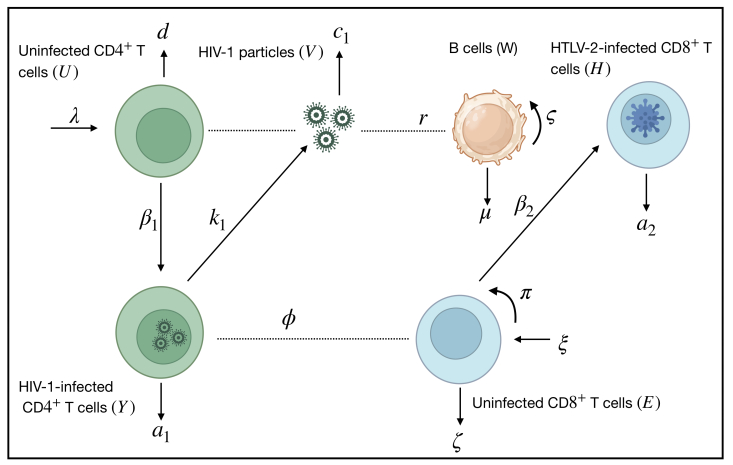
Human T-lymphotropic virus type 2 (HTLV-2) and human immunodeficiency virus type 1 (HIV-1) are two infectious retroviruses that infect immune cells, CD8+ T cells and CD4+ T cells, respectively. Multiple studies have revealed co-infected patients with HTLV-2 and HIV-1. In this paper, we formulated a new mathematical model for the co-infection of HTLV-2 and HIV-1 in vivo. The HIV-1-specific B-cell response is included. Six ordinary differential equations made up the model, which depicted the interactions between uninfected CD4+ T cells, HIV-1-infected CD4+ T cells, HIV-1 particles, uninfected CD8+ T cells, HTLV-2-infected CD8+ T cells, and HIV-1-specific B cells. We carried out a thorough study of the model, demonstrating the boundedness and nonnegativity of the solutions. Additionally, we determined the equilibrium points and demonstrated, under specific conditions, their global stability. The global asymptotic stability of all equilibria was established by constructing appropriate Lyapunov functions and applying the Lyapunov-LaSalle asymptotic stability theorem. We provide numerical simulations to corroborate the theoretical findings. We investigated how the B-cell response affects the dynamics of HIV-1 and HTLV-2 co-infection. The results suggested that the B-cell response regulates and inhibits the spread of HIV-1. We present a comparison between HTLV-2 or HIV-1 mono-infections and co-infections with HTLV-2 and HIV-1. Our findings support earlier research, suggesting that co-infection with HTLV-2 may be able to maintain the behavior dynamics of the CD4+ T cells, inhibit HIV-1 replication, and postpone the onset of AIDS. However, co-infected patients with HTLV-2 and HIV-1 may experience a greater occurrence of HTLV-2-related T-cell malignant diseases.
Citation: A. M. Elaiw, E. A. Almohaimeed, A. D. Hobiny. Modeling the co-infection of HTLV-2 and HIV-1 in vivo[J]. Electronic Research Archive, 2024, 32(11): 6032-6071. doi: 10.3934/era.2024280
[1] | Danqi Feng, Yu Chen, Quanbao Ji . Contribution of a Ca2+-activated K+ channel to neuronal bursting activities in the Chay model. Electronic Research Archive, 2023, 31(12): 7544-7555. doi: 10.3934/era.2023380 |
[2] | Ziang Chen, Chunguang Dai, Lei Shi, Gaofang Chen, Peng Wu, Liping Wang . Reaction-diffusion model of HIV infection of two target cells under optimal control strategy. Electronic Research Archive, 2024, 32(6): 4129-4163. doi: 10.3934/era.2024186 |
[3] | Yapeng Zhang, Yu Chen, Quanbao Ji . Dynamical analysis of spontaneous Ca2+ oscillations in astrocytes. Electronic Research Archive, 2024, 32(1): 405-417. doi: 10.3934/era.2024020 |
[4] | Ting-Ying Chang, Yihong Du . Long-time dynamics of an epidemic model with nonlocal diffusion and free boundaries. Electronic Research Archive, 2022, 30(1): 289-313. doi: 10.3934/era.2022016 |
[5] | Qin Ye . Space-time decay rate of high-order spatial derivative of solution for 3D compressible Euler equations with damping. Electronic Research Archive, 2023, 31(7): 3879-3894. doi: 10.3934/era.2023197 |
[6] | Yan Geng, Jinhu Xu . Modelling and analysis of a delayed viral infection model with follicular dendritic cell. Electronic Research Archive, 2024, 32(8): 5127-5138. doi: 10.3934/era.2024236 |
[7] | Kingshook Biswas, Rudra P. Sarkar . Dynamics of $ L^p $ multipliers on harmonic manifolds. Electronic Research Archive, 2022, 30(8): 3042-3057. doi: 10.3934/era.2022154 |
[8] | Chengchun Hao, Tao Luo . Some results on free boundary problems of incompressible ideal magnetohydrodynamics equations. Electronic Research Archive, 2022, 30(2): 404-424. doi: 10.3934/era.2022021 |
[9] | Fanqi Zeng . Some almost-Schur type inequalities and applications on sub-static manifolds. Electronic Research Archive, 2022, 30(8): 2860-2870. doi: 10.3934/era.2022145 |
[10] | Abdelmajid El Hakoume, Lekbir Afraites, Amine Laghrib . An improved coupled PDE system applied to the inverse image denoising problem. Electronic Research Archive, 2022, 30(7): 2618-2642. doi: 10.3934/era.2022134 |
Human T-lymphotropic virus type 2 (HTLV-2) and human immunodeficiency virus type 1 (HIV-1) are two infectious retroviruses that infect immune cells, CD8+ T cells and CD4+ T cells, respectively. Multiple studies have revealed co-infected patients with HTLV-2 and HIV-1. In this paper, we formulated a new mathematical model for the co-infection of HTLV-2 and HIV-1 in vivo. The HIV-1-specific B-cell response is included. Six ordinary differential equations made up the model, which depicted the interactions between uninfected CD4+ T cells, HIV-1-infected CD4+ T cells, HIV-1 particles, uninfected CD8+ T cells, HTLV-2-infected CD8+ T cells, and HIV-1-specific B cells. We carried out a thorough study of the model, demonstrating the boundedness and nonnegativity of the solutions. Additionally, we determined the equilibrium points and demonstrated, under specific conditions, their global stability. The global asymptotic stability of all equilibria was established by constructing appropriate Lyapunov functions and applying the Lyapunov-LaSalle asymptotic stability theorem. We provide numerical simulations to corroborate the theoretical findings. We investigated how the B-cell response affects the dynamics of HIV-1 and HTLV-2 co-infection. The results suggested that the B-cell response regulates and inhibits the spread of HIV-1. We present a comparison between HTLV-2 or HIV-1 mono-infections and co-infections with HTLV-2 and HIV-1. Our findings support earlier research, suggesting that co-infection with HTLV-2 may be able to maintain the behavior dynamics of the CD4+ T cells, inhibit HIV-1 replication, and postpone the onset of AIDS. However, co-infected patients with HTLV-2 and HIV-1 may experience a greater occurrence of HTLV-2-related T-cell malignant diseases.
Persistent viral infections, such those brought on by two different families of retroviruses human immunodeficiency viruses (HIVs) and the human T-lymphotropic viruses (HTLVs), are one of the largest clinical problems. In addition to sharing an in vivo preference for immune system cells, particularly T lymphocytes, the viruses are known to transmit along both vertical and horizontal pathways [1]. UNAIDS 2024 reports that in 2023, there were 630,000 HIV-related deaths, 1.3 million new HIV infections, and 39.9 million HIV-positive people globally [2]. HIV comes in two varieties: HIV-1 and HIV-2 [3]. HIV-2 has a slower rate of development and spread than HIV-1, despite the fact that both impair immunity by infecting and destroying the cental component of the adaptive immune response, CD4+ T cells. Certain drugs that are used to treat HIV-1 do not work on HIV-2. Acquired immune deficiency syndrome (AIDS) can result from either HIV-1 or HIV-2 [3]. In a healthy person, the expected number of CD4+ T cells is 1000 cells/mm3. Following HIV-1 infection, there is a reduction in CD4+ T cells that can last for years. An individual is considered to have acquired immunodeficiency syndrome (AIDS) when the count of these cells falls below 200 cells/mm3 [4].
Of the four HTLV types, only two—HTLV-1 and HTLV-2—have been connected to diseases [5]. Both HTLV-1 and HTLV-2 are closely related retroviruses that share shared mechanisms of transmission and comparable biological characteristics [6]. In 2012, there were an estimated 5 million to 10 million individuals worldwide who were infected with HTLV-1 [7]. Murphy et al. [8] estimated that the number of known cases of HTLV-2 infection is thought to be between 670,000 and 890,000 in 2015, a far smaller number than there are for HTLV-1. HTLV-1 mainly targets the CD4+ T cells and can cause two diseases, adult T-cell leukemia (ATL) and HTLV-1-associated myelopathy/tropical spastic paraparesis (HAM/TSP) [9]. In contrast, HTLV-2 mainly targets CD8+ T cells, also called cytotoxic T-lymphocytes (CTLs), which eliminate the cells that are infected with the virus [9]. HTLV-2 has been linked to peripheral neuropathy and may also be connected to tropical spastic paraparesis [9]. HTLV-1 and HTLV-2 depend on direct cell-to-cell contact for efficient transmission. Both viruses use the Envelope (Env) glycoproteins to facilitate cell attachment and entry into the host cells, enabling them to establish infection effectively. This mechanism plays a critical role in their persistence and ability to spread within the host [10]. Martinez et al. [10] conducted a comprehensive review comparing HTLV-1 and HTLV-2, focusing on key areas such as epidemiology, pathobiology, gene products, and genomic structure. Their work highlights the similarities and differences in how these viruses spread, their genetic makeup, and the diseases they cause, providing a deeper understanding of their biological characteristics and public health impact.
Co-infections between HIV-1 and HTLV-1/-2 are known to happen more often, because the viruses have the same pathways of acquisition and dissemination [11]. This is especially true in big cities where injection drug users (IDUs) and sexual activity are the major ways that HIV-1 and HTLV-1/-2 viruses spread [11]. IDUs may found in the US, Europe, Asia, South America, and many Native American Indian groups are endemic to HTLV-2 infection. It would seem that injectable drug users who are also HIV-1 infected are more likely to have HTLV-2 infection in several countries [6]. Since routine HTLV-1/-2 testing is not often done in outpatient clinics, HTLV-1/HIV-1 and HTLV-2 and HIV-1 coinfections likely occur more frequently than doctors realize [11]. HIV-1-positive people are thought to have rates of HTLV-1 or HTLV-2 coinfections that are at least 100–500 times higher than those in the general population. Five to ten percent of those living with HIV-1 infection may also have HTLV-1 or HTLV-2 co-infection in particular geographical areas [11]. Several publications have described cases of co-infection between HTLV-2 and HIV-1 (see the review articles [12,13,14]).
Our knowledge of viral dynamics has significantly increased thanks to rigorous mathematical modeling and analysis, which can help us come up with workable and efficient management plans to eradicate viral infections. One of the areas of mathematical immunology that is progressing the fastest is the formulation of mathematical models of the dynamics of HIV-1 infection. Three populations are included in the classic model of HIV-1 mono-infection [15]: Free HIV-1 particles, uninfected CD4+ T cells and infected cells. The model was expanded to incorporate the influence of CD8+ T cells in several publications (see e.g., [15,16,17,18,19,20]). B cells is another arm of the adaptive immune response, which produce antibodies to attack the viruses. Models with B-cell response have been investigated in many papers (see e.g., [21,22,23,24,25,26]). The HIV-1 mono-infection model under the impact of both B cells and CD8+ T cells can be written as [27]:
˙U=λ⏟production rate of CD4+ T cells−dU⏟death rate−β1UV⏟HIV-1 infectious transmission rate,˙Y=β1UV⏟HIV-1 infectious transmission rate−a1Y⏟death rate−ϕYE⏟killing rate of HIV-1-infected cells by CD8+ T cells,˙V=k1Y⏟generation rate of HIV-1−c1V⏟clearance rate of HIV-1−rVW⏟neutralization rate of HIV-1 by B cells,˙E=Ψ(Y,E)⏟proliferation rate of CD8+ T cells−ζE⏟death rate,˙W=Φ(V,W)⏟proliferation rate of HIV-1-specific B cells−μW⏟death rate, |
where U=U(t), Y=Y(t), V=V(t), E=E(t), and W=W(t) are the concentrations of uninfected CD4+ T cells, HIV-1-infected CD4+ T cells, HIV-1 particles, uninfected CD8+ T cells and HIV-1-specific B cells at time t. Here, the proliferation rate of CD8+ T cells and B cells are represented, respectively, by Ψ(Y,E) and Φ(V,W). The model has been extended in several works (see e.g., [28,29,30]). The literature took into consideration the following particular forms of Ψ(Y,E) and Φ(V,W) as follows:
SS-(I): Self-regulating immune response, Ψ(Y,E)=ξ and Φ(V,W)=ϰ, where ξ,ϰ>0 [31],
SS-(II): Linear immune response, Ψ(Y,E)=˜πY [32,33,34] and Φ(V,W)=˜ςV [24,35], where ˜π,˜ς>0,
SS-(III): Predator-prey like immune response, Ψ(Y,E)=πYE [15,20,31] and Φ(V,W)=ςVW [22,24,31,36], where π,ς>0
SS-(IV): Combination of SS-(Ⅰ), SS-(Ⅱ) and SS-(Ⅲ), Ψ(Y,E)=ξ+˜πY+πYE [31,37] and Φ(V,W)=ϰ+˜ςV+ςVW [31],
SS-(V): Combination of predator-prey like immune and self-proliferation immune responses: Ψ(Y,E)=πYE+qE(1−EEmax), where q,Emax>0 [17].
SS-(VI): Saturated immune response: Ψ(Y,E)=πYEϑ+E [18,38,39,40], Φ(V,W)=ςVWϑ+W [41,42], where ϑ>0.
The infection rate of cells, denoted as β1UV, is influenced by some biological factors, such as saturation and pyroptosis. Saturation is considered when the concentration of the viruses is high. In this case the infection rate is reduced and given by β1UV1+νV, where ν is the saturation constant [43]. Pyroptosis is a highly inflammatory type of programmed cell death triggered during incomplete HIV-1 infection, leads to the release of pro-inflammatory cytokines. These cytokines have the ability to attract more CD4+ T cells to the infection site, expanding the number of cells susceptible to HIV infection. This makes the deterioration of the immune system worse [44,45]. The impact of pyroptosis on HIV-1 dynamics was initially explored in a model presented in [46]. The infection rate was modeled by a bilinear incidence β1UV, which is enhanced by the inflammatory cytokine (C) with a factor γ as β1(1+γC)UV. This model was later extended to incorporate reaction-diffusion processes, as studied in subsequent works [47,48,49,50]. These extensions aimed to capture spatial effects and the spread of infection in tissues, providing deeper insights into the role of pyroptosis in HIV-1 infection.
The co-infection of HIV-1 and HTLV-1 has been modeled in a number of recent research (see e.g., [43,51,52,53]). The models presented in [43,51,52] were built on the premise that both HIV-1 and HTLV-1 compete for the same target cells, CD4+ T cells. The effect of CTL response and latently infected cells have been included in model presented in [51,52]. In [52], it was assumed that HIV spreads through two main pathways: Virus-to-cell transmission and direct cell-to-cell contact. In contrast, HTLV-1 is transmitted via two distinct mechanisms: (ⅰ) horizontally through direct cell-to-cell interactions, and (ⅱ) vertically during the mitotic division of Tax-expressing HTLV-1-infected cells. In [43], both uninfected and infected CD4+ T cells are modeled to proliferate according to a full logistic growth form. Additionally, the infection rate is modeled using a saturated incidence form. A stochastic model for the co-infection dynamics of HIV and HTLV-1, which also includes the effects of AIDS-related cancer cells, was explored in [53].
To the best of our knowledge, no earlier studies on modeling HTLV-2 and HIV-1 co-infection within a host have been conducted. In this study, we construct a new in vivo model of co-infection between HIV-1 and HTLV-2. Apart from the global stability of the equilibria, we investigate the fundamental characteristics of the solutions to the model. By constructing suitable Lyapunov functions and employing Lyapunov-LaSalle asymptotic stability theorem (L-LAST), the six equilibria's global stability is demonstrated. We conduct numerical simulations to demonstrate and validate the theoretical findings. We conclude by discussing the results.
Our proposed model and its analysis may provide valuable insights into the dynamics of co-infection between different human viruses. By capturing the interactions between multiple infections and the immune system's response to chronic viral co-infections, this model serves as a tool to explore how co-infection influences disease progression. Additionally, the framework has the potential to forecast new treatment approaches, offering predictions on optimal therapies that could address viral co-infections more effectively. This comprehensive analysis could contribute to developing strategies for improving patient outcomes.
In this section, we propose a new model for the co-infection of HTLV-2 and HIV-1 in vivo. To formulate our model, we need the following hypothesis:
(H1) The key components of the model include the concentration of, uninfected CD4+ T cells (U(t)), HIV-1-infected CD4+ T cells (Y(t)), HIV-1 particles (V(t)), uninfected CD8+ T cells (E(t)), HTLV-2-infected CD8+ T cells (H(t)) and HIV-1-specific B cells (W(t)) at time t. The death (or clearance or decay) rates of compartments U, Y, V, E, H and W are denoted by dU, a1Y, c1V, ζE, a2H and μW, respectively. The HTLV-2 and HIV-1 co-dynamics is depicted in the schematic diagram in Figure 1.
(H2) HIV-1 primarily targets uninfected CD4+ T cells. Uninfected CD4+ T cells are produced at a constant rate λ and become infected by HIV-1 particles through virus-to-cell transmission at a rate β1UV [15] (see Eq (2.1)).
(H3) HIV-1-infected CD4+ T cells are generated at a rate of β1UV due to the interaction between uninfected CD4+ T cells and free HIV-1 particles. These infected cells are subsequently eliminated by CD8+ T cells at a rate of ϕYE [15] (see Eq (2.2)).
(H4) Free HIV-1 particles are generated from HIV-1-infected CD4+ T cells at the rate of k1Y. These viral particles are then neutralized at a rate of rVW, where the neutralization is carried out by HIV-1-specific antibodies which are produced by the HIV-1-specific B cells [31] (see Eq (2.3)).
(H5) HTLV-2 mainly infects CD8 + T cells [9]. We assume that, in the absence of both HIV-1 and HTLV-2 infections, the baseline level of CD8+ T cells is represented by ξ/ζ, where ξ signifies the source of CD8+ T cells that are specific to HIV-1. Upon HIV-1 infection, the immune system is triggered, leading to an expansion of CD8+ T cells at a rate πYE, which is influenced by the concentrations of CD4+ T cells infected with HIV-1 and CD8+ T cells [31]. Therefore, the production rate of uninfected CD8+ T cells results from a combination of self-regulation mechanisms (ξ) and immune responses similar to a predator-prey interaction (πYE), modeled by ξ+πYE. Uninfected CD8+ T cells become infected upon contact with HTLV-2-infected CD8+ T cells via cell-to-cell transmission, occurring at a rate β2EH [10] (see Eq (2.4)).
(H6) HTLV-2-infected CD8+ T cells are generated at rate of β2EH due to the cell-to-cell interaction between uninfected CD8+ T cells and HTLV-2-infected CD8+ T cells [10] (see Eq (2.5)).
(H7) HIV-1-specific B cells are stimulated at rate ςVW, which is influenced by the concentrations of free HIV-1 particles and HIV-1-specific B cells [22,31] (see Eq (2.6)).
Based on hypothesis H1-H7, our proposed HTLV-2 and HIV-1 co-infection model is given by:
˙U=λ−dU−β1UV, | (2.1) |
˙Y=β1UV−a1Y−ϕYE, | (2.2) |
˙V=k1Y−c1V−rVW, | (2.3) |
˙E=ξ+πYE−ζE−β2EH, | (2.4) |
˙H=β2EH−a2H, | (2.5) |
˙W=ςVW−μW. | (2.6) |
The definition of variables and parameters are given in Table 1. The model's parameters are all positive. The initial condition is given by:
U(0)>0,Y(0)≥0, V(0)≥0, E(0)>0, H(0)≥0, W(0)≥0. |
We emphasize that our proposed HTLV-2 and HIV-1 co-infection model (2.1)–(2.6) is different from the HTLV-1 and HIV-1 co-infection models presented in [43,51,52] in such away that both HTLV-1 and HIV-1 compete for the same target cells, CD4+ T cells (i.e., Eq (2.1) becomes ˙U=λ−dU−β1UV−ˉβ1UK, where, K denotes the HTLV-1-infected CD4+ T cells). A detailed analysis of the system described in model (2.1)–(2.6) will be addressed in next sections, where an in-depth examination of the model's dynamics will be carried out.
Symbol | Description | Value | Source |
U | Concentration of uninfected CD4+T cells | cells mm-3 | |
Y | Concentration of HIV-1-infected CD4+T cells | cells mm-3 | |
V | Concentration of HIV-1 particles | viruses mm-3 | |
E | Concentration of uninfected CD8+T cells | cells mm-3 | |
H | Concentration of HTLV-2-infected CD8+T cells | cells mm-3 | |
W | Concentration of HIV-1-specific B cells | cells mm-3 | |
λ | Production rate of uninfected CD4+T cells | 10 cells mm-3day-1 | [54,55] |
d | Death rate of uninfected CD4+T cells | 0.01 day-1 | [25,56] |
β1 | Infection rate of uninfected CD4+T cells by HIV-1 | varied | |
a1 | Death rate of HIV-1-infected CD4+T cells | 0.4 day-1 | [54] |
k1 | Production rate of HIV-1 | 38 viruses cells-1 day-1 | [56,57] |
c1 | Clearance rate of HIV-1 | 2.4 day-1 | [54,55,56] |
r | Neutralization rate of HIV-1 particles by B cells | 0.1 cells-1mm3 day-1 | |
ϕ | Killing rate of HIV-1-infected CD4+T cells | 0.01 cells -1 mm3 day-1 | [58] |
by CD8+T cells | |||
ξ | Production rate of uninfected CD8+T cells | 20 cells mm-3 day-1 | [59] |
π | Stimulation rate of uninfected CD8+T cells | 0.0000005 cells-1 mm3 day-1 | [59] |
0.2 cells-1 mm3 day-1 | [58] | ||
ζ | Death rate of uninfected CD8+T cells | 0.06 day-1 | [59] |
β2 | Infection rate of uninfected CD8+T cells by HTLV-2 | varied | |
a2 | Death rate of HTLV-2-infected CD8+T cells | 0.3 day-1 | Assumed |
ς | Stimulation rate of HIV-1-specific B cells | varied | |
μ | Decay rate of HIV-1-specific B cells | 0.24 day-1 | [60] |
In this section, the fundamental qualitative characteristics of the system (2.1)–(2.6), such as non-negativity and boundedness of solutions, are examined. We find the model's equilibria and determine a set of threshold parameters which determine the existence of the model's equilibria.
We demonstrate that the model (2.1)–(2.6) is well-posed by establishing the nonnegativity and boundedness of the solutions.
Lemma 1. Solution of the system (2.1)–(2.6) are non-negative and bounded.
Proof. From Eqs (2.1)–(2.6) We get
˙U|U=0=λ>0,˙Y|Y=0=β1UV≥0,∀V,U≥0,˙V|V=0=k1Y≥0,∀Y≥0, ˙E|E=0=ξ>0,˙H|H=0=0, ˙W|W=0=0. |
Therefore, in accordance with the Proposition B.7 of [61]
(U(t),Y(t),V(t),E(t),H(t),W(t))∈R6≥0 for any t≥0 when (U(0),Y(0),V(0),E(0),H(0),W(0))∈R6≥0. |
To demonstrate the solutions' boundedness, let define Γ(t) as:
Γ=U+Y+a12k1V+ϕπ[E+H]+a1r2k1ςW. |
Next, we get
˙Γ=˙U+˙Y+a12k1˙V+ϕπ[˙E+˙H]+a1r2k1ς˙W=λ−dU−β1UV+β1UV−a1Y−ϕYE+a12k1[k1Y−c1V−rVW]+ϕπ[ξ+πYE−ζE−β2EH+β2EH−a2H]+a1r2k1ς[ςVW−μW]=λ+ξϕπ−dU−a12Y−a1c12k1V−ϕζπE−a2ϕπH−a1rμ2k1ςW≤λ+ξϕπ−ϱ[U+Y+a12k1V+ϕπ(E+H)−a1r2k1ςW]=λ+ξϕπ−ϱΓ, |
where ϱ=min{d,a1/2,c1,ζ,a2,μ}. Thus, Γ≤λϱ+ξϕπϱ=τ1, ifΓ(0)≤τ1. It follows that
0≤U(t),Y(t)≤τ1,0≤V(t)≤τ2,0≤E(t),H(t)≤τ3,0≤W(t)≤τ4 |
if
U(0)+Y(0)+a12k1V(0)+ϕπ[E(0)+H(0)]+a1r2k1ςW(0)≤τ1, |
where τ2=2k1a1τ1,τ3=πϕτ1 and τ4=2k1ςa1rτ1.
Lemma 2. For model (2.1)–(2.6), there exist six equilibria besides seven threshold parameters (Ri, i=1,2,…,7) such that
(I) Infection-free equilibrium, Δ0, is always presented, where Δ0=(U0,0,0,E0,0,0).
(II) If R1>1, then an HTLV-2 mono-infection equilibrium, Δ1, exists besides Δ0, where Δ1=(U1,0,0,E1,H1,0).
(III) If R2>1, then an HIV-1 mono-infection equilibrium in the absence of HIV-1-specific B-cell response, Δ2, exists besides Δ0, where Δ2=(U2,Y2,V2,E2,0,0).
(IV) If R3>1, then an HIV-1 mono-infection equilibrium with an active HIV-1-specific B-cell response, Δ3, exists besides Δ0, where Δ3=(U3,Y3,V3,E3,0,W3).
(V) If R7≤1<R4 and R5>1, then an HTLV-2 and HIV-1 co-infection equilibrium in the absence of HIV-1-specific B-cell response, Δ4, exists besides Δ0, where Δ4=(U4,Y4,V4,E4,H4,0).
(VI) If R6>1 and R7>1, then an HTLV-2 and HIV-1 co-infection equilibrium with an active HIV-1-specific B-cell response, Δ5, exists besides Δ0, where Δ5=(U5,Y5,V5,E5,H5,W5).
Proof. Equilibria of (2.1)–(2.6) fulfill
{0=λ−dU−β1UV,0=β1UV−a1Y−ϕYE,0=k1Y−c1V−rVW,0=ξ+πYE−ζE−β2EH,0=β2EH−a2H,0=ςVW−μW. |
We get that the provided model (2.1)–(2.6) has six equilibria:
1) Infection-free equilibrium, Δ0=(U0,0,0,E0,0,0), where U0=λd and E0=ξζ.
2) HTLV-2 mono-infection equilibrium, Δ1=(U1,0,0,E1,H1,0), where
U1=λd=U0,E1=a2β2=E0R1,H1=ζβ2(R1−1), |
where
R1=ξβ2a2ζ, |
which stands for the HTLV-2 mono-infection basic reproduction ratio. The parameter R1 plays a critical role in clinical settings, as it helps determine whether an HTLV-2 infection will persist chronically. It quantifies the average number of new HTLV-2-infected CD8+ T cells generated from the interaction between HTLV-2-infected CD8+ T cells and uninfected CD8+ T cells, indicating the potential for viral spread within the host.
3) HIV-1 mono-infection equilibrium in the absence of HIV-1-specific B-cell response, Δ2=(U2,Y2,V2,E2,0,0), where
U2=a1Y2+ϕY2E2β1V2,V2=k1Y2c1,E2=ξζ−πY2 |
and Y2 fulfills the following:
Ω1Y2+Ω2Y+Ω3ζ−πY=0, |
where
Ω1=β1a1k1π,Ω2=c1da1π−λβ1k1π−a1β1ζk1−ϕξβ1k1,Ω3=λβ1k1ζ−c1da1ζ−c1ϕdξ. |
We define a function G1(Y) as:
G1(Y)=Ω1Y2+Ω2Y+Ω3ζ−πY,(0,ζπ). |
Note that, G1 is continuous on (0,ζπ). We have
G1(0)=λβ1k1ζ−c1da1ζ−c1ϕdξζ=c1d(a1ζ+ϕξ)ζ(R2−1) |
where
R2=λβ1k1ζc1d(a1ζ+ϕξ), |
indicates HIV-1 mono-infection basic reproduction ratio, which determines whether or not an HIV-1 mono-infection can be established. R2, refers to the average number of newly HIV-1-infected CD4+ T cells generated by a single infected cell in a situation where nearly all CD4+ T cells are uninfected. It provides a measure of how efficiently a virus can spread within the host at the early stages of infection, influencing whether the infection will proliferate or die out.
Since G1(0)>0 if R2>1 in addition to limY→(ζπ)−G1(Y)=−∞, there exist Y2 such that 0<Y2<ζπ and satisfies G1(Y2)=0. Consequently, we get U2>0,V2>0 and E2>0.
4) HIV-1 mono-infection equilibrium with an active HIV-1-specific B-cell response, Δ3=(U3,Y3,V3,E3,0,W3), where
U3=ςλdς+β1μ, V3=μς, E3=ξζ−πY3, W3=c1r(k1ςμc1Y3−1) |
and Y3 fulfills the following:
ω1Y2+ω2Y+ω3ζ−πY=0, |
where
ω1=a1π(dς+β1μ), ω2=−λβ1μπ−(dς+β1μ)(a1ζ+ϕξ), ω3=λβ1ζμ. |
We define a function G2(Y) as:
G2(Y)=ω1Y2+ω2Y+ω3ζ−πY. |
Note that G2 is continuous on (0,ζπ). We have G2(0)=λβ1ζμζ>0. Moreover, limY→(ζπ)−G2(Y)=−∞, there is Y3 such that 0<Y3<ζπ and satisfies G2(Y3)=0. Consequently, we get
U3=ςλdς+β1μ>0, V3=μς>0, E3=ξζ−πY3>0, W3=c1r(R3−1)>0 |
where
R3=k1ςμc1Y3. |
Here, R3 is the activation number of HIV-1-specific B-cell response in the case of HIV-1 mono-infection. The parameter R3 indicates whether the HIV-1-specific B-cell response will be activated in the absence of HTLV-2 infection. It serves as a threshold to assess the immune system's ability to respond to HIV-1 without the influence of co-infection, determining whether an effective immune response is triggered.
5) HTLV-2/HIV-I co-infection equilibrium in the absence of HIV-1-specific B-cell response, Δ4=(U4,Y4,V4,E4,H4,0), where
U4=c1(a1β2+a2ϕ)k1β2β1, Y4=c1dk1β1(R4−1), V4=dβ1(R4−1), E4=a2β2, H4=c1dπ+β1k1ζk1β2β1(R5−1) |
where
R4=λk1β2β1c1d(a1β2+a2ϕ),R5=k1β2β1c1dπ+β1k1ζ(ξa2+πλa1β2+a2ϕ). |
Consequently, if R4>1 and R5>1, then the co-infection equilibrium in the absence of HIV-1-specific B-cell response, Δ4, exists. In this scenario, the threshold parameters R4 and R5 determine the likelihood of HIV-1 and HTLV-2 co-infection in the absence of an HIV-1-specific B-cell response. These values help assess whether conditions are favorable for the co-infection to establish and persist, particularly when the immune response to HIV-1 is not fully activated.
6) HTLV-2 and HIV-1 co-infection equilibrium with an active HIV-1-specific B-cell response, Δ5=(U5,Y5,V5,E5,H5,W5), where
U5=ςλdς+β1μ, Y5=β2β1λμ(a1β2+a2ϕ)(dς+β1μ), V5=μς,E5=a2β2,H5=ζβ2(R6−1), W5=c1r(R7−1), |
where
R6=β2ζ(ξa2+β1πλμ(a1β2+a2ϕ)(dς+β1μ)),R7=λk1β2β1ςc1(a1β2+a2ϕ)(dς+β1μ). |
Thus, when R6>1 and R7>1, then the co-infection equilibrium with an active HIV-1-specific B-cell response, Δ5, exists. The parameter R6 serves as an indicator of whether individuals infected with HIV-1 could also become co-infected with HTLV-2. It reflects the conditions under which co-infection may occur, based on the dynamics of the two viruses and the patient's immune response. In addition, R7 indicates the activation number of HIV-1-specific B-cell response in the case HTLV-2/HIV-I co-infection. We note that
R7=λk1β2β1ςc1(a1β2+a2ϕ)(dς+β1μ)=R41+β1μdς<R4. |
Hence, R7<R4.
In this section, we aim to examine the global asymptotic stability of the all model's equilibria (2.1)–(2.6) constructing Lyapunov functions [62] and applying Lyapunov-LaSalle asymptotic stability theorem (L-LAST) [63,64,65]. Define a function S(x)=x−1−lnx where S(x)≥0 for all x>0 and S(1)=0. Furthermore, the arithmetic mean-geometric mean inequality presented below is employed to prove Theorems 1–6.
n∑i=1Xin≥(n∏i=1Xi)1n | (4.1) |
Consider the Lyapunov function candidate Li and define Λ′i as the largest invariant set of
Λi={(U,Y,V,E,H,W):dLidt=0},i=0,1,2,3,4,5. |
Theorem 1. The infection-free equilibrium Δ0 is globally asymptotically stable (GAS) if R1≤1 and R2≤1. In addition, if R1>1 and/or R2>1, then the Δ0 is unstable.
Proof. Define L0(U,Y,V,E,H,W) as:
L0=U0S(UU0)+Y+β1U0c1V+ϕπE0S(EE0)+ϕπH+β1rU0c1ςW. |
Obviously, L0(U,Y,V,E,H,W)>0 for any U,Y,V,E,H,W>0 and L0(U0,0,0,E0,0,0)=0. The derivative of L0 along the solutions of system (2.1)–(2.6) can be calculated as:
dL0dt=(1−U0U)˙U+˙Y+β1U0c1˙V+ϕπ(1−E0E)˙E+ϕπ˙H+β1rU0c1ς˙W. |
By replacing the equations mentioned in model (2.1)–(2.6), we obtain
dL0dt=(1−U0U)(λ−dU−β1UV)+(β1UV−a1Y−ϕYE)+β1U0c1(k1Y−c1V−rVW)+ϕπ(1−E0E)(ξ+πYE−ζE−β2EH)+ϕπ(β2EH−a2H)+β1rU0c1ς(ςVW−μW). |
Collecting the terms and using λ=dU0 and ξ=ζE0, we obtain
dL0dt=−dU(U−U0)2−ϕπζE(E−E0)2+(β1k1c1U0−a1−ϕE0)Y+ϕπ(β2E0−a2)H−β1rμU0c1ςW=−dU(U−U0)2−ϕπζE(E−E0)2+a1ζ+ϕξζ(λk1β1ζc1d(a1ζ+ϕξ)−1)Y+ϕa2π(ξβ2a2ζ−1)H−β1rμλc1dςW. |
Ultimately, we obtain
dL0dt=−dU(U−U0)2−ϕπζE(E−E0)2+a1ζ+ϕξζ(R2−1)Y+ϕa2π(R1−1)H−β1rμλc1dςW. |
Hence, dL0dt≤0 satisfies if R1≤1 and R2≤1. Moreover, dL0dt=0 when U=U0, E=E0, W=0,(R2−1)Y=0 and (R1−1)H=0. Solutions of the system tend to Λ′0 [66]. Any element in Λ′0 satisfies U=U0, E=E0, W=0,
(R2−1)Y=0 and (R1−1)H=0. | (4.2) |
There are four cases:
(I) R1=1 and R2=1. Then from Eq (2.1) we get
0=˙U=λ−dU0−β1U0V⟹V(t)=0 for any t. | (4.3) |
From Eq (2.3) we have
0=˙V=k1Y⟹Y(t)=0 for any t. | (4.4) |
Equation (2.4) suggests that
0=˙E=ξ−ζE0−β2E0H⟹H(t)=0 for any t. | (4.5) |
Hence Λ′0={Δ0}.
(II) R1<1 and R2<1. Then from Eq (4.2) we have Y=H=0 and Eq (4.3) indicates V=0. Consequently, Λ′0={Δ0}.
(III) R1=1 and R2<1. Then from Eq (4.2) we get Y=0. Equations (4.3) and (4.5) imply V=H=0. Thus Λ′0={Δ0}.
(IV) R1<1 and R2=1. Equation (4.2) gives H=0 while Eqs (4.3) and (4.4) give, V=Y=0. Thus Λ′0={Δ0}.
By L-LAST [63,64,65], Δ0 is GAS.
To establish the instability of Δ0 if R1>1 and/or R2>1, it is necessary to construct the Jacobian matrix J=J(U,Y,V,E,H,W) of model (2.1)–(2.6) as:
J=(−d−β1V0−β1U000β1V−a1−ϕEβ1U−ϕY000k1−c1−rW00−rV0πE0πY−ζ−β2H−β2E0000β2H−a2+β2E000ςW00ςV−μ). | (4.6) |
Therefore, at Δ0, the characteristic equation is provided by
det(J−σI)=(σ+d)(σ+ζ)(σ+μ)(b1σ+b0)(˜b2σ2+˜b1σ+˜b0)=0, | (4.7) |
where I is the identity matrix and σ is the eigenvalue and
b1=ζ,b0=a2ζ(1−R1),˜b2=dζ,˜b1=d(ϕξ+ζ(a1+c1)),˜b0=c1d(a1ζ+ϕξ)(1−R2). |
If R1>1 and/or R2>1, then b0<0 and/or ˜b0<0, respectively. Hence, Eq (4.7) has positive root and then Δ0 is unstable.
Theorem 2. HTLV-2 mono-infection equilibrium Δ1 is GAS if R1>1 and R4≤1. Moreover, if R4>1 then Δ1 is unstable.
Proof. construct L1(U,Y,V,E,H,W) as:
L1=U1S(UU1)+Y+β1U1c1V+ϕπE1S(EE1)+ϕπH1S(HH1)+β1rU1c1ςW. |
Clearly L1(U,Y,V,E,H,W)>0 for any U,Y,V,E,H,W>0 and L1(U1,0,0,E1,H1,0)=0. Calculating dL1dt as:
dL1dt=(1−U1U)(λ−dU−β1UV)+(β1UV−a1Y−ϕYE)+β1U1c1(k1Y−c1V−rVW)+ϕπ(1−E1E)(ξ+πYE−ζE−β2EH)+ϕπ(1−H1H)(β2EH−a2H)+β1rU1c1ς(ςVW−μW). |
Collecting terms results to
dL1dt=(1−U1U)(λ−dU)−a1Y+β1k1c1U1Y+ϕπ(1−E1E)(ξ−ζE)−ϕE1Y+ϕβ2πE1H−ϕβ2πH1E−a2ϕπH+a2ϕπH1−β1rμU1c1ςW. |
By using the subsequent equilibrium conditions
λ=dU1, ξ=ζE1+β2E1H1,β2E1H1=a2H1, |
we get
dL1dt=−dU(U−U1)2−ϕπζE(E−E1)2+(β1k1c1U1−a1−ϕE1)Y+ϕπ(1−E1E)β2E1H1+ϕπ(β2E1−a2)H−ϕβ2πH1E+ϕβ2πE1H1−β1rμU1c1ςW. |
Thus,
dL1dt=−dU(U−U1)2−ϕπζE(E−E1)2+a1β2+a2ϕβ2(λk1β2β1c1d(a1β2+a2ϕ)−1)Y+ϕπβ2E1H1(2−E1E−EE1)−β1rμU1c1ςW=−dU(U−U1)2−ϕπξEE1(E−E1)2+a1β2+a2ϕβ2(R4−1)Y−β1rμU1c1ςW. |
Thus, if R1>1, R4≤1 and using inequality (4.1), we conclude that dL1dt≤0 for any U,Y,V,E,H,W>0. In addition, dL1dt=0 if U=U1,E=E1,W=0 and (R4−1)Y=0. Λ′1 is reached via the solutions of model (2.1)–(2.6). In Λ′1 we have U=U1,E=E1, W=0 and
(R4−1)Y=0. | (4.8) |
Two cases are at hand:
(I) R4=1, then from Eq (2.1)
0=˙U=λ−dU1−β1U1V⟹V(t)=0 for any t. | (4.9) |
From Eq (2.3) we have
˙V=0=k1Y⟹Y(t)=0 for any t. | (4.10) |
Equation (2.4) implies that
0=˙E=ξ−ζE1−β2E1H⟹H(t)=H1 for any t. | (4.11) |
The Λ′1={Δ1}.
(II) R4<1, then Eq (4.8) implies that Y=0 and Eqs (4.9) and (4.11) give V=0 and H=H1, respectively. Hence, Λ′1={Δ1}.
Thus, by L-LAST, Δ1 is GAS. To determine whether Δ1 is unstable when R4>1, we compute the characteristic equation at Δ1 utilizing the Jacobian matrix provided in (4.6) as:
det(J−σI)=(σ+d)(σ+μ)(m2σ2+m1σ+m0)(n2σ2+n1σ+n0)=0, | (4.12) |
where
m2=a2,m1=β2ξ,m0=a22ζ(R1−1),n2=dβ2,n1=a1dβ2+a2dϕ+c1dβ2n0=c1d(a1β2+a2ϕ)(1−R4). |
If R4>1, then n0<0, and hence, Eq (4.12) has a positive root. Consequently, Δ1 is unstable.
Theorem 3. HIV-1 mono-infection equilibrium in the absence of HIV-1-specific B-cell response, Δ2 is GAS if R2>1, R6≤1 and R7≤1.
Proof. Define L2(U,Y,V,E,H,W) as:
L2=U2S(UU2)+Y2S(YY2)+β1U2c1V2S(VV2)+ϕπE2S(EE2)+ϕπH+β1rU2c1ςW. |
Evidently, L2(U,Y,V,E,H,W)>0for anyU,Y,V,E,H,W>0andL2(U2,Y2,V2,E2,0,0)=0. Calculating dL2dt as:
dL2dt=(1−U2U)(λ−dU−β1UV)+(1−Y2Y)(β1UV−a1Y−ϕYE)+β1U2c1(1−V2V)(k1Y−c1V−rVW)+ϕπ(1−E2E)(ξ+πYE−ζE−β2EH)+ϕπ(β2EH−a2H)+β1rU2c1ς(ςVW−μW). |
Collecting the above terms leads to
dL2dt=(1−U2U)(λ−dU)−β1UVY2Y−a1Y+a1Y2+ϕY2E+β1k1c1U2Y−β1k1c1U2V2VY+β1U2V2+β1rU2c1V2W+ϕπ(1−E2E)(ξ−ζE)−ϕE2Y+ϕβ2πE2H−ϕa2πH−β1rμU2c1ςW. |
Utilizing the equilibrium conditions
λ=dU2+β1U2V2,β1U2V2=a1Y2+ϕY2E2,k1Y2=c1V2,ξ=−πY2E2+ζE2, |
we obtain
dL2dt=−dU(U−U2)2−ϕπζE(E−E2)2+(1−U2U)β1U2V2−ϕ(1−E2E)Y2E2+(β1k1c1U2−a1−ϕE2)Y+ϕπ(β2E2−a2)H+β1rU2c1(V2−μς)W−β1UVY2Y+a1Y2+ϕY2E−β1k1c1U2V2VY+β1U2V2+ϕY2E2−ϕY2E2=−dU(U−U2)2−ϕπξEE2(E−E2)2+β1U2V2(3−U2U−UVY2U2V2Y−YV2Y2V)+ϕβ2π(E2−E5)H+β1rU2c1(V2−V5)W. |
In case R6≤1 and R7≤1, then co-infection equilibrium with an active HIV-1-specific B-cell response Δ5 does not exist since H5≤0 and W5≤0. Thus,
˙H=β2EH−a2H=β2(E−a2β2)H≤0, for any H>0.˙W=ςVW−μW=ς(V−μς)W≤0, for any W>0. |
This happens when E2≤a2β2=E5 and V2≤μς=V5. Then, using inequality (4.1), we obtain that dL2dt≤0 for any U, Y, V, E, H, W>0. Moreover, dL2dt=0 if U=U2, Y=Y2, V=V2, E=E2,(V2−V5)W=0 and (E2−E5)H=0. Λ′2 is reached by the model's solutions. Λ′2 has elements with U=U2, Y=Y2, V=V2, E=E2,
(V2−V5)W=0 and (E2−E5)H=0. | (4.13) |
We have four cases:
(I) V2=V5 and E2=E5. From Eq (2.4) we have
0=˙E=ξ+πY2E2−ζE2−β2E2H⟹H(t)=0for any t. | (4.14) |
From Eq (2.3) implies that
0=˙V=k1Y2−c1V2−rV2W⟹W(t)=0 for any t. | (4.15) |
Hence Λ′2={Δ2}.
(II) V2<V5 and E2<E5. Then from Eq (4.13) we get H=W=0. Thus Λ′2={Δ2}.
(III) V2<V5 and E2=E5. Equation (4.13) leads to W=0 while Eq (4.14) implies that H=0. Thus, Λ′2={Δ2}.
(IV) V2=V5 and E2<E5. Equations (4.13) and (4.15) imply that H=W=0. Hence, Λ′2={Δ2}.
Consequently, by L-LAST, Δ2 is GAS.
Theorem 4. HIV-1 mono-infection equilibrium with an active HIV-1-specific B-cell response, Δ3 is GAS if R3>1, R5≤1.
Proof. Define L3(U,Y,V,E,H,W) as:
L3=U3S(UU3)+Y3S(YY3)+β1U3V3k1Y3V3S(VV3)+ϕπE3S(EE3)+ϕπH+β1rU3V3k1ςY3S(WW3). |
Evidently, L3(U,Y,V,E,H,W)>0for anyU,Y,V,E,H,W>0andL3(U3,Y3,V3,E3,0,W3)=0. Calculating dL3dt as:
dL3dt=(1−U3U)(λ−dU−β1UV)+(1−Y3Y)(β1UV−a1Y−ϕYE)+β1U3V3k1Y3(1−V3V)(k1Y−c1V−rVW)+ϕπ(1−E3E)(ξ+πYE−ζE−β2EH)+ϕπ(β2EH−a2H)+β1rU3V3k1ςY3(1−W3W)(ςVW−μW). |
Collecting the above terms leads to
dL3dt=(1−U3U)(λ−dU)+β1U3V−β1UVY3Y−a1Y+a1Y3+ϕY3E+β1U3V3Y3Y−β1U3V3Y3V3VY−β1c1U3V3k1Y3V+β1c1U3V3k1Y3V3+β1rU3V3k1Y3V3W+ϕπ(1−E3E)(ξ−ζE)−ϕE3Y+ϕβ2πE3H−ϕa2πH−β1rU3V3k1Y3VW3−β1rμU3V3k1ςY3W+β1rμU3V3k1ςY3W3. |
Utilizing the equilibrium conditions
λ=dU3+β1U3V3, β1U3V3=a1Y3+ϕY3E3,k1Y3=c1V3+rV3W3, ξ=−πY3E3+ζE3, V3=μ/ς, |
We obtain
dL3dt=−dU(U−U3)2−ϕπζE(E−E3)2+(1−U3U)β1U3V3−ϕ(1−E3E)Y3E3+(β1U3V3−a1Y3−ϕY3E3)YY3+β1U3(1−c1V3k1Y3−rV3W3k1Y3)V+ϕπ(β2E3−a2)H+β1U3V3(c1V3k1Y3+rV3W3k1Y3)+β1rU3V3k1Y3(V3−μς)W−β1UVY3Y+a1Y3+ϕY3E−β1U3V3Y3V3VY+ϕY3E3−ϕY3E3=−dU(U−U3)2−ϕπξEE3(E−E3)2+β1U3V3(3−U3U−UVY3U3V3Y−YV3Y3V)+ϕβ2π(E3−E4)H. |
In case R5≤1, then Δ4 does not exist since H4≤0. Hence,
˙H=β2EH−a2H=β2(E−a2β2)H≤0 for any H>0. |
This occurs when E3≤a2β2=E4 and using inequality (4.1), we obtain that dL3dt≤0 for any U, Y, V, E, H, W>0. Moreover, dL3dt=0 if U=U3, Y=Y3, V=V3, E=E3 and (E3−E4)H=0. The model's solutions converge to Λ′3 where U=U3, Y=Y3, V=V3, E=E3 and
(E3−E4)H=0. | (4.16) |
We have two cases:
(I) E3=E4. From Eq (2.4) we have
0=˙E=ξ+πY3E3−ζE3−β2E3H⟹H(t)=0for any t. | (4.17) |
From Eq (2.3) implies that
0=˙V=k1Y3−c1V3−rV3W⟹W(t)=W3 for any t. | (4.18) |
Hence Λ′3={Δ3}.
(II) E3<E4. Then from Eq (4.16), we get H=0 and from Eq (4.18), we obtain W=W3. Thus Λ′3={Δ3}.
Consequently, by L-LAST, Δ3 is GAS.
Theorem 5. HTLV-2/HIV-I co-infection equilibrium in the absence of HIV-1-specific B-cell response, Δ4 is GAS if R7≤1<R4 and R5>1.
Proof. Define L4(U,Y,V,E,H,W) as:
L4=U4S(UU4)+Y4S(YY4)+β1U4c1V4S(VV4)+ϕπE4S(EE4)+ϕπH4S(HH4)+β1rU4c1ςW. |
Calculating dL4dt as:
dL4dt=(1−U4U)(λ−dU−β1UV)+(1−Y4Y)(β1UV−a1Y−ϕYE)+β1U4c1(1−V4V)(k1Y−c1V−rVW)+ϕπ(1−E4E)(ξ+πYE−ζE−β2EH)+ϕπ(1−H4H)(β2EH−a2H)+β1rU4c1ς(ςVW−μW). |
Then we get
dL4dt=(1−U4U)(λ−dU)−β1UVY4Y−a1Y+a1Y4+ϕY4E+β1k1c1U4Y−β1k1c1U4V4VY+β1U4V4+β1rU4c1V4W+ϕπ(1−E4E)(ξ−ζE)−ϕE4Y+ϕβ2πE4H−ϕβ2πH4E−a2ϕπH+a2ϕπH4−β1rμU4c1ςW. |
Using the equilibrium conditions
λ=dU4+β1U4V4,β1U4V4=a1Y4+ϕY4E4,k1Y4=c1V4,ξ=−πY4E4+ζE4+β2E4H4, E4=a2/β2, |
we finally get
dL4dt=−dU(U−U4)2−ϕπξEE4(E−E4)2+β1U4V4(3−U4U−UY4VU4YV4−YV4Y4V)+β1r(dς+β1μ)U4c1β1ς(R7−1)W. |
Thus, if R7≤1<R4 and R5>1 and using inequality (4.1), we conclude that dL4dt≤0 for any U,Y,V,E,H,W>0. In addition, dL4dt=0 if U=U4,Y=Y4,V=V4,E=E4 and (R7−1)W=0. Solutions of model (2.1)–(2.6) converge to Λ′4 where U=U4,Y=Y4,V=V4,E=E4 and
(R7−1)W=0. | (4.19) |
We have two cases:
(I) R7=1, hence from Eq (2.4)
0=˙E=ξ+πY4E4−ζE4−β2E4H⟹H(t)=H4 for any t, | (4.20) |
and Eq (2.3) implies that
0=˙V=k1Y4−c1V4−rV4W⟹W(t)=0 for any t. | (4.21) |
Hence, Λ′4={Δ4}.
(II) R7<1, then from Eq (4.19) we get W=0 and from Eq (4.20) we get H=H4. Thus Λ′4={Δ4}.
Thus, by L-LAST Λ′4={Δ4} and Δ4 is GAS.
Theorem 6. HTLV-2 and HIV-1 co-infection equilibrium with an active HIV-1-specific B-cell response, Δ5 is GAS if R6>1 and R7>1.
Proof. Define L5(U,Y,V,E,H,W) as:
L5=U5S(UU5)+Y5S(YY5)+β1U5V5k1Y5V5S(VV5)+ϕπE5S(EE5)+ϕπH5S(HH5)+β1rU5V5k1ςY5S(WW5). |
Evidently, L5(U,Y,V,E,H,W)>0 for any U,Y,V,E,H,W>0andL5(U5,Y5,V5,E5,H5,W5)=0. Calculating dL5dt as:
dL5dt=(1−U5U)(λ−dU−β1UV)+(1−Y5Y)(β1UV−a1Y−ϕYE)+β1U5V5k1Y5(1−V5V)(k1Y−c1V−rVW)+ϕπ(1−E5E)(ξ+πYE−ζE−β2EH)+ϕπ(1−H5H)(β2EH−a2H)+β1rU5V5k1ςY5(1−W5W)(ςVW−μW). |
Collecting the above terms leads to
dL5dt=(1−U5U)(λ−dU)+β1U5V−β1UVY5Y−a1Y+a1Y5+ϕY5E+β1U5V5Y5Y−β1U5V5Y5V5VY−β1c1U5V5k1Y5V+β1c1U5V5k1Y5V5+β1rU5V5k1Y5V5W+ϕπ(1−E5E)(ξ−ζE)−ϕE5Y+ϕβ2πE5H−ϕβ2πEH5−ϕa2πH+ϕa2πH5−β1rU5V5k1Y5VW5−β1rμU5V5k1ςY5W+β1rμU5V5k1ςY5W5. |
Utilizing the equilibrium conditions
λ=dU5+β1U5V5, β1U5V5=a1Y5+ϕY5E5, k1Y5=c1V5+rV5W5, ξ=−πY5E5+ζE5+β2E5H5, E5=a2/β2, V5=μ/ς, |
We finally obtain
dL5dt=−dU(U−U5)2−ϕπζE(E−E5)2+(1−U5U)β1U5V5−ϕY5E5(2−E5E−EE5)+ϕβ2π(2−E5E−EE5)E5H5−β1U5V5UVY5U5V5Y+β1U5V5−β1U5V5YV5Y5V+β1U5V5=−dU(U−U5)2−ϕπξEE5(E−E5)2+β1U5V5(3−U5U−UVY5U5V5Y−YV5Y5V). |
Therefore, if R6>1 and R7>1 then based on inequality (4.1), we deduce that dL5dt≤0 for any U,Y,V,E,H,W>0. In addition, dL5dt=0 if U=U5,Y=Y5,V=V5, and E=E5. Solutions of model (2.1)–(2.6) converge to Λ′5 where U=U5,Y=Y5,V=V5,E=E5 and from Eq (2.4), we get
0=˙E=ξ+πY5E5−ζE5−β2E5H⟹H(t)=H5 for any t. |
Equation (2.3) implies that
0=˙V=k1Y5−c1V5−rV5W⟹W(t)=W5 for any t. |
Thus, using L-LAST, Λ′5={Δ5} and Δ5 is GAS.
Table 2 provides an overview of the existence and global stability conditions for each equilibria of the model (2.1)–(2.6).
Equilibrium | Existence condition | Stability condition |
D0=(U0,0,0,E0,0,0) | - | R1≤1 and R2≤1 |
D1=(U1,0,0,E1,H1,0) | R1>1 | R1>1 and R4≤1 |
D2=(U2,Y2,V2,E2,0,0) | R2>1 | R2>1, R6≤1, and R7≤1 |
D3=(U3,Y3,V3,E3,0,W3) | R3>1 | R3>1 and R5≤1 |
D4=(U4,Y4,V4,E4,H4,0) | R4>1 and R5>1 | R7≤1<R4 and R5>1 |
D5=(U5,Y5,V5,E5,H5,W5) | R6>1,R7>1 | R6>1 and R7>1 |
In this section, we conduct numerical simulations to validate and expand on our theoretical findings, using specific parameter values to demonstrate the model's behavior under different conditions. We numerically investigate the stability of the model's equilibria, analyze the impact of HIV-1-specific B-cell response on the co-dynamics of HIV-1 and HTLV-2, and compare the proposed model with cases of HIV-1 and HTLV-2 mono-infections.
In this part, we solve system (2.1)–(2.6) numerically using the parameter values from Table 1. We present the numerical outcomes through graphical representations to clearly demonstrate the global stability findings outlined in Theorems 1–6. The numerical solutions of the system is performed using MATLAB solver ode45 which is widely recognized for its ability to solve ODEs efficiently. Its strengths lie in its accuracy, adaptability, robustness, and ease of use, making it highly suitable for various applications. These features make ode45 highly practical for general-purpose ODEs solving, particularly for problems where solutions are relatively smooth and do not exhibit stiff behavior.
We consider the following initial points:
IP.1:U(0)=300,Y(0)=3,V(0)=30,E(0)=100,H(0)=15,W(0)=3,IP.2:U(0)=500,Y(0)=2,V(0)=20,E(0)=250,H(0)=10,W(0)=4,IP.3:U(0)=700,Y(0)=1,V(0)=10,E(0)=350,H(0)=5,W(0)=5. |
We select various initial conditions just to demonstrate that, for any starting point within the feasible region, the system's solution will consistently converge to an equilibrium where the chosen parameter values meet the corresponding stability criteria.
The following circumstances result from the selection of values for the parameters β1, β2, ς and fixing π=0.0000005:
Circumstance-1: β1=0.0001, β2=0.0005 and ς=0.01. Our results show that R1=0.56<1 and R2=0.42<1 for these parameter values. As seen in Figure 2, the trajectories that starting with the three different initials lead to the equilibrium Δ0=(1000,0,0,333.33,0,0). This illustrates that Δ0 is GAS in accordance with Theorem 1. HIV-1 and HTLV-2 will be eliminated as a result of this.
Circumstance-2: β1=0.0001, β2=0.002 and ς=0.01. Hence, R1=2.22>1 and R4=0.83<1 are obtained. The results shown in Figure 3 show how the solutions go closer to the equilibrium Δ1=(1000,0,0,150,36.67,0). As a result, Theorem 2 and the numerical results agree. This case demonstrates what occurs when an individual is infected with HTLV-2 but not HIV-1. While CD4+T cell concentrations are within normal limits, it is clear that HTLV-2 infection has almost led to a drop to the half in CD8+T cell counts.
Circumstance-3: β1=0.0003, β2=0.0002 and ς=0.01. Next, we compute R2=1.27>1, R6=0.22<1, and R7=0.18<1. It is clear that the standards stated in Table 2 are clearly met. Figure 4, which illustrates how the solutions converge to the equilibrium Δ2=(785.97,0.57,9.08,333.34,0,0), and thus validates Theorem 3. This case demonstrates what occurs when an individual is infected only with HIV-1 in the absence of HIV-1-specific B-cell response. It is obvious that a reduction in CD4+T cell counts has been caused by HIV-1 infection.
Circumstance-4: β1=0.0003, β2=0.0002 and ς=0.1. Next, we compute R3=1.19>1 and R5=0.22<1. The conditions outlined in Table 2 are therefore evidently satisfied. As shown in Figure 5, the solutions converge to the equilibrium Δ3=(932.84,0.18,2.4,333.33,0,4.48) and validates Theorem 4. This case illustrates what transpires when an individual infected only with HIV-1 and possesses an active HIV-1-specific B-cell response. Compared with circumstance-3, the concentration of uninfected CD4+ T cells is higher in patients with active HIV-1-specific B cells than in those who don't have it. Furthermore, the activation of HIV-1-specific B cells leads to a decrease in the concentrations of HIV-1-infected CD4+ T cells and HIV-1 particles. That means that HIV-1-specific B cells can control HIV-1 infection.
Circumstance-5: β1=0.0005, β2=0.00093 and ς=0.01. The parameters R4=2.18>1, R5=1.03>1 and R7=0.99<1 are provided by these data. As we have proven in Theorem 5, Figure 6 demonstrates that Δ4=(458,1.49,23.67,322.58,2.15,0) exists and is GAS. Here, an individual has co-infections with HIV-1 and HTLV-2. Additionally, the patient may be experiencing a decline in their immune system, which could result in an increase in the symptoms of their condition. The patient might be more likely to die as a result of this.
Circumstance-6: β1=0.0005, β2=0.00093 and ς=0.1. The threshold parameters R6=1.03>1 and R7=1.95>1 are provided by these data. Figure 7 shows that Δ5=(892.86,0.3,2.4,322.58,2.15,22.79) exists and is GAS, as we mentioned in Theorem 6. In this case, an individual has co-infections with HTLV-2 and HIV-1 with an active HIV-1-specific B-cell response. Compared to circumstance-5, the counts of uninfected CD4+T cells is higher, while the counts of HIV-1-infected CD4+ T cells and HIV-1 particles are lower. This result suggest that, even when there is HTLV-2 and HIV-1 co-infection, B cell activation is essential for infection control.
To provide additional verification, a comprehensive examination of the local stability of every given equilibrium is presented. The Jacobian matrix, denoted as J=J(U,Y,V,E,H,W), is calculated with respect to the variables U,Y,V,E,H and W in the model (2.1)–(2.6) as described in (4.6). In the case of each equilibrium, the eigenvalues λi,i=1,⋯,6 of J are computed. An equilibrium is considered to be locally stable if the eigenvalues of the system satisfy Re(λi)<0 for all i=1,2,⋯,6. By doing calculations for every nonnegative equilibrium points and utilizing the parameter values specified in Circumstance 1–6, we infer the eigenvalues associated with each equilibria. In Table 3, the positive equilibria and the real part of the eigenvalues are presented. These result support the global stability results provided in Theorem 1–6.
Circumstance | Equilibrium | Re(λi)<0,i=1,⋯,6 | Stability |
1 | Δ0=(1000,0,0,333.33,0,0) | (−5.13,−1.01,−0.24,−0.13,−0.06,−0.01) | stable |
2 | Δ0=(1000,0,0,333.33,0,0) | (−5.13,−1.01,0.37,−0.24,−0.06,−0.01) | unstable |
Δ1=(1000,0,0,150,36.67,0) | (−4.12,−0.24,−0.18,−0.07,−0.07,−0.01) | stable | |
3 | Δ0=(1000,0,0,333.33,0,0) | (−6.51,0.37,−0.24,−0.23,−0.06,−0.01) | unstable |
Δ2=(785.97,0.57,9.08,333.34,0,0) | (−6.13,−0.23,−0.15,−0.01,−0.01,−0.06) | stable | |
4 | Δ0=(1000,0,0,333.33,0,0) | (−6.51,0.37,−0.24,−0.23,−0.06,−0.01) | unstable |
Δ2=(785.97,0.57,9.08,333.34,0,0) | (−6.13,0.67,−0.23,−0.01,−0.01,−0.06) | unstable | |
Δ3=(932.84,0.18,2.4,333.33,0,4.48) | (−6.57,−0.01,−0.01,−0.23,−0.06,−0.01) | stable | |
5 | Δ0=(1000,0,0,333.33,0) | (−7.48,1.34,−0.24,−0.06,−0.01,0.01) | unstable |
Δ1=(1000,0,0,322.58,2.15,0) | (−7.41,1.39,−0.24,−0.05,−0.01,−0.01) | unstable | |
Δ2=(471.58,1.42,22.41,333.34,0,0) | (−6.14,−0.01,−0.01,−0.06,−0.02,0.01) | unstable | |
Δ4=(458,1.49,23.67,322.58,2.15,0) | (−6.03,−0.01,−0.01,−0.05,−0.01,−0.01) | stable | |
6 | Δ0=(1000,0,0,333.33,0,0) | (−7.48,1.34,−0.24,−0.06,−0.01,0.01) | unstable |
Δ1=(1000,0,0,322.58,2.15,0) | (−7.41,1.39,−0.24,−0.05,−0.01,−0.01) | unstable | |
Δ2=(471.58,1.42,22.41,333.34,0,0) | (−6.14,2,−0.01,−0.01,−0.06,0.01) | unstable | |
Δ3=(892.86,0.29,2.4,333.33,0,21.44) | (−8.24,−0.02,−0.02,−0.06,−0.01,0.01) | unstable | |
Δ4=(458,1.49,23.67,322.58,2.15,0) | (−6.03,2.13,−0.01,−0.01,−0.05,−0.01) | unstable | |
Δ5=(892.86,0.3,2.4,322.58,2.15,22.79) | (−8.27,−0.02,−0.02,−0.05,−0.01,−0.01) | stable |
This subsection examines the impact of stimulated rate constants of HIV-1-specific B-cell, denoted as ς, on the system dynamics described by (2.1)–(2.6). To investigate the impact of HIV-1-specific B cells on the model's solutions, we hold the values of β1=0.0007, β2=0.0009, and π=0.0000005 while varying the parameter ς. By choosing the following initial points:
IP.4:U(0)=600,Y(0)=2,V(0)=20,E(0)=250,H(0)=10,W(0)=4. |
We can see from Figure 8 that as ς increases, the numbers of uninfected CD4+ T cells increase. In contrast, the numbers of HIV-1-infected CD4+T cells and free HIV-1 decrease. It is noteworthy to mention that an increase in the HIV-1-specific B cells does not have an effect on the numbers of uninfected CD8+T cells and HTLV-2-infected CD8+T cells. Consequently, HIV-1-specific B cells aid only in the controlling of HIV-1 infection. Due to the fact that R1 and R2 are independent of ς, increasing ς does not result in the attainment of Δ0. As a result, HIV-1-specific B cells can't completely eradicate the HIV-1 infections; however, they are useful in suppressing HIV-1 progression.
In this section, we present a comparison of the dynamics of HTLV-2 or HIV-1 mono-infection and HTLV-2 and HIV-1 co-infection. Our aim is to examine the influence of HTLV-2 or HIV-1 mono-infection on each other. In this part we will fix the value of π=0.2 and ς=0.1.
We compare the solutions of the HTLV-2 and HIV-1 co-infection model, represented by Eqs (2.1)–(2.6), with the solutions of the following HIV-1 mono-infection with HIV-1-specific B-cell response system (5.1)–(5.5):
˙U=λ−dU−β1UV, | (5.1) |
˙Y=β1UV−a1Y−ϕYE, | (5.2) |
˙V=k1Y−c1V, | (5.3) |
˙E=ξ+πYE−ζE, | (5.4) |
˙W=ςVW−μW. | (5.5) |
We choose the values of the parameters β1=0.001 and β2=0.002, together with the given initial condition:
IP.5:U(0)=200, Y(0)=0.5, V(0)=2.5, E(0)=300, H(0)=200, W(0)=40. |
The solutions of two systems, denoted as (2.1)–(2.6) and (5.1)–(5.5), are presented in Figure 9. We can see from Figure 9 that, HIV-1-positive individuals who also have HTLV-2 infection had lower levels of free HIV-1 and uninfected CD8+ T cells and higher levels of HIV-1-specific B cells. We also observe from Figure 9 that after co-infection, the quantity of uninfected CD4+ T cells remains unchanged. Note that
W5=c1r(λk1β2β1ςc1(a1β2+a2ϕ)(dς+β1μ)−1),∂W5∂β2=a2k1ςβ1λϕr(a1β2+a2ϕ)2(dς+β1μ)>0. |
Thus, W5 is an increasing function of the HTLV-2 infection rate constant β2. This suggests that the presence of HTLV-2 may activate B cells specific to HIV-1, which might lead to a drop in the HIV-1's level. These results are consistent with the findings of other studies (see e.g., [67,68]) showing that co-infection with HTLV-2 is linked to the ability to control HIV-1 replication, the higher chance of survival and the delayed onset of AIDS. HTLV-2 may work as a barrier against HIV-1 infection [69].
We compare the solutions of the HTLV-2 and HIV-1 co-infection model (2.1)–(2.6) with the solutions of the HTLV-2 mono-infection system.
˙E=ξ−ζE−β2EH, | (5.6) |
˙H=β2EH−a2H. | (5.7) |
We select the values β1=0.007 and β2=0.003 in addition to use the subsequent initial condition:
IP.6:U(0)=200, Y(0)=1.5, V(0)=2.5, E(0)=15, H(0)=100, W(0)=20. |
Solutions of the two systems (2.1)–(2.6) and (5.6)–(5.7) that are illustrated in Figure 10. It can be seen the numbers of uninfected CD8+T cells in both systems gradually tend to the same values. The numbers of HTLV-2-infected CD8+ T cells are more prevalent in HTLV-2 and HIV-1 co-infection patients than in HTLV-2 mono-infection patients. Nevertheless, in the context of HIV-1 infection, the emergence of malignant CD8+T-lymphoproliferative diseases appears to be feasible. It is anticipated that people who are co-infected with HTLV-2 and HIV-1 may experience a greater occurrence of HTLV-2-related T-cell malignant diseases [1]. This may be an explanation for why the number of HTLV-2-infected CD8+T cells is higher in patients with co-infection with HIV-1 compared to those without it as shown in Figure 10(b).
HTLV-2 and HIV-1 are both retroviruses that target different immune cells–HTLV-2 infects CD8+ T cells, while HIV-1 primarily targets CD4+ T cells. Several studies have documented cases of patients co-infected with HTLV-2 and HIV-1. To optimize treatment strategies for such coinfections, mathematical models have been developed to better understand the interactions between these viruses and their effects on the immune system. These models help in predicting disease progression and evaluating potential therapeutic approaches. In this article, we propose a new mathematical model that describes the co-dynamics of HIV-1 and HTLV-2 in vivo. The model was given as a system of nonlinear ODEs, which describes the interactions between six compartments: uninfected CD4+ T cells, HIV-1-infected CD4+ T cells, HIV-1 particles, uninfected CD8+ T cells, HTLV-2-infected CD8+ T cells, and HIV-1-specific B cells. Initially, we demonstrated that the model's solutions are bounded and non-negative. Additionally, we identified six equilibrium points, with their existence and stability conditions described in terms of seven threshold parameters. A summary of all the equilibria presented in our model is given as follows:
● Infection-free equilibrium, Δ0, which usually exists. When R1≤1 and R2≤1, Δ0 is GAS. HTLV-2 and HIV-1 will be eradicated as a consequence of this. From a control perspective, making R1≤1 and R2≤1 would be an effective strategy. Controlling these parameters through the effectiveness of antiviral drugs. For physicians and researchers, curing patients of chronic viral co-infections such as HTLV2 and HIV-1 is a top priority. Effective antiviral medication can stop viruses from replicating and lower R1 and R2 to less than one. There is no specific antiviral treatment approved for HTLV-2. Unlike HIV-1, where antiretroviral therapy (ART) is well-established.
● HTLV-2 mono-infection equilibrium, Δ1, exists if R1>1 and is GAS if R4≤1. An individual who is infected with only HTLV-2 is the outcome illustrated in this case.
● HIV-1 mono-infection equilibrium in the absence of HIV-1-specific B-cell response, Δ2 is presented if R2>1 and is GAS if R6≤1 and R7≤1. This case illustrates the consequences that ensue when an individual acquires only HIV-1 infection in the absence of HIV-1-specific B-cell response. This could be due to the body's low concentration of HIV-1 particles (i.e., V≤μ/ς), which could not be sufficient to elicit an immune response.
● HIV-1 mono-infection equilibrium with an active HIV-1-specific B-cell response, Δ3 exists when R3>1 and is GAS when R5≤1. This case study the outcomes that occur when an individual is only infected with HIV-1 and exhibits an active HIV-1-specific B-cell response. The body contains enough free HIV-1 particles (i.e., V>μ/ς) in this case to activate the immune system.
● HTLV-2 and HIV-1 co-infection equilibrium in the absence of HIV-1-specific B-cell response, Δ4, exists when R4>1 and R5>1. Moreover, Δ4 is GAS if R7≤1. In this case, an individual is co-infected with HTLV-2 and HIV-1, but without HIV-1-specific B-cell response.
● HTLV-2 and HIV-1 co-infection equilibrium with an active HIV-1-specific B-cell response, Δ5 exists and is GAS if R6>1 and R7>1. A person in this instance is co-infected with HTLV-2 and HIV-1 and has an active HIV-1-specific B-cell response. This instance demonstrates that B-cell activation is a crucial factor in infection control.
The global asymptotic stability of all equilibria was established by constructing appropriate Lyapunov functions and utilizing the Lyapunov-LaSalle asymptotic stability theorem. We have provided numerical simulations to demonstrate the validity and robustness of our theoretical findings. We observed that the numerical and analytical results align closely, demonstrating consistency between the two approaches. The influence of HIV-1-specific B-cell response on the dynamics of HTLV-2 and HIV-1 co-infection was presented. We demonstrated that increasing the stimulation rate of the HIV-1-specific B-cell response in HTLV-2 and HIV-1 co-infection enhances the concentration of CD4+ T cells and reduces the level of free HIV-1 particles, thereby boosting the immune system's overall effectiveness. Therefore, HIV-1-specific B-cell response plays the role of controlling and suppressing HIV-1 progression. We compared between HIV-1 or HTLV-2 mono-infection with HTLV-2 and HIV-1 co-infection, separately. We observed that patients with HIV-1 who are also infected with HTLV-2 had larger levels of HIV-1-specific B cells and lower levels of uninfected CD8+ T cells and HIV-1. Furthermore, we note that following co-infection, the behavior dynamics of uninfected CD4+ T cells did not alter. These outcomes agree with prior research (see [70]), which indicates that co-infection with HTLV-2 is associated with the capacity to regulate HIV-1 replication, a greater likelihood of survival, and a postponed start of AIDS. However, co-infected patients with HTLV-2 and HIV-1 may experience a greater occurrence of HTLV-2-related T-cell malignant diseases.
When comparing HTLV-1 and HIV-1 co-infection and HTLV-2 and HIV-1 co-infection, studies such as [10] report that HTLV-1 co-infection can worsen the clinical course of HIV-1, accelerating progression to AIDS and complicating treatment. In contrast, HIV-1 and HTLV-2 co-infection appears to be more benign, showing a neutral or even protective association, with delayed AIDS progression, longer survival, and reduced mortality rates [71].
The main limitation of our study is the inability to estimate the model's parameter values using real data. This is due to several factors: Comprehensive data on HTLV-2 and HIV-1 co-infection is scarce, even though some data exists for patients with single HTLV-2 or HIV-1 infections. Moreover, comparing our results to a limited number of available studies may not yield reliable conclusions. Furthermore, obtaining real patient data for HTLV-2 and HIV-1 co-infection remains challenging.
This study could be extended by: (ⅰ) Employing real data to accurately estimate parameter values, (ⅱ) accounting for viral mutation, (ⅲ) developing the model using fractional differential equations to explore the effects of memory on viral co-dynamics, and (ⅳ) integrating reaction-diffusion dynamics and stochastic interactions. Additionally, the model can be treated as a nonlinear control system, where antiviral drug efficacy functions as the control input. Then, one can focus on designing optimal control strategies based on this framework. These research areas require further investigation, and we reserve them for future studies.
The authors declare they have not used Artificial Intelligence (AI) tools in the creation of this article.
This Project was funded by the Deanship of Scientific Research (DSR) at King Abdulaziz University, Jeddah, Saudi Arabia, under grant No. (GPIP-283-130-2024). The authors, therefore, acknowledge with thanks DSR for technical and financial support.
The authors declare there is no conflicts of interest.
[1] |
A. Araujo, N. Sheehy, H. Takahashi, W. W. Hall, Concomitant Infections with Human Immunodeficiency Virus Type 1 and Human T-Lymphotropic Virus Types 1 and 2, Polymicrobial Diseases, (2002), 75–97. https://doi.org/10.1128/9781555817947.ch5 doi: 10.1128/9781555817947.ch5
![]() |
[2] | UNAIDS: Global HIV & AIDS statistics-Fact sheet, 2024. Available from: https://www.unaids.org/sites/default/files/media_asset/UNAIDS_FactSheet_en.pdf. |
[3] |
J. Esbjörnsson, M. Jansson, S. Jespersen, F. Månsson, B. L. Hønge, J. Lindman, et al., HIV-2 as a model to identify a functional HIV cure, AIDS Research and Therapy, 16 (2019), 24. https://doi.org/10.1186/s12981-019-0239-x doi: 10.1186/s12981-019-0239-x
![]() |
[4] | D. Wodarz, D. N. Levy, Human immunodeficiency virus evolution towards reduced replicative fitness in vivo and the development of AIDS, in Proceedings of the Royal Society B: Biological Sciences, 274 (2007), 2481–2491. https://doi.org/10.1098/rspb.2007.0413 |
[5] |
M. T. Raza, S. Mizan, F. Yasmin, A. S. Akash, S. M. Shahik, Epitope-based universal vaccine for Human T-lymphotropic virus-1(HTLV-1), PLoS One, 16 (2021), e0248001. https://doi.org/10.1371/journal.pone.0248001 doi: 10.1371/journal.pone.0248001
![]() |
[6] |
A. Araujo, W. W. Hall, Human T-lymphotropic virus type Ⅱ and neurological disease, Ann. Neurol., 56 (2004), 10–19. https://doi.org/10.1002/ana.20126 doi: 10.1002/ana.20126
![]() |
[7] |
A. Gessain, O. Cassar, Epidemiological aspects and world distribution of HTLV-1 infection, Front. Microbiol., 3 (2012), 388. https://doi.org/10.3389/fmicb.2012.00388 doi: 10.3389/fmicb.2012.00388
![]() |
[8] |
E. L. Murphy, O. Cassar, A. Gessain, Estimating the number of HTLV-2 infected persons in the world, Retrovirology, 12 (2015), O5. https://doi.org/10.1186/1742-4690-12-S1-O5 doi: 10.1186/1742-4690-12-S1-O5
![]() |
[9] |
K. S. Jones, K. Fugo, C. Petrow-Sadowski, Y. Huang, D. C. Bertolette, I. Lisinski, et al., Human T-cell leukemia virus type 1 (HTLV-1) and HTLV-2 use different receptor complexes to enter T cells, J. Virol., 80 (2006), 8291–8302. https://doi.org/10.1128/jvi.00389-06 doi: 10.1128/jvi.00389-06
![]() |
[10] |
M. P. Martinez, J. Al-Saleem, P. L. Green, Comparative virology of HTLV-1 and HTLV-2, Retrovirology, 16 (2019), 1–12. https://doi.org/10.1186/s12977-019-0483-0 doi: 10.1186/s12977-019-0483-0
![]() |
[11] |
M. A. Beilke, Retroviral coinfections: HIV and HTLV: Taking stock of more than a quarter century of research, AIDS Res. Hum. Retroviruses, 28 (2012), 139–147. https://doi.org/10.1089/aid.2011.0342 doi: 10.1089/aid.2011.0342
![]() |
[12] |
D. Dhasmana, G. P. Taylor, Human T-lymphotropic virus/HIV co-infection: A clinical review, Curr. Opin. Infect. Dis., 27 (2014), 16–28. https://doi.org/10.1097/QCO.0000000000000027 doi: 10.1097/QCO.0000000000000027
![]() |
[13] |
C. C. Koech, R. M. Lwembe, E. O. Odari, N. L. M. Budambula, Prevalence and associated risk factors of HTLV/HIV co-infection among people who inject drugs (PWIDs): A review, J. Hum. Virol. Retrovirol., 6 (2018), 00188. https://doi.org/10.15406/jhvrv.2018.06.00188 doi: 10.15406/jhvrv.2018.06.00188
![]() |
[14] |
A. Caterino-de-Araujo, Sex, age, and risk group variations among individuals infected with HIV, HTLV-1, and HTLV-2: Review of data records (1983–2017) from a public health laboratory in São Paulo, Brazil, Sexes, 4 (2023), 638–655. https://doi.org/10.3390/sexes4040041 doi: 10.3390/sexes4040041
![]() |
[15] |
M. A. Nowak, C. R. M. Bangham, Population dynamics of immune responses to persistent viruses, Science, 272 (1996), 74–79. https://doi.org/10.1126/science.272.5258.74 doi: 10.1126/science.272.5258.74
![]() |
[16] |
D. Wodarz, R. M. May, M. A. Nowak, The role of antigen-independent persistence of memory cytotoxic T lymphocytes, Int. Immunol., 12 (2000), 467–477. https://doi.org/10.1093/intimm/12.4.467 doi: 10.1093/intimm/12.4.467
![]() |
[17] |
C. Jiang, H. Kong, G. Zhang, K. Wang, Global properties of a virus dynamics model with self-proliferation of CTLs, Math. Appl. Sci. Eng., 2 (2021), 123–133. https://doi.org/10.5206/mase/13822 doi: 10.5206/mase/13822
![]() |
[18] |
Y. Yang, R. Xu, Mathematical analysis of a delayed HIV infection model with saturated CTL immune response and immune impairment, J. Appl. Math. Comput., 68 (2022), 2365–2380. https://doi.org/10.1007/s12190-021-01621-x doi: 10.1007/s12190-021-01621-x
![]() |
[19] |
C. Chen, Y. Zhou, Dynamic analysis of HIV model with a general incidence, CTLs immune response and intracellular delays, Math. Comput. Simul., 212 (2023), 159–181. https://doi.org/10.1016/j.matcom.2023.04.029 doi: 10.1016/j.matcom.2023.04.029
![]() |
[20] |
C. Lv, L. Huang, Z. Yuan, Global stability for an HIV-1 infection model with Beddington-DeAngelis incidence rate and CTL immune response, Commun. Nonlinear Sci. Numer. Simul., 19 (2014), 121–127. https://doi.org/10.1016/j.cnsns.2013.06.025 doi: 10.1016/j.cnsns.2013.06.025
![]() |
[21] |
A. Murase, T. Sasaki, T. Kajiwara, Stability analysis of pathogen-immune interaction dynamics, J. Math. Biol., 51 (2005), 247–267. https://doi.org/10.1007/s00285-005-0321-y doi: 10.1007/s00285-005-0321-y
![]() |
[22] |
S. Wang, D. Zou, Global stability of in host viral models with humoral immunity and intracellular delays, Appl. Math. Modell., 36 (2012), 1313–1322. https://doi.org/10.1016/j.apm.2011.07.086 doi: 10.1016/j.apm.2011.07.086
![]() |
[23] |
T. Kajiwara, T. Sasaki, Y. Otani, Global stability for an age-structured multistrain virus dynamics model with humoral immunity, J. Appl. Math. Comput., 62 (2020), 239–279. https://doi.org/10.1007/s12190-019-01283-w doi: 10.1007/s12190-019-01283-w
![]() |
[24] |
M. Dhar, S. Samaddar, P. Bhattacharya, Modeling the effect of non-cytolytic immune response on viral infection dynamics in the presence of humoral immunity, Nonlinear Dyn., 98 (2019), 637–655. https://doi.org/10.1007/s11071-019-05219-8 doi: 10.1007/s11071-019-05219-8
![]() |
[25] |
J. Lin, R. Xu, X. Tian, Threshold dynamics of an HIV-1 virus model with both virus-to-cell and cell-to-cell transmissions, intracellular delay, and humoral immunity, Appl. Math. Comput., 315 (2017), 516–530. https://doi.org/10.1016/j.amc.2017.08.004 doi: 10.1016/j.amc.2017.08.004
![]() |
[26] |
Z. She, X. Jiang, Threshold dynamics of a general delayed within-host viral infection model with humoral immunity and two modes of virus transmission, Discrete Contin. Dyn. Syst.-Ser. B, 26 (2021), 3835–3861. https://doi.org/10.3934/dcdsb.2020259 doi: 10.3934/dcdsb.2020259
![]() |
[27] |
D. Wodarz, Hepatitis C virus dynamics and pathology: The role of CTL and antibody responses, J. Gen. Virol., 84 (2003), 1743–1750. https://doi.org/10.1099/vir.0.19118-0 doi: 10.1099/vir.0.19118-0
![]() |
[28] |
Z. Zhang, Y. Chen, X. Wang, L. Rong, Dynamic analysis of a latent HIV infection model with CTL immune and antibody responses, Int. J. Biomath., 17 (2024), 2350079. https://doi.org/10.1142/S1793524523500791 doi: 10.1142/S1793524523500791
![]() |
[29] |
J. Lin, R. Xu, X. Tian, Threshold dynamics of an HIV-1 model with both viral and cellular infections, cell-mediated and humoral immune responses, Math. Biosci. Eng., 16 (2018), 292–319. https://doi.org/10.3934/mbe.2019015 doi: 10.3934/mbe.2019015
![]() |
[30] |
T. Guo, Z. Qiu, L. Rong, Analysis of an HIV model with immune responses and cell-to-cell transmission, Bull. Malays. Math. Sci. Soc., 43 (2018), 581–607. https://doi.org/10.1007/s40840-018-0699-5 doi: 10.1007/s40840-018-0699-5
![]() |
[31] | M. A. Nowak, R. M. May, Virus Dynamics, Oxford University Press, New York, 2000. |
[32] |
R. Arnaout, M. Nowak, D. Wodarz, HIV-1 dynamics revisited: Biphasic decay by cytotoxic lymphocyte killing?, Proc. R. Soc. London. Ser. B: Biol. Sci., 267 (1450), 1347–1354. https://doi.org/10.1098/rspb.2000.1149 doi: 10.1098/rspb.2000.1149
![]() |
[33] |
B. J. Nath, K. Sadri, H. K. Sarmah, K. Hosseini, An optimal combination of antiretroviral treatment and immunotherapy for controlling HIV infection, Math. Comput. Simul., 217 (2024), 226–243. https://doi.org/10.1016/j.matcom.2023.10.012 doi: 10.1016/j.matcom.2023.10.012
![]() |
[34] |
D. Adak, N. Bairagi, Bifurcation analysis of a multidelayed HIV model in presence of immune response and understanding of in-host viral dynamics, Math. Methods Appl. Sci., 42 (2019), 4256–4272. https://doi.org/10.1002/mma.5645 doi: 10.1002/mma.5645
![]() |
[35] |
M. Dhar, S. Samaddar, P. Bhattacharya, Modeling the cell-to-cell transmission dynamics of viral infection under the exposure of non-cytolytic cure, J. Appl. Math. Comput., 65 (2021), 885–911. https://doi.org/10.1007/s12190-020-01420-w doi: 10.1007/s12190-020-01420-w
![]() |
[36] |
H. Miao, Z. Teng, C. Kang, A. Muhammadhaji, Stability analysis of a virus infection model with humoral immunity response and two time delays, Math. Methods Appl. Sci., 39 (2016), 3434–3449. https://doi.org/10.1002/mma.3790 doi: 10.1002/mma.3790
![]() |
[37] |
P. Dubey, U. S. Dubey, B. Dubey, Modeling the role of acquired immune response and antiretroviral therapy in the dynamics of HIV infection, Math. Comput. Simul., 144 (2018), 120–137. https://doi.org/10.1016/j.matcom.2017.07.006 doi: 10.1016/j.matcom.2017.07.006
![]() |
[38] |
A.P. Wang, M. Y. Li, Viral dynamics of HIV-1 with CTL immune response, Discrete Contin. Dyn. Syst.-Ser. B, 26 (2021), 2257–2272. https://doi.org/10.3934/dcdsb.2020212 doi: 10.3934/dcdsb.2020212
![]() |
[39] |
Z. Hu, J. Yang, Q. Li, S. Liang, D. Fan, Mathematical analysis of stability and Hopf bifurcation in a delayed HIV infection model with saturated immune response, Math. Methods Appl. Sci., 47 (2024), 9834–9857. https://doi.org/10.1002/mma.10097 doi: 10.1002/mma.10097
![]() |
[40] |
M. Tan, G. Lan, C. Wei, Dynamic analysis of HIV infection model with CTL immune response and cell-to-cell transmission, Appl. Math. Lett., 156 (2024), 109140. https://doi.org/10.1016/j.aml.2024.109140 doi: 10.1016/j.aml.2024.109140
![]() |
[41] |
X. Duan, S. Yuan, Global dynamics of an age-structured virus model with saturation effects, Math. Methods Appl. Sci., 40 (2017), 1851–1864. https://doi.org/10.1002/mma.4102 doi: 10.1002/mma.4102
![]() |
[42] |
E. Avila-Vales, Á. G. Pérez, Global properties of an age-structured virus model with saturated antibody-immune response, multi-target cells, and general incidence rate, Bol. Soc. Mat. Mex., 27 (2021), 26. https://doi.org/10.1007/s40590-021-00315-5 doi: 10.1007/s40590-021-00315-5
![]() |
[43] |
S. Chowdhury, J. K. Ghosh, U. Ghosh, Co-infection dynamics between HIV-HTLV-I disease with the effects of Cytotoxic T-lymphocytes, saturated incidence rate and study of optimal control, Math. Comput. Simul., 223 (2024), 195–218. https://doi.org/10.1016/j.matcom.2024.04.015 doi: 10.1016/j.matcom.2024.04.015
![]() |
[44] |
G. Doitsh, N. Galloway, X. Geng, Z. Yang, K. M. Monroe, O. Zepeda, et al., Cell death by pyroptosis drives CD4 T-cell depletion in HIV-1 infection, Nature, 505 (2014), 509–514. http://doi.org/10.1038/nature12940 doi: 10.1038/nature12940
![]() |
[45] |
W. Wang, W. Ma, Z. Feng, Complex dynamics of a time periodic nonlocal and time-delayed model of reaction-diffusion equations for modeling CD4+ T cells decline, J. Comput. Appl. Math., 367 (2020), 112430. http://doi.org/10.1016/j.cam.2019.112430 doi: 10.1016/j.cam.2019.112430
![]() |
[46] |
S. Wang, P. Hottz, M. Schechter, L. Rong, Modeling the slow CD4+ T cell decline in HIV-infected individuals, PLoS Comput. Biol., 11 (2015), e1004665. https://doi.org/10.1371/journal.pcbi.1004665 doi: 10.1371/journal.pcbi.1004665
![]() |
[47] |
W. Wang, T. Zhang, Caspase-1-mediated pyroptosis of the predominance for driving CD4+ T cells death: a nonlocal spatial mathematical model, Bull. Math. Biol., 80 (2018), 540–582. https://doi.org/10.1007/s11538-017-0389-8 doi: 10.1007/s11538-017-0389-8
![]() |
[48] |
W. Wang, Z. Feng, Global dynamics of a diffusive viral infection model with spatial heterogeneity, Nonlinear Anal. Real World Appl., 72 (2023), 103763. https://doi.org/10.1016/j.nonrwa.2022.10376 doi: 10.1016/j.nonrwa.2022.10376
![]() |
[49] |
W. Wang, X. Ren, W. Ma, X. Lai, New insights into pharmacologic inhibition of pyroptotic cell death by necrosulfonamide: A PDE model, Nonlinear Anal. Real World Appl., 56 (2020), 103173. https://doi.org/10.1016/j.nonrwa.2020.103173 doi: 10.1016/j.nonrwa.2020.103173
![]() |
[50] |
W. Wang, X. Ren, X. Wang, Spatial-temporal dynamics of a novel PDE model: Applications to pharmacologic inhibition of pyroptosis by necrosulfonamide, Commun. Nonlinear Sci. Numer. Simul., 103 (2021), 106025. https://doi.org/10.1016/j.cnsns.2021.106025 doi: 10.1016/j.cnsns.2021.106025
![]() |
[51] |
A. M. Elaiw, N. H. AlShamrani, A. D. Hobiny, Mathematical modeling of HIV/HTLV co-infection with CTL-mediated immunity, AIMS Math., 6 (2021), 1634–1676. https://doi.org/10.3934/math.2021098 doi: 10.3934/math.2021098
![]() |
[52] |
A. M. Elaiw, N. H. AlShamrani, Analysis of a within-host HIV/HTLV-I co-infection model with immunity, Virus Res., 295 (2021), 198204. https://doi.org/10.1016/j.virusres.2020.198204 doi: 10.1016/j.virusres.2020.198204
![]() |
[53] |
H. Yang, X. Li, W. Zhang, A stochastic HIV/HTLV-I co-infection model incorporating the aids-related cancer cells, Discrete Contin. Dyn. Syst.-Ser. B, 29 (2024), 702–730. https://doi.org/10.3934/dcdsb.2023110 doi: 10.3934/dcdsb.2023110
![]() |
[54] |
A. S. Perelson, D. E. Kirschner, R. De Boer, Dynamics of HIV-1 infection of CD4+ T cells, Math. Biosci., 114 (1993), 81–125. https://doi.org/10.1016/0025-5564(93)90043-a doi: 10.1016/0025-5564(93)90043-a
![]() |
[55] |
R. V. Culshaw, S. Ruan, A delay-differential equation model of HIV infection of CD4+T-cells, Math. Biosci., 165 (2000), 27–39. https://doi.org/10.1016/S0025-5564(00)00006-7 doi: 10.1016/S0025-5564(00)00006-7
![]() |
[56] |
M. M. Hadjiandreou, R. Conejeros, V. S. Vassiliadis, Towards a long-term model construction for the dynamic simulation of HIV-1 infection, Math. Biosci. Eng., 4 (2007), 489–504. https://doi.org/10.3934/mbe.2007.4.489 doi: 10.3934/mbe.2007.4.489
![]() |
[57] |
E. A. Hernandez-Vargas, R. H. Middleton, Modeling the three stages in HIV infection, J. Theor. Biol., 320 (2013), 33–40. https://doi.org/10.1016/j.jtbi.2012.11.028 doi: 10.1016/j.jtbi.2012.11.028
![]() |
[58] |
B. E. L. Boukari, N. Yousfi, A delay differential equation model of HIV infection, with therapy and CTL response, Bull. Math. Sci. Appl., 9 (2014), 53–68. https://doi.org/10.18052/www.scipress.com/BMSA.9.53 doi: 10.18052/www.scipress.com/BMSA.9.53
![]() |
[59] |
P. Ngina, R. W. Mbogo, L. S. Luboobi, HIV drug resistance: insights from mathematical modelling, Appl. Math. Modell., 75 (2019), 141–161. https://doi.org/10.1016/j.apm.2019.04.040 doi: 10.1016/j.apm.2019.04.040
![]() |
[60] |
B. Szomolay, E. M. Lungu, A mathematical model for the treatment of AIDS-related Kaposi's sarcoma, J. Biol. Syst., 22 (2014), 495–522. https://doi.org/10.1142/S0218339014500247 doi: 10.1142/S0218339014500247
![]() |
[61] | H. L. Smith, P. Waltman, The Theory of the Chemostat: Dynamics of Microbial Competition, Cambridge University Press, 1995. http://doi.org/10.1017/CBO9780511530043 |
[62] |
A. Korobeinikov, Global properties of basic virus dynamics models, Bull. Math. Biol., 66 (2004), 879–883. https://doi.org/10.1016/j.bulm.2004.02.001 doi: 10.1016/j.bulm.2004.02.001
![]() |
[63] | E. A. Barbashin, Introduction to the Theory of Stability, Wolters-Noordhoff, Groningen, 1970. https://doi.org/10.1007/978-1-4612-4046-4 |
[64] | J. P. LaSalle, The Stability of Dynamical Systems, SIAM, Philadelphia, 1976. https://doi.org/10.1137/1.9781611970432 |
[65] | A. M. Lyapunov, The General Problem of the Stability of Motion, Taylor & Francis, Ltd., London, 1992. https://doi.org/10.1080/00207179208934253 |
[66] | J. K. Hale, S. M. V. Lunel, Introduction to Functional Differential Equations, Springer-Verlag, New York, 1993. https://doi.org/10.1007/978-1-4612-4342-7 |
[67] |
C. Casoli, E. Vicenzi, A. Cimarelli, G. Magnani, P. Ciancianaini, E. Cattaneo, et al., HTLV-Ⅱ down-regulates HIV-1 replication in IL-2–stimulated primary PBMC of coinfected individuals through expression of MIP-1α, Blood, 95 (2000), 2760–2769. https://doi.org/10.1182/blood.V95.9.2760.009k04_2760_2769 doi: 10.1182/blood.V95.9.2760.009k04_2760_2769
![]() |
[68] |
A. Q. C. Araujo, Neurological aspects of HIV-1/HTLV-1 and HIV-1/HTLV-2 coinfection, Pathogens, 9 (2020), 250. https://doi.org/10.3390/pathogens9040250 doi: 10.3390/pathogens9040250
![]() |
[69] |
E. Pilotti, M. V. Bianchi, A. D. Maria, F. Bozzano, M. G. Romanelli, U. Bertazzoni, et al., HTLV-1/-2 and HIV-1 co-infections: retroviral interference on host immune status, Front. Microbiol., 4 (2013), 372. https://doi.org/10.3389/fmicb.2013.00372 doi: 10.3389/fmicb.2013.00372
![]() |
[70] |
M. Turci, E. Pilotti, P. Ronzi, G. Magnani, A. Boschini, S. G. Parisi, et al., Coinfection with HIV-1 and human T-Cell lymphotropic virus type Ⅱ in intravenous drug users is associated with delayed progression to AIDS, JAIDS J. Acquired Immune Defic. Syndr., 41 (2006), 100–106. https://doi.org/10.1097/01.qai.0000179426.04166.12 doi: 10.1097/01.qai.0000179426.04166.12
![]() |
[71] |
S. Bassani, M. López, C. Toro, V. Jimenez, J. M. Sempere, V. Soriano, et al., Influence of human T cell Lymphotropic virus type 2 coinfection on virological and immunological parameters in HIV type 1–infected patients, Clin. Infect. Dis., 44 (2007), 105–110. https://doi.org/10.1086/510076 doi: 10.1086/510076
![]() |
1. | Shirali Kadyrov, Farkhod Haydarov, Khudoyor Mamayusupov, Komil Mustayev, Endemic coexistence and competition of virus variants under partial cross-immunity, 2025, 33, 2688-1594, 1120, 10.3934/era.2025050 | |
2. | E. A. Almohaimeed, A. M. Elaiw, A. D. Hobiny, Modeling HTLV-1 and HTLV-2 co-infection dynamics, 2025, 10, 2473-6988, 5696, 10.3934/math.2025263 |
Symbol | Description | Value | Source |
U | Concentration of uninfected CD4+T cells | cells mm-3 | |
Y | Concentration of HIV-1-infected CD4+T cells | cells mm-3 | |
V | Concentration of HIV-1 particles | viruses mm-3 | |
E | Concentration of uninfected CD8+T cells | cells mm-3 | |
H | Concentration of HTLV-2-infected CD8+T cells | cells mm-3 | |
W | Concentration of HIV-1-specific B cells | cells mm-3 | |
λ | Production rate of uninfected CD4+T cells | 10 cells mm-3day-1 | [54,55] |
d | Death rate of uninfected CD4+T cells | 0.01 day-1 | [25,56] |
β1 | Infection rate of uninfected CD4+T cells by HIV-1 | varied | |
a1 | Death rate of HIV-1-infected CD4+T cells | 0.4 day-1 | [54] |
k1 | Production rate of HIV-1 | 38 viruses cells-1 day-1 | [56,57] |
c1 | Clearance rate of HIV-1 | 2.4 day-1 | [54,55,56] |
r | Neutralization rate of HIV-1 particles by B cells | 0.1 cells-1mm3 day-1 | |
ϕ | Killing rate of HIV-1-infected CD4+T cells | 0.01 cells -1 mm3 day-1 | [58] |
by CD8+T cells | |||
ξ | Production rate of uninfected CD8+T cells | 20 cells mm-3 day-1 | [59] |
π | Stimulation rate of uninfected CD8+T cells | 0.0000005 cells-1 mm3 day-1 | [59] |
0.2 cells-1 mm3 day-1 | [58] | ||
ζ | Death rate of uninfected CD8+T cells | 0.06 day-1 | [59] |
β2 | Infection rate of uninfected CD8+T cells by HTLV-2 | varied | |
a2 | Death rate of HTLV-2-infected CD8+T cells | 0.3 day-1 | Assumed |
ς | Stimulation rate of HIV-1-specific B cells | varied | |
μ | Decay rate of HIV-1-specific B cells | 0.24 day-1 | [60] |
Equilibrium | Existence condition | Stability condition |
D0=(U0,0,0,E0,0,0) | - | R1≤1 and R2≤1 |
D1=(U1,0,0,E1,H1,0) | R1>1 | R1>1 and R4≤1 |
D2=(U2,Y2,V2,E2,0,0) | R2>1 | R2>1, R6≤1, and R7≤1 |
D3=(U3,Y3,V3,E3,0,W3) | R3>1 | R3>1 and R5≤1 |
D4=(U4,Y4,V4,E4,H4,0) | R4>1 and R5>1 | R7≤1<R4 and R5>1 |
D5=(U5,Y5,V5,E5,H5,W5) | R6>1,R7>1 | R6>1 and R7>1 |
Circumstance | Equilibrium | Re(λi)<0,i=1,⋯,6 | Stability |
1 | Δ0=(1000,0,0,333.33,0,0) | (−5.13,−1.01,−0.24,−0.13,−0.06,−0.01) | stable |
2 | Δ0=(1000,0,0,333.33,0,0) | (−5.13,−1.01,0.37,−0.24,−0.06,−0.01) | unstable |
Δ1=(1000,0,0,150,36.67,0) | (−4.12,−0.24,−0.18,−0.07,−0.07,−0.01) | stable | |
3 | Δ0=(1000,0,0,333.33,0,0) | (−6.51,0.37,−0.24,−0.23,−0.06,−0.01) | unstable |
Δ2=(785.97,0.57,9.08,333.34,0,0) | (−6.13,−0.23,−0.15,−0.01,−0.01,−0.06) | stable | |
4 | Δ0=(1000,0,0,333.33,0,0) | (−6.51,0.37,−0.24,−0.23,−0.06,−0.01) | unstable |
Δ2=(785.97,0.57,9.08,333.34,0,0) | (−6.13,0.67,−0.23,−0.01,−0.01,−0.06) | unstable | |
Δ3=(932.84,0.18,2.4,333.33,0,4.48) | (−6.57,−0.01,−0.01,−0.23,−0.06,−0.01) | stable | |
5 | Δ0=(1000,0,0,333.33,0) | (−7.48,1.34,−0.24,−0.06,−0.01,0.01) | unstable |
Δ1=(1000,0,0,322.58,2.15,0) | (−7.41,1.39,−0.24,−0.05,−0.01,−0.01) | unstable | |
Δ2=(471.58,1.42,22.41,333.34,0,0) | (−6.14,−0.01,−0.01,−0.06,−0.02,0.01) | unstable | |
Δ4=(458,1.49,23.67,322.58,2.15,0) | (−6.03,−0.01,−0.01,−0.05,−0.01,−0.01) | stable | |
6 | Δ0=(1000,0,0,333.33,0,0) | (−7.48,1.34,−0.24,−0.06,−0.01,0.01) | unstable |
Δ1=(1000,0,0,322.58,2.15,0) | (−7.41,1.39,−0.24,−0.05,−0.01,−0.01) | unstable | |
Δ2=(471.58,1.42,22.41,333.34,0,0) | (−6.14,2,−0.01,−0.01,−0.06,0.01) | unstable | |
Δ3=(892.86,0.29,2.4,333.33,0,21.44) | (−8.24,−0.02,−0.02,−0.06,−0.01,0.01) | unstable | |
Δ4=(458,1.49,23.67,322.58,2.15,0) | (−6.03,2.13,−0.01,−0.01,−0.05,−0.01) | unstable | |
Δ5=(892.86,0.3,2.4,322.58,2.15,22.79) | (−8.27,−0.02,−0.02,−0.05,−0.01,−0.01) | stable |
Symbol | Description | Value | Source |
U | Concentration of uninfected CD4+T cells | cells mm-3 | |
Y | Concentration of HIV-1-infected CD4+T cells | cells mm-3 | |
V | Concentration of HIV-1 particles | viruses mm-3 | |
E | Concentration of uninfected CD8+T cells | cells mm-3 | |
H | Concentration of HTLV-2-infected CD8+T cells | cells mm-3 | |
W | Concentration of HIV-1-specific B cells | cells mm-3 | |
λ | Production rate of uninfected CD4+T cells | 10 cells mm-3day-1 | [54,55] |
d | Death rate of uninfected CD4+T cells | 0.01 day-1 | [25,56] |
β1 | Infection rate of uninfected CD4+T cells by HIV-1 | varied | |
a1 | Death rate of HIV-1-infected CD4+T cells | 0.4 day-1 | [54] |
k1 | Production rate of HIV-1 | 38 viruses cells-1 day-1 | [56,57] |
c1 | Clearance rate of HIV-1 | 2.4 day-1 | [54,55,56] |
r | Neutralization rate of HIV-1 particles by B cells | 0.1 cells-1mm3 day-1 | |
ϕ | Killing rate of HIV-1-infected CD4+T cells | 0.01 cells -1 mm3 day-1 | [58] |
by CD8+T cells | |||
ξ | Production rate of uninfected CD8+T cells | 20 cells mm-3 day-1 | [59] |
π | Stimulation rate of uninfected CD8+T cells | 0.0000005 cells-1 mm3 day-1 | [59] |
0.2 cells-1 mm3 day-1 | [58] | ||
ζ | Death rate of uninfected CD8+T cells | 0.06 day-1 | [59] |
β2 | Infection rate of uninfected CD8+T cells by HTLV-2 | varied | |
a2 | Death rate of HTLV-2-infected CD8+T cells | 0.3 day-1 | Assumed |
ς | Stimulation rate of HIV-1-specific B cells | varied | |
μ | Decay rate of HIV-1-specific B cells | 0.24 day-1 | [60] |
Equilibrium | Existence condition | Stability condition |
D0=(U0,0,0,E0,0,0) | - | R1≤1 and R2≤1 |
D1=(U1,0,0,E1,H1,0) | R1>1 | R1>1 and R4≤1 |
D2=(U2,Y2,V2,E2,0,0) | R2>1 | R2>1, R6≤1, and R7≤1 |
D3=(U3,Y3,V3,E3,0,W3) | R3>1 | R3>1 and R5≤1 |
D4=(U4,Y4,V4,E4,H4,0) | R4>1 and R5>1 | R7≤1<R4 and R5>1 |
D5=(U5,Y5,V5,E5,H5,W5) | R6>1,R7>1 | R6>1 and R7>1 |
Circumstance | Equilibrium | Re(λi)<0,i=1,⋯,6 | Stability |
1 | Δ0=(1000,0,0,333.33,0,0) | (−5.13,−1.01,−0.24,−0.13,−0.06,−0.01) | stable |
2 | Δ0=(1000,0,0,333.33,0,0) | (−5.13,−1.01,0.37,−0.24,−0.06,−0.01) | unstable |
Δ1=(1000,0,0,150,36.67,0) | (−4.12,−0.24,−0.18,−0.07,−0.07,−0.01) | stable | |
3 | Δ0=(1000,0,0,333.33,0,0) | (−6.51,0.37,−0.24,−0.23,−0.06,−0.01) | unstable |
Δ2=(785.97,0.57,9.08,333.34,0,0) | (−6.13,−0.23,−0.15,−0.01,−0.01,−0.06) | stable | |
4 | Δ0=(1000,0,0,333.33,0,0) | (−6.51,0.37,−0.24,−0.23,−0.06,−0.01) | unstable |
Δ2=(785.97,0.57,9.08,333.34,0,0) | (−6.13,0.67,−0.23,−0.01,−0.01,−0.06) | unstable | |
Δ3=(932.84,0.18,2.4,333.33,0,4.48) | (−6.57,−0.01,−0.01,−0.23,−0.06,−0.01) | stable | |
5 | Δ0=(1000,0,0,333.33,0) | (−7.48,1.34,−0.24,−0.06,−0.01,0.01) | unstable |
Δ1=(1000,0,0,322.58,2.15,0) | (−7.41,1.39,−0.24,−0.05,−0.01,−0.01) | unstable | |
Δ2=(471.58,1.42,22.41,333.34,0,0) | (−6.14,−0.01,−0.01,−0.06,−0.02,0.01) | unstable | |
Δ4=(458,1.49,23.67,322.58,2.15,0) | (−6.03,−0.01,−0.01,−0.05,−0.01,−0.01) | stable | |
6 | Δ0=(1000,0,0,333.33,0,0) | (−7.48,1.34,−0.24,−0.06,−0.01,0.01) | unstable |
Δ1=(1000,0,0,322.58,2.15,0) | (−7.41,1.39,−0.24,−0.05,−0.01,−0.01) | unstable | |
Δ2=(471.58,1.42,22.41,333.34,0,0) | (−6.14,2,−0.01,−0.01,−0.06,0.01) | unstable | |
Δ3=(892.86,0.29,2.4,333.33,0,21.44) | (−8.24,−0.02,−0.02,−0.06,−0.01,0.01) | unstable | |
Δ4=(458,1.49,23.67,322.58,2.15,0) | (−6.03,2.13,−0.01,−0.01,−0.05,−0.01) | unstable | |
Δ5=(892.86,0.3,2.4,322.58,2.15,22.79) | (−8.27,−0.02,−0.02,−0.05,−0.01,−0.01) | stable |