numerical bifurcation analysis for a prey-predator type interactions with a time lag and habitat complexity, Bitlis Eren Ü niv. Fen Bilim. Derg., 10 (2021), 57–66. https://doi.org/10.17798/bitlisfen.840245 doi: 10.17798/bitlisfen.840245
![]() |
[33] | K. Gopalsamy, G. Ladas, On the oscillation and asymptotic behavior of ˙N(t)=N(t)[a+bN(t−τ)−cN2(t−τ)], Quart. Appl. Math., 48 (1990), 433–440. |
[34] |
M. Umar, Z. Sabir, F. Amin, J. L. Guirao, M. A. Z. Raja, Stochastic numerical technique for solving HIV infection model of CD4+ T cells, Eur. Phys. J. Plus, 135 (2020), 1–19. https://doi.org/10.1140/epjp/s13360-020-00417-5 doi: 10.1140/epjp/s13360-020-00417-5
![]() |
[35] |
Z. Sabir, Stochastic numerical investigations for nonlinear three-species food chain system, Int. J. Biomath., 15 (2022), 2250005. https://doi.org/10.1142/S179352452250005X doi: 10.1142/S179352452250005X
![]() |
[36] |
Z. Sabir, M. R. Ali, R. Sadat, Gudermannian neural networks using the optimization procedures of genetic algorithm and active set approach for the three-species food chain nonlinear model, J. Ambien. Intell. Human. Comput., 13 (2022), 1–10. https://doi.org/10.1007/s12652-021-03638-3 doi: 10.1007/s12652-021-03638-3
![]() |
[37] | M. Umar, Z. Sabir, M. A. Z. Raja, M. Shoaib, M. Gupta, Y. G. Sánchez, A stochastic intelligent computing with neuro-evolution heuristics for nonlinear SITR system of novel COVID-19 dynamics, Symmetry, 12 (2020), 1–17. https://doi.org/10.3390/sym12101628 |
[38] |
M. Umar, F. Amin, H. A. Wahab, D. Baleanu, Unsupervised constrained neural network modeling of boundary value corneal model for eye surgery, Appl. Soft Comput., 85 (2019), 1–16. https://doi.org/10.1016/j.asoc.2019.105826 doi: 10.1016/j.asoc.2019.105826
![]() |
[39] | B. Wang, J. F. Gomez-Aguilar, Z. Sabir, M. A. Z. Raja, W. F. Xia, H. Jahanshahi, et al., Numerical computing to solve the nonlinear corneal system of eye surgery using the capability of Morlet wavelet artificial neural networks, Fractals, 30 (2022), 1–19. https://doi.org/10.1142/S0218348X22401478 |
[40] |
Z. Sabir, Neuron analysis through the swarming procedures for the singular two-point boundary value problems arising in the theory of thermal explosion, Eur. Phys. J. Plus, 137 (2022), 1–18. https://doi.org/10.1140/epjp/s13360-022-02869-3 doi: 10.1140/epjp/s13360-022-02869-3
![]() |
[41] |
Z. Sabir, H. A. Wahab, Evolutionary heuristic with Gudermannian neural networks for the nonlinear singular models of third kind, Phys. Scr., 96 (2021), 1–12. https://doi.org/10.1088/1402-4896/ac3c56 doi: 10.1088/1402-4896/ac3c56
![]() |
[42] | T. Saeed, Z. Sabir, M. S. Alhodaly, H. H. Alsulami, Y. G. Sánchez, An advanced heuristic approach for a nonlinear mathematical based medical smoking model, Results Phys., 32 (2022), 1–13. https://doi.org/10.1016/j.rinp.2021.105137 |
[43] |
A. Gökçe, A dynamic interplay between Allee effect and time delay in a mathematical model with weakening memory, Appl. Math. Comput., 430 (2022), 127306. https://doi.org/10.1016/j.amc.2022.127306 doi: 10.1016/j.amc.2022.127306
![]() |
[44] |
M. R. Ali, S. Raut, S. Sarkar, U. Ghosh, Unraveling the combined actions of a Holling type III predator–prey model incorporating Allee response and memory effects, Comp. Math. Methods., 3 (2021), 1–18. https://doi.org/10.1002/cmm4.1130 doi: 10.1002/cmm4.1130
![]() |
[45] | A. Rojas-Palma, E. González-Olivares, Optimal harvesting in a predator-prey model with Allee effect and sigmoid functional response, Appl. Math. Model., 36 (2012), 1864–1874. https://doi.org/10.1016/j.apm.2011.07.081 |
[46] |
T. Botmart, N. Yotha, P. Niamsup, W. Weera, Hybrid adaptive pinning control for function projective synchronization of delayed neural networks with mixed uncertain couplings, Complexity, 2017 (2017), 4654020. https://doi.org/10.1155/2017/4654020 doi: 10.1155/2017/4654020
![]() |
[47] |
P. Lakshminarayana, K. Vajravelu, G. Sucharitha, S. Sreenadh, Peristaltic slip flow of a Bingham fluid in an inclined porous conduit with Joule heating, Appl. Math. Nonlinear Sci., 3 (2018), 41–54. https://doi.org/10.21042/AMNS.2018.1.00005 doi: 10.21042/AMNS.2018.1.00005
![]() |
[48] |
T. Sajid, S. Tanveer, Z. Sabir, J. L. G. Guirao, Impact of activation energy and temperature-dependent heat source/sink on Maxwell–Sutterby fluid, Math. Probl. Eng., 2020 (2020), 1–15. https://doi.org/10.1155/2020/5251804 doi: 10.1155/2020/5251804
![]() |
[49] |
R. Ahmad, A. Farooqi, J. Zhang, N. Ali, Steady flow of a power law fluid through a tapered non-symmetric stenotic tube, Appl. Math. Nonlinear Sci., 4 (2019), 255–266. https://doi.org/10.2478/AMNS.2019.1.00022 doi: 10.2478/AMNS.2019.1.00022
![]() |
[50] |
Z. Sabir, A. Imran, M. Umar, M. Zeb, M. Shoaib, M. A. Z. Raja, A numerical approach for 2-D Sutterby fluid-flow bounded at a stagnation point with an inclined magnetic field and thermal radiation impacts, Therm. Sci., 25 (2021), 1975–1987. https://doi.org/10.2298/TSCI191207186S doi: 10.2298/TSCI191207186S
![]() |
[51] |
Z. Sabir, M. A. Z. Raja, M. Shoaib, J. F. Aguilar, FMNEICS: Fractional Meyer neuro-evolution-based intelligent computing solver for doubly singular multi-fractional order Lane–Emden system, Comp. Appl. Math., 39 (2020), 1–18. https://doi.org/10.1007/s40314-020-01350-0 doi: 10.1007/s40314-020-01350-0
![]() |
[52] | H. Günerhan, E. Çelik, Analytical and approximate solutions of fractional partial differential-algebraic equations, Appl. Math. Nonlinear Sci., 5 (2020), 109–120. https://doi.org/10.2478/amns.2020.1.00011 |
[53] |
K. A. Touchent, Z. Hammouch, T. Mekkaoui, A modified invariant subspace method for solving partial differential equations with non-singular kernel fractional derivatives, Appl. Math. Nonlinear Sci., 5 (2020), 35–48. https://doi.org/10.2478/amns.2020.2.00012 doi: 10.2478/amns.2020.2.00012
![]() |
[54] |
Z. Sabir, M. A. Z. Raja, J. L. Guirao, T. Saeed, Meyer wavelet neural networks to solve a novel design of fractional order pantograph Lane-Emden differential model, Chaos Solitons Fract., 152 (2021), 1–14. https://doi.org/10.1016/j.chaos.2021.111404 doi: 10.1016/j.chaos.2021.111404
![]() |
[55] |
E. İlhan, İ. O. Kıymaz, A generalization of truncated M-fractional derivative and applications to fractional differential equations, Appl. Math. Nonlinear Sci., 5 (2020), 171–188. https://doi.org/10.2478/amns.2020.1.00016 doi: 10.2478/amns.2020.1.00016
![]() |
[56] |
H. M. Baskonus, H. Bulut, T. A. Sulaiman, New complex hyperbolic structures to the lonngren-wave equation by using sine-gordon expansion method, Appl. Math. Nonlinear Sci., 4 (2019), 129–138. https://doi.org/10.2478/AMNS.2019.1.00013 doi: 10.2478/AMNS.2019.1.00013
![]() |
1. | Gulaly Sadiq, Amir Ali, Shabir Ahmad, Kamsing Nonlaopon, Ali Akgül, Bright Soliton Behaviours of Fractal Fractional Nonlinear Good Boussinesq Equation with Nonsingular Kernels, 2022, 14, 2073-8994, 2113, 10.3390/sym14102113 | |
2. | Hamood Ur Rehman, Mustafa Inc, Muhammad Imran Asjad, Azka Habib, Qamar Munir, New soliton solutions for the space-time fractional modified third order Korteweg–de Vries equation, 2022, 24680133, 10.1016/j.joes.2022.05.032 | |
3. | Hamood ur Rehman, Aziz Ullah Awan, Azka Habib, Fehmi Gamaoun, ElSayed M. Tag El Din, Ahmed M. Galal, Solitary wave solutions for a strain wave equation in a microstructured solid, 2022, 39, 22113797, 105755, 10.1016/j.rinp.2022.105755 | |
4. | Muhammad Imran Asjad, Mustafa Inc, Ifrah Iqbal, Exact solutions for new coupled Konno–Oono equation via Sardar subequation method, 2022, 54, 0306-8919, 10.1007/s11082-022-04208-3 | |
5. | Kang-Jia Wang, Diverse soliton solutions to the Fokas system via the Cole-Hopf transformation, 2023, 272, 00304026, 170250, 10.1016/j.ijleo.2022.170250 | |
6. | Hamood Ur Rehman, Aziz Ullah Awan, Seham Ayesh Allahyani, ElSayed M. Tag-ElDin, Muhammad Ahsan Binyamin, Sadia Yasin, Exact solution of paraxial wave dynamical model with Kerr Media by using ϕ6 model expansion technique, 2022, 42, 22113797, 105975, 10.1016/j.rinp.2022.105975 | |
7. | Muhammad Zafarullah Baber, Nauman Ahmed, Muhammad Waqas Yasin, Muhammad Sajid Iqbal, Ali Akgül, Muhammad Bilal Riaz, Muhammad Rafiq, Ali Raza, Comparative analysis of numerical with optical soliton solutions of stochastic Gross–Pitaevskii equation in dispersive media, 2023, 44, 22113797, 106175, 10.1016/j.rinp.2022.106175 | |
8. | Aqilah Aljuaidan, Mamdouh Elbrolosy, Adel Elmandouh, Nonlinear Wave Propagation for a Strain Wave Equation of a Flexible Rod with Finite Deformation, 2023, 15, 2073-8994, 650, 10.3390/sym15030650 | |
9. | Kang-Jia Wang, Jing Si, Jing-Hua Liu, Diverse optical soliton solutions to the Kundu-Mukherjee-Naskar equation via two novel techniques, 2023, 273, 00304026, 170403, 10.1016/j.ijleo.2022.170403 | |
10. | Shao-Wen Yao, Selahattin Gulsen, M.S. Hashemi, Mustafa Inc, Harun Bicer, Periodic Hunter–Saxton equation parametrized by the speed of the Galilean frame: Its new solutions, Nucci’s reduction, first integrals and Lie symmetry reduction, 2023, 22113797, 106370, 10.1016/j.rinp.2023.106370 | |
11. | Tahira Sumbal Shaikh, Muhammad Zafarullah Baber, Nauman Ahmed, Muhammad Sajid Iqbal, Ali Akgül, Sayed M. El Din, Investigation of solitary wave structures for the stochastic Nizhnik–Novikov–Veselov (SNNV) system, 2023, 48, 22113797, 106389, 10.1016/j.rinp.2023.106389 | |
12. | Waqas Ali Faridi, Muhammad Abu Bakar, Zhaidary Myrzakulova, Ratbay Myrzakulov, Mawahib Elamin, Lakhdar Ragoub, Lanre Akinyemi, The Construction of Analytical Exact Soliton Waves of Kuralay Equation, 2024, 18, 2300-5319, 603, 10.2478/ama-2024-0064 | |
13. | Hassan Eltayeb, Said Mesloub, A Note on the Application of the Double Sumudu–Generalized Laplace Decomposition Method and 1+1- and 2+1-Dimensional Time-Fractional Boussinesq Equations, 2024, 16, 2073-8994, 665, 10.3390/sym16060665 | |
14. | Kang-Jia Wang, Feng Shi, Peng Xu, Multiple soliton, soliton molecules and the other diverse wave solutions to the (2+1)-dimensional Kadomtsev–Petviashvili equation, 2024, 38, 0217-9849, 10.1142/S0217984924502592 | |
15. | Rajesh Kumar Gupta, Vikash Yadav, On weakly nonlinear electron-acoustic waves in the fluid ions, bifurcation analysis, generalized symmetries and series solution propagated via Biswas–Milovic equation, 2023, 55, 0306-8919, 10.1007/s11082-023-04925-3 | |
16. | Asghar Ali, Jamshad Ahmad, Sara Javed, Shafqat-Ur- Rehman, Analysis of chaotic structures, bifurcation and soliton solutions to fractional Boussinesq model, 2023, 98, 0031-8949, 075217, 10.1088/1402-4896/acdcee | |
17. | Khalid K. Ali, M. S. Mehanna, Mohamed S. Mohamed, Optical soliton solutions for Kudryashov’s quintuple power-law coupled with dual form of non-local refractive index, 2023, 55, 0306-8919, 10.1007/s11082-023-05512-2 | |
18. | Nazek Alessa, Muhammad Shoaib Saleem, Hamood Ur Rehman, Sidra Noreen, Nonlinear wave dynamics in ferromagnetic media: A study with the Kuralay-IIA equation, 2024, 0217-9849, 10.1142/S0217984925500356 | |
19. | Hamood Ur Rehman, Sadia Yasin, Ifrah Iqbal, Optical soliton for (2+1)-dimensional coupled integrable NLSE using Sardar-subequation method, 2024, 38, 0217-9849, 10.1142/S0217984924500441 | |
20. | Tayyaba younas, Jamshad Ahmad, Dynamical behavior of the higher-order cubic-quintic nonlinear Schrödinger equation with stability analysis, 2024, 0972-8821, 10.1007/s12596-024-01864-4 | |
21. | Mashael M. ALBaidani, Umair Ali, Abdul Hamid Ganie, The closed-form solution by the exponential rational function method for the nonlinear variable-order fractional differential equations, 2024, 12, 2296-424X, 10.3389/fphy.2024.1347636 | |
22. | Hamood Ur Rehman, Aziz Ullah Awan, Sayed M. Eldin, Ifrah Iqbal, Study of optical stochastic solitons of Biswas-Arshed equation with multiplicative noise, 2023, 8, 2473-6988, 21606, 10.3934/math.20231101 | |
23. | Renfei Luo, Homan Emadifar, Mati ur Rahman, Bifurcations, chaotic dynamics, sensitivity analysis and some novel optical solitons of the perturbed non-linear Schrödinger equation with Kerr law non-linearity, 2023, 54, 22113797, 107133, 10.1016/j.rinp.2023.107133 | |
24. | Mi Chen, Zhen Wang, Analytical three-periodic solution and interaction for nonlocal Boussinesq equation, 2023, 138, 2190-5444, 10.1140/epjp/s13360-023-04518-9 | |
25. | Ifrah Iqbal, Hamood Ur Rehman, Mohammad Mirzazadeh, Mir Sajjad Hashemi, Retrieval of optical solitons for nonlinear models with Kudryashov’s quintuple power law and dual-form nonlocal nonlinearity, 2023, 55, 0306-8919, 10.1007/s11082-023-04866-x | |
26. | Khadija Shakeel, Alina Alb Lupas, Muhammad Abbas, Pshtiwan Othman Mohammed, Farah Aini Abdullah, Mohamed Abdelwahed, Construction of Soliton Solutions of Time-Fractional Caudrey–Dodd–Gibbon–Sawada–Kotera Equation with Painlevé Analysis in Plasma Physics, 2024, 16, 2073-8994, 824, 10.3390/sym16070824 | |
27. | Jamshad Ahmad, Tayyaba Younas, Dynamical behavior of soliton solutions to the fractional phi-four model via two analytical techniques, 2024, 38, 0217-9849, 10.1142/S021798492450310X | |
28. | Jamshad Ahmad, Tayyaba Younas, Wave structures of the (3+1)-dimensional nonlinear extended quantum Zakharov–Kuznetsov equation: analytical insights utilizing two high impact methods, 2024, 56, 1572-817X, 10.1007/s11082-024-06691-2 | |
29. | Kang-Jia Wang, Guo-Dong Wang, Feng Shi, Nonlinear dynamics of soliton molecules, hybrid interactions and other wave solutions for the (3+1)-dimensional generalized Kadomtsev–Petviashvili–Boussinesq equation, 2024, 38, 0217-9849, 10.1142/S021798492450194X | |
30. | Muhammad Shakeel, Xinge Liu, Almetwally M. Mostafa, Salman A. AlQahtani, Nouf F. AlQahtani, Mohamed R. Ali, Exploring of soliton solutions in optical metamaterials with parabolic law of nonlinearity, 2024, 56, 1572-817X, 10.1007/s11082-024-06452-1 | |
31. | Sidra Ghazanfar, Nauman Ahmed, Muhammad Sajid Iqbal, Syed Mansoor Ali, Ali Akgül, Shah Muhammad, Mubasher Ali, Murad Khan Hassani, Analysis of multi-wave solitary solutions of (2+1)-dimensional coupled system of Boiti–Leon–Pempinelli, 2024, 14, 2045-2322, 10.1038/s41598-024-67698-z | |
32. | Hamood Ur Rehman, Rehan Akber, Abdul-Majid Wazwaz, Hashim M. Alshehri, M.S. Osman, Analysis of Brownian motion in stochastic Schrödinger wave equation using Sardar sub-equation method, 2023, 289, 00304026, 171305, 10.1016/j.ijleo.2023.171305 | |
33. | Hamood Ur Rehman, Ghada S. Said, Aamna Amer, Hameed Ashraf, M.M. Tharwat, Mahmoud Abdel-Aty, Nasser S. Elazab, M.S. Osman, Unraveling the (4+1)-dimensional Davey-Stewartson-Kadomtsev-Petviashvili equation: Exploring soliton solutions via multiple techniques, 2024, 90, 11100168, 17, 10.1016/j.aej.2024.01.058 | |
34. | Dean Chou, Hamood Ur Rehman, Aamna Amer, Aatika Amer, New solitary wave solutions of generalized fractional Tzitzéica-type evolution equations using Sardar sub-equation method, 2023, 55, 0306-8919, 10.1007/s11082-023-05425-0 | |
35. | Karim K. Ahmed, Hamdy M. Ahmed, Wafaa B. Rabie, Mohammed F. Shehab, Effect of noise on wave solitons for (3+1)-dimensional nonlinear Schrödinger equation in optical fiber, 2024, 0973-1458, 10.1007/s12648-024-03222-3 | |
36. | Kang-Jia Wang, Manuel De León, The Perturbed Chen–Lee–Liu Equation: Diverse Optical Soliton Solutions and Other Wave Solutions, 2024, 2024, 1687-9120, 10.1155/2024/4990396 | |
37. | Anwar Ja’afar Mohamad Jawad, Anjan Biswas, Yakup Yildirim, Ali Saleh Alshomrani, Highly dispersive optical solitons with generalized quadratic—cubic form of self—phase modulation by Sardar sub—equation scheme, 2024, 0972-8821, 10.1007/s12596-024-01848-4 | |
38. | Kang-Jia Wang, Resonant multiple wave, periodic wave and interaction solutions of the new extended (3 + 1)-dimensional Boiti-Leon-Manna-Pempinelli equation, 2023, 111, 0924-090X, 16427, 10.1007/s11071-023-08699-x | |
39. | Mohammed F. Shehab, Mohamed M.A. El-Sheikh, Hamdy M. Ahmed, A.A. El-Gaber, Soliman Alkhatib, Effects of Wiener process on analytical wave solutions for (3+1) dimensional nonlinear Schrödinger equation using modified extended mapping method, 2024, 56, 22113797, 107297, 10.1016/j.rinp.2023.107297 | |
40. | Sibel Tarla, Karmina K Ali, Abdullahi Yusuf, Retracted: Exploring new optical solutions for nonlinear Hamiltonian amplitude equation via two integration schemes, 2023, 98, 0031-8949, 095218, 10.1088/1402-4896/aceb40 | |
41. | Karmina K. Ali, Abdullahi Yusuf, Soliton waves with optical solutions to the three-component coupled nonlinear Schrödinger equation, 2024, 39, 0217-7323, 10.1142/S0217732324500688 | |
42. | Muhammad Ishfaq Khan, Aamir Farooq, Kottakkaran Sooppy Nisar, Nehad Ali Shah, Unveiling new exact solutions of the unstable nonlinear Schrödinger equation using the improved modified Sardar sub-equation method, 2024, 59, 22113797, 107593, 10.1016/j.rinp.2024.107593 | |
43. | Kang-Jia Wang, Feng Shi, Jing-Hua Liu, Soliton molecules and the novel hybrid interaction solutions of the new extended (3 + 1)-dimensional Boiti–Leon–Manna–Pempinelli equation, 2024, 98, 0973-7111, 10.1007/s12043-024-02747-w | |
44. | Khalid K. Ali, Salman A. AlQahtani, M. S. Mehanna, Ahmet Bekir, New optical soliton solutions for the (2+1) Fokas system via three techniques, 2023, 55, 0306-8919, 10.1007/s11082-023-04900-y | |
45. | Chanidaporn Pleumpreedaporn, Elvin J. Moore, Sekson Sirisubtawee, Nattawut Khansai, Songkran Pleumpreedaporn, Exact Solutions for the Sharma–Tasso–Olver Equation via the Sardar Subequation Method with a Comparison between Atangana Space–Time Beta-Derivatives and Classical Derivatives, 2024, 12, 2227-7390, 2155, 10.3390/math12142155 | |
46. | Sibel Tarla, Karmina K. Ali, Hatıra Günerhan, Optical soliton solutions of generalized Pochammer Chree equation, 2024, 56, 1572-817X, 10.1007/s11082-024-06711-1 | |
47. | Rajib Mia, Arjun Kumar Paul, New exact solutions to the generalized shallow water wave equation, 2024, 38, 0217-9849, 10.1142/S0217984924503019 | |
48. | PENG XU, HUAN HUANG, HUI LIU, SEMI-DOMAIN SOLUTIONS TO THE FRACTAL (3+1)-DIMENSIONAL JIMBO–MIWA EQUATION, 2024, 32, 0218-348X, 10.1142/S0218348X24400425 | |
49. | Kang-Jia Wang, Feng Shi, Non-singular complexiton, singular complexiton and complex N-soliton solutions of the new extended (3+1)-dimensional Boiti-Leon-Manna-Pempinelli equation, 2024, 99, 0031-8949, 035251, 10.1088/1402-4896/ad2966 | |
50. | Hira Tariq, Hira Ashraf, Hadi Rezazadeh, Ulviye Demirbilek, Travelling wave solutions of nonlinear conformable Bogoyavlenskii equations via two powerful analytical approaches, 2024, 39, 1005-1031, 502, 10.1007/s11766-024-5030-7 | |
51. | Waqas Ali Faridi, Muhammad Abu Bakar, Zhaidary Myrzakulova, Ratbay Myrzakulov, Ali Akgül, Sayed M. El Din, The formation of solitary wave solutions and their propagation for Kuralay equation, 2023, 52, 22113797, 106774, 10.1016/j.rinp.2023.106774 | |
52. | Yan-Nan Chen, Kang-Jia Wang, On the Wave Structures to the (3+1)-Dimensional Boiti–Leon–Manna–Pempinelli Equation in Incompressible Fluid, 2023, 12, 2075-1680, 519, 10.3390/axioms12060519 | |
53. | Manar S. Ahmed, Ahmed H. Arnous, Khaled A. Gepreel, Aydin Secer, Analyzing the influence of multiplicative white noise on optical solitons in birefringent fibers through the perturbed Gerdjikov–Ivanov model, 2024, 56, 1572-817X, 10.1007/s11082-024-07329-z | |
54. | Mehmet Şenol, Mehmet Gençyiğit, Ulviye Demirbilek, Emad A. Az-Zo’bi, Sensitivity and wave propagation analysis of the time-fractional (3+1)-dimensional shallow water waves model, 2024, 75, 0044-2275, 10.1007/s00033-024-02216-9 | |
55. | Tariq Alraqad, Muntasir Suhail, Hicham Saber, Khaled Aldwoah, Nidal Eljaneid, Amer Alsulami, Blgys Muflh, Investigating the Dynamics of a Unidirectional Wave Model: Soliton Solutions, Bifurcation, and Chaos Analysis, 2024, 8, 2504-3110, 672, 10.3390/fractalfract8110672 | |
56. | Muhammad Zain Yousaf, Muhammad Abbas, Muhammad Kashif Iqbal, Imen Ali Kallel, Adequate dynamical perspective of traveling wave solutions to the perturbed Boussinesq equation appearing in ocean engineering, 2024, 2198-6444, 10.1007/s40722-024-00359-5 | |
57. | Zeting Li, Ben Gao, Investigation for the exact solutions of two classes of extended Sakovich equations, 2025, 533, 03759601, 130203, 10.1016/j.physleta.2024.130203 | |
58. | Mingyue Wang, Youhe Zhou, Jizeng Wang, New exact traveling wave solutions of the coupled Boussinesq equations, 2025, 20950349, 100568, 10.1016/j.taml.2024.100568 | |
59. | Kang-Jia Wang, Xiao-Lian Liu, Feng Shi, Geng Li, Bifurcation and sensitivity analysis, chaotic behaviors, variational principle, Hamiltonian and diverse wave solutions of the new extended integrable Kadomtsev–Petviashvili equation, 2025, 03759601, 130246, 10.1016/j.physleta.2025.130246 | |
60. | Kang‐Jia Wang, Bo‐Rong Zou, Hong‐Wei Zhu, Shuai Li, Geng Li, Phase Portrait, Bifurcation and Chaotic Analysis, Variational Principle, Hamiltonian, Novel Solitary, and Periodic Wave Solutions of the New Extended Korteweg–de Vries–Type Equation, 2025, 0170-4214, 10.1002/mma.10852 | |
61. | Sibel Tarla, Karmina K. Ali, Abdullahi Yusuf, Berna Uzun, Soheil Salahshour, Exact solutions of the (2+1)-dimensional Konopelchenko–Dubrovsky system using Sardar sub-equation method, 2025, 39, 0217-9849, 10.1142/S0217984924504852 |