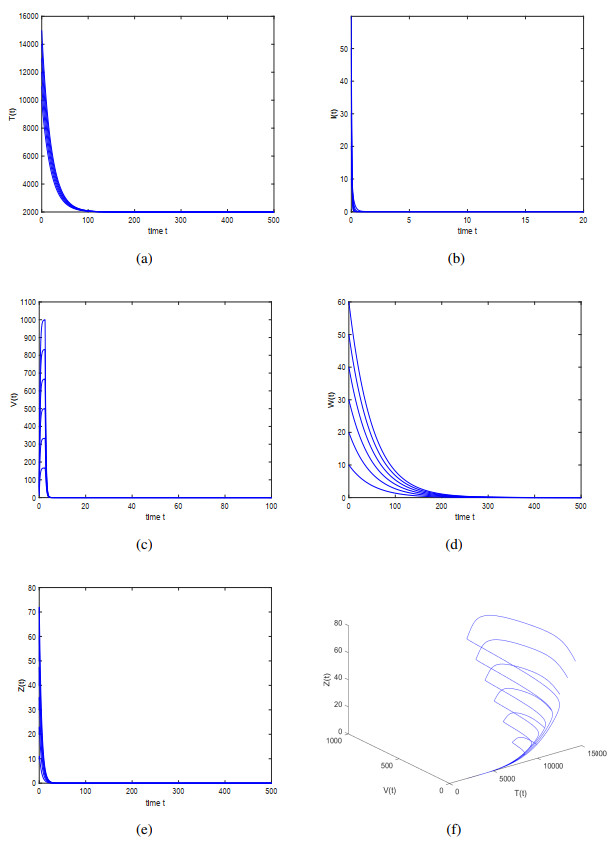
In this paper, we propose a new viral infection model by incorporating a new compartment for follicular dendritic cell (FDC), nonlinear incidence, CTL immune response, and two intracellular delays. The main purpose of the paper is to make an improvement and supplement to the global dynamics of the model proposed by Callaway and Perelson (2002), in which global stability has not been studied. The global stabilities of equilibria are established by constructing corresponding Lyapunov functionals in terms of two threshold parameters, R0 and R1. The obtained results imply that both nonlinear incidence and intracellular time delays have no impact on the stability of the model.
Citation: Yan Geng, Jinhu Xu. Modelling and analysis of a delayed viral infection model with follicular dendritic cell[J]. Electronic Research Archive, 2024, 32(8): 5127-5138. doi: 10.3934/era.2024236
[1] | Weijie Ding, Xiaochen Mao, Lei Qiao, Mingjie Guan, Minqiang Shao . Delay-induced instability and oscillations in a multiplex neural system with Fitzhugh-Nagumo networks. Electronic Research Archive, 2022, 30(3): 1075-1086. doi: 10.3934/era.2022057 |
[2] | A. M. Elaiw, E. A. Almohaimeed, A. D. Hobiny . Modeling the co-infection of HTLV-2 and HIV-1 in vivo. Electronic Research Archive, 2024, 32(11): 6032-6071. doi: 10.3934/era.2024280 |
[3] | Songbai Guo, Xin Yang, Zuohuan Zheng . Global dynamics of a time-delayed malaria model with asymptomatic infections and standard incidence rate. Electronic Research Archive, 2023, 31(6): 3534-3551. doi: 10.3934/era.2023179 |
[4] | Xudong Hai, Chengxu Chen, Qingyun Wang, Xiaowei Ding, Zhaoying Ye, Yongguang Yu . Effect of time-varying delays' dynamic characteristics on the stability of Hopfield neural networks. Electronic Research Archive, 2025, 33(3): 1207-1230. doi: 10.3934/era.2025054 |
[5] | Fang Yan, Changyong Dai, Haihong Liu . Oscillatory dynamics of p53 pathway in etoposide sensitive and resistant cell lines. Electronic Research Archive, 2022, 30(6): 2075-2108. doi: 10.3934/era.2022105 |
[6] | Dongbao Jia, Zhongxun Xu, Yichen Wang, Rui Ma, Wenzheng Jiang, Yalong Qian, Qianjin Wang, Weixiang Xu . Application of intelligent time series prediction method to dew point forecast. Electronic Research Archive, 2023, 31(5): 2878-2899. doi: 10.3934/era.2023145 |
[7] | Ziang Chen, Chunguang Dai, Lei Shi, Gaofang Chen, Peng Wu, Liping Wang . Reaction-diffusion model of HIV infection of two target cells under optimal control strategy. Electronic Research Archive, 2024, 32(6): 4129-4163. doi: 10.3934/era.2024186 |
[8] | Yuan Xue, Jinli Xu, Yuting Ding . Dynamics analysis of a diffusional immunosuppressive infection model with Beddington-DeAngelis functional response. Electronic Research Archive, 2023, 31(10): 6071-6088. doi: 10.3934/era.2023309 |
[9] | Xin Du, Quansheng Liu, Yuanhong Bi . Bifurcation analysis of a two–dimensional p53 gene regulatory network without and with time delay. Electronic Research Archive, 2024, 32(1): 293-316. doi: 10.3934/era.2024014 |
[10] | Yang Yang, Yun-Rui Yang, Xin-Jun Jiao . Traveling waves for a nonlocal dispersal SIR model equipped delay and generalized incidence. Electronic Research Archive, 2020, 28(1): 1-13. doi: 10.3934/era.2020001 |
In this paper, we propose a new viral infection model by incorporating a new compartment for follicular dendritic cell (FDC), nonlinear incidence, CTL immune response, and two intracellular delays. The main purpose of the paper is to make an improvement and supplement to the global dynamics of the model proposed by Callaway and Perelson (2002), in which global stability has not been studied. The global stabilities of equilibria are established by constructing corresponding Lyapunov functionals in terms of two threshold parameters, R0 and R1. The obtained results imply that both nonlinear incidence and intracellular time delays have no impact on the stability of the model.
Samples cannot always be taken frequently from patients, or detection techniques of the virus may not be accurate, and testing specific hypotheses based on clinical statistic data is a challengeable task, which justifies the key role played by mathematical models in this area. Mathematical models have been proposed to understand the in vivo infection dynamics of viruses. Particularly, theses within-host viral infection models were used to describe the viral infection process, estimate some key parameters, and provide support for research and development of antiviral drugs [1,2,3,4]. The classical viral dynamics can be described by the differential equations of three compartments: the uninfected target cells, the infected cells, and the free virus. Sometimes, a fourth compartment corresponding to the immune response was introduced into the model [5,6,7,8]. One of the main immune responses is the cytotoxic T-lymphocyte cells (CTLs), which play a crucial role in antiviral defense by attacking virus-infected cells in most virus infections. A basic and simple model with CTL immune response was proposed to explore the interaction between the CTLs and infected cells [5]. Then many kinds of models with immune response were further developed to investigate the impact of immune response on the dynamics of virus infection [6,7,8] and references therein.
Though antiretroviral therapy can effectively suppress viral replication to a low level, it cannot eradicate the virus permanently. An reliable and possible explanation is that there exists a viral reservoir, which is a possible impediment to virus eradication. Currently, researchers have shown that follicular dendritic cells (FDC) reside in secondary lymphoid organs known as germinal centers and bind HIV-antibody complexes, thus creating an archive of infectious viruses that can perpetuate infection. As such, HIV trapped on FDC is thought to be a significant viral reservoir [9,10,11,12,13]. Even with antiretroviral therapy, the FDC can still provide a suitable microenvironment for ongoing infection and impair the B cell response [14,15,16,17]. It is thus important to consider this factor in viral infection models, and some earlier studies of FDC can be found in [9,10,11]. For example, Callaway and Perelson [11] proposed the following simple model with FDC to understand what the effect FDC could have on the steady state viral load:
{dTdt=λ−dT−(1−ε)kTV,dIdt=(1−ε)kTV−δI,dVdt=NδI−(c1+μ)V+αW,dWdt=μV−(α+c2)W, | (1.1) |
where T(t), I(t), V(t), and W(t) are the concentrations of uninfected target cells, infected cells, free viruses, and virus particles bound to FDC at time t, respectively. λ is a constant production rate of uninfected cells that die at a rate d. The uninfected cells are infected by free virus at a rate of (1−ε)kTV, where ε represents the efficacy of RT inhibitors. The infected cells produce virions at a rate NδI and die at a rate δ, where N is the number of virions produced by an infected cell during its life span (burst size). The virions are cleared at a rate of c1. FDC binds free virus at rate μ, bound virus dissociates from FDC at rate α, and bound virus is cleared at rate c2.
In addition, it needs to go through a period between initial viral entry into a cell and subsequent viral production, and a maturation time is necessary for the released virions before they become infectious. Moreover, as pointed out by Ciup et al. [18], allowing for time delays in the models better predicts viral load data when compared to models without delays. Thus taking time delays into account makes the models more realistic. Then motivated by [11], a general viral infection model with two intracellular time delays and CTL immune response takes as follows:
{dTdt=λ−dT−(1−ε)kTf(V),dIdt=(1−ε)kT(t−τ1)f(V(t−τ1))−δI−rIZ,dVdt=NδI(t−τ2)−(c1+μ)V+αW,dWdt=μV−(α+c2)W,dZdt=pIZ−qZ, | (1.2) |
where Z(t) be the concentrations of CTLs at time t. The uninfected cells are infected by free virus at a rate of (1−ε)kTf(V). The infected cells are cleared by CTLs at a rate of rIZ. The CTLs are proliferated at a rate pIZ and decay at a rate q. τ1 represents the time period from being infected to becoming productive infected cells, and τ2 represents the time necessary for the newly produced virions to become mature. Throughout this paper, we assume that the incidence function f(V) satisfies the following conditions:
f(0)=0,f′(V)>0,f″(V)≤0. | (1.3) |
Based on condition (1.3), it follows that
f′(V)V≤f(V)≤f′(0)V,forV≥0. | (1.4) |
The initial conditions for model (1.2) are
T(θ)=φ1(θ),I(θ)=φ2(θ),V(θ)=φ3(θ),W(θ)=φ4(θ),Z(θ)=φ5(θ), | (1.5) |
where τ=max{τ1,τ2} and (φ1(θ),φ2(θ),φ3(θ),φ4(θ),φ5(θ))∈C([−τ,0],R5+). It is obviously that the model (1.2) includes the model [11] as an special case, in which the global dynamics that has not been investigated will be solved in this paper.
The rest of the paper is organized as follows: In Section 2, we derive the basic reproduction number for the viral infection, the basic reproduction number for the immune response, and the existence of equilibria. In Section 3, we prove that the global dynamics of the model are determined by the two threshold parameters. Simulations are carried out to validate the obtained results in Section 4. A summary and a discussion are presented in Section 5.
Some preliminary results will be presented in this part before analyzing the dynamics of model (1.2). According to literature [19], it is easy to show that the solutions of model (1.2) are non-negative. Moreover, let P1(t)=T+I(t+τ1)+rpZ(t+τ1), then we have
P′1=λ−dT−δI(t+τ1)−rpqZ(t+τ1)≤λ−m1P1(t), |
which implies that lim supt→+∞P1(t)≤λm1, where m1=min{d,δ,q}. Then, let P2(t)=V(t)+W(t), it follows that P′2(t)≤Nδλm1−m2P2(t), which leads to that lim supt→+∞P2(t)≤Nδλm1m2 with m2=min{c1,c2}. Therefore, the above analysis leads to the following result.
Lemma 2.1. The solutions (T(t),I(t),V(t),W(t),Z(t)) of model (1.2) with initial conditions (1.5) are non-negative and ultimately bounded.
It is easily seen that the model (1.2) possesses an infection-free equilibrium E0=(T0,0,0,0,0) with T0=λd. Define the basic reproduction number for viral infection R0 of model (1.2) as
R0=λ(1−ε)kf′(0)N(α+c2)d(c1α+c1c2+c2μ). |
When Z=0, an immune-inactivated equilibrium E1=(T1,I1,V1,W1,0) exists if T1,I1,V1,W1>0 satisfy
λ−dT1=(1−ε)kT1f(V1)=δI1=c1α+c1c2+c2μN(c2+α)V1=c1α+c1c2+c2μNμW1. | (2.1) |
Since T1,V1>0, it then follows from (2.1) that V1≤λN(c2+α)c1α+c1c2+c2μ=:˜V. In order to show the existence of E1, we define
F(V)=d(c1α+c1c2+c2μ)VkN(1−ε)(c2+α)f(V)+c1c2+c1α+c2μN(c2+α)V−λ. |
Together with (1.3) and (1.4), it follows that F′(V)>0. Moreover, for R0>1 a simple calculation gives
limV→0+F(V)=λ(1−R0)R0>0,F(˜V)=dλ(1−ε)kf(˜V)>0. |
Thus, if R0>1, there exists V1∈(0,˜V) such that F(V1)=0. This proves the existence of E1 when R0>1.
An immune-activated equilibrium E2=(T2,I2,V2,W2,Z2) exists if T2,I2,V2,W2,Z2>0 satisfy the following equilibrium equations:
T2=λd+(1−ε)kf(V2),I2=qp,V2=NδI2(c2+α)c1α+c1c2+c2μ,W2=μV2c2+α,Z2=1rI2[λ(1−ε)kf(V2)δI2(d+(1−ε)kf(V2))−1]=1rI2(R1−1). | (2.2) |
Thus, an immune-activated equilibrium E2 exists if and only if R1>1, where
R1=λ(1−ε)kf(V2)δI2(d+(1−ε)kf(V2))<λ(1−ε)kf′(0)N(c2+α)d(c1α+c1c2+c2μ)=R0. |
Lemma 2.2. Sign{T2−T1}=Sign{I1−I2}=Sign{V1−V2} =Sign{W1−W2}=Sign{R1−1}.
Proof. It follows from (2.1) and (2.2) that
d(T1−T2)=(1−ε)k(T2−T1)f(V2)+(1−ε)kT1(f(V2)−f(V1)). |
Then we have (d+(1−ε)kf(V2))(T1−T2)=(1−ε)kT1(f(V2)−f(V1)), which implies that Sign{T2−T1}=Sign{V1−V2}.
Moreover, it follows from (2.1) and (2.2) that Sign{V1−V2}=Sign{W1−W2} and
Nδ(I1−I2)=(c1+μ)(V1−V2)−α(W1−W2)=((c1+μ)(c2+α)μ−α)(W1−W2), |
we then have Sign{I1−I2}=Sign{V1−V2}=Sign{W1−W2}.
Then
R1−1=(1−ε)kT2f(V2)δI2−1=(1−ε)kT2f(V2)(c1+μ)V2−αW2−(1−ε)kT1f(V1)(c1+μ)V1−αW1=(1−ε)kN(c1+μ)(c2+α)−αμ{(T2−T1)f(V2)V2+T1(f(V2)V2−f(V1)V1)}. |
It follows from (1.4) that Sign{V1−V2}=Sign{f(V2)V2−f(V1)V1}, then we have Sign{R1−1}=Sign{V1−V2}=Sign{I1−I2}. This completes the proof.
In this part, the global stability of the equilibria E0, E1, and E2 will be established by constructing Lyapunov functionals, which are motivated by [20,21,22,23]. Here, we will use the function φ(x)=1+lnx−x, which satisfies φ(x)≤0 for x>0 and φ(x)=0 if and only if x=1.
Theorem 3.1. If R0≤1, then the infection-free equilibrium of E0 is globally asymptotically stable.
Proof. Let T(t),I(t),V(t),W(t),Z(t) be any arbitrary positive solution of model (1.2) and recall that T0=λd. Define a Lyapunov functional G1(t) as
G1(t)=φ(TT0)+I(t)+V(t)N+αWN(c2+α)+rpZ+∫tt−τ1(1−ε)kT(θ)f(V(θ))dθ+∫tt−τ2δI(θ)dθ. |
Computing the time derivative of G1(t) along the solution of model (1.2), which leads to
dG1dt=dT0(1−T0T)(1−TT0)+(1−ε)kT0f(V)+(αμN(c2+α)−c1+μN)V−qrpZ≤dT0(1−T0T)(1−TT0)+c1α+c1c2+c2μN(c2+α)(R0−1)V−qrpZ. |
Clearly, if R0≤1, then dG1dt≤0, for all T,I,V,W,Z≤0 and dG1dt=0 is satisfied if and only if T=T0,I=V=W=Z=0. Thus, the maximal compact invariant set in {G′1(t)=0} is the singleton {E0}. This proves the global stability of E0 by applying the LaSalle invariance principle [24].
Theorem 3.2. If R1<1<R0, then the immune-inactivated equilibrium E1 is globally asymptotically stable.
Proof. Define
G2(t)=φ(TT1)+φ(II1)+1Nφ(VV1)+αN(c2+α)φ(WW1)+rpZ+∫tt−τ2δI1φ(I(θ)I1)dθ+∫tt−τ1(1−ε)kT1f(V1)φ(T(θ)f(V(θ))T1f(V1))dθ. |
For convenience, let uτ=u(t−τ). Taking the time derivative of G1(t) along the solution of model (1.2) and using the equilibrium conditions (2.1) for E1, we have
dG2dt=dT1(1−TT1)(1−T1T)+(1−ε)kT1f(V1){3−T1T−VV1−V1Iτ2VI1−Tτ1I1f(Vτ1)T1If(V1)+f(V)f(V1)+lnTτ1f(Vτ1)Iτ2Tf(V)I}+rZ(I1−I2)+αW1N(2−V1WVW1−VW1V1W)=dT1(1−TT1)(1−T1T)+(1−ε)kT1f(V1){φ(T1T)+φ(Tτ1I1f(Vτ1)T1If(V1))+φ(V1Iτ2VI1)+φ(f(V1)Vf(V)V1)+(f(V)f(V1)−VV1)(1−f(V1)f(V))}+rZ(I1−I2)+αW1N(2−V1WVW1−VW1V1W). |
From Lemma 2.2, we have I1−I2<0. Moreover, (f(V)f(V1)−VV1)(1−f(V1)f(V))≤0 follows from (1.3) and (1.4). Then, we have dG2dt≤0 and dG2dt=0 if and only if T=T1,I=I1,V=V1,W=W1,Z=Z1. Thus, the largest compact invariant set of {G′2=0} is the singleton {E1}. Therefore, the global stability of E1 follows from the LaSalle invariance principle [24].
Theorem 3.3. If R1>1, then the immune-activated equilibrium E2 is globally asymptotically stable.
Proof. Define
G3(t)=φ(TT2)+φ(II2)+δ+rZ2Nδφ(VV2)+(δ+rZ2)αNδ(c2+α)φ(WW2)+rpφ(ZZ2)+∫tt−τ1(1−ε)kT2f(V2)φ(T(θ)f(V(θ))T2f(V2))dθ+∫tt−τ2(δ+rZ2)I2φ(I(θ)I2)dθ. |
Taking the time derivative of G3(t) along the solution of model (1.2) and using the equilibrium conditions (2.1) for E2, we have
dG3dt=dT2(1−TT2)(1−T2T)+(1−ε)kT2f(V2){3−T2T−VV2−V2Iτ2VI2−Tτ1I2f(Vτ1)T2If(V2)+f(V)f(V2)+lnTτ1f(Vτ1)Iτ2Tf(V)I}+αW2(δ+rZ2)Nδ(2−V2WVW2−VW2V2W)=dT2(1−TT2)(1−T2T)+(1−ε)kT2f(V2){φ(T2T)+φ(Tτ1I2f(Vτ1)T2If(V2))+φ(V2Iτ2VI2)+φ(f(V2)Vf(V)V2)+(f(V)f(V2)−VV2)(1−f(V2)f(V))}+αW2(δ+rZ2)Nδ(2−V2WVW2−VW2V2W). |
Similar to the proof of Theorem 3.2, we have dG3dt≤0 and dG3dt=0 if and only if T=T2,I=I2,V=V2,W=W2,Z=Z2. Thus, the largest compact invariant set of {G′2=0} is the singleton {E2}. Therefore, the global stability of E2 follows from the LaSalle invariance principle [24].
In this part, numerical simulations are carried out to validate the obtained results. Here, we select the function f(V)=V1+mV. Choosing a certain parameter value of the model (1.2), and a simple calculation show that R0=0.016<1, which implies that the infection-free equilibrium E0 is globally asymptotically stable and the infection dies out (see Figure 1). When choosing parameter values, we have R1=0.1894<1<R0=15.9999, then the immune-inactivated equilibrium E1 is globally asymptotically stable, which means the immune response is not enough to inhibit the infection and the virus dominates the infection process, as shown in Figure 2. Moreover, choosing parameter values such that R1=1.8936>1, which implies that the immune-activated equilibrium E2 is globally asymptotically stable, and then immune cells can coexist with viruses within the host, as shown in Figure 3.
In this paper, we studied an improved delayed viral infection model by incorporating nonlinear incidence and CTL immune response into the proposed model [11]. Thus, the model investigated here is including some existing literatures. We have shown that the model admits three equilibria E0, E1, and E2. Moreover, two threshold parameters, R0 and R1 are defined. By constructing corresponding Lyapunov functionals, we have demonstrated that the infection can be inhibited when R0≤1. The infection will persist and the immune response can not be activated when R1<1<R0. Both the viruses and immune cells can coexist and reach a steady state provided that R1>1. The obtained results reveal that both nonlinear incidence and intracellular delay cannot change the stability of the model. Besides, the obtained global dynamics of the model are a theoretical supplement to [11], which has not been considered.
Literatures reveals that cell-to-cell transmission is vital to the spread of viruses in vivo [25,26,27], and only virus-to-cell infection is taken into consideration in this paper, and whether the cell-to-cell infection can retain the stability or not is an interesting question. Besides, the time for activating the immune response is not included in the model, i.e., the immune time delay. How immune time delays impact the dynamical behavior of the model is also worth studying. We leave these for future work.
The authors declare they have not used Artificial Intelligence (AI) tools in the creation of this article.
This work was founded by National Natural Science Foundation of China (#11701445, #11971379, #12271431), by Natural Science Basic Research Programm in Shaanxi Province of China (#2022JM-042, #23IP114).
The authors declare there is no conflicts of interest.
[1] |
A. S. Perelson, D. E. Kirschner, R. D. Boer, Dynamics of HIV infection of CD4+ T cells, Math. Biosci., 114 (1993), 81–125. https://doi.org/10.1016/0025-5564(93)90043-A doi: 10.1016/0025-5564(93)90043-A
![]() |
[2] |
A. S. Perelson, P. W. Nelson, Mathematical analysis of HIV-1 dynamics in vivo, SIAM Rev., 41 (1999), 3–44. https://doi.org/10.1137/S0036144598335107 doi: 10.1137/S0036144598335107
![]() |
[3] |
A. S. Perelson, A. U. Neumann, M. Markowitz, J. M. Leonard, D. D. Ho, HIV-1 dynamics in vivo: virion clearance rate, infected cell life-span, and viral generation time, Science, 271 (1996), 1582–1586. https://doi.org/10.1126/science.271.5255.1582 doi: 10.1126/science.271.5255.1582
![]() |
[4] |
S. Bonhoeffer, R. M. May, G. M. Shaw, M. A. Nowak, Virus dynamics and drug therapy, Proc. Natl. Acad. Sci., 94 (1997), 6971–6976. https://doi.org/10.1073/pnas.94.13.6971 doi: 10.1073/pnas.94.13.6971
![]() |
[5] |
M. A. Nowak, C. R. M. Bangham, Population dynamics of immune responses to persistent viruses, Science, 272 (1996), 74–79. https://doi.org/10.1126/science.272.5258.74 doi: 10.1126/science.272.5258.74
![]() |
[6] |
X. Wang, Y. Tao, X. Song, Global stability of a virus dynamics model with Beddington-DeAngelis incidence tate and CTL immune response, Nonlinear Dynam., 66 (2011), 825–830. https://doi.org/10.1007/s11071-011-9954-0 doi: 10.1007/s11071-011-9954-0
![]() |
[7] |
H. Shu, L. Wang, J. Watmough, Global stability of a nonlinear viral infection model with infinitely distributed intracellular delays and CTL immune responses, SIAM J. Appl. Math., 73 (2013), 1280–1302. https://doi.org/10.1137/120896463 doi: 10.1137/120896463
![]() |
[8] |
S. S. Chen, C. Y. Cheng, Y. Takeuchi, Stability analysis in delayed within-host viral dynamics with both viral and cellular infections, J. Math. Anal. Appl., 442 (2016), 642–672. https://doi.org/10.1016/j.jmaa.2016.05.003 doi: 10.1016/j.jmaa.2016.05.003
![]() |
[9] |
W. S. Hlavacek, C. Wofsy, A. S. Perelson, Dissociation of HIV-1 from follicular dendritic cells during HAART: mathematical analysis, Proc. Nat. Acad. Sci., 96 (1999), 14681–14686. https://doi.org/10.1073/pnas.96.26.14681 doi: 10.1073/pnas.96.26.14681
![]() |
[10] |
W. S. Hlavacek, N. I. Stilianakis, D. W. Notermans, S. A. Danner, A. S. Perelson, Influence of follicular dendritic cells on decay of HIV during antiretroviral therapy, Proc. Nat. Acad. Sci., 97 (2000), 10966–10971. https://doi.org/10.1073/pnas.190065897 doi: 10.1073/pnas.190065897
![]() |
[11] |
D. S. Callaway, A. S. Perelson, HIV-1 infection and low steady state viral loads, Bull. Math. Biol., 64 (2002), 29–64. https://doi.org/10.1006/bulm.2001.0266 doi: 10.1006/bulm.2001.0266
![]() |
[12] |
T. C. Thacker, X. Zhou, J. D. Estes, Y. Jiang, B. F. Keele, T. S. Elton, et al., Follicular dendritic cells and human immunodeficiency virus type 1 transcription in CD4+ T cells, J. Virol., 83 (2009), 150–158. https://doi.org/10.1128/jvi.01652-08 doi: 10.1128/jvi.01652-08
![]() |
[13] |
E. L. Shikh, E. M. Mohey, Costantino Pitzalis. Follicular dendritic cells in health and disease, Front. Immunol., 3 (2012), 31755. https://doi.org/10.3389/fimmu.2012.00292 doi: 10.3389/fimmu.2012.00292
![]() |
[14] |
J. Zhang, A. S. Perelson, Contribution of follicular dendritic cells to persistent HIV viremia, J. Virol., 87 (2013), 7893–7901. https://doi.org/10.1128/jvi.00556-13 doi: 10.1128/jvi.00556-13
![]() |
[15] |
F. Sabri, A. Prados, R. Mu˜noz-Fernˊandez, R. Lantto, P. Fernandez-Rubio, A. Nasi, et al., Impaired B cells survival upon production of inflammatory cytokines by HIV-1 exposed follicular dendritic cells, Retrovirology, 13 (2016). https://doi.org/10.1186/s12977-016-0295-4 doi: 10.1186/s12977-016-0295-4
![]() |
[16] |
C. V. Fletcher, K. Staskus, S. W. Wietgrefe, et al., Persistent HIV-1 replication is associated with lower antiretroviral drug concentrations in lymphatic tissues, Proc. Natl. Acad. Sci., 111 (2014), 2307–2312. https://doi.org/10.1073/pnas.1318249111 doi: 10.1073/pnas.1318249111
![]() |
[17] |
M. T. Ollerton, E. A. Berger, E. Connick, G. F. Burton, HIV-1-specific chimeric antigen receptor T cells fail to recognize and eliminate the follicular dendritic cell HIV reservoir in vitro, J. Virol., 94 (2020). https://doi.org/10.1128/jvi.00190-20 doi: 10.1128/jvi.00190-20
![]() |
[18] |
M. S. Ciupe, B. L. Bivort, D. M. Bortz, P. W. Nelson, Estimating kinetic parameters from HIV primary infection data through the eyes of three different mathematical models, Math. Biosci., 200 (2006). https://doi.org/10.1016/j.mbs.2005.12.006 doi: 10.1016/j.mbs.2005.12.006
![]() |
[19] |
X. Yang, L. Chen, J Chen, Permanence and positive periodic solution for the single-species nonautonomous delay diffusive models, Comput. Math. Appl., 32 (1996), 109–116. https://doi.org/10.1016/0898-1221(96)00129-0 doi: 10.1016/0898-1221(96)00129-0
![]() |
[20] |
A. Korobeinikov, Global properties of basic virus dynamics models, Bull. Math. Biol., 66 (2004), 879–883. https://doi.org/10.1016/j.bulm.2004.02.001 doi: 10.1016/j.bulm.2004.02.001
![]() |
[21] |
C. C. McCluskey, Complete global stablity for an SIR epidemic model with delay-distributed or discrete, Nonlinear Anal. Real World Appl., 11 (2010), 55–59. https://doi.org/10.1016/j.nonrwa.2008.10.014 doi: 10.1016/j.nonrwa.2008.10.014
![]() |
[22] |
K. Hattaf, N. Yousfi, Global stability for reaction-diffusion equations in biology, Comput. Math. Appl., 66 (2013), 1488–1497. https://doi.org/10.1016/j.camwa.2013.08.023 doi: 10.1016/j.camwa.2013.08.023
![]() |
[23] |
K. Hattaf, N. Yousfi, A generalized HBV model with diffusion and two delays, Comput. Math. Appl., 69 (2015), 31–40. https://doi.org/10.1016/j.camwa.2014.11.010 doi: 10.1016/j.camwa.2014.11.010
![]() |
[24] | J. K. Hale, S. M. V. Lunel, Introduction to Functional Differential Equations, Springer-Verlag, New York, 1993. https://doi.org/10.1007/978-1-4612-4342-7 |
[25] |
S. Gummuluru, C. M. Kinsey, M. Emerman, An in vitro rapid-turnover assay for human immunodeficiency virus type 1 replication selects for cell-to-cell spread of virus, J. Virol., 74 (2000), 10882–10891. https://doi.org/10.1128/JVI.74.23.10882-10891.2000 doi: 10.1128/JVI.74.23.10882-10891.2000
![]() |
[26] |
C. R. M. Bangham, The immune control and cell-to-cell spread of human T-lymphotropic virus type 1, J. Gen. Virol., 84 (2003), 3177–3189. https://doi.org/10.1099/vir.0.19334-0 doi: 10.1099/vir.0.19334-0
![]() |
[27] |
A. Sigal, J. T. Kim, A. B. Balazs, E. Dekel, A. Mayo, R. Milo, et al., Cell-to-cell spread of HIV permits ongoing replication despite antiretroviral therapy, Nature, 477 (2011), 95–98. https://doi.org/10.1038/nature10347 doi: 10.1038/nature10347
![]() |