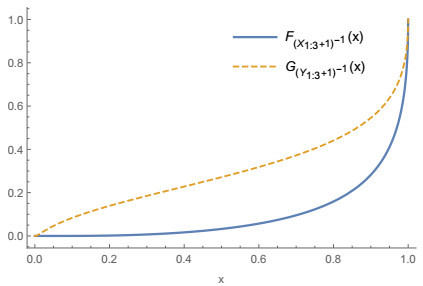
Citation: Miaomiao Zhang, Bin Lu, Rongfang Yan. Ordering results of extreme order statistics from dependent and heterogeneous modified proportional (reversed) hazard variables[J]. AIMS Mathematics, 2021, 6(1): 584-606. doi: 10.3934/math.2021036
[1] | Bin Lu . Stochastic comparisons of second-order statistics from dependent and heterogeneous modified proportional (reversed) hazard rates scale models. AIMS Mathematics, 2024, 9(4): 8904-8919. doi: 10.3934/math.2024434 |
[2] | Mingxia Yang . Orderings of the second-largest order statistic with modified proportional reversed hazard rate samples. AIMS Mathematics, 2025, 10(1): 311-337. doi: 10.3934/math.2025015 |
[3] | Xiao Zhang, Rongfang Yan . Stochastic comparisons of extreme order statistic from dependent and heterogeneous lower-truncated Weibull variables under Archimedean copula. AIMS Mathematics, 2022, 7(4): 6852-6875. doi: 10.3934/math.2022381 |
[4] | Li Zhang, Rongfang Yan . Stochastic comparisons of series and parallel systems with dependent and heterogeneous Topp-Leone generated components. AIMS Mathematics, 2021, 6(3): 2031-2047. doi: 10.3934/math.2021124 |
[5] | Bin Lu, Rongfang Yan . Ordering results of second order statistics from random and non-random number of random variables with Archimedean copulas. AIMS Mathematics, 2021, 6(6): 6390-6405. doi: 10.3934/math.2021375 |
[6] | H. M. Barakat, M. A. Alawady, I. A. Husseiny, M. Nagy, A. H. Mansi, M. O. Mohamed . Bivariate Epanechnikov-exponential distribution: statistical properties, reliability measures, and applications to computer science data. AIMS Mathematics, 2024, 9(11): 32299-32327. doi: 10.3934/math.20241550 |
[7] | Fawaz K. Alalhareth, Seham M. Al-Mekhlafi, Ahmed Boudaoui, Noura Laksaci, Mohammed H. Alharbi . Numerical treatment for a novel crossover mathematical model of the COVID-19 epidemic. AIMS Mathematics, 2024, 9(3): 5376-5393. doi: 10.3934/math.2024259 |
[8] | Mansour Shrahili, Mohamed Kayid . Laplace transform ordering of bivariate inactivity times. AIMS Mathematics, 2022, 7(7): 13208-13224. doi: 10.3934/math.2022728 |
[9] | Mohamed Kayid, Adel Alrasheedi . Weighted proportional mean inactivity time model. AIMS Mathematics, 2022, 7(3): 4038-4060. doi: 10.3934/math.2022223 |
[10] | Ze Gu . On weakly semiprime segments of ordered semihypergroups. AIMS Mathematics, 2021, 6(9): 9882-9885. doi: 10.3934/math.2021573 |
In reliability theory, to model the lifetime data with different hazard shapes, it is desirable to introduce flexible families of distributions, and to this end, there are two methods have been commonly used to characterise lifetime distribution with considerable flexibility. One method is to adopt the well-known families of distributions, for example, Gamma, Weibull and Log-normal, which have been studied quite extensively in the literature, for more discussions on this topic, we refer readers to [1,2,3]. Marshall and Olkin [4] developed a new method to introduce one parameter to a base distribution results in a new family of distribution with more flexibility. For example, for a baseline distribution function F with support R+=(0,∞) and corresponding survival function ˉF, the new distribution functions can be defined as
G(x;α)=F(x)1−ˉαˉF(x),x,α∈R+,ˉα=1−α, | (1.1) |
H(x;α)=αF(x)1−ˉαF(x),x,α∈R+,ˉα=1−α. | (1.2) |
Marshall and Olkin [4] originally proposed the family of distributions in (1.1) and studied it for the case when F is a Weibull distribution. When F has probability density and hazard rate functions as f and hF, respectively, then the hazard rate function of G is given by
hF(x;α)=11−ˉαˉF(x)hF(x),x,α∈R+,ˉα=1−α, |
Therefore, one can observe that if hF(x) is decreasing (increasing) in x, then for 0<α≤1(α≥1), hF(x;α) is also decreasing in x. Moreover, one can observe that hF(x)≤hF(x;α) for 0<α≤1, and hF(x;α)≤hF(x) for α≥1. For this reason, the parameter α in (1.1) is referred to as a tilt parameter (see [5]). Note that (1.1) is equivalent to (1.2) if α in (1.1) is changed to 1/α. The proportional hazard rates (PHR) and the proportional reversed hazard rates (PRHR) models have important applications in reliability and survival analysis. The random variables X1,⋯,Xn are said to follow: (ⅰ) PHR model if Xi has the survival function ˉFXi(x)=ˉFλi(x), i=1,⋯,n, where ˉF is the baseline survival function and (λ1,⋯,λn) is the frailty vector; (ii) PRHR model if Xi has the distribution function FXi(x)=Fβi(x), i=1,⋯,n, where F is the baseline distribution and (β1,⋯,βn) is the resilience vector. It is well-known that the Exponential, Weibull, Lomax and Pareto distributions are special cases of the PHR model, and Fréchet distribution is a special case of the PRHR model. Balakrishnan et al. [6] introduced two new statistical models by adding a parameter to PHR and PRHR models, which are regarded as the baseline distributions in G(x;α) and H(x;α), respectively. The two new models are referred to as the modified proportional hazard rates (MPHR) and modified proportional reversed hazard rates (MPRHR) models, respectively. These are given by
G(x;α,λ)=1−(ˉF(x))λ1−ˉα(ˉF(x))λ,x,α∈R+,ˉα=1−α, | (1.3) |
H(x;α,β)=α(F(x))β1−ˉα(F(x))β,x,α∈R+,ˉα=1−α, | (1.4) |
where λ and β are the proportional hazard rate and proportional reversed hazard rate parameters, respectively. We denote X∼MPHR(α,λ;ˉF) and X∼MPRHR(α,β;F) if X has the distribution functions G(x;α,λ) and H(x;α,β), respectively. For the case λ=β=1, (1.3) and (1.4) simply reduce to (1.1) and (1.2), respectively. For the case α=1, (1.3) and (1.4) simply reduce to the PHR and PRHR models, respectively. According to the Theorem 2.1 of Navarro et al.[14], (1.3) and (1.4) can be rewritten the distorted distribution of h1 and h2, respectively, where
h1(u;α,λ)=1−(u)λ1−ˉα(u)λ,h2(u;α,β)=α(1−u)β1−ˉα(1−u)β,u=ˉF(x),x,α∈R+,ˉα=1−α, | (1.5) |
if λ=β=1, (1.5) just as the distorted distributions of (1.1) and (1.2), respectively. For some special models, please refer to multiple-outlier models ([7], [8]), extended exponential and extend Weibull distribution ([4], [9]), extended Pareto distribution ([10]) and extended Lomax distribution ([11]).
Order statistics play an important role in reliability theory, auction theory, operations research, and many applied probability areas. Xk:n denotes the kth smallest of random variables X1,…,Xn,k=1,…,n. In reliability theory, Xk:n characterizes the lifetime of a (n−k+1)-out-of-n system, which works if at least n−k+1 of all the n components function normally. Specifically, X1:n and Xn:n denote the lifetimes of series and parallel systems, respectively. In auction theory, X1:n and Xn:n represent the final price of the first-price procurement auction and the first-price sealed-bid auction (see [12]), respectively. In the past decades, researchers devoted themselves to stochastic comparisons of order statistics from heterogeneous independent or dependent samples. For example. Belzunce et al. [13] established some results and applications concerning the likelihood ratio order of random vectors of order statistics in the case of independent but not necessarily identically distributed observations and for the case of possible dependent observations. Navarro et al.[14] obtained ordering properties for coherent systems with possibly dependent identically distributed components. Balakrishnan and Zhao[15] studied the stochastic comparison of order statistics from independent and heterogeneous proportional hazard rates models, gamma variables, geometric variables, and negative binomial variables in the stochastic orders and majorization orders. For more discussions related to order statistics, one may refer to [16,17,18,19,20]. Besides, many authors have studied stochastic comparisons of order statistics from heterogeneous samples following some families of lifetime distributions. For example, Fang et al.[21] conducted stochastic comparisons on sample extremes of dependent and heterogeneous observations from PHR and PRHR models. Balakrishnan et al. [6] introduced MPHR and MPRHR model, and established some stochastic comparisons between the corresponding order statistics with independent samples. Li and Li [22] developed sufficient conditions for the (reversed) hazard rate order on maximums (minimums) of samples following PRHR (PHR) model under Archimedean copula. Das and Kayal [23] introduced a scale parameter into the MPHR and MPRHR models lead to new models, which are called as modified proportional hazard rate scale (MPHRS) and modified proportional reversed hazard rate scale (MPRHRS) models, respectively, and obtained some stochastic comparison results on independent samples in term of the usual stochastic, (reversed) hazard rate orders. Barmalzan et al. [24] discussed the hazard rate order and reversed hazard rate order of series and parallel systems with dependent components following either MPHR or MPRHR models under Archimedean copula. Motivated by the work of Balakrishnan et al.[6], this paper devotes to studying stochastic comparisons of sample extremes arising from heterogeneous and dependent MPHR and MPRHR models, and we derive the usual stochastic, (reversed) hazard rate orders of extremes with the heterogeneity considered in the model parameters.
The remaining part of the paper is organized as follows: Section 2 recalls some basic concepts and notations that will be used in the sequel. Section 3 deals with stochastic comparisons between minimums of MPRHR (MPHR) sample with Archimedean (survival) copula, respectively. Section 4 presents the corresponding results on maximums of the sample. Section 5 summarizes our research findings and future directions.
In this section, let us first review some basic concepts that will be used in the sequel. Let X and Y be two random variables with distribution functions F(x) and G(x), survival functions ˉF(x)=1−F(x) and ˉG(x)=1−G(x), probability density functions f(x) and g(x), the hazard rate functions hX(x)=f(x)/ˉF(x) and hY(x)=g(x)/ˉG(x), and reversed hazard rate functions rX(x)=f(x)/F(x) and rY(x)=g(x)/G(x), respectively.
Definition 1. X is said to be smaller than Y in the sense of
(i) the usual stochastic order (denoted by X≤stY) if ˉF(x)≤ˉG(x) for all x∈R+;
(ii) the hazard rate order (denoted by X≤hrY) if hX(x)≥hY(x) for all x∈R+ or equivalently, if ˉG(x)/ˉF(x) is increasing in x∈R+;
(iii) the reversed hazard rate order (denoted by X≤rhY) if rX(x)≤rY(x) for all x∈R+ or equivalently, if G(x)/F(x) is increasing in x∈R+.
It is well known that the hazard rate and reversed hazard rate orders imply the usual stochastic order, but the converse is not true. For a comprehensive discussion on various stochastic orders and their applications, one may refer to Shaked and Shanthikumar[25] and Li and Li [26].
Next, we introduce the notion of the weak majorization order. For two real vectors x=(x1,...,xn) and y=(y1,...,yn)∈Rn, x(1)≤x(2)≤⋯≤x(n) and y(1)≤y(2)≤⋯≤y(n) denote the increasing arrangement of the components of x and y, respectively. Denote In={1,…,n}.
Definition 2. The vector x is said to be weakly supermajorized by the vector y (write as xw⪯y) if ∑ji=1x(i)≥∑ji=1y(i), for all j∈In.
Now, let us review the concept of Archimedean Copulas.
Definition 3. For a decreasing and continuous function ψ:[0,+∞]↦[0,1] such that ψ(0)=1 and ψ(+∞)=0, let ϕ=ψ−1 be the pseudo-inverse. Then
Cψ(u1,...,un)=ψ(ϕ(u1)+...+ϕ(un)),ui∈[0,1],i∈In, |
is said to be an Archimedean copula with generator ψ if (−1)kψk(x)≥0 for k=0,…,n−2 and (−1)n−2ψn−2(x) is decreasing and convex.
For detailed discussions on copulas and its applications, one may refer to Nelsen [27].
The following lemmas are useful to establish the main results.
Lemma 1. ([28]) Let I⊆R be an open interval, a continuously differentiable h:In→R is Schur-convex(Schur-concave) if and only if h is symmetric on In and for all i≠j
(xi−xj)(∂h(x)∂xi−∂h(x)∂xj)≥(≤)0. |
Lemma 2. ([29]) For a real function h on A⊆Rn, xw⪯y implies h(x)≤h(y) if and only if h is decreasing and Schur-convex on A.
h(x) is Schur-concave on A if and only if −h(x) is Schur-convex. For more details on majorization and Schur-convexity (concavity), please refer to [29].
Lemma 3. For two n-dimensional Archimedean copulas Cψ1 and Cψ2, if ϕ2∘ψ1 is super-additive, then Cψ1(u)≤Cψ2(u) for all u∈[0,1]n.
Throughout this paper, all concerned random variables are assumed to be absolutely continuous and nonnegative, and the terms increasing and decreasing stand for non-decreasing and non-increasing, respectively. Denote ei=(0,…,0,1,⏟i0,…,0⏟n−i) for i∈In, and “sgn=” means equality of sign.
In this section, we carry out stochastic comparison of smallest order statistics from dependent and heterogeneous MPHR and MPRHR samples, and the heterogeneity has been considered in the model parameters.
In this subsection, we deal with the case of MPHR samples in the sense of the usual stochastic and hazard rate orders. Let X=(X1,…,Xn) be the random vector, we denote X∼MPHR(α;λ;ˉF;ψ) the sample follows MPHR model, where ˉF is the baseline survival function, ψ is generator of the associated Archimedean survival copula, and α=(α1,…,αn) and λ=(λ1,…,λn) are the tilt parameter vector and modified proportional hazard rate vector, respectively.
Next, we establish sufficient conditions for the usual stochastic order, whenever the modified proportional hazard rate parameters may be different with the tilt parameters being equal.
Theorem 1. For X∼MPHR(α;λ;ˉF;ψ1) and Y∼MPHR(α;μ;ˉF;ψ2), where 0<α≤1. If ψ1 or ψ2 is log-concave, and ϕ2∘ψ1 is super-additive, then λw⪯μ implies
X1:n≤stY1:n. |
Proof. The survival function of X1:n can be expressed as
ˉFX1:n(x)=ψ1(n∑i=1ϕ1(α(ˉF(x))λi1−ˉα(ˉF(x))λi))=J1(α,λ,ψ1,ˉF(x)),x≥0. |
Assume that ψ1 is log-concave. In order to obtain the required result, according to Lemma 2, it is sufficient to show that J1(α,λ,ψ1,ˉF(x)) is decreasing in λi and Schur-convex in λ for given x≥0 and 0<α≤1. Taking the partial derivative of J1(α,λ,ψ1,ˉF(x)) with respect to λi, i∈In, we have
∂J1(α,λ,ψ1,ˉF(x))∂λi=ψ′1(n∑i=1ϕ1(α(ˉF(x))λi1−ˉα(ˉF(x))λi))lnˉF(x)α(ˉF(x))λi1−ˉα(ˉF(x))λiψ′(ϕ1(α(ˉF(x))λi1−ˉα(ˉF(x))λi))(1−ˉα(ˉF(x))λi)≤0. |
That is, J1(α,λ,ψ1,ˉF(x)) is decreasing in λi. Furthermore, for i≠j, we have
(λi−λj)(∂J1(α,λ,ψ1,ˉF(x))∂λi−∂J1(α,λ,ψ1,ˉF(x))∂λj)=ψ′1(n∑i=1ϕ1(α(ˉF(x))λi1−ˉα(ˉF(x))λi))lnˉF(x)(λi−λj)(h1(λi)−h1(λj)), |
where
h1(λ)=α(ˉF(x))λ1−ˉα(ˉF(x))λψ′1(ϕ1(α(ˉF(x))λ1−ˉα(ˉF(x))λ))(1−ˉα(ˉF(x))λ). |
Since the log-concavity of ψ1 implies the increasing property of ψ1/ψ′1, and consider that ϕ1(α(ˉF(x))λ/(1−ˉα(ˉF(x))λ)) is increasing in λ, then we have
α(ˉF(x))λ1−ˉα(ˉF(x))λψ′1(ϕ1(α(ˉF(x))λ1−ˉα(ˉF(x))λ))=ψ1(ϕ1(α(ˉF(x))λ1−ˉα(ˉF(x))λ))ψ′1(ϕ1(α(ˉF(x))λ1−ˉα(ˉF(x))λ))≤0. |
On the other hand, 1/(1−ˉα(ˉF(x))λ) is nonnegative and decreasing in λ. Consequently, h1(λ) is increasing in λ, which in turn implies that
(λi−λj)(∂J1(α,λ,ψ1,ˉF(x))∂λi−∂J1(α,λ,ψ1,ˉF(x))∂λj)≥0. |
Thus, Schur-convexity of J1(α,λ,ψ1,ˉF(x)) follows from Lemma 1. Due to Lemma 2, λw⪯μ implies J1(α,λ,ψ1,ˉF(x))≤J1(α,μ,ψ1,ˉF(x)), and note that ϕ2∘ψ1 is super-additive, by Lemma 3, we have J1(α,μ,ψ1,ˉF(x))≤J1(α,μ,ψ2,ˉF(x)). Hence, it holds that
J1(α,λ,ψ1,ˉF(x))≤J1(α,μ,ψ1,ˉF(x))≤J1(α,μ,ψ2,ˉF(x)). |
As a consequence, we conclude that X1:n≤stY1:n. For the case of ψ2 is log-concave, the proof can be obtained in a similar way. Then we complete the proof.
Remark 1. When α=1, MPHR model reduces to PHR model, which just the result established in Theorem 4.1 (ii) of [21].
The next example illustrates the result of Theorem 1.
Example 1. Consider the case of n=3. Let ˉF(x)=e−(ax)b, a>0, 0<b≤1, and generators ψ1(x)=e1−exθ1, 0<θ1≤1, ψ2(x)=(θ2x+1)−1/θ2, θ2>0. Set α=0.4,a=1.2,b=0.5,θ1=0.1,θ2=1.2,λ=(0.4,0.5,0.6)w⪯(0.3,0.4,0.5)=μ. One can check chat ψ1(x) is log-concave. It can be seen that [ϕ2∘ψ1(x)]″=ex(e1θ1−exθ1)−θ2(θ1+exθ2)θ1−2≥0, that is, ϕ2∘ψ1(x) is convex function in x, which implies that ϕ2∘ψ1 is super-additive. To plot the whole of survival curves of X1:3 and Y1:3 on [0,∞), we perform the transformation (x+1)−1:[0,∞)⟼[0,1]. Then it is obvious that X1:3≤stY1:3 is equivalent to (Y1:3+1)−1≤st(X1:3+1)−1. As is seen in Figure 1, the distribution curve of (X1:3+1)−1 is always beneath that of (Y1:3+1)−1, that is, X1:3≤stY1:3, which coincides with the result of Theorem 1.
The following counterexample shows that the condition ψ1(x) or ψ2(x) is log-concave given in the above Theorem 1 can't be dropped.
Counterexample 1. Under the setup of Example 1, let generators ψ1(x)=(θ1x+1)−1/θ1,ψ2(x)=(θ2x+1)−1/θ2, θi>0,i=1,2. Note that [logψi(x)]″=θi(1+θix)−2≥0 for x≥0, thus, ψi(x) is log-convex. Take θ1=10,θ2=1.2. As is seen in Figure 2, the survival function ˉFX1:3(x) is not always beneath that of ˉGY1:3(x), that is, neither X1:3≤stY1:3 nor X1:3≥stY1:3.
The following theorem presents the usual stochastic order on sample minimum, here, we assume that the two samples have common modified proportional hazard rates parameters.
Theorem 2. For X∼MPHR(α;λ;ˉF;ψ1) and Y∼MPHR(β;λ;ˉF;ψ2). If ϕ1∘ψ2 is super-additive, then αw⪯β implies
X1:n≥stY1:n. |
Proof. The survival function of X1:n can be written as
ˉFX1:n(x)=ψ1(n∑i=1ϕ1(αi(ˉF(x))λ1−ˉαi(ˉF(x))λ))=J2(α,λ,ψ1,ˉF(x)),x≥0. |
To obtain the required result, it suffices to show that the J2(α,λ,ψ1,ˉF(x)) is increasing in αi and Schur-concave in α, i∈In. Differentiating J2(α,λ,ψ1,ˉF(x)) with respect to αi, we obtain
∂J2(α,λ,ψ1,ˉF(x))∂αi=ψ′1(n∑i=1ϕ1(αi(ˉF(x))λ1−ˉαi(ˉF(x))λ))(ˉF(x))λ(1−(ˉF(x))λ)ψ′1(ϕ1(αi(ˉF(x))λ1−ˉαi(ˉF(x))λ))(1−ˉαi(ˉF(x))λ)2≥0. |
That is, J2(α,λ,ψ1,ˉF(x)) is increasing in αi. Furthermore, for i≠j, we have
(αi−αj)(∂J2(α,λ,ψ1,ˉF(x))∂αi−∂J2(α,λ,ψ1,ˉF(x))∂αj)=ψ′1(n∑i=1ϕ1(αi(ˉF(x))λ1−ˉαi(ˉF(x))λ))(ˉF(x))λ(1−(ˉF(x))λ)(αi−αj)(1h2(αi)−1h2(αj)), |
where
h2(α)=(1−ˉα(ˉF(x))λ)2ψ′1(ϕ1(α(ˉF(x))λ1−ˉα(ˉF(x))λ)). |
By the decreasing and convexity of ψ1, it holds that
∂h2(α)∂α=2(ˉF(x))λ(1−ˉα(ˉF(x))λ)ψ′1(ϕ1(α(ˉF(x))λ1−ˉα(ˉF(x))λ))+(ˉF(x))λ(1−(ˉF(x))λ)ψ″1(ϕ1(α(ˉF(x))λ1−ˉα(ˉF(x))λ))ψ′1(ϕ1(α(ˉF(x))λ1−ˉα(ˉF(x))λ))≤0. |
Hence, h2(α) is negative and decreasing in α, or equivalently, 1/h2(α) is negative and increasing in α. Then, for i≠j,
(αi−αj)(∂J2(α,λ,ψ1,ˉF(x))∂αi−∂J2(α,λ,ψ1,ˉF(x))∂αj)≤0. |
It follows from Lemma 1 that J2(α,λ,ψ1,ˉF(x)) is Schur-concave, which is equivalent to −J2(α,λ,ψ1,ˉF(x)) is Schur-convex. According to Lemma 2, αw⪯β implies −J2(α,λ,ψ1,ˉF(x))≤−J2(β,λ,ψ1,ˉF(x)), and note that ϕ1∘ψ2 is super-additive, it holds by Lemma 3 that J2(β,λ,ψ1,ˉF(x))≥J2(β,λ,ψ2,ˉF(x)). Hence,
J2(α,λ,ψ1,ˉF(x))≥J2(β,λ,ψ1,ˉF(x))≥J2(β,λ,ψ2,ˉF(x)). |
That is, X1:n≥stY1:n. The desired result then follows.
The following example demonstrates the theoretical result of Theorem 2.
Example 2. Let ˉF(x)=e−(ax)b,a>0, 0<b≤1, and generators ψ1(x)=(θx+1)−1/θ, θ>0,ψ2(x)=e−x. Take n=3,λ=0.4,a=0.5,b=0.8,θ=0.7 and α=(0.3,0.5,0.7)w⪯(0.2,0.4,0.5)=β. It is easy to check that the conditions of Theorem 2 are all satisfied. The distribution functions of (X1:3+1)−1 and (Y1:3+1)−1 are displayed in Figure 3, which confirms X1:3≥stY1:3.
The next theorem gives sufficient conditions guaranteeing the hazard rate order between two modified proportional hazard rates models with the same modified proportional hazard rates parameters and the heterogeneous tilt parameters.
Theorem 3. For X∼MPHR(α;λ;ˉF;ψ) and Y∼MPHR(β;λ;ˉF;ψ) with log-concave ψ, and −ψ′/ψ is log-convex. If αw⪯β, then
X1:n≥hrY1:n. |
Proof. For convenience, let us give the following facts for any x≥0.
ϱ1: ψ(x)≥0 and ψ′(x)≤0.
ϱ2: The log-concave ψ implies the decreasing ψ′/ψ and hence ψ″(x)ψ(x)≤[ψ′(x)]2.
ϱ3: The log-convex −ψ′/ψ implies that {ψ″(x)ψ(x)−[ψ′(x)]2}/ψ(x)ψ′(x)≥0 increases in x≥0.
Denote h(x) the hazard rate function corresponding to the baseline survival function ˉF(x). The survival function of X1:n can be written as
ˉFX1:n(x)=ψ(n∑i=1ϕ(αi(ˉF(x))λ1−ˉαi(ˉF(x))λ)),x≥0, |
and the hazard rate function of X1:n is
hX1:n(x)=ψ′(∑ni=1ϕ(αi(ˉF(x))λ1−ˉαi(ˉF(x))λ))ψ(∑ni=1ϕ(αi(ˉF(x))λ1−ˉαi(ˉF(x))λ))n∑i=1λh(x)1−ˉαi(ˉF(x))λψ(ϕ(αi(ˉF(x))λ1−ˉαi(ˉF(x))λ))ψ′(ϕ(αi(ˉF(x))λ1−ˉαi(ˉF(x))λ))=L1(x,α,λ,ψ). |
Likewise, Y1:n gets the hazard rate function hY1:n(x)=L1(x,β,λ,ψ) for x≥0. Further denote
A1(α(s,t),x)=∑i≠s,tλh(x)1−ˉαi(ˉF(x))λB1(αiei,x),B1(α,x)=ψ(∑ni=1ϕ(αi(ˉF(x))λ1−ˉαi(ˉF(x))λ))ψ′(∑ni=1ϕ(αi(ˉF(x))λ1−ˉαi(ˉF(x))λ)), |
Jψ(x)=ψ″(x)ψ(x)−(ψ′(x))2(ψ(x))2,C1(α,x)=Jψ(n∑i=1ϕ(αi(ˉF(x))λ1−ˉαi(ˉF(x))λ))B1(α,x). |
Then, for any s,t∈In with s≠t and all ui∈[0,1] (i∈In), we have
∂L1(x,α,λ,ψ)∂αs=(ˉF(x))λ(1−(ˉF(x))λ)(1−ˉαs(ˉF(x))λ)2ψ′(ϕ(αs(ˉF(x))λ1−ˉαs(ˉF(x))λ))n∑i=1λh(x)1−ˉαi(ˉF(x))λB1(αiei,x)Jψ(n∑i=1ϕ(αi(ˉF(x))λ1−ˉαi(ˉF(x))λ))−B1(αses,x)B1(α,x)λh(x)(ˉF(x))λ(1−ˉαs(ˉF(x))λ)2−B21(αses,x)B1(α,x)λh(x)(1−ˉαs(ˉF(x))λ)ψ′(ϕ(αs(ˉF(x))λ1−ˉαs(ˉF(x))λ))(ˉF(x))λ(1−(ˉF(x))λ)(1−ˉαs(ˉF(x))λ)2Jψ(ϕ(αs(ˉF(x))λ1−ˉαs(ˉF(x))λ))=[(ˉF(x))λ(1−(ˉF(x))λ)A1(α(s,t),x)(1−ˉαs(ˉF(x))λ)2ψ′(ϕ(αs(ˉF(x))λ1−ˉαs(ˉF(x))λ))+(ˉF(x))λ(1−(ˉF(x))λ)(1−ˉαs(ˉF(x))λ)2ψ′(ϕ(αs(ˉF(x))λ1−ˉαs(ˉF(x))λ))λh(x)B1(αses,x)1−ˉαs(ˉF(x))λ+(ˉF(x))λ(1−(ˉF(x))λ)(1−ˉαs(ˉF(x))λ)2ψ′(ϕ(αs(ˉF(x))λ1−ˉαs(ˉF(x))λ))λh(x)1−ˉαt(ˉF(x))λB1(αtet,x)]⋅Jψ(n∑i=1ϕ(αi(ˉF(x))λ1−ˉαi(ˉF(x))λ))−B1(αses,x)B1(α,x)λh(x)(ˉF(x))λ(1−ˉαs(ˉF(x))λ)2−B1(αses,x)C1(αses,x)B1(α,x)ψ′(ϕ(αs(ˉF(x))λ1−ˉαs(ˉF(x))λ))λh(x)1−ˉαs(ˉF(x))λ(ˉF(x))λ(1−(ˉF(x))λ)(1−ˉαs(ˉF(x))λ)2. |
By ϱ3, it holds that C1(α,x)≥C1(αses,x), and in combination ϱ1 with ϱ2, we have
∂L1(x,α,λ,ψ)∂αs=[(ˉF(x))λ(1−(ˉF(x))λ)A1(α(s,t),x)(1−ˉαs(ˉF(x))λ)2ψ′(ϕ(αs(ˉF(x))λ1−ˉαs(ˉF(x))λ))+(ˉF(x))λ(1−(ˉF(x))λ)(1−ˉαs(ˉF(x))λ)2ψ′(ϕ(αs(ˉF(x))λ1−ˉαs(ˉF(x))λ))λh(x)B1(αtet,x)1−ˉαt(ˉF(x))λ]⋅Jψ(n∑i=1ϕ(αi(ˉF(x))λ1−ˉαi(ˉF(x))λ))−B1(αses,x)B1(α,x)λh(x)(ˉF(x))λ(1−ˉαs(ˉF(x))λ)2+(ˉF(x))λ(1−(ˉF(x))λ)(1−ˉαs(ˉF(x))λ)2ψ′(ϕ(αs(ˉF(x))λ1−ˉαs(ˉF(x))λ))λh(x)1−ˉαs(ˉF(x))λB1(αses,x)(C1(α,x)−C1(αses,x))B1(α,x)≤0. |
Therefore, L1(x,α,λ,ψ) is decreasing in αi for any i∈In. Furthermore, for s,t∈In with s≠t, we obtain
(αs−αt)(∂L1(x,α,λ,ψ)∂αs−∂L1(x,α,λ,ψ)∂αt)=(αs−αt)Jψ(n∑i=1ϕ(αi(ˉF(x))λ1−ˉαi(ˉF(x))λ))(ˉF(x))λ(1−(ˉF(x))λ)A1(α(s,t),x)⋅[1(1−ˉαs(ˉF(x))λ)2ψ′(ϕ(αs(ˉF(x))λ1−ˉαs(ˉF(x))λ))−1(1−ˉαt(ˉF(x))λ)2ψ′(ϕ(αt(ˉF(x))λ1−ˉαt(ˉF(x))λ))]+(αs−αt)(1αs−1αt)Jψ(n∑i=1ϕ(αi(ˉF(x))λ1−ˉαi(ˉF(x))λ))(1−(ˉF(x))λ)λh(x)B1(αses,x)B1(αtet,x)(1−ˉαs(ˉF(x))λ)(1−ˉαt(ˉF(x))λ)+(αs−αt)λh(x)(ˉF(x))λB1(α,x)(B1(αtet,x)(1−ˉαt(ˉF(x))λ)2−B1(αses,x)(1−ˉαs(ˉF(x))λ)2)+(αs−αt)λ h(x)(ˉF(x))λ(1−(ˉF(x))λ)B1(α,x)⋅[B1(αses,x)(C1(α,x)−C1(αses,x))(1−ˉαs(ˉF(x))λ)3ψ′(ϕ(αs(ˉF(x))λ1−ˉαs(ˉF(x))λ))−B1(αtet,x)(C1(α,x)−C1(αtet,x))(1−ˉαt(ˉF(x))λ)3ψ′(ϕ(αt(ˉF(x))λ1−ˉαt(ˉF(x))λ))]sgn=−(αs−αt)C1(α,x)(ˉF(x))λ(1−(ˉF(x))λ)A1(α(s,t),x)⋅[1(1−ˉαs(ˉF(x))λ)2ψ′(ϕ(αs(ˉF(x))λ1−ˉαs(ˉF(x))λ))−1(1−ˉαt(ˉF(x))λ)2ψ′(ϕ(αt(ˉF(x))λ1−ˉαt(ˉF(x))λ))]−(αs−αt)(1αs−1αt)C1(α,x)(1−(ˉF(x))λ)λh(x)B1(αses,x)B1(αtet,x)(1−ˉαs(ˉF(x))λ)(1−ˉαt(ˉF(x))λ)−(αs−αt)λh(x)(ˉF(x))λ(B1(αtet,x)(1−ˉαt(ˉF(x))λ)2−B1(αses,x)(1−ˉαs(ˉF(x))λ)2)−(αs−αt)λh(x)(ˉF(x))λ(1−(ˉF(x))λ)⋅[B1(αses,x)(C1(α,x)−C1(αses,x))(1−ˉαs(ˉF(x))λ)3ψ′(ϕ(αs(ˉF(x))λ1−ˉαs(ˉF(x))λ))−B1(αtet,x)(C1(α,x)−C1(αtet,x))(1−ˉαt(ˉF(x))λ)3ψ′(ϕ(αt(ˉF(x))λ1−ˉαt(ˉF(x))λ))]. |
As 1/h2(α) is increasing in \alpha , it holds that
\begin{eqnarray} \begin{split} &-(\alpha_s-\alpha_t) C_1(\boldsymbol \alpha,x) (\bar F(x))^{\lambda} (1-(\bar F(x))^{\lambda}) A_1(\boldsymbol \alpha_{(s,t)},x) \\ &\cdot \Bigg[\frac{1}{(1 -\bar\alpha_s(\bar F(x))^{\lambda})^2\psi'\left(\phi\left(\frac{ \alpha_s(\bar F(x))^{\lambda}}{1 -\bar \alpha_s(\bar F(x))^{\lambda}} \right)\right)}-\frac{1}{(1 -\bar\alpha_t(\bar F(x))^{\lambda})^2\psi'\left(\phi\left(\frac{\alpha_t(\bar F(x))^{\lambda}}{1 -\bar \alpha_t(\bar F(x))^{\lambda}} \right)\right)}\Bigg]\ge 0. \end{split} \end{eqnarray} | (3.1) |
Likewise, for \alpha_s \ge \alpha_t, we have
\begin{eqnarray} -(\alpha_s-\alpha_t)\left(\frac{1}{\alpha_s}-\frac{ 1 }{\alpha_t}\right)C_1(\boldsymbol \alpha,x)\frac{(1-(\bar F(x))^{\lambda})\lambda h(x) B_1(\alpha_s\mathit{\boldsymbol{e}}_s,x) B_1(\alpha_t\mathit{\boldsymbol{e}}_t,x)} {(1 -\bar\alpha_s(\bar F(x))^{\lambda})(1 -\bar\alpha_t(\bar F(x))^{\lambda})}\ge0. \end{eqnarray} | (3.2) |
Consider that {1}/{(1-\bar\alpha_i(\bar F(x))^{\lambda})^2}\ge0 is decreasing in \alpha_i , \varrho2 implies that B_1(\alpha_i\mathit{\boldsymbol{e}}_i, x) is increasing in \alpha_i , and B_1(\alpha_t\mathit{\boldsymbol{e}}_t, x)\le B_1(\alpha_s\mathit{\boldsymbol{e}}_s, x)\le 0 for \alpha_s\ge \alpha_t . Then
\begin{eqnarray} -(\alpha_s-\alpha_t) \lambda h(x)(\bar F(x))^{\lambda}\Bigg(\frac{B_1(\alpha_t\mathit{\boldsymbol{e}}_t,x)}{(1 -\bar\alpha_t(\bar F(x))^{\lambda})^2 }-\frac{B_1( \alpha_s\mathit{\boldsymbol{e}}_s,x)}{(1 -\bar\alpha_s(\bar F(x))^{\lambda}) ^2}\Bigg)\ge0. \end{eqnarray} | (3.3) |
Note that { 1}/{(1-\bar\alpha_i(\bar F(x))^{\lambda})}\ge0 is decreasing in \alpha_i and {1}/{h_2(\alpha_i)} is increasing in \alpha_i . It is clear that
\frac{1}{(1 -\bar\alpha_s(\bar F(x))^{\lambda})^3\psi'\left( \phi\left(\frac{\alpha_s(\bar F(x))^{\lambda}}{1 -\bar \alpha_s(\bar F(x))^{\lambda}} \right)\right)} |
is increasing in \alpha_i for \alpha_s\ge \alpha_t . By \varrho3 , for \alpha_s\ge \alpha_t , we have C_1(\boldsymbol \alpha, x)-C_1(\alpha_t\mathit{\boldsymbol{e}}_t, x)\ge C_1(\boldsymbol \alpha, x)- C_1(\alpha_s\mathit{\boldsymbol{e}}_s, x)\ge0. It holds that
\begin{eqnarray} \begin{split} &-(\alpha_s-\alpha_t)\lambda h(x) (\bar F(x))^{\lambda} (1-(\bar F(x))^{\lambda})\\ &\cdot\Bigg[\frac{B_1(\alpha_s\mathit{\boldsymbol{e}}_s,x)\Big(C_1(\boldsymbol \alpha,x)-C_1( \alpha_s\mathit{\boldsymbol{e}}_s,x)\Big)} {(1 -\bar\alpha_s(\bar F(x))^{\lambda})^3\psi'\left( \phi\left(\frac{\alpha_s(\bar F(x))^{\lambda}}{1 -\bar \alpha_s(\bar F(x))^{\lambda}}\right)\right) }-\frac{B_1(\alpha_t\mathit{\boldsymbol{e}}_t,x)\Big(C_1(\boldsymbol \alpha,x)-C_1( \alpha_t\mathit{\boldsymbol{e}}_t,x)\Big)} {(1 -\bar\alpha_t(\bar F(x))^{\lambda})^3\psi'\left( \phi\left(\frac{\alpha_t(\bar F(x))^{\lambda}}{1-\bar \alpha_t(\bar F(x))^{\lambda}}\right)\right)}\Bigg] \ge0. \end{split} \end{eqnarray} | (3.4) |
Combing (3.1)-(3.4), we conclude that L_1(x, \mathit{\boldsymbol{\alpha}}, {\lambda}, \psi) is Schur-convex with respect to \mathit{\boldsymbol{\alpha}} . Thus, according to Lemma 1 and Lemma 2, \mathit{\boldsymbol{\alpha}}\overset{w}\preceq \mathit{\boldsymbol{\beta}} implies h_{X_{1:n}}(x) = L_1(x, \mathit{\boldsymbol{\alpha}}, {\lambda}, \psi)\le L_1(x, \mathit{\boldsymbol{\beta}}, {\lambda}, \psi) = h_{Y_{1:n}}(x) for all x , that is, X_{1:n}\ge_{hr} Y_{1:n}.
Remark 2. For two independent samples, we have \psi(x) = e^{-{x}} , thus Theorem 3 serves as a generalization of Theorem 3.4 (ii) of [6] to the case of dependent samples with Archimedean survival copulas.
We present the following example to illustrate the result of Theorem 3.
Example 3. Let \bar F(x) = e^{-(a x)^b}, a > 0 , 0 < b\le1 , and generator \psi(x) = e^{\frac{1-e^{x}}{\theta}} , 0 < \theta\le 0.5(3-\sqrt5) . Set n = 3, \lambda = 0.03, a = 5, b = 0.007, \theta = 0.1 and \mathit{\boldsymbol{\alpha}} = (0.8, 0.6, 0.4)\overset{w}\preceq(0.7, 0.5, 0.3) = \mathit{\boldsymbol{\beta}} . One can verify that the conditions of Theorem 3 are all satisfied. Figure 4 plots the reversed hazard rate functions of {({X_{1:3}+1})^{-1}} and {({Y_{1:3}+1})^{-1}} , and it is obvious that X_{1:3}\ge_{hr}Y_{1:3} is equivalent to {({X_{1:3}+1})^{-1}}\le_{rh} ({Y_{1:3}+1})^{-1} , which asserts X_{1:3}\ge_{hr} Y_{1:3}.
In this subsection, we consider the case of MPRHR samples. Let \textbf{X} = (X_1, \dots, X_n) be the random vector, and \boldsymbol X \sim MPRHR (\mathit{\boldsymbol{\alpha}}; \mathit{\boldsymbol{\beta}}; F;\psi) , where F is the baseline distribution function, \psi is generator of the associated Archimedean copula, and \mathit{\boldsymbol{\alpha}} = (\alpha_1, \dots, \alpha_n) and \mathit{\boldsymbol{\beta}} = (\beta_1, \dots, \beta_n) are the tilt parameter vector and modified proportional reversed hazard rate vector, respectively.
Next, we present two results on the heterogeneity among parameters in terms of the usual stochastic order. The proofs can be obtained along the same way with that of Theorem 1, and thus omitted here.
Theorem 4. For \mathit{\boldsymbol{X}} \sim MPRHR(\alpha; \mathit{\boldsymbol{\beta}}; F; \psi_1) and \mathit{\boldsymbol{Y}} \sim MPRHR (\alpha; \mathit{\boldsymbol{\gamma}}; F; \psi_2) , where 0 < \alpha\le1 . If \psi_1 or \psi_2 is log-concave, and \phi_1 \circ \psi_2 is super-additive, then \mathit{\boldsymbol{\beta}}\overset{w}\preceq \mathit{\boldsymbol{\gamma}} implies
\ X_{1:n}\ge_{st} Y_{1:n}. |
Theorem 5. For \mathit{\boldsymbol{X}} \sim MPRHR (\mathit{\boldsymbol{\alpha}}; \beta; F; \psi_1) and \mathit{\boldsymbol{Y}} \sim MPRHR (\mathit{\boldsymbol{\upsilon}}; \beta; F; \psi_2) . If \psi_1 or \psi_2 is log-concave, and \phi_2 \circ \psi_1 is super-additive, then \mathit{\boldsymbol{\alpha}}\overset{w}\preceq \mathit{\boldsymbol{\upsilon}} implies
\ X_{1:n}\le_{st} Y_{1:n}. |
The next example is provided to illustrate the result of Theorem 4.
Example 4. Let \bar F(x) = e^{-(a x)^b}, a > 0 , 0 < b\le1 , and generators \psi_1(x) = (\theta_1 {x}+1)^{-1/\theta_1} , \theta_1 > 0, \; \psi_2(x) = e^{\frac{1-e^{x}}{\theta_2}} , 0 < \theta_2\le1 . Set n = 3, \alpha = 0.3, \theta_1 = 0.8, \theta_2 = 0.9, a = 0.1, b = 0.9, \; \mathit{\boldsymbol{\beta}} = (0.3, 0.3, 0.4)\overset{w}\preceq(0.2, 0.2, 0.3) = \mathit{\boldsymbol{\gamma}} . It is easy to check that all conditions of Theorem 4 are satisfied. The distribution functions of ({X_{1:3}+1})^{-1} and ({Y_{1:3}+1})^{-1} are plotted in Figure 5, from which we can confirm that \ X_{1:3}\ge_{st} Y_{1:3}.
The following theorem compares two sample minimums with common modified proportional reversed hazard rates parameters and heterogeneous tilt parameters in the sense of the hazard rate order. The proof is similar to that of Theorem 3 and hence omitted.
Theorem 6. For \mathit{\boldsymbol{X}} \sim MPRHR (\mathit{\boldsymbol{\alpha}}; \beta; F; \psi) and \mathit{\boldsymbol{Y}} \sim MPRHR (\mathit{\boldsymbol{\upsilon}}; \beta; F; \psi) with log-concave \psi , and -{\psi'}/{\psi } is log-convex. If \boldsymbol \alpha \overset{w}\preceq \boldsymbol \upsilon , then
\ X_{1:n}\le_{hr} Y_{1:n}. |
Remark 3. It is mentioned that Theorem 6 generalizes Theorem 4.5 (ii) of [6] to the case of dependent samples with Archimedean copulas.
Remark 4. According to the Theorem 2.1 of Navarro et al.[10], the coherent system reliability function can be written as \bar F_{X}(x) = h(\bar F(x)) , where h only depends on structure function and on the survival copula of (X_1, \dots, X_n) . Owing to the complexity of coherent system, we only studied the series system in Section 3 by distored distribution. Based on Archimedean copula K , we obtained the distorted function representations of series system as follows:
\begin{eqnarray} h_3(u;\boldsymbol \alpha,\boldsymbol \lambda) = K\left(\frac{\alpha_1(u)^{\lambda_1}}{1 -\bar \alpha_1(u)^{\lambda_1}},\dots,\frac{\alpha_i(u)^{\lambda_i}}{1 -\bar \alpha_i(u)^{\lambda_i}},\dots, \frac{\alpha_n(u)^{\lambda_n}}{1 -\bar \alpha_n(u)^{\lambda_n}}\right), \end{eqnarray} | (3.5) |
\begin{eqnarray} h_4(u;\boldsymbol \alpha,\boldsymbol \beta) = K\left(\frac{1-(1-u)^{\beta_1}}{1 -\bar \alpha_1(1-u)^{\beta_1}},\dots, \frac{1-(1-u)^{\beta_i}}{1 -\bar \alpha_i(1-u)^{\beta_i}},\dots, \frac{1-(1-u)^{\beta_n}}{1 -\bar \alpha_n(1-u)^{\beta_n}}\right), \quad u = \bar F(x), \end{eqnarray} | (3.6) |
where h_3(u; \boldsymbol \alpha, \boldsymbol \lambda) and h_4(u; \boldsymbol \alpha, \boldsymbol \beta) are obtained by MPHR and MPRHR models. In (3.5) , if \alpha_i = \alpha (i = 1, \dots, n), we can obtain representation of Theorem 1, when \lambda_i = \lambda (i = 1, \dots, n) , we can obtain representation of Theorem 2 and Theorem 3. As for in other Theorem 4, Theorem 5, and Theorem 6, we can obtain in similar way by (3.6) .
In parallel to the previous section, here, we consider the largest order statistics from dependent and heterogeneous MPHR and MPRHR samples.
In this subsection, we deal with the case of MPHR samples in the sense of the usual stochastic and reversed hazard rate orders. The first theorem establishes sufficient conditions for the usual stochastic order, which can be verified in a similar method with that of Theorem 1, and thus omitted for brevity.
Theorem 7. For \mathit{\boldsymbol{X}} \sim MPHR(\alpha; \mathit{\boldsymbol{\lambda}}; \bar F; \psi_1) and \mathit{\boldsymbol{Y}} \sim MPHR(\alpha; \mathit{\boldsymbol{\mu}}; \bar F; \psi_2) , where 0 < \alpha\le1 . If \psi_1 or \psi_2 is log-concave, and \phi_1 \circ \psi_2 is super-additive, then \mathit{\boldsymbol{\lambda}}\overset{w}\preceq \mathit{\boldsymbol{\mu}} implies
\ X_{n:n}\le_{st}Y_{n:n}. |
In the following, we give a numerical example to illustrate the effectiveness of Theorem 7.
Example 5. Under the setup of Example 4, set n = 3, \alpha = 0.9, \theta_1 = 0.9, \theta_2 = 2, a = 0.5, b = 0.3, \; \mathit{\boldsymbol{\lambda}} = (4, 5, 6)\overset{w}\preceq(3, 4, 5) = \mathit{\boldsymbol{\mu}} . One can check that the conditions of Theorem 7 are satisfied. The survival functions of {({X_{3:3}+1})^{-1}} and {({Y_{3:3}+1})^{-1}} are plotted in Figure 6, which verifies that F_{X_{3:3}}(x) \ge G_{Y_{3:3}}(x) . That is, \ X_{3:3}\le_{st}Y_{3:3} .
The following theorem conducts stochastic comparisons of samples with heterogeneous tilt parameters in the sense of the usual stochastic order.
Theorem 8. For \mathit{\boldsymbol{X}} \sim MPHR (\mathit{\boldsymbol{\alpha}}; \lambda; \bar F; \psi_1) and {\bf {Y}} \sim MPHR (\mathit{\boldsymbol{\beta}}; \lambda; \bar F; \psi_2) . If \psi_1 or \psi_2 is log-concave, and \phi_2 \circ \psi_1 is super-additive, then \mathit{\boldsymbol{\alpha}}\overset{w}\preceq\mathit{\boldsymbol{\beta}} implies
\ X_{n:n}\ge_{st} Y_{n:n}. |
Proof. The distribution function X_{n:n} is given by
F_{X_{n:n}}(x) = \psi_1\left(\sum\limits_{i = 1}^n\phi_1\left(\frac{1-(\bar F(x))^{\lambda}}{1 -\bar\alpha_i(\bar F(x))^{\lambda}}\right)\right) = J_3(\mathit{\boldsymbol{\alpha}},{\lambda},\psi_1,\bar F(x)),\qquad x\ge0. |
Without loss of generality, we assume that \psi_1 is log-concave. In order to obtain the desired result, we just need to prove that J_3(\mathit{\boldsymbol{\alpha}}, {\lambda}, \psi_1, \bar F(x)) is decreasing in {\alpha_i} and Schur-convex in \mathit{\boldsymbol{\alpha}} , i \in \mathcal{I}_n . Taking the partial derivative of J_3(\mathit{\boldsymbol{\alpha}}, \lambda, \psi_1, \bar F(x)) with respect to \alpha_i , we get
\begin{eqnarray*} \frac{\partial J_3(\mathit{\boldsymbol{\alpha}},{\lambda},\psi_1,\bar F(x)) }{\partial\alpha_i} = \psi_1'\left( \sum\limits_{i = 1}^n \phi_1\left(\frac{ 1-(\bar F(x))^{\lambda}} {1 -\bar \alpha_i(\bar F(x))^{\lambda}}\right)\right) \frac{-(\bar F(x))^{\lambda}\Big(\frac{ 1-(\bar F(x))^{\lambda}}{1 -\bar \alpha_i(\bar F(x))^{\lambda}}\Big) }{\psi_1'\left( \phi_1\left(\frac{ 1-(\bar F(x))^{\lambda}}{1 -\bar \alpha_i(\bar F(x))^{\lambda}}\right)\right)(1 -\bar\alpha_i(\bar F(x))^{\lambda})}\le 0. \end{eqnarray*} |
That is, J_3(\mathit{\boldsymbol{\alpha}}, {\lambda}, \psi_1, \bar F(x)) is decreasing in \alpha_i . Furthermore, for i\not = j, it holds that
\begin{eqnarray*} & & (\alpha_i-\alpha_j)\left(\frac{\partial J_3(\mathit{\boldsymbol{\alpha}},{\lambda},\psi_1,\bar F(x)) }{\partial\alpha_i}-\frac{\partial J_3(\mathit{\boldsymbol{\alpha}},{\lambda},\psi_1,\bar F(x))}{\partial\alpha_j}\right)\\ && = -\psi_1'\left(\sum\limits_{i = 1}^n \phi_1\left(\frac{1-(\bar F(x))^{\lambda}} {1-\bar \alpha_i(\bar F(x))^{\lambda}}\right)\right)(\bar F(x))^{\lambda}(\alpha_i-\alpha_j)\Big(h_3(\alpha_i)-h_3(\alpha_j)\Big), \end{eqnarray*} |
where
h_3(\alpha) = \frac{\frac{ 1-(\bar F(x))^{\lambda}}{1 -\bar \alpha(\bar F(x))^{\lambda}} }{\psi_1'\left( \phi_1\left(\frac{ 1-(\bar F(x))^{\lambda}}{1 -\bar \alpha(\bar F(x))^{\lambda}}\right)\right)(1 -\bar\alpha(\bar F(x))^{\lambda})}. |
Since the log-concavity of \psi_1 implies the increasing property of {\psi_1}/{\psi_1'} , and consider that \phi_1({1-(\bar F(x))^{\lambda}}/{(1 -\bar \alpha(\bar F(x))^{\lambda})}) is increasing in \alpha, then we have
\frac{\frac{1-(\bar F(x))^{\lambda}}{1 -\bar \alpha(\bar F(x))^{\lambda}}}{\psi_1'\left( \phi_1\left(\frac{ 1-(\bar F(x))^{\lambda}}{1 -\bar \alpha(\bar F(x))^{\lambda}} \right)\right)} = \frac{\psi_1\left( \phi_1\left(\frac{ 1-(\bar F(x))^{\lambda}}{1 -\bar \alpha(\bar F(x))^{\lambda}} \right)\right)}{\psi_1'\left( \phi_1\left(\frac{ 1-(\bar F(x))^{\lambda}}{1 -\bar \alpha(\bar F(x))^{\lambda}} \right)\right)} |
is negative and is increasing in \alpha for every fixed x\ge0 . In addition, {1}/{(1 -\bar\alpha(\bar F(x))^{\lambda})}\ge0 is decreasing in \alpha . Consequently, h_3(\alpha) is increasing in \alpha which in turn implies that
(\alpha_i-\alpha_j)\left(\frac{\partial J_3(\mathit{\boldsymbol{\alpha}},{\lambda},\psi_1,\bar F(x)) }{\partial\alpha_i}-\frac{\partial J_3(\mathit{\boldsymbol{\alpha}},{\lambda},\psi_1,\bar F(x))}{\partial\alpha_j}\right)\ge 0. |
Therefore, J_3(\mathit{\boldsymbol{\alpha}}, {\lambda}, \psi_1, \bar F(x)) is decreasing in {\alpha_i} and Schur-convex in \mathit{\boldsymbol{\alpha}}. By Lemma 2, \mathit{\boldsymbol{\alpha}}\overset{w}\preceq \mathit{\boldsymbol{\beta}} implies J_3(\mathit{\boldsymbol{\alpha}}, {\lambda}, \psi_1, \bar F(x))\le J_3(\mathit{\boldsymbol{\beta}}, {\lambda}, \psi_1, \bar F(x)), and note that \phi_2 \circ \psi_1 is super-additive, we can conclude by Lemma 3 that J_3(\mathit{\boldsymbol{\beta}}, {\lambda}, \psi_1, \bar F(x)) \le J_3(\mathit{\boldsymbol{\beta}}, {\lambda}, \psi_2, \bar F(x)) . Hence,
J_3(\mathit{\boldsymbol{\alpha}},{\lambda},\psi_1,\bar F(x))\le J_3(\mathit{\boldsymbol{\beta}},{\lambda},\psi_1,\bar F(x))\le J_3(\mathit{\boldsymbol{\beta}},{\lambda},\psi_2,\bar F(x)), |
which implies that \ X_{n:n}\ge_{st}Y_{n:n}. The proof can be completed.
The following numerical example is provided to demonstrate the theoretical result of Theorem 8.
Example 6. Let \bar F(x) = e^{-(a x)^b}, a > 0 , 0 < b\le1 , and generators \psi_1(x) = e^{-{x}} , \psi_2(x) = (\theta {x}+1)^{-1/\theta} , \theta > 0. Take n = 3, \lambda = 0.8, a = 0.5, b = 0.2, \theta = 2 and \mathit{\boldsymbol{\alpha}} = (0.3, 0.5, 0.7)\overset{w}\preceq(0.2, 0.4, 0.6) = \mathit{\boldsymbol{\beta}}. One can check chat the conditions of Theorem 8 are satisfied. The survival functions of {({X_{3:3}+1})^{-1}} and {({Y_{3:3}+1})^{-1}} are plotted in Figure 7, that is X_{3:3}\ge_{st}Y_{3:3} , which verifies the result of Theorem 8.
In the next theorem, we provide a sufficient condition to obtain the reversed hazard rate order between two modified proportional hazard rates models when the modified proportional hazard rates parameters are equal and the tilt parameters are heterogeneous.
Theorem 9. For \mathit{\boldsymbol{X}} \sim MPHR (\mathit{\boldsymbol{\alpha}}; \lambda; \bar F; \psi) and \mathit{\boldsymbol{Y}} \sim MPHR (\mathit{\boldsymbol{\beta}}; \lambda; \bar F; \psi) with log-concave \psi . Assume that -{\psi'}/{\psi} is log-convex. If \mathit{\boldsymbol{\alpha}}\overset{w}\preceq \mathit{\boldsymbol{\beta}} , then
X_{n:n}\ge_{rh}Y_{n:n}. |
Proof. The distribution function X_{n:n} is given by
\begin{eqnarray*} F_{X_{n:n}}(x) = \psi\left(\sum\limits_{i = 1}^n \phi\left(\frac{1-(\bar F(x))^{\lambda}} {1 -\bar\alpha_i(\bar F(x))^{\lambda}}\right)\right),\qquad x\ge0, \end{eqnarray*} |
and the reversed hazard rate function of X_{n:n} is
\begin{eqnarray*} r_{X_{n:n}}(x)& = &\frac{\psi'\left( \sum_{i = 1}^n \phi\left(\frac{1-(\bar F(x))^{\lambda}} {1 -\bar\alpha_i(\bar F(x))^{\lambda} } \right)\right)}{\psi\left(\sum_{i = 1}^n \phi\left(\frac{1-(\bar F(x))^{\lambda}}{1 -\bar\alpha_i(\bar F(x))^{\lambda}} \right)\right)}\sum\limits_{i = 1}^n\frac{\lambda (\bar F(x))^{\lambda} h(x)}{1-(\bar F(x))^{\lambda}}\frac{\alpha_i }{1 -\bar\alpha_i(\bar F(x))^{\lambda}}\frac{\psi\left( \phi\left(\frac{ 1-(\bar F(x))^{\lambda}}{1 -\bar \alpha_i(\bar F(x))^{\lambda}}\right )\right)}{\psi'\left(\phi\left(\frac{ 1-(\bar F(x))^{\lambda}}{1 -\bar \alpha_i(\bar F(x))^{\lambda}}\right)\right)}\\ & = &L_2(x,\mathit{\boldsymbol{\alpha}},\lambda,\psi). \end{eqnarray*} |
Similiarly, Y_{n:n} gets the reversed hazard rate function r_{Y_{n:n}}(x) = L_2(x, \mathit{\boldsymbol{\beta}}, \lambda, \psi) for x \ge 0. Further denote
A_2(\boldsymbol \alpha_{(s,t)},x) = \sum\limits_{i\neq s,t}\frac{\lambda (\bar F(x))^{\lambda} h(x)}{1-(\bar F(x))^{\lambda}}\frac{\alpha_iB_2(\alpha_i\mathit{\boldsymbol{e}}_i,x)}{1 -\bar\alpha_i(\bar F(x))^{\lambda}}, \quad B_2(\boldsymbol \alpha,x) = \frac{\psi\left(\sum_{i = 1}^n \phi\left(\frac{1-(\bar F(x))^{\lambda}}{1 -\bar\alpha_i(\bar F(x))^{\lambda}} \right)\right)}{\psi'\left(\sum_{i = 1}^n \phi\left(\frac{1-(\bar F(x))^{\lambda}}{1 -\bar\alpha_i(\bar F(x))^{\lambda}} \right)\right)}, |
J_{\psi}(x) = \frac{\psi''(x)\psi(x)-(\psi'(x))^2} {(\psi(x))^2},\quad C_2(\boldsymbol \alpha,x) = J_{\psi}\left(\sum\limits_{i = 1}^n \phi\left(\frac{1-(\bar F(x))^{\lambda}}{1 -\bar \alpha_i(\bar F(x))^{\lambda}}\right)\right) B_2(\boldsymbol \alpha,x). |
Note that, for s, t \in \mathcal I_n with s\not = t , and u_i\in[0, 1] ( i\in \mathcal I_n ), we have
\begin{eqnarray*} &&\frac {\partial L_2(x,\mathit{\boldsymbol{\alpha}},{\lambda},\psi)}{\partial \alpha_s}\\ & = &\frac{(\bar F(x))^{\lambda}((\bar F(x))^{\lambda}-1)}{(1 -\bar\alpha_s(\bar F(x))^{\lambda}) ^2\psi'\left( \phi\left(\frac{1-(\bar F(x))^{\lambda}} {1 -\bar\alpha_s(\bar F(x))^{\lambda}}\right )\right)}\sum\limits_{i = 1}^n\frac{\lambda (\bar F(x))^{\lambda}h(x)}{1-(\bar F(x))^{\lambda}}\frac{\alpha_i B_2( \alpha_i\mathit{\boldsymbol{e}}_i,x)}{1 -\bar\alpha_i(\bar F(x))^{\lambda}} J_{\psi}\left(\sum\limits_{i = 1}^n \phi\left(\frac{ 1-(\bar F(x))^{\lambda}}{1 -\bar \alpha_i(\bar F(x))^{\lambda}} \right)\right)\\ &&+\frac{B_2(\alpha_s\mathit{\boldsymbol{e}}_s,x)}{B_2(\boldsymbol \alpha,x)} \frac{\lambda h(x)(\bar F(x))^{\lambda}}{(1 -\bar\alpha_s(\bar F(x))^{\lambda})^2}\\ &&-\frac{B_2^2(\alpha_s\mathit{\boldsymbol{e}}_s,x)}{B_2(\boldsymbol \alpha,x)}\frac{(\bar F(x))^{\lambda} ((\bar F(x))^{\lambda}-1) }{(1 -\bar\alpha_s(\bar F(x))^{\lambda}) ^3\psi'\left(\phi\left(\frac{1-(\bar F(x))^{\lambda}} {1 -\bar\alpha_s(\bar F(x))^{\lambda}}\right)\right)} \frac{\alpha_s \lambda h(x) (\bar F(x))^{\lambda}}{(1-(\bar F(x))^{\lambda})}J_{\psi}\left(\phi\left(\frac{ 1-(\bar F(x))^{\lambda}}{1 -\bar \alpha_s(\bar F(x))^{\lambda}} \right)\right) \\ & = &\Bigg[\frac{(\bar F(x))^{\lambda} ((\bar F(x))^{\lambda}-1) A_2(\boldsymbol \alpha_{(s,t)},x)}{(1 -\bar\alpha_s(\bar F(x))^{\lambda}) ^2\psi'\left(\phi\left(\frac{1-(\bar F(x))^{\lambda}}{1 -\bar\alpha_s(\bar F(x))^{\lambda} } \right)\right)}+ \frac{(\bar F(x))^{\lambda}((\bar F(x))^{\lambda}-1)\alpha_s B_2( \alpha_s\mathit{\boldsymbol{e}}_s,x)}{(1 -\bar\alpha_s(\bar F(x))^{\lambda}) ^3\psi'\left(\phi\left(\frac{1-(\bar F(x))^{\lambda}} {1 -\bar\alpha_s(\bar F(x))^{\lambda} }\right )\right)}\frac{\lambda (\bar F(x))^{\lambda} h(x) }{1-(\bar F(x))^{\lambda}}\\ & &+\frac{(\bar F(x))^{\lambda}((\bar F(x))^{\lambda}-1)}{(1 -\bar\alpha_s(\bar F(x))^{\lambda}) ^2\psi'\left( \phi\left(\frac{1-(\bar F(x))^{\lambda}}{1 -\bar\alpha_s(\bar F(x))^{\lambda}} \right)\right)}\frac{\lambda (\bar F(x))^{\lambda} h(x)}{1-(\bar F(x))^{\lambda}}\frac{\alpha_tB_2( \alpha_t\mathit{\boldsymbol{e}}_t,x)}{1 -\bar\alpha_t(\bar F(x))^{\lambda}} \Bigg]J_{\psi} \left(\sum\limits_{i = 1}^n \phi\left(\frac{1-(\bar F(x))^{\lambda}}{1 -\bar \alpha_i(\bar F(x))^{\lambda}}\right )\right) \\ & &+\frac{B_2(\alpha_s\mathit{\boldsymbol{e}}_s,x)}{B_2(\boldsymbol \alpha,x)} \frac{\lambda h(x) (\bar F(x))^{\lambda}}{(1 -\bar\alpha_s(\bar F(x))^{\lambda})^2}\\ & & -\frac{B_2( \alpha_s\mathit{\boldsymbol{e}}_s,x)C_2( \alpha_s\mathit{\boldsymbol{e}}_s,x)}{B_2(\boldsymbol \alpha,x)}\frac{(\bar F(x))^{\lambda} ((\bar F(x))^{\lambda}-1)}{(1 -\bar\alpha_s(\bar F(x))^{\lambda})^3\psi'\left(\phi\left(\frac{1-(\bar F(x))^{\lambda}}{1 -\bar\alpha_s(\bar F(x))^{\lambda}}\right )\right)}\frac{\alpha_s \lambda h(x) (\bar F(x))^{\lambda} }{(1-(\bar F(x))^{\lambda})}. \end{eqnarray*} |
By \varrho1 , \varrho2 and C_1(\boldsymbol \alpha, x)\ge C_1(\alpha_s\mathit{\boldsymbol{e}}_s, x) , it holds that
\begin{eqnarray*} &&\frac{\partial L_2(x,\mathit{\boldsymbol{\alpha}},{\lambda},\psi)} {\partial \alpha_s}\\ & = &\Bigg[\frac{ (\bar F(x))^{\lambda} ((\bar F(x))^{\lambda}-1) A_2(\boldsymbol \alpha_{(s,t)},x)}{(1 -\bar\alpha_s(\bar F(x))^{\lambda}) ^2\psi'\left(\phi\left(\frac{1-(\bar F(x))^{\lambda}}{1 -\bar\alpha_s(\bar F(x))^{\lambda} }\right )\right)}+\frac{ (\bar F(x))^{\lambda} ((\bar F(x))^{\lambda}-1) }{(1 -\bar\alpha_s(\bar F(x))^{\lambda}) ^2\psi'\left(\phi\left(\frac{1-(\bar F(x))^{\lambda}} {1 -\bar\alpha_s(\bar F(x))^{\lambda}}\right)\right)} \frac{\lambda (\bar F(x))^{\lambda}h(x) }{(1-(\bar F(x))^{\lambda})}\\ &&\cdot \frac{\alpha_tB_2( \alpha_t\mathit{\boldsymbol{e}}_t,x)}{(1 -\bar\alpha_t(\bar F(x))^{\lambda})}\Bigg] J_{\psi} \left(\sum\limits_{i = 1}^n \phi\left(\frac{ 1-(\bar F(x))^{\lambda}}{1 -\bar \alpha_i(\bar F(x))^{\lambda}}\right )\right) +\frac{B_2( \alpha_s\mathit{\boldsymbol{e}}_s,x)}{B_2(\boldsymbol \alpha,x)}\frac{\lambda h(x) (\bar F(x))^{\lambda}}{(1 -\bar\alpha_s(\bar F(x))^{\lambda})^2}\\ &&+\frac{(\bar F(x))^{\lambda}((\bar F(x))^{\lambda}-1)}{(1 -\bar\alpha_s(\bar F(x))^{\lambda}) ^3\psi'\left(\phi\left(\frac{1-(\bar F(x))^{\lambda}} {1 -\bar\alpha_s(\bar F(x))^{\lambda}}\right)\right)} \frac{\alpha_s \lambda h(x) (\bar F(x))^{\lambda} }{(1-(\bar F(x))^{\lambda})}\frac{B_2(\alpha_s\mathit{\boldsymbol{e}}_sx)\Big(C_2(\boldsymbol \alpha,x)-C_2( \alpha_s\mathit{\boldsymbol{e}}_s,x)\Big)}{B_2(\boldsymbol \alpha,x)} \ge0. \end{eqnarray*} |
As a result, L_2(x, \mathit{\boldsymbol{\alpha}}, \lambda, \psi) is increasing in \alpha_i, for any i\in\mathcal I_n , and for s, t \in \mathcal I_n with s\not = t ,
\begin{eqnarray*} & &(\alpha _s-\alpha _t)\left(\frac{\partial L_2(x,\mathit{\boldsymbol{\alpha}},{\lambda},\psi)} {\partial \alpha_s}-\frac {\partial L_2(x,\mathit{\boldsymbol{\alpha}},{\lambda},\psi)} {\partial \alpha_t}\right)\\ & = &-(\alpha _s-\alpha _t)J_{\psi} \left(\sum\limits_{i = 1}^n \phi\left(\frac{1-(\bar F(x))^{\lambda}}{1 -\bar \alpha_i(\bar F(x))^{\lambda}}\right)\right)(\bar F(x))^{\lambda} A_1(\boldsymbol \alpha_{(s,t)},x)\\ & & \cdot \Bigg[\frac{(1-(\bar F(x))^{\lambda})} {(1 -\bar\alpha_s(\bar F(x))^{\lambda})^2\psi'\left( \phi\left(\frac{ 1-(\bar F(x))^{\lambda}}{1 -\bar \alpha_s(\bar F(x))^{\lambda}}\right)\right)}-\frac{(1-(\bar F(x))^{\lambda})} {(1 -\bar\alpha_t(\bar F(x))^{\lambda})^2\psi'\left( \phi\left(\frac{ 1-(\bar F(x))^{\lambda}}{1 -\bar \alpha_t(\bar F(x))^{\lambda}}\right )\right) }\Bigg]\\ & & +(\alpha_s-\alpha_t)^2J_{\psi} \left(\sum\limits_{i = 1}^n \phi\left(\frac{1-(\bar F(x))^{\lambda}}{1 -\bar \alpha_i(\bar F(x))^{\lambda}}\right)\right) \frac{\lambda (\bar F(x))^{2\lambda} h(x)}{1-(\bar F(x))^{\lambda}} \frac{B_2(\alpha_s\mathit{\boldsymbol{e}}_s,x) B_2(\alpha_t\mathit{\boldsymbol{e}}_t,x)} {(1 -\bar\alpha_s(\bar F(x))^{\lambda})(1 -\bar\alpha_t(\bar F(x))^{\lambda})}\\ & &-(\alpha_s-\alpha_t)\frac{\lambda h(x) (\bar F(x))^{\lambda} }{B_2(\boldsymbol \alpha,x)}\Bigg(\frac{B_2(\alpha_t\mathit{\boldsymbol{e}}_t,x)}{(1 -\bar\alpha_t(\bar F(x))^{\lambda})^2 }-\frac{B_2(\alpha_s\mathit{\boldsymbol{e}}_s,x)}{(1 -\bar\alpha_s(\bar F(x))^{\lambda})^2}\Bigg)\\ & &+(\alpha_s-\alpha_t)\frac{\lambda h(x)(\bar F(x))^{2\lambda}((\bar F(x))^{\lambda}-1)}{(1-(\bar F(x))^{\lambda})B_2(\boldsymbol \alpha,x)}\\ && \cdot\Bigg[\frac{\alpha_s B_2(\alpha_s\mathit{\boldsymbol{e}}_s,x)\Big(C_2(\boldsymbol \alpha,x)-C_2(\alpha_s\mathit{\boldsymbol{e}}_s ,x)\Big)}{(1 -\bar\alpha_s(\bar F(x))^{\lambda})^3\psi'\left(\phi\left(\frac{ 1-(\bar F(x))^{\lambda}}{1 -\bar \alpha_s(\bar F(x))^{\lambda}}\right)\right)} -\frac{\alpha_t B_2(\alpha_t\mathit{\boldsymbol{e}}_t,x)\Big(C_2(\boldsymbol \alpha,x)-C_2( \alpha_t\mathit{\boldsymbol{e}}_t,x)\Big)}{(1 -\bar\alpha_t(\bar F(x))^{\lambda})^3\psi'\left( \phi\left(\frac{ 1-(\bar F(x))^{\lambda}}{1 -\bar\alpha_t(\bar F(x))^{\lambda}}\right)\right)}\Bigg]\\ &\overset{sgn} = &(\alpha_s-\alpha_t) C_2(\boldsymbol \alpha,x)(\bar F(x))^{\lambda} A_2(\boldsymbol \alpha_{(s,t)},x) \\ & &\cdot\Bigg[\frac{(1-(\bar F(x))^{\lambda})} {(1-\bar\alpha_s(\bar F(x))^{\lambda})^2\psi'\left( \phi\left(\frac{ 1-(\bar F(x))^{\lambda}}{1 -\bar \alpha_s(\bar F(x))^{\lambda}}\right)\right)}-\frac{(1-(\bar F(x))^{\lambda})} {(1 -\bar\alpha_t(\bar F(x))^{\lambda})^2\psi'\left( \phi\left(\frac{ 1-(\bar F(x))^{\lambda}}{1 -\bar \alpha_t(\bar F(x))^{\lambda}}\right)\right)}\Bigg] \\ & & -(\alpha_s-\alpha_t)^2 C_2(\boldsymbol \alpha,x) \frac{\lambda (\bar F(x))^{2\lambda} h(x)}{1-(\bar F(x))^{\lambda}} \frac{B_2(\alpha_s\mathit{\boldsymbol{e}}_s,x) B_2(\alpha_t\mathit{\boldsymbol{e}}_t,x)} {(1 -\bar\alpha_s(\bar F(x))^{\lambda})(1 -\bar\alpha_t(\bar F(x))^{\lambda})}\\ &&+(\alpha_s-\alpha_t) \lambda h(x)(\bar F(x))^{\lambda} \Bigg(\frac{B_2(\alpha_t\mathit{\boldsymbol{e}}_t,x)}{(1 -\bar\alpha_t(\bar F(x))^{\lambda})^2}-\frac{B_2(\alpha_s\mathit{\boldsymbol{e}}_s,x)}{(1 -\bar\alpha_s(\bar F(x))^{\lambda})^2}\Bigg)\\ & &+(\alpha_s-\alpha_t)\frac{\lambda h(x)(\bar F(x))^{2\lambda}(1-(\bar F(x))^{\lambda})}{(1-(\bar F(x))^{\lambda})}\\ & & \cdot\Bigg[\frac{\alpha_s B_2(\alpha_s\mathit{\boldsymbol{e}}_s,x)\Big(C_2(\boldsymbol \alpha,x)-C_2( \alpha_s\mathit{\boldsymbol{e}}_s ,x)\Big)}{(1 -\bar\alpha_s(\bar F(x))^{\lambda})^3\psi'\left( \phi\left(\frac{ 1-(\bar F(x))^{\lambda}}{1 -\bar \alpha_s(\bar F(x))^{\lambda}}\right)\right)} -\frac{\alpha_t B_2(\alpha_t\mathit{\boldsymbol{e}}_t,x)\Big(C_2(\boldsymbol \alpha,x)-C_2( \alpha_t\mathit{\boldsymbol{e}}_t,x)\Big)}{(1 -\bar\alpha_t(\bar F(x))^{\lambda})^3\psi'\left(\phi\left(\frac{1-(\bar F(x))^{\lambda}}{1-\bar \alpha_t(\bar F(x))^{\lambda}}\right )\right)}\Bigg]. \end{eqnarray*} |
Since h_3(\alpha) is increasing in \alpha , it holds that
\begin{eqnarray} \begin{split} &(\alpha_s-\alpha_t) C_2(\boldsymbol \alpha,x) (\bar F(x))^{\lambda} A_2(\boldsymbol \alpha_{(s,t)},x)\\ &\cdot\Bigg[\frac{(1-(\bar F(x))^{\lambda})} {(1 -\bar\alpha_s(\bar F(x))^{\lambda})^2\psi'\left( \phi\left(\frac{1-(\bar F(x))^{\lambda}}{1 -\bar \alpha_s(\bar F(x))^{\lambda}}\right)\right)}-\frac{(1-(\bar F(x))^{\lambda}) }{(1 -\bar\alpha_t(\bar F(x))^{\lambda})^2\psi'\left( \phi\left(\frac{1-(\bar F(x))^{\lambda}}{1 -\bar \alpha_t(\bar F(x))^{\lambda}}\right)\right)}\Bigg]\le0. \end{split} \end{eqnarray} | (4.1) |
Obviously, for \alpha_s\ge \alpha_t,
\begin{eqnarray} \begin{split} -(\alpha_s-\alpha_t)^2 C_2(\boldsymbol \alpha,x) \frac{\lambda (\bar F(x))^{2\lambda} h(x)}{1-(\bar F(x))^{\lambda}} \frac{B_2(\alpha_s\mathit{\boldsymbol{e}}_s,x) B_2(\alpha_t\mathit{\boldsymbol{e}}_t,x)} {(1 -\bar\alpha_s(\bar F(x))^{\lambda})(1 -\bar\alpha_t(\bar F(x))^{\lambda})}\le 0. \end{split} \end{eqnarray} | (4.2) |
Note that {1}/{(1-\bar\alpha_i(\bar F(x))^{\lambda})^2}\ge0 is decreasing in \alpha_i , \varrho2 implies that B_1(\alpha_i\mathit{\boldsymbol{e}}_i, x) is increasing in \alpha_i , and B_1(\alpha_t\mathit{\boldsymbol{e}}_t, x)\le B_1(\alpha_s\mathit{\boldsymbol{e}}_s, x)\le 0 for \alpha_s\ge \alpha_t . Then, we have
\begin{eqnarray} \begin{split} (\alpha_s-\alpha_t) \lambda h(x)(\bar F(x))^{\lambda} \Bigg(\frac{B_2(\alpha_t\mathit{\boldsymbol{e}}_t,x) }{(1 -\bar\alpha_t(\bar F(x))^{\lambda})^2}-\frac{B_2(\alpha_s\mathit{\boldsymbol{e}}_s,x) }{(1 -\bar\alpha_s(\bar F(x))^{\lambda})^2}\Bigg)\le 0. \end{split} \end{eqnarray} | (4.3) |
Since {\alpha_i}/{(1-\bar\alpha_i(\bar F(x))^{\lambda})} and {h_3(\alpha_i)} are increasing in \alpha_i , and by \varrho3 , we have C_1(\boldsymbol \alpha, x)-C_1(\alpha_t\mathit{\boldsymbol{e}}_t, x)\ge C_1(\boldsymbol \alpha, x)- C_1(\alpha_s\mathit{\boldsymbol{e}}_s, x)\ge0 for \alpha_s\ge \alpha_t . It is verified that
\begin{eqnarray} \begin{split} &(\alpha_s-\alpha_t)\frac{\lambda h(x) (\bar F(x))^{2\lambda}(1-(\bar F(x))^{\lambda})}{(1-(\bar F(x))^{\lambda})}\\ &\cdot\Bigg[\frac{\alpha_s B_2(\alpha_s\mathit{\boldsymbol{e}}_s,x)\Big(C_2(\boldsymbol \alpha,x)-C_2( \alpha_s\mathit{\boldsymbol{e}}_s ,x)\Big)}{(1 -\bar\alpha_s(\bar F(x))^{\lambda})^3\psi'\left( \phi\left(\frac{ 1-(\bar F(x))^{\lambda}}{1 -\bar \alpha_s(\bar F(x))^{\lambda}}\right)\right)} -\frac{\alpha_t B_2(\alpha_t\mathit{\boldsymbol{e}}_t,x)\Big(C_2(\boldsymbol \alpha,x)-C_2( \alpha_t\mathit{\boldsymbol{e}}_t,x)\Big)}{(1 -\bar\alpha_t(\bar F(x))^{\lambda})^3\psi'\left(\phi\left(\frac{1-(\bar F(x))^{\lambda}}{1-\bar \alpha_t(\bar F(x))^{\lambda}}\right )\right)}\Bigg]\le0. \end{split} \end{eqnarray} | (4.4) |
By (4.1)-(4.4), it is plain that L_2(x, \mathit{\boldsymbol{\alpha}}, \lambda, \psi) is increasing in \alpha_i and Schur-concave with respect to \mathit{\boldsymbol{\alpha}}. According to Lemma 1 and Lemma 2, \mathit{\boldsymbol{\alpha}}\overset{w}\preceq \mathit{\boldsymbol{\beta}} implies -r_{X_{n:n}}(x) = -L_2(x, \mathit{\boldsymbol{\alpha}}, {\lambda}, \psi) \le -L_2(x, \mathit{\boldsymbol{\beta}}, {\lambda}, \psi) = -r_{Y_{n:n}}(x) for all x , that is, \ X_{n:n}\ge_{rh} Y_{n:n}. This completes the proof.
Remark 5. Theorem 9 extends Theorem 3.4 (i) of [6] to the case of dependent samples with Archimedean survival copulas.
The following example illustrates the result of Theorem 9.
Example 7. Let \bar F = e^{-(a x)^b}, a > 0, 0 < b\le 1 , and \psi(x) = e^{\frac{1-e^{x}}{\theta}} , 0 < \theta\le 0.5(3-\sqrt5). Set n = 3, \lambda = 3, a = 6, b = 0.08, \theta = 0.3 and \mathit{\boldsymbol{\alpha}} = (0.8, 0.6, 0.4)\overset{w}\preceq(0.7, 0.5, 0.3) = \mathit{\boldsymbol{\beta}} . These satisfy all conditions of Theorem 9, and the hazard rate functions of {({X_{3:3}+1})^{-1}} and {({Y_{3:3}+1})^{-1}} are displayed in Figure 8, which confirms that \ X_{3:3}\ge_{rh} Y_{3:3}.
In this subsection, we consider the case of MPRHR samples. The first two theorems present the comparison results on the heterogeneity among parameters in terms of the usual stochastic order. The proofs can be completed in a similar way with that of Theorem 1, and thus are omitted.
Theorem 10. For \mathit{\boldsymbol{X}} \sim MPRHR(\alpha; \mathit{\boldsymbol{\beta}}; F; \psi_1) and \mathit{\boldsymbol{Y}} \sim MPRHR(\alpha; \mathit{\boldsymbol{\gamma}}; F; \psi_2) , where 0 < \alpha\le1 . If \psi_1 or \psi_2 is log-concave, and \phi_2 \circ \psi_1 is super-additive, then \mathit{\boldsymbol{\beta}}\overset{w}\preceq \mathit{\boldsymbol{\gamma}} implies
\begin{eqnarray*} X_{n:n}\ge_{st} Y_{n:n}. \end{eqnarray*} |
Proof. The distribution function of X_{n:n} can be represented as
F_{X_{n:n}}(x) = \psi_1\left(\sum\limits_{i = 1}^n\phi_1(\frac{\alpha( F(x))^{\beta_i}}{1 -\bar\alpha(F(x))^{\beta_i}})\right) = J_4({\alpha},\mathit{\boldsymbol{\beta}},\psi_1, F(x)), \quad x\ge0. |
Suppose that \psi_1 is log-concave. To establish the desired result, along with Lemma 2, it suffices to show that for fixed x\ge0 and 0 < \alpha\le1 . J_4({\alpha}, \mathit{\boldsymbol{\beta}}, \psi_1, F(x)) is decreasing in {\beta_i} and Schur-convex in \mathit{\boldsymbol{\beta}} . The partial derivative of J_4({\alpha}, \mathit{\boldsymbol{\beta}}, \psi_1, F(x)) with respect to {\beta_i} is
\begin{eqnarray*} \frac{\partial J_4({\alpha},\mathit{\boldsymbol{\beta}},\psi_1, F(x)) }{\partial\beta_i} = \psi_1'\left(\sum\limits_{i = 1}^n \phi_1\left(\frac{ \alpha( F(x))^{\beta_i}}{1 -\bar \alpha( F(x))^{\beta_i}}\right)\right)\frac{\ln F(x)\frac{ \alpha( F(x))^{\beta_i}}{1-\bar \alpha( F(x))^{\beta_i}}}{\psi_1'\left(\phi_1\left(\frac{ \alpha(F(x))^{\beta_i}}{1 -\bar \alpha( F(x))^{\beta_i}}\right)\right)(1-\bar\alpha( F(x))^{\beta_i})}\le 0. \end{eqnarray*} |
Which clearly shows that J_4({\alpha}, \mathit{\boldsymbol{\beta}}, \psi_1, F(x)) is decreasing in \beta_i . Now, for i\not = j, we get
\begin{eqnarray*} &&(\beta_i-\beta_j)\left (\frac{\partial J_4({\alpha},\mathit{\boldsymbol{\beta}},\psi_1, F(x)) }{\partial\beta_i}-\frac{\partial J_4({\alpha},\mathit{\boldsymbol{\beta}},\psi_1, F(x))}{\partial\beta_j}\right) \\ && = \psi'_1\left(\sum\limits_{i = 1}^n\phi_1\left(\frac{\alpha( F(x))^{\beta_i}}{1 -\bar \alpha( F(x))^{\beta_i}}\right)\right)\ln F(x)(\beta_i-\beta_j)\Big(h_4(\beta_i)-h_4(\beta_j)\Big), \end{eqnarray*} |
where
h_4(\beta) = \frac{\frac{ \alpha( F(x))^{\beta}}{1 -\bar \alpha( F(x))^{\beta}} }{\psi_1'\left(\phi_1\left(\frac{\alpha( F(x))^{\beta}}{1 -\bar \alpha( F(x))^{\beta}}\right)\right)(1 -\bar\alpha( F(x))^{\beta})}. |
As dicussed in the proof of Theorem 1, for each fixded x > 0 , we have
\frac{\frac{\alpha( F(x))^{\beta}}{1 -\bar\alpha( F(x))^{\beta}}}{\psi_1'\left(\phi_1\left(\frac{\alpha( F(x))^{\beta}}{1 -\bar\alpha( F(x))^{\beta}}\right)\right)} = \frac{\psi_1\left( \phi_1\left(\frac{ \alpha( F(x))^{\beta}}{1 -\bar\alpha( F(x))^{\beta}}\right)\right)}{\psi_1'\left( \phi_1\left(\frac{ \alpha( F(x))^{\beta}}{1 -\bar \alpha( F(x))^{\beta}}\right)\right)}\le0. |
Moreover, we readily observe that {1}/{(1 -\bar\alpha(F(x))^{\beta})} is nonnegative and decreasing in \beta for 0 < \alpha\le1 . Upon combining these observations, we find h_4(\beta) to be increasing in \beta . Consequently
(\beta_i-\beta_j)\left (\frac{\partial J_4({\alpha},\mathit{\boldsymbol{\beta}},\psi_1, F(x)) }{\partial\beta_i}-\frac{\partial J_4({\alpha},\mathit{\boldsymbol{\beta}},\psi_1, F(x))}{\partial\beta_j}\right) \ge0. |
Therefore, Schur-convexity of J_4({\alpha}, \mathit{\boldsymbol{\beta}}, \psi_1, F(x)) follows from Lemma 1. According to Lemma 2, \mathit{\boldsymbol{\beta}}\overset{w}\preceq \mathit{\boldsymbol{\gamma}} implies J_4({\alpha}, \mathit{\boldsymbol{\beta}}, \psi_1, F(x)) \le J_4({\alpha}, \mathit{\boldsymbol{\gamma}}, \psi_1, F(x)) , and the assumption \phi_2 \circ \psi_1 is super-additive, by Lemma 3, we have J_4({\alpha}, \mathit{\boldsymbol{\gamma}}, \psi_1, F(x)) \le J_4({\alpha}, \mathit{\boldsymbol{\gamma}}, \psi_2, F(x)) . So, we obtain
J_4({\alpha},\mathit{\boldsymbol{\beta}},\psi_1, F(x)) \le J_4({\alpha},\mathit{\boldsymbol{\gamma}},\psi_1, F(x))\le J_4({\alpha},\mathit{\boldsymbol{\gamma}},\psi_2, F(x)). |
It is clear that we conclude F_{X_{n:n}}(x)\le F_{Y_{n:n}}(x) , that is, \ X_{n:n}\ge_{st}Y_{n:n}. For the case of \psi_2 is log-concave, the proof can be obtained in a similar way. This completes the desired proof.
Remark 6. Theorem 10 generalizes the result of Theorem 5.1 (ii) of [21] to the case of MPHR model.
Theorem 11. For \mathit{\boldsymbol{X}} \sim MPRHR (\mathit{\boldsymbol{\alpha}}; \beta; F; \psi_1) and \mathit{\boldsymbol{Y}} \sim MPRHR (\mathit{\boldsymbol{\upsilon}}; \beta; F; \psi_2) . If \phi_1 \circ \psi_2 is super-additive, then \mathit{\boldsymbol{\alpha}}\overset{w}\preceq \mathit{\boldsymbol{\upsilon}} implies
\begin{eqnarray*} X_{n:n}\le_{st}Y_{n:n}. \end{eqnarray*} |
The following example is provided to illustrate the Theorem 11.
Example 8. Under the setup of Example 2, take n = 3, \lambda = 0.8, a = 0.5, b = 0.4, \theta = 0.6 and \mathit{\boldsymbol{\alpha}} = (0.4, 0.5, 0.6)\overset{w}\preceq(0.3, 0.4, 0.5) = \mathit{\boldsymbol{\upsilon}}. These satisfy all conditions of Theorem 11, and the survival functions of {({X_{3:3}+1})^{-1}} and {({Y_{3:3}+1})^{-1}} are poltted in Figure 9, which asserts {X_{3:3}} \le_{st}{Y_{3:3}} .
Now, we present comparison result of sample having common modified proportional reversed hazard rates parameters and heterogeneous tilt parameters in terms of the reversed hazard rate order.
Theorem 12. For \mathit{\boldsymbol{X}} \sim MPRHR (\mathit{\boldsymbol{\alpha}}; \beta; F; \psi) and \mathit{\boldsymbol{Y}} \sim MPRHR (\mathit{\boldsymbol{\upsilon}}; \beta; F; \psi) with log-concave \psi , and -{\psi'}/{\psi} is log-convex. If \boldsymbol \alpha \overset{w}\preceq \boldsymbol \upsilon , then
\begin{eqnarray*} X_{n:n}\le_{rh} Y_{n:n}. \end{eqnarray*} |
Remark 7. It should be pointed out that Theorem 12 extends Theorem 4.5 (i) of [6] to the case of dependent samples with Archimedean copulas.
Remark 8. Similarly, according to the Remark 2, based on Archimedean copula K , we obtained the distorted function representations of parallel system as follows:
\begin{eqnarray} h_5(u;\boldsymbol \alpha,\boldsymbol \lambda) = K\left(\frac{1-(1-u)^{\lambda_1}}{1 -\bar \alpha_1(1-u)^{\lambda_1}},\dots,\frac{1-(1-u)^{\lambda_i}}{1 -\bar \alpha_i(1-u)^{\lambda_i}},\dots, \frac{1-(1-u)^{\lambda_n}}{1 -\bar \alpha_n(1-u)^{\lambda_n}}\right), \end{eqnarray} | (4.5) |
\begin{eqnarray} h_6(u;\boldsymbol \alpha,\boldsymbol \beta) = K\left(\frac{\alpha_1(u)^{\beta_1}}{1 -\bar \alpha_1(u)^{\beta_1}},\dots,\frac{\alpha_i(u)^{\beta_i}}{1 -\bar \alpha_i(u)^{\beta_i}},\dots, \frac{\alpha_n(u)^{\beta_n}}{1 -\bar \alpha_n(u)^{\beta_n}}\right),\quad u = \bar F(x), \end{eqnarray} | (4.6) |
where h_5(u; \boldsymbol \alpha, \boldsymbol \lambda) and h_6(u; \boldsymbol \alpha, \boldsymbol \beta) are obtained by MPHR and MPRHR models. In (4.5) , when \alpha_i = \alpha(i = 1, \dots, n), we can obtain representation of Theorem 7, if \lambda_i = \lambda (i = 1, \dots, n) , we can obtain representation of Theorem 8 and Theorem 9. As for in other Theorem 10, Theorem 11, and Theorem 12, we can obtain in similar way by (4.6) .
In this paper, we study stochastic comparisons on minimums and maximums from heterogeneous MPRHR (MPHR) samples with Archimedean (survival) copulas. Some ordering results are established for the usual stochastic, hazard rate and reversed rate orders on the smallest and largest order statistics. These results generalize some known results in the literature. As a further study, it is of interest to consider other stochastic orders such as likelihood ratio order, star order and dispersive order.
The authors would like to thank the two anonymous reviewers and the Editor for their valuable and constructive comments and suggestions to improve the presentation of this manuscript. This research was supported by the National Natural Science Foundation of China (11861058, 71471148).
The authors declare no conflict of interest.
[1] | N. L. Johnson, S. Kotz, N. Balakrishnan, Continuous univariate distributions-Vol.1, 2 Eds., New York: John Wiley & Sons, 1994. |
[2] | N. L. Johnson, S. Kotz, N. Balakrishnan, Continuous univariate distributions-Vol.2, 2 Eds., New York: John Wiley & Sons, 1995. |
[3] |
G. S. Mudholkar, D. K. Srivastava, Exponentiated Weibull family for analyzing bathtub failure-rate data, IIEEE Trans. Reliab., 42 (1993), 299-302. doi: 10.1109/24.229504
![]() |
[4] | A. W. Marshall, I. Olkin, A new method for adding a parameter to a family of distributions with application to the exponential and Weibull families, Biometrika, 84 (1997), 641-652. |
[5] | A. W. Marshall, I. Olkin, Life distributions, New York: Springer, 2007. |
[6] |
N. Balakrishnan, G. Barmalzan, A. Haidari, Modified proportional hazard rates and proportional reversed hazard rates models via Marshall-Olkin distribution and some stochastic comparisons, J. Korean Stat. Soc., 47 (2018), 127-138. doi: 10.1016/j.jkss.2017.12.003
![]() |
[7] | N. Balakrishnan, N. Torrado, Comparisons between largest order statistics from multiple-outlier models, Statistics, 50 (2016), 176-189. |
[8] |
J. Navarro, N. Torrado, Y. D. Aguila, Comparisons between largest order statistics from multipleoutlier models with dependence, Methodol. Comput. Appl. Probab., 20 (2018), 411-433. doi: 10.1007/s11009-017-9562-7
![]() |
[9] |
G. Barmalzan, S. M. Ayat, N. Balakrishnan, R. Roozegar, Stochastic comparisons of series and parallel systems with dependent heterogeneous extended exponential components under Archimedean copula, J. Comput. Appl. Math., 380 (2020), 112965. doi: 10.1016/j.cam.2020.112965
![]() |
[10] | M. E. Ghitany, Marshall-Olkin extended Pareto distribution and its application, International Journal of Applied Mathematics, 18 (2005), 17-32. |
[11] | M. E. Ghitany, F. A. Al-Awadhi, L. A. Al-khalfan, Marshall-Olkin extended Lomax distribution and its application to censored data, Commun. Stat.-Theory Methods, 36 (2007), 1855-1866. |
[12] |
R. Fang, X. H. Li, Advertising a second-price auction, J. Math. Econ., 61 (2015), 246-252. doi: 10.1016/j.jmateco.2015.04.003
![]() |
[13] |
F. Belzunce, S. Gurler, J. M. Ruiz, Revisiting multivariate likelihood ratio ordering results for order statistics, Probab. Eng. Inform. Sci., 25 (2011), 355-368. doi: 10.1017/S0269964811000052
![]() |
[14] | J. Navarro, Y. D. Águila, M. A. Sordo, A. Suarez-Llorens, Stochastic ordering properties for systems with dependent identically distributed components, Appl. Stoch. Models Bus. Ind., 29 (2012), 264-278. |
[15] |
N. Balakrishnan, P. Zhao, Ordering properties of order statistics from heterogeneous populations: A review with an emphasis on some recent development, Probab. Eng. Inform. Sci., 27 (2013), 403-443. doi: 10.1017/S0269964813000156
![]() |
[16] |
S. Kochar, M. Xu, Stochastic comparisons of parallel systems when components have proportional hazard rates, Probab. Eng. Inform. Sci., 21 (2007), 597-609. doi: 10.1017/S0269964807000344
![]() |
[17] |
J. Navarro, F. Spizzichino, Comparisons of series and parallel systems with components sharing the same copula, Appl. Stoch. Models. Bus. Ind., 26 (2010), 775-791. doi: 10.1002/asmb.819
![]() |
[18] | R. F. Yan, G. F. Da, P. Zhao, Further results for parallel systems with two heterogeneous exponential components, Statistics, 47 (2013), 1128-1140. |
[19] | G. Barmalzan, A. T. P. Najafabadi, N. Balakrishnan, Ordering properties of the smallest and largest claim amounts in a general scale model, Scand. Actuar. J., 2017 (2015), 105-124. |
[20] | J. R. Wang, R. F. Yan, B. Lu, Stochastic comparisons of parallel and series systems with type II half logistic-resilience scale components, Mathematics, 8 (2020), 470. |
[21] | R. Fang, C. Li, X. H. Li, Stochastic comparisons on sample extremes of dependent and heterogenous observations, Statistics, 50 (2016), 930-955. |
[22] |
C. Li, X. H. Li, Hazard rate and reversed hazard rate orders on extremes of heterogeneous and dependent random variables, Stat. Probab. Lett., 146 (2019), 104-111. doi: 10.1016/j.spl.2018.11.005
![]() |
[23] | S. Das, S. Kayal, Some ordering results for the Marshall and Olkin's family of distributions, Commun. Math. Stat., (2019), 1-27. |
[24] | G. Barmalzan, N. Balakrishnan, S. M. Ayat, A. Akrami, Orderings of extremes dependent modified proportional hazard and modified proportional reversed hazard variables under Archimedean copula, Commun. Stat.-Theory Methods, (2020), 1-22. |
[25] | M. Shaked, J. G. Shanthikumar, Stochastic orders, New York: Springer, 2007. |
[26] | H.J. Li, X. H. Li, Stochastic orders in reliability and risk, New York: Springer, 2013. |
[27] | R. B. Nelsen, An introduction to copulas, New York: Springer, 2006. |
[28] | I. Schur, Uber eine Klasse von Mittelbildungen mit Anwendungen auf die Determinantentheorie, Sitzungsberichte der Berliner Mathematischen Gesellschaft, 22 (1923), 9-20. |
[29] | A. W. Marshall, I. Olkin, B. C. Arnold, Inequalities: Theory of majorization and its applications, 2 Eds., New York: Springer, 2011. |
1. | Bin Lu, Rongfang Yan, Ordering results of second order statistics from random and non-random number of random variables with Archimedean copulas, 2021, 6, 2473-6988, 6390, 10.3934/math.2021375 | |
2. | Rongfang Yan, Jiale Niu, Stochastic comparisons of second-order statistics from dependent and heterogenous modified proportional hazard rate observations, 2023, 0233-1888, 1, 10.1080/02331888.2023.2177999 | |
3. | Xiao Zhang, Rongfang Yan, Stochastic comparisons of extreme order statistic from dependent and heterogeneous lower-truncated Weibull variables under Archimedean copula, 2022, 7, 2473-6988, 6852, 10.3934/math.2022381 | |
4. | Liang Jiao, Rongfang Yan, Stochastic Comparisons of Lifetimes of Series and Parallel Systems with Dependent Heterogeneous MOTL-G Components under Random Shocks, 2021, 13, 2073-8994, 2248, 10.3390/sym13122248 | |
5. | Sangita Das, Suchandan Kayal, Nuria Torrado, Ordering results between extreme order statistics in models with dependence defined by Archimedean [survival] copulas, 2022, 0035-5038, 10.1007/s11587-022-00715-3 | |
6. | Mansour Shrahili, Mohamed Kayid, Mhamed Mesfioui, Relative Orderings of Modified Proportional Hazard Rate and Modified Proportional Reversed Hazard Rate Models, 2023, 11, 2227-7390, 4652, 10.3390/math11224652 | |
7. | Bin Lu, Stochastic comparisons of second-order statistics from dependent and heterogeneous modified proportional (reversed) hazard rates scale models, 2024, 9, 2473-6988, 8904, 10.3934/math.2024434 | |
8. | Mingxia Yang, Orderings of the second-largest order statistic with modified proportional reversed hazard rate samples, 2025, 10, 2473-6988, 311, 10.3934/math.2025015 |