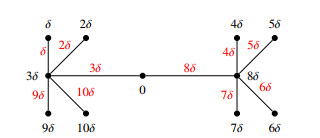
In this paper, we propose a Dickey-Fuller difference statistic to sequentially detect the change-point that shift from an unit root process to a long-memory process. The limiting distribution of monitoring statistic under the unit root process null hypothesis as well as its consistency under the alternative hypothesis are proved. Simulations indicate that the new method can control the empirical size well even for the heavy-tailed unit root process when using the sieve bootstrap method computing its critical values. In particular, it performs significantly better than the available method in the literature under the alternative hypothesis. Finally, we illustrate the new monitoring procedure by a set of foreign exchange rate data.
Citation: Zhanshou Chen, Muci Peng, Li Xi. A new procedure for unit root to long-memory process change-point monitoring[J]. AIMS Mathematics, 2022, 7(4): 6467-6477. doi: 10.3934/math.2022360
[1] | Huda Alsaud, Muhammad Owais Kulachi, Aqeel Ahmad, Mustafa Inc, Muhammad Taimoor . Investigation of SEIR model with vaccinated effects using sustainable fractional approach for low immune individuals. AIMS Mathematics, 2024, 9(4): 10208-10234. doi: 10.3934/math.2024499 |
[2] | Muhammad Farman, Aqeel Ahmad, Ali Akgül, Muhammad Umer Saleem, Kottakkaran Sooppy Nisar, Velusamy Vijayakumar . Dynamical behavior of tumor-immune system with fractal-fractional operator. AIMS Mathematics, 2022, 7(5): 8751-8773. doi: 10.3934/math.2022489 |
[3] | Tahir Khan, Fathalla A. Rihan, Muhammad Bilal Riaz, Mohamed Altanji, Abdullah A. Zaagan, Hijaz Ahmad . Stochastic epidemic model for the dynamics of novel coronavirus transmission. AIMS Mathematics, 2024, 9(5): 12433-12457. doi: 10.3934/math.2024608 |
[4] | Muhammad Farman, Ali Akgül, Sameh Askar, Thongchai Botmart, Aqeel Ahmad, Hijaz Ahmad . Modeling and analysis of fractional order Zika model. AIMS Mathematics, 2022, 7(3): 3912-3938. doi: 10.3934/math.2022216 |
[5] | Hasib Khan, Jehad Alzabut, Anwar Shah, Sina Etemad, Shahram Rezapour, Choonkil Park . A study on the fractal-fractional tobacco smoking model. AIMS Mathematics, 2022, 7(8): 13887-13909. doi: 10.3934/math.2022767 |
[6] | Aziz Khan, Thabet Abdeljawad, Manar A. Alqudah . Neural networking study of worms in a wireless sensor model in the sense of fractal fractional. AIMS Mathematics, 2023, 8(11): 26406-26424. doi: 10.3934/math.20231348 |
[7] | Zhengwen Yin, Yuanshun Tan . Threshold dynamics of stochastic SIRSW infectious disease model with multiparameter perturbation. AIMS Mathematics, 2024, 9(12): 33467-33492. doi: 10.3934/math.20241597 |
[8] | Shah Hussain, Naveed Iqbal, Elissa Nadia Madi, Mohsen Bakouri, Ilyas Khan, Wei Sin Koh . On the stochastic modeling and forecasting of the SVIR epidemic dynamic model under environmental white noise. AIMS Mathematics, 2025, 10(2): 3983-3999. doi: 10.3934/math.2025186 |
[9] | Amir Ali, Abid Ullah Khan, Obaid Algahtani, Sayed Saifullah . Semi-analytical and numerical computation of fractal-fractional sine-Gordon equation with non-singular kernels. AIMS Mathematics, 2022, 7(8): 14975-14990. doi: 10.3934/math.2022820 |
[10] | Najat Almutairi, Sayed Saber, Hijaz Ahmad . The fractal-fractional Atangana-Baleanu operator for pneumonia disease: stability, statistical and numerical analyses. AIMS Mathematics, 2023, 8(12): 29382-29410. doi: 10.3934/math.20231504 |
In this paper, we propose a Dickey-Fuller difference statistic to sequentially detect the change-point that shift from an unit root process to a long-memory process. The limiting distribution of monitoring statistic under the unit root process null hypothesis as well as its consistency under the alternative hypothesis are proved. Simulations indicate that the new method can control the empirical size well even for the heavy-tailed unit root process when using the sieve bootstrap method computing its critical values. In particular, it performs significantly better than the available method in the literature under the alternative hypothesis. Finally, we illustrate the new monitoring procedure by a set of foreign exchange rate data.
Labeling graphs have attracted the attention of numerous researchers in different disciplines. The importance of this research line is in fact due to the following:
(1) Finding different coding techniques for securing the communication networks and database managements.
(2) Providing a high-level secrecy to military services which is the most important factor for coding.
(3) Providing confidentiality, and integrity of messages transferred between group members which is a critical networking issue. For more application, see [1,2].
Coding through special kinds of graphs with different kinds of labeling is structured by many papers. Coding with Fibonacci web graph using super mean labeling was introduced by Uma Maheswari et al. [3]. Prasad et al. developed a technique of coding secret messages using sun flower graphs SFn. Furthermore, every labeling graph can be converted to a code by using GMJ coding methods (see, [4] and the references therein)
A graph G is a pair (V,E), where V(G) and E(G) denote the vertex set and edge set of a graph G. The position of the vertices and the length of the edges do not concern us, what is important is the size of a graph (number of vertices) and the pairs of vertices which are connected by an edge. If e={u,v} is an edge of a graph G, then u and v are adjacent while u and e are incident. Let q=|E(G)| be the cardinality of E(G) and p=|V(G)| be that of V(G). For every vertex u∈V(G), the open neighborhood set N(u) is the set of all vertices adjacent to u in G. Graph operations[5] allow us to generate many new graphs from old ones. A fan graph Fn is defined as the join Pn+K1 where Pn is the path graph on n vertices and K1 is a complete graph on one vertex. The crown graph Crn(Sunlet graph) is the graph on 2n vertices obtained by attaching n pendant edges to a cycle graph Cn, i.e., the coronas Cn⊙K1.
Definition 1.1. (i) The splitting graph S′(G) of a connected graph G is the graph obtained by adding new vertex ui corresponding to each vertex vi of V(G) such that N(vi)=N(ui).
(ii) The shadow graph D2(G) of a connected graph G is constructed by taking two copies of G, say G1 and G2. Join each vertex vi in G1 to the neighbors of the corresponding vertex ui in G2. The shadow graph D2(G) can be obtained from the splitting graph S′(G) by adding edge between any two new vertices ui and uj if the corresponding original vertices vi and vj are adjacent. V[D2(G)]=V[S′(G)], E[D2(G)]=E[S′(G)]∪{uiui+1,i=1,2,⋯,n}.
(iii) The middle graph M(G) of a connected graph G is the graph whose vertex set is V(G)∪E(G) and in which two vertices in M(G) are adjacent whenever either they are adjacent edges of G or one is a vertex of G and the other is an edge incident with it.
(iv) The total graph T(G) of a connected graph G is a graph such that the vertex set of T(G) corresponds to the vertices and edges of G and two vertices are adjacent in T(G) if their corresponding elements are either adjacent or incident in G. The total graph T(G) can be obtained from the middle graph M(G) by adding edge between any two original vertices vi and vj if the corresponding new vertices ui and uj are adjacent. V[T(G)]=V[M(G)], E[T(G)]=E[M(G)]∪E(G).
There are many different kinds of graph labeling[6,7,8,9,10,11,12,13], all that kinds of labeling problem will have following three common characteristics. A set of numbers from which vertex or edge labels are chosen, A rule that assigns a value to each edge or vertex, A condition that these values must satisfy. For a comprehensive survey on graph labeling refers to a dynamic survey of graph labeling [14].
Zeen El Deen [15] introduced the edge δ− graceful labeling of graphs by using the numbers {δ,2δ,3δ, ⋯,qδ} to label the edges of a graph, for any positive integer δ. He showed edge δ− graceful labeling for some graphs related to cycles.
Definition 1.2. An edge δ− graceful labeling of a graph G=(V(G),E(G)) with p=|V(G)| vertices and q=|E(G)| edges is a bijective mapping f of the edge set E(G) into the set {δ,2δ,3δ,⋯,qδ} such that the induced mapping f∗:V(G)→{0,δ,2δ,3δ,⋯,kδ−δ}, given by: f∗(u)=(∑uv∈E(G)f(uv))mod(δk), where k=max(p,q), is an injective function. The graph that admits an edge δ− graceful labeling is called an edge δ− graceful graph, if δ=2 we have the edge even labeling also if δ=3 we have the edge triple labeling and so on.
Example 1.1. In Figure 1 we present an edge δ− graceful labeling of a tree graph on p=11 vertices and q=10 edges f:E(G)→{δ,2δ,3δ,⋯,10δ} and f∗:V(G)→{0,δ,2δ,3δ,⋯,10δ}, given by: f∗(u)=(∑uv∈E(G)f(uv))mod(11δ).
Example 1.2. Duplication of an edge e=(xy) by a new vertex u in a graph G produces a new graph H such that N(u)={x,y}. Let {v1,v2,⋯,vn} be the vertex set in the path Pn and G be the graph obtained by duplication of each edge vivi+1 of path Pn by vertex ui,(1≤i<n). Then V(G)=V(Pn)∪{u1,u2,⋯,un−1} and the edge set are {vivi+1,viui,uivi+1,i=1,2,⋯,n−1}, so the graph G has p=2n−1 vertices and q=3n−3 edges, k=max(p,q)=3n−3. An edge 5− graceful labeling of a graph obtained duplication of each edge of P11 by a vertex is shown in Figure 2.
Theorem 2.1. For any positive integer δ, the splitting graph S′(Cn) of the cycle Cn,n>3 is an edge δ− graceful graph.
Proof. Let {v1,v2,⋯,vn} be the vertices of Cn where these vertices are in their natural order module n. To form the splitting graph S′(Cn) we add new vertices {u1,u2,⋯,un} corresponding to vertices of Cn. The edges set of S′(Cn) are {vivi+1,viui+1,uivi+1,i=1,2,⋯,n}, the graph S′(Cn) has p=2n vertices and q=3n edges, k=max(p,q)=3n.
Case (1): When n≡2mod4,n>3. We define the labeling function f:E(S′(Cn))⟶{δ,2δ,⋯,(3n)δ} as follows:
f(viui+1)=δ(i),for1≤i≤n, |
f(vivi+1)={δ(n+1),if i=1;δ(2n−1+i),if 2≤i≤n. |
f(uivi+1)={δ(2n),if i=1;δ(n+i),if 2≤i≤n−1; δ(3n),if i=n. |
In view of the above labeling pattern then the induced vertex labels are:
f∗(v1)=(n+1)δ,f∗(v2)=(2n+4)δ,f∗(u1)=0,f∗(un)=(n−1)δ, and
f∗(vi)=(2n+4i−4)δmod(3nδ),for3≤i≤n,
f∗(ui)=(n+2i−1)δmod(3nδ),for2≤i≤n−1.
Hence the vertex labels are all distinct and a multiple of δ.
Case (2): When n≡0mod4,n=4, the graph S′(C4) is an edge δ− graceful graph for any positive integer δ define the labeling function f:E(S′(C4))⟶{δ,2δ,⋯,(12)δ} as shown in Figure 3.
∙ When n≡0mod4,n>4, we define the labeling function f as follows:
f(uivi+1)=δ(n+i),for1≤i≤n, |
f(viui+1)={δ(i+1),if 2≤i≤n−1;δ,if i=n. |
f(vivi+1)={δ(2n+i),if 1≤i≤n−3;δ(4n−2−i),if n−2≤i≤n. |
In view of the above labeling pattern then the induced vertex labels are:
f∗(v1)=(n+1)δ,f∗(vn−2)=(3n−7)δ,f∗(vn−1)=(3n−3)δ,f∗(vn)=(2n−3)δ,
f∗(vi)=(2n+4i−1)δmod(3nδ),for2≤i≤n−3,
f∗(u1)=(n+2)δ,f∗(ui)=(n+2i)δmod(3nδ),for2≤i≤n.
Hence the vertex labels are all distinct and a multiple of δ.
Case (3): When n is odd, we define the labeling function f as follows:
f(uivi+1)=δ(3i−2),for1≤i≤n,f(vivi+1)=δ(3i−1),for1≤i≤n,f(viui+1)=δ(3i),for1≤i≤n. |
In view of the above labeling pattern then the induced vertex labels are:
f∗(vi)=(12i−10)δmod(3nδ),for1≤i≤n, and f∗(ui)=(6i−5)δmod(3nδ),for1≤i≤n |
Hence the vertex labels are all distinct and a multiple of δ. Therefore S′(Cn) admits an edge δ− graceful labeling.
Illustration: The splitting graphs S′(C8) with an edge 4− graceful labeling, S′(C9) with an edge 5− graceful labeling and splitting graph S′(C10) with an edge 3− graceful labeling are shown in Figure 4.
Theorem 2.2. For any positive integer δ, the splitting graph S′(Fn) of the fan graph Fn is an edge δ− graceful graph.
Proof. Let {v0,v1,v2,⋯,vn} be the vertices of the fan Fn, to form the splitting graph S′(Fn) we add new vertices {u0,u1,u2,⋯,un} corresponding to the vertices of the fan Fn. The edges set of S′(Fn) are {viui+1,vivi+1,uivi+1,1≤i≤n−1}∪{uiv0,viu0,viv0,1≤i≤n}, the graph S′(Fn) has p=2n+2 vertices and q=6n−3 edges, k=max(p,q)=6n−3, see Figure 5.
Case (1): When n is even, we define the labeling function f:E(S′(Fn))⟶{δ,2δ,⋯,(6n−3)δ} as follows:
f(v0vi)={δ(n),if i=1;δ(i),if 2≤i≤n−1; δ,if i=n. |
f(uivi+1)=δ(n+i),for1≤i≤n−1, |
f(viui+1)=δ(2n−1+i),for1≤i≤n−1, |
f(u0vi)={δ(3n−2+i),if 1≤i≤n−1;δ(6n−3),if i=n. |
f(vivi+1)=δ(5n−3−i),for1≤i≤n−1, |
f(v0ui)=δ(6n−3−i),for1≤i≤n. |
In view of the above labeling pattern then the induced vertex labels are:
f∗(v0)=0,f∗(v1)=(5n−2)δ,f∗(vn)=δ,
f∗(u1)=(n)δ,f∗(un)=(2n−2)δ,
f∗(vi)=(4n−3+2i)δmod[(6n−3)δ],for2≤i≤n−1,
f∗(ui)=(3n−2+i)δmod[(6n−3)δ],for2≤i≤n−1,
Finally, f∗(u0)=[n−1∑i=1f(u0vi)+f(u0vn)]mod(6n−3)δ=[n−1∑i=1(3n−2+i)δ]mod(6n−3)δ
=[(7n22−11n2+2)δ]mod(6n−3)δ.
If n≡2mod12⟹n=12k+2
f∗(u0)=[(504k2+102k+5)δ]mod(72k+9)δ=[(39k+5)δ]mod(72k+9)δ
=[(13n−64)δ]mod(6n−3)δ.
Similarly, If n≡0mod12⟹n=12k then f∗(u0)=[(9n−44)δ]mod(6n−3)δ.
If n≡4mod12⟹n=12k+4 then f∗(u0)=[(17n−84)δ]mod(6n−3)δ.
If n≡6mod12⟹n=12k+6, then f∗(u0)=[(21n−104)δ]mod(6n−3)δ.
If n≡8mod12⟹n=12k+8 then f∗(u0)=[(n4)δ]mod(6n−3)δ.
If n≡10mod12⟹n=12k+10 then f∗(u0)=[(5n−24)δ]mod(6n−3)δ.
Case (2): When n is odd, n>3, we define the labeling function f as follows:
f(viui+1)=δ(i),for1≤i≤n−1, |
f(v0ui)=δ[32(n−1)+i],for1≤i≤n, |
f(v0vi)=δ(9n−32−i),for1≤i≤n. |
f(vivi+1)={δ(5n−1),if i=1;δ(9n−52+i2),if i=2,4,⋯,n−3,n−1; δ(n+i−32),if i=3,5,⋯,n−4,n−2. |
f(uivi+1)={δ(32(n−1)),if i=1;δ(6n−2−i),if i=2,3,⋯,n−2; δ(5n−12),if i=n−1. |
f(viu0)={δ(6n−3),if i=1;δ(5n−32+i),if i=2,3,⋯,n−1; δ(5n−2),if i=n. |
In view of the above labeling pattern then the induced vertex labels are:
f∗(v0)=0,f∗(v1)=(7n+12)δ,f∗(v2)=(4)δ,f∗(vn)=(4n−1)δ,
f∗(u1)=(3n−2)δ,f∗(un−1)=(6n−5)δ,f∗(un)=(7n−52)δ,
f∗(vi)=(372n−172+i)δmod[(6n−3)δ]=(n+12+i)δ,for3≤i≤n−1,
f∗(ui)=(15n−92+i)δmod[(6n−3)δ]=(3n−32+i)δ,for2≤i≤n−2,
Finally, f∗(u0)=∑ni=1f(u0vi)=[∑n−1i=2f(u0vi)+f(u0v1)+f(u0vn)]mod(6n−3)δ
=[∑n−1i=2(52n−32+i)δ+(5n−2)δ]mod(6n−3)δ=[(3n2−2n)δ]mod(6n−3)δ
∵n≡1mod2⟹n=2k+1
f∗(u0)=[(12k2+8k+1)δ]mod(12k+3)δ=[(5k+1)δ]mod(12k+3)δ=(5n−32)δ.
Hence the vertex labels are all distinct and a multiple of δ. Therefore S′(Fn) admits an edge δ− graceful labeling.
Illustration: The splitting graphs S′(F8) with an edge 5− graceful labeling andS′(F9) with an edge 4− graceful labeling are presented in Figure 6.
Theorem 2.3. For any positive integer δ, the splitting graph S′(Crn) of the crown graph is an edge δ− graceful graph.
Proof. Let {v1,v2,⋯,vn} and {u1,u2,⋯,un} be the vertices of the crown Crn, to form the splitting graph S′(Crn) we add newly vertices {v′1,v′2,⋯,v′n} and {u′1,u′2,⋯,u′n} corresponding to the vertices of the crown Crn. The edges set of the splitting graph S′(Crn) are {viu′i,v′ivi+1, viv′i+1,vivi+1,v′iui,uivi,1≤i≤n}, see Figure 7. The graph S′(Crn) has p=4n vertices and q=6n edges, k=max(p,q)=6n.
Case (1): When n is odd. We define the labeling function f:E(S′(Crn))⟶{δ,2δ,⋯,(6n)δ} as follows:
f(v′ivi+1)=δ(n+i),for1≤i≤n, |
f(v′iui)=δ(5n−i),for1≤i≤n, |
f(uivi)=δ(6n−i),for1≤i≤n, |
f(viv′i+1)=δ(3n−i),for1≤i≤n−1, and f(vnv′1)=δ(6n), |
f(vivi+1)=δ(3n+i),for1≤i≤n−1, and f(vnv1)=δ(3n), |
f(viu′i)={δ(n),if i=1;δ(i),if for2≤i≤n−1; δ,if i=n. |
In view of the above labeling pattern then the induced vertex labels are:
f∗(u′1)=nδ,f∗(u′n)=δ,f∗(v′1)=0,f∗(v1)=(6n−1)δ,f∗(vn)=(2n−1)δ,
f∗(ui)=(5n−2i)δ,for1≤i≤n,
f∗(u′i)=(i)δ,for2≤i≤n−1,
f∗(v′i)=(3n−i+1)δ,for2≤i≤n,
f∗(vi)=(4n+2i−2)δ,for2≤i≤n−1.
Case (2): When n is even. The labeling function f:E(S′(Crn))⟶{δ,2δ,⋯,(6n)δ} defined as follows:
f(uivi)=δ(i),for1≤i≤n,
f(v′ivi+1)=δ(3n+i),for1≤i≤n,
f(viv′i+1)=δ(5n+1−i),for1≤i≤n,
f(v1u′1)=δ(6n),f(viu′i)=δ(2n+1−i),for2≤i≤n,
f(v′1u1)=δ(6n−2),f(v′iui)=δ(2n+i),for2≤i≤n,
f(vivi+1)={δ(5n−1+2i),if 1≤i≤n2; δ(2n+1),if i=n2+1;δ(4n+2i−2),if n2+2≤i≤n−1;δ(2n),if i=n. |
In view of the above labeling pattern then the induced vertex labels are:
f∗(v1)=(4n+2)δ,f∗(vn2+1)=δ,f∗(vn2+2)=(5n+4)δ,f∗(vn)=(6n−3)δ,
f∗(u1)=(6n−1)δ,f∗(u′1)=0,f∗(v′1)=(n)δ,
f∗(ui)=(2n+2i)δ,for2≤i≤n,
f∗(u′i)=(2n+1−i)δ,for2≤i≤n,
f∗(v′i)=(4n+i+2)δ,for2≤i≤n,
f∗(vi)=(2n+4i−3)δ,for2≤i≤n2,
f∗(vi)=(4i−5)δ,forn2+3≤i≤n−1.
Hence the vertex labels are all distinct. Therefore S′(Crn) admits an edge δ− graceful labeling.
Illustration: The splitting graphs S′(Cr8) of the crown graph with an edge 5− graceful labeling and S′(Cr9) with an edge 3− graceful labeling are shown in Figure 8.
Theorem 3.1. For any positive integer δ, the shadow graph D2(Pn),n>2 of the path Pn is an edge δ− graceful graph.
Proof. Let {v1,v2,⋯,vn} be the vertices in first copy of Pn and {u1,u2,⋯,un} be that in second copy of Pn. The edges set in the shadow graph D2(Pn) are {vivi+1,viui+1,uivi+1,uiui+1,1≤i≤n−1}. The four vertices v1,vn,u1 and un are of degree 2 and the remaining vertices are of degree 4, so the graph D2(Pn) has p=2n vertices and q=4n−4 edges, k=max(p,q)=4n−4.
∙ If n=2 the graph D2(P2) is not an edge δ− graceful graph since it isomorphic to C4 [2].
∙ If n=3 the graph D2(P3) is an edge δ− graceful graph for any positive integer δ define the labeling function f:E(D2(P3))⟶{δ,2δ,⋯,8δ} as shown in Figure 9.
∙ If n≥4. We define the labeling function f:E(D2(Pn))⟶{δ,2δ,⋯,(4n−4)δ} as follows:
f(viui+1)=δi,for1≤i≤n−1, |
f(vivi+1)=δ(4n−4−i),for1≤i≤n−1, |
f(uivi+1)=δ(2n−i−1),for1≤i≤n−1, |
f(u1u2)=δ(4n−4),f(u2u3)=δ(2n),f(u3u4)=δ(2n−1), |
f(uiui+1)=δ(2n+i−3),for4≤i≤n−1. |
In view of the above labeling pattern then the induced vertex labels are:
f∗(v1)=0,f∗(vn)=δ,f∗(u1)=(2n−2)δ,f∗(un)=(4n−5)δ,
f∗(u2)=2δ,f∗(u3)=(2n+1)δ,f∗(u4)=(2n+2)δ,
f∗(vi)=[f(vivi+1)+f(vi−1vi)+f(viui−1)+f(viui+1)]mod[(4n−4)δ]
=(2n+1−2i)δ,for2≤i≤n−1.
Similarly, f∗(ui)=(2n−5+2i)δ,for5≤i≤n−1.
Hence the labels of the vertices are all distinct numbers and a multiple of δ. Thus D2(Pn) is an edge δ− graceful graph.
Illustration: The shadow graph D2(P13) with edge 3− graceful labeling is presented in Figure 10.
Theorem 3.2. For any positive integer δ, the shadow graph D2(Cn),n≥3 of the cycle Cn is an edge δ− graceful graph.
Proof. Let {v1,v2,⋯,vn} be the vertices in first copy of Cn and {u1,u2,⋯,un} be that in second copy of Cn. According to the construction of the shadow graph D2(Cn) of the cycle Cn, the edges set in the shadow graph D2(Cn) are {vivi+1,viui+1,uivi+1,uiui+1,i=1,2,⋯,n}. All the vertices vi and ui are of degree 4, so the graph D2(Cn) has p=2n vertices and q=4n edges, k=max(p,q)=4n. There are two cases:
Case (1): When n is odd, we define the labeling f:E(D2(Cn)⟶{δ,2δ,3δ,⋯,4nδ} as follows:
f(v1vn)=δn,f(vivi+1)=δifor1≤i≤n−1, f(u1un)=δ2n,f(uiui+1)=δ(n+i)for1≤i≤n−1, f(v1un)=δ3n,f(viui+1)=δ(4n−i)for1≤i≤n−1, f(u1vn)=δ4n,f(uivi+1)=δ(2n+i)for1≤i≤n−1. |
In view of the above labeling pattern we have:
f∗(v1)=nδ and f∗(vi)=[2δ(n+i−1)]mod(4n)δ,for2≤i≤n,
f∗(un)=3nδ and f∗(ui)=(2δi)mod(4n)δ,for1≤i≤n−1.
Hence the labels of the vertices v2,v3,v4,⋯,vn−1,vn are 2δ(n+1),2δ(n+2),2δ(n+3),⋯,2δ(2n−2),2δ(2n−1), respectively, and the labels of the vertices u1,u2,u3,⋯,un−1,un are 2δ,4δ,6δ,⋯,2δ(n−1),3δn, respectively, which are distinct numbers.
Case (2): When n is even, we define the labeling f as follows:
f(uivi+1)=iδ,for1≤i≤n,
f(v1vn)=δ(4n−1),f(vivi+1)=δ(2n+i),for1≤i≤n−1,
f(vnu1)=δ2n,f(viui+1)=δ(4n−1−i),for1≤i≤n−1,
f(unu1)=δ4n,f(uiui+1)=δ(n+i),for1≤i≤n−1.
In view of the above labeling pattern we have:
f∗(u1)=(3n+2)δ,f∗(un)=(2n−1)δ,f∗(v1)=(3n−2)δ,f∗(vn)=(2n−3)δ,
f∗(ui)=(2n−1+2i)δmod(4n)δ,for2≤i≤n−1,
f∗(vi)=[δ(2i−3)]mod(4n)δ,for2≤i≤n−1.
Obviously the vertex labels are all distinct. Thus, the graph D2(Cn) is an edge δ− graceful graph for all n.
Illustration: The shadow graph D2(C9) with an edge 5− graceful labeling and D2(C10) with an edge 3− graceful labeling are shown in Figure 11.
Theorem 3.3. For any positive integer δ, the shadow graph D2(Fn),n≥3 of the fan graph Fn is an edge δ− graceful graph.
Proof. Let {v0,v1,v2,⋯,vn} be the vertices in first copy of Fn and {u0,u1,u2,⋯,un} be that in second copy of Fn. The edges set in the shadow graph D2(Fn) are {viui+1,vivi+1,uivi+1,uiui+1,1≤i≤n−1}∪{uiv0,viu0,viv0,u0ui,1≤i≤n}, so the graph D2(Fn) has number of total vertices p=|V(D2(Fn)|=2n+2 and edges q=|E(D2(Fn)|=8n−4,n=2,3,4,⋯, see Figure 12. There are three cases:
Case (1): When n≡0mod4 and n≡2mod4, we define the labeling
f:E[D2(Fn)]⟶{δ,2δ,3δ,⋯,(8n−4)δ} as follows:
f(v0vi)=δi,for1≤i≤n,f(v0ui)=δ(8n−4−i),for1≤i≤n,f(vivi+1)=δ(n+i),for1≤i≤n−1,f(uiui+1)=δ(3n−1−i),for1≤i≤n−1,f(viui+1)=δ(6n−3−i),for1≤i≤n−1,f(uivi+1)=δ(7n−4−i),for1≤i≤n−1,f(u0ui)=δ(4n−1−i),for1≤i≤n,f(u0v1)=δ(8n−4),f(u0vi)=δ(5n−1−i)for1≤i≤n. |
In view of the above labeling pattern, we can check that
(i) [f(v0vi)+f(v0ui)]mod[(8n−4)δ]=0,for1≤i≤n.
(ii) [f(u0un−i)+f(u0vi+2)]mod[(8n−4)δ]=0,for0≤i≤n2.
Then, the induced vertex labels are:
f∗(v0)=∑ni=1[f(v0vi)+f(v0ui)]mod[(8n−4)δ]=0,
f∗(u0)=∑ni=1[f(u0vi)+f(u0ui)]mod[(8n−4)δ]=[f(u0v1)+f(u0u1)]mod(8n−4)δ=(4n−2)δ,
f∗(v1)=(n−1)δ,f∗(vn)=(5n)δ,f∗(u1)=(4n−3)δ,f∗(un)=nδ,
f∗(vi)=[f(vivi+1)+f(vi−1vi)+f(viu0)+f(viv0)+f(viui+1)+f(ui−1vi)]mod[(8n−4)δ]
=[δ(8n−4i)]mod[(8n−4)δ],for2≤i≤n−1.
f∗(ui)=[f(uiui+1)+f(ui−1ui)+f(uiu0)+f(uiv0)+f(uivi+1)+f(vi−1ui)]mod(8n−4)δ
=[δ(3n−2i)]mod[(8n−4)δ],for2≤i≤n−1.
Hence the labels of the vertices v2,v3,v4,⋯,vn−2,vn−1 are δ(8n−8),δ(8n−12),δ(8n−16),⋯,δ(4n+8),δ(4n+4), respectively, and the labels of the vertices u2,u3,u4,⋯,un−2,un−1 are δ(3n−4),δ(3n−6),δ(3n−8),⋯,δ(n+4),δ(n+2), respectively, which are distinct numbers.
Case (2): When n≡1mod4, we define the labeling f as follows:
f(v0vi)=δ(n−1+i),for1≤i≤n,f(v0ui)=δ(7n−3−i),for1≤i≤n,f(uiui+1)=δ(4n−3−i),for1≤i≤n−1,f(u0vi)=δ(n−i),for1≤i≤n−1,f(u0vn)=δ(4n−3),f(u0ui)=δ(8n−4−i),for1≤i≤n−1,f(u0un)=δ(8n−4),f(viui+1)=δ(2n−1+i),for1≤i≤n−2,f(vn−1un)=δ(4n−2),f(u1v2)=δ(4n−1),f(uivi+1)=δ(6n−2−i),for2≤i≤n−1,f(vivi+1)={δ(4n+i),if i=1; δ(4n),if i=2; δ(4n−1+i),if 3≤i≤n−1. |
In view of the above labeling pattern, the induced vertex labels are:
f∗(v0)=0,f∗(v1)=4δ,f∗(v2)=8δ,
f∗(v3)=δ(2n+7),f∗(vn−1)=5nδ,f∗(vn)=δ,
f∗(u0)=(4n−3)δ,f∗(u1)=(7n−6)δ,f∗(un)=(5n−3)δ,
f∗(vi)=[δ(2n+2+2i)]mod[(8n−4)δ],for4≤i≤n−2.
Finally, f∗(ui)=[δ(7n−4−4i)]mod[(8n−4)δ],for2≤i≤n−1.
Hence the labels of the vertices v2,v3,v4,⋯,vn−2,vn−1 are δ(8n−8),δ(8n−12),δ(8n−16),⋯,δ(4n+8),δ(4n+4), respectively, and the labels of the vertices u2,u3,u4,⋯,un−2,un−1 are δ(7n−12),δ(7n−16),δ(7n−20),⋯,δ(3n+4),δ(3n), respectively, which are distinct numbers.
In this labeling, the induced labeling of the vertex u0 will equal the induced labeling of the vertex
ui when i=3n−14 i.e., when n=4k+3⇒n≡3mod4.
Case (3): When n≡3mod4, we define the labeling f as in the case n≡1mod4 but we change the labeling of two edges (u0vn) and (u1v2) as follows:
f(u0vn)=δ(4n−1) and f(u1v2)=δ(4n−3).
The induced vertex labels are:
f∗(v0)=0,f∗(v1)=4δ,f∗(v2)=6δ,
f∗(v3)=δ(2n+7),f∗(vn−1)=5nδ,f∗(vn)=3δ,
f∗(u0)=(4n−1)δ,f∗(u1)=(7n−8)δ,f∗(un)=(5n−3)δ,
f∗(vi)=[δ(2n+2+2i)]mod[(8n−4)δ],for4≤i≤n−2
f∗(ui)=[δ(7n−4−4i)]mod[(8n−4)δ],for2≤i≤n−1.
Hence the vertex labels are all distinct and a multiple of δ. Therefore the shadow graph D2(Fn) admits an edge δ− graceful labeling.
Illustration: The shadow graphs D2(F8) with edge 4− graceful labeling, D2(F9) with edge 3− graceful labeling and D2(F7) with an edge 5− graceful labeling. are presented in Figure 13.
Theorem 4.1. For any positive integer δ, the middle graph M(Pn) of path Pn is an edge δ− graceful graph when n is even and n≡1mod4.
Proof. Let {v1,v2,⋯,vn} be the vertices and {u1,u2,⋯,un−1} be the edges of path Pn. Then V[M(Pn)]=V(Pn)∪E(Pn) and E[M(Pn)]={viui;1≤i≤n−1,viui−1;2≤i≤n,uiui+1;1≤i≤n−2}. Here p=2n−1 vertices and q=3n−4 edges, k=max(p,q)=3n−4.
Case (1): When n is even, We define the labeling function f:E(M(Pn))⟶{δ,2δ,⋯,(3n−4)δ} as follows:
f(uiui+1)={δ(n−2i−2),if 1≤i≤n2−2; δ(n−1),if i=n2−1;δ(2n−2i−5),if n2≤i≤n−3;δ(n−2),if i=n−2. |
f(uivi)={δ(n−3),if i=1; δ(n+i−2),if 2≤i≤n−1. |
f(viui−1)=δ(2n−4+i),for2≤i≤n. |
Then the induced vertex labels are:
f∗(v1)=δ(n−3),f∗(vn)=0,f∗(u1)=(n−5)δ,f∗(un−1)=(3n−5)δ,
f∗(un−2)=(3n−6)δ,f∗(un2)=(3n−7)δ,andf∗(un2−1)=(2n−2)δ,
f∗(vi)=[f(viui−1)+f(viui)]mod[(3n−4)δ]=(2i−2)δ,for2≤i≤n−1;
f∗(ui)=[f(ui−iui)+f(uiui+1)+f(uivi)+f(uivi+1)]mod[(3n−4)δ]
=(2n−3−2i)δ,for2≤i≤n2−2, and
f∗(ui)=(4n−9−2i)δ,forn2+1≤i≤⋯,n−3.
Case (2): When n≡1mod4, We define the labeling function f:E(M(Pn))⟶{δ,2δ,⋯,(3n−4)δ} as follows:
f(viui)=δi,for1≤i≤n−1, |
f(uiui+1)=δ(n+i),for1≤i≤n−2, and |
f(viui−1)={δ(2n+i−3),if 2≤i≤n−1; δn,if i=n. |
Then the induced vertex labels are:
f∗(v1)=δ,f∗(vn)=nδ,f∗(u1)=5δ,f∗(un−1)=(n+1)δ,
f∗(vi)=[f(viui−1)+f(viui)]mod[(3n−4)δ]=(2n−3+2i)δmod[(3n−4)δ]2≤i≤n−1,
f∗(ui)=[f(ui−iui)+f(uiui+1)+f(uivi)+f(uivi+1)]mod[(3n−4)δ]
=[(n+1+4i)δ]mod[(3n−4)δ],for2≤i≤n−2.
Hence the labels of the vertices v2,v3,⋯,vn−2,vn−1 are (2n+1)δ,(2n+3)δ,⋯,(n−3)δ,(n−1)δ, respectively, and the labels of the vertices u2,u3,⋯,un−3,un−2 will be (n+9)δ,(n+13)δ,⋯,(2n−7)δ,(2n−3)δ, respectively. Hence the vertex labels are all distinct and a multiple of δ. Therefore M(Pn) admits an edge δ graceful labeling when n is odd.
Illustration: The middle graphs M(P14) with an edge 2− graceful labeling and M(P13) with an edge 3− graceful labeling are shown in Figure 14.
Theorem 4.2. For any positive integer δ, the middle graph M(Cn),n≥3 of the cycle Cn is an edge δ− graceful graph when n is odd number.
Proof. Let {v1,v2,⋯,vn} be the vertices of the cycle Cn and {u1,u2,⋯,un−1} be the edges of the cycle Cn. The V[M(Cn)]=V(Cn)∪E(Cn) and E[M(Cn)]={uiui+1,viui,uivi+1;1≤i≤n}, so the number of vertices p=|V[M(Cn]|=2n and edges q=|E[M(Cn)]|=3n,n=3,4,⋯.
When n is odd, we define the labeling function f:E(M(Cn))⟶{δ,2δ,⋯,(3n)δ} as follows:
f(uivi+1)=δ(3i−2),for1≤i≤n, |
f(uiui+1)=δ(3i−1),for1≤i≤n, |
f(v1u1)=3nδ,f(viui)=3δ(i−1),for2≤i≤n. |
In view of the above labeling pattern, the induced vertex labels are:
f∗(v1)=δ(3n−2),f∗(vi)=(6i−8)δmod[(3n)δ],2≤i≤n,
f∗(u1)=2δ,f∗(ui)=(12i−10)δmod[(3n)δ],2≤i≤n.
Hence the vertex labels are all distinct and a multiple of δ. Therefore M(Cn) admits an edge δ− graceful labeling.
Illustration: The middle graph M(C9) of the cycle C9 with an edge 6− graceful labeling is shown in Figure 15.
Theorem 4.3. For any positive integer δ, the middle graph of the crown graph Crn is an edge δ− graceful graph.
Proof. Let {v1,v2,⋯,vn} and {u1,u2,⋯,un−1} be the vertices of the crown graph Crn and v′1,v′2,⋯,v′n and u′1,u′2,⋯,u′n−1 be the edges of the crown graph Crn. Then V(M(Crn))=V(Crn)∪E(Crn) and E(M(Crn))={viu′i,v′ivi+1,viv′i,uiu′i,u′iv′i,v′iu′i+1,v′iv′i+1;1≤i≤n}, so p=4n vertices and q=7n edges, k=max(p,q)=7n, see Figure 16.
Case (1): When n is odd, we define the labeling function f:E(M(Crn))⟶{δ,2δ,⋯,7nδ} as follows:
f(viv′i)=δ(n+i),for1≤i≤n, |
f(v′ivi+1)=δ(3n−i+1),for1≤i≤n, |
f(v′iu′i+1)=δ(4n+i),for1≤i≤n, |
f(v′iu′i)=δ(3n+i),for1≤i≤n, |
f(v1u′1)=δ(7n),f(viu′i)=δi,for2≤i≤n, |
f(u1u′1)=δ,f(uiu′i)=δ(7n+1−i),for2≤i≤n, |
f(v′iv′i+1)={δ(6n−2i),if 1≤i≤n−12; δ(6n−1),if i=n+12; δ(7n−2i),if n+32≤i≤n−1;δ(6n),if i=n. |
Then the induced vertex labels are:
f∗(v′1)=(2n+1)δ,f∗(v′n+12)=(2n+2)δ,f∗(v′n+32)=(3n)δ,f∗(v′n)=(3n+3)δ,
f∗(v1)=(3n+2)δ,f∗(u1)=δ,f∗(u′1)=(n+2)δ,
f∗(vi)=(4n+i+2)δ,for2≤i≤n,
f∗(ui)=(7n−i+1)δ,for2≤i≤n,
f∗(u′i)=(2i)δ,for2≤i≤n,
f(v′i)={δ(2n−2i+3)mod[(7n)δ],if 2≤i≤n−12; δ(4n−2i+3)mod[(7n)δ],if n+52≤i≤n−1. |
Hence the labels of the vertices v′2,v′3,⋯,v′n−32,v′n−12 are (2n−1)δ,(2n−3)δ,⋯,(n+6)δ,(n+4)δ, respectively, and the labels of the vertices v′n+52,v′n+72,⋯,v′n−2,v′n−1 are (3n−2)δ,(3n−4)δ,⋯,(2n+7)δ,(2n+5)δ, respectively.
Case (2): When n≡0mod6, and n≡2mod6. We define the labeling function f:E(M(Crn))⟶{δ,2δ,⋯,7nδ} as follows:
f(viu′i)=δi,for1≤i≤n, |
f(v′ivi+1)=δ(3n−i+1),for1≤i≤n, |
f(viv′i)=δ(n+i),for1≤i≤n, |
f(uiu′i)={δ(3n+1),if i=1; δ(7n−i+1),if 2≤i≤n. |
f(u′iv′i)={δ(7n),if i=1; δ(3n+i),if 2≤i≤n. |
f(v′iu′i+1)={δ(4n+1+i),if 1≤i≤n−1; δ(4n+1),if i=n. |
f(v′iv′i+1)={δ(5n+2i−1),if 1≤i≤n2; δ(4n+2i),if n2+1≤i≤n. |
Then the induced vertex labels are:
f∗(v1)=(3n+3)δ,f∗(vi)=(4n+i+2)δ,for2≤i≤n,
f∗(u1)=(3n+1)δ,f∗(ui)=(7n−i+1)δ,for2≤i≤n,
f∗(u′1)=3δ,f∗(u′i)=(2i+1)δ,for2≤i≤n,
f∗(v′1)=(5n+4)δ,f∗(v′n2+1)=(2n+5)δ,f∗(v′n)=(3n)δ and
f(v′i)={δ(6i−2)mod[(7n)δ]if 2,≤i≤n2; δ(5n+6i)mod[(7n)δ]if n2+2≤i≤n−1. |
Hence the labels of the vertices v′2,v′3,⋯,v′n2−1,v′n2 are 10δ,16δ,⋯,(3n−8)δ,(3n−2)δ, respectively, and the labels of the vertices v′n2+2,v′n2+3,⋯,v′n−2,v′n−1 are (n+12)δ,(n+18)δ,⋯,(4n−12)δ,(4n−6)δ, respectively.
We notice that, these values are distinct for all n even except when n≡4mod6, since
v′n2+2=(n+12)δ=v′3=16δ,whenn=4
v′n2+2=(n+12)δ=v′4=22δ,whenn=10
v′n2+2=(n+12)δ=v′5=28δ,whenn=16
Case (3): When n≡4mod6. Define the labeling function f:E(M(Crn))⟶{δ,2δ,⋯,7nδ} as in the first case with changes in the following edges
f(v′iv′i+1)={δ(5n+2i),if 1≤i≤n2; δ(4n+2i−1),if n2+1≤i≤n. |
Then the induced vertex labels are:
f∗(v′1)=(5n+4)δ,f∗(v′n2+1)=(2n+5)δ,f∗(v′n)=(3n−2)δ and
f(v′i)={δ(6i)mod[(7n)δ]if 2≤i≤n2; δ(5n+6i−2)mod[(7n)δ]if n2+2≤i≤n−1. |
Hence the labels of the vertices v′2,v′3,⋯,v′n2−1,v′n2 are 12δ,18δ,⋯,(3n−6)δ,(3n)δ, respectively, and the labels of the vertices v′n2+2,v′n2+3,⋯,v′n−2,v′n−1 are (n+10)δ,(n+16)δ,⋯,(4n−14)δ,(4n−8)δ, respectively. Hence there are no repetition in the vertex labels which completes the proof.
Illustration: The middle graphs M(Cr10) with an edge 3− graceful labeling and M(Cr9) with an edge 2− graceful labeling are shown in Figure 17.
Theorem 5.1. For any positive integer δ, the total graph T(Pn) of the path Pn is an edge δ− graceful graph.
Proof. Let {v1,v1,⋯,vn} be the vertices of the path Pn, the total graph T(Pn) of path Pn has vertices set V(T(Pn))={vi,1≤i≤n}∪{ui,1≤i≤n−1} and edges set E(T(Pn))={vivi+1,viui,uivi+1,1≤i≤n−1}∪{uiui+1,1≤i≤n−2}, so the graph T(Pn) has p=2n−1 and q=4n−5, k=max(p,q)=4n−5.
If n=2 the graph T(P2) is an edge δ− graceful graph since it isomorphic to C3 [15]. There are two cases:
Case (1): When n is even, n≥4. We define the labeling function f:E(T(Pn))⟶{δ,2δ,⋯,(4n−5)δ} as follows:
f(viui)=δi,for1≤i≤n−1, $ |
f(vivi+1)=δ(n−1+i),for1≤i≤n−1, |
f(uivi+1)=δ(4n−5−i),for1≤i≤n−1, |
f(uiui+1)={δ(4n−5),if i=1; δ(2n−3+i),if 2≤i≤n−2. |
In view of the above labeling pattern then the induced vertex labels are:
f∗(v1)=(n+1)δ,f∗(vn)=(n−1)δ,f∗(u1)=0,f∗(u2)=(2n−1)δ,f∗(un−1)=(3n−5)δ,
f∗(vi)=[(2n−2+2i)δ]mod[(4n−5)δ],for2≤i≤n−1,
f∗(ui)=(2i−2)δ,for3≤i≤n−2.
Hence the labels of the vertices are all distinct numbers.
Case (2): When n is odd:
∙ If n=3 the graph T(P3) is an edge δ− graceful graph for any positive integer δ define the labeling function f:E(T(P3))⟶{δ,2δ,⋯,7δ} as shown in Figure 18.
∙ If n≥5. Define the labeling function f:E(T(Pn))⟶{δ,2δ,⋯,(4n−5)δ} as follows:
f(viui)=δi,for1≤i≤n−1, |
f(uiui+1)=δ(2n−2+i),for1≤i≤n−2, |
f(uivi+1)=δ(2n−1−i),for1≤i≤n−1, and |
f(vivi+1)={δ(4n−5),if i=1;δ(3n−4+i),if 2≤i≤n−2; δ(3n−3),if i=n−1. |
In view of the above labeling pattern then the induced vertex labels are:
f∗(v1)=δ,f∗(v2)=(n+3)δ,f∗(vn−1)=(n+1)δ,
f∗(vn)=2δ,f∗(u1)=3δ,f∗(un−1)=nδ,
f∗(vi)=[(1+2i)δ]mod[(4n−5)δ],for3≤i≤n−2, and
f∗(ui)=[(2n−1+2i)δ]mod[(4n−5)δ],for2≤i≤n−2.
Hence the labels of the vertices v3,v4,⋯,vn−3,vn−2 will be 7δ,9δ,⋯,(2n−5)δ,(2n−3)δ, respectively, and the labels of the vertices u2,u3,⋯,un−3,un−2 will be (2n+3)δ,(2n+5)δ,⋯,(4n−7)δ,0, respectively.
In this case we have f∗(vn−12) will equal f∗(un−1), so we change the labeling of two edges (vn−52vn−32) and (vn−32vn−12) as follows f(vn−52vn−32)=δ2(7n−11). and f(vn−32vn−12)=δ2(7n−13).
Then f∗(vn−52)=(n−3)δ and f∗vn−12=(n−1)δ Hence the vertex labels are all distinct and a multiple of δ. Therefore T(Pn) admits an edge δ− graceful labeling.
Illustration: The total graphs T(P10) and T(P11) with an edge 6− graceful labeling are shown in Figure 19.
Theorem 5.2. For any positive integer δ, the total graph T(Cn),n≥3 of the cycle Cn is an edge δ− graceful graph.
Proof. Let {vi,i=1,2,⋯,n} be the vertices of the path Cn, the total graph T(Cn) of path Cn has vertices set V(T(Cn))={vi,ui,i=1,2,⋯,n} and E(T(Cn))={vivi+1,viui,uivi+1,uiui+1,i=1,2,⋯,n}, so the graph T(Cn) has p=2n and q=4n, k=max(p,q)=4n. There are two cases:
Case (1): When n is even, we define the labeling f:E(T(Cn)⟶{δ,2δ,3δ,⋯,4nδ} as follows:
f(vivi+1)=δ(n+i),for1≤i≤n,f(uivi)=δ(4n−i),for1≤i≤n,f(u1un)=δ4n,f(uiui+1)=δi,for1≤i≤n−1,f(v1un)=δn,f(uivi+1)=δ(2n+i),for1≤i≤n−1, |
In view of the above labeling pattern we have:
f∗(v1)=0,f∗(u1)=(2n+1)δ,f∗(un)=(n−1)δ,
f∗(vi)=2δ(i−1),for2≤i≤n,
f∗(ui)=δ(2i+2n−1),for2≤i≤n−1.
Hence the labels of the vertices v2,v3,v4,⋯,vn−1,vn are 2δ,4δ,6δ,⋯,2δ(n−2),2δ(n−1), respectively, and the labels of the vertices u2,u3,u4,⋯,un−2,un−1 are δ(2n+3),δ(2n+5),δ(2n+7),⋯,δ(4n−5),δ(4n−3), respectively.
It is easy to see that: f∗(un)=f∗(vi) when i=n+12, but n is even number, so all the labels are distinct numbers.
Case (2): When n is odd, we define the labeling f as in the above case where n is even but we change the labeling of two edges (unv1) and (vnv1) as follows f(unv1)=2nδ and f(vnv1)=nδ.
The induced vertex labels are:
f∗(v1)=[f(v1v2)+f(vnv1)+f(u1v1)+f(unv1)]mod(4n)δ=0,
f∗(vn)=[f(vnv1)+f(vn−1vn)+f(unvn)+f(un−1vn)]mod(4n)δ=δ(n−2),
f∗(un)=[f(unu1)+f(un−1un)+f(unv1)+f(unvn)]mod(4n)δ=(2n−1)δ.
It is easy to see that: f∗(vn)=f∗(vi) when i=n2, but n is odd number, so all the labels are distinct numbers.
Illustration: The total graph T(C10) of the cycle C10 with an edge 5− graceful labeling and the total graph T(C9) of the cycle C9 with an edge 6− graceful labeling are shown in Figure 20.
Theorem 5.3. For any positive integer δ, the total graph of the crown graph Crn is an edge δ− graceful graph.
Proof. Let {v1,v2,⋯,vn} and {u1,u2,⋯,un} be the vertices of the crown graph Crn and {v′1,v′2,⋯,v′n} and {u′1,u′2,⋯,u′n} be the edges of the crown graph Crn. Then V(T(Crn))=V(Crn)∪E(Crn) and E(T(Crn))={viu′i,v′ivi+1,viv′i,uiu′i,u′iv′i,v′iu′i+1,v′iv′i+1,viui,vivi+1;1≤i≤n}, see Figure 21.
Here p=4n vertices and q=9n edges, k=max(p,q)=9n.
Case (1): When n is odd. Define the labeling function f:E(T(Crn))⟶{δ,2δ,⋯,9nδ} as follows:
f(viu′i)={δ(n+1),if i=1; δi,if 2≤i≤n. |
f(uiu′i)=δ(2n+2−i),for1≤i≤n, |
f(v′iu′i)={δ(3n+1),if i=1; δ(2n+i),if 2≤i≤n. |
f(viv′i)={δ,if i=1; δ(3n+i),if 2≤i≤n. |
f(v′ivi+1)=δ(4n+i),for1≤i≤n, |
f(vivi+1)=δ(5n+i),for1≤i≤n, |
f(v′iv′i+1)={δ(7n−i),if 1≤i≤n−1; δ(7n),if i=n. |
f(v′iu′i+1)=δ(7n+i),for1≤i≤n, |
f(viui)=δ(9n+1−i),for1≤i≤n. |
Then the induced vertex labels are:
f∗(v1)=(8n+3)δ,f∗(vi)=(8n+4i−1)δmod[(9n)δ],for2≤i≤n,
f∗(u′1)=(5n+3)δ,f∗(u′i)=(2n+2i+1)δ,for2≤i≤n,
f∗(ui)=(2n+3−2i)δ,for1≤i≤n,
f∗(v′1)=(n+3)δ,f∗(v′n)=(6n+1)δ,f(v′i)=(3n+1+2i)δ,for2≤i≤n−1.
Case (2): When n≡2mod4. The labeling function f:E(T(Crn))⟶{δ,2δ,⋯,9nδ} defined as follows:
f(uiu′i)=δ(i),for1≤i≤n, |
f(u′ivi)=δ(n+i),for1≤i≤n, |
f(v′iu′i)=δ(2n+i),for1≤i≤n, |
f(vivi+1)=δ(3n+i),for1≤i≤n, |
f(v′ivi+1)=δ(5n+1−i),for1≤i≤n, |
f(v′iv′i+1)=δ(5n+i+1),for1≤i≤n−1,f(v′nv′1)=δ(5n+1), |
f(viv′i)=δ(6n+i),for1≤i≤n, |
f(v′iu′i+1)=δ(7n+i),for1≤i≤n, |
f(viui)=δ(8n+i),for1≤i≤n. |
Then the induced vertex labels are:
f∗(ui)=(8n+2i)δmod[(9n)δ],for1≤i≤n,
f∗(u′1)=(2n+3)δ,f∗(u′i)=(n+4i−1)δ,for2≤i≤n,
f∗(vi)=(8n+4i+1)δmod[(9n)δ],for1≤i≤n,
f∗(v′1)=(3n+6)δ,f∗(v′n)=(6n+2)δ,f(v′i)=(3n+2+4i)δ,for2≤i≤n−1.
These values are distinct for all n≡2mod4, but
f∗(v′i)=(3n+4i+2)δ=f∗(v′n)=(6n+3)δ, when i=3n4 i.e., when n≡0mod4.
Case (3): When n≡0mod4 define the labeling function f as follows:
f(uiu′i)=δ(i),for1≤i≤n, |
f(u′ivi)=δ(n+i),for1≤i≤n, |
f(vivi+1)={δ(3n+i),if 1≤i≤n−1; δ(2n+1),if i=n. |
f(v′iu′i)={δ(4n),if i=1;δ(2n+i),if 2≤i≤n; |
f(v′ivi+1)=δ(5n+1−i),for1≤i≤n, |
f(v′iv′i+1)=δ(5n+i),for1≤i≤n, |
f(viv′i)=δ(6n+i),for1≤i≤n, |
f(v′iu′i+1)=δ(7n+i),for1≤i≤n, |
f(viui)=δ(8n+i),for1≤i≤n. |
Then the induced vertex labels are:
f∗(ui)=(8n+2i)δmod[(9n)δ],for1≤i≤n,
f∗(u′1)=(4n+2)δ,f∗(u′i)=(n+4i−1)δ,for2≤i≤n,
f∗(v′1)=(6n+3)δ,f(v′i)=(3n+4i)δ,for2≤i≤n,
f∗(v1)=(6n+6)δ,f∗(vn)=(n+2)δ,
f∗(vi)=(8n+4i+1)δmod[(9n)δ],for2≤i≤n−1.
We notice that, these values are distinct for all n≡0mod4. It should be notice that:
f∗(v′i)=(3n+4i)δ=f∗(u′1)=(4n+2)δ, when i=n+24 i.e., when n≡2mod4. Hence there are no repetition in the vertex labels which completes the proof.
Illustration: The total graphs T(Cr8) and T(Cr9) of the crown graph with an edge 3− graceful labeling are shown in Figure 22.
Definition 6.1. The twig graph TWn,n≥4 is the graph obtained from path Pn={v1,v2,⋯,vn} by attaching exactly two pendant edges to each internal vertex of the path Pn.
Theorem 6.1. For any positive integer δ, the twig graph TWn is an edge δ− graceful graph when n is odd.
Proof. Let {v1,v2,⋯,vn} be the vertices of the path Pn and the new attaching vertices are {u2,u3,⋯,un−1} and {w2,w3,⋯,wn−1}. The edges of TWn are denoted by {ai,bi,ci,di}, where ai={v(n−4i+32)v(n−4i+52)}, bi={v(n+4i−32)v(n+4i−12)}, di={v(n−4i+12)v(n−4i+32)} and ci={v(n+4i−12)v(n+4i+12)} where,
i={1,2,⋯,n−14if i≡1mod4;1,2,⋯,n+14if i≡3mod4. |
The graph TWn has p=3n−4 vertices and q=3n−5 edges, k=max(p,q)=3n−4. see, Figure 23 We define the labeling function f:E(TWn)⟶{δ,2δ,⋯,(3n−4)δ} as follows:
At the beginning, we determine the middle vertex vn+12 in the path Pn and we start the labeling from this vertex by using the following algorithm.
First step: Label the edges a1,b1,c1 and d1 in the following order:
f(a1)=δ, f(b1)=δ(3n−5), f(c1)=2δ and
f(d1)=[f(b1)−f(a1)]mod[δ(3n−4)]=δ(3n−6).
Second step: Label the vertices ai,bi,ci and di by the following algorithm respectively
(i) f(ai)=[f(ci−1)−f(di−1)]mod[δ(3n−4)], for i=2,3,⋯,n−34,
(ii) f(bi)=(3n−4)δ−f(ai),
(iii) f(ci)=[f(ai)−f(bi)]mod[δ(3n−4)], for i=2,3,⋯,n−74,
(iv) f(di)=[f(bi)−f(ai)]mod[δ(3n−4)]. for i=2,3,⋯,n−74,
and repeats the second step until we fish the labeling of all edges in the path Pn.
At the end, the edges (viui) and (viwi),2≤i≤n−1 take any number from the reminder set of the labeling such that [f(viui)+f(viwi)]mod[δ(3n−4)]≡0mod[δ(3n−4)]fori=2≤i≤n−1.
In view of the above labeling algorithm, the labels of the vertices are,
f∗(vn+12)=[f(a1)+f(b1)+f(un+12)+f(wn+12)]mod[δ(3n−4)]=0,
f∗(vn−12)=f(b1)=δ(3n−5),f∗(vn+32)=f(a1)=δ,
f∗(vn−32)=f(c1)=2δ,f∗(vn+52)=f(d1)=δ(3n−6),
f∗(vn−52)=f(b2)=δ(3n−8),f∗(vn+72)=f(a2)=4δ,
f∗(vn+(4i−1)2)=f(ai),f∗(vn−(4i−3)2)=f(bi), for i=1,2,⋯,n−14,
f∗(vn+(4i+1)2)=f(di),f∗(vn−(4i−1)2)=f(ci), for i=1,2,⋯,n−54.
The pendant vertices v1andvn of the path Pn will take the labels of its pendant edges, i.e.,
∙ If n≡1(mod4), then f∗(v1)=f(dn−14), and f∗(v2n−1)=f(cn−14).
∙ If n≡3(mod4), then f∗(v1)=f(an+14), and f∗(vn)=f(bn+14).
It is clear that the labels of the vertices of the path Pn take the labels of the edges of the path and each pendant vertex takes the labels of its incident edge. Then there are no repeated vertex labels, which completes the proof.
Illustration: The twig graph TW13 is presented in Figure 24 with an edge 4− graceful labeling.
Definition 7.1. A snail graph Sn is obtained from path Pn={v1,v2,⋯,vn} by attaching two parallel edges between vi and vn−i+1 for i=1,2,⋯,⌊n2⌋.
Theorem 7.1. For any positive integer δ, the snail graph Sn, n>2 is an edge δ− graceful graph.
Proof.
Case (1): When n is even, the vertices of the graph Sn are {v0,v1,v2,⋯,vn} and the edges are {e1,e1,⋯,en−1,a1,a2⋯,an2,b1,b2⋯,bn2} where {ei=vivi+1,i=1,2,⋯,n−1} and {aj=bj=vivn−i+1,j=1,2,⋯,n2}, see Figure 25, in this case p=n,q=2n−1 and k=max(p,q)=2n−1.
We define the labeling function f:E(Sn))⟶{δ,2δ,⋯,(2n−1)δ} as follows:
f(ai)=δi,for1≤i≤n2,f(bi)=δ(2n−i−1),for1≤i≤n2,f(ei)={δ(i+n2),if 1≤i≤n2−1; δ(2n−1),if i=n2; δ(n2+i−1),if n2+1≤i≤n−1. |
The induced vertex labels are:
f∗(v1)=[f(a1)+f(b1)+f(e1)]mod[(2n−1)δ]=δ[n2+1],
f∗(vn)=[f(a1)+f(b1)+f(en−1)]mod[(2n−1)δ]=δ[3n2−2],
f∗(vn2)=δ(n−1),f∗(vn2+1)=δn.
f∗(vi)=[f(ai)+f(bi)+f(ei)+f(ei−1)][mod(2n−1)δ]
={δ(n+2i−1)mod[(2n−1)δ]if 2≤i≤n2−1;δ(n+2i−3)mod[(2n−1)δ]if n2+2≤i≤n−1.
Hence the labels of the vertices v2,v3,⋯,vn2−1 are δ(n+3),δ(n+5),⋯,δ(2n−3), respectively, and the labels of the vertices vn2+2,vn2+3,⋯,vn−1 are 2δ,4δ,⋯,δ(n−4), respectively.
Note that S4 is an edge δ− graceful graph but not follow this rule, see Figure 26.
Case (2): When n is odd. Let {e1,e1,⋯,en−1,a1,a2,⋯,an−12,b1,b2⋯,bn−12} be the edges of Sn which are denoted as in the Figure 27, in this case p=n,q=2n−2,k=max(p,q)=2n−2.
Define the labeling function f:E(Sn))⟶{δ,2δ,⋯,(2n−2)δ} as follows:
f(ei)=iδ,for1≤i≤n−1,f(ai)=δ(n+i−1),for1≤i≤n−12,f(bi)=δ(2n−i−1),for1≤i≤n−12. |
Therefor, the induced vertex labels are
f∗(v1)=δ(n+1),f∗(vn+12)=nδ,f∗(vn)=δ and
f∗(vi)=[f(ai)+f(bi)+f(ei)+f(ei−1)]mod[(2n−2)δ]
=δ(n+2i−1)mod[(2n−2)δ],ifi=2,3,⋯,n−12,n+22,⋯,n−1.
Hence the labels of the vertices v2,v3,⋯,vn−32,vn−12 are δ(n+3),δ(n+5),⋯,δ(2n−4),0, respectively, and the labels of the vertices vn+32,vn+52,⋯,vn−2,vn−1 are 4δ,6δ,⋯,(n−3)δ,(n−1)δ, respectively. Overall all vertex labels are distinct and a multiple of δ numbers. Hence, the snail graph Sn is an edge δ− graceful for all n.
Illustration: In Figure 28, we present a triple graceful labeling of the graph S12 and an edge 4 graceful labeling of S13.
Recently, edge graceful labeling of graphs has been studied too much and these objects continue to be an attraction in the field of graph theory and discrete mathematics. A senior number of published papers and results exist. So far, numerous graphs are unknown if it is edge graceful or not.
In this work, we pushed the new type of labeling (edge δ− graceful labeling), by finding more graphs that have edge δ− graceful labeling. We prove the existence of an edge δ− graceful labeling, for any positive integer δ, for the following graphs. The splitting graphs of the cycle, fan, and crown graphs. The shadow graphs of the path, cycle, and fan graphs. The middle graphs and the total graphs of the path, cycle, and crown graphs. Finally, we display the existence of an edge δ− graceful labeling, for the twig and snail graphs.
The authors declare that they have no competing interests.
[1] |
F. Busetti, A. M. R. Taylor, Tests of stationarity against a change in persistence, J. Econom., 123 (2004), 33–66. https://doi.org/10.1016/j.jeconom.2003.10.028 doi: 10.1016/j.jeconom.2003.10.028
![]() |
[2] |
M. Caporin, R. Gupta, Time-varying persistence in US inflation, Empir. Econ., 53 (2017), 423–439. https://doi.org/10.1007/s00181-016-1144-y doi: 10.1007/s00181-016-1144-y
![]() |
[3] |
Z. S. Chen, Z. Jin, Z. Tian, P. Y. Qi, Bootstrap testing multiple changes in persistence for a heavy-tailed sequence, Comput. Stat. Data Anal., 56 (2012), 2303–2316. https://doi.org/10.1016/j.csda.2012.01.011 doi: 10.1016/j.csda.2012.01.011
![]() |
[4] |
Z. Chen, Z. Tian, Y. Xing, Sieve bootstrap monitoring persistence change in long memory process, Stat. Interface, 9 (2016), 37–45. https://doi.org/10.4310/SII.2016.v9.n1.a4 doi: 10.4310/SII.2016.v9.n1.a4
![]() |
[5] |
Z. Chen, Y. Xing, Z. A. Chen, Y. H. Xing, F. X. Li, Sieve bootstrap monitoring for change from short to long memory, Econ. Lett., 140 (2016), 53–56. https://doi.org/10.1016/j.econlet.2015.12.023 doi: 10.1016/j.econlet.2015.12.023
![]() |
[6] |
Z. S. Chen, F. X. Li, L. Zhu, Y. H. Xing, Monitoring mean and variance change-points in long-memory time series, J. Syst. Sci. Complex., 2021. https://doi.org/10.1007/s11424-021-0222-1 doi: 10.1007/s11424-021-0222-1
![]() |
[7] |
Z. S. Chen, Y. T. Xiao, F. X. Li, Monitoring memory parameter change-points in long-memory time series, Empir. Econ., 60 (2021), 2365–2389. https://doi.org/10.1007/s00181-020-01840-4 doi: 10.1007/s00181-020-01840-4
![]() |
[8] |
H. Dette, J. Gösmann, A likelihood ratio approach to sequential change point detection for a general class of parameters, J. Am. Stat. Assoc., 115 (2019), 1361–1377. https://doi.org/10.1080/01621459.2019.1630562 doi: 10.1080/01621459.2019.1630562
![]() |
[9] |
J. Gösmann, T. Kley, H. Dette, A new approach for open-end sequential change point monitoring, J. Time Ser. Anal., 42 (2021), 63–84. https://doi.org/10.1111/jtsa.12555 doi: 10.1111/jtsa.12555
![]() |
[10] |
D. I. Harvey, S. J. Leybourne, A. M. R. Taylor, Modified tests for a change in persistence, J. Econom., 134 (2006), 441–469. https://doi.org/10.1016/j.jeconom.2005.07.002 doi: 10.1016/j.jeconom.2005.07.002
![]() |
[11] |
U. Hassler, J. Scheithauer, Detecting changes from short to long memory, Stat. Papers, 52 (2011), 847–870. https://doi.org/10.1007/s00362-009-0292-y doi: 10.1007/s00362-009-0292-y
![]() |
[12] |
U. Hassler, B. Meller, Detecting multiple breaks in long memory the case of U.S. inflation, Empir. Econ., 46 (2014), 653–680. https://doi.org/10.1007/s00181-013-0691-8 doi: 10.1007/s00181-013-0691-8
![]() |
[13] |
F. Iacone, Š. Lazarová, Semiparametric detection of changes in long range dependence, J. Time Ser. Anal., 40 (2019), 693–706. https://doi.org/10.1111/jtsa.12448 doi: 10.1111/jtsa.12448
![]() |
[14] |
M. Kejriwal, P. Perron, J. Zhou, Wald tests for detecting multiple structural changes in persistence, Economet. Theor., 29 (2013), 289–323. https://doi.org/10.1017/S0266466612000357 doi: 10.1017/S0266466612000357
![]() |
[15] |
J. Y. Kim, Detection of change in persistence of a linear time series, J. Econ., 95 (2000), 97–116. https://doi.org/10.1016/S0304-4076(99)00031-7 doi: 10.1016/S0304-4076(99)00031-7
![]() |
[16] |
C. Kirch, S. Weber, Modified sequential change point procedures based on estimating functions, Electron. J. Stat., 12 (2018), 1579–1613. https://doi.org/10.1214/18-EJS1431 doi: 10.1214/18-EJS1431
![]() |
[17] |
F. Lavancier, R. Leipus, A. Philippe, D. Surgailis, Detection of nonconstant long memory parameter, Economet. Theor., 29 (2013), 1009–1056. https://doi.org/10.1017/S0266466613000303 doi: 10.1017/S0266466613000303
![]() |
[18] |
S. Leybourne, R. Taylor, T. H. Kim, CUSUM of squares-based tests for a change in persistence, J. Time Ser. Anal., 28 (2007), 408–433. https://doi.org/10.1111/j.1467-9892.2006.00517.x doi: 10.1111/j.1467-9892.2006.00517.x
![]() |
[19] |
F. X. Li, Z. S. Chen, Y. T. Xiao, Sequential change-point detection in a multinomial logistic regression model, Open Math., 18 (2020), 807–819. https://doi.org/10.1515/math-2020-0037 doi: 10.1515/math-2020-0037
![]() |
[20] |
R. B. Qin, Y. Liu, Block bootstrap testing for changes in persistence with heavy-tailed innovations, Commun. Stat.-Theor. M., 47 (2018), 1104–1116. https://doi.org/10.1080/03610926.2017.1316398 doi: 10.1080/03610926.2017.1316398
![]() |
[21] |
P. Sibbertsen, R. Kruse, Testing for a break in persistence under long-range dependences, J. Time Ser. Anal., 30 (2009), 263–285. https://doi.org/10.1111/j.1467-9892.2009.00611.x doi: 10.1111/j.1467-9892.2009.00611.x
![]() |
[22] |
M. S. Taqqu, Weak convergence to fractional Brownian motion and to the Rosenblatt process, Z. Wahrscheinlichkeitstheorie verw. Gebiete, 31 (1975), 287–302. https://doi.org/10.1007/BF00532868 doi: 10.1007/BF00532868
![]() |
[23] |
W. Z. Zhao, Y. X. Xue, X. Liu, Monitoring parameter change in linear regression model based on the efficient score vector, Physica A, 527 (2019), 121135. https://doi.org/10.1016/j.physa.2019.121135 doi: 10.1016/j.physa.2019.121135
![]() |
1. | Saima Rashid, Fahd Jarad, Sobhy A. A. El-Marouf, Sayed K. Elagan, Global dynamics of deterministic-stochastic dengue infection model including multi specific receptors via crossover effects, 2023, 8, 2473-6988, 6466, 10.3934/math.2023327 | |
2. | Saima Rashid, Shazia Karim, Ali Akgül, Abdul Bariq, S. K. Elagan, Novel insights for a nonlinear deterministic-stochastic class of fractional-order Lassa fever model with varying kernels, 2023, 13, 2045-2322, 10.1038/s41598-023-42106-0 | |
3. | Yu-Ming Chu, Saima Rashid, Shazia Karim, Aasma Khalid, S. K. Elagan, Deterministic-stochastic analysis of fractional differential equations malnutrition model with random perturbations and crossover effects, 2023, 13, 2045-2322, 10.1038/s41598-023-41861-4 | |
4. | V. Suganya, S. Padmasekaran, S. Dickson, Stability analysis of a dynamic cancer mathematical model with the impact of smoking and alcohol consumption, 2024, 30, 1405-213X, 10.1007/s40590-024-00599-3 | |
5. | S. Fathima Nasrin, C. Rajivganthi, Dynamical Analysis of a Stochastic Ebola Model with Nonlinear Incidence Functions, 2025, 35, 0938-8974, 10.1007/s00332-025-10127-w |