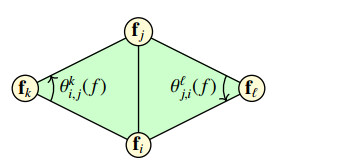
Terrain surface roughness, often described abstractly, poses challenges in quantitative characterization with various descriptors found in the literature. In this study, we compared five commonly used roughness descriptors, exploring correlations among their quantified terrain surface roughness maps across three terrains with distinct spatial variations. Additionally, we investigated the impacts of spatial scales and interpolation methods on these correlations. Dense point cloud data obtained through Light Detection and Ranging technique were used in this study. The findings highlighted both global pattern similarities and local pattern distinctions in the derived roughness maps, emphasizing the significance of incorporating multiple descriptors in studies where local roughness values play a crucial role in subsequent analyses. The spatial scales were found to have a smaller impact on rougher terrain, while interpolation methods had minimal influence on roughness maps derived from different descriptors.
Citation: Lei Fan, Yang Zhao. Comparing roughness maps generated by five typical roughness descriptors for LiDAR-derived digital elevation models[J]. AIMS Geosciences, 2024, 10(2): 228-241. doi: 10.3934/geosci.2024013
[1] | Mohd Danish Siddiqi, Fatemah Mofarreh . Schur-type inequality for solitonic hypersurfaces in (k,μ)-contact metric manifolds. AIMS Mathematics, 2024, 9(12): 36069-36081. doi: 10.3934/math.20241711 |
[2] | Nasser Bin Turki, Sharief Deshmukh, Olga Belova . A note on closed vector fields. AIMS Mathematics, 2024, 9(1): 1509-1522. doi: 10.3934/math.2024074 |
[3] | Amira Ishan . On concurrent vector fields on Riemannian manifolds. AIMS Mathematics, 2023, 8(10): 25097-25103. doi: 10.3934/math.20231281 |
[4] | Mohammad Aamir Qayyoom, Rawan Bossly, Mobin Ahmad . On CR-lightlike submanifolds in a golden semi-Riemannian manifold. AIMS Mathematics, 2024, 9(5): 13043-13057. doi: 10.3934/math.2024636 |
[5] | Mehmet Gülbahar . Qualar curvatures of pseudo Riemannian manifolds and pseudo Riemannian submanifolds. AIMS Mathematics, 2021, 6(2): 1366-1376. doi: 10.3934/math.2021085 |
[6] | Xiaosheng Li . New Einstein-Randers metrics on certain homogeneous manifolds arising from the generalized Wallach spaces. AIMS Mathematics, 2023, 8(10): 23062-23086. doi: 10.3934/math.20231174 |
[7] | Rabia Cakan Akpınar, Esen Kemer Kansu . Metallic deformation on para-Sasaki-like para-Norden manifold. AIMS Mathematics, 2024, 9(7): 19125-19136. doi: 10.3934/math.2024932 |
[8] | Ghodratallah Fasihi-Ramandi . Hamilton’s gradient estimate for fast diffusion equations under geometric flow. AIMS Mathematics, 2019, 4(3): 497-505. doi: 10.3934/math.2019.3.497 |
[9] | Ehtesham Akhter, Musavvir Ali, Mohd Bilal . Special type convex functions on Riemannian manifolds with application. AIMS Mathematics, 2023, 8(7): 15081-15091. doi: 10.3934/math.2023770 |
[10] | Yusuf Dogru . η-Ricci-Bourguignon solitons with a semi-symmetric metric and semi-symmetric non-metric connection. AIMS Mathematics, 2023, 8(5): 11943-11952. doi: 10.3934/math.2023603 |
Terrain surface roughness, often described abstractly, poses challenges in quantitative characterization with various descriptors found in the literature. In this study, we compared five commonly used roughness descriptors, exploring correlations among their quantified terrain surface roughness maps across three terrains with distinct spatial variations. Additionally, we investigated the impacts of spatial scales and interpolation methods on these correlations. Dense point cloud data obtained through Light Detection and Ranging technique were used in this study. The findings highlighted both global pattern similarities and local pattern distinctions in the derived roughness maps, emphasizing the significance of incorporating multiple descriptors in studies where local roughness values play a crucial role in subsequent analyses. The spatial scales were found to have a smaller impact on rougher terrain, while interpolation methods had minimal influence on roughness maps derived from different descriptors.
This paper proposes a new method for computing spherical area-preserving mappings of topological spheres using a Riemannian optimization framework.
Area-preserving mappings can serve as parameterization of surfaces and have been applied to the field of computer graphics and medical imaging [6]. State-of-the-art methods for the computation of area-preserving parameterizations include diffusion-based methods [8], optimal mass transportation-based methods [18], and nonlinear optimization methods [20]. It is worth noting that most previous works consider area-preserving parameterization from simply connected open surfaces to planar regions. For the spherical area-preserving mapping of genus-zero closed surfaces, recently developed methods include the adaptive area-preserving parameterization method [7], the spherical optimal transportation mapping [10], and the stretch energy minimization method [21].
Riemannian optimization generalizes unconstrained, continuous optimization (defined on Euclidean space) following the same principle that Riemannian geometry is a generalization of Euclidean space. In traditional optimization methods, nonlinear matrix manifolds rely on the Euclidean vector space structure. In contrast, in the Riemannian optimization framework, algorithms and convergence analysis are formulated based on the language of differential geometry, and numerical linear algebra techniques are then used for implementation. Riemannian optimization focuses on matrix manifolds, which are manifolds in the sense of classical Riemannian geometry that admit a natural representation of their elements in the form of matrices. Retraction mappings play a vital role in this framework, as they are used to turn objective functions defined in Euclidean space into functions defined on an abstract manifold so that constraints are taken into account explicitly. A vast body of literature on the theory and implementation of Riemannian optimization now exists. Some of the earliest references for this field, especially for line-search methods, go back to Luenberger [15], Gabay [14], and Udrişte [19]. More recent literature that encompasses the findings and developments of the last three decades is [2,5,12].
Our Riemannian gradient descent (RGD) method involves classical components from computational geometry (simplicial surfaces and mappings) and Riemannian optimization (line search, retractions, and Riemannian gradients). To the best of the authors' knowledge, this is the first time this approach has been used in computational geometry. In this paper, we minimize the normalized stretch energy [20] for simplicial mappings subject to the constraint that the image of the mapping belongs to a power manifold of n unit spheres embedded in R3.
In this paper, we propose an RGD method to compute spherical area-preserving mappings on a power manifold of unit spheres. In particular, the main contributions are as follows:
(i) We combine the tools from the Riemannian optimization framework and the components from computational geometry to propose an RGD method for computing spherical area-preserving mappings of topological spheres.
(ii) We explore two different line-search strategies: one using MATLAB's fminbnd, and the other using the quadratic/cubic interpolant approximation from [11,§ 6.3.2].
(iii) We conduct extensive numerical experiments on several mesh models to demonstrate the accuracy and efficiency of the algorithm.
(iv) We demonstrate the competitiveness and efficiency of our algorithm over three state-of-the-art methods for computing area-preserving mappings.
(v) We show that our algorithm is stable with respect to small perturbations in the initial mesh model.
(vi) We apply the algorithm to the concrete application of landmark-aligned surface registration between two human brain models.
The remaining part of this paper is organized as follows. Section 2 introduces the main concepts on simplicial surfaces and mappings and presents the formulation of the objective function. Section 3 provides some preliminaries on the Riemannian optimization framework, briefly recalls the geometry of the unit sphere, and then describes the tools needed to perform optimization on the power manifold. Section 4 is about the RGD method and reports known convergence results. Section 5 discusses the extensive numerical experiments to evaluate our algorithm in terms of accuracy and efficiency, and provides a concrete application. Finally, we wrap our paper with conclusions and a future outlook in Section 6. The appendices contain other details about the calculations of the area of the simplicial mapping, the Hessian of the objective function, and the line-search procedure used in the RGD method.
In this section, we list the notations and symbols adopted in order of appearance in the paper. Symbols specific to a particular section are usually not included in this list.
τ | A triangular face |
|τ| | The area of the triangle τ |
M | Simplicial surface |
V(M) | Set of vertices of M |
F(M) | Set of faces of M |
E(M) | Set of edges of M |
vi, vj, vk | Vertices of a triangular face |
f | Simplicial mapping |
f | Representative matrix of f |
fℓ | Coordinates of a vertex f(vℓ) |
vec | Column-stacking vectorization operator |
S2 | Unit sphere in R3 |
(S2)n | Power manifold of n unit spheres in R3 |
EA(f) | The authalic energy |
ES(f) | The stretch energy |
LS(f) | Weighted Laplacian matrix |
ωS | Modified cotangent weights |
A(f) | Area of the image of f |
E(f) | The normalized stretch energy |
TxS2 | Tangent space to S2 at x |
PTxS2 | Orthogonal projector onto the tangent space to S2 at x |
PTfℓ(S2)n | Orthogonal projector onto the tangent space to (S2)n at fℓ |
R | Retraction mapping |
∇E(f) | Euclidean gradient of E(f) |
grad E(f) | Riemannian gradient of E(f) |
We introduce the simplicial surfaces and mappings in subsection 2.1 and the objective function in subsection 2.2.
A simplicial surface M is the underlying set of a simplicial 2-complex K(M)=F(M)∪E(M)∪V(M) composed of vertices
V(M)={vℓ=(v1ℓ,v2ℓ,v2ℓ)⊤∈R3}nℓ=1, |
oriented triangular faces
F(M)={τℓ=[viℓ,vjℓ,vkℓ]∣viℓ,vjℓ,vkℓ∈V(M)}mℓ=1, |
and undirected edges
E(M)={[vi,vj]∣[vi,vj,vk]∈F(M) for some vk∈V(M)}. |
A simplicial mapping f:M→R3 is a particular type of piecewise affine mapping with the restriction mapping f|τ being affine, for every τ∈F(M). We denote
fℓ:=f(vℓ)=(f1ℓ,f2ℓ,f3ℓ)⊤, for every vℓ∈V(M). |
The mapping f can be represented as a matrix
f=[f⊤1⋮f⊤n]=[f11f21f31⋮⋮⋮f1nf2nf3n]=:[f1f2f3], | (2.1) |
or a vector
vec(f)=[f1f2f3]. |
The authalic energy for simplicial mappings f:M→R3 is defined as [20]
EA(f)=ES(f)−A(f), |
where ES is the stretch energy defined as
ES(f)=12vec(f)⊤(I3⊗LS(f))vec(f), |
where I3 is the identity matrix of size 3-by-3, ⊗ denotes the Kronecker product, and LS(f) is the weighted Laplacian matrix LS(f). The latter is defined by
[LS(f)]i,j={−∑[vi,vj,vk]∈F(M)[ωS(f)]i,j,kif [vi,vj]∈E(M),−∑ℓ≠i[LS(f)]i,ℓif j=i,0otherwise, | (2.2) |
in which ωS(f) is the modified cotangent weight defined as
[ωS(f)]i,j,k=cot(θki,j(f))|f([vi,vj,vk])|2|[vi,vj,vk]|, | (2.3) |
with θki,j(f) being the angle opposite to the edge f([vi,vj]) at the point f(vk) on the image f(M), as illustrated in Figure 1.
It is proved that EA(f)≥0 and the equality holds if and only if f preserves the area [20,Corollary 3.4]. Due to the optimization process, the image area A(f) is not constant, hence we introduce a prefactor |M|/A(f) and define the normalized stretch energy as
E(f)=|M|A(f)ES(f). | (2.4) |
To perform numerical optimization via the RGD method, we need to compute the Euclidean gradient. By applying the formula ∇ES(f)=2(I3⊗LS(f))vec(f) from [20,(3.6)], the gradient of E(f) can be formulated as
∇E(f)=∇(|M|A(f)ES(f))=|M|A(f)∇ES(f)+ES(f)∇|M|A(f)=2|M|A(f)(I3⊗LS(f))vec(f)−|M|ES(f)A(f)2∇A(f). | (2.5) |
The following proposition gives an explicit formula for calculating ∇A(f).
Proposition 2.1 (Formula for ∇A). The gradient of A can be explicitly formulated as
∇A(f|τ)=|τ|A(f|τ)vec(LS(f|τ)fτ). | (2.6) |
Proof. By applying the explicit formulas ES(f|τ)=A(f|τ)2|τ| and ∇ES(f|τ)=2vec(LS(f|τ)fτ) from [20], the chain rule yields
2vec(LS(f|τ)fτ)=∇ES(f|τ)=2A(f|τ)|τ|∇A(f|τ), |
which is equivalent to (2.6).
Other details about the calculation of A(f) are reported in Appendix 7.
The Riemannian optimization framework [2,5,12] solves constrained optimization problems where the constraints have a geometric nature. This approach utilizes the underlying geometric structure of the problems, which allows the constraints to be taken explicitly into account. The optimization variables are constrained to a smooth manifold, and the optimization is performed on that manifold. Typically, the manifolds considered are matrix manifolds, meaning there is a natural representation of their elements in matrix form. In particular, in this paper, the problem is formulated on a power manifold of n unit spheres embedded in R3, and we use the RGD method for minimizing the cost function (2.4) on this power manifold.
Traditional optimization methods rely on the Euclidean vector space structure. For instance, the steepest descent method for minimizing a function g:Rn→R updates the current iterate xk by moving in the direction dk of the anti-gradient of g, by a step size αk chosen according to an appropriate line-search rule. However, on a nonlinear manifold, the vector addition xk+αkdk does not make sense in general due to the manifold curvature. We need the notion of tangent vectors and their length to generalize the steepest descent direction to a Riemannian manifold. The retraction mapping is also a vital tool in the Riemannian optimization framework. It is a mapping from the tangent space to the manifold, used to transform objective functions defined in Euclidean space into functions defined on a manifold while explicitly taking the constraints into account.
Similarly to the line-search method in Euclidean space, a line-search method in the Riemannian framework determines at a current iterate xk on a manifold M a search direction ξ on the tangent space TxM. The next iterate xk+1 is then determined by a line search along a curve α↦Rx(αξ) where Rx:TxM→M is the retraction mapping. The procedure is then repeated for xk+1 taking the role of xk. Similarly to optimization methods in Euclidean space, search directions can be the negative of the Riemannian gradient, leading to the Riemannian steepest descent method. Other choices of search directions lead to other methods, e.g., Riemannian versions of the trust-region method [1] or BFGS [17].
In what follows, we introduce some fundamental geometry concepts used in Riemannian optimization, which are necessary to formulate the RGD method for our problems. We first briefly recall the geometry of the unit sphere S2 embedded in R3, and then we switch to the power manifold of n unit spheres, denoted by (S2)n.
The unit sphere S2 is a Riemannian submanifold of R3 defined as
S2={x∈R3:x⊤x=1}. |
The Riemannian metric (inner product) on the sphere is inherited from the embedding space R3, i.e.,
⟨ξ,η⟩x=ξ⊤η,ξ,η∈TxS2, |
where TxS2 is the tangent space to S2 at x∈S2, defined as the set of all vectors orthogonal to x in R3, i.e.,
TxS2={z∈R3:x⊤z=0}. |
The projector PTxS2 from R3 onto the tangent space TxS2 is a mapping PTxS2:R3→TxS2, defined by
PTxS2(z)=(I3−xx⊤)z. | (3.1) |
In the following, points on the unit sphere are denoted by fℓ (the vertices of the simplicial mapping f), and tangent vectors are represented by ξℓ.
We aim to minimize the function E(f)=E(f1,…,fn) (2.4), where each fℓ, ℓ=1,…,n, lives on the same manifold S2. This leads us to consider the power manifold of n unit spheres
(S2)n=S2×S2×⋯S2⏟n times, |
with the metric of S2 extended elementwise. In the remaining part of this section, we present the tools from Riemannian geometry needed to generalize gradient descent to this manifold. The projector onto the tangent space is used to compute the Riemannian gradient, as it will be explained in Section 4. The projection onto the power manifold turns points of Rn×3 into points of (S2)n. Finally, the retraction turns an objective function defined on Rn×3 into an objective function defined on the manifold (S2)n.
As seen above, the projector onto the tangent space to the unit sphere at the point x is given by (3.1). Here, the points are denoted by fℓ∈R3, ℓ=1,…,n, so we write
PTfℓS2=I3−fℓf⊤ℓ. |
It clearly changes for every vertex fℓ. The projector from Rn×3 onto the tangent space at f to the power manifold (S2)n is a mapping
PTf(S2)n:Rn×3→Tf(S2)n, |
and can be represented by a block diagonal matrix of size 3n×3n, i.e.,
PTf(S2)n:=blkdiag(PTf1S2,PTf2S2,…,PTfnS2)=[PTf1S2PTf2S2⋱PTfnS2]. | (3.2) |
In practice, we never actually create this matrix. Instead, we implement an efficient version using vectorized operations (MATLAB's bsxfun).
The projection of a single vertex fℓ from R3 to the unit sphere S2 is given by the normalization
˜fℓ=fℓ‖fℓ‖2. |
Hence, the projection of the whole of f onto the power manifold (S2)n is given by
P(S2)n:Rn×3→(S2)n, |
defined by
f↦˜f:=diag(1‖f1‖2,1‖f2‖2,…,1‖fn‖2)[f1f2⋯fn]⊤. |
Again, this representative matrix is only shown for illustrative purposes; in the actual implementation, we use row-wise normalization of f.
A retraction is a mapping from the tangent space to the manifold used to turn tangent vectors into points on the manifold and functions defined on the manifold into functions defined on the tangent space; see [2,3] for more details.
The retraction of a single tangent vector ξℓ from TfℓS2 to S2 is a mapping Rfℓ:TfℓS2→S2, defined by [2,Example 4.1.1]
Rfℓ(ξℓ)=fℓ+ξℓ‖fℓ+ξℓ‖. |
Figure 2 provides an illustration of the retraction from TfℓS2 to S2.
For the power manifold (S2)n, the retraction of all the tangent vectors ξℓ, ℓ=1,…,n, is a mapping
Rf:Tf(S2)n→(S2)n, |
defined by
[ξ1⋯ξn]⊤↦diag(1‖f1+ξ1‖2,…,1‖fn+ξn‖2)[f1+ξ1⋯fn+ξn]⊤. | (3.3) |
Again, this retraction is implemented by row-wise normalization of f+ξ.
We are now in the position of introducing the RGD method. In this section, we will first provide the formula for the Riemannian gradient on the power manifold (S2)n. Then, we will explain the RGD method by providing its pseudocode, and finally, we will recall the known theoretical results that ensure the convergence of RGD.
The Riemannian gradient of the objective function E in (2.4) is given by the projection onto Tf(S2)n of the Euclidean gradient of E, namely,
grad E(f)=PTf(S2)n(∇E(f)), | (4.1) |
where ∇E is explicitly formulated in (2.5). This is always the case for embedded submanifolds; see [2,§ 3.6.1]. Figure 3 illustrates the difference between the Euclidean and the Riemannian gradient for one point on the unit sphere.
Given an initial iterate f(0)∈(S2)n, the RGD algorithm generates a sequence of iterates {f(k)} as follows. At each iteration k=0,1,2,…, it chooses a search direction d(k)=−grad E(f(k)) in the tangent space Tf(k)(S2)n such that the sequence {d(k)} is gradient related. Then, the new point f(k+1) is chosen such that it satisfies the sufficient decrease condition
E(f(k))−E(f(k+1))≥c(E(f(k))−E(Rf(k)(αkd(k)))), | (4.2) |
where c>0 and αk is the step size for the given d(k).
The RGD method on the power manifold (S2)n is summarized in Algorithm 1. It has been adapted from [2,p. 63]. In practice, in our numerical experiments of Section 5, the initial mapping f(0)∈(S2)n is computed by applying the fixed-point iteration (FPI) method of [21] until the first increase in energy is detected; see subsection 5.3, Algorithm 2. The line-search procedure used in line 7 is described in detail in Appendix 9.
Algorithm 1: The RGD method on (S2)n. |
1 Given objective function E, power manifold (S2)n, initial iterate f(0)∈(S2)n, projector PTf(S2)n from Rn×3 to Tf(S2)n, retraction Rf from Tf(S2)n to (S2)n;
Result: Sequence of iterates {f(k)}. 2 k←0; ![]() |
Known convergence results
The RGD method has theoretically guaranteed convergence. For the reader's convenience, we report the two main results on the convergence of RGD. The first result is about the convergence of Algorithm 1 to critical points of the objective function. The second result is about the convergence of RGD to a local minimizer with a line-search technique.
Theorem 4.1 ([2,Theorem 4.3.1]). Let {f(k)} be an infinite sequence of iterates generated by Algorithm 1. Then, every accumulation point f(∗) of {f(k)} is a critical point of the cost function E.
Remark 4.1. We are implicitly saying that a sequence can have more than one accumulation point; for example, from a sequence {f(k)}, we may extract two subsequences such that they have two distinct accumulation points.
The proof of Theorem 4.1 can be done by contradiction, but it remains pretty technical, so we refer the interested reader to [2,p. 65]. It should be pointed out that Theorem 4.1 only guarantees the convergence to critical points. It does not tell us anything about their nature, i.e., whether the critical points are local minimizers, local maximizers, or saddle points. However, if the smallest eigenvalue of the Hessian of E at f(∗), λH,min, then the critical point f^{(\ast)} is a local minimizer of E . Under this assumption (i.e., that \lambda_{H, \min} > 0 ), [2,§ 4.5.2] gives an asymptotic convergence bound for Algorithm 1. Indeed, the following result uses the smallest and largest eigenvalues of the Hessian of the objective function at a critical point f^{(\ast)} .
Theorem 4.2 ([2,Theorem 4.5.6]). Let \lbrace f^{(k)} \rbrace be an infinite sequence of iterates generated by Algorithm 1, converging to a point f^{(\ast)} . (By Theorem 4.1, f^{(\ast)} is a critical point of E .) Let \lambda_{H, \min} and \lambda_{H, \max} be the smallest and largest eigenvalues of the Hessian of E at f^{(\ast)} . Assume that \lambda_{H, \min} > 0 (hence f^{(\ast)} is a local minimizer of E ). Then, given r in the interval (r_{\ast}, 1) with r_{\ast} = 1-\min\left(2\sigma \bar{\alpha}\lambda_{H, \min}, 4\sigma(1-\sigma)\beta \frac{\lambda_{H, \min}}{\lambda_{H, \max}} \right) , there exists an integer K \geq 0 such that
\begin{equation} E(f^{(k+1)}) - E(f^{(\ast)}) \leq (r+(1-r)(1-c))\left( E(f^{(k)}) - E(f^{(\ast)}) \right), \end{equation} | (4.3) |
for all k\geq K , where c is the parameter in (4.2) of Algorithm 1. Note that 0 < r_{\ast} < 1 since \beta, \sigma \in (0, 1) .
As noted in [2,p. 71], in typical cases of Algorithm 1, the constant c in the descent condition (4.2) equals 1, hence (4.3) reduces to E(f^{(k+1)}) - E(f^{(\ast)}) \leq r\left(E(f^{(k)}) - E(f^{(\ast)}) \right) .
In this section, we demonstrate the convergence behavior of the RGD method using twelve mesh models and two line-search techniques. We present numerical results in tables and provide convergence plots. The computational time cost of the RGD in every table does not include the time cost for the initial mapping by the FPI method. In subsection 5.2, we introduce a correction for bijectivity, which helps to unfold the folding triangles. We then compare RGD to the FPI method of [21] in subsection 5.3 and the adaptive area-preserving parameterization method of [7] in subsection 5.4. In subsection 5.5, we compare our RGD to the spherical optimal transportation mapping proposed by Cui et al. [10]. Then, in subsection 5.6, we show that the algorithm is robust to noise by starting the algorithm from an initial guess with small perturbations. Finally, in subsection 5.7, we apply our algorithm to the concrete application of surface registration of two brain models.
We conducted all our experiments on a laptop Lenovo ThinkPad T460s, with Windows 10 Pro and MATLAB R2021a installed, with Intel Core i7-6600 CPU, 20GB RAM, and Mesa Intel HD Graphics 520. The benchmark triangular mesh models used in our numerical experiments are shown in Figure 4, arranged per increasing number of vertices and faces, from the top left to bottom right.
To provide the RGD method with a good initial mapping, we first apply the FPI method of [21], described in subsection 5.3. Specifically, we apply the FPI until the first increase in energy occurs. We adopted two different line-search strategies: one that uses MATLAB's \texttt{fminbnd} and another that uses the quadratic/cubic interpolant of [11,§ 6.3.2], described in Appendix 9.
In all the experiments, we monitor the authalic energy E_A(f) := E_S(f) - {\mathcal A}(f) defined in subsection 2.2 instead of the normalized stretch energy (2.4) because when E_{A} = 0 , we know from the theory that f is an area-preserving mapping. Strictly speaking, in practice, we never obtain a mapping that exactly preserves the area, but we obtain a mapping that is area-distortion minimizing, since E_{A} is never identically zero. We also monitor the ratio between the standard deviation and the mean of the area ratio. This quantity has been considered in [8]. Finally, the computational time is always reported in seconds, and #Fs denotes the number of folding triangles.
Tables 1 and 2 report the numerical results for RGD for minimizing the normalized stretch energy E , when run for a fixed maximum number of iterations (10 on the left and 100 on the right). Table 1 is for the RGD that uses the \texttt{fminbnd} line-search strategy, while Table 2 is for the RGD that uses the quadratic/cubic interpolant from [11,§ 6.3.2]. Similarly to the tables, Figures 5 and 6 illustrate the convergence behavior of RGD in the same setting when run for 100 iterations.
10 Iterations | 100 Iterations | |||||||||
Model Name | SD/Mean | E_{A}(f) | Time | #Fs | SD/Mean | E_{A}(f) | Time | #Fs | ||
Right Hand | 0.1950 | 2.17 \times 10^{-1} | 0.85 | 4 | 0.1459 | 1.28 \times 10^{-1} | 9.37 | 2 | ||
David Head | 0.0178 | 4.04 \times 10^{-3} | 1.66 | 0 | 0.0156 | 3.05 \times 10^{-3} | 14.95 | 0 | ||
Cortical Surface | 0.0214 | 4.68 \times 10^{-3} | 3.62 | 0 | 0.0200 | 4.15 \times 10^{-3} | 32.40 | 0 | ||
Bull | 0.1492 | 2.58 \times 10^{-1} | 4.99 | 4 | 0.1380 | 2.31 \times 10^{-1} | 43.27 | 1 | ||
Bulldog | 0.0369 | 1.41 \times 10^{-2} | 13.59 | 0 | 0.0343 | 1.27 \times 10^{-2} | 136.63 | 0 | ||
Lion Statue | 0.1935 | 4.77 \times 10^{-1} | 16.83 | 0 | 0.1922 | 4.69 \times 10^{-1} | 160.20 | 0 | ||
Gargoyle | 0.0690 | 5.28 \times 10^{-2} | 19.55 | 0 | 0.0653 | 4.84 \times 10^{-2} | 192.62 | 0 | ||
Max Planck | 0.0537 | 3.54 \times 10^{-2} | 16.52 | 0 | 0.0525 | 3.38 \times 10^{-2} | 161.01 | 0 | ||
Bunny | 0.0417 | 2.18 \times 10^{-2} | 20.59 | 0 | 0.0404 | 2.04 \times 10^{-2} | 226.99 | 0 | ||
Chess King | 0.0687 | 6.07 \times 10^{-2} | 52.36 | 21 | 0.0639 | 5.14 \times 10^{-2} | 518.18 | 17 | ||
Art Statuette | 0.0408 | 2.14 \times 10^{-2} | 140.59 | 0 | 0.0405 | 2.10 \times 10^{-2} | 1 111.67 | 0 | ||
Bimba Statue | 0.0514 | 3.31 \times 10^{-2} | 270.63 | 1 | 0.0511 | 3.29 \times 10^{-2} | 2 320.19 | 1 |
10 Iterations | 100 Iterations | |||||||||
Model Name | SD/Mean | E_{A}(f) | Time | #Fs | SD/Mean | E_{A}(f) | Time | #Fs | ||
Right Hand | 0.1936 | 2.16 \times 10^{-1} | 0.36 | 4 | 0.1204 | 9.40 \times 10^{-2} | 4.07 | 1 | ||
David Head | 0.0178 | 4.04 \times 10^{-3} | 0.99 | 0 | 0.0156 | 3.04 \times 10^{-3} | 9.16 | 0 | ||
Cortical Surface | 0.0216 | 4.75 \times 10^{-3} | 1.40 | 0 | 0.0200 | 3.72 \times 10^{-3} | 16.01 | 0 | ||
Bull | 0.1492 | 2.59 \times 10^{-1} | 1.77 | 4 | 0.1348 | 2.19 \times 10^{-1} | 18.89 | 1 | ||
Bulldog | 0.0369 | 1.41 \times 10^{-2} | 6.60 | 0 | 0.0343 | 1.27 \times 10^{-2} | 61.93 | 0 | ||
Lion Statue | 0.1935 | 4.77 \times 10^{-1} | 7.75 | 0 | 0.1894 | 4.54 \times 10^{-1} | 76.76 | 0 | ||
Gargoyle | 0.0688 | 5.26 \times 10^{-2} | 7.81 | 0 | 0.0646 | 4.76 \times 10^{-2} | 80.52 | 0 | ||
Max Planck | 0.0537 | 3.54 \times 10^{-2} | 7.18 | 0 | 0.0525 | 3.39 \times 10^{-2} | 75.60 | 0 | ||
Bunny | 0.0417 | 2.18 \times 10^{-2} | 8.30 | 0 | 0.0390 | 1.91 \times 10^{-2} | 89.62 | 0 | ||
Chess King | 0.0692 | 6.07 \times 10^{-2} | 20.06 | 21 | 0.0647 | 5.23 \times 10^{-2} | 207.47 | 17 | ||
Art Statuette | 0.0408 | 2.14 \times 10^{-2} | 57.71 | 0 | 0.0405 | 2.10 \times 10^{-2} | 654.57 | 0 | ||
Bimba Statue | 0.0514 | 3.31 \times 10^{-2} | 70.83 | 1 | 0.0512 | 3.29 \times 10^{-2} | 775.36 | 1 |
A comparison of the computational times between the two line-search strategies shows that the line-search strategy with a quadratic/cubic interpolant (Table 2) is much more efficient than the line-search strategy that uses MATLAB's \texttt{fminbnd} (Table 1). In many cases, the former even yields more accurate results than the latter. This is particularly evident for the Art Statuette (1 111.67 s versus 654.57 s) and the Bimba Statue (2 320.19 s versus 775.36 s) mesh models. The numerical results show that, in general, RGD can still decrease energy as the number of iterations increases.
Table 3 presents the results using a different stopping criterion instead of a fixed maximum number of iterations. Specifically, we keep track of the following quantity
\Delta E_{A}^{(k)} := \frac{E_{A}(f^{(k-1)})-E_{A}(f^{(k)})}{E_{A}(f^{(k-1)})}, |
Before bijectivity correction | After bijectivity correction | ||||||||||
Model Name | SD/Mean | E_{A}\big(f\big) | #Fs | SD/Mean | E_{A}\big(f\big) | #Fs | Time | #Its | |||
Right Hand | 0.2063 | 8.01 \times 10^{-2} | 3 | 0.1071 | 8.12 \times 10^{-2} | 0 | 6.67 | 127 | |||
David Head | 0.0123 | 1.87 \times 10^{-3} | 0 | --------------------- | 83.66 | 432 | |||||
Cortical Surface | 0.0187 | 4.23 \times 10^{-3} | 0 | --------------------- | 9.22 | 52 | |||||
Bull | 0.1520 | 2.37 \times 10^{-1} | 11 | 0.1403 | 2.40 \times 10^{-1} | 3 | 12.20 | 50 | |||
Bulldog | 0.0351 | 1.31 \times 10^{-2} | 0 | --------------------- | 72.64 | 60 | |||||
Lion Statue | 0.1940 | 4.79 \times 10^{-1} | 2 | 0.1938 | 4.79 \times 10^{-1} | 0 | 2.63 | 2 | |||
Gargoyle | 0.0704 | 5.17 \times 10^{-2} | 3 | 0.0682 | 5.14 \times 10^{-2} | 0 | 26.71 | 17 | |||
Max Planck | 0.0464 | 3.54 \times 10^{-2} | 0 | --------------------- | 10.58 | 8 | |||||
Bunny | 0.0417 | 2.17 \times 10^{-2} | 0 | --------------------- | 20.77 | 13 | |||||
Chess King | 0.0715 | 5.17 \times 10^{-2} | 43 | 0.0641 | 5.38 \times 10^{-2} | 17 | 370.68 | 107 | |||
Art Statuette | 0.0409 | 2.14 \times 10^{-2} | 0 | --------------------- | 31.53 | 3 | |||||
Bimba Statue | 0.0515 | 3.32 \times 10^{-2} | 2 | 0.0514 | 3.32 \times 10^{-2} | 0 | 19.24 | 2 |
which represents the relative improvement in the authalic energy E_{A}(f) between two successive iterates, and we stop the RGD method if the value of \Delta E_{A}^{(k)} is smaller than 10^{-3} .
It is apparent from Table 3 that RGD stops at different numbers of iterations for different mesh models. Comparing with Table 2 above, it suggests that the improvement after doing more iterations is not significant for a few mesh models, especially Lion Statue, Art Statuette, and Bimba Statue. Table 3 also shows the values of the monitored quantities SD/Mean, E_{A}(f) , and #Fs before and after the bijectivity correction described in subsection 5.2. In all cases where the bijectivity correction is applied, the number of folding triangles #Fs decreases or reduces to zero. Moreover, the post-processing for bijectivity correction improves the SD/Mean value in all the cases. For the Right Hand mesh model, the SD/Mean value even improves significantly from 0.2063 to 0.1071 before and after the bijectivity correction. In one case (Gargoyle), the value of authalic energy E_{A}(f) also improves.
Figure 7 shows the convergence behavior for the first three smallest mesh models considered, namely Right Hand, David Head, and Cortical Surface, when the algorithm is run for many more iterations; here, 10 000 iterations. It shows that RGD keeps decreasing the authalic energy E_{A} , albeit very slowly. The values of SD/Mean are also improved for all three mesh models. The corresponding numerical results are reported in Table 4; results for 100 iterations are also reported for easier comparison.
100 Iterations | 10 000 Iterations | |||||||||
Model Name | SD/Mean | E_{A}(f) | Time | #Fs | SD/Mean | E_{A}(f) | Time | #Fs | ||
Right Hand | 0.1204 | 9.40 \times 10^{-2} | 4.07 | 1 | 0.0545 | 2.07 \times 10^{-2} | 431.28 | 0 | ||
David Head | 0.0156 | 3.04 \times 10^{-3} | 9.16 | 0 | 0.0029 | 1.01 \times 10^{-4} | 1 018.87 | 0 | ||
Cortical Surface | 0.0200 | 3.72 \times 10^{-3} | 16.01 | 0 | 0.0045 | 2.06 \times 10^{-4} | 1 328.56 | 0 |
We compute with MATLAB the eigenvalues of the Hessian matrix at the minimizer. Table 5 reports on the smallest eigenvalue of the Hessian of the initial mapping (produced by the FPI method) and eigenvalues of the Hessian of the mapping when the prescribed maximum number of RGD iterations is achieved. The eigenvalues of the Hessian are computed by the MATLAB built-in function \texttt{eigs} with the option \texttt{smallestabs} and the number of eigenvalues to compute being 2 000. We observe that the Hessian eigenvalues are significantly closer to zero after running the RGD method, as we expected.
Smallest eigenvalue of the Hessian | ||
Model Name | After FPI | After RGD |
Right Hand | -2.4429 \times 10^{0} | -1.9013 \times 10^{-1} |
David Head | -1.8481 \times 10^{0} | -2.8445 \times 10^{-6} |
Cortical Surface | -4.4940 \times 10^{-1} | -3.6768 \times 10^{-3} |
Figure 8 displays the spherical mappings resulting from applying our RGD algorithm to the benchmark mesh models considered, offering a qualitative insight into the goodness of the mappings obtained.
The proposed RGD method does not guarantee the produced mapping is bijective. To remedy this drawback, we introduce a post-processing method to ensure bijectivity in the mapping. This is achieved by employing a modified version of the mean value coordinates, as described in [13].
Suppose we have a spherical mapping f\colon {\mathcal M}\to S^{2} that may not be bijective. First, we map the spherical image to the extended complex plane \overline{\mathbb{C}} = \mathbb{C}\cup\{\infty\} by the stereographic projection
\begin{equation} \varPi_{ S^2}(x, y, z) = \frac{x}{1-z} + \mathrm{i}\, \frac{y}{1-z}. \end{equation} | (5.1) |
Denote the mapping h = \varPi_{ S^{2}}\circ f and the complex-valued vector \mathbf{h}_i = h(v_i) , for v_i\in\mathcal{V}({\mathcal M}) . The folding triangular faces in the southern hemisphere are now mapped in \mathbb{D}\subset\overline{\mathbb{C}} , which can be unfolded by solving the linear systems
\begin{equation} [L_M(h)]_{\mathtt{I}, \mathtt{I}} \widetilde{\mathbf{h}_{\mathtt{I}}} = -[L_M(h)]_{\mathtt{I}, \mathtt{B}} \mathbf{h}_\mathtt{B}, \end{equation} | (5.2) |
where \mathtt{I} = \{ i \mid | h(v_i) | < r \} denotes the vertex index set with r being a value slightly larger than 1 , e.g., r = 1.2 , \mathtt{B} = \{1, \ldots, n\} \backslash \mathtt{I} , and L_{M} is the Laplacian matrix defined as
\begin{equation} {[L_M(h)]}_{i, j} = \begin{cases} -\sum\limits_{[v_i, v_j, v_k]\in\mathcal{F}( {\mathcal M})} [\omega_M(h)]_{i, j, k} &\mbox{if } [{v}_i, {v}_j]\in\mathcal{E}( {\mathcal M}) , \\ -\sum\limits_{\ell\neq i} [L_M(h)]_{i, \ell} &\mbox{if } j = i , \\ 0 &\mbox{otherwise, } \end{cases} \end{equation} | (5.3a) |
with \omega_M(h) being a variant of the mean value weight [13] defined as
\begin{equation} [\omega_M(h)]_{i, j, k} = \frac{1}{\|\mathbf{h}_i - \mathbf{h}_j\|} \tan\frac{\varphi_{i, j}^k(h)}{2}, \end{equation} | (5.3b) |
in which \varphi_{i, j}^k(h) is the angle opposite to the edge [h(v_j), h(v_k)] at the point h(v_i) on h({\mathcal M}) , as illustrated in Figure 9. Then, the mapping is updated by replacing \mathbf{h}_{\mathtt{I}} with \widetilde{\mathbf{h}_{\mathtt{I}}} . Next, an inversion
\begin{equation} \mathrm{Inv}(z) = \frac{1}{\bar{z}} \end{equation} | (5.4) |
is performed to reverse the positions of the southern and northern hemispheres. Then, the linear system (5.2) is solved again to unfold the triangular faces originally located in the northern hemisphere. We denote the updated mapping as \widetilde{h} . Ultimately, the corrected mapping is given by \varPi_{ S^2}^{-1}\circ \widetilde{h} , where \varPi_{ S^2}^{-1} denotes the inverse stereographic projection
\begin{equation} \varPi_{ S^2}^{-1}(u+\mathrm{i}\, v) = \left( \frac{2 u}{u^2+v^2+1}, \ \frac{2 v}{u^2+v^2+1}, \ \frac{u^2+v^2-1}{u^2+v^2+1} \right). \end{equation} | (5.5) |
In our numerical experiments, we perform the bijectivity correction both after the FPI method and after the RGD method, if needed.
To validate the algorithm, we compare it with the numerical results on the same models obtained using the FPI method. This method was proposed by Yueh et al. [21] to calculate area-preserving boundary mapping while parameterizing 3-manifolds.
The FPI method of stretch energy minimization (SEM) is performed as follows. First, a spherical harmonic mapping f^{(0)}\colon {\mathcal M}\to S^2 is computed by the Laplacian linearization method [4]. Suppose a spherical mapping f^{(k)} is computed. Then, we map the spherical image to the extended complex plane by the stereographic projection (5.1) together with an inversion (5.4). Then, we define the mapping h^{(k)}\colon {\mathcal M}\to\mathbb{C}\cup\{\infty\} as
h^{(k)}(v) = \mathrm{Inv} \circ\varPi_{ S^2}\circ f^{(k)}(v). |
The mapping h^{(k)} is represented as the complex-valued vector by \mathbf{h}^{(k)}_i = h^{(k)}(v_i) . Next, we define the interior vertex index set as
\mathtt{I}^{(k)} = \{ i \mid | h^{(k)}(v_i) | < r \}, |
which collects the indices of vertices in the circle centered at the origin of radius r . Other vertices are defined as boundary vertices and the associated index set is defined as
\mathtt{B}^{(k)} = \{1, \ldots, n\} \backslash \mathtt{I}^{(k)}. |
Then, the interior mapping is updated by solving the linear system
[L_S(f^{(k)})]_{\mathtt{I}^{(k)}, \mathtt{I}^{(k)}} \mathbf{h}^{(k+1)}_{\mathtt{I}^{(k)}} = -[L_S(f^{(k)})]_{\mathtt{I}^{(k)}, \mathtt{B}^{(k)}} \mathbf{h}^{(k)}_{\mathtt{B}^{(k)}}, |
while the boundary mapping remains the same, i.e., \mathbf{h}^{(k)}_{\mathtt{B}^{(k+1)}} = \mathbf{h}^{(k)}_{\mathtt{B}^{(k)}} . The updated spherical mapping f^{(k+1)}\colon {\mathcal M}\to S^2 is computed by
f^{(k+1)}(v_i) = \varPi_{ S^2}^{-1}(\mathbf{h}^{(k)}_i), |
where \varPi_{ S^2}^{-1} is the inverse stereographic projection (5.5). This procedure is summarized by Algorithm 2.
Algorithm 2: FPI method of the SEM [21]. |
1 Given a genus-zero closed mesh {\mathcal M} , a tolerance \varepsilon , a radius r (e.g., \varepsilon = 10^{-6} , r = 1.2 );
Result: A spherical area-preserving parameterization \mathbf{f} . 2 Compute a spherical conformal map \mathbf{g} using the Laplacian linearization method [4]; 3 Perform the stereographic projection \mathbf{h}_\ell = \varPi_{ S^2}(\mathbf{g}_\ell), \ell = 1, \ldots, n , as in (5.1); 4 Let \delta = \infty ; ![]() |
Table 6 reports the numerical results for the FPI method applied to the twelve benchmark mesh models considered. Two different stopping criteria are considered: increase in authalic energy (columns 2 to 6) and maximum number of iterations (columns 7 to 10). From Table 6, it appears that performing more iterations of the FPI method does not necessarily improve the mapping f . In most cases, except for David Head and Cortical Surface, the authalic energy and the values of SD/Mean increase. This motivates us to use the FPI method only to calculate a good initial mapping and then switch to RGD.
Energy E_{A} Increased | 100 Iterations | ||||||||||
Model Name | SD/Mean | E_{A}(f) | Time | #Fs | #Its | SD/Mean | E_{A}(f) | Time | #Fs | ||
Right Hand | 0.2050 | 2.86 \times 10^{-1} | 0.08 | 12 | 6 | 0.4598 | 2.92 \times 10^{0} | 1.35 | 67 | ||
David Head | 0.0191 | 4.66 \times 10^{-3} | 0.35 | 0 | 8 | 0.0169 | 3.58 \times 10^{-3} | 4.30 | 0 | ||
Cortical Surface | 0.0220 | 4.93 \times 10^{-3} | 0.85 | 0 | 15 | 0.0174 | 3.21 \times 10^{-3} | 5.62 | 0 | ||
Bull | 0.1504 | 2.74 \times 10^{-1} | 1.29 | 8 | 18 | 0.1876 | 4.59 \times 10^{-1} | 6.90 | 40 | ||
Bulldog | 0.0381 | 1.49 \times 10^{-2} | 2.61 | 0 | 10 | 0.1833 | 3.99 \times 10^{-1} | 22.22 | 53 | ||
Lion Statue | 0.1940 | 5.10 \times 10^{-1} | 1.12 | 1 | 4 | 0.2064 | 5.28 \times 10^{-1} | 23.67 | 38 | ||
Gargoyle | 0.0704 | 5.47 \times 10^{-2} | 2.64 | 0 | 11 | 4.1020 | 4.85 \times 10^{2} | 36.10 | 1955 | ||
Max Planck | 0.0544 | 3.67 \times 10^{-2} | 1.35 | 0 | 5 | 0.1844 | 1.67 \times 10^{1} | 25.99 | 144 | ||
Bunny | 0.0423 | 2.24 \times 10^{-2} | 6.40 | 0 | 20 | 0.0394 | 3.96 \times 10^{-2} | 35.78 | 2 | ||
Chess King | 0.0713 | 6.91 \times 10^{-2} | 6.35 | 9 | 8 | 1.0903 | 1.79 \times 10^{1} | 88.04 | 1655 | ||
Art Statuette | 0.0411 | 2.15 \times 10^{-2} | 23.27 | 0 | 7 | 0.0908 | 1.07 \times 10^{-1} | 342.95 | 126 | ||
Bimba Statue | 0.0515 | 3.32 \times 10^{-2} | 29.94 | 6 | 9 | 0.0932 | 7.42 \times 10^{-2} | 305.00 | 144 |
In this section, we compare the numerical results of our RGD method with the adaptive area-preserving parameterization for genus-zero closed surfaces proposed by Choi et al. [7]. The computational procedure is summarized as follows. First, the mesh is punctured by removing two triangular faces \tau_1 and \tau_2 that share a common edge. Then, the FPI of the SEM [22] is applied to compute an area-preserving initial mapping {g}_0\colon {\mathcal M}\backslash\{\tau_1, \tau_2\}\to\mathbb{D} := \{\mathbf{x}\in\mathbb{R}^2 \mid \|\mathbf{x}\|_2\leq 1\} . The Beltrami coefficient of the mapping {g}_0 is denoted by \mu_{g_0} . Next, a quasi-conformal map g\colon {\mathcal M}\backslash\{\tau_1, \tau_2\}\to\mathbb{D} with \|\mu_g\|_\infty = \|\lambda\mu_{g_0}\|_\infty < 1 is constructed [9]. The scaling factor \lambda\in[0, 1] is chosen to be 0.2 in practice. Then, the optimal mass transport mapping h_r\colon {\mathcal M}\backslash\{\tau_1, \tau_2\}\to r\mathbb{D} is computed by the method proposed by Zhao et al. [23], with the optimal radius r satisfying
r = \mathop {{\rm{argmin}}}\limits_{} r\int |\mu_{(h_r\circ rg)^{-1}(z)}|^2 \, \mathrm{d}z. |
The final spherical area-preserving parameterization is obtained by the composition mapping f = \varPi_{ S^{2}}^{-1}\circ h_r \circ rg , where \varPi_{ S^{2}}^{-1} denotes the inverse stereographic projection (5.5).
Table 7 reports on the numerical results. The algorithm of Choi et al. [7] was run with the default parameters found in the code package*. The only satisfactory results are those for the David Head mesh model. However, even in this case, the values of the monitored quantities (SD/Mean, E_{A}(f) , and computation time) do not compete with those obtained by running our RGD method for only ten iterations; compare with the fourth column of Table 2. Our method is much more efficient and accurate and provides mappings with better bijectivity properties.
*Available at https://www.math.cuhk.edu.hk/ptchoi/files/adaptiveareapreservingmap.zip.
Model Name | SD/Mean | E_{A}(f) | Time | #Foldings |
Right Hand | 18.3283 | 4.84 \times 10^{3} | 218.03 | 672 |
David Head | 0.0426 | 2.27 \times 10^{-2} | 298.76 | 0 |
Cortical Surface | 0.6320 | 1.14 \times 10^{0} | 420.20 | 10 |
Bull | 8.5565 | 1.82 \times 10^{3} | 34.42 | 335 |
Bulldog | 9.2379 | 1.22 \times 10^{3} | 183.94 | 338 |
Lion Statue | 0.2626 | 8.96 \times 10^{-1} | 1498.91 | 540 |
Gargoyle | 0.3558 | 1.30 \times 10^{0} | 1483.35 | 571 |
Max Planck | 11.6875 | 1.49 \times 10^{3} | 195.39 | 575 |
Bunny | 27.6014 | 8.94 \times 10^{3} | 157.87 | 208 |
Chess King | 11.8300 | 1.65 \times 10^{3} | 608.55 | 948 |
Art Statuette | 394.4414 | 9.93 \times 10^{0} | 2284.79 | 2242 |
Bimba Statue | 0.5110 | 2.01 \times 10^{0} | 16 773.34 | 11 821 |
In this section, we compare the numerical results of our RGD method with the spherical optimal transportation mapping proposed by Cui et al. [10]. The computational procedure can be summarized as follows. First, the algorithm calculates a conformal map from a genus-zero closed surface {\mathcal M} to the unit sphere and then normalizes the area of {\mathcal M} . Iteratively, a power diagram is computed from the sphere and the power radii. The gradient of the energy is calculated from the vertices, followed by the Hessian matrix, which depends on the source measure. A linear equation is solved at every step to update the power radii, and a line search strategy is used. The step length parameter is chosen so that all cells of the power diagram are non-degenerated. If some cells are degenerated, the step length is reduced by half, and the power diagram is computed again until all cells are non-degenerated. After the loop, the centroid of each power cell in the power diagram is computed, and the mapping from each vertex to each centroid of the power cell is returned. The algorithmic details can be found in [10,Algorithm 1].
Here, we run the algorithm of [10] with the default parameters found in the code package†. The target mesh for the mesh model was adapted to be its spherical harmonic mapping as suggested in [10].
†Available at https://www3.cs.stonybrook.edu/gu/software/SOT/index.html
From Table 8, it appears that the method of [10] is capable, at least when working, of computing bijective spherical mappings within a relatively moderate number of iterations. However, the area-preserving properties, as measured by SD/Mean and E_{A}(f) , are inferior to those obtained with our RGD method for all four mesh models considered.
Model Name | SD/Mean | E_{A}(f) | #Foldings | # Iterations |
David Head | 0.4189 | 2.25 \times 10^{0} | 0 | 27 |
Cortical Surface | 0.5113 | 3.11 \times 10^{0} | 0 | 27 |
Bulldog | 0.8665 | 1.00 \times 10^{1} | 0 | 33 |
Max Planck | 0.5619 | 4.38 \times 10^{0} | 0 | 25 |
In this section, we investigate the numerical stability of our scheme. To this aim, we introduce Gaussian random noise to the vertex normal of each vertex in every mesh model according to a given value of noise variance \sigma_{\mathrm{noise}} . We then re-run the entire algorithm, i.e., we first perform a few iterations of the FPI method (Algorithm 2) to obtain a mapping that is used as an initial mapping for the RGD method (Algorithm 1). We then calculate a relative error on the authalic energy, as defined below in (5.6). We repeat this procedure for different values of noise variance \sigma_{\mathrm{noise}} .
Figure 10 shows the original mesh model of the Lion Statue (panel (a)) and two noisy versions (panels (b) and (c)).
We compute the following relative error on the authalic energy
\begin{equation} \text{err-}E_{A}\big(f, \tilde{f}\big) := \#\text{Vertices} \times \frac{\lvert {E}_{A}\big(\tilde{f}\big) - E_{A}(f) \rvert}{\sum\limits_{v\in\mathcal{V}( {\mathcal M})} \| \widetilde{v} - v\|_2 }, \end{equation} | (5.6) |
where {E}_{A}\big(\tilde{f}\big) and E_{A}(f) are the authalic energies after 100 iterations of RGD for the mesh model with and without noise, respectively, and \widetilde{v} and v denote the coordinates of the vertices of the mesh model with and without noise, respectively.
Tables 9 and 10 report the numerical results for all the noisy mesh models, with \sigma_{\mathrm{noise}} = 1 \times 10^{-3} and 5 \times 10^{-3} , respectively. The values of authalic energy for the non-noisy mesh models from Table 2 are reported in the second last column for easier comparison. We observe that the values for SD/Mean and E_{A}\big(\tilde{f}\big) remain bounded and reasonable with respect to the original mesh models, demonstrating that our method is stable to noise.
\sigma_{\mathrm{noise}} = 1 \times 10^{-3} | |||||||||
Model Name | SD/Mean | E_{A}\big(\tilde{f}\big) | Time | #Fs | #Fs after b.c. | E_{A}(f) | err- E_{A}\big(f, \tilde{f}\big) | ||
Right Hand | 0.1254 | 1.03 \times 10^{-1} | 3.13 | 14 | 1 | 9.40 \times 10^{-2} | 5.84 \times 10^{-2} | ||
David Head | 0.0108 | 1.44 \times 10^{-3} | 9.98 | 0 | 0 | 3.04 \times 10^{-3} | 4.47 \times 10^{-3} | ||
Cortical Surface | 0.0187 | 3.82 \times 10^{-3} | 12.78 | 0 | 0 | 3.72 \times 10^{-3} | 3.45 \times 10^{-3} | ||
Bull | 0.2528 | 9.23 \times 10^{-1} | 16.74 | 19 | 6 | 2.19 \times 10^{-1} | 1.12 \times 10^{0} | ||
Bulldog | 0.0273 | 6.94 \times 10^{-3} | 59.26 | 0 | 0 | 1.27 \times 10^{-2} | 7.19 \times 10^{-6} | ||
Lion Statue | 0.1630 | 3.27 \times 10^{-1} | 66.78 | 1 | 0 | 4.54 \times 10^{-1} | 1.36 \times 10^{-4} | ||
Gargoyle | 0.1677 | 3.66 \times 10^{-1} | 65.72 | 0 | 0 | 4.76 \times 10^{-2} | 4.45 \times 10^{-3} | ||
Max Planck | 0.0464 | 2.67 \times 10^{-2} | 64.30 | 0 | 0 | 3.39 \times 10^{-2} | 5.27 \times 10^{-5} | ||
Bunny | 0.0297 | 1.11 \times 10^{-2} | 81.31 | 0 | 0 | 1.91 \times 10^{-2} | 5.32 \times 10^{-3} | ||
Chess King | 0.0747 | 6.08 \times 10^{-2} | 193.04 | 119 | 38 | 5.23 \times 10^{-2} | 1.03 \times 10^{-3} | ||
Art Statuette | 0.0235 | 6.88 \times 10^{-3} | 636.30 | 0 | 0 | 2.10 \times 10^{-2} | 2.54 \times 10^{-2} | ||
Bimba Statue | 0.0541 | 3.33 \times 10^{-2} | 759.36 | 2 | 0 | 3.29 \times 10^{-2} | 5.81 \times 10^{-4} |
\sigma_{\mathrm{noise}} = 5 \times 10^{-3} | |||||||||
Model Name | SD/Mean | E_{A}\big(\tilde{f}\big) | Time | #Fs | #Fs after b.c. | E_{A}(f) | err- E_{A}\big(f, \tilde{f}\big) | ||
Right Hand | 0.2304 | 5.77 \times 10^{-1} | 3.12 | 10 | 4 | 9.40 \times 10^{-2} | 1.45 \times 10^{0} | ||
David Head | 0.0211 | 5.51 \times 10^{-3} | 9.43 | 0 | 0 | 3.04 \times 10^{-3} | 6.84 \times 10^{-3} | ||
Cortical Surface | 0.0301 | 1.02 \times 10^{-2} | 12.72 | 0 | 0 | 3.72 \times 10^{-3} | 2.28 \times 10^{-1} | ||
Bull | 0.1723 | 2.98 \times 10^{-1} | 17.40 | 18 | 4 | 2.19 \times 10^{-1} | 1.19 \times 10^{-1} | ||
Bulldog | 0.0629 | 3.86 \times 10^{-2} | 61.75 | 2 | 0 | 1.27 \times 10^{-2} | 3.23 \times 10^{-5} | ||
Lion Statue | 0.1044 | 1.36 \times 10^{-1} | 69.55 | 0 | 0 | 4.54 \times 10^{-1} | 3.36 \times 10^{-4} | ||
Gargoyle | 0.0974 | 9.68 \times 10^{-2} | 69.32 | 1 | 0 | 4.76 \times 10^{-2} | 6.87 \times 10^{-4} | ||
Max Planck | 0.0782 | 7.11 \times 10^{-2} | 67.30 | 0 | 0 | 3.39 \times 10^{-2} | 2.75 \times 10^{-4} | ||
Bunny | 0.0455 | 2.57 \times 10^{-2} | 80.93 | 0 | 0 | 1.91 \times 10^{-2} | 4.40 \times 10^{-3} | ||
Chess King | 0.1521 | 2.72 \times 10^{-1} | 187.57 | 95 | 35 | 5.23 \times 10^{-2} | 2.64 \times 10^{-2} | ||
Art Statuette | 0.0981 | 5.41 \times 10^{-2} | 617.75 | 0 | 0 | 2.10 \times 10^{-2} | 5.92 \times 10^{-2} | ||
Bimba Statue | 0.1083 | 1.25 \times 10^{-1} | 743.00 | 76 | 0 | 3.29 \times 10^{-2} | 1.68 \times 10^{-1} |
A registration mapping between surfaces {\mathcal M}_0 and {\mathcal M}_1 refers to a bijective mapping g \colon {\mathcal M}_0\to {\mathcal M}_1 . An ideal registration mapping keeps important landmarks aligned while preserving specified geometry properties. In this section, we demonstrate a framework for the computation of landmark-aligned area-preserving parameterizations of genus-zero closed surfaces.
Suppose a set of landmark pairs \{(p_i, q_i) \mid p_i\in {\mathcal M}_0, \ q_i\in {\mathcal M}_1\}_{i = 1}^m is given. The goal is to compute an area-preserving simplicial mapping g\colon {\mathcal M}_0\to {\mathcal M}_1 that satisfies g(p_i) \approx q_i , for i = 1, \ldots, m . First, we compute area-preserving parameterizations f_0\colon {\mathcal M}_0\to S^{2} and f_1\colon {\mathcal M}_1\to S^{2} of surfaces {\mathcal M}_0 and {\mathcal M}_1 , respectively. The simplicial registration mapping h \colon S^{2} \to S^{2} that satisfies h\circ f_0(p_i) = f_1(q_i) , for i = 1, \ldots, m , can be carried out by minimizing the registration energy
E_R(h) = E_S(h) + \sum\limits_{i = 1}^m \lambda_i \| h\circ f_0(p_i) - f_1(q_i) \|^2. |
Let
\mathbf{h} = \begin{bmatrix} (h\circ f_0(v_1)) ^{\top} \\ \vdots \\ (h\circ f_0(v_n)) ^{\top} \end{bmatrix} = \begin{bmatrix} \mathbf{h}^1 & \mathbf{h}^2 & \mathbf{h}^3 \end{bmatrix} |
be the matrix representation of h . The gradient of E_R with respect to \mathbf{h} can be formulated as
\nabla E_R(h) = 2 \, (I_3\otimes L_S(h)) {\rm{vec}}(\mathbf{h}) + {\rm{vec}}(\mathbf{r}), |
where \mathbf{r} is the matrix of the same size as \mathbf{h} given by
\mathbf{r}(i, :) = \begin{cases} 2\lambda_i\left(\mathbf{h}(i, :) - (f_1(q_i)) ^{\top}\right) & \text{if} ~~ p_i ~~ \text{is a landmark}, \\ (0, 0, 0) & \text{otherwise}. \end{cases} |
In practice, we define the midpoints c_i of each landmark pairs on S^{2} as
c_i = \frac{1}{2}( f_0(p_i) + f_1(q_i)), |
for i = 1, \ldots, m , and compute h_0 and h_1 on S^{2} that satisfy h_0\circ f_0(p_i) = c_i and h_1\circ f_1(q_i) = c_i , respectively. Ultimately, the registration mapping g\colon {\mathcal M}_0\to {\mathcal M}_1 is obtained by the composition mapping g = f_1^{-1} \circ h_1^{-1} \circ h_0 \circ f_0 . Figure 11 schematizes this composition of functions for the landmark-aligned morphing process from one brain to another.
A landmark-aligned morphing process from {\mathcal M}_0 to {\mathcal M}_1 can be constructed by the linear homotopy H\colon {\mathcal M}_0\times[0, 1]\to\mathbb{R}^3 defined as
\begin{equation} H(v, t) = (1-t) \, v + t \, g(v). \end{equation} | (5.7) |
In Figure 12, we demonstrate the morphing process from one brain to another brain by four snapshots at four different values of t . The brain surfaces are obtained from the source code package in [9].
In this paper, we introduced an RGD method for computing spherical area-preserving mappings of genus-zero closed surfaces. Our approach combines the tools of Riemannian optimization and computational geometry to develop a method based on the minimization of the normalized stretch energy. The proposed algorithm has theoretically guaranteed convergence and is accurate, efficient, and robust. We tested two different line-search strategies and conducted extensive numerical experiments on various mesh models to demonstrate the algorithm's stability and effectiveness. By comparing with three existing methods for computing area-preserving mappings, we demonstrated that our algorithm is more efficient than these state-of-the-art methods. Moreover, we show that our approach is stable and robust even when the mesh model undergoes small perturbations. Finally, we applied our algorithm to the practical problem of landmark-aligned surface registration between two human brain models.
There are some directions in which we could conduct further research. Specifically, we would like to enhance the speed of convergence of the algorithm we have proposed while keeping the computational cost low. One potential way to improve this would be to use appropriate Riemannian generalizations of the conjugate gradient method or the limited memory BFGS (L-BFGS) method, as suggested in [17]. Another research direction may target genus-one or higher genus closed surfaces.
The image area of the simplicial mapping f can be calculated as follows. Let \tau = [v_i, v_j, v_k] and denote f_{ij}^\ell = f_{i}^\ell - f_{j}^\ell , f_{ik}^\ell = f_{i}^\ell - f_{k}^\ell , and f_{jk}^\ell = f_{ij}^\ell - f_{k}^\ell , for \ell = 1, 2, 3 . The image area of a simplicial mapping f can be formulated as
{\mathcal A}(f) = \sum\limits_{\tau\in {\mathcal F}( {\mathcal M})} |f(\tau)| = \sum\limits_{\tau\in {\mathcal F}( {\mathcal M})} \frac{1}{2} \sqrt{ {\mathcal A}_{12}(f|_\tau)^2 + {\mathcal A}_{13}(f|_\tau)^2 + {\mathcal A}_{23}(f|_\tau)^2}, |
where
{\mathcal A}_{12}(f|_{\tau}) = f_{ij}^1 f_{ik}^2 - f_{ij}^2 f_{ik}^1, \quad {\mathcal A}_{13}(f|_{\tau}) = f_{ij}^1 f_{ik}^3 - f_{ij}^3 f_{ik}^1, \quad {\mathcal A}_{23}(f|_{\tau}) = f_{ij}^2 f_{ik}^3 - f_{ij}^3 f_{ik}^2. |
The functionals {\mathcal A}_{12} , {\mathcal A}_{13} and {\mathcal A}_{23} measure the image area of mappings \varPi_{P_{xy}}\circ f , \varPi_{P_{xz}}\circ f and \varPi_{P_{yz}}\circ f , respectively. The partial derivatives of {\mathcal A}(f|_{\tau}) can be formulated as
\begin{align*} \frac{\partial}{\partial f_i^1} {\mathcal A}(f|_{\tau}) & = \frac{ 1}{4 {\mathcal A}(f|_{\tau})} \left( {\mathcal A}_{12}(f|_{\tau}) f_{jk}^2 + {\mathcal A}_{13}(f|_{\tau}) f_{jk}^3 \right), \\ \frac{\partial}{\partial f_i^2} {\mathcal A}(f|_{\tau}) & = \frac{-1}{4 {\mathcal A}(f|_{\tau})} \left( {\mathcal A}_{12}(f|_{\tau}) f_{jk}^1 - {\mathcal A}_{23}(f|_{\tau}) f_{jk}^3 \right), \\ \frac{\partial}{\partial f_i^3} {\mathcal A}(f|_{\tau}) & = \frac{-1}{4 {\mathcal A}(f|_{\tau})} \left( {\mathcal A}_{13}(f|_{\tau}) f_{jk}^1 - {\mathcal A}_{23}(f|_{\tau}) f_{jk}^2 \right), \\ \frac{\partial}{\partial f_j^1} {\mathcal A}(f|_{\tau}) & = \frac{-1}{4 {\mathcal A}(f|_{\tau})} \left( {\mathcal A}_{12}(f|_{\tau}) f_{ik}^2 + {\mathcal A}_{13}(f|_{\tau}) f_{ik}^3 \right), \\ \frac{\partial}{\partial f_j^2} {\mathcal A}(f|_{\tau}) & = \frac{ 1}{4 {\mathcal A}(f|_{\tau})} \left( {\mathcal A}_{12}(f|_{\tau}) f_{ik}^1 - {\mathcal A}_{23}(f|_{\tau}) f_{ik}^3 \right), \\ \frac{\partial}{\partial f_j^3} {\mathcal A}(f|_{\tau}) & = \frac{ 1}{4 {\mathcal A}(f|_{\tau})} \left( {\mathcal A}_{13}(f|_{\tau}) f_{ik}^1 + {\mathcal A}_{23}(f|_{\tau}) f_{ik}^2 \right), \\ \frac{\partial}{\partial f_k^1} {\mathcal A}(f|_{\tau}) & = \frac{ 1}{4 {\mathcal A}(f|_{\tau})} \left( {\mathcal A}_{12}(f|_{\tau}) f_{ij}^2 + {\mathcal A}_{13}(f|_{\tau}) f_{ij}^3 \right), \\ \frac{\partial}{\partial f_k^2} {\mathcal A}(f|_{\tau}) & = \frac{-1}{4 {\mathcal A}(f|_{\tau})} \left( {\mathcal A}_{12}(f|_{\tau}) f_{ij}^1 - {\mathcal A}_{23}(f|_{\tau}) f_{ij}^3 \right), \\ \frac{\partial}{\partial f_k^3} {\mathcal A}(f|_{\tau}) & = \frac{-1}{4 {\mathcal A}(f|_{\tau})} \left( {\mathcal A}_{13}(f|_{\tau}) f_{ij}^1 + {\mathcal A}_{23}(f|_{\tau}) f_{ij}^2 \right). \end{align*} |
In this appendix, we describe the calculation of the Hessian of the stretch energy functional. Let \tau = [v_i, v_j, v_k] . From [20,Theorem 3.5 and (3.1)], we know that
\begin{align*} \nabla_{\mathbf{f}^\ell} E_S(f|_\tau) = 2L_S(f|_\tau) \, \mathbf{f}|_\tau^\ell, \; \ell = 1, 2, 3, \end{align*} |
where
\begin{align*} L_S(f|_\tau) = \frac{1}{4|\tau |} \begin{bmatrix} \begin{array}{c} (\mathbf{f}_i-\mathbf{f}_k)^\top (\mathbf{f}_j-\mathbf{f}_k)\\+(\mathbf{f}_i-\mathbf{f}_j)^\top (\mathbf{f}_k-\mathbf{f}_j) \end{array} & -(\mathbf{f}_i-\mathbf{f}_k)^\top (\mathbf{f}_j-\mathbf{f}_k) & - (\mathbf{f}_i-\mathbf{f}_j)^\top (\mathbf{f}_k-\mathbf{f}_j) \\[0.5cm] -(\mathbf{f}_i-\mathbf{f}_k)^\top (\mathbf{f}_j-\mathbf{f}_k) & \begin{array}{c} (\mathbf{f}_i-\mathbf{f}_k)^\top (\mathbf{f}_j-\mathbf{f}_k) \\ +(\mathbf{f}_j-\mathbf{f}_i)^\top (\mathbf{f}_k-\mathbf{f}_i) \end{array} & -(\mathbf{f}_j-\mathbf{f}_i)^\top (\mathbf{f}_k-\mathbf{f}_i) \\[0.5cm] -(\mathbf{f}_i-\mathbf{f}_j)^\top (\mathbf{f}_k-\mathbf{f}_j) & -(\mathbf{f}_j-\mathbf{f}_i)^\top (\mathbf{f}_k-\mathbf{f}_i) & \begin{array}{c} (\mathbf{f}_i-\mathbf{f}_j)^\top (\mathbf{f}_k-\mathbf{f}_j) \\ + (\mathbf{f}_j-\mathbf{f}_i)^\top (\mathbf{f}_k-\mathbf{f}_i) \end{array} \end{bmatrix}, \end{align*} |
and \mathbf{f}|_\tau^\ell = (\mathbf{f}_i^\ell, \mathbf{f}_j^\ell, \mathbf{f}_k^\ell)^\top , \ell = 1, 2, 3 . A direct calculation yields that the Hessian matrix
\mathrm{Hess}(E_S(f|_{\tau})) = \begin{bmatrix} \frac{\partial E_S(f|_\tau)}{\partial f_i^s \partial f_i^t} & \frac{\partial E_S(f|_\tau)}{\partial f_i^s \partial f_j^t} & \frac{\partial E_S(f|_\tau)}{\partial f_i^s \partial f_k^t} \\[0.5cm] \frac{\partial E_S(f|_\tau)}{\partial f_j^s \partial f_i^t} & \frac{\partial E_S(f|_\tau)}{\partial f_j^s \partial f_j^t} & \frac{\partial E_S(f|_\tau)}{\partial f_j^s \partial f_k^t} \\[0.5cm] \frac{\partial E_S(f|_\tau)}{\partial f_k^s \partial f_i^t} & \frac{\partial E_S(f|_\tau)}{\partial f_k^s \partial f_j^t} & \frac{\partial E_S(f|_\tau)}{\partial f_k^s \partial f_k^t} \end{bmatrix}_{s, t = 1}^3 |
can be formulated as
\mathrm{Hess}(E_S(f|_{\tau})) = \frac{1}{2|\tau|} \begin{bmatrix} \mathbf{h}_{ijk}^2 {\mathbf{h}_{ijk}^2} ^{\top} + \mathbf{h}_{ijk}^3 {\mathbf{h}_{ijk}^3} ^{\top} & \mathbf{h}_{ijk}^1 {\mathbf{h}_{ijk}^2} ^{\top} - 2\mathbf{h}_{ijk}^2 {\mathbf{h}_{ijk}^1} ^{\top} & \mathbf{h}_{ijk}^1 {\mathbf{h}_{ijk}^3} ^{\top} - 2\mathbf{h}_{ijk}^3 {\mathbf{h}_{ijk}^1}^\top \\[0.5cm] \mathbf{h}_{ijk}^2 {\mathbf{h}_{ijk}^1}^\top - 2\mathbf{h}_{ijk}^1 {\mathbf{h}_{ijk}^2}^\top & \mathbf{h}_{ijk}^1 {\mathbf{h}_{ijk}^1}^\top + \mathbf{h}_{ijk}^3 {\mathbf{h}_{ijk}^3}^\top & \mathbf{h}_{ijk}^2 {\mathbf{h}_{ijk}^3}^\top - 2\mathbf{h}_{ijk}^3 {\mathbf{h}_{ijk}^2}^\top \\[0.5cm] \mathbf{h}_{ijk}^3 {\mathbf{h}_{ijk}^1}^\top - 2\mathbf{h}_{ijk}^1 {\mathbf{h}_{ijk}^3}^\top & \mathbf{h}_{ijk}^3 {\mathbf{h}_{ijk}^2}^\top - 2\mathbf{h}_{ijk}^2 {\mathbf{h}_{ijk}^3}^\top & \mathbf{h}_{ijk}^1 {\mathbf{h}_{ijk}^1}^\top + \mathbf{h}_{ijk}^2 {\mathbf{h}_{ijk}^2}^\top \end{bmatrix}, |
where \mathbf{h}_{ijk}^\ell = (f_j^\ell-f_k^\ell, f_k^\ell-f_i^\ell, f_i^\ell-f_j^\ell) ^{\top} , \ell = 1, 2, 3 .
In this appendix, we describe the line-search procedure used in our RGD method. We start by detailing how to compute the derivative appearing in the sufficient decrease condition, and then we describe the interpolant line-search strategy from [11,§ 6.3.2].
In Euclidean space \mathbb{R}^{n} , the steepest descent method updates a current iterate by moving in the direction of the anti-gradient, by a step size chosen according to an appropriate line-search rule. The step size is related to the sufficient decrease condition [16]
\begin{equation} \phi(\alpha_{k}) \leq \phi(0) + c_{1} \alpha_{k} \phi'(0). \end{equation} | (C.1) |
In the Euclidean setting, the univariate function \phi(\alpha) in the line-search procedure is
\phi(\alpha) = E\big( \mathbf{f}^{(k)} + \alpha \, \mathbf{d}^{(k)}\big), |
where E is the objective function considered, and \mathbf{f}^{(k)} is the current iterate. However, this function changes in the Riemannian optimization framework because we cannot directly perform the vector addition \mathbf{f}^{(k)} + \alpha \, \mathbf{d}^{(k)} without leaving the manifold.
Let \mathbf{f}^{(k)} be a point of \big(S^{2}\big)^{n} at the k th iteration of our RGD algorithm, and \text{R}_{ \mathbf{f}^{(k)}} the retraction at \mathbf{f}^{(k)} , as defined in subsection 3.2.3. Let the objective function be E \colon \big(S^{2}\big)^{n} \to \mathbb{R} . For a fixed point \mathbf{f}^{(k)} and a fixed tangent vector \mathbf{d}^{(k)} , we introduce the vector-valued function \boldsymbol{\psi} \colon \mathbb{R} \to \big(S^{2}\big)^{n} , defined by \alpha \mapsto \text{R}_{ \mathbf{f}^{(k)}}(\alpha\, \mathbf{d}^{(k)}) . Hence, by composition of functions, we have \phi \colon \mathbb{R} \to \mathbb{R} , defined by
\phi(\alpha) := E(\boldsymbol{\psi} (\alpha)). |
Note that \phi(0) = E(\boldsymbol{\psi}(0)) = E(\mathbf{f}^{(k)}) =: E^{(k)} , due to the definition of retraction.
To evaluate the sufficient decrease condition (9.1), we need to calculate \phi'(0) , and to compute \phi'(0) we need to calculate the derivative of the retraction \text{R}_{ \mathbf{f}^{(k)}} (\alpha \, \mathbf{d}^{(k)}) with respect to \alpha . The derivative of \phi(\alpha) is given by the chain rule
\phi'(\alpha) = \nabla E(\boldsymbol{\psi}(\alpha)) ^{\top} \boldsymbol{\psi}'(\alpha), |
and then we evaluate it at \alpha = 0 , i.e.,
\phi'(0) = \nabla E( \mathbf{f}^{(k)}) ^{\top} \boldsymbol{\psi}'(0). |
In general, the retraction and the derivative \boldsymbol{\psi}'(\alpha) depend on the choice of the manifold. The differentials of a retraction provide the so-called vector transports, and the derivative of the retraction on the unit sphere appears in [2,§ 8.1.2].
In the following formulas, we omit the superscript ^{(k)} referring to the current iteration of RGD and assume that the line-search direction \mathbf{d} is also partitioned as the matrix \mathbf{f} ; see (2.1). Recalling the retraction on the power manifold \big(S^{2}\big)^{n} from (3.3), we can write \boldsymbol{\psi}(\alpha) as
\boldsymbol{\psi}(\alpha) = \text{R}_{ \mathbf{f}}(\alpha \, \mathbf{d}) = \begin{bmatrix} \dfrac{1}{\| \mathbf{f}_{1} + \alpha \, \mathbf{d}_{1}\|_{2}} & & & \\ & \dfrac{1}{\| \mathbf{f}_{2} + \alpha \, \mathbf{d}_{2}\|_{2}} & & \\ & & \ddots & \\ & & & \dfrac{1}{\| \mathbf{f}_{n} + \alpha \, \mathbf{d}_{n}\|_{2}} \end{bmatrix} \begin{bmatrix} \mathbf{f}_{1} ^{\top} + \alpha \, \mathbf{d}_{1} ^{\top} \\ \mathbf{f}_{2} ^{\top} + \alpha \, \mathbf{d}_{2} ^{\top} \\ \vdots \\ \mathbf{f}_{n} ^{\top} + \alpha \, \mathbf{d}_{n} ^{\top} \end{bmatrix}. |
The derivative \boldsymbol{\psi}'(\alpha) can be computed via the formula (cf. [2,Example 8.1.4])
\boldsymbol{\psi}'(\alpha) = \begin{bmatrix} -\dfrac{( \mathbf{f}_{1} + \alpha \, \mathbf{d}_{1}) ^{\top} \mathbf{d}_{1}}{\| \mathbf{f}_{1} + \alpha \, \mathbf{d}_{1}\|_{2}^{3}} \, ( \mathbf{f}_{1} + \alpha \, \mathbf{d}_{1}) ^{\top} \\[12pt] -\dfrac{( \mathbf{f}_{2} + \alpha \, \mathbf{d}_{2}) ^{\top} \mathbf{d}_{2}}{\| \mathbf{f}_{2} + \alpha \, \mathbf{d}_{2}\|_{2}^{3}} \, ( \mathbf{f}_{2} + \alpha \, \mathbf{d}_{2}) ^{\top} \\[12pt] \vdots \\[12pt] -\dfrac{( \mathbf{f}_{n} + \alpha \, \mathbf{d}_{n}) ^{\top} \mathbf{d}_{n}}{\| \mathbf{f}_{n} + \alpha \, \mathbf{d}_{n}\|_{2}^{3}} \, ( \mathbf{f}_{n} + \alpha \, \mathbf{d}_{n}) ^{\top} \end{bmatrix} + \begin{bmatrix} \dfrac{ \mathbf{d}_{1} ^{\top}}{\| \mathbf{f}_{1} + \alpha \, \mathbf{d}_{1}\|_{2}} \\[12pt] \dfrac{ \mathbf{d}_{2} ^{\top}}{\| \mathbf{f}_{2} + \alpha \, \mathbf{d}_{2}\|_{2}} \\[12pt] \vdots \\[12pt] \dfrac{ \mathbf{d}_{n} ^{\top}}{\| \mathbf{f}_{n} + \alpha \, \mathbf{d}_{n}\|_{2}} \end{bmatrix}, |
At \alpha = 0 , this simplifies into
\boldsymbol{\psi}'(0) = \begin{bmatrix} \dfrac{ \mathbf{d}_{1} ^{\top}}{\| \mathbf{f}_{1} \|_{2}} \\[12pt] \dfrac{ \mathbf{d}_{2} ^{\top}}{\| \mathbf{f}_{2} \|_{2}} \\[12pt] \vdots \\[12pt] \dfrac{ \mathbf{d}_{n} ^{\top}}{\| \mathbf{f}_{n} \|_{2}} \end{bmatrix} - \begin{bmatrix} \dfrac{( \mathbf{f}_{1} ) ^{\top} \mathbf{d}_{1}}{\| \mathbf{f}_{1} \|_{2}^{3}} \, \mathbf{f}_{1} ^{\top} \\[12pt] \dfrac{( \mathbf{f}_{2} ) ^{\top} \mathbf{d}_{2}}{\| \mathbf{f}_{2} \|_{2}^{3}} \, \mathbf{f}_{2} ^{\top} \\[12pt] \vdots \\[12pt] \dfrac{( \mathbf{f}_{n} ) ^{\top} \mathbf{d}_{n}}{\| \mathbf{f}_{n} \|_{2}^{3}} \, \mathbf{f}_{n} ^{\top} \end{bmatrix}. |
This value is needed to evaluate the sufficient decrease condition (9.1).
Quadratic/cubic approximation
At every step of our RGD algorithm, we want to satisfy the sufficient decrease condition (9.1). Here, we adopt the safeguarded quadratic/cubic approximation strategy described in [11,§ 6.3.2].
In the following, we let \alpha_{k} and \alpha_{k-1} denote the step lengths used at iterations k and k-1 of the optimization algorithm, respectively. We denote the initial guess using \alpha_{0} . We suppose that the initial guess is given; alternatively, one can use [16,(3.60)] as initial guess, i.e.,
\alpha_{0} = \frac{2(E^{(k)} - E^{(k-1)})}{\phi'(0)}. |
If \alpha_{0} satisfies the sufficient decrease condition (9.1), i.e.,
\phi(\alpha_{0}) \leq \phi(0) + c_{1} \alpha_{0} \phi'(0), |
then \alpha_{0} is accepted as step length, and we terminate the search. Otherwise, we build a quadratic approximation \phi_{\mathrm{q}}(\alpha) of \phi(\alpha) using the information we have, that is, \phi(0) , \phi'(0) , and \phi(\alpha_{0}) . The quadratic model is
\phi_{\mathrm{q}}(\alpha) = \left[\phi(\alpha_{0}) - \phi(0) -\phi'(0) \, \alpha_{0}\right]\alpha^{2} + \phi'(0)\, \alpha + \phi(0). |
The new trial value \alpha_{1} is defined as the minimizer of this quadratic, i.e.,
\alpha_{1} = - \frac{\phi'(0) \, \alpha_{0}^{2}}{2 \left[\phi(\alpha_{0}) - \phi(0) -\phi'(0) \, \alpha_{0}\right]}. |
We terminate the search if the sufficient decrease condition is satisfied at \alpha_{1} , i.e.,
\phi(\alpha_{1}) \leq \phi(0) + c_{1} \alpha_{1} \phi'(0). |
Otherwise, we need to backtrack again. We now have four pieces of information about \phi(\alpha) , so it is desirable to use all of them. Hence, at this and any subsequent backtrack steps during the current iteration of RGD, we use a cubic model \phi_{\mathrm{c}}(\alpha) that interpolates the four pieces of information \phi(0) , \phi'(0) , and the last two values of \phi(\alpha) , and set \alpha_{k} to the value of \alpha at which \phi_{\mathrm{c}}(\alpha) has its local minimizer.
Let \alpha_{\mathrm{prev}} and \alpha_{\mathrm{2prev}} be the last two previous values of \alpha_{k} tried in the backtrack procedure. The cubic that fits \phi(0) , \phi'(0) , \phi(\alpha_{\mathrm{prev}}) , and \phi(\alpha_{\mathrm{2prev}}) is
\phi_{\mathrm{c}}(\alpha) = a\alpha^{3} + b\alpha^{2} + \phi'(0)\, \alpha + \phi(0), |
where
\begin{bmatrix} a \\ b \end{bmatrix} = \dfrac{1}{\alpha_{\mathrm{prev}}-\alpha_{\mathrm{2prev}}} \begin{bmatrix} \dfrac{1}{\alpha_{\mathrm{prev}}^{2}} & \dfrac{-1}{\alpha_{\mathrm{2prev}}^{2}} \\[14pt] \dfrac{-\alpha_{\mathrm{2prev}}}{\alpha_{\mathrm{prev}}^{2}} & \dfrac{\alpha_{\mathrm{prev}}}{\alpha_{\mathrm{2prev}}^{2}} \end{bmatrix} \begin{bmatrix} \phi(\alpha_{\mathrm{prev}}) - \phi(0) - \phi'(0) \, \alpha_{\mathrm{prev}} \\[6pt] \phi(\alpha_{\mathrm{2prev}}) - \phi(0) - \phi'(0) \, \alpha_{\mathrm{2prev}} \end{bmatrix}. |
The local minimizer of this cubic is given by [11,(6.3.18)]
\frac{-b+\sqrt{b^{2}-3a\phi'(0)}}{3a}, |
and we set \alpha_{k} equal to this value. If necessary, this process is repeated, using a cubic interpolant of \phi(0) , \phi'(0) , and the two most recent values of \phi , namely, \phi(\alpha_{\mathrm{prev}}) and \phi(\alpha_{\mathrm{2prev}}) , until a step size that satisfies the sufficient decrease condition is located. Numerical experiments in Section 5 demonstrate the usage and effectiveness of this line-search technique.
The authors declare they have not used Artificial Intelligence (AI) tools in the creation of this article.
Marco Sutti and Mei-Heng Yueh: conceptualization, data curation, formal analysis, funding acquisition, investigation, methodology development, project administration, resource provision, software development, supervision, validation, visualization, writing of the original draft, review, and editing. All authors of this article have been contributed equally. All authors have read and approved the final version of the manuscript for publication.
The work of the first author was supported by the National Center for Theoretical Sciences in Taiwan (R.O.C.) under the NSTC grant 112-2124-M-002-009-. The work of the second author was supported by the National Science and Technology Council and the National Center for Theoretical Sciences in Taiwan.
The authors declare that they have no conflict of interest.
[1] |
Fernando JA, Francisco A, Manuel AA, et al. (2005) Effects of terrain morphology, sampling density, and interpolation methods on grid DEM accuracy. Photogramm Eng Remote Sens 71: 805–816. https://doi.org/10.14358/pers.71.7.805 doi: 10.14358/pers.71.7.805
![]() |
[2] |
Grohmann CH, Smith MJ, Riccomini C (2011) Multiscale analysis of topographic surface roughness in the Midland Valley, Scotland. IEEE Trans Geosci Remote Sens 49: 1200–1213. https://doi.org/10.1109/tgrs.2010.2053546 doi: 10.1109/tgrs.2010.2053546
![]() |
[3] |
Peter JB, Vernon HS, Jiro S, et al. (2004) Methods for Remote Engineering Geology Terrain Analysis in Boreal Forest Regions of Ontario, Canada. Environ Eng Geosci 10: 229–241. https://doi.org/10.2113/10.3.229 doi: 10.2113/10.3.229
![]() |
[4] |
Fan L, Atkinson PM (2015) Accuracy of digital elevation models derived from terrestrial laser scanning data. IEEE Geosci Remote Sens Lett 12: 1923-1927. https://doi.org/10.1109/LGRS.2015.2438394 doi: 10.1109/LGRS.2015.2438394
![]() |
[5] | Fan L (2014) Uncertainty in terrestrial laser scanning for measuring surface movements at a local scale. University of Southampton: Southampton, UK. |
[6] |
Fan L, Powrie W, Smethurst JA, et al. (2014) The effect of short ground vegetation on terrestrial laser scans at a local scale. ISPRS J Photogramm Remote Sens 95: 42–52. https://doi.org/10.1016/j.isprsjprs.2014.06.003 doi: 10.1016/j.isprsjprs.2014.06.003
![]() |
[7] |
Frankel KL, Dolan JF (2007) Characterizing arid region alluvial fan surface roughness with airborne laser swath mapping digital topographic data. J Geophys Res 112: F02025. https://doi.org/10.1029/2006JF000644 doi: 10.1029/2006JF000644
![]() |
[8] |
Nield JM, Wiggs GFS (2011) The application of terrestrial laser scanning to aeolian saltation cloud measurement and its response to changing surface moisture. Earth Surf Processes Landforms 36: 273–278. https://doi.org/10.1002/esp.2102 doi: 10.1002/esp.2102
![]() |
[9] |
Kristen MB, Wayne LM, Patrick JD, et al. (2013) The Use of LiDAR Terrain Data in Characterizing Surface Roughness and Microtopography. Appl Environ Soil Sci 2013: 891534. https://doi.org/10.1155/2013/891534 doi: 10.1155/2013/891534
![]() |
[10] |
Milenković M, Pfeifer N, Glira P (2015) Applying Terrestrial Laser Scanning for Soil Surface Roughness Assessment. Remote Sens 7: 2007–2045. https://doi.org/10.3390/rs70202007 doi: 10.3390/rs70202007
![]() |
[11] |
Fan L (2020) A comparison between structure-from-motion and terrestrial laser scanning for deriving surface roughness: A case study on a sandy terrain surface. Int Arch Photogramm Remote Sens Spatial Inf Sci 42: 1225–1229. https://doi.org/10.5194/isprs-archives-XLⅡ-3-W10-1225-2020 doi: 10.5194/isprs-archives-XLⅡ-3-W10-1225-2020
![]() |
[12] |
Glenn NF, Streutker DR, Chadwick DJ, et al. (2006) Analysis of LiDAR-derived topographic information for characterizing and differentiating landslide morphology and activity. Geomorphology 73: 131–148. https://doi.org/10.1016/j.geomorph.2005.07.006 doi: 10.1016/j.geomorph.2005.07.006
![]() |
[13] |
Brightman N, Fan L, Zhao Y (2023) Point cloud registration: a mini-review of current state, challenging issues and future directions. AIMS Geosci 9: 68–85. https://doi.org/10.3934/geosci.2023005 doi: 10.3934/geosci.2023005
![]() |
[14] |
Zhao Y, Fan L (2023) Review on deep learning algorithms and benchmark datasets for pairwise global point cloud registration. Remote Sens. 15: 2060. https://doi.org/10.3390/rs15082060 doi: 10.3390/rs15082060
![]() |
[15] |
Trevisani S, Teza G, Guth PL (2023) Hacking the topographic ruggedness index. Geomorphology 439: 108838. https://doi.org/10.1016/j.geomorph.2023.108838 doi: 10.1016/j.geomorph.2023.108838
![]() |
[16] |
Andrle R, Abrahams AD (1989) Fractal techniques and the surface roughness of talus slopes. Earth Surf Processes Landforms 14: 197–209. https://doi.org/10.1002/esp.3290140303 doi: 10.1002/esp.3290140303
![]() |
[17] |
Dusséaux R, Vannier E (2022) Soil surface roughness modelling with the bidirectional autocorrelation function. Biosyst Eng 220: 87–102. https://doi.org/10.1016/j.biosystemseng.2022.05.012 doi: 10.1016/j.biosystemseng.2022.05.012
![]() |
[18] |
Huang CH, Bradford JM (1992) Applications of a Laser Scanner to Quantify Soil Microtopography. Soil Sci Soc Am J 56: 14–21. https://doi.org/10.2136/sssaj1992.03615995005600010002x doi: 10.2136/sssaj1992.03615995005600010002x
![]() |
[19] |
Trevisani S, Teza G, Guth P (2023) A Simplified Geostatistical Approach for Characterizing Key Aspects of Short-Range Roughness. CATENA 223: 106927. https://doi.org/10.1016/j.catena.2023.106927 doi: 10.1016/j.catena.2023.106927
![]() |
[20] |
Shepard MK, Campbell BA, Bulmer MH, et al. (2001) The roughness of natural terrain: A planetary and remote sensing perspective. J Geophys Res Planets 106: 32777–32795. https://doi.org/10.1029/2000JE001429 doi: 10.1029/2000JE001429
![]() |
[21] |
Fan L, Atkinson PM (2019) An Iterative Coarse-to-Fine Sub-Sampling Method for Density Reduction of Terrain Point Clouds. Remote Sens 11: 947. https://doi.org/10.3390/rs11080947 doi: 10.3390/rs11080947
![]() |
[22] | LiDAR data access was based on[LiDAR, ground] services provided by the OpenTopography Facility. Lidar data acquisition completed by the National Centre for Airborne Laser Mapping. Available from: https://doi.org/10.5069/G9PR7SX0 |
[23] |
Fan L, Atkinson PM (2018) A new multi-resolution based method for estimating local surface roughness from point clouds. ISPRS J Photogramm Remote Sens 144,369–378. https://doi.org/10.1016/j.isprsjprs.2018.08.003 doi: 10.1016/j.isprsjprs.2018.08.003
![]() |
[24] |
Zevenbergen LW, Thorne CR (1987) Quantitative analysis of land surface topography. Earth Surf Processes Landforms 12: 47–56. https://doi.org/10.1002/esp.3290120107 doi: 10.1002/esp.3290120107
![]() |
[25] |
Moore ID, Grayson RB, Ladson AR (1991) Digital terrain modelling: A review of hydrological, geomorphological, and biological applications. Hydrol Processes 5: 3–30. https://doi.org/10.1002/hyp.3360050103 doi: 10.1002/hyp.3360050103
![]() |
[26] |
Lindsay JB, Newman DR, Francioni A (2019) Scale-optimized surface roughness for topographic analysis. Geosciences 9: 322. https://doi.org/10.3390/geosciences9070322 doi: 10.3390/geosciences9070322
![]() |
[27] |
Fan L, Smethurst JA, Atkinson PM, et al. (2014) Propagation of vertical and horizontal source data errors into a TIN with linear interpolation. Int J Geogr Inf Sci 28: 1378–1400. https://doi.org/10.1080/13658816.2014.889299 doi: 10.1080/13658816.2014.889299
![]() |
[28] |
Pollyea RM, Fairley JP (2011) Estimating surface roughness of terrestrial laser scan data using orthogonal distance regression. Geology 39: 623–626. https://doi.org/10.1130/G32078.1 doi: 10.1130/G32078.1
![]() |
10 Iterations | 100 Iterations | |||||||||
Model Name | SD/Mean | E_{A}(f) | Time | #Fs | SD/Mean | E_{A}(f) | Time | #Fs | ||
Right Hand | 0.1950 | 2.17 \times 10^{-1} | 0.85 | 4 | 0.1459 | 1.28 \times 10^{-1} | 9.37 | 2 | ||
David Head | 0.0178 | 4.04 \times 10^{-3} | 1.66 | 0 | 0.0156 | 3.05 \times 10^{-3} | 14.95 | 0 | ||
Cortical Surface | 0.0214 | 4.68 \times 10^{-3} | 3.62 | 0 | 0.0200 | 4.15 \times 10^{-3} | 32.40 | 0 | ||
Bull | 0.1492 | 2.58 \times 10^{-1} | 4.99 | 4 | 0.1380 | 2.31 \times 10^{-1} | 43.27 | 1 | ||
Bulldog | 0.0369 | 1.41 \times 10^{-2} | 13.59 | 0 | 0.0343 | 1.27 \times 10^{-2} | 136.63 | 0 | ||
Lion Statue | 0.1935 | 4.77 \times 10^{-1} | 16.83 | 0 | 0.1922 | 4.69 \times 10^{-1} | 160.20 | 0 | ||
Gargoyle | 0.0690 | 5.28 \times 10^{-2} | 19.55 | 0 | 0.0653 | 4.84 \times 10^{-2} | 192.62 | 0 | ||
Max Planck | 0.0537 | 3.54 \times 10^{-2} | 16.52 | 0 | 0.0525 | 3.38 \times 10^{-2} | 161.01 | 0 | ||
Bunny | 0.0417 | 2.18 \times 10^{-2} | 20.59 | 0 | 0.0404 | 2.04 \times 10^{-2} | 226.99 | 0 | ||
Chess King | 0.0687 | 6.07 \times 10^{-2} | 52.36 | 21 | 0.0639 | 5.14 \times 10^{-2} | 518.18 | 17 | ||
Art Statuette | 0.0408 | 2.14 \times 10^{-2} | 140.59 | 0 | 0.0405 | 2.10 \times 10^{-2} | 1 111.67 | 0 | ||
Bimba Statue | 0.0514 | 3.31 \times 10^{-2} | 270.63 | 1 | 0.0511 | 3.29 \times 10^{-2} | 2 320.19 | 1 |
10 Iterations | 100 Iterations | |||||||||
Model Name | SD/Mean | E_{A}(f) | Time | #Fs | SD/Mean | E_{A}(f) | Time | #Fs | ||
Right Hand | 0.1936 | 2.16 \times 10^{-1} | 0.36 | 4 | 0.1204 | 9.40 \times 10^{-2} | 4.07 | 1 | ||
David Head | 0.0178 | 4.04 \times 10^{-3} | 0.99 | 0 | 0.0156 | 3.04 \times 10^{-3} | 9.16 | 0 | ||
Cortical Surface | 0.0216 | 4.75 \times 10^{-3} | 1.40 | 0 | 0.0200 | 3.72 \times 10^{-3} | 16.01 | 0 | ||
Bull | 0.1492 | 2.59 \times 10^{-1} | 1.77 | 4 | 0.1348 | 2.19 \times 10^{-1} | 18.89 | 1 | ||
Bulldog | 0.0369 | 1.41 \times 10^{-2} | 6.60 | 0 | 0.0343 | 1.27 \times 10^{-2} | 61.93 | 0 | ||
Lion Statue | 0.1935 | 4.77 \times 10^{-1} | 7.75 | 0 | 0.1894 | 4.54 \times 10^{-1} | 76.76 | 0 | ||
Gargoyle | 0.0688 | 5.26 \times 10^{-2} | 7.81 | 0 | 0.0646 | 4.76 \times 10^{-2} | 80.52 | 0 | ||
Max Planck | 0.0537 | 3.54 \times 10^{-2} | 7.18 | 0 | 0.0525 | 3.39 \times 10^{-2} | 75.60 | 0 | ||
Bunny | 0.0417 | 2.18 \times 10^{-2} | 8.30 | 0 | 0.0390 | 1.91 \times 10^{-2} | 89.62 | 0 | ||
Chess King | 0.0692 | 6.07 \times 10^{-2} | 20.06 | 21 | 0.0647 | 5.23 \times 10^{-2} | 207.47 | 17 | ||
Art Statuette | 0.0408 | 2.14 \times 10^{-2} | 57.71 | 0 | 0.0405 | 2.10 \times 10^{-2} | 654.57 | 0 | ||
Bimba Statue | 0.0514 | 3.31 \times 10^{-2} | 70.83 | 1 | 0.0512 | 3.29 \times 10^{-2} | 775.36 | 1 |
Before bijectivity correction | After bijectivity correction | ||||||||||
Model Name | SD/Mean | E_{A}\big(f\big) | #Fs | SD/Mean | E_{A}\big(f\big) | #Fs | Time | #Its | |||
Right Hand | 0.2063 | 8.01 \times 10^{-2} | 3 | 0.1071 | 8.12 \times 10^{-2} | 0 | 6.67 | 127 | |||
David Head | 0.0123 | 1.87 \times 10^{-3} | 0 | --------------------- | 83.66 | 432 | |||||
Cortical Surface | 0.0187 | 4.23 \times 10^{-3} | 0 | --------------------- | 9.22 | 52 | |||||
Bull | 0.1520 | 2.37 \times 10^{-1} | 11 | 0.1403 | 2.40 \times 10^{-1} | 3 | 12.20 | 50 | |||
Bulldog | 0.0351 | 1.31 \times 10^{-2} | 0 | --------------------- | 72.64 | 60 | |||||
Lion Statue | 0.1940 | 4.79 \times 10^{-1} | 2 | 0.1938 | 4.79 \times 10^{-1} | 0 | 2.63 | 2 | |||
Gargoyle | 0.0704 | 5.17 \times 10^{-2} | 3 | 0.0682 | 5.14 \times 10^{-2} | 0 | 26.71 | 17 | |||
Max Planck | 0.0464 | 3.54 \times 10^{-2} | 0 | --------------------- | 10.58 | 8 | |||||
Bunny | 0.0417 | 2.17 \times 10^{-2} | 0 | --------------------- | 20.77 | 13 | |||||
Chess King | 0.0715 | 5.17 \times 10^{-2} | 43 | 0.0641 | 5.38 \times 10^{-2} | 17 | 370.68 | 107 | |||
Art Statuette | 0.0409 | 2.14 \times 10^{-2} | 0 | --------------------- | 31.53 | 3 | |||||
Bimba Statue | 0.0515 | 3.32 \times 10^{-2} | 2 | 0.0514 | 3.32 \times 10^{-2} | 0 | 19.24 | 2 |
100 Iterations | 10 000 Iterations | |||||||||
Model Name | SD/Mean | E_{A}(f) | Time | #Fs | SD/Mean | E_{A}(f) | Time | #Fs | ||
Right Hand | 0.1204 | 9.40 \times 10^{-2} | 4.07 | 1 | 0.0545 | 2.07 \times 10^{-2} | 431.28 | 0 | ||
David Head | 0.0156 | 3.04 \times 10^{-3} | 9.16 | 0 | 0.0029 | 1.01 \times 10^{-4} | 1 018.87 | 0 | ||
Cortical Surface | 0.0200 | 3.72 \times 10^{-3} | 16.01 | 0 | 0.0045 | 2.06 \times 10^{-4} | 1 328.56 | 0 |
Smallest eigenvalue of the Hessian | ||
Model Name | After FPI | After RGD |
Right Hand | -2.4429 \times 10^{0} | -1.9013 \times 10^{-1} |
David Head | -1.8481 \times 10^{0} | -2.8445 \times 10^{-6} |
Cortical Surface | -4.4940 \times 10^{-1} | -3.6768 \times 10^{-3} |
Energy E_{A} Increased | 100 Iterations | ||||||||||
Model Name | SD/Mean | E_{A}(f) | Time | #Fs | #Its | SD/Mean | E_{A}(f) | Time | #Fs | ||
Right Hand | 0.2050 | 2.86 \times 10^{-1} | 0.08 | 12 | 6 | 0.4598 | 2.92 \times 10^{0} | 1.35 | 67 | ||
David Head | 0.0191 | 4.66 \times 10^{-3} | 0.35 | 0 | 8 | 0.0169 | 3.58 \times 10^{-3} | 4.30 | 0 | ||
Cortical Surface | 0.0220 | 4.93 \times 10^{-3} | 0.85 | 0 | 15 | 0.0174 | 3.21 \times 10^{-3} | 5.62 | 0 | ||
Bull | 0.1504 | 2.74 \times 10^{-1} | 1.29 | 8 | 18 | 0.1876 | 4.59 \times 10^{-1} | 6.90 | 40 | ||
Bulldog | 0.0381 | 1.49 \times 10^{-2} | 2.61 | 0 | 10 | 0.1833 | 3.99 \times 10^{-1} | 22.22 | 53 | ||
Lion Statue | 0.1940 | 5.10 \times 10^{-1} | 1.12 | 1 | 4 | 0.2064 | 5.28 \times 10^{-1} | 23.67 | 38 | ||
Gargoyle | 0.0704 | 5.47 \times 10^{-2} | 2.64 | 0 | 11 | 4.1020 | 4.85 \times 10^{2} | 36.10 | 1955 | ||
Max Planck | 0.0544 | 3.67 \times 10^{-2} | 1.35 | 0 | 5 | 0.1844 | 1.67 \times 10^{1} | 25.99 | 144 | ||
Bunny | 0.0423 | 2.24 \times 10^{-2} | 6.40 | 0 | 20 | 0.0394 | 3.96 \times 10^{-2} | 35.78 | 2 | ||
Chess King | 0.0713 | 6.91 \times 10^{-2} | 6.35 | 9 | 8 | 1.0903 | 1.79 \times 10^{1} | 88.04 | 1655 | ||
Art Statuette | 0.0411 | 2.15 \times 10^{-2} | 23.27 | 0 | 7 | 0.0908 | 1.07 \times 10^{-1} | 342.95 | 126 | ||
Bimba Statue | 0.0515 | 3.32 \times 10^{-2} | 29.94 | 6 | 9 | 0.0932 | 7.42 \times 10^{-2} | 305.00 | 144 |
Model Name | SD/Mean | E_{A}(f) | Time | #Foldings |
Right Hand | 18.3283 | 4.84 \times 10^{3} | 218.03 | 672 |
David Head | 0.0426 | 2.27 \times 10^{-2} | 298.76 | 0 |
Cortical Surface | 0.6320 | 1.14 \times 10^{0} | 420.20 | 10 |
Bull | 8.5565 | 1.82 \times 10^{3} | 34.42 | 335 |
Bulldog | 9.2379 | 1.22 \times 10^{3} | 183.94 | 338 |
Lion Statue | 0.2626 | 8.96 \times 10^{-1} | 1498.91 | 540 |
Gargoyle | 0.3558 | 1.30 \times 10^{0} | 1483.35 | 571 |
Max Planck | 11.6875 | 1.49 \times 10^{3} | 195.39 | 575 |
Bunny | 27.6014 | 8.94 \times 10^{3} | 157.87 | 208 |
Chess King | 11.8300 | 1.65 \times 10^{3} | 608.55 | 948 |
Art Statuette | 394.4414 | 9.93 \times 10^{0} | 2284.79 | 2242 |
Bimba Statue | 0.5110 | 2.01 \times 10^{0} | 16 773.34 | 11 821 |
Model Name | SD/Mean | E_{A}(f) | #Foldings | # Iterations |
David Head | 0.4189 | 2.25 \times 10^{0} | 0 | 27 |
Cortical Surface | 0.5113 | 3.11 \times 10^{0} | 0 | 27 |
Bulldog | 0.8665 | 1.00 \times 10^{1} | 0 | 33 |
Max Planck | 0.5619 | 4.38 \times 10^{0} | 0 | 25 |
\sigma_{\mathrm{noise}} = 1 \times 10^{-3} | |||||||||
Model Name | SD/Mean | E_{A}\big(\tilde{f}\big) | Time | #Fs | #Fs after b.c. | E_{A}(f) | err- E_{A}\big(f, \tilde{f}\big) | ||
Right Hand | 0.1254 | 1.03 \times 10^{-1} | 3.13 | 14 | 1 | 9.40 \times 10^{-2} | 5.84 \times 10^{-2} | ||
David Head | 0.0108 | 1.44 \times 10^{-3} | 9.98 | 0 | 0 | 3.04 \times 10^{-3} | 4.47 \times 10^{-3} | ||
Cortical Surface | 0.0187 | 3.82 \times 10^{-3} | 12.78 | 0 | 0 | 3.72 \times 10^{-3} | 3.45 \times 10^{-3} | ||
Bull | 0.2528 | 9.23 \times 10^{-1} | 16.74 | 19 | 6 | 2.19 \times 10^{-1} | 1.12 \times 10^{0} | ||
Bulldog | 0.0273 | 6.94 \times 10^{-3} | 59.26 | 0 | 0 | 1.27 \times 10^{-2} | 7.19 \times 10^{-6} | ||
Lion Statue | 0.1630 | 3.27 \times 10^{-1} | 66.78 | 1 | 0 | 4.54 \times 10^{-1} | 1.36 \times 10^{-4} | ||
Gargoyle | 0.1677 | 3.66 \times 10^{-1} | 65.72 | 0 | 0 | 4.76 \times 10^{-2} | 4.45 \times 10^{-3} | ||
Max Planck | 0.0464 | 2.67 \times 10^{-2} | 64.30 | 0 | 0 | 3.39 \times 10^{-2} | 5.27 \times 10^{-5} | ||
Bunny | 0.0297 | 1.11 \times 10^{-2} | 81.31 | 0 | 0 | 1.91 \times 10^{-2} | 5.32 \times 10^{-3} | ||
Chess King | 0.0747 | 6.08 \times 10^{-2} | 193.04 | 119 | 38 | 5.23 \times 10^{-2} | 1.03 \times 10^{-3} | ||
Art Statuette | 0.0235 | 6.88 \times 10^{-3} | 636.30 | 0 | 0 | 2.10 \times 10^{-2} | 2.54 \times 10^{-2} | ||
Bimba Statue | 0.0541 | 3.33 \times 10^{-2} | 759.36 | 2 | 0 | 3.29 \times 10^{-2} | 5.81 \times 10^{-4} |
\sigma_{\mathrm{noise}} = 5 \times 10^{-3} | |||||||||
Model Name | SD/Mean | E_{A}\big(\tilde{f}\big) | Time | #Fs | #Fs after b.c. | E_{A}(f) | err- E_{A}\big(f, \tilde{f}\big) | ||
Right Hand | 0.2304 | 5.77 \times 10^{-1} | 3.12 | 10 | 4 | 9.40 \times 10^{-2} | 1.45 \times 10^{0} | ||
David Head | 0.0211 | 5.51 \times 10^{-3} | 9.43 | 0 | 0 | 3.04 \times 10^{-3} | 6.84 \times 10^{-3} | ||
Cortical Surface | 0.0301 | 1.02 \times 10^{-2} | 12.72 | 0 | 0 | 3.72 \times 10^{-3} | 2.28 \times 10^{-1} | ||
Bull | 0.1723 | 2.98 \times 10^{-1} | 17.40 | 18 | 4 | 2.19 \times 10^{-1} | 1.19 \times 10^{-1} | ||
Bulldog | 0.0629 | 3.86 \times 10^{-2} | 61.75 | 2 | 0 | 1.27 \times 10^{-2} | 3.23 \times 10^{-5} | ||
Lion Statue | 0.1044 | 1.36 \times 10^{-1} | 69.55 | 0 | 0 | 4.54 \times 10^{-1} | 3.36 \times 10^{-4} | ||
Gargoyle | 0.0974 | 9.68 \times 10^{-2} | 69.32 | 1 | 0 | 4.76 \times 10^{-2} | 6.87 \times 10^{-4} | ||
Max Planck | 0.0782 | 7.11 \times 10^{-2} | 67.30 | 0 | 0 | 3.39 \times 10^{-2} | 2.75 \times 10^{-4} | ||
Bunny | 0.0455 | 2.57 \times 10^{-2} | 80.93 | 0 | 0 | 1.91 \times 10^{-2} | 4.40 \times 10^{-3} | ||
Chess King | 0.1521 | 2.72 \times 10^{-1} | 187.57 | 95 | 35 | 5.23 \times 10^{-2} | 2.64 \times 10^{-2} | ||
Art Statuette | 0.0981 | 5.41 \times 10^{-2} | 617.75 | 0 | 0 | 2.10 \times 10^{-2} | 5.92 \times 10^{-2} | ||
Bimba Statue | 0.1083 | 1.25 \times 10^{-1} | 743.00 | 76 | 0 | 3.29 \times 10^{-2} | 1.68 \times 10^{-1} |
10 Iterations | 100 Iterations | |||||||||
Model Name | SD/Mean | E_{A}(f) | Time | #Fs | SD/Mean | E_{A}(f) | Time | #Fs | ||
Right Hand | 0.1950 | 2.17 \times 10^{-1} | 0.85 | 4 | 0.1459 | 1.28 \times 10^{-1} | 9.37 | 2 | ||
David Head | 0.0178 | 4.04 \times 10^{-3} | 1.66 | 0 | 0.0156 | 3.05 \times 10^{-3} | 14.95 | 0 | ||
Cortical Surface | 0.0214 | 4.68 \times 10^{-3} | 3.62 | 0 | 0.0200 | 4.15 \times 10^{-3} | 32.40 | 0 | ||
Bull | 0.1492 | 2.58 \times 10^{-1} | 4.99 | 4 | 0.1380 | 2.31 \times 10^{-1} | 43.27 | 1 | ||
Bulldog | 0.0369 | 1.41 \times 10^{-2} | 13.59 | 0 | 0.0343 | 1.27 \times 10^{-2} | 136.63 | 0 | ||
Lion Statue | 0.1935 | 4.77 \times 10^{-1} | 16.83 | 0 | 0.1922 | 4.69 \times 10^{-1} | 160.20 | 0 | ||
Gargoyle | 0.0690 | 5.28 \times 10^{-2} | 19.55 | 0 | 0.0653 | 4.84 \times 10^{-2} | 192.62 | 0 | ||
Max Planck | 0.0537 | 3.54 \times 10^{-2} | 16.52 | 0 | 0.0525 | 3.38 \times 10^{-2} | 161.01 | 0 | ||
Bunny | 0.0417 | 2.18 \times 10^{-2} | 20.59 | 0 | 0.0404 | 2.04 \times 10^{-2} | 226.99 | 0 | ||
Chess King | 0.0687 | 6.07 \times 10^{-2} | 52.36 | 21 | 0.0639 | 5.14 \times 10^{-2} | 518.18 | 17 | ||
Art Statuette | 0.0408 | 2.14 \times 10^{-2} | 140.59 | 0 | 0.0405 | 2.10 \times 10^{-2} | 1 111.67 | 0 | ||
Bimba Statue | 0.0514 | 3.31 \times 10^{-2} | 270.63 | 1 | 0.0511 | 3.29 \times 10^{-2} | 2 320.19 | 1 |
10 Iterations | 100 Iterations | |||||||||
Model Name | SD/Mean | E_{A}(f) | Time | #Fs | SD/Mean | E_{A}(f) | Time | #Fs | ||
Right Hand | 0.1936 | 2.16 \times 10^{-1} | 0.36 | 4 | 0.1204 | 9.40 \times 10^{-2} | 4.07 | 1 | ||
David Head | 0.0178 | 4.04 \times 10^{-3} | 0.99 | 0 | 0.0156 | 3.04 \times 10^{-3} | 9.16 | 0 | ||
Cortical Surface | 0.0216 | 4.75 \times 10^{-3} | 1.40 | 0 | 0.0200 | 3.72 \times 10^{-3} | 16.01 | 0 | ||
Bull | 0.1492 | 2.59 \times 10^{-1} | 1.77 | 4 | 0.1348 | 2.19 \times 10^{-1} | 18.89 | 1 | ||
Bulldog | 0.0369 | 1.41 \times 10^{-2} | 6.60 | 0 | 0.0343 | 1.27 \times 10^{-2} | 61.93 | 0 | ||
Lion Statue | 0.1935 | 4.77 \times 10^{-1} | 7.75 | 0 | 0.1894 | 4.54 \times 10^{-1} | 76.76 | 0 | ||
Gargoyle | 0.0688 | 5.26 \times 10^{-2} | 7.81 | 0 | 0.0646 | 4.76 \times 10^{-2} | 80.52 | 0 | ||
Max Planck | 0.0537 | 3.54 \times 10^{-2} | 7.18 | 0 | 0.0525 | 3.39 \times 10^{-2} | 75.60 | 0 | ||
Bunny | 0.0417 | 2.18 \times 10^{-2} | 8.30 | 0 | 0.0390 | 1.91 \times 10^{-2} | 89.62 | 0 | ||
Chess King | 0.0692 | 6.07 \times 10^{-2} | 20.06 | 21 | 0.0647 | 5.23 \times 10^{-2} | 207.47 | 17 | ||
Art Statuette | 0.0408 | 2.14 \times 10^{-2} | 57.71 | 0 | 0.0405 | 2.10 \times 10^{-2} | 654.57 | 0 | ||
Bimba Statue | 0.0514 | 3.31 \times 10^{-2} | 70.83 | 1 | 0.0512 | 3.29 \times 10^{-2} | 775.36 | 1 |
Before bijectivity correction | After bijectivity correction | ||||||||||
Model Name | SD/Mean | E_{A}\big(f\big) | #Fs | SD/Mean | E_{A}\big(f\big) | #Fs | Time | #Its | |||
Right Hand | 0.2063 | 8.01 \times 10^{-2} | 3 | 0.1071 | 8.12 \times 10^{-2} | 0 | 6.67 | 127 | |||
David Head | 0.0123 | 1.87 \times 10^{-3} | 0 | --------------------- | 83.66 | 432 | |||||
Cortical Surface | 0.0187 | 4.23 \times 10^{-3} | 0 | --------------------- | 9.22 | 52 | |||||
Bull | 0.1520 | 2.37 \times 10^{-1} | 11 | 0.1403 | 2.40 \times 10^{-1} | 3 | 12.20 | 50 | |||
Bulldog | 0.0351 | 1.31 \times 10^{-2} | 0 | --------------------- | 72.64 | 60 | |||||
Lion Statue | 0.1940 | 4.79 \times 10^{-1} | 2 | 0.1938 | 4.79 \times 10^{-1} | 0 | 2.63 | 2 | |||
Gargoyle | 0.0704 | 5.17 \times 10^{-2} | 3 | 0.0682 | 5.14 \times 10^{-2} | 0 | 26.71 | 17 | |||
Max Planck | 0.0464 | 3.54 \times 10^{-2} | 0 | --------------------- | 10.58 | 8 | |||||
Bunny | 0.0417 | 2.17 \times 10^{-2} | 0 | --------------------- | 20.77 | 13 | |||||
Chess King | 0.0715 | 5.17 \times 10^{-2} | 43 | 0.0641 | 5.38 \times 10^{-2} | 17 | 370.68 | 107 | |||
Art Statuette | 0.0409 | 2.14 \times 10^{-2} | 0 | --------------------- | 31.53 | 3 | |||||
Bimba Statue | 0.0515 | 3.32 \times 10^{-2} | 2 | 0.0514 | 3.32 \times 10^{-2} | 0 | 19.24 | 2 |
100 Iterations | 10 000 Iterations | |||||||||
Model Name | SD/Mean | E_{A}(f) | Time | #Fs | SD/Mean | E_{A}(f) | Time | #Fs | ||
Right Hand | 0.1204 | 9.40 \times 10^{-2} | 4.07 | 1 | 0.0545 | 2.07 \times 10^{-2} | 431.28 | 0 | ||
David Head | 0.0156 | 3.04 \times 10^{-3} | 9.16 | 0 | 0.0029 | 1.01 \times 10^{-4} | 1 018.87 | 0 | ||
Cortical Surface | 0.0200 | 3.72 \times 10^{-3} | 16.01 | 0 | 0.0045 | 2.06 \times 10^{-4} | 1 328.56 | 0 |
Smallest eigenvalue of the Hessian | ||
Model Name | After FPI | After RGD |
Right Hand | -2.4429 \times 10^{0} | -1.9013 \times 10^{-1} |
David Head | -1.8481 \times 10^{0} | -2.8445 \times 10^{-6} |
Cortical Surface | -4.4940 \times 10^{-1} | -3.6768 \times 10^{-3} |
Energy E_{A} Increased | 100 Iterations | ||||||||||
Model Name | SD/Mean | E_{A}(f) | Time | #Fs | #Its | SD/Mean | E_{A}(f) | Time | #Fs | ||
Right Hand | 0.2050 | 2.86 \times 10^{-1} | 0.08 | 12 | 6 | 0.4598 | 2.92 \times 10^{0} | 1.35 | 67 | ||
David Head | 0.0191 | 4.66 \times 10^{-3} | 0.35 | 0 | 8 | 0.0169 | 3.58 \times 10^{-3} | 4.30 | 0 | ||
Cortical Surface | 0.0220 | 4.93 \times 10^{-3} | 0.85 | 0 | 15 | 0.0174 | 3.21 \times 10^{-3} | 5.62 | 0 | ||
Bull | 0.1504 | 2.74 \times 10^{-1} | 1.29 | 8 | 18 | 0.1876 | 4.59 \times 10^{-1} | 6.90 | 40 | ||
Bulldog | 0.0381 | 1.49 \times 10^{-2} | 2.61 | 0 | 10 | 0.1833 | 3.99 \times 10^{-1} | 22.22 | 53 | ||
Lion Statue | 0.1940 | 5.10 \times 10^{-1} | 1.12 | 1 | 4 | 0.2064 | 5.28 \times 10^{-1} | 23.67 | 38 | ||
Gargoyle | 0.0704 | 5.47 \times 10^{-2} | 2.64 | 0 | 11 | 4.1020 | 4.85 \times 10^{2} | 36.10 | 1955 | ||
Max Planck | 0.0544 | 3.67 \times 10^{-2} | 1.35 | 0 | 5 | 0.1844 | 1.67 \times 10^{1} | 25.99 | 144 | ||
Bunny | 0.0423 | 2.24 \times 10^{-2} | 6.40 | 0 | 20 | 0.0394 | 3.96 \times 10^{-2} | 35.78 | 2 | ||
Chess King | 0.0713 | 6.91 \times 10^{-2} | 6.35 | 9 | 8 | 1.0903 | 1.79 \times 10^{1} | 88.04 | 1655 | ||
Art Statuette | 0.0411 | 2.15 \times 10^{-2} | 23.27 | 0 | 7 | 0.0908 | 1.07 \times 10^{-1} | 342.95 | 126 | ||
Bimba Statue | 0.0515 | 3.32 \times 10^{-2} | 29.94 | 6 | 9 | 0.0932 | 7.42 \times 10^{-2} | 305.00 | 144 |
Model Name | SD/Mean | E_{A}(f) | Time | #Foldings |
Right Hand | 18.3283 | 4.84 \times 10^{3} | 218.03 | 672 |
David Head | 0.0426 | 2.27 \times 10^{-2} | 298.76 | 0 |
Cortical Surface | 0.6320 | 1.14 \times 10^{0} | 420.20 | 10 |
Bull | 8.5565 | 1.82 \times 10^{3} | 34.42 | 335 |
Bulldog | 9.2379 | 1.22 \times 10^{3} | 183.94 | 338 |
Lion Statue | 0.2626 | 8.96 \times 10^{-1} | 1498.91 | 540 |
Gargoyle | 0.3558 | 1.30 \times 10^{0} | 1483.35 | 571 |
Max Planck | 11.6875 | 1.49 \times 10^{3} | 195.39 | 575 |
Bunny | 27.6014 | 8.94 \times 10^{3} | 157.87 | 208 |
Chess King | 11.8300 | 1.65 \times 10^{3} | 608.55 | 948 |
Art Statuette | 394.4414 | 9.93 \times 10^{0} | 2284.79 | 2242 |
Bimba Statue | 0.5110 | 2.01 \times 10^{0} | 16 773.34 | 11 821 |
Model Name | SD/Mean | E_{A}(f) | #Foldings | # Iterations |
David Head | 0.4189 | 2.25 \times 10^{0} | 0 | 27 |
Cortical Surface | 0.5113 | 3.11 \times 10^{0} | 0 | 27 |
Bulldog | 0.8665 | 1.00 \times 10^{1} | 0 | 33 |
Max Planck | 0.5619 | 4.38 \times 10^{0} | 0 | 25 |
\sigma_{\mathrm{noise}} = 1 \times 10^{-3} | |||||||||
Model Name | SD/Mean | E_{A}\big(\tilde{f}\big) | Time | #Fs | #Fs after b.c. | E_{A}(f) | err- E_{A}\big(f, \tilde{f}\big) | ||
Right Hand | 0.1254 | 1.03 \times 10^{-1} | 3.13 | 14 | 1 | 9.40 \times 10^{-2} | 5.84 \times 10^{-2} | ||
David Head | 0.0108 | 1.44 \times 10^{-3} | 9.98 | 0 | 0 | 3.04 \times 10^{-3} | 4.47 \times 10^{-3} | ||
Cortical Surface | 0.0187 | 3.82 \times 10^{-3} | 12.78 | 0 | 0 | 3.72 \times 10^{-3} | 3.45 \times 10^{-3} | ||
Bull | 0.2528 | 9.23 \times 10^{-1} | 16.74 | 19 | 6 | 2.19 \times 10^{-1} | 1.12 \times 10^{0} | ||
Bulldog | 0.0273 | 6.94 \times 10^{-3} | 59.26 | 0 | 0 | 1.27 \times 10^{-2} | 7.19 \times 10^{-6} | ||
Lion Statue | 0.1630 | 3.27 \times 10^{-1} | 66.78 | 1 | 0 | 4.54 \times 10^{-1} | 1.36 \times 10^{-4} | ||
Gargoyle | 0.1677 | 3.66 \times 10^{-1} | 65.72 | 0 | 0 | 4.76 \times 10^{-2} | 4.45 \times 10^{-3} | ||
Max Planck | 0.0464 | 2.67 \times 10^{-2} | 64.30 | 0 | 0 | 3.39 \times 10^{-2} | 5.27 \times 10^{-5} | ||
Bunny | 0.0297 | 1.11 \times 10^{-2} | 81.31 | 0 | 0 | 1.91 \times 10^{-2} | 5.32 \times 10^{-3} | ||
Chess King | 0.0747 | 6.08 \times 10^{-2} | 193.04 | 119 | 38 | 5.23 \times 10^{-2} | 1.03 \times 10^{-3} | ||
Art Statuette | 0.0235 | 6.88 \times 10^{-3} | 636.30 | 0 | 0 | 2.10 \times 10^{-2} | 2.54 \times 10^{-2} | ||
Bimba Statue | 0.0541 | 3.33 \times 10^{-2} | 759.36 | 2 | 0 | 3.29 \times 10^{-2} | 5.81 \times 10^{-4} |
\sigma_{\mathrm{noise}} = 5 \times 10^{-3} | |||||||||
Model Name | SD/Mean | E_{A}\big(\tilde{f}\big) | Time | #Fs | #Fs after b.c. | E_{A}(f) | err- E_{A}\big(f, \tilde{f}\big) | ||
Right Hand | 0.2304 | 5.77 \times 10^{-1} | 3.12 | 10 | 4 | 9.40 \times 10^{-2} | 1.45 \times 10^{0} | ||
David Head | 0.0211 | 5.51 \times 10^{-3} | 9.43 | 0 | 0 | 3.04 \times 10^{-3} | 6.84 \times 10^{-3} | ||
Cortical Surface | 0.0301 | 1.02 \times 10^{-2} | 12.72 | 0 | 0 | 3.72 \times 10^{-3} | 2.28 \times 10^{-1} | ||
Bull | 0.1723 | 2.98 \times 10^{-1} | 17.40 | 18 | 4 | 2.19 \times 10^{-1} | 1.19 \times 10^{-1} | ||
Bulldog | 0.0629 | 3.86 \times 10^{-2} | 61.75 | 2 | 0 | 1.27 \times 10^{-2} | 3.23 \times 10^{-5} | ||
Lion Statue | 0.1044 | 1.36 \times 10^{-1} | 69.55 | 0 | 0 | 4.54 \times 10^{-1} | 3.36 \times 10^{-4} | ||
Gargoyle | 0.0974 | 9.68 \times 10^{-2} | 69.32 | 1 | 0 | 4.76 \times 10^{-2} | 6.87 \times 10^{-4} | ||
Max Planck | 0.0782 | 7.11 \times 10^{-2} | 67.30 | 0 | 0 | 3.39 \times 10^{-2} | 2.75 \times 10^{-4} | ||
Bunny | 0.0455 | 2.57 \times 10^{-2} | 80.93 | 0 | 0 | 1.91 \times 10^{-2} | 4.40 \times 10^{-3} | ||
Chess King | 0.1521 | 2.72 \times 10^{-1} | 187.57 | 95 | 35 | 5.23 \times 10^{-2} | 2.64 \times 10^{-2} | ||
Art Statuette | 0.0981 | 5.41 \times 10^{-2} | 617.75 | 0 | 0 | 2.10 \times 10^{-2} | 5.92 \times 10^{-2} | ||
Bimba Statue | 0.1083 | 1.25 \times 10^{-1} | 743.00 | 76 | 0 | 3.29 \times 10^{-2} | 1.68 \times 10^{-1} |