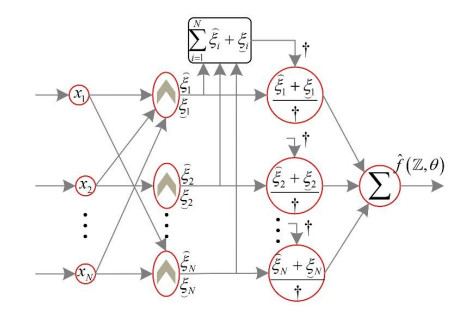
The present study explores the effects of viscous dissipation, the thermal dependent conductivity and the thermal dependent viscosity on the steady motion of a Powell-Eyring fluid over a stratified stretching sheet which embedded in a porous medium. The fact that the nature of non-Newtonian flows problems are highly nonlinear equations has been taken into consideration here and this was the motive objective to determine numerical solutions. So, the emphasis is on the methodology adopted for obtaining numerical solutions that yielded after employing the Chebyshev spectral method. The temperature distributions and the velocity components are evaluated by solving numerically the boundary value problems that correspond to the proposed problem. Then, some figures have been plotted to elucidates the effect of different physical parameters appearing in the problem on both the temperature and the velocity profiles. The presence of the thermal radiation and the viscous dissipation in the fluid flow are shown to have quite a dramatic effect on the temperature profiles. In culmination, cooling process in nuclear reactors and geothermal engineering especially in the presence of thermal stratification phenomenon can be adopted as an application of this study. The theoretical and the observed results provide a fairly good qualitative agreement.
Citation: W. Abbas, Ahmed M. Megahed. Powell-Eyring fluid flow over a stratified sheet through porous medium with thermal radiation and viscous dissipation[J]. AIMS Mathematics, 2021, 6(12): 13464-13479. doi: 10.3934/math.2021780
[1] | Shufen Zhao, Xiaoqian Li, Jianzhong Zhang . S-asymptotically ω-periodic solutions in distribution for a class of stochastic fractional functional differential equations. Electronic Research Archive, 2023, 31(2): 599-614. doi: 10.3934/era.2023029 |
[2] | Seda IGRET ARAZ, Mehmet Akif CETIN, Abdon ATANGANA . Existence, uniqueness and numerical solution of stochastic fractional differential equations with integer and non-integer orders. Electronic Research Archive, 2024, 32(2): 733-761. doi: 10.3934/era.2024035 |
[3] | Changling Xu, Huilai Li . Two-grid methods of finite element approximation for parabolic integro-differential optimal control problems. Electronic Research Archive, 2023, 31(8): 4818-4842. doi: 10.3934/era.2023247 |
[4] | Jingyun Lv, Xiaoyan Lu . Convergence of finite element solution of stochastic Burgers equation. Electronic Research Archive, 2024, 32(3): 1663-1691. doi: 10.3934/era.2024076 |
[5] | Meng Gao, Xiaohui Ai . A stochastic Gilpin-Ayala nonautonomous competition model driven by mean-reverting OU process with finite Markov chain and Lévy jumps. Electronic Research Archive, 2024, 32(3): 1873-1900. doi: 10.3934/era.2024086 |
[6] | Kai Gao . Global boundedness of classical solutions to a Keller-Segel-Navier-Stokes system involving saturated sensitivity and indirect signal production in two dimensions. Electronic Research Archive, 2023, 31(3): 1710-1736. doi: 10.3934/era.2023089 |
[7] | Dongxing Fu, Xiaowei Xu, Zhibing Zhao . Generalized tilting modules and Frobenius extensions. Electronic Research Archive, 2022, 30(9): 3337-3350. doi: 10.3934/era.2022169 |
[8] | Zihan Zheng, Juan Wang, Liming Cai . Global boundedness in a Keller-Segel system with nonlinear indirect signal consumption mechanism. Electronic Research Archive, 2024, 32(8): 4796-4808. doi: 10.3934/era.2024219 |
[9] | Zhiwei Hao, Huiqin Zheng . Existence and multiplicity of solutions for fractional p(x)-Kirchhoff-type problems. Electronic Research Archive, 2023, 31(6): 3309-3321. doi: 10.3934/era.2023167 |
[10] | Guaiqi Tian, Hongmin Suo, Yucheng An . Multiple positive solutions for a bi-nonlocal Kirchhoff-Schr¨odinger-Poisson system with critical growth. Electronic Research Archive, 2022, 30(12): 4493-4506. doi: 10.3934/era.2022228 |
The present study explores the effects of viscous dissipation, the thermal dependent conductivity and the thermal dependent viscosity on the steady motion of a Powell-Eyring fluid over a stratified stretching sheet which embedded in a porous medium. The fact that the nature of non-Newtonian flows problems are highly nonlinear equations has been taken into consideration here and this was the motive objective to determine numerical solutions. So, the emphasis is on the methodology adopted for obtaining numerical solutions that yielded after employing the Chebyshev spectral method. The temperature distributions and the velocity components are evaluated by solving numerically the boundary value problems that correspond to the proposed problem. Then, some figures have been plotted to elucidates the effect of different physical parameters appearing in the problem on both the temperature and the velocity profiles. The presence of the thermal radiation and the viscous dissipation in the fluid flow are shown to have quite a dramatic effect on the temperature profiles. In culmination, cooling process in nuclear reactors and geothermal engineering especially in the presence of thermal stratification phenomenon can be adopted as an application of this study. The theoretical and the observed results provide a fairly good qualitative agreement.
The control problems of nonlinear systems have received a great deal of attention, and a considerable amount of literatures have been published [1,2,3,4,5]. A two-layer neural networks (NNs) based robust control for nonlinear induction motors is proposed in [1]. In [2] the unknown nonlinear items in the dynamic model of robotic manipulators are identified by NNs. Dynamic properties and optimal stabilization issues of fractional-order (FO) self-sustained electromechanical seismograph system is investigated in [3]. A neural adaptive control scheme is raised for a class of uncertain multi-input/multi-output nonlinear systems [4] and only one learning parameter is updated in the parameter identification. And coupled FO chaotic electromechanical devices are studied in [5]. Specifically, an adaptive dynamic programming policy is proposed to address the zero-sum differential game issue in the optimal neural feedback controller. Obviously, approximation-based adaptive backstepping control has been widely utilized in designing controllers for various nonlinear systems [6,7,8]. However, traditional approximation-based adaptive backstepping technique faces two crucial issues that hinder its application. The first issue is so-called "EOC" arising from the derivations of virtual control input [7]. The second one is a large computational burden caused by high precision approximation requirements [8].
For the backstepping technique, the design of the control law relies on intermediate state variables as virtual control signals. The controller of each subsystem requires the virtual control signal and its derivative. The lower-order derivatives of the virtual control signals are likely simple in theory, but the higher-order derivatives in higher-order systems are quite complex, which is called the "EOC" problem. For handling the "EOC" problem, a first-order filter was used to calculate the virtual control signal derivatives in each recursive step [9]. This technique is the so-called dynamic surface control (DSC). The utilization of the first-order filter overcomes the EOC problem, but its own characteristics lead to the derivative errors of virtual control signal, which will certainly affect the tracking performance of the system. Based on this, a modified scheme of the DSC method named command filter based control (CFC) method was proposed in [10]. On the one hand, the EOC problem is avoided by substituting a first-order filter with a second-order one to obtain the derivatives of the virtual control signal. On the other hand, the filter errors are compensated by a constructed error compensation mechanism for obtaining better tracking performance of the system. Moreover, constructing an effective error compensation mechanism needs to be further studied.
For the approximation-based control, the NNs or fuzzy logic systems (FLSs) are utilized to approximate unknown functions and external disturbances for ensuring better tracking performance [11,12], which is also a kind of learning control [13]. In [11] a NNs-based approximator is utilized to solve the unmodeled dynamics of the system. FLSs are employed to identify unknown functions existing in systems [12]. Iterative learning control schemes are designed to suppress the vibrations in bending and twisting of the flexible micro aerial vehicle [13]. The approximation accuracy improves with the increase of the number of neural network nodes or fuzzy rules in general, but it also significantly increases the number of estimated parameters. Therefore, the burdens of computation required for online learning will become very heavy. For decreasing the computational burden of approximation-based control, a tuning-function is inserted in the controller of strict-feedback systems [14,15], in which the number of parameter to be updated is the same as the number of unknown parameters. Recently, a kind of one-parameter estimation approach is proposed in [16,17], which needs only one parameter to be updated online and can significantly reduce the computational burden. Nevertheless, the mentioned control schemes do not take issues of input constraints, state constraints and prescribed performance into account. Therefore, for applying to a broader range of control problems with security, reliability and performance consideration in reality, further research is needed.
In the real world, many physical constraints are generated with security and reliability consideration, such as the output of MEMS resonator [18], the state constraint of aircraft engine [19] and the input constraint of magnetic-field electromechanical transducer [20]. Obviously, severe security matters, performance degradation, and other troubles can be caused without considering these constraints. For the issue of input constraint, a considerable amount of literatures have been published about it [21,22,23]. A non-smooth and piecewise input constraint model is described in [21]. Furthermore, the model in [22] is a smooth but piecewise function. The input constraint nonlinearity is tackled by asymmetric smooth input constraint model in [23]. For the issues of output constraint and state constraint, Barrier Lyapunov Function (BLF) is seen as an effective tool, and a significant number of typical works have been published including symmetric BLF [24,25] and asymmetric BLF [26,27]. However, the aforementioned BLF-based controllers have the following three drawbacks: 1) Discontinuous actions exist when constructing asymmetric BLF deals with asymmetric constraints; 2) Output/state constraints are achieved indirectly through error constraint, which leads to a more conservative initial output and state; 3) It is not allowed to handle both constrained and unconstrained systems without changing the controller structure. Although the integral BLF (IBLF)-based approach is possible to tackle output/state constraints directly [28], it can only overcome the disadvantages 1) and 2). By constructing a novel state transformation nonlinear function in [29,30], all those shortcomings can be overcome simultaneously. However, in practical applications, ensuring system security and reliability is the foundation, and achieving high performance control of the system is surely the ultimate goal. To be the best of our knowledge, no relevant results have been reported which can overcome the all above drawbacks and ensure safety reliability and high performance of systems simultaneously.
In this paper, with consideration of security, reliability and high performance, a LAFN-PPC of strict-feedback systems considering asymmetric full-state and input constraints is raised. In the controller design procedure, the constrained system is transformed into an unconstrained system using one-to-one nonlinear state transformation technique. One-to-one nonlinear error transformation technique is used to guarantee the prescribed performance. Furthermore, the unknown control direction and the input constraint nonlinearity are resolved by Nussbaum gain technique simultaneously. By introducing command filter and an error compensation mechanism, the constructed scheme can not only overcomes the so-called "EOC" problem, but also reduces filter errors. Moreover, the maximum values of the norm of optimal weight vector in FWNN is constructed as a virtual parameter, and the only one virtual parameter is estimated instead of the optimal weight vectors (OWVs). Regardless of the order of the system and the dimension of the system parameters, only one parameter is required to be updated online, which significantly reduces the computational burdens. The major contributions comparing with the existing ones are listed as:
1) In order to ensure the controlled systems with higher security, faster response speed and lower tracking error simultaneously, we combine a simple state transformation function with an error transformation function. All states and tracking error are always in symmetric or asymmetric prescribed bounds. Compared with the BLF-based methods [24,25,26,27], the LAFN-PPC can overcome all the three drawbacks, because we utilize the state transformation function instead of BLF, by which the constrained system is converted to an unconstrained system. In contrast to state transformation based methods [29,30], the tracking error is always remained within the prescribed performance bound by using an error transformation function.
2) By using command filtering to get the virtual control signal derivatives, the "EOC" problem of traditional backstepping method is overcome, the filter error caused by command filter is compensated by the carefully constructed error compensation mechanism. Compared with [1,2,3,4,5], the method we take only requires the reference signal and its first derivative, which greatly reduces the amount of calculation and meets many practical engineering requirements.
3) To significantly improve the computational efficiency of FWNN-based approximator and to replace estimating the OWVs in each step of backstepping, we construct the maximum value of the norm of OWVs in the FWNN as a virtual parameter. Only one virtual parameter needs to be estimated in the FWNN-based approximator, with this one-parameter estimation-based approach, the number of parameters updated online is independent of the order of the system and the dimension of OWVs, and the computational burden is significantly reduced, while the computational efficiency is significantly improved.
The considered strict-feedback systems with input constraint nonlinearity are given as
{˙xi=fi(¯xi,ℓi)+xi+1,˙xn=fn(¯xn,ℓn)+gu,y=x1i=1,…,n−1u=C(v) | (2.1) |
where ¯xi=[x1,…,xi]T∈Ri, i=1,…,n and v∈R are the states and the system input. y∈R denotes the system output. x=[x1,…,xn]T∈Rn are the whole states of the system, fi(ˉxi,ℓi), i=1,…,n are unknown smooth functions, Specifically, fi(ˉxi,ℓi) denote system uncertainties and external disturbances, ℓi are the unknown constant parameters inseparable from fi(ˉxi,ℓi). g denotes the unknown control gain. Here, u is the actual control signal which is subjected to the input constraint nonlinearity C(v):R→R. And the input constraint nonlinearity will be given later. In this paper, xi(t) are constrained in the open sets (−κ_i,¯κi), i.e., xi(t)∈Ωi.
As is known that input saturation of actuator is a common problem. The input saturation nonlinearity can seriously affect the safety and performance of the system. How to cope with the saturation nonlinearity has become an urgent and challenging research issue. In this paper, we take the input constraint nonlinearity into account. Mostly, the input constraint nonlinearity [21] can be expressed as
u=C(v)={u−,vi<u−,vi,u−≤vi≤u+,u+,vi>u+, | (2.2) |
where u+ and u− are the upper/lower bounds of u(t).
To simplify the design of the control, we can define Δ(v)=u−cvv, where cv is a positive constant. We can rewrite the input constraint model as:
u=cvv+Δ(v). | (2.3) |
Based on the above strict-feedback systems, the control object of this paper is to design a LAFN-PPC for system (2.1) to realize the following purposes:
(a) All signals of the system are in the sense of uniformly ultimate boundedness.
(b) The input signal and full state variables can be strictly restricted in asymmetric upper and lower bounds.
(c) The output signal can track the reference signal very well. And the output tracking error can be strictly restricted in upper and lower bounds.
The FWNN [20] has strong power in function approximation, which consists of a series of fuzzy IF-THEN rules as:
If Z1 is Mj1 and … Zn is Mjn, then ˆfs is ωj, j=1,…Ns, where Mji, i=1,…,ns, j=1,…,Ns, is jth member function for ith input, ns and Ns represent the number of inputs and rulers (fuzzy logical system), respectively.
The FWNN shown in Figure 1 consists of five lawyers, including an input layer, a fuzzification layer, a membership layer, a rule layer, and an output layer. The firing degrees of rulers are defined as
⌢ξi=∑nj=1(1+(Zi−cji)2ωji)e−(Zi−cji)2ωji, |
ξ⌣i=∑nj=1(1−(Zi−cji)2ωji)e−(Zi−cji)2ωji, |
where i=1,…,N, j=1,…,n, n and N denote the number of inputs and rulers (neural network system). cji and ωji represent the center and width of member function.
The firing degrees are defined as
ξi=ˉξi+ξ−iN∑i=1(ˉξi+ξ−i),i=1,…,N. |
The FWNN can be described as
f(θ,Z)=θTξ(Z)+ε(Z) | (2.4) |
where f(θ,Z) is a continuous function which is bounded in closed compact set ℧→Rn, Z=[Z1,Z2,⋯,ZN]∈℧⊂Rn is the input vector, n is the input dimension of neural network. θ=[θ1,θ2,⋯θN]T∈RN denotes the weight vector, and N>1 is the node number of neuron. and ξ(Z)=[ξ1(Z),ξ2(Z),⋯,ξN(Z)]T∈RN indicates the basic function vector. ε is the estimation error. And there is a positive constant εMi which satisfies |εi|⩽εMi, i=1,…,n.
Lemma 1 [20]. Continuous function f(Z) is defined on a compact set ℧. And for ε(Z)>0, there is a FWNN satisfying
supx∈Ω|f(Z)−ˆf(Z,θ)|≤ε(Z). |
The optimal parameter ⌢θ is equal to argminθ∈℧θ[supZ∈℧|f(Z)−ˆf(Z,θ)|], where ℧θ is a compact set, and ˜θ=⌢θ−θ, where ⌢θ represents the estimation of θ.
For resolving physical constraints generated with security and reliability consideration, the following state transformation function [29,30] is introduced to achieve asymmetric constraints, symmetric constraints and no constraints on states simultaneously in a unified form:
si(t)=κ_iˉκixi(t)(κ_i+xi(t))(ˉκi−xi(t)) | (2.5) |
where κ_i and ˉκi are positive constants, the initial state xi(0)∈Ωi, i=1,2,…,n. It is obvious that if the states xi(t)→−κ_i or xi(t)→ˉκi, the transformed states si(t)→±∞. Therefore, the state transformation function can constrain xi(t) within the open sets (−κ_i,ˉκi), i.e., xi(t)∈Ωi.
Remark 1. If asymmetric constraints need to be addressed, i.e., let κ_i≠ˉκi, i=1,2,…,n. Else symmetric constraints need to be tackled, i.e., let κi=κ_i=ˉκi. Then (2.5) becomes
si(t)=κ2ixi(t)κ2i−x2i(t). | (2.6) |
If no constraints need to be handled, let κi→+∞. It is clear that si(t)→xi(t), i.e.,
limκi→+∞si(t)=xi(t). | (2.7) |
The above state transformation function can solve the control problems with asymmetric, symmetric and no state constraints in a unified form. Furthermore, it can handle above three kinds of control problems without changing adaptive laws. Figure 2 shows that the state transformation function can constrain xi(t) within the open set (−κ_i,ˉκi) (For the symmetric one: κ_i=ˉκi=4; For the asymmetric one: κ_i=4, ˉκi=6).
The state transformation function can be rewritten as
xi(t)=ηisi(t) | (2.8) |
where
ηi=(κ_i+xi(t))(ˉκi−xi(t))κ_iˉκi. |
Remark 2. ηi are also bounded in the sets Ωηi, i.e., 0<ηi⩽ˉηi, and ˉηi=(κ_i+ˉκi)24κ_iˉκi, i=1,2,…,n.
Proof. See Appendix A. Taking the time derivative of the state transformation function (2.5):
˙si(t)=ρi˙xi(t) | (2.9) |
where
ρi=κ_iˉκi(κ_iˉκi+x2i(t))(κ_i+xi(t))2(ˉκi−xi(t))2. |
The constrained system is converted to an unconstrained system as
{˙si=ρifi(¯xi,ℓi)+ρixi+1,˙sn=ρnfn(¯xn,ℓn)+ρngu,y=η1s1i=1,…,n−1u=C(v). | (2.10) |
For achieving the prescribed performance and guaranteeing the transformed output tracking error to converge within the prescribed performance bounds. Firstly, we define transformed output tracking error, virtual control errors and the command filters [10] as:
{z1=s1−α1cei=si−αici=2,3,…,n, | (2.11) |
{˙αjc=ωαjc,j˙αjc,j=−2ϖωαjc,j−ω(αjc−αj−1), | (2.12) |
where z1 is the transformed output tracking error, ei are intermediate tracking errors, αic are the outputs of the command filters, and the virtual controlαi are the inputs of the command filters. ω and ϖ∈(0,1) are positive design parameters. The initial values satisfy αjc(0)=αj−1(0) and αjc,j(0)=0 for j=2,3,…,n. α1c and its time derivative are calculated as:
{α1c=κ_1¯κ1yr(κ_1+yr)(¯κ1−yr)˙α1c=κ_1¯κ1(κ_1¯κ1+y2r)(κ_1+yr)2(¯κ1−yr)2˙yr=ρ1r˙yr | (2.13) |
where yr is the reference signal.
To ensure the transformed output tracking error z1 strictly converges in the prescribed performance region during the whole time, we define
−k−μ(t)<z1(t)<ˉkμ(t),∀t⩾0, | (2.14) |
where the design parameters k− and ˉk are positive constants, and
μ(t)=(μ0−μ∞)e−ℏt+μ∞ |
is the prescribed performance function, μ(0)=μ0, 0<μ∞<μ0. The parameter ℏ is also a positive constant.
Remark 3. It is obvious that the transformed output tracking error is the output tracking error of unconstrained system and is restricted in the prescribed domain, i.e. z1(t)∈(−k−μ(t),ˉkμ(t)). However, our control objective is that the output tracking error of original constrained system er=x1−yr is restricted in a prescribed domain. The output tracking error is bounded in a set
er∈(−k−μ(t)(κ_1+ˉκ1)2/κ_1ˉκ1,ˉkμ(t)(κ_1+ˉκ1)2/κ_1ˉκ1). |
Proof. See Appendix B. The error transformation can be defined as:
z1(t)=μ(t)Υ(Z1(t)),∀t≥0 | (2.15) |
where Z1(t) is the transformed error and Υ(Z1) is defined as:
Υ(Z1)=¯κeZ1−κ_e−Z1eZ1+e−Z1. | (2.16) |
Remark 4. It is obvious that Υ(t) is strictly constrained in symmetric or asymmetric domain (−k−,ˉk) showed in Figure 3 (For the symmetric one: k−=ˉk=0.5. For the asymmetric one: k−=0.5, ˉk=0.7). It means that the error transformation function can deal with the issues considering symmetric or asymmetric exponential constraint simultaneously.
According to (2.15) and (2.16), one can obtain:
Z1(t)=Υ−1(z1(t)μ(t))=12lnΥ+κ_¯κ−Υ | (2.17) |
and
˙Z1(t)=ρ(˙z1(t)−˙μ(t)z1(t)μ(t)) | (2.18) |
where
ρ=[(1(Υ(Z1)+κ_))+(1(¯κ−Υ(Z1)))]2μ(t). |
We finally define the transformed tracking error as
e1=Z1(t). | (2.19) |
In order to reduce the errors caused by the command filters, we introduce compensation signals ςi, and the compensation errors can be defined as vi=ei−ςi, i=1,…,n.
FWNN is used to approximate the system uncertainties and external disturbances fi:
fi=θTiξi(Z)+εi(Z). | (3.1) |
For facilitating adaptive law design, we define
{ˉθi=max{‖θi‖2,ε2Mi}θ=max{ˉθ1,…,ˉθn},i=1,…,n, | (3.2) |
where ˉθi are unknown virtual parameters with ˉθi<θ. θi is the ideal constant weight of ith NN and εMi is the upper bound of approximation error εi.
Assumption 1. The reference trajectory yr is continuous, and satisfies
[yr,˙yr,¨yr]T∈Ξr, |
Ξr is a known compact set
{[yr,˙yr,¨yr]T:y2d+˙y2d+¨y2d≤Br}⊂R3. |
Br is a known positive constant. Furthermore, −κ−1<yr<ˉκ1 holds.
Assumption 2. There are some constants ˉΔ, g− and ˉg which satisfied Δ(v)⩽ˉΔ and g−⩽|g|⩽ˉg.
For solving the unknown gain and input constraint of systems simultaneously, Nussbaum function is introduced [10]. Obviously, a Nussbaum-type function N(χ) needs to hold following the properties:
{lims→+∞sup1s∫s0N(χ)dχ=+∞lims→+∞inf1s∫s0N(χ)dχ=−∞. | (3.3) |
In general, ln(χ+1)cos√ln(χ+1), χ2cos(χ) and eχ2cos(πχ/2) are commonly used Nussbaum-type function, and N(χ)=χ2cos(χ) is employed in this paper.
Lemma 2 [10]. Let V(⋅) and χ(⋅) be smooth functions defined on [0,tf) with V(t)⩾0. ∀t∈[0,tf), and N(⋅) is a smooth Nussbaum-type function. If the following inequality holds:
V(t)≤c0+∫t0(gfN(χ)+1)˙χdτ | (3.4) |
where gf is a non-zero parameter and c0 is an appropriate constant, then V(t), χ(t) and ∫t0(gN(χ)+1)˙χdτ must be bounded on [0,tf).
Step 1. Taking the time derivative of e1 based on (2.17)–(2.19), it has:
˙e1=ρ(ρ1x2+ρ1f1−ρ1r˙yr−˙μ(t)z1(t)μ(t)). | (3.5) |
The error compensation signal is constructed as
˙ς1=−k1ς1+ρρ1η2(ς2+α2c−α1). | (3.6) |
Choosing a Lyapunov function and taking its time derivative, one can obtain
{V1=12v21+12γ˜θ2˙V1=v1(ρ(ρ1η2(e2+α2c)+ρ1f1−ρ1r˙yr−˙μ(t)z1(t)μ(t))−˙ς1)+1γ˜θ˙⌢θ | (3.7) |
where the compensation error v1=e1−ς1.
Using a FWNN to approximate the unknown item f1, one can obtain:
˙V1=v1(ρ(ρ1η2(e2+α2c)+ρ1(θT1ξ1+ε1)−ρ1r˙yr−˙μ(t)z1(t)μ(t))−˙ς1)+1γ˜θ˙⌢θ. | (3.8) |
By the Young's inequality, it has
{ρρ1v1θT1ξ1⩽ρ2ρ21v21‖θ1‖2‖ξ1‖2+14ρρ1v1ε1⩽ρ2ρ21v21ε2M1+14. | (3.9) |
Therefore, we have
ρρ1v1(θT1ξ1+ε1)⩽ˉθ1v21ζ1+12⩽θv21ζ1+12, | (3.10) |
with
ˉθ1=max{‖θ1‖2,ε2M1}, | (3.11) |
ζ1=ρ2ρ21‖ξ1‖2+ρ2ρ21>0. | (3.12) |
Hence, it has
˙V1≤v1(ρ(ρ1η2(e2+α2c)−ρ1r˙yr−˙μ(t)z1(t)μ(t))−˙ς1)+θv21ζ1+12+1γ˜θ˙⌢θ. | (3.13) |
By substituting compensation signal ˙ς1 from (3.6) into (3.13), it has
˙V1≤v1(ρρ1η2v2−ρρ1r˙yr−ρ˙μ(t)z1(t)μ(t)+k1ς1+ρρ1η2α1)+θv21ζ1+12+1γ˜θ˙⌢θ. | (3.14) |
Then, the virtual control law α1 is designed as the following
α1=(−k1e1−v1⌢θζ1+ρρ1r˙yr+ρ˙μ(t)z1(t)μ(t))ρ−1ρ−11η−12. | (3.15) |
Substituting the virtual control α1 into (3.14) results in
˙V1≤−k1v21+v1(ρρ1η2v2−v1⌢θζ1)+θv21ζ1+12+1γ˜θ˙⌢θ. | (3.16) |
The adaptive law is given as
˙⌢θ=γ∑nk=1v2kζk−σ⌢θ. | (3.17) |
By integrating (3.16) and (3.17), we have
˙V1≤−k1v21+v1ρρ1η2v2+12+˜θ∑nk=2v2kζk−σγ˜θ⌢θ. | (3.18) |
Note that
−σγ˜θ⌢θ≤−σ2γ˜θ2+σ2γθ2. | (3.19) |
One has
˙V1≤−k1v21−σ2γ˜θ2+v1ρρ1η2v2+˜θ∑nk=2v2kζk+σ2γθ2+12. | (3.20) |
Step 2. Taking the time derivative of e2, it has:
˙e2=ρ2˙x2−˙α2c=ρ2x3+ρ2f2−˙α2c. | (3.21) |
The error compensation signal is constructed as
˙ς2=−k2ς2−ρρ1η2ς1+ρ2η3(ς3+α3c−α2). | (3.22) |
Choosing a Lyapunov function and taking its time derivative, one can obtain
{V2=V1+12v22˙V2=˙V1+v2(ρ2η3(e3+α3c)+ρ2f2−˙α2c−˙ς2) | (3.23) |
where the compensation error v2=e2−ς2.
By integrating compensation signal ˙ς2 and (3.23), it has
˙V2≤−k1v21−σ2γ˜θ2+v2(ρ2η3v3+ρ2f2+ρρ1η2e1−˙α2c+k2ς2+ρ2η3α2)+˜θ∑nk=2v2kζk+σ2γθ2+12. | (3.24) |
Using a FWNN to approximate the unknown item f2, one can obtain:
˙V2≤−k1v21−σ2γ˜θ2+˜θn∑k=2v2kζk+σ2γθ2+12+v2(ρ2η3v3+ρ2(θT2ξ2+ε2)+ρρ1η2e1−˙α2c+k2ς2+ρ2η3α2). | (3.25) |
By the Young's inequality, it has
{ρ2v2θT2ξ2⩽ρ22v22‖θ2‖2‖ξ2‖2+14ρ2v2ε2⩽ρ22v22ε2M2+14. | (3.26) |
Therefore, we have
ρ2v2(θT2ξ2+ε2)⩽ˉθ2v22ζ2+12⩽θv22ζ2+12, | (3.27) |
with
ˉθ2=max{‖θ2‖2,ε2M2}, | (3.28) |
ζ2=ρ22‖ξ2‖2+ρ22>0. | (3.29) |
Hence, it has
˙V2≤−k1v21−σ2γ˜θ2+˜θn∑k=2v2kζk+σ2γθ2+12+v2(ρ2η3v3+ρρ1η2e1−˙α2c+k2ς2+ρ2η3α2)+θv22ζ2+12. | (3.30) |
Then, the virtual control law α2 is designed as the following
α2=(−k2e2−v2⌢θζ2−ρρ1η2e1+˙α2c)ρ−12η−13. | (3.31) |
Substituting the virtual control α2 into (3.30) results in
˙V2≤−k1v21−k2v22−σ2γ˜θ2+v2ρ2η3v3+˜θ∑nk=3v2kζk+σ2γθ2+22. | (3.32) |
Step i. Taking the time derivative of ei, it has:
˙ei=ρi˙xi−˙αic=ρixi+1−˙αic. | (3.33) |
The error compensation signal is constructed as
˙ςi=−kiςi−ρi−1ηiςi−1+ρiηi+1(ςi+1+αi+1c−αi). | (3.34) |
Choosing a Lyapunov function and taking its time derivative, one can obtain
{Vi=Vi−1+12v2i˙Vi=˙Vi−1+vi(ρiηi+1(ei+1+αi+1c)+ρifi−˙αic−˙ςi) | (3.35) |
where the compensation error vi=ei−ςi.
By integrating compensation signal ˙ςi and (3.35), it has
˙Vi≤−i−1∑k=1kkv2k−σ2γ˜θ2+˜θn∑k=iv2kζk+σ2γθ2+i−12+vi(ρiηi+1vi+1+ρifi−˙αic+kiςi+ρi−1ηiei−1+ρiηi+1αi). | (3.36) |
Using a FWNN to approximate the unknown item fi, one can obtain:
˙Vi≤−i−1∑k=1kkv2k−σ2γ˜θ2+˜θn∑k=iv2kζk+σ2γθ2+i−12+vi(ρiηi+1vi+1+ρi(θTiξi+εi)−˙αic+kiςi+ρi−1ηiei−1+ρiηi+1αi). | (3.37) |
By the Young's inequality, it has
{ρiviθTiξi⩽ρ2iv2i‖θi‖2‖ξi‖2+14ρiviεi⩽ρ2iv2iε2Mi+14. | (3.38) |
Therefore, we have
ρivi(θTiξi+εi)⩽ˉθiv2iζi+12⩽θv2iζi+12, | (3.39) |
with
ˉθi=max{‖θi‖2,ε2Mi}, | (3.40) |
ζi=ρ2i‖ξi‖2+ρ2i>0. | (3.41) |
Hence, it has
˙Vi⩽−i−1∑k=1kkv2k−σ2γ˜θ2+˜θn∑k=iv2kζk+σ2γθ2+i−12+vi(ρiηi+1vi+1−˙αic+kiςi+ρi−1ηiei−1+ρiηi+1αi)+θv2iζi+12. | (3.42) |
Then, the virtual control law αi is designed as the following
αi=(−kiei−vi⌢θζi−ρi−1ηiei−1+˙αic)ρ−1iη−1i+1. | (3.43) |
Substituting the virtual control αi into (3.42) results in
˙Vi≤−∑ik=1kkv2k−σ2γ˜θ2+˜θ∑nk=i+1v2kζk+σ2γθ2+i2+viρiηi+1vi+1. | (3.44) |
Step n. Taking the time derivative of en, it has:
˙en=ρn˙xn−˙αnc=ρngu+ρnfn−˙αnc. | (3.45) |
The error compensation signal is constructed as
˙ςn=−knςn−ρn−1ηnςn−1. | (3.46) |
Choosing a Lyapunov function and taking its time derivative, one can obtain
{Vn=Vn−1+12v2n˙Vn=˙Vn−1+vn(ρngu+ρnfn−˙αnc−˙ςn) | (3.47) |
where the compensation error vn=en−ςn.
By integrating compensation signal ˙ςn and (3.47), it has
˙Vn=˙Vn−1+vn(ρngu+ρnfn−˙αnc+knςn+ρn−1ηnςn−1). | (3.48) |
Using a FWNN to approximate the unknown item fn, one can obtain:
˙Vn=˙Vn−1+vn(ρngu+ρn(θTnξn+εn)−˙αnc+knςn+ρn−1ηnςn−1). | (3.49) |
By the Young's inequality, it has
{ρnvnθTnξn⩽ρ2nv2n‖θn‖2‖ξn‖2+14ρnvnεn⩽ρ2nv2nε2Mn+14. | (3.50) |
Therefore, we have
ρnvn(θTnξn+εn)⩽ˉθnv2nζn+12⩽θv2nζn+12 | (3.51) |
with
ˉθn=max{‖θn‖2,ε2Mn}, | (3.52) |
ζn=ρ2n‖ξn‖2+ρ2n>0. | (3.53) |
Hence, it has
˙Vn≤˙Vn−1+vn(ρngcvv+12ρ2nvn−˙αnc+knςn+ρn−1ηnςn−1)+θv2nζn+12+12¯g2¯Δ2. | (3.54) |
Then, the control input v is designed as the following
v=N(χ)ψρ−1n, | (3.55) |
ψ=knen+vn⌢θζn+ρn−1ηnen−1−˙αnc+12ρ2nvn, | (3.56) |
˙χ=vnψ. | (3.57) |
Substituting the virtual control v into (3.54) results in
˙Vn≤−∑nk=1kkv2k+gcvN(χ)˙χ+˙χ−σ2γ˜θ2+σ2γθ2+12¯g2¯Δ2+n2. | (3.58) |
Up to now, the whole construction of LAFN-PPC is completed.
For any given positive constant p, consider a closed set
{Θ1={(v1,⌢θ):v21+1γ˜θ2≤2p}Θ2={(v1,v2,⌢θ):∑2k=1v2k+1γ˜θ2≤2p}Θi={(v1,v2,…,vi,⌢θ):∑ik=1v2k+1γ˜θ2≤2p}Θn={(v1,v2,…,vn,⌢θ):∑nk=1v2k+1γ˜θ2≤2p}. | (4.1) |
Theorem 1. For the strict-feedback systems (2.1) with full-state and input constraints under Assumptions 1 and 2, the controllers (3.15), (3.31), (3.43) and (3.55)–(3.57), adaptive law (3.17) and compensation signal (3.6), (3.22), (3.34) and (3.46) are constructed. If initial conditions satisfy Θi, i=1,2,…,n, xi(0)∈(−κ_i,ˉκi), i=1,2,…,n, and yr(0)∈(−κ_1,ˉκ1), then the proposed control scheme ensures the achievement of objectives (a)–(c).
Proof. The Lyapunov function choosing as
V=12∑nk=1v2k+12γ˜θ2. | (4.2) |
From (3.58), we have
˙V≤−∑nk=1kkv2k+gcvN(χ)˙χ+˙χ−σ2γ˜θ2+σ2γθ2+12¯g2¯Δ2+n2. | (4.3) |
To facilitate analysis, the above inequality can be written as
˙V≤−AV+B+(gcvN(χ)+1)˙χ, | (4.4) |
where
A⩽min{2k1,…,2kn,σ}, |
B=σ2γθ2+12ˉg2ˉΔ2+n2. |
By computing the integration of the above differentiation inequality at the interval [0,t), one can obtain
0≤V≤V(0)e−At+BA(1−e−At)+e−At∫t0(gcvN(χ)+1)˙χeAτdτ. | (4.5) |
From Lemma 2, we can get that V, χ and ∫t0(gcvN(χ)+1)˙χdτ are bounded at the interval [0,t). And the result holds even if t→∞. Furthermore, let C be the upper bound of e−At∫t0(gcvN(χ)+1)˙χeAτdτ. The following inequality will holds
V≤(V(0)−BA)e−At+BA+C. | (4.6) |
We denote F∞ as the set of all bounded functions. According to the above analysis, we can get V⩽p. Therefore, we can obtain that v1, v2, ⋯, vn, ⌢θ, ς1, ς2, ⋯, ςn are bounded. Furthermore, ei are also bounded due to vi=ei−ςi. As e1=Z1(t) and e1∈F∞ it has Z1(t)∈F∞. It implies z1(t),ρ∈F∞ and s1=z1+α1c∈F∞. Noting (2.9), we obtain ρ1∈F∞. From (2.18), it yields ρ,μ1(t),˙μ1(t)∈F∞. With the help of (3.15) and (2.12), we have α1∈F∞ and α2c,˙α2c∈F∞. Since s2=v2+α2c+ς2, we get s2∈F∞. Noting (2.9), we obtain ρ2∈F∞. From (3.31) and (2.12), we get α2∈F∞ and α3c,˙α3c∈F∞. Similarly, we can easily get
s3,ρ3,α4c,˙α4c,⋯,sn−1,ρn−1,αnc,˙αnc,sn,ρn,v∈F∞. |
From (2.8), it yields x1,x2,⋯,xn∈F∞. This finishes the proof.
Remark 5. In the proposed low-cost adaptive neural prescribed performance control (LAFN-PPC) scheme, we can obtain a satisfactory performance by reasonably adjusting ki, γ, σ, ˉκi, κ_i, ˉk, k−, μ0, μ∞ and ℏ, where i=1,2,…,n. The larger ki and smallerγ, σ can improve the convergence speed and tracking accuracy of the controller. But too large ki and small γ, σ values can result in large control input, which may be far beyond physical limitations of actuator. The adaptive parameters γ and σ play the part of regulators between controller and control output. To avoid the large control input, the values of the parameters ki, γ, σ, i=1,2,…,n are limited to a certain interval. Meanwhile, ˉκi and κ_i are used to constrain the states xi(t) within the open sets (−κ_i,ˉκi), which can be selected according to the actual application requirements. From this it can be concluded that the time varying parameter
ρi=(κ_iˉκi(κ_iˉκi+x2i(t)))/((κ_i+xi(t))2(ˉκi−xi(t))2). |
The parameters ˉk, k−, μ0, μ∞ and ℏ are designed to constrain the transformed output tracking error within the open set (−k−μ(t),ˉkμ(t)), where
μ(t)=(μ0−μ∞)e−ℏt+μ∞. |
μ0, ℏ and μ∞ determine the initial error, the error convergence rate and the steady-state error of the transformed output tracking error bound, which can be selected according to the performance requirements. ˉk and k− are always within (0,1] and can deal with the issues considering symmetric or asymmetric transformed output tracking error constraint.
In order to prove the effectiveness and feasibility of our control scheme, this section provides comparison simulation cases. Meanwhile, the control schemes based on works in [29,30] are compared with our suggested control scheme.
A rigid manipulator system [30] is given as
{JL¨θ+MgvLsinθ+TE=uu=H(v) | (5.1) |
where θ, ˙θ and ¨θ are the position, velocity and acceleration of the link, respectively. M, gv and L denote the link mass, gravity constant and the distance from the joint to the mass center of the link, respectively. TE indicates the unknown external load. u is the actual control torque which is subjected to the unknown input constraint nonlinearity H(v), and v is system control input signal.
To facilitate the controller design, we transfer the system (5.1) with new variables. Let x1=θ, x2=˙θ. Then the dynamic model of system (5.1) can be expressed as follows:
{˙x1=x2˙x2=J−1L(u−MgLsinx1−TE)u=H(v)y=x1. | (5.2) |
To simplify the expression, the dynamic model is rewritten as
{˙x1=x2˙x2=GLu+fLu=H(v)=cvv+Δ(v)y=x1 | (5.3) |
where
fL=−MgLsinx1−TEJL,GL=J−1L. |
In the simulation, we set f1(x1)=0, f2(x1,x2)=fL and g=GL. M=0.35Kg, gv = 9.8 m/s2, L=1.47m and TE=0.1sint. The tracking signal of following cases are set as yr=0.5sint.
Case 1. The initial system states and output are x(0)=[0.1,0]. And ⌢θ(0)=αjc(0)=αjc,j(0)=0, ς1(0)=ς2(0)=0. The proposed controller, adaptive laws and error compensation schemes are constructed as
{α1=(−k1e1+ρρ1r˙yr+ρ˙μ(t)z1(t)μ(t))ρ−1ρ−11η−12v=N(χ)ψρ−12ψ=k2e2+v2⌢θζ+ρρ1η2e1−˙αnc+12ρ22v2˙χ=v2ψ, | (5.4) |
\left\{\begin{array}{l}{\dot{\varsigma }}_{1} = -{k}_{1}{\varsigma }_{1}+\rho {\rho }_{1}{\eta }_{2}\left({\varsigma }_{2}+{\alpha }_{2c}-{\alpha }_{1}\right)\\ {\dot{\varsigma }}_{2} = -{k}_{2}{\varsigma }_{2}-\rho {\rho }_{1}{\eta }_{2}{\varsigma }_{1}\end{array}\right. , | (5.5) |
\dot{{\overset\frown{\theta }}} = \gamma {v}_{2}^{2}{\zeta }_{2}-\sigma \overset\frown{\theta }, | (5.6) |
where the parameters are chosen as {k_1} = 50, {k_2} = 15, \gamma = 10, \sigma = 0.7, \omega = 300, \varpi = 0.95. The parameters of FWNN are chosen as: The nodes of neural network is 7, the center c_1^j is distributed in the field of \left[{- 3, 3} \right], and its width \omega _1^j = 1. The parameters of full-state constraints, input constraint and prescribed performance constraint are set as: {\bar \kappa _1} = 1.5, {\underset{\raise0.3em\hbox{$\smash{\scriptscriptstyle-}$}}{\kappa } _1} = 1.5, {\underset{\raise0.3em\hbox{$\smash{\scriptscriptstyle-}$}}{\kappa } _2} = 3, {\bar \kappa _2} = 4, {u^ + } = 30, {u^ - } = - 30, {\mu _0} = 0.2, {\mu _\infty } = 0.02, \hbar = 1, \underset{\raise0.3em\hbox{$\smash{\scriptscriptstyle-}$}}{k} = 1, \bar k = 1.
Case 2. Base on the case 1, we further study the output tracking errors when give different prescribed performance. {e_{r1}} for {\mu _\infty } = 0.02, {e_{r2}} for {\mu _\infty } = 0.016, {e_{r3}} for {\mu _\infty } = 0.012, {e_{r4}} for {\mu _\infty } = 0.008, {e_{r5}} for {\mu _\infty } = 0.004, {e_{r6}} for {\mu _\infty } = 0.002.
Case 3. Based on the case 2, we set {\mu _\infty } = 0.004 and {y_d} = {y_r}, two comparative simulation from [29,30] are carried out to further show the advantage of our control scheme, which is still based on the rigid manipulator system (5.3). The controller, adaptive laws and first-order filter of work in [29] are given as
\left\{\begin{array}{l}{\alpha }_{1} = -{c}_{1}\frac{{{\underline{F } }}_{1}{{\overline {F} }}_{1}\left({{\underline{F } }}_{1}{{\overline {F} }}_{1}-{x}_{1}^{2}\right)}{{\left({{\underline{F } }}_{1}+{x}_{1}\right)}^{2}{\left({{\overline {F} }}_{1}-{x}_{1}\right)}^{2}}{z}_{1}+\frac{{{\underline{F } }}_{1}{{\overline {F} }}_{1}\left({{\underline{F } }}_{1}{{\overline {F} }}_{1}-{y}_{d}^{2}\right)}{{\left({{\underline{F } }}_{1}+{y}_{d}\right)}^{2}{\left({{\overline {F} }}_{1}-{y}_{d}\right)}^{2}}{\dot{y}}_{d}\\ u = -\left({c}_{2}+\widehat{\theta }{\mathit{\Phi}} \right)\frac{{{\underline{F } }}_{2}{{\overline {F} }}_{2}\left({{\underline{F } }}_{2}{{\overline {F} }}_{2}-{x}_{2}^{2}\right)}{{\left({{\underline{F } }}_{2}+{x}_{2}\right)}^{2}{\left({{\overline {F} }}_{2}-{x}_{2}\right)}^{2}}{z}_{2}\\ {\mathit{\Phi}} = {‖\frac{{{\underline{F } }}_{1}{{\overline {F} }}_{1}\left({{\underline{F } }}_{1}{{\overline {F} }}_{1}-{x}_{1}^{2}\right)}{{\left({{\underline{F } }}_{1}+{x}_{1}\right)}^{2}{\left({{\overline {F} }}_{1}-{x}_{1}\right)}^{2}}‖}^{2}\left(1+{\phi }^{2}\right)+{‖{\dot{\alpha }}_{2f}‖}^{2}\end{array}\right. , | (5.7) |
\dot{\widehat{\theta }} = \gamma {‖{z}_{2}‖}^{2}{\mathit{\Phi}} -\sigma \widehat{\theta , } | (5.8) |
\varepsilon {\dot{\alpha }}_{2f}+{\alpha }_{2f} = \frac{{{\underline{F } }}_{2}{{\overline {F} }}_{2}}{\left({{\underline{F } }}_{2}+{x}_{2}\right)\left({{\overline {F} }}_{2}-{x}_{2}\right)}{\alpha }_{1}, | (5.9) |
where {z_1} = {x_1} - {y_d}, {z_2} = {x_2} - {\alpha _{2f}}, \theta \left(0 \right) = 0, {\alpha _{2f}}\left(0 \right) = 0, {c_1} = 40, {c_2} = 80, {\underset{\raise0.3em\hbox{$\smash{\scriptscriptstyle-}$}}{F} _1} = 1.5, {\bar F_1} = 1.5, {\underset{\raise0.3em\hbox{$\smash{\scriptscriptstyle-}$}}{F} _2} = 3, {\bar F_2} = 4, \gamma = 15, \sigma = 0.5, \varphi = 5 and \varepsilon = 0.03. Detailed controller design and parameter meaning is found in [29].
The controller, adaptive laws and first-order filter of works in [30]. are given as
\left\{\begin{array}{l}{\alpha }_{1} = -{\left(\frac{{{\underline{ \kappa } }}_{1}{{\overline {\kappa} }}_{1}\left({{\underline{ \kappa } }}_{1}{{\overline {\kappa} }}_{1}+{x}_{1}^{2}\right)}{{\left({{\underline{ \kappa } }}_{1}+{x}_{1}\right)}^{2}{\left({{\overline {\kappa} }}_{1}-{x}_{1}\right)}^{2}}\right)}^{-1}\left({k}_{1}{z}_{1}-\frac{{{\underline{ \kappa } }}_{1}{{\overline {\kappa} }}_{1}\left({{\underline{ \kappa } }}_{1}{{\overline {\kappa} }}_{1}+{y}_{d}^{2}\right)}{{\left({{\underline{ \kappa } }}_{1}+{y}_{d}\right)}^{2}{\left({{\overline {\kappa} }}_{1}-{y}_{d}\right)}^{2}}{\dot{y}}_{d}\right)\\ \mu = N\left({\zeta }_{2}\right){v}_{2}\\ {v}_{2} = {\left(\frac{{{\underline{ \kappa } }}_{2}{{\overline {\kappa} }}_{2}\left({{\underline{ \kappa } }}_{2}{{\overline {\kappa} }}_{2}+{x}_{2}^{2}\right)}{{\left({{\underline{ \kappa } }}_{2}+{x}_{2}\right)}^{2}{\left({{\overline {\kappa} }}_{2}-{x}_{2}\right)}^{2}}\right)}^{-1}\left({k}_{2}{z}_{2}+{z}_{2}{\widehat{\theta }}^{T}\varphi \left(Z\right)-{\dot{\psi }}_{2}\right)\\ {\dot{\zeta }}_{2} = \frac{{{\underline{ \kappa } }}_{2}{{\overline {\kappa} }}_{2}\left({{\underline{ \kappa } }}_{2}{{\overline {\kappa} }}_{2}+{x}_{2}^{2}\right)}{{\left({{\underline{ \kappa } }}_{2}+{x}_{2}\right)}^{2}{\left({{\overline {\kappa} }}_{2}-{x}_{2}\right)}^{2}}{z}_{2}{v}_{2}\end{array}\right. , | (5.10) |
\dot{\widehat{\theta }} = \Gamma \left(\varphi \left(Z\right){z}_{2}^{2}-\sigma \widehat{\theta }\right), | (5.11) |
\delta {\dot{\psi }}_{2}+{\psi }_{2} = \frac{{{\underline{ \kappa } }}_{2}{{\overline {\kappa} }}_{2}}{\left({{\underline{ \kappa } }}_{2}+{x}_{2}\right)\left({{\overline {\kappa} }}_{2}-{x}_{2}\right)}{\alpha }_{1}, | (5.12) |
where {z_1} = {x_1} - {y_d}, {z_2} = {x_2} - {\psi _2}, {\zeta _2}\left(0 \right) = 0, {\psi _2}\left(0 \right) = 0, {k_1} = 50, {k_2} = 30, {\underset{\raise0.3em\hbox{$\smash{\scriptscriptstyle-}$}}{k} _1} = 1.5, {\bar k_1} = 1.5, {\underset{\raise0.3em\hbox{$\smash{\scriptscriptstyle-}$}}{k} _2} = 3, {\bar k_2} = 4, \Gamma = 15 and \sigma = 0.5.\phi \left(Z \right) is the activation function of neural network, Z is the input of neural network. The parameters of RBF are chosen as: The nodes of neural network is 7, the center {\mu _1} is distributed in the field of \left[{- 0.3, 0.3} \right], and its width {\sigma _1} = 0.1. Detailed controller design and parameter meaning is found in [30].
Figures 4–7 reflect the main results of our control scheme. Figure 4 shows that output y of rigid manipulator system can track desired trajectory well without violating the output constraint. Figure 5 reveals the output tracking error {e_r} is in the region boundary all the times. The actual controls uis showed in Figure 6. And the state {x_2} is illustrated in Figure 7. From Figure 8, the proposed control scheme tracks the desired signal well with different prescribed performance, and the boundedness of prescribed performance is not violated.
Figures 9–11 present the comparative results of tracking trajectories, output tracking errors and input signals. We can easily find that the results of LAFN-PPC is better than controllers in [29,30]. Hence, for strict-feedback systems with purpose of high-precision tracking performance, full-state constraints and input constraint, we can conclude that our suggested control scheme has a potential to control them.
The LAFN-PPC is newly constructed for strict-feedback systems with prescribed output performance, full-state constraints and input constraint. The newly constructed command filter based adaptive control scheme with an error compensation mechanism can not only overcome the so-called "EOC" problem, but also reduce filter errors. By introducing a one-to-one nonlinear state transformation function, the full-state constraints are resolved. The prescribed performance can be guaranteed by using the one-to-one nonlinear error transformation function. The unknown control direction and the input constraint nonlinearity are resolved by introducing the Nussbaum function simultaneously. Moreover, the large computational cost is solved by introducing a virtual parameter of adaptive laws. Only one parameter needs to be updated online. Future works can focus on command-filter based optimal control of nonstrict-feedback systems considering performance constraint and address how to minimize resources consumption while ensuring performance.
Proof. According to the state transformation function (2.8), we have
{\eta _i} = \frac{{ - x_i^2 \;+ \;\left( {{{\bar \kappa }_i} - {{\underline{\kappa }}_{i}}} \right){x_i} + {{\underline{\kappa }}_{i}}\;{{\bar \kappa }_i}}}{{{{\underline{\kappa }}_{i}}\;{{\bar \kappa }_i}}}. | (A.1) |
{x_i} are always constrained in the open sets \left({ - {{\underline{\kappa }}_{i}}, {{\bar \kappa }_i}} \right), i.e., {x_i}\left(t \right) \in {{{\Omega}} _i}. Therefore, we can easily obtain that {\eta _i} > 0 and {\eta _i}take the maximal value at the points x_i^0 = {{\left({{{\bar \kappa }_i} - {{\underline{\kappa }}_{i}}} \right)} /2} according to the properties of the quadratic function. It is obvious that x_i^0 is in the interval \left({ - {{\underline{\kappa }}_{i}}, {{\bar \kappa }_i}} \right). Thus, the maximal value of {\eta _i} is
{\bar \eta _i} = \frac{{{{\left( {{{\bar \kappa }_i} + {{\underline{\kappa }}_{i}}} \right)}^2}}}{{4{{\underline{\kappa }}_{i}}{{\bar \kappa }_i}}}, i = 1, 2, \cdots , n. | (A.2) |
This finishes the proof.
Proof. According to (2.5), (2.11), (2.13) and (2.14), we have
\begin{gathered} {s_1} - {\alpha _{1c}} = \frac{{{{\underline{\kappa }}_{1}}{{\bar \kappa }_1}{x_1}}}{{\left( {{{\underline{\kappa }}_{1}} + {x_1}} \right)\left( {{{\bar \kappa }_1} - {x_1}} \right)}} - \frac{{{{\underline{\kappa }}_{1}}{{\bar \kappa }_1}{y_r}}}{{\left( {{{\underline{\kappa }}_{1}} + {y_r}} \right)\left( {{{\bar \kappa }_1} - {y_r}} \right)}} \hfill \\ = \left( { - \frac{{\underset{\raise0.3em\hbox{$\smash{\scriptscriptstyle-}$}}{\kappa } _1^2{{\bar \kappa }_1}}}{{{{\underline{\kappa }}_{1}} + {{\bar \kappa }_1}}}\frac{1}{{{{\underline{\kappa }}_{1}} + {x_1}}} + \frac{{{{\underline{\kappa }}_{1}}\bar \kappa _1^2}}{{{{\underline{\kappa }}_{1}} + {{\bar \kappa }_1}}}\frac{1}{{{{\bar \kappa }_1} - {x_1}}}} \right) - \left( { - \frac{{\underset{\raise0.3em\hbox{$\smash{\scriptscriptstyle-}$}}{\kappa } _1^2{{\bar \kappa }_1}}}{{{{\underline{\kappa }}_{1}} + {{\bar \kappa }_1}}}\frac{1}{{{{\underline{\kappa }}_{1}} + {y_r}}} + \frac{{{{\underline{\kappa }}_{1}}\bar \kappa _1^2}}{{{{\underline{\kappa }}_{1}} + {{\bar \kappa }_1}}}\frac{1}{{{{\bar \kappa }_1} - {y_r}}}} \right) \hfill \\ = \frac{{\underset{\raise0.3em\hbox{$\smash{\scriptscriptstyle-}$}}{\kappa } _1^2{{\bar \kappa }_1}}}{{{{\underline{\kappa }}_{1}} + {{\bar \kappa }_1}}}\left( {\frac{1}{{{{\underline{\kappa }}_{1}} + {y_r}}} - \frac{1}{{{{\underline{\kappa }}_{1}} + {x_1}}}} \right) + \frac{{{{\underline{\kappa }}_{1}}\bar \kappa _1^2}}{{{{\underline{\kappa }}_{1}} + {{\bar \kappa }_1}}}\left( {\frac{1}{{{{\bar \kappa }_1} - {x_1}}} - \frac{1}{{{{\bar \kappa }_1} - {y_r}}}} \right) \hfill \\ = \frac{{\underset{\raise0.3em\hbox{$\smash{\scriptscriptstyle-}$}}{\kappa } _1^2{{\bar \kappa }_1}}}{{{{\underline{\kappa }}_{1}} + {{\bar \kappa }_1}}}\frac{{{x_1} - {y_r}}}{{\left( {{{\underline{\kappa }}_{1}} + {y_r}} \right)\left( {{{\underline{\kappa }}_{1}} + {x_1}} \right)}} + \frac{{{{\underline{\kappa }}_{1}}\bar \kappa _1^2}}{{{{\underline{\kappa }}_{1}} + {{\bar \kappa }_1}}}\frac{{{x_1} - {y_r}}}{{\left( {{{\bar \kappa }_1} - {x_1}} \right)\left( {{{\bar \kappa }_1} - {y_r}} \right)}} \hfill \\ = \left[ {\frac{{\underset{\raise0.3em\hbox{$\smash{\scriptscriptstyle-}$}}{\kappa } _1^2{{\bar \kappa }_1}}}{{\left( {{{\underline{\kappa }}_{1}} + {{\bar \kappa }_1}} \right)\left( {{{\underline{\kappa }}_{1}} + {y_r}} \right)\left( {{{\underline{\kappa }}_{1}} + {x_1}} \right)}} + \frac{{{{\underline{\kappa }}_{1}}\bar \kappa _1^2}}{{\left( {{{\underline{\kappa }}_{1}} + {{\bar \kappa }_1}} \right)\left( {{{\bar \kappa }_1} - {x_1}} \right)\left( {{{\bar \kappa }_1} - {y_r}} \right)}}} \right]\left( {{x_1} - {y_r}} \right) \hfill \\ \end{gathered} . | (B.1) |
{x_1} and {y_r} are constrained in the open sets \left({ - {{\underline{\kappa }}_{i}}, {{\bar \kappa }_i}} \right). Thus, 0 < {\underset{\raise0.3em\hbox{$\smash{\scriptscriptstyle-}$}}{\kappa } _1} + {y_r} < {\underset{\raise0.3em\hbox{$\smash{\scriptscriptstyle-}$}}{\kappa } _1} + {\bar \kappa _1}, 0 < {\underset{\raise0.3em\hbox{$\smash{\scriptscriptstyle-}$}}{\kappa } _1} + {x_1} < {\underset{\raise0.3em\hbox{$\smash{\scriptscriptstyle-}$}}{\kappa } _1} + {\bar \kappa _1}, 0 < {\bar \kappa _1} - {x_1} < {\underset{\raise0.3em\hbox{$\smash{\scriptscriptstyle-}$}}{\kappa } _1} + {\bar \kappa _1} and 0 < {\bar \kappa _1} - {y_r} < {\underset{\raise0.3em\hbox{$\smash{\scriptscriptstyle-}$}}{\kappa } _1} + {\bar \kappa _1}. One obtains
\begin{gathered} 0 < \frac{{\underset{\raise0.3em\hbox{$\smash{\scriptscriptstyle-}$}}{\kappa } _1^2{{\bar \kappa }_1}}}{{\left( {{{\underline{\kappa }}_{1}} + {{\bar \kappa }_1}} \right)\left( {{{\underline{\kappa }}_{1}} + {y_r}} \right)\left( {{{\underline{\kappa }}_{1}} + {x_1}} \right)}} + \frac{{{{\underline{\kappa }}_{1}}\bar \kappa _1^2}}{{\left( {{{\underline{\kappa }}_{1}} + {{\bar \kappa }_1}} \right)\left( {{{\bar \kappa }_1} - {x_1}} \right)\left( {{{\bar \kappa }_1} - {y_r}} \right)}} \hfill \\ < \frac{{\underset{\raise0.3em\hbox{$\smash{\scriptscriptstyle-}$}}{\kappa } _1^2{{\bar \kappa }_1}}}{{{{\left( {{{\underline{\kappa }}_{1}} + {{\bar \kappa }_1}} \right)}^3}}} + \frac{{{{\underline{\kappa }}_{1}}\bar \kappa _1^2}}{{{{\left( {{{\underline{\kappa }}_{1}} + {{\bar \kappa }_1}} \right)}^3}}} = \frac{{{{\underline{\kappa }}_{1}}{{\bar \kappa }_1}}}{{{{\left( {{{\underline{\kappa }}_{1}} + {{\bar \kappa }_1}} \right)}^2}}} \hfill \\ \end{gathered} . | (B.2) |
The transformed output tracking error {z_1} = {s_1} - {\alpha _{1c}} strictly converges in the prescribed performance region - \underset{\raise0.3em\hbox{$\smash{\scriptscriptstyle-}$}}{k} \mu \left(t \right) < {z_1}\left(t \right) < \bar k\mu \left(t \right) . Hence, it has
- \frac{{\underset{\raise0.3em\hbox{$\smash{\scriptscriptstyle-}$}}{k} \mu \left( t \right){{\left( {{{\underline{\kappa }}_{1}} + {{\bar \kappa }_1}} \right)}^2}}}{{{{\underline{\kappa }}_{1}}{{\bar \kappa }_1}}} < {x_1} - {y_r} < \frac{{\bar k\mu \left( t \right){{\left( {{{\underline{\kappa }}_{1}} + {{\bar \kappa }_1}} \right)}^2}}}{{{{\underline{\kappa }}_{1}}{{\bar \kappa }_1}}} . | (B.3) |
This finishes the proof.
The authors would like to appreciate all the editors and reviewers for improving the quality of this article. This work was supported by the National Key Research and Development Program of China (2018YFB1304800) and Key Research and Development Program of Guangdong Province (2020B090926002).
All authors declare no conflicts of interest in this paper.
[1] | L. J. Crane, Flow past a stretching plate, Z. Angew. Math. Phys., 21 (1970), 645–647. |
[2] |
C. H. Chen, Laminar mixed convection adjacent to vertical, continuously stretching sheets, Heat Mass Transfer, 33 (1998), 471–476. doi: 10.1007/s002310050217
![]() |
[3] |
A. J. Chamkha, A. M. Aly, M. A. Mansour, Similarity solution for unsteady heat and mass transfer from a stretching surface embedded in a porous medium with suction/injection and chemical reaction effects, Chem. Eng. Commun., 197 (2010), 846–858. doi: 10.1080/00986440903359087
![]() |
[4] |
I. C. Liu, A. M. Megahed, Hung-Hsun Wang, Heat transfer in a liquid film due to an unsteady stretching surface with variable heat flux, J. Appl. Mech., 80 (2013), 041003. doi: 10.1115/1.4007966
![]() |
[5] |
T. Muhammad, K. Rafique, M. Asma, M. Alghamdi, Darcy-Forchheimer flow over an exponentially stretching curved surface with Cattaneo-Christov double diffusion, Physica A, 556 (2020), 123968. doi: 10.1016/j.physa.2019.123968
![]() |
[6] |
A. M. Megahed, M. R. Gnaneswara, W. Abbas, Modeling of MHD fluid flow over an unsteady stretching sheet with thermal radiation, variable fluid properties and heat flux, Math. Comput. Simul., 185 (2021), 583–593. doi: 10.1016/j.matcom.2021.01.011
![]() |
[7] |
P. M. Patil, M. Kulkarni, P. S. Hiremath, Effects of surface roughness on mixed convective nanofluid flow past an exponentially stretching permeable surface, Chinese J. Phys., 64 (2020), 203–218. doi: 10.1016/j.cjph.2019.12.006
![]() |
[8] |
N. S. Khashiie, N. M. Arifin, I. Pop, R. Nazar, E. H. Hafidzuddin, N. Wahi, Three-dimensional hybrid nanofluid flow and heat transfer past a permeable stretching/shrinking sheet with velocity slip and convective condition, Chinese J. Phys., 66 (2020), 157–171. doi: 10.1016/j.cjph.2020.03.032
![]() |
[9] |
D. Pal, D. Chatterjee, K. Vajravelu, Influence of magneto-thermo radiation on heat transfer of a thin nanofluid film with non-uniform heat source/sink, Propuls. Power Res., 9 (2020), 169–180. doi: 10.1016/j.jppr.2020.03.003
![]() |
[10] |
W. Abbas, A. M. Megahed, Numerical solution for chemical reaction and viscous dissipation phenomena on non-Newtonian MHD fluid flow and heat mass transfer due to a nonuniform stretching sheet with thermal radiation, Int. J. Mod. Phys. C, 32 (2021), 2150124. doi: 10.1142/S0129183121501242
![]() |
[11] |
H. Rosali, A. Ishak, I. Pop, Micropolar fluid flow towards a stretching/shrinking sheet in a porous medium with suction, Int. Commun. Heat Mass Transfer, 39 (2012), 826–829. doi: 10.1016/j.icheatmasstransfer.2012.04.008
![]() |
[12] |
I. A. Muhammad, S. Sarwar, M. Imran, Effects of slip on free convection flow of Casson fluid over an oscillating vertical plate, Bound. Value Probl., 2016 (2016), 1–11. doi: 10.1186/s13661-015-0477-3
![]() |
[13] |
A. Usman, K. U. Rehman, M. Y. Malik, Thermal energy statistics for Jeffery fluid flow regime: A generalized Fourier's law outcomes, Physica A, 542 (2020), 123428. doi: 10.1016/j.physa.2019.123428
![]() |
[14] | S. Shateyi, H. Muzara, On the numerical analysis of unsteady MHD boundary layer flow of Williamson fluid over a stretching sheet and heat and mass transfers, Comput., 8 (2020), 55. |
[15] |
T. Hayat, Z. Iqbal, M. Qasim, S. Obaidat, Steady flow of an Eyring Powell fluid over a moving surface with convective boundary conditions, Int. J. Heat Mass Tran., 55 (2012), 1817–1822. doi: 10.1016/j.ijheatmasstransfer.2011.10.046
![]() |
[16] |
N. A. Khan, S. Aziz, N. A. Khan, MHD flow of Powell-Eyring fluid over a rotating disk, J. Taiwan Inst. Chem. Eng., 45 (2014), 2859–2867. doi: 10.1016/j.jtice.2014.08.018
![]() |
[17] |
T. Hayat, M. I. Khan, M. Waqas, A. Alsaedi, On Cattaneo-Christov heat flux in the flow of variable thermal conductivity Eyring-Powell fluid, Results Phys., 7 (2017), 446–450. doi: 10.1016/j.rinp.2016.12.034
![]() |
[18] | N. Ali, F. Nazeer, M. Nazeer, Flow and heat transfer of Eyring-powell fluid in a pipe, Z. Naturforsch. A, 73 (2018), 265–274. |
[19] |
M. Nazeer, F. Ahmad, A. Saleem, M. Saeed, S. Naveed, M. Shaheen, et al., Effects of constant and space dependent viscosity on Eyring-Powell fluid in a pipe: Comparison of perturbation and explicit finite difference method, Z. Naturforsch. A, 74 (2019), 961–969. doi: 10.1515/zna-2019-0095
![]() |
[20] |
M. Nazeer, F. Ahmad, M. Saeed, A. Saleem, S. Naveed, Z. Akram, Numerical solution for flow of a Eyring-powell fluid in a pipe with prescribed surface temperature, J. Braz. Soc. Mech. Sci., 41 (2019), 518. doi: 10.1007/s40430-019-2005-3
![]() |
[21] |
A. Alsaedi, T. Hayat, S. Qayyum, R. Yaqoob, Eyring-Powell nanofluid flow with nonlinear mixed convection: Entropy generation minimization, Comput. Meth. Prog. Bio., 186 (2020), 105183. doi: 10.1016/j.cmpb.2019.105183
![]() |
[22] |
T. Muhammad, H. Waqas, S. A. Khan, R. Ellahi, S. M. Sait, Significance of nonlinear thermal radiation in 3D Eyring-Powell nanofluid flow with Arrhenius activation energy, J. Therm. Anal. Calorim., 143 (2021), 929–944. doi: 10.1007/s10973-020-09459-4
![]() |
[23] |
V. S. Patil, A. B. Patil, S. Ganesh, P. H. Pooja, N. S. Patil, Unsteady MHD flow of a nano powell-eyring fluid near stagnation point past a convectively heated stretching sheet in the existence of chemical reaction with thermal radiation, Mater. Today: Proc., 44 (2021), 3767–3776. doi: 10.1016/j.matpr.2020.11.860
![]() |
[24] |
M. Hamid, T. Zubair, M. Usman, R. U. Haq, Numerical investigation of fractional-order unsteady natural convective radiating flow of nanofluid in a vertical channel, AIMS Math., 4 (2019), 1416–1429. doi: 10.3934/math.2019.5.1416
![]() |
[25] | W. Abbas, M. M. Magdy, Heat and mass transfer analysis of nanofluid flow based on, and over a moving rotating plate and impact of various nanoparticle shapes, Math. Probl. Eng., 2020 (2020), Article ID 9606382, 12 pages. |
[26] |
W. Abbas, A. S. Emad, Hall current and joule heating effects on free convection flow of a nanofluid over a vertical cone in presence of thermal radiation, Therm. Sci., 21 (2017), 2609–2620. doi: 10.2298/TSCI160413083A
![]() |
[27] |
S. S. Ghadikolaei, K. H. Hosseinzadeh, D. D. Ganji, B. Jafari, Nonlinear thermal radiation effect on magneto Casson nanofluid flow with Joule heating effect over an inclined porous stretching sheet, Case Stud. Therm. Eng., 12 (2018), 176–187. doi: 10.1016/j.csite.2018.04.009
![]() |
[28] |
E. O. Fatunmbi, A. Adeniyan, Nonlinear thermal radiation and entropy generation on steady flow of magneto-micropolar fluid passing a stretchable sheet with variable properties, Results Eng., 6 (2020), 100142. doi: 10.1016/j.rineng.2020.100142
![]() |
[29] |
W. Abbas, K. S. Mekheimer, M. M. Ghazy, A. M. A. Moawad, Thermal radiation effects on oscillatory squeeze flow with a particle-fluid suspension, Heat Transfer, 50 (2021), 2129–2149. doi: 10.1002/htj.21971
![]() |
[30] |
T. Muhammad, H. Waqas, U. Farooq, M. S. Alqarni, Numerical simulation for melting heat transport in nanofluids due to quadratic stretching plate with nonlinear thermal radiation, Case Stud. Therm. Eng., 27 (2021), 101300. doi: 10.1016/j.csite.2021.101300
![]() |
[31] | M. Bilal, S. Ashbar, Flow and heat transfer analysis of Eyring-Powell fluid over stratified sheet with mixed convection, JOEMS, 28 (2020), 40. |
[32] | I. Jabeen, M. Farooq, M. Rizwan, R. Ullah, S. Ahmad, Analysis of nonlinear stratified convective flow of Powell-Eyring fluid: Application of modern diffusion, Adv. Mech. Eng., 12 (2020), 1–10. |
[33] |
R. E. Powell, H. Eyring, Mechanism for relaxation theory of viscosity, Nature, 154 (1944), 427–428. doi: 10.1038/154594a0
![]() |
[34] |
N. A. Khan, S. Khan, A. Arab, Flow of micropolar fluid over an off centered rotating disk with modified Darcy's law, Propuls. Power Res., 6 (2017), 285–295. doi: 10.1016/j.jppr.2017.11.006
![]() |
[35] |
N. A. Khan, F. Naz, F. Sultan, Entropy generation analysis and effects of slip conditions on micropolar fluid flow due to a rotating disk, Open Eng., 7 (2017), 185–198. doi: 10.1515/eng-2017-0025
![]() |
[36] | A. Raptis, C. Perdikis, H. S. Takhar, Effect of thermal radiation on MHD flow, Appl. Math. Comput., 153 (2004), 645–649. |
[37] |
A. Raptis, Radiation and viscoelastic flow, Int. Commun. Heat Mass, 26 (1999), 889–895. doi: 10.1016/S0735-1933(99)00077-9
![]() |
[38] | T. Hayat, S. Ali, M. A. Farooq, A. Alsaedi, On comparison of series and numerical solutions for flow of Eyring-Powell fluid with newtonian heating and internal heat generation/absorption, PLOS ONE, 10 (2015), 1–13. |
[39] |
S. E. El-Gendi, Chebyshev solution of differential, integral and integro-differential equations, Comput. J., 12 (1969), 282–287. doi: 10.1093/comjnl/12.3.282
![]() |
1. | Yankui Song, Gong Cheng, Yaoyao Tuo, Adaptive output feedback control for chaotic PMSMs stochastic system considering constraints, 2024, 187, 09600779, 115321, 10.1016/j.chaos.2024.115321 |