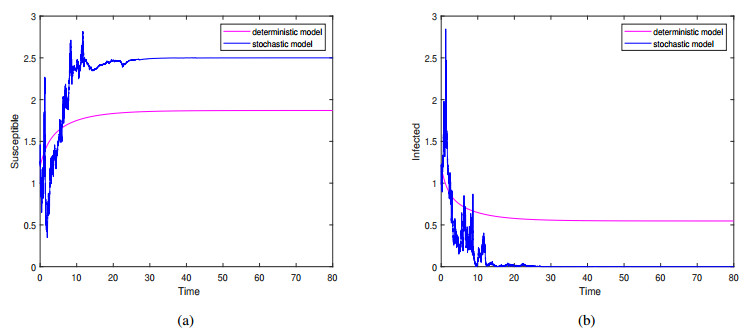
In this paper, we investigated a stochastic SIRS epidemic infectious disease model that accounted for environmentally driven infection and incorporated multiparameter perturbations. In addition to establishing the existence and uniqueness of the global positive solution of the model, we derived the threshold conditions for the extinction and persistence of the disease using the comparison theorem and Itˆo's formula of stochastic differential equations. Subsequently, we obtained the asymptotic stability of both the disease-free equilibrium and the endemic equilibrium of the deterministic model corresponding to the stochastic model through stochastic stability theory. The results indicated that high-intensity noise perturbation can inhibit the spread of the disease, and the dynamic behavior of the disease transitioned from persistence to extinction as noise intensity increased. Our study also demonstrated that, compared to perturbations in the indirect infection rate, changes in noise intensity that affect the direct infection rate will have a more significant impact on disease transmission.
Citation: Zhengwen Yin, Yuanshun Tan. Threshold dynamics of stochastic SIRSW infectious disease model with multiparameter perturbation[J]. AIMS Mathematics, 2024, 9(12): 33467-33492. doi: 10.3934/math.20241597
[1] | Shah Hussain, Naveed Iqbal, Elissa Nadia Madi, Thoraya N. Alharthi, Ilyas Khan . Vaccination strategies in a stochastic SIVR epidemic model. AIMS Mathematics, 2025, 10(2): 4441-4456. doi: 10.3934/math.2025204 |
[2] | Asad Khan, Anwarud Din . Stochastic analysis for measles transmission with Lévy noise: a case study. AIMS Mathematics, 2023, 8(8): 18696-18716. doi: 10.3934/math.2023952 |
[3] | Xiangming Zhao, Jianping Shi . Dynamic behavior of a stochastic SIR model with nonlinear incidence and recovery rates. AIMS Mathematics, 2023, 8(10): 25037-25059. doi: 10.3934/math.20231278 |
[4] | Yanfang Zhang, Fuchang Wang, Yibin Zhao . Statistical characteristics of earthquake magnitude based on the composite model. AIMS Mathematics, 2024, 9(1): 607-624. doi: 10.3934/math.2024032 |
[5] | Yue Wu, Shenglong Chen, Ge Zhang, Zhiming Li . Dynamic analysis of a stochastic vector-borne model with direct transmission and media coverage. AIMS Mathematics, 2024, 9(4): 9128-9151. doi: 10.3934/math.2024444 |
[6] | Roshan Ara, Saeed Ahmad, Zareen A. Khan, Mostafa Zahri . Threshold dynamics of stochastic cholera epidemic model with direct transmission. AIMS Mathematics, 2023, 8(11): 26863-26881. doi: 10.3934/math.20231375 |
[7] | Ishtiaq Ali, Sami Ullah Khan . Dynamics and simulations of stochastic COVID-19 epidemic model using Legendre spectral collocation method. AIMS Mathematics, 2023, 8(2): 4220-4236. doi: 10.3934/math.2023210 |
[8] | Mireia Besalú, Giulia Binotto . Time-dependent non-homogeneous stochastic epidemic model of SIR type. AIMS Mathematics, 2023, 8(10): 23218-23246. doi: 10.3934/math.20231181 |
[9] | Butsayapat Chaihao, Sujin Khomrutai . Extinction and permanence of a general non-autonomous discrete-time SIRS epidemic model. AIMS Mathematics, 2023, 8(4): 9624-9646. doi: 10.3934/math.2023486 |
[10] | Yubo Liu, Daipeng Kuang, Jianli Li . Threshold behaviour of a triple-delay SIQR stochastic epidemic model with Lévy noise perturbation. AIMS Mathematics, 2022, 7(9): 16498-16518. doi: 10.3934/math.2022903 |
In this paper, we investigated a stochastic SIRS epidemic infectious disease model that accounted for environmentally driven infection and incorporated multiparameter perturbations. In addition to establishing the existence and uniqueness of the global positive solution of the model, we derived the threshold conditions for the extinction and persistence of the disease using the comparison theorem and Itˆo's formula of stochastic differential equations. Subsequently, we obtained the asymptotic stability of both the disease-free equilibrium and the endemic equilibrium of the deterministic model corresponding to the stochastic model through stochastic stability theory. The results indicated that high-intensity noise perturbation can inhibit the spread of the disease, and the dynamic behavior of the disease transitioned from persistence to extinction as noise intensity increased. Our study also demonstrated that, compared to perturbations in the indirect infection rate, changes in noise intensity that affect the direct infection rate will have a more significant impact on disease transmission.
Epidemic dynamics is a crucial method for the quantitative study of infectious diseases, developing mathematical models that represent the process of disease progression and the principles of transmission. Mathematical research has achieved significant advances in both theory and application, leading to several well-known models of infectious disease dynamics [1,2,3,4].
Numerous researchers have explored mathematical models concerning population-level transmission dynamics, such as SIR model that susceptible-infected-recovered-infectious disease model and SIRS model is based on the SIR model with the addition of the process of loss of immunity, among others[1]. These models have been studied and analyzed from various perspectives, including incidence, treatment functions, and age structure. Many reliable conclusions have emerged, contributing to the advancement of infectious disease dynamics [3,4,5,6]. For example, Li et al. [5] investigated epidemic models of the SIR and SIRS types with a general contact rate and constant immigration, particularly emphasizing the impact of the influx of infectious individuals. The model complexity and greater stochastic volatility of multiparameter stochastic perturbations have resulted in relatively little relevant research. The purpose of this study is to investigate the extinction and persistence of stochastic SIRSW model solutions under multiparameter stochastic perturbations, the propagation laws of infectious disease dynamics, and the effects of different parameters on disease spread. Among these, the SIRSW model is based on the SIRS model, adding the environmental pathogen infection process.
In recent years, due to environmental degradation and varying degrees of contamination of water and food, people have faced numerous infectious diseases stemming from environmental issues[7]. Infectious diseases such as cholera, tuberculosis, and COVID-19 exhibit spatial-temporal and multi-scale characteristics, including direct transmission between humans at the macroscale population level and indirect transmission between humans and environmental pathogens at the microscale [8,9,10,11]. In the environmental transmission of certain infectious diseases, the excretion of pathogens and their transmission are two major processes. Additionally, between-host disease transmission and within-host viral load are interdependent[12]. To investigate the effects of individual movement and pathogen dispersal in space on disease transmission, Xiao et al.[13] developed a coupled model that considers direct infection resulting from random human movements and indirect infection following pathogen shedding in the environment, which took the following form
{dS(t)dt=−βSI−vSW,dI(t)dt=βSI+νSW−γI,dR(t)dt=γI,dW(t)dt=ηI−(μ+νN)W(t), | (1.1) |
where N denotes the total population, S,I,R are the number of susceptible, infected, and recovered individuals, W(t) is the pathogen concentration in the environment at time t, respectively, β is the direct transmission rate, ν is the indirect transmission rate, η is the environmental virus shedding rate, μ is the natural mortality rate, and γ is the rate of recovery of infected individuals. Xiao et al. performed a numerical simulation analysis from a data-driven perspective and reached many interesting conclusions, but no theoretical analysis was performed. In the following section, refining the theoretical analyses of the corresponding models is also an important research objective of this paper.
Deterministic modeling of viral infections has been studied by some researchers[14,15,16]. Edoardo[14] proposes a mathematical model of the marine bacteriophage infection and analyzes its basic mathematical features. Ivo et al.[15] extended the Beretta-Kuang model to allow the estimation of virus growth parameters under model-specific parameterizations. Depending on the characteristics of the infection, the recovering person loses immunity after a period of time and becomes susceptible to infection again, which is called SIRS infection[17]. On this basis, by considering birth and death rates, the following deterministic model is obtained:
{dS=(Λ−βSI−νSW−μS+ζR)dt,dI=(βSI+νSW−γI−μI)dt,dR=(γI−(μ+ζ)R)dt,dW=(ηI−cW)dt, | (1.2) |
where Λ is the input rate of susceptible individuals, ζ denotes the rate of immune loss, and c denotes the rate of viral failure. The environmental pathogen concentration level is denoted by W. Denote
Rn+={x∈Rn|xi>0,1≤i≤n}. |
By calculation, model (1.2) has a disease-free equilibrium
E0=(S0,I0,R0,W0)=(Λμ,0,0,0) |
and exists an endemic equilibrium
E∗=(S∗,I∗,R∗,W∗)=(c(μ+γ)(βc+νη),Λ(μ+ζ)μ(μ+ζ+γ)(1−1R0),Λγμ(μ+ζ+γ)(1−1R0),Λη(μ+ζ)μc(μ+ζ+γ)(1−1R0)). |
The basic reproduction number
R0=Λ(βc+νη)μc(μ+γ) |
is obtained by using the next generation matrix method. Further, if R0<1, E0 is globally asymptotically stable in D (in Remark 1). If R0>1, E∗ is globally asymptotically stable in D.
In the realm of infectious diseases transmission, the coefficients governing this process are frequently affected by stochastic environmental disturbances [18,19]. This environmental interference can be mathematically characterized as standard Brownian motion. Relying solely on deterministic models to describe and predict the evolution of disease dynamics and transmission mechanisms is often inadequate. Hence, there is an increasing recognition of the practical significance of investigating infectious disease models that incorporate stochastic factors, leading to a growing scholarly focus on these stochastic frameworks in recent years [20,21,22]. For instance, Ji et al.[23,24] explored the threshold behavior of the SIR infection model in the presence of stochastic noise perturbations, examining both the persistence and extinction dynamics of the SIR model under various stochastic perturbation patterns. They derived threshold conditions for disease extinction and persistence utilizing Itô's formula and the stochastic comparison theorem. In another study, Zhao et al. [25] investigated a class of stochastic SIRS models characterized by saturated incidence, taking into account the dynamics of recovered individuals who lose immunity and revert to susceptibility after a period. They established conditions for disease extinction and persistence through the stochastic comparison theorem, supported by numerical simulations that corroborated their theoretical findings. Yang et al. [26] developed a stochastic multi-scale COVID-19 model that integrates both within-host and between-host dynamics, employing interval parameters. This model was derived through fast-slow decoupling via singular perturbation theory, distinguishing between a rapid within-host model and a slower between-host stochastic model. Rihan et al.[27,28] developed a stochastic epidemiological SIRC model to study the transmission of COVID-19 with cross-immunity classes and time-delayed transmission terms. Which the SIRC model is a new partition added to the SIR model, cross immunity (C). Echoing the insights of Mao et al. [29], this paper posits that fluctuations in the environment predominantly manifest as variations in the transmission coefficient
β→β+σ1B1(t),ν→ν+σ2B2(t), |
where Bi(t) is a standard Brownian motion and σi>0 indicates the white noise intensity, i=1,2. Then we have
{dS=(Λ−βSI−νSW−μS+ζR)dt−σ1SIdB1(t)−σ2SWdB2(t),dI=(βSI+νSW−γI−μI)dt+σ1SIdB1(t)+σ2SWdB2(t),dR=(γI−(μ+ζ)R)dt,dW=(ηI−cW)dt. | (1.3) |
Our model considers the loss of immunity rate and the effects of random disturbances based on Xiao et al.[13], investigating a stochastic SIRSW model that accounts for environmentally driven infection and incorporates multiparameter perturbations. It innovatively examines the impacts of both direct and indirect transmission rates on the spread of the disease through multiparameter perturbations. This model is more comprehensive and aligns more closely with real-world situations. However, as a multi-scale model that integrates macro and micro perspectives, it is challenging to unify the data across temporal and spatial scales. The numerical simulation remains relatively idealized, and there is still a significant gap between the results and the actual inter-evolutionary outcomes. It considers environmental pathogen infections with stochastic perturbations in two key parameters: direct and indirect transmission rates. We conclude that, compared to perturbations in the indirect infection rate, changes in noise intensity affecting the direct infection rate have a more significant impact on disease transmission. Additionally, we find that the direct transmission rate notably influences the threshold of Rs0. These parameters are crucial for analyzing the impact of environmental fluctuations on disease dynamics.
The structure of the paper is delineated as follows: In Sections 1 and 2, we present the foundational concepts, along with relevant notations and lemmas essential for our analysis. Section 3 is devoted to establishing the existence and uniqueness of global positive solutions for the SIRS infectious disease system under consideration. In Sections 4 and 5, we explore the sufficient conditions that govern the persistence and extinction of the stochastic SIRS infectious disease model. Section 6 focuses on analyzing the asymptotic stability of the disease-free equilibrium, as well as the endemic equilibrium of the deterministic counterpart to the stochastic model. To conclude, we provide a series of numerical simulations accompanied by a summary of our principal findings, aimed at elucidating the theoretical results presented throughout the paper.
In this paper, unless otherwise stated, let (Ω,F,{Ft}t≥0,P) denote the complete probability space of the filtration Ftt≥0 that satisfies the regularity condition. Let Bi(t)(i=1,2) denote the independent standard Brownian motions defined on this complete probability space. For any a,b∈R, note that
a∨b=max{a,b}. |
For convenience, the following symbols have been introduced,
⟨x(t)⟩=1t∫t0x(r)dr. |
Lemma 1. [30] (Strong law of large number) Let
M={Mt}t≥0 |
be a real-valued continuous local martingale, and
M(0)=0. |
Then,
limt→∞⟨M,M⟩t=∞,a.s.⇒limt→∞Mt⟨M,M⟩t=0a.s. |
and
lim supt→∞⟨M,M⟩tt<∞,a.s.⇒limt→∞Mtt=0a.s. |
Lemma 2. [23] Suppose f∈C[Ω×[0,+∞),R+] if there exists a positive ordinal λ,λ0, such that
lnf(t)≥λt−λ0∫t0f(s)ds+F(t),a.s. |
Then, for any t≥0, there are F∈C[Ω×[0,+∞),(−∞,+∞)] and
limt→∞F(t)t=0 |
a.s., such that
lim inft→∞1t⋅∫t0f(s)ds≥λλ0a.s. |
Lemma 3. Suppose f∈C[Ω×[0,+∞),R+] if there exists a positive ordinal λ,λ0, such that
lnf(t)≤λt−λ0∫t0f(s)ds+F(t),a.s. |
Then, for any t≥0, there are F∈C[Ω×[0,+∞),(−∞,+∞)] and
limt→∞F(t)t=0 |
a.s., such that
lim supt→∞1t⋅∫t0f(s)ds≤λλ0a.s. |
Remark 1. For the model (1.3), we have
d[S(t)+I(t)+R(t)]≤[Λ−μ(S(t)+I(t)+R(t))]dt, |
and we assume the initial values (S0,I0,R0,W0)∈D, which shows that the positive invariant set of the model is
D={(S,I,R,W)T∈R4+|0≤S(t)+I(t)+R(t)≤Λμ,0≤W(t)≤ηΛcμ}. |
The following theorem will show the existence and uniqueness of global positive solutions of system (1.3).
Theorem 1. For any initial value (S(0),I(0),R(0),W(0))∈R4+, there exists a positive salutation (S(t),I(t),R(t),W(t)) of the stochastic model (1.3) for t≥0, and the solution will hold in R4+ with probability one.
Proof. Since the coefficients of the model (1.3) satisfy the local Lipschits condition, for any S(0),I(0),R(0),W(0))∈R4+, there exists a locally unique solution (S(t),I(t),R(t),W(t)) on t∈[0,τε), where τe denotes the moment of explosion. It is sufficient to show that
τε=+∞a.s. |
Let k0≥1 denote a sufficiently large constant, and we have that S(0),I(0),R(0),W(0) are all in the interval [1k0,k0]. For any constant k>k0, define the stopping time,
τk=inf{t∈[0,τε):min[S(t),I(t),R(t),W(t)]≤1k |
or
max[S(t),I(t),R(t),W(t)]≥k}. |
Let
inf∅=∞, |
usually, ∞ denotes the empty set. Clearly, {τk}k≥k0 is a monotonically increasing function. If
τ∞=limk→∞τk, |
then τ∞≤τe a.s. If
τ∞=∞a.s., |
then for any t≥0,τe=∞, and (S(t),I(t),R(t),W(t))∈R4+a.s. Assuming that τ∞≠∞, there exist a constant T≥0 and ε∈(0,1) such that
P{τ∞≤T}>ε |
where, for any k≥k0, there exists a constant k1≥k0 such that, when all the k≥k1, there is
P{τk≤T}>ε. | (3.1) |
Define a C2-equation V: R4+→R+ as follows:
V(S,I,R,W)=(S+I+R+W)2+1S. |
Apply the Itˆo's formula
dV(S,I,R,W)=LV(S,I,R,W)dt+1Sσ1IdB1(t)+1Sσ2WdB2(t), | (3.2) |
where,
LV(S,I,R,W)=2(S+I+R+W)(Λ+ηI−cW−μS−μI−μR)−1S2(Λ−βSI−νSW+ζR−μS)+(2S2+1S)(σ21I2+σ22W2). | (3.3) |
Because of the mean value theorem, we can obtain
LV(S,I,R,W)≤Λ2+(S+I+R+W)2+2ηI(S+I+R+W)+1S(βI+νW+μ)+(2S2+1S)(σ21I2+σ22W2)=Λ2+S2(1+σ21I2+σ22W2)+2(SR+SW+WR)+I2(1+2η)+R2+W2+(SI+RI+WI)(2+2η)+1S(βI+νW+μ+σ21I2+σ22W2)≤Λ2+S2(1+σ21Λ2μ2+σ22Λ2η2c2μ2)+2(SR+SW+WR)+I2(1+2η)+R2+W2+(SI+RI+WI)(2+2η)+1S(βΛμ+νηΛcμ+μ+σ21Λ2μ2+σ22Λ2η2c2μ2)≤Λ2+H1(S+I+R+W)2+1S(βΛμ+νηΛcμ+μ+σ21Λ2μ2+σ22Λ2η2c2μ2)≤Λ2+H2[(S+I+R+W)2+1S]≤H(1+V), | (3.4) |
where,
H1=Max{1,1+2η,1+σ21Λ2μ2+σ22Λ2η2c2μ2},H2=Max{H1,βΛμ+νηΛcμ+μ+σ21Λ2μ2+σ22Λ2η2c2μ2},H=Max{H1,H2,Λ2}. | (3.5) |
H is a positive constant which is independent of S(t),I(t),R(t),W(t),t. Integrate both sides of the Eq (3.2) from 0 to
T∧τk=min{T,τk}, |
and then take the expectation as follows:
EV(S(T∧τk),I(T∧τk),R(T∧τk),W(T∧τk))≤E∫T∧τk0LV(s)dt+V(S(0),I(0),R(0),W(0))≤H∫T0EV(S(T∧τk),I(T∧τk),R(T∧τk),W(T∧τk))dt+V(S(0),I(0),R(0),W(0))+HT. | (3.6) |
By using Gronwall inequality [31], we have
EV(S(T∧τk),I(T∧τk),R(T∧τk),W(T∧τk))≤(V(S(0),I(0),R(0),W(0))+HT)eHT. | (3.7) |
Let
Ωk={τk≤T}, |
and by the inequality (3.1), it is known that
P{Ωk}≥ε. |
For any ω∈Ωk, S(τk,ω),I(τk,ω),R(τk,ω),W(τk,ω), at least one of them equals 1k or k, therefore,
V(S(τk,ω),I(τk,ω),R(τk,ω),W(τk,ω))≥(16k2+k)∧(16k2+1k). | (3.8) |
Combined with the above Eqs (3.2) and (3.8), we can get
(V(S(0),I(0),R(0),W(0))+HT)eHT≥E[IΩk(ω)V(S(τk,ω),I(τk,ω),R(τk,ω),W(τk,ω))]≥ε[(16k2+k)∧(16k2+1k)]. |
Here IΩk(ω) is the indicator function for Ωk. When k→+∞, there are
+∞>(V(S(0),I(0),R(0),W(0))+HT)eHT=+∞. |
This is a clear contradiction, and it is proved that
τ∞=+∞ |
a.s. Thus, the theorem can be proved.
As a stochastic infectious disease model, when diseases become extinct it is a major concern. In this section, we study the conditions for disease extinction and give a better condition for when a phenomenon like disease extinction will occur. Denote
RS0=R0−Λ2(σ21c2+σ22η2)2μ2c2(μ+γ),Rs=σ21+σ22η2c2−μ(βc+νη)cΛ. | (4.1) |
Theorem 2. Let (S(t),I(t),R(t),W(t)) be the solution of the model (1.3) with initial values (S(0),I(0),R(0),W(0))∈R4+. If
(σ21+σ22η2c2)>max{μ(βc+νη)cΛ,(β+νηc)22(μ+γ)} | (4.2) |
or
Rs0<1andRs≤0, | (4.3) |
then we have
lim supt→∞lnI(t)t<0a.s. |
It can be shown that the I(t) index tends to 0, the disease will become die out with probability one, and there are
limt→∞S(t)=Λμ,limt→∞I(t)=0,limt→∞R(t)=0,limt→∞W(t)=0a.s. |
Proof. Integration of the model (1.3) is obtained,
{S(t)−S(0)t=Λ−μ⟨S(t)⟩−β⟨S(t)I(t)⟩−ν⟨S(t)W(t)⟩+ζ⟨R(t)⟩ −σ1t∫t0S(r)I(r)dB1(r)−σ2t∫t0S(r)W(r)dB2(r),I(t)−I(0)t=β⟨S(t)I(t)⟩+ν⟨S(t)W(t)⟩−(μ+γ)⟨I(t)⟩ +σ1t∫t0S(r)I(r)dB1(r)+σ2t∫t0S(r)W(r)dB2(r),R(t)−R(0)t=γ⟨I(t)⟩−(μ+ζ)⟨R(t)⟩,W(t)−W(0)t=η⟨I(t)⟩−c⟨W(t)⟩. | (4.4) |
According to Eq (4.4), it can be obtained that
S(t)−S(0)t+I(t)−I(0)t+ζμ+ζR(t)−R(0)t=Λ−μ⟨S(t)⟩−(μ+μγμ+ζ)⟨I(t)⟩. | (4.5) |
From Eqs (4.4) and (4.5), we can get
⟨S(t)⟩=1t∫t0S(s)ds=Λμ−(μ+γ+ζμ+ζ)⟨I(t)⟩−φ(t),⟨W(t)⟩=1t∫t0W(s)ds=ηc⟨I(t)⟩−ϕ(t), | (4.6) |
where
φ(t)=1μt[S(t)−S(0)+I(t)−I(0)+ζμ+ζ(R(t)−R(0))],ϕ(t)=1ct[W(t)−W(0)]. | (4.7) |
Obviously,
limt→∞φ(t)=0,limt→∞ϕ(t)=0. | (4.8) |
Applying Itô's formula to the second equation of model (1.3) and integrating from 0 to t leads to
lnI(t)−lnI(0)=β∫t0S(s)ds+ν∫t0S(s)W(s)I(s)ds−σ212∫t0S2(s)ds−σ222∫t0S2(s)W2(s)I2(s)ds+σ1∫t0S(s)dB(s)−(μ+γ)t+σ2∫t0S(s)W(s)I(s)dB(s)⩽β∫t0S(s)ds+ν∫t0S(s)W(s)I(s)ds−σ212(∫t0S(s)ds)2−σ222(∫t0S(s)W(s)I(s)ds)2−(μ+γ)t+M1(t)+M2(t), | (4.9) |
where
M1(t)=σ1∫t0S(s)dB(s),M2(t)=σ2∫t0S(s)W(s)I(s)dB(s). |
Note that M1(t) and M2(t) are a real-valued continuous local martingale vanishing at time zero and
lim supt→∞⟨M1,M1⟩tt⩽σ21Λ2μ2<∞,lim supt→∞⟨M2,M2⟩tt⩽σ22η2Λ2c2μ2<∞, | (4.10) |
then by Lemma 1, it leads to
limt→∞M1(t)t=limt→∞σ1t∫t0S(s)dB(s)=0 a.s.,limt→∞M2(t)t=limt→∞σ2t∫t0S(s)W(s)I(s)dB(s)=0 a.s. | (4.11) |
Bringing Eq (4.6) into the above Eq (4.9), we have
lnI(t)−lnI(0)t=[Λμ−(μ+γ+ζμ+ζ)⟨I(t)⟩−φ(t)](β+νηc−νϕ(t)⟨I(t)⟩)−σ222[Λμ−(μ+γ+ζμ+ζ)⟨I(t)⟩−φ(t)]2[ηc−ϕ(t)⟨I(t)⟩]2−σ212[Λμ−(μ+γ+ζμ+ζ)⟨I(t)⟩−φ(t)]2−(μ+γ)+M1(t)t+M2(t)t=βΛμ−(μ+γ)−σ21Λ22μ2−(σ212+σ22η22c2)(μ+γ+ζμ+ζ)2⟨I(t)⟩2+(μ+γ+ζμ+ζ)(σ21Λμ+σ22Λη2μc2−νηc−β)⟨I(t)⟩+νΛημc−σ22Λ2η22μ2c2+Ψ(t), | (4.12) |
where
Ψ(t)=[σ21Λμ+σ22Λη2μc2−β−νηc−(σ21+σ22η2c2)((μ+γ+ζ)μ+ζ)⟨I(t)⟩]φ(t)−σ222[Λμ−(μ+γ+ζμ+ζ)⟨I(t)⟩−φ(t)]2[(ϕ(t)⟨I(t)⟩)2−2ηcϕ(t)⟨I(t)⟩]+ν[μ+γ+ζμ+ζ−Λμ⟨I(t)⟩+φ(t)⟨I(t)⟩]ϕ(t)+M1(t)t+M2(t)t. | (4.13) |
From Eqs (4.8) and (4.11), we obtain
limt→∞Ψ(t)=0 a.s. | (4.14) |
Case 1. Assume that Rs0<1 and Rs≤0, then the above Eq (4.12) gets
lnI(t)−lnI(0)t⩽βΛμ−(μ+γ)−σ21Λ22μ2−σ22Λ2η22μ2c2+νΛημc+Ψ(t), | (4.15) |
and combining (4.14), it can be obtained that
lim supt→∞lnI(t)t⩽βΛμ−(μ+γ)−σ21Λ22μ2−σ22Λ2η22μ2c2+νΛημc=(μ+γ)(ˉRs0−1)<0 a.s. | (4.16) |
Case 2. Assume that
(σ21+σ22η2c2)>max{μ(βc+νη)cΛ,(β+νηc)22(μ+γ)}, |
since
h(x)=−(σ212+σ22η22c2)(μ+γ+ζμ+ζ)2x2+(μ+γ+ζμ+ζ)(σ21Λμ+σ22Λη2μc2−νηc−β)x≤(σ21Λμ+σ22Λη2μc2−νηc−β)22(σ21+σ22η2c2). | (4.17) |
Therefore, from Eqs (4.12) and (4.17), we have
lnI(t)−lnI(0)t⩽βΛμ−(μ+γ)−σ21Λ22μ2−σ22Λ2η22μ2c2+νΛημc+(σ21Λμ+σ22Λη2μc2−νηc−β)22(σ21+σ22η2c2)+Ψ(t)=(β+νηc)22(σ21+σ22η2c2)−(μ+γ)+Ψ(t), | (4.18) |
and combining (4.14), it can be obtained that
lim supt→∞lnI(t)t⩽(β+νηc)22(σ21+σ22η2c2)−(μ+γ)<0 a.s. | (4.19) |
From the proofs of Cases 1 and 2, it can be shown that the I(t) index tends to 0 and the disease will become die out with probability one, then we have
limt→∞I(t)=0 a.s. | (4.20) |
Let
Ωi={ω∈Ω:limt→∞I(t,ω)=0}, |
Accroding to (4.20), we have
P(Ωi)=1. |
For any θ>0 and ω∈Ωi, there exists
Ti=Ti (ω,θ)>0, |
such that for any t≥Ti, there exists
I(t,ω)≤θ. |
Substituting it into the third equation of model (1.3), and according to the comparison theorem for stochastic differential equations, we obtain
lim supt→∞R(t,ω)≤γθμ+ζ, ω∈Ωi,t≥Ti. | (4.21) |
For all ω∈Ωi and t>0, such that R(t,ω), since the arbitrariness of θ, we can get
limt→∞R(t,ω)=0, ω∈Ωi,t≥Ti. | (4.22) |
It follows from
P(Ωi)=1 |
that, consequently,
limt→∞R(t)=0 a.s. | (4.23) |
Similarly, we have
limt→∞W(t)=0 a.s. | (4.24) |
Through model (1.3), we can obtain
d(S(t)+I(t)+R(t))dt=Λ−μ(S+I+R), |
therefore,
limt→∞[S(t)+I(t)+R(t)]=Λμ a.s., |
which, together with Eqs (4.20) and (4.23), yields
limt→∞S(t)=Λμ a.s. | (4.25) |
This completes the proof.
Disease persistence is an important characteristic of infectious disease dynamics, meaning that the disease persists in the population. Theorem 3 will show the persistence of disease.
Theorem 3. If RS0>1,Rs≤0, let (S(t),I(t),R(t),W(t)) be the solution of the model (1.3) with initial values (S(0),I(0),R(0),W(0))∈Ω. It has the following properties:
lim supt→∞1t∫t0I(s)ds⩽(μ+γ)(Rs0−1)(μ+γ+ζμ+ζ)(νηc+β−σ21Λμ−σ22Λη2μc2),lim inft→∞1t∫t0I(s)ds⩾(μ+γ)(Rs0−1)(1+ηc)(μ+γ+ζμ+ζ)a.s. |
Proof. From the above Eq (4.12), we get:
lnI(t)−lnI(0)t≤βΛμ−(μ+γ)−σ21Λ22μ2+νΛημc−σ22Λ2η22μ2c2+Ψ(t)−(σ212+σ22η22c2)(μ+γ+ζμ+ζ)2(1t∫t0I(s)ds)2+(μ+γ+ζμ+ζ)(σ21Λμ+σ22Λη2μc2−νηc−β)1t∫t0I(s)ds. | (5.1) |
Therefore,
lnI(t)t=−(μ+γ+ζμ+ζ)(νηc+β−σ21Λμ−σ22Λη2μc2)1t∫t0I(s)ds+(μ+γ)(Rs0−1)+lnI(0)t+Ψ(t). | (5.2) |
By Eq (4.14) and Lemma 3, we have
lim supt→∞1t∫t0I(s)ds⩽(μ+γ)(Rs0−1)(μ+γ+ζμ+ζ)(νηc+β−σ21Λμ−σ22Λη2μc2) a.s. |
Bring Eq (4.24) to Eq (4.9), and by Remark 1, we have
lnI(t)−lnI(0)t=β⟨S(t)⟩+ν⟨S(t)⟩⟨W(t)⟩⟨I(t)⟩−(μ+γ)−σ21Λ22μ2−σ22Λ2η22μ2c2+M1(t)t+M2(t)t. | (5.3) |
Bringing Eq (4.6) into the above Eq (5.3), we have
lnI(t)−lnI(0)t=βΛμ+νΛημc−(μ+γ)−σ21Λ22μ2−σ22Λ2η22μ2c2−(1+ηc)(μ+γ+ζμ+ζ)∫t0I(s)ds+Φ(t). | (5.4) |
Therefore, it is possible to get
lnI(t)t=(μ+γ)(Rs0−1)−(1+ηc)(μ+γ+ζμ+ζ)∫t0I(s)ds+lnI(0)t+Φ(t), | (5.5) |
where
Φ(t)=νϕ(t)[φ(t)⟨I(t)⟩−Λμ⟨I(t)⟩+μ+γ+ζμ+ζ]−(ηc+β)φ(t)+M1(t)t+M2(t)t. | (5.6) |
From Eqs (4.8) and (4.11), we can get
limt→∞Φ(t)=0. |
By Lemma 2 and Eq (5.5), one obtains
lim inft→∞1t∫t0I(s)ds⩾(μ+γ)(Rs0−1)(1+ηc)(μ+γ+ζμ+ζ). |
The proof is complete.
In epidemiology, stability is of high practical importance. This section discusses the asymptotical stability of the disease-free equilibrium and the endemic equilibrium of the deterministic model corresponding to the stochastic model.
The basic reproduction number plays an important role in the study of infectious disease dynamics and determines whether a disease becomes extinct or not. We know that the basic reproduction number of a deterministic system
R0=Λ(βc+νη)μc(μ+γ), |
and the disease-free equilibrium of the deterministic model is E0(Λμ,0,0).
Theorem 4. Let (S(t),I(t),R(t),W(t)) be the solution of the model (1.3) with initial values (S(0),I(0),R(0),W(0))∈Ω. If R0<1 and σ1,σ2 are small enough, then
limt→∞1tE∫t0(μ(S−Λμ)2+(1−R0)(γ+μ)I+ΛνμW+(Λ+μ)ζμR)ds≤Λ2μ2(βΛμ+(Λ+μ)μημc+ζ)+ζγΛ(μ+ζ)μ+Λ22μ2(σ21+σ22η2c2)a.s. |
Proof. Define a Lyapunov function V: R4+→R+ as follows:
V1(S,I)=12(S−Λμ)2+I,V2(R,W)=ΛνμcW+ζμ+ζR,V3(S,I,R,W)=V1(S,I)+V2(R,W). | (6.1) |
Applying Itˆo's formula, we have
LV1=(S−Λμ)(Λ−βSI−νSW−μS+ζR)+(βSI+νSW−γI−μI)+12σ21S2I2+12σ22S2W2=−μ(S−Λμ)2−S(βSI+νSW)+Λμ(βSI+νSW)+(S−Λμ)ζR+(βSI+νSW−γI−μI)+12σ21S2I2+12σ22S2W2≤−μ(S−Λμ)2+Λμ(βSI+νSW)+ζSR−ΛζμR+ΛβμI+νSW−(γ+μ)I+12σ21S2I2+12σ22S2W2,LV2=Λνμc(ηI−cW)+ζμ+ζ(γI−μR−ζR)≤ΛνημcI−ΛνμW+ζγμ+ζI−ζR. |
Therefore,
LV3≤−μ(S−Λμ)2+ΛνημcI+βΛμI−(γ+μ)I−ΛνμW−(Λζμ+ζ)R+Λμ(βSI+νSW)+ζSR+νSW+ζγμ+ζI+12σ21S2I2+12σ22S2W2,≤−μ(S−Λμ)2+(R0−1)(γ+μ)I−ΛνμW−(Λ+μ)ζμR+βΛ3μ3+(Λ+μ)μηΛ2μ3c+ζΛ2μ2+ζγΛ(μ+ζ)μ+σ21Λ22μ2+σ22Λ2η22μ2c2. |
Due to
dV3(S,I,R,W)=(LV3)dt+(1+Λμ−S)(σ1SIdB1(t)+σ2SWdB2(t)), | (6.2) |
integrating both sides of the above Eq (6.2) from 0 to t, and then taking the expectation as follows:
0≤EV3(t)−V3(0)=E∫t0LV3(s)ds≤−E∫t0[μ(S(s)−Λμ)2+(1−R0)(γ+μ)I(s)+(Λ+μ)ζμR(s)+ΛνμW(s)]ds+(Λ2μ2(βΛμ+(Λ+μ)μημc+ζ)+ζγΛ(μ+ζ)μ+Λ22μ2(σ21+σ22η2c2))t. | (6.3) |
Therefore,
limt→∞1tE∫t0(μ(S−Λμ)2+(1−R0)(γ+μ)I+ΛνμW+(Λ+μ)ζμR)ds≤Λ2μ2(βΛμ+(Λ+μ)μημc+ζ)+ζγΛ(μ+ζ)μ+Λ22μ2(σ21+σ22η2c2). |
The proof is complete.
In studying epidemic dynamics, the stochastic infectious disease model has no endemic equilibrium, but it is obtained from a deterministic model in which the infectious rate is subjected to a random perturbation. Therefore, the asymptotic behavior of the solution of the stochastic model in the region around E∗(S∗,I∗,R∗,W∗) is studied. The expressions E∗ is as follows:
E∗=(c(μ+γ)(βc+νη),Λ(μ+ζ)μ(μ+ζ+γ)(1−1R0),Λγμ(μ+ζ+γ)(1−1R0),Λη(μ+ζ)μc(μ+ζ+γ)(1−1R0)). |
Theorem 5. Let (S(t),I(t),R(t),W(t)) be the solution of the model (1.3) with initial values (S(0),I(0),R(0),W(0))∈Ω. If R0>1,
μ>ϵ2ζ, γ+μ>ϵη+ϵγ+ϵ2ζ, 2γI∗R∗>γ+ζϵ, 2ηI∗W∗>ηϵ. |
Then,
limt→∞1tE∫t0[(μ−ϵ2ζ)(S−S∗)2+(γ+μ−ϵη−ϵγ−ϵ2ζ)(I−I∗)2+(2γI∗R∗−γ+ζϵ)(R−R∗)2+(2ηI∗W∗−ηϵ)(W−W∗)2]ds≤Λμ(γ+2μ)(S∗+I∗)+σ21Λ4μ4+σ22Λ4η2μ4c2. |
Proof. The deterministic system obeys the following relationship at the endemic equilibrium:
Λ=βS∗I∗+νS∗W∗+μS∗−ζR∗,βS∗I∗+νS∗W∗=(γ+μ)I∗,γI∗=(μ+ζ)R∗,ηI∗=cW∗. | (6.4) |
Define a Lyapunov function V: R4+→R+ as follows:
V4(S,I)=12((S−S∗)+(I−I∗))2,V5(R,W)=(R−R∗)2+(W−W∗)2,V6(S,I,R,W)=V4+V5. | (6.5) |
Applying Itˆo's formula, we have
LV4=(S−S∗+I−I∗)(Λ−μS+ζR−γI−μI)+σ21S2I2+σ22S2W2=−μ(S−S∗)2−(γ+μ)(I−I∗)2−(γ+2μ)(S−S∗)(I−I∗)+ζ(R−R∗)(S−S∗)+ζ(R−R∗)(I−I∗)+σ21S2I2+σ22S2W2,LV5=2(R−R∗)(γI−μR−ζR)+2(W−W∗)(ηI−cW)=2(R−R∗)γI∗(II∗−I∗I∗+R∗R∗−RR∗)+2(W−W∗)ηI∗(II∗−I∗I∗+W∗W∗−WW∗)=2γ(R−R∗)(I−I∗)−2γI∗R∗(R−R∗)2+2η(W−W∗)(I−I∗)−2ηI∗W∗(W−W∗)2. |
Therefore,
LV6=−μ(S−S∗)2−(γ+μ)(I−I∗)2−2γI∗R∗(R−R∗)2−2ηI∗W∗(W−W∗)2−(γ+2μ)(SI−S∗I−SI∗+S∗I∗)+(2γ+ζ)(R−R∗)(I−I∗)+ζ(R−R∗)(S−S∗)+2η(W−W∗)(I−I∗)+σ21S2I2+σ22S2W2, |
and because a≤|a|, we can get
LV6≤−μ(S−S∗)2−(γ+μ)(I−I∗)2−2γI∗R∗(R−R∗)2−2ηI∗W∗(W−W∗)2+(γ+2μ)(S∗I+SI∗)+|(2γ+ζ)||(R−R∗)||(I−I∗)|+|ζ||(R−R∗)||(S−S∗)|+|2η||(W−W∗)||(I−I∗)|+σ21S2I2+σ22S2W2. |
Take a positive number ϵ, such that
2ab≤ϵa2+b2ϵ, |
and from Remark 1, we have
LV6≤−μ(S−S∗)2−(γ+μ)(I−I∗)2−2γI∗R∗(R−R∗)2−2ηI∗W∗(W−W∗)2+Λμ(γ+2μ)(S∗+I∗)+12ϵζ(R−R∗)2+ϵ2ζ(S−S∗)2+12ϵ(2γ+ζ)(R−R∗)2+ϵ2(2γ+ζ)(I−I∗)2+ηϵ(W−W∗)2+ϵη(I−I∗)2+σ21S2I2+σ22S2W2≤(ϵ2ζ−μ)(S−S∗)2−(γ+μ−ϵη−ϵγ−ϵ2ζ)(I−I∗)2−(2γI∗R∗−γ+ζϵ)(R−R∗)2−(2ηI∗W∗−ηϵ)(W−W∗)2+Λμ(γ+2μ)(S∗+I∗)+σ21Λ4μ4+σ22Λ4η2μ4c2. |
Due to
dV6(S,I,R,W)=(LV4+LV5)dt+(S−S∗+I−I∗)(−σ1SIdB1(t)−σ2SWdB2(t))+(S−S∗+I−I∗)(σ1SIdB1(t)+σ2SWdB2(t)), | (6.6) |
integrating both sides of the above Eq (6.6) from 0 to t, and then taking the expectation as follows,
E∫t0LV6(s)ds=EV6(t)−V6(0)≤−E∫t0[(μ−ϵ2ζ)(S−S∗)2+(γ+μ−ϵη−ϵγ−ϵ2ζ)(I−I∗)2+(2γI∗R∗−γ+ζϵ)(R−R∗)2+(2ηI∗W∗−ηϵ)(W−W∗)2]ds+(Λμ(γ+2μ)(S∗+I∗)+σ21Λ4μ4+σ22Λ4η2μ4c2)t. | (6.7) |
Because of
E∫t0LV6(s)ds≥0, |
we obtain
limt→∞1tE∫t0[(μ−ϵ2ζ)(S−S∗)2+(γ+μ−ϵη−ϵγ−ϵ2ζ)(I−I∗)2+(2γI∗R∗−γ+ζϵ)(R−R∗)2+(2ηI∗W∗−ηϵ)(W−W∗)2]ds≤Λμ(γ+2μ)(S∗+I∗)+σ21Λ4μ4+σ22Λ4η2μ4c2. |
The proof is complete.
Numerical simulations are presented below to illustrate the theoretical results of this chapter. We provide some numerical examples to support our results. The numerical simulations of epidemic dynamics are carried out for academic purposes, using arbitrary parameter values that do not correspond to any specific epidemic and only demonstrate the theoretical properties of the numerical solutions of the models considered. We present our results using the Milstein's higher order method developed in [32]. The discrete form of the model (1.3) is as follows:
{Si+1=Si+(Λ−βSiIi−νSiWi−μSi+ζRi)Δt−σ1SiIiξ1,i√Δt−σ212SiIi(ξ21,i−1)Δt−σ2SiWiξ2,i√Δt−σ222SiWi(ξ22,i−1)Δt,Ii+1=Ii+(βSiIi+νSiWi−μIi−γIi)Δt+σ1SiIiξ1,i√Δt+σ212SiIi(ξ21,i−1)Δt+σ2SiWiξ2,i√Δt+σ222SiWi(ξ22,i−1)Δt,Ri+1=Ri+(γIi−μRi−ζRi)Δt,Wi+1=Wi+(ηIi−cWi)Δt, | (7.1) |
where ξj,ij=1,2 are Gaussian random variables following the standard normal distribution N(0,1) and the time increment
Δt=0.01. |
Let
Λ=0.9,μ=0.36,ζ=0.3,Γ=0.1,η=0.3,c=0.5,(S(0),I(0),R(0),W(0))=(1.2,1.2,0.1,0.5). | (7.2) |
In different examples, parameters β,ν,σ1, and σ2 will take different values.
Example 1. To start, we choose
β=0.15, ν=0.16, σ1=0.55, σ2=0.11, |
such that
(σ21+σ22η2c2)−max{μ(βc+νη)cΛ,(β+νηc)22(μ+γ)}=0.2411>0, |
then from Theorem 2, the disease of model (1.3) will become extinct; see Figure 1.
Let
β=0.14, ν=0.1, σ1=0.12, σ2=0.32, |
and the other parameters are shown in (7.2) such that
RS0=R0−Λ2(σ21c2+σ22η2)2μ2c2(μ+γ)=0.7387<1 |
and
(σ21+σ22η2c2)−μ(βc+νη)cΛ=−0.0287<0. |
According to Theorem 2, the disease of model (1.3) will be extinct; see Figure 2.
In order to verify Theorem 2, numerical simulations were carried out with the parameters selected above. The results are shown in Figures 1 and 2. From the Figures 1b and 2b, it can be concluded that the disease I(t) in stochastic model (1.3) will die out with probability one, and compared to deterministic model (1.2), white noise accelerates disease extinction and inhibits disease transmission.
Example 2. To begin, we choose
β=0.1, ν=0.1, σ1=0.05, σ2=0.05, |
and the other parameters are shown in (7.2) such that
RS0=R0−Λ2(σ21c2+σ22η2)2μ2c2(μ+γ)=1.1618>1 |
and
(σ21+σ22η2c2)−μ(βc+νη)cΛ=−0.0499<0. |
According to Theorem 3, the disease of model (1.3) will be persist. Figure 3 supports the result.
In order to verify Theorem 3, numerical simulations were carried out with the parameters selected above. The results are shown in Figure 3. From the Figure 3b, it can be concluded that the disease I(t) in stochastic model (1.3) will be permanent in the time mean. This suggests that the disease will persist.
Example 3. Take
β=0.1, ν=0.1,andϵ=0.4, |
and the other parameters are shown in (7.2) such that
R0=1.2000>1,E∗=(2.4995,0.4290,0.0715,0.2575), |
and
μ−ϵ2ζ=0.24>0,γ+μ−ϵη+ϵγ+ϵ2ζ=0.18>0,2γI∗R∗−γ+ζϵ=0.2>0,2ηI∗W∗−ηϵ=0.8320>0. |
According to Theorem 5, solutions of stochastic model (1.3) fluctuate in time average around endemic equilibrium E∗ of the deterministic model, which can be verified by using Figure 4, and the oscillation amplitude increases with white noise intensity.
Example 4. Take
β=0.15, ν=0.16 |
and the other parameters are shown in (7.2) such that
R0=1.3370>1. |
Figure 5 shows that σ1 and σ2 have a significant impact on both extinction and persistence of disease. With the intensity of σ1,σ2, the disease of model (1.3) will accelerate extinction.
The results show that large amounts of white noise can lead to disease extinction, while even small amounts of white noise were found to inhibit disease outbreaks. We conclude that changes in noise intensity affecting direct transmission rates have a more pronounced effect on disease spread than perturbations in indirect infection rates. This tells us that in the prevention and control of infectious diseases, as well as in public health practice, cutting off direct sources of infection and reducing the rate of direct infection are very useful measures.
Example 5. Take σ1=0.25, σ2=0.1, and the other parameters are shown in (7.2). Figure 6a shows how threshold Rs0 varies with β and ν, as well as shows the positive correlation. Figure 6b shows how threshold Rs0 varies with white noise intensity σ1 and σ2, and as the noise intensity increases, Rs0 becomes smaller and smaller.
In this paper, we investigate a stochastic SIRS epidemic model that incorporates environmentally driven transmission dynamics alongside multiparameter perturbations. The purpose of this study is to investigate the extinction and persistence of stochastic SIRW model solutions under multiparameter stochastic perturbations, the propagation laws of infectious disease dynamics, and the effects of different parameters on disease spread. We commence our study by establishing the existence and uniqueness of the global positive solution for the model presented in Eq (1.3). Subsequently, we derive the threshold conditions necessary for disease extinction and persistence, employing the comparison theorem in conjunction with Itˆo's formula for stochastic differential equations. The theoretical findings are substantiated through a series of numerical simulations, as depicted in Figures 1–3. These simulations show that large amounts of white noise can lead to disease extinction, whereas even small amounts of white noise can suppress disease outbreaks, with the dynamics transitioning from persistence to extinction as the noise intensity increases. Furthermore, we analyze the asymptotic stability of both the disease-free equilibrium and the endemic equilibrium of the deterministic model corresponding to our stochastic framework, utilizing principles from stochastic stability theory. Our results demonstrate that the solutions of the stochastic model (1.3) exhibit fluctuations around the endemic equilibrium E∗ of the deterministic counterpart, with the oscillation amplitude increasing in response to higher levels of white noise intensity, as shown in Figure 4. Additionally, we observe that variations in noise intensity affecting the direct transmission rate exert a more pronounced influence on disease transmission compared to perturbations in the indirect infection rate, as illustrated in Figure 5. Finally, we find that the direct transmission rate plays a critical role in determining the threshold Rs0, as highlighted in Figure 6. This suggests that disruption of direct source links and isolation controls to reduce the rate of direct infection are very useful measures in the prevention and control of infectious diseases and in public health practice.
In the future, we will consider the dynamics of infectious disease processes across different temporal scales. It is also interesting to incorporate the immunological processes occurring within the host into system (1.2), and we will leave this for future research.
Zhengwen Yin: responsible for mathematical modelling and analysis, paper writing, numerical simulations; Yuanshun Tan: responsible for mathematical modelling, model analysis, paper framework construction. All authors have read and agreed to the published version of the manuscript.
This work was supported by the National Natural Scicnce Foundation of China (No. 12271068), the Rescarch and Innovation Project for Graduate Research in Chongqing Jiaotong University (No. 2024S0138).
The authors declare that they have no conflicts of interest concerning this article.
[1] |
W. O. Kermack, A. G. McKendrick, Contributions to the mathematical theory of epidemics–I, Bull. Math. Biol., 53 (1991), 33–55. https://doi.org/10.1007/BF02464423 doi: 10.1007/BF02464423
![]() |
[2] |
W. M. Liu, H. W. Hethcote, S. A. Levin, Dynamical behavior of epidemiological models with nonlinear incidence rates, J. Math. Biol., 25 (1987), 359–380. https://doi.org/10.1007/BF00277162 doi: 10.1007/BF00277162
![]() |
[3] |
H. C. Tuckwell, R. J. Williams, Some properties of a simple stochastic epidemic model of SIR type, Math. Biosci., 208 (2007), 76–97. https://doi.org/10.1016/j.mbs.2006.09.018 doi: 10.1016/j.mbs.2006.09.018
![]() |
[4] |
X. Wei, Global analysis of a network-based SIR epidemic model with a saturated treatment function, Int. J. Biomath., 17 (2024), 2350112. https://doi.org/10.1142/S1793524523501127 doi: 10.1142/S1793524523501127
![]() |
[5] |
J. Li, J. Zhang, Z. Ma, Global analysis of some epidemic models with general contact rate and constant immigration, Appl. Math. Mech., 25 (2004), 396–404. https://doi.org/10.1007/BF02437523 doi: 10.1007/BF02437523
![]() |
[6] |
C. Y. Ji, D. Q. Jiang, The extinction and persistence of a stochastic SIR model, Adv. Differ. Equations, 2017 (2017), 30. https://doi.org/10.1186/s13662-016-1068-z doi: 10.1186/s13662-016-1068-z
![]() |
[7] |
N. Wang, L. Zhang, Z. D. Teng, Dynamics in a reaction-diffusion epidemic model via environmental driven infection in heterogenous space, J. Biol. Dyn., 16 (2021), 373–396. https://doi.org/10.1080/17513758.2021.1900428 doi: 10.1080/17513758.2021.1900428
![]() |
[8] |
L. Liu, X. Q. Zhao, Y. Zhou, A tuberculosis model with seasonality, Bull. Math. Biol., 72 (2010), 931–952. https://doi.org/10.1007/s11538-009-9477-8 doi: 10.1007/s11538-009-9477-8
![]() |
[9] |
D. Posny, J. Wang, Modelling cholera in periodic environments, J. Biol. Dyn., 8 (2014), 1–19. https://doi.org/10.1080/17513758.2014.896482 doi: 10.1080/17513758.2014.896482
![]() |
[10] |
X. Y. Wang, S. P. Wang, A multiscale model of COVID-19 dynamics, Bull. Math. Biol., 84 (2022), 99. https://doi.org/10.1007/s11538-022-01058-8 doi: 10.1007/s11538-022-01058-8
![]() |
[11] |
A. Abulajiang, Z. D. Teng, L. Zhang, Dynamics in a disease transmission model coupled virus infection in host with incubation delay and environmental effects, J. Appl. Math. Comput., 68 (2022), 4331–4359. https://doi.org/10.1007/s12190-022-01709-y doi: 10.1007/s12190-022-01709-y
![]() |
[12] |
Z. L. Feng, J. Velasco-Hernandez, B. Tapia-Santos, A mathematical model for coupling within-host and between-host dynamics in an environmentally-driven infectious disease, Math. Biosci., 241 (2013), 49–55. https://doi.org/10.1016/j.mbs.2012.09.004 doi: 10.1016/j.mbs.2012.09.004
![]() |
[13] |
Y. N. Xiao, C. C. Xiang, R. A. Cheke, S. Tang, Coupling the macroscale to the microscale in a spatiotemporal context to examine effects of spatial diffusion on disease transmission, Bull. Math. Biol., 82 (2020), 58. https://doi.org/10.1007/s11538-020-00736-9 doi: 10.1007/s11538-020-00736-9
![]() |
[14] |
B. Edoardo, Y. Kuang, Modeling and analysis of a marine bacteriophage infection, Math. Biosci., 149 (1998), 57–76. https://doi.org/10.1016/S0025-5564(97)10015-3 doi: 10.1016/S0025-5564(97)10015-3
![]() |
[15] |
I. Siekmann, H. Malchow, E. Venturino, An extension of the Beretta-Kuang model of viral diseases, Math. Biosci. Eng., 5 (2008), 549–565. https://doi.org/10.3934/mbe.2008.5.549 doi: 10.3934/mbe.2008.5.549
![]() |
[16] |
L. N. Nkamba, J. M. Ntaganda, H. Abboubakar, J. C. Kamgang, L. Castelli, Global stability of a SVEIR epidemic model: application to poliomyelitis transmission dynamics, Open J. Modell. Simul., 5 (2017), 98–112. https://doi.org/10.4236/ojmsi.2017.51008 doi: 10.4236/ojmsi.2017.51008
![]() |
[17] |
C. Li, C. Tsai, S. Yang, Analysis of epidemic spreading of an SIRS model in complex heterogeneous networks, Commun. Nonlinear Sci. Numer. Simul., 19 (2014), 1042–1054. https://doi.org/10.1016/j.cnsns.2013.08.033 doi: 10.1016/j.cnsns.2013.08.033
![]() |
[18] |
T. Gard, Persistence in stochastic food web models, Bull. Math. Biol., 46 (1984), 357–370. https://doi.org/10.1007/BF02462011 doi: 10.1007/BF02462011
![]() |
[19] | R. May, Stability and complexity in model ecosystems, Princeton University Press, 2019. https://doi.org/10.2307/j.ctvs32rq4 |
[20] |
Y. M. Wang, G. R. Liu, Dynamics analysis of a stochastic SIRS epidemic model with nonlinear incidence rate and transfer from infectious to susceptible, Math. Biosci. Eng., 16 (2019), 6047–6070. https://doi.org/10.3934/mbe.2019303 doi: 10.3934/mbe.2019303
![]() |
[21] |
X. Zhou, X. Shi, M. Wei, Dynamical behavior and optimal control of a stochastic mathematical model for cholera, Chaos Solitons Fract., 156 (2022), 111854. https://doi.org/10.1016/j.chaos.2022.111854 doi: 10.1016/j.chaos.2022.111854
![]() |
[22] |
Y. Tan, Y. Cai, X. Wang, Z. Peng, K. Wang, R. Yao, et al., Stochastic dynamics of an SIS epidemiological model with media coverage, Math. Comput. Simul., 204 (2023), 1–27. https://doi.org/10.1016/j.matcom.2022.08.001 doi: 10.1016/j.matcom.2022.08.001
![]() |
[23] |
C. Y. Ji, D. Q. Jiang, Threshold behaviour of a stochastic SIR model, Appl. Math. Modell., 38 (2014), 5067–5079. https://doi.org/10.1016/j.apm.2014.03.037 doi: 10.1016/j.apm.2014.03.037
![]() |
[24] |
C. Y. Ji, D. Q. Jiang, N. Z. Shi, The behavior of an SIR epidemic model with stochastic perturbation, Stochastic Anal. Appl. Appl., 30 (2012), 755–773. https://doi.org/10.1080/07362994.2012.684319 doi: 10.1080/07362994.2012.684319
![]() |
[25] |
Y. Zhao, D. Jiang, The threshold of a stochastic SIRS epidemic model with saturated incidence, Appl. Math. Lett., 34 (2014), 90–93. https://doi.org/10.1016/j.aml.2013.11.002 doi: 10.1016/j.aml.2013.11.002
![]() |
[26] |
Q. Yang, J. Huang, A stochastic multi-scale COVID-19 model With interval parameters, J. Appl. Anal. Comput., 14 (2024), 515–542. http://doi.org/10.11948/20230298 doi: 10.11948/20230298
![]() |
[27] |
F. A. Rihan, H. J. Alsakaji, Dynamics of a stochastic delay differential model for COVID-19 infection with asymptomatic infected and interacting people: case study in the UAE, Results Phys., 28 (2021), 104658. https://doi.org/10.1016/j.rinp.2021.104658 doi: 10.1016/j.rinp.2021.104658
![]() |
[28] |
F. A. Rihan, H. J. Alsakaji, C. Rajivganthi, Stochastic SIRC epidemic model with time-delay for COVID-19, Adv. Differ. Equations, 2020 (2020), 502. https://doi.org/10.1186/s13662-020-02964-8 doi: 10.1186/s13662-020-02964-8
![]() |
[29] |
X. Mao, G. Marion, E. Renshaw, Environmental Brownian noise suppresses explosions in population dynamics, Stochastic Process. Appl., 97 (2002), 95–110. https://doi.org/10.1016/S0304-4149(01)00126-0 doi: 10.1016/S0304-4149(01)00126-0
![]() |
[30] | X. Mao, Stochastic differential equations and applications, Elsevier, 2007. https://doi.org/10.1007/978-3-642-11079-5-2 |
[31] |
T. H. Gronwall, Note on the derivatives with respect to a parameter of the solutions of a system of differential equations, Ann. Math., 40 (1919), 292–296. https://doi.org/10.2307/1967124 doi: 10.2307/1967124
![]() |
[32] |
D. J. Higham, An algorithmic introduction to numerical simulation of stochastic differential equations, Soc. Ind. Appl. Math. Rev., 43 (2001), 525–546. https://doi.org/10.1137/S0036144500378302 doi: 10.1137/S0036144500378302
![]() |