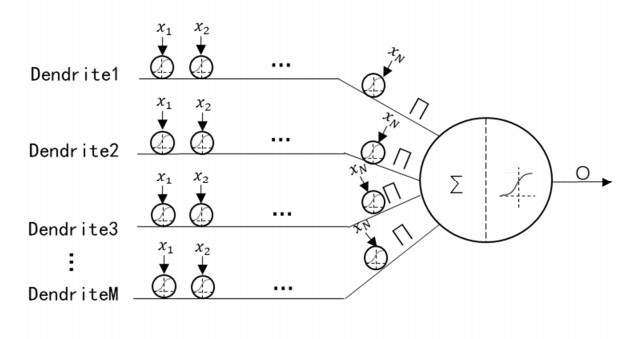
With the rapid development of meteorology, there requires a great need to better forecast dew point temperatures contributing to mild building surface and rational chemical control, while researches on time series forecasting barely catch the attention of meteorology. This paper would employ the seasonal-trend decomposition-based simplified dendritic neuron model (STLDNM*) to predict the dew point temperature. We utilize the seasonal-trend decomposition based on LOESS (STL) to extract three subseries from the original sequence, among which the residual part is considered as an input of an improved dendritic neuron model (DNM*). Then the back-propagation algorithm (BP) is used for training DNM* and the output is added to another two series disposed. Four datasets, which record dew points of four cities, along with eight algorithms are put into the experiments for comparison. Consequently, the combination of STL and simplified DNM achieves the best speed and accuracy.
Citation: Dongbao Jia, Zhongxun Xu, Yichen Wang, Rui Ma, Wenzheng Jiang, Yalong Qian, Qianjin Wang, Weixiang Xu. Application of intelligent time series prediction method to dew point forecast[J]. Electronic Research Archive, 2023, 31(5): 2878-2899. doi: 10.3934/era.2023145
[1] | Peng Lu, Yuchen He, Wenhui Li, Yuze Chen, Ru Kong, Teng Wang . An Informer-based multi-scale model that fuses memory factors and wavelet denoising for tidal prediction. Electronic Research Archive, 2025, 33(2): 697-724. doi: 10.3934/era.2025032 |
[2] | Xianfei Hui, Baiqing Sun, Indranil SenGupta, Yan Zhou, Hui Jiang . Stochastic volatility modeling of high-frequency CSI 300 index and dynamic jump prediction driven by machine learning. Electronic Research Archive, 2023, 31(3): 1365-1386. doi: 10.3934/era.2023070 |
[3] | Shaohu Zhang, Jianxiao Ma, Boshuo Geng, Hanbin Wang . Traffic flow prediction with a multi-dimensional feature input: A new method based on attention mechanisms. Electronic Research Archive, 2024, 32(2): 979-1002. doi: 10.3934/era.2024048 |
[4] | Gang Cheng, Changliang He . Analysis of bus travel characteristics and predictions of elderly passenger flow based on smart card data. Electronic Research Archive, 2022, 30(12): 4256-4276. doi: 10.3934/era.2022217 |
[5] | Miguel Arantes, Wenceslao González-Manteiga, Javier Torres, Alberto Pinto . Striking a balance: navigating the trade-offs between predictive accuracy and interpretability in machine learning models. Electronic Research Archive, 2025, 33(4): 2092-2117. doi: 10.3934/era.2025092 |
[6] | Xinyi Xu, Shaojuan Ma, Cheng Huang . Uncertainty prediction of wind speed based on improved multi-strategy hybrid models. Electronic Research Archive, 2025, 33(1): 294-326. doi: 10.3934/era.2025016 |
[7] | Nihar Patel, Nakul Vasani, Nilesh Kumar Jadav, Rajesh Gupta, Sudeep Tanwar, Zdzislaw Polkowski, Fayez Alqahtani, Amr Gafar . F-LSTM: Federated learning-based LSTM framework for cryptocurrency price prediction. Electronic Research Archive, 2023, 31(10): 6525-6551. doi: 10.3934/era.2023330 |
[8] | Boshuo Geng, Jianxiao Ma, Shaohu Zhang . Ensemble deep learning-based lane-changing behavior prediction of manually driven vehicles in mixed traffic environments. Electronic Research Archive, 2023, 31(10): 6216-6235. doi: 10.3934/era.2023315 |
[9] | Changhai Wang, Jiaxi Ren, Hui Liang . MSGraph: Modeling multi-scale K-line sequences with graph attention network for profitable indices recommendation. Electronic Research Archive, 2023, 31(5): 2626-2650. doi: 10.3934/era.2023133 |
[10] | Shengming Hu, Yongfei Lu, Xuanchi Liu, Cheng Huang, Zhou Wang, Lei Huang, Weihang Zhang, Xiaoyang Li . Stability prediction of circular sliding failure soil slopes based on a genetic algorithm optimization of random forest algorithm. Electronic Research Archive, 2024, 32(11): 6120-6139. doi: 10.3934/era.2024284 |
With the rapid development of meteorology, there requires a great need to better forecast dew point temperatures contributing to mild building surface and rational chemical control, while researches on time series forecasting barely catch the attention of meteorology. This paper would employ the seasonal-trend decomposition-based simplified dendritic neuron model (STLDNM*) to predict the dew point temperature. We utilize the seasonal-trend decomposition based on LOESS (STL) to extract three subseries from the original sequence, among which the residual part is considered as an input of an improved dendritic neuron model (DNM*). Then the back-propagation algorithm (BP) is used for training DNM* and the output is added to another two series disposed. Four datasets, which record dew points of four cities, along with eight algorithms are put into the experiments for comparison. Consequently, the combination of STL and simplified DNM achieves the best speed and accuracy.
Weather forecasting is of great importance to human beings [1,2]. It means to analyze various atmospheric data, including air pressure, surrounding temperature, wind intensity and direction, rainfall, and so on. This study impacts multiple fields such as industry, agriculture [3], disaster protection [4] and energy management [5]. For instance, weather forecasting can be used to ensure storage as well as transportation in order to increase quantity and improve quality in agricultural planting and production. Moreover, by accurate prediction of local wind power, hydropower, and solar energy, we can make a better use of these renewable resources under sustainable strategy [6]. Certainly, the most important purpose is to reduce unnecessary losses of properties and lives as much as possible [7].
Among various parameters of weather is there an important parameter called dew point. The dew point temperature reflects the humidity of the air and is widely used in industrial production and weather forecasting. Additionally, in the pneumatic system, researches on dew point are beneficial to improvement of equipment reliability and service life [8]. As current dew point studies mainly rely on instrumental measurement in specific areas, equation solving remains the first choice. Most of these expressions are differential equations, whose results are almost determined by initial conditions. The prediction quality is greatly influenced even if there are few deviations from true values.
Traditional method for weather forecasting is the numerical weather prediction (NWP) [9]. Its core idea is to construct a general circulation model following the physical principles and perform numerical analysis using computers. Therefore, weather forecasting has often been troubled due to its complicated computation, large amounts of changeable parameters and latent continuity among data. As is discussed in [10], although better improving measures are undertaken, these problems still exist and call the appearance of machine learning.
Machine learning (ML), along with the subsequent deep learning (DL) as a great idea has multiple contributions to weather forecasting. Compared with classical NWP methods which take various complex equations and complicated computation into account, all that machine learning needs are almost the input data, a transmitting model and a modification step [11]. Accompanied by the huge improvement of computing power and increasingly big-data processing technology, machine learning is playing a significant role in weather forecasting, especially for one-dimension time series, since these series are more suitable for data with certain regularity and duplicate procedures [12,13].
As for time series, there are a good many forecasting models. Time series are a series of values observed in a sequence. They are characteristic of nonlinearity, correlation, and volatility [14,15,16]. Researchers have been devoted to its prediction for a long time. At the beginning, they choose linear fitting methods such as the autoregressive moving average (ARIMA) [17,18], the exponential smoothing model [19] and the naive method [20]. But most of them suffer from their trouble of solving nonlinear problems. Nowadays, with the development of ML and DL, nonlinearity is well resolved. Meanwhile, researchers can obtain better forecasting results benefiting from them. These methods include support vector machine (SVM) [21], fuzzy-series analysis [22], artificial neural networks (ANN) [23,24,25,26] and their variants. But when predicting dew point temperatures using SVM and random forest alike [27], they are still troubled by complex mathematical models.
ANN is a representative researching framework among all the methods due to its data-driven and big-data mining features [28]. By putting existing data into the ANN model to train, the hidden connection among data can be found, thus obtaining appropriate results. Its another advantage is adaptability [29]. The ANN can be adaptive based on settings of some updating parameters, whose modification can significantly improve forecasting results. Although the ANN reveals a good many advantages, the model performs not so well in the time series prediction due to their changeable features and unstable volatility under the circumstance of weather.
Multilayer Perceptron (MLP) [30] develops on the basis of perceptron, which is a one-layer classifier for the linear separable case. Given enough hidden layers, it can achieve high-level results. Nevertheless, it lacks flexibility when addressing problems about time series.
Elman [31] is a kind of ANN adding a context unit to basic neural networks, which makes it a BP-like forward mechanism. The context unit receives previous state output as the input of hidden units. The Elman network boasts nonlinearity and time-memory feature, which is sensitive to historical data and help construct required models. However, it is troubled by computational costs and overfitting problems.
Support Vector Regression (SVR) [32] is an application to series prediction based on SVM, which utilizes kernel functions, sparse solution to decide the number of support vectors. Although the dimension of the input data has no impact on the computing complexity, the absence of preprocessing leads to biased forecasts.
AR is the abbreviation of Autoregressive Recurrent Network. DeepAR [33], which is involved in the study, creatively combines conceptions of time-variability, continuity, and dynamicity. Moreover, it introduces the probability distribution and improves the accuracy of prediction results. However, when confronted with multi-step prediction, time cost is an unbearable problem. And taking the mean values as final results may result in distortion as well.
Below are other state-of-the-art models with memorability for time series. As a classical variant of recurrent neural network (RNN), long short-term memory (LSTM) is widely used due to its ability to avoid vanishing gradients in RNN [34]. The modification is to introduce more layers so as to alleviate multiplication of past time units. Existing researches show that LSTM performs well in financial time series [35]. Furthermore, for simplification, researchers proposed the gate recurrent unit (GRU) with less layers [36]. A few years later, Jaeger put forward a novel network called echo state network (ESN) [37]. The ESN develops based on RNN as well and absorbs the nonlinear high-dimensional transformation feature as the SVM does. ESN has widely applied to temperature time series [38] but suffer from unstable probability distributions which is harmful to its prediction. In addition, the three memorable algorithms above are time-consuming when series become longer.
Putting the above factors aside, original series are chaotic and disorganized, which needs a preprocessing method to reduce their adverse effects on predicting. A classical method is empirical mode decomposition (EMD) [39]. It is a mathematical method to separate a sequence into the sum of multiple subseries based on the Fourier transformation. This approach has strong interpretability but has little connection with basic features of the original data.
Given the situation above, we need a model concerning both data preprocessing and close relation with features of series. In recent studies, instead of traditional mathematical EMD, a novel decomposing method [40] based on sequence features called STL is used. The seasonal and trend elements of the original series are successfully extracted using the STL, an abbreviation for the seasonal-trend decomposition procedure based on LOESS. Then the original problem is simplified and become a prediction of the third subseries. Here, a model called dendritic neuron model (DNM) [41] inspired by biological excitement is introduced. By deciding which branch is activated, researchers can construct a neural network with complex relations. With the use of sigmoid functions and multiplicated units, nonlinearity is well solved. Furthermore, as time series are basically one-dimension, the network can be further improved to DNM* by simplifying several functions of DNM. So far, a STLDNM* approach is constructed in order to predict time series.
Considering the huge significance of weather forecasting, and the urgent need to overcome difficulties mentioned above, we propose the STLDNM* model to make a solution. The model successfully combines the decomposition technology STL and the machine learning model DNM, and experiments demonstrate that the proposed model performs better in predicting instant weather conditions than other cutting-edge methods. It excels in both aspects of accuracy and efficiency, thus has a promising prospect in weather forecasting in the real world.
The main contributions are summarized as follows:
• To tackle the problem of predicting dew point series, a new combination of the decomposing method STL and the forecasting model DNM is proposed. This combination boasts both consistency and accuracy in the application of weather forecasting.
• The DNM model is improved to be DNM* which is more suitable for time series. DNM* is better on both stability and accuracy and has potentials for more chaotic series. BP is chosen as the optimization method due to its simplicity. In this paper, DNM* manages to predict dew points of different cities nationwide.
The STL is a method of decomposing time series data into three elements: the seasonal, trend and residual elements. Its formula is represented as follows:
Yk=Tk+Sk+Rk | (1) |
where Tk, Sk, Rk respectively denotes the trend, seasonal and residual element, for k∈[1,M], M is the length of time series.
LOESS, or locally weighted regression, is its full name. It aims to smooth the trend and seasonal parts of time series [42]. Two variables, xi and yi (i=1,2,…,n), are adopted to fit the regression curve, denoted by ˆh(x). Given x, fitting value of ˆh(x) is the smooth of y along the scale of the independent variable. Therefore, we shall deal with datasets with missing values.
ˆh(x) is obtained considering two cases. Assume a positive integer r, then we shall launch a discussion below:
1) If r≤n, we choose the r points from {xi} which stand closest to x. Then every point will be given a specific local weight according to how far it is from x. Below are two related equations. λr(x) denotes how far x is from the r th farthest point xi. W is tricube weight function. vi(x) is the local weight of xi :
W(t)={(1−t3)3,for 0≤t<10,for t≥1 | (2) |
vi(x)=W(|xi−x|λr(x)) | (3) |
2) If r>n, the definition of λr(x) becomes:
λr(x)=λn(x)rn | (4) |
From these formulae above, we can see that xi closest to x have the largest weights. As xi becomes farther from x, the weight decreases and eventually down to 0. This time xi is the r th farthest point. The polynomial function of ˆh(x) is at last computed at the point (xi,yi), then the locally-fitted value of x is ˆh(x).
The STL embeds two iterated loops: the inner loop and the outer loop. The seasonal element is updated on the detrending series in the inner loop. Then the resulting series are robustly weighted and run into the outer loop, the weight of which is computed in the outer loop. After that, the method implements multiple times of cycles as mentioned above.
In this paper, python programmer is adopted and packaged STL module is imported. The specific periodicity during one observation np is set to 365 and percentage of data used in LOESS regression is set to 0.6. Other pertinent parameters are set by the recommended settings.
Dendritic neuron model (DNM) is the simulation of the real neuron, which contains four connecting layers. The first and the last layer utilizes a sigmoid function as their processing tools. The second layer has a multiplication unit for received inputs. The third layer is an addition unit for inputs of the second layer. The four layers are denoted as the synaptic layer, the dendrite layer, the membrane layer and the soma layer, respectively in order. Figure 1 shows the DNM's organizational structure. The model is explained in detail below:
A synapse is the contact between neurons or inside the neuron itself. The original signal transfers from a presynaptic neuron to another one. By implementing the ionotropy, we determine whether the synapse is excitatory or inhibitory according to changes in the potential of postsynaptic. The synaptic layer serves to connects the i th (i=1,2,...,N) synaptic input to the j th (j=1,2,...,N) synaptic layer, shown as:
Yij=11+e−k(ωij xi−θij) | (5) |
where xi∈[0,1] denotes the input signals. Yij represents the output of the j th synaptic layer. And k represents a constant parameter. The weight ωij and threshold θij are connection parameters. Here four types of connection samples can be listed as follows:
• Case 1 (Constant 0 connection): under the condition of ωij<0<θij or 0<ωij<θij, the input xi changes from 0 to 1, whereas the output result Yij is roughly 0.
• Case 2 (Constant 1 connection): under the condition of θij<ωij<0 or θij<0<ωij, t the input xi changes from 0 to 1, whereas the output result Yij is roughly 1.
• Case 3 (Excitatory connection): under the condition of 0<θij<ωij, while the input xi transforms from 0 to 1, input xi and the output Yij are positively correlated.
• Case 4 (Inhibitory connection): under the condition of ωij<θij<0, the output Yij is inversely proportional to the input, while the input xi transforms from 0 to 1.
As xi is normalized before entering the model, only the conforming part is worth paying attention to. Figure 2 displays four cases above in detail.
In this layer, a multiplicative function is implemented on the outputs from the synaptic layer. Given the non-linearity of synapses transforming from 0 to 1, a multiplicative operation is introduced. This is equal to the logic AND operation, denoted in Figure 1 by the symbol ∏. Thus the output function for the j th dendrite is:
Zj=∏Ni=1Yij | (6) |
Similar to the second layer, this layer implements an addition function of the outputs on each dendrite. This function makes it equal to the logic OR operation under normalization between 0 and 1. Then, the summed result is sent to the fourth layer. The function of the membrane layer is:
V=∑Mj=1Zj | (7) |
At last, the soma layer utilizes another sigmoid function for the output V of the membrane layer. Once V exceeds its threshold, the neuron fires. Thus according to the following formula, the final result of the entire model is as follows:
O=11+e−ks(V−θs) | (8) |
The proposed model used in this paper is the combination of STL and DNM with their benefits. It makes the best adjustment for better forecasting results. Given the dataset, we implement the STL on it at first. Then, decomposed components are respectively processed. The seasonal element Sk remains unchanged. Tk is disposed using the least squares method (LSM). For the third part, the residual element Rk is regarded as the input of DNM (*), where the sign* means there is a simplification of the model. At last, the three elements are added after being processed. Figure 3 is a clear illustration of the STLDNM (*).
As the seasonal element Sk is an objective reflation of the original series, it tends to keep unchanged in this paper. As for Tk, a tricube continuous polynomial function is used to fit. Eq (9) describes the function:
Tk=pk3+qk2+uk+v | (9) |
where values of p,q,u,v are obtained using LSM. Hence, the first two elements of the decomposed results are processed in an easy way.
According to our previous studies, DNM is broadly applied due to its advantage of high efficiency and theoretical explanation. For better prediction results, here we utilize a simplified DNM meant for time series according to our previous study [43]. Figure 4 is the structure of DNM*. In particular, the summation at the middle is omitted and becomes a line with a weight of one. Additionally, the sigmoid function reduces to a linear expression. The other two layers remain unchanged. The details are as follows:
Yi=wixi+θi | (10) |
Z=∏Ni=1Yi | (11) |
V=Z | (12) |
O=11+e−kS(V−θs) | (13) |
Hence, the structure of proposed DNM* is greatly simplified. The performance of either fitting or calculation is significantly improved. By implementing a model of a single line, the DNM* performs a better effect for time series prediction.
As for time series, a phase space reconstruction (PSR) [44] is introduced to improve the experimenting results. It reconstructs the sequence into higher dimension. In this study, the embedding dimension and time delay are set to be 2 and 1 respectively. Thus, two adjacent values in the time series lay the foundation of later prediction. Generally, we can describe the input matrix as:
I=[x1x2…xM−2x2x3…xM−1] | (14) |
The corresponding target vector T is
T=[x3x4…xM] | (15) |
where M represents the length of the series, and each dataset is divided into two parts: training part and testing part. The former possesses 70% and the latter is 30%.
When dealing with problems about time series, it is most suitable to take back-propagation as a choice during the process of supervised learning. BP is a method using gradient descent learning to update and adjust the parameters of the neural network, whose purpose is to reduce the error at the terminal. We define the error by Eq (16):
Ep=12(Tp−Op)2 | (16) |
where the Tp is the teaching signal, while Op is the real output of the model. In BP algorithm, we decrease the error by correcting the values of wij and θij of the DNM model, their variations are shown as:
Δwij(t)=∑Pp=1∂Ep∂wij | (17) |
Δθij(t)=∑Pp=1∂Ep∂θij | (18) |
The parameters of wij and θij are updated as follows:
wij(k+1)=wij(k)−ηΔwij(k) | (19) |
θij(k+1)=θij(k)−ηΔθij(k) | (20) |
where η represents the learning rate of the BP, and k represents the learning iteration. η is set to be 0.1 in this paper. Then, the partial differentials of Ep with respect to wij and θij are obtained using the chain rule:
∂E∂wi=∂E∂O∙∂O∂V∙∂V∂Z∙∂Z∂Yi∙∂Yi∂wi | (21) |
∂E∂θi=∂E∂O∙∂O∂V∙∂V∂Z∙∂Z∂Yi∙∂Yi∂θi | (22) |
Repeating the application of the chain rule, a list of partial differentials are displayed below:
∂E∂O=O−T | (23) |
∂O∂V=kse−ks(V−θs)(1+e−ks(V−θs))2 | (24) |
∂V∂Z=1 | (25) |
∂Z∂Yi=∏Nl=1 and l≠iYi | (26) |
∂Yi∂wi=xi | (27) |
∂Yi∂θi=1 | (28) |
The STL decomposing method is meant to extract the seasonal and trend elements to maintain the main and unique features of the original series, and the whole disposing procedures are implemented by the almost best appropriate measures. The three subseries are comprehensively considered as the global reflection of the original data, with no part neglected. Besides, the method has also robust properties due to its relatively stable parameters and computing steps. Therefore, the STL is an excellent choice to operate weather-related time series forecasting.
The data sets of this paper are the yearly dew point temperatures of four cities from 2010 to 2015 as shown in Table 1. Cities chosen are four different regions from mainland. Hence, the predicting result of STLDNM* can be fully experimented. In view of the same length of these sequences, original series are filtered before implementing STL.
Weather data | Original Period | Chosen Sequence Length | Maximum Lyapunov Exponent |
Beijing's dew points (BJ) | 2010.01–2015.12 | 2187 | 0.6238 |
Shenyang's dew points (SY) | 2010.01–2015.12 | 2175 | 0.7034 |
Guangzhou's dew points (GZ) | 2010.01–2015.12 | 2183 | 0.1897 |
Shanghai's dew points (SY) | 2010.01–2015.12 | 2183 | 0.5258 |
In order to decide whether the original series can be short-termly forecasted or not, a maximum Lyapunov exponent (MLE) is computed before experiments [45]. This metric is obtained by the Wolf method. Given t0 (the beginning time) and yt0 (the reconstructed first phase point), we can get the minimum length between yt0 and its nearest neighbor. This distance is called L0. At a later time t1, the initial distance becomes L'0=‖yt1−yt0‖>ϵ, where ϵ is a positive threshold value. The L'0 will be replaced when another phase point y1t1 with L1=‖yt1−y1t1‖<L'0 appears. This calculation process is carried out until yt reaches yN. As a result, we can obtain MLE as:
λmax=1tm−t0∑mi=0lnL'iLi | (29) |
where m is the generation. Table 1 also gives MLEs of four cities. As the fourth column shows, all these series are characterized by chaotic features as their MLEs are positive and less than one.
As the four chosen datasets are long, the original series are initially shortened. We firstly choose values on five or eight o'clock as they rarely involve invalidate data like "NaN". Then, from the initial point, each five values are averaged. Finally, a new series is constructed from each dataset.
Moreover, to reduce time cost, a normalization method is introduced to make inputs range from 0 to 1. We choose the simplest formula below:
x(ti)normalized=x(ti)−MINMAX−MIN | (30) |
where MIN and MAX manifest the minimal and maximal values of a series x(t). Meanwhile, the teaching signal is going to be normalized under the same parameters. At last, outputs are disposed with similar rules.
Four user defined parameters affect predicting results of STLDNM*, three of which are parameters of the DNM model. Another important parameter is the np of the STL, especially for series with relatively stable periods. The np is decided by observing the sequence to be put into the STL. We make efforts to give a value closest to original period by using the scale line in the MATLAB toolbox. Besides, three structure parameters of DNM* are listed as 27 teams in Table 2. In order to provide a relatively comprehensive impression of the STLDNM*, three metrics mentioned above are included. Then, we execute a Friedman test [46] on the resulting values, thus obtaining the ranks of each dataset on each model as shown in Table 3. And Table 4 shows the final rank of each model, and the conclusion is that the Team 18 (ks=1,θs=1,η=0.1) performs the best under this evaluation.
Parameter | ks | θs | η | Parameter | ks | θs | η | Parameter | ks | θs | η |
Team 1 | 5 | 0 | 0.01 | Team 10 | 1 | 0 | 0.01 | Team 19 | 10 | 0 | 0.01 |
Team 2 | 5 | 0.5 | 0.01 | Team 11 | 1 | 0.5 | 0.01 | Team 20 | 10 | 0.5 | 0.01 |
Team 3 | 5 | 1 | 0.01 | Team 12 | 1 | 1 | 0.01 | Team 21 | 10 | 1 | 0.01 |
Team 4 | 5 | 0 | 0.05 | Team 13 | 1 | 0 | 0.05 | Team 22 | 10 | 0 | 0.05 |
Team 5 | 5 | 0 | 0.1 | Team 14 | 1 | 0 | 0.1 | Team 23 | 10 | 0 | 0.1 |
Team 6 | 5 | 0.5 | 0.05 | Team 15 | 1 | 0.5 | 0.05 | Team 24 | 10 | 0.5 | 0.05 |
Team 7 | 5 | 0.5 | 0.1 | Team 16 | 1 | 0.5 | 0.1 | Team 25 | 10 | 0.5 | 0.1 |
Team 8 | 5 | 1 | 0.05 | Team 17 | 1 | 1 | 0.05 | Team 26 | 10 | 1 | 0.05 |
Team 9 | 5 | 1 | 0.1 | Team 18 | 1 | 1 | 0.1 | Team 27 | 10 | 1 | 0.1 |
Team index | Ranks of parameters on | |||
BJ | SY | GZ | SH | |
1 | 15 | 18 | 6 | 14 |
2 | 2 | 10 | 13 | 12 |
3 | 14 | 16 | 12 | 23 |
4 | 25 | 8 | 9 | 13 |
5 | 10 | 13 | 7 | 19 |
6 | 3 | 15 | 15 | 8 |
7 | 5 | 9 | 14 | 6 |
8 | 12 | 14 | 23 | 24 |
9 | 11 | 11 | 19 | 21 |
10 | 26 | 21 | 11 | 10 |
11 | 9 | 6 | 24 | 5 |
12 | 6 | 5 | 10 | 2 |
13 | 21 | 22 | 16 | 15 |
14 | 16 | 12 | 21 | 18 |
15 | 7 | 1 | 1 | 9 |
16 | 8 | 2 | 4 | 4 |
17 | 4 | 3 | 3 | 3 |
18 | 1 | 4 | 5 | 1 |
19 | 13 | 17 | 18 | 7 |
20 | 18 | 23 | 20 | 17 |
21 | 23 | 27 | 25 | 27 |
22 | 19 | 7 | 8 | 22 |
23 | 17 | 19 | 2 | 11 |
24 | 24 | 20 | 17 | 16 |
25 | 20 | 25 | 26 | 26 |
26 | 22 | 26 | 22 | 20 |
27 | 27 | 24 | 27 | 25 |
Rank | Team Index | Rank | Team Index | Rank | Team Index |
1 | 12 | 10 | 19 | 19 | 14 |
2 | 7 | 11 | 9 | 20 | 23 |
3 | 17 | 12 | 5 | 21 | 26 |
4 | 13 | 13 | 21 | 22 | 15 |
5 | 10 | 14 | 18 | 23 | 11 |
6 | 8 | 15 | 3 | 24 | 22 |
7 | 6 | 16 | 4 | 25 | 25 |
8 | 20 | 17 | 2 | 26 | 24 |
9 | 16 | 18 | 1 | 27 | 27 |
Eight models are compared with STLDNM* in the experiment, including STLDNM*, STLDNM, DNM*, DNM, Elman, MLP, SVR, DeepAR and LSTM. As different conditions may provide different results, this paper chooses optimal parameters of each model shown in Table 5. Tables 6–8 shows the MSE, MAPE and MAE of each method on each dataset. These three evaluation metrics are simply described as follows:
Basic model | Using condition |
Elman | 10 hidden layers |
MLP | 15 hidden layers |
SVM | Radial basis function kernel |
DeepAR | GluonTS Toolbox |
DNM | —— |
DNM* | Simplified DNM model |
STLDNM | STL added |
STLDNM* | STL added |
LSTM | 100 hidden layers |
Data sets | MSE of | ||||||||
STLDNM* | STLDNM | DNM* | DNM | Elman | MLP | SVR | DeepAR | LSTM | |
BJ | 2.45E-01 | 4.79E+00 | 7.96E-01 | 4.74E+01 | 5.88E-01 | 3.40E-01 | 3.48E+00 | 3.49E+01 | 1.11E+00 |
SY | 2.65E-01 | 9.24E+00 | 1.49E+00 | 3.94E+01 | 4.71E-01 | 4.02E+00 | 3.20E+00 | 1.97E+01 | 1.09E+00 |
GZ | 5.42E-02 | 9.99E+00 | 6.65E-01 | 9.89E+00 | 1.07E-01 | 4.87E-01 | 1.69E-01 | 2.93E+02 | 4.53E-01 |
SH | 1.97E-01 | 1.12E+01 | 5.40E-01 | 1.70E+01 | 1.47E+00 | 1.90E+00 | 1.56E+00 | 1.45E+02 | 6.58E-01 |
Data sets | MAPE of | ||||||||
STLDNM* | STLDNM | DNM* | DNM | Elman | MLP | SVR | DeepAR | LSTM | |
BJ | 5.94E-06 | 4.42E-06 | 1.66E-05 | 3.90E-04 | 5.10E-06 | 4.68E-06 | 4.80E-06 | 1.53E-04 | 1.18E-05 |
SY | 8.31E-06 | 3.65E-05 | 1.30E-05 | 4.26E-04 | 1.14E-05 | 1.82E-07 | 8.75E-06 | 2.08E-04 | 7.73E-06 |
GZ | 8.10E-07 | 3.98E-05 | 1.15E-05 | 6.61E-06 | 6.98E-06 | 3.26E-06 | 1.37E-06 | 1.07E-03 | 4.03E-05 |
SH | 1.97E-06 | 2.13E-05 | 4.34E-06 | 4.95E-06 | 7.58E-06 | 6.72E-06 | 1.94E-06 | 7.94E-04 | 4.77E-06 |
Data sets | MAE of | ||||||||
STLDNM* | STLDNM | DNM* | DNM | Elman | MLP | SVR | DeepAR | LSTM | |
BJ | 3.03E-01 | 1.42E+00 | 7.62E-01 | 4.59E+00 | 5.46E-01 | 4.83E-01 | 1.33E+00 | 4.34E+00 | 7.69E-01 |
SY | 2.59E-01 | 1.99E+00 | 1.00E+00 | 3.53E+00 | 5.39E-01 | 1.70E+00 | 1.24E+00 | 3.49E+00 | 7.64E-01 |
GZ | 1.71E-01 | 1.99E+00 | 6.99E-01 | 1.77E+00 | 2.75E-01 | 5.29E-01 | 2.92E-01 | 1.58E+01 | 5.96E-01 |
SH | 2.26E-01 | 2.52E+00 | 6.15E-01 | 2.54E+00 | 9.38E-01 | 1.10E+00 | 8.70E-01 | 1.08E+01 | 5.93E-01 |
To measure how well the proposed model and others perform, we utilize three criterions to assess each model's performances. These metrics are computed between targeted vectors and outputs as the following formulas:
• MSE : Mean square error
MSE=1n∑ni=1(Ti−Oi)2 | (31) |
• MAPE : Mean absolute percentage error
MAPE=1n∑ni=1|Ti−OiTi| | (32) |
• MAE : Mean absolute error
MAE=1n∑ni=1|Ti−Oi| | (33) |
Later we employ the Friedman test on all the eight models to display which ranks the highest. Table 9 gives the rank of each model under the standard of MSE, MAPE and MAE. It concludes that STLDNM* performs the best. According to previous researches [47,48], to compensate the drawback that Friedman test can only judge whether there are differences among these models, Wilcoxon test is used for comparison between STLDNM* and other models. As can be seen from Table 10, STLDNM* outperforms its peers, except for MLP and SVM on MAPE. Besides, Table 11 shows the average time cost of each model. It can be seen that our model is the most efficient in terms of time cost. Tables 9–11 show that DNM* is better than other machine learning models, both in efficiency and precision.
Model | MSE | Model | MAPE | Model | MAE |
STLDNM* | 1 | STLDNM* | 2 | STLDNM* | 1 |
STLDNM | 7 | STLDNM | 7 | STLDNM | 7 |
DNM* | 4 | DNM* | 6 | DNM* | 4 |
DNM | 8 | DNM | 8 | DNM | 8 |
Elman | 2 | Elman | 5 | Elman | 2 |
MLP | 5 | MLP | 3 | MLP | 5 |
SVR | 5 | SVR | 1 | SVR | 5 |
DeepAR | 9 | DeepAR | 9 | DeepAR | 9 |
LSTM | 3 | LSTM | 4 | LSTM | 3 |
Compared models | Exact p -values of | ||
MSE | MAPE | MAE | |
STLDNM* vs Elman | 1.312E-03 | 3.906E-03 | 9.155E-05 |
STLDNM* vs MLP | 3.052E-05 | ≥ 0.2 | 3.052E-05 |
STLDNM* vs SVR | 4.272E-04 | ≥ 0.2 | 6.104E-05 |
STLDNM* vs DeepAR | 3.052E-05 | 3.052E-05 | 3.052E-05 |
STLDNM* vs DNM | 3.052E-05 | 2.441E-04 | 3.052E-05 |
STLDNM* vs DNM* | 3.052E-05 | 3.357E-04 | 3.052E-05 |
STLDNM* vs STLDNM | 3.052E-05 | 6.104E-05 | 3.052E-05 |
STLDNM* vs LSTM | 3.052E-05 | 2.533E-03 | 3.052E-05 |
Model | Average time cost (seconds) | Rank |
STLDNM* | 2.003739 | 1 |
STLDNM | 7.709 | 5 |
DNM* | 2.132047 | 2 |
DNM | 14.33868 | 6 |
Elman | 6.26621 | 4 |
MLP | 4.766181 | 3 |
SVR | 113.448 | 8 |
DeepAR | Greater than 2 hours | 9 |
LSTM | 21.4106 | 7 |
The predicting results of four cities using several algorithms are shown in the Figures 5–8, respectively. Typically, we extract the Shenyang's averages of each algorithm and plot another graph as shown in Figure 9. Thus, a group of curves surrounding "y=0" line is generated. Although the STLDNM* has the least advantage on the SY dataset, it remains more stable than most algorithms except MLP. For the other three series, it maintains the minimum deviation value.
Finally, to verify the stability of each model, the convergence curve of each model is shown in Figure 10. Overall, DNM and STLDNM are worse than other models and converge rapidly after about 10 generations. While the improved STLDNM* and DNM* have obtained excellent performance, obviously superior to MLP and Elman. It is further verified that the improved DNM is suitable and efficient for time series.
Although DNM is less popular than SVR and DeepAR for weather forecasting, it stands out for its simplicity. The experimental results show that the combination of preprocessing technology, neural network, and classical learning algorithm can achieve excellent results.
It is significantly important that a precise prediction of weather data reminds people to realize anormal phenomenon and make instant preparations, aiming to reduce loss to the least. However, forecasting consequences with high precision and efficiency is difficult due to various factors, such as variability and volatility.
In this paper, we present a new method combining decomposition method STL and prediction model DNM for dew point series. The STLDNM* model is applied to meteorology and the dew point of different cities in China is predicted successfully. Regarding STL as the preprocessing method, LSM and DNM* as the forecast method, BP as the learning algorithm, this combination has achieved excellent accuracy in the application of weather forecast.
DNM*, as a variation of DNM, is a single-input-single-output model that is more suitable to one-dimensional time series. The results show that the proposed model is superior to MLP, Elman, SVR, DeepAR in terms of time cost, convergence rate and prediction accuracy. And although the LSTM performs well in financial field, it does not fit weather data. In addition, Friedman test and Wilcoxon test were used to verify the above conclusions in terms of MSE, MAPE and MAE. Moreover, STLDNM* and DNM* are significantly better than DNM in stability. As a consequence, STLDNM* has great potential and prospects for development in more areas, whose performance in other types of series will be further discussed in the future.
This research was supported by the National Natural Science Foundation of China (NSFC) under Grant No. 12105120.
The authors declare there is no conflict of interest.
[1] |
M. Fathi, M. H. Kashani, S. M. Jameii, E. Mahdipour, Big data analytics in weather forecasting: A systematic review, Arch. Comput. Methods Eng., 29 (2022), 1247–1275. https://doi.org/10.1007/s11831-021-09616-4 doi: 10.1007/s11831-021-09616-4
![]() |
[2] |
J. S. Leu, K. W. Su, C. T. Chen, Ambient mesoscale weather forecasting system featuring mobile augmented reality, Multimedia Tools Appl., 72 (2014), 1585–1609. https://doi.org/10.1007/s11042-013-1462-4 doi: 10.1007/s11042-013-1462-4
![]() |
[3] |
P. Roudier, B. Muller, P. d'Aquino, C. Roncoli, M. A. Soumaré, L. Batté, et al., The role of climate forecasts in smallholder agriculture: Lessons from participatory research in two communities in Senegal, Clim. Risk Manage., 2 (2014), 42–55. https://doi.org/10.1016/j.crm.2014.02.001 doi: 10.1016/j.crm.2014.02.001
![]() |
[4] |
S. C. Gao, M. C. Zhou, Z. Q. Wang, D. Sugiyama, J. Cheng, J. Wang, et al., Fully complex-valued dendritic neuron model, IEEE Trans. Neural Networks Learn. Syst., 2021 (2021), 1–14. https://doi.org/10.1109/TNNLS.2021.3105901 doi: 10.1109/TNNLS.2021.3105901
![]() |
[5] |
J. Shi, W. J. Lee, Y. Liu, Y. Yang, P. Wang, Forecasting power output of photovoltaic systems based on weather classification and support vector machines, IEEE Trans. Ind. Appl., 48 (2012), 1064–1069. https://doi.org/10.1109/TIA.2012.2190816 doi: 10.1109/TIA.2012.2190816
![]() |
[6] |
D. Lazos, A. B. Sproul, M. Kay, Optimisation of energy management in commercial buildings with weather forecasting inputs: A review, Renewable Sustainable Energy Rev., 39 (2014), 587–603. https://doi.org/10.1016/j.rser.2014.07.053 doi: 10.1016/j.rser.2014.07.053
![]() |
[7] |
D. B. Jia, W. X. Xu, D. Z. Liu, Z. X. Xu, Z. M. Zhong, X. X. Ban, Verification of classification model and dendritic neuron model based on machine learning, Discrete Dyn. Nat. Soc., 2022 (2022). https://doi.org/10.1155/2022/3259222 doi: 10.1155/2022/3259222
![]() |
[8] |
Q. H. Li, X. L. Wang, H. B. Yang, X. C. Liu, Research on water vapor release and adsorption mechanism to improve the measurement of dew Point humidity sensor, IEEE Sens. J., 21 (2021), 14666–14676. https://doi.org/10.1109/JSEN.2021.3074647 doi: 10.1109/JSEN.2021.3074647
![]() |
[9] |
J. J. Cheng, G. Y. Yuan, M. C. Zhou, S. Gao, C. Liu, H. Duan, A fluid mechanics-based data flow model to estimate VANET capacity, IEEE Trans. Intell. Transp. Syst., 21 (2020), 2603–2614. https://doi.org/10.1109/TITS.2019.2921074 doi: 10.1109/TITS.2019.2921074
![]() |
[10] |
M. G. Schultz, C. Betancourt, B. Gong, F. Kleinert, M. Langguth, L. H. Leufen, et al., Can deep learning beat numerical weather prediction, Phil. Trans. R. Soc. A, 379 (2021). https://doi.org/10.1098/rsta.2020.0097 doi: 10.1098/rsta.2020.0097
![]() |
[11] |
J. J. Cheng, X. Wu, M. C. Zhou, S. C. Gao, Z. H. Huang, C. Liu, A novel method for detecting new overlapping community in complex evolving networks, IEEE Trans. Syst. Man Cybern. Syst., 49 (2019), 1832–1844. https://doi.org/10.1109/TSMC.2017.2779138 doi: 10.1109/TSMC.2017.2779138
![]() |
[12] |
D. B. Jia, Y. Fujishita, C. H. Li, Y. Todo, H. W. Dai, Validation of large-scale classification problem in dendritic neuron model using particle antagonism mechanism, Electronics, 9 (2020). https://doi.org/10.3390/electronics9050792 doi: 10.3390/electronics9050792
![]() |
[13] |
J. J. Cheng, M. J. Chen, M. C. Zhou, S. C. Gao, C. N. Liu, C Liu, Overlapping community change-point detection in an evolving network, IEEE Trans. Big Data, 6 (2020), 189–200. https://doi.org/10.1109/TBDATA.2018.2880780 doi: 10.1109/TBDATA.2018.2880780
![]() |
[14] |
K. Fu, H. Li, P. Deng, Chaotic time series prediction using DTIGNet based on improved temporal-inception and GRU, Chaos, Solitons Fractals, 159 (2022), 2022, 112183. https://doi.org/10.1016/j.chaos.2022.112183 doi: 10.1016/j.chaos.2022.112183
![]() |
[15] |
H. Abbasimehr, F. S. Baghery, A novel time series clustering method with fine-tuned support vector regression for customer behavior analysis, Expert Syst. Appl., 204 (2022), 117584. https://doi.org/10.1016/j.eswa.2022.117584 doi: 10.1016/j.eswa.2022.117584
![]() |
[16] |
M. M. Öztürk, Initializing hyper-parameter tuning with a metaheuristic-ensemble method: A case study using time series weather data, Evol. Intell., 2022 (2022). https://doi.org/10.1007/s12065-022-00717-y doi: 10.1007/s12065-022-00717-y
![]() |
[17] |
D. B. Jia, H. W. Dai, Y. Takashima, T. Nishio, K. Hirobayashi, M. Hasegawa, et al., EEG processing in internet of medical things using non-harmonic analysis: Application and evolution for SSVEP responses, IEEE Access, 7 (2019), 11318–11327. https://doi.org/10.1109/ACCESS.2019.2892188 doi: 10.1109/ACCESS.2019.2892188
![]() |
[18] |
W. X. Xu, D. B. Jia, Z. M. Zhong, C. H. Li, Z. X. Xu, Intelligent dendritic neural model for classification problems, Symmetry, 14 (2022). https://doi.org/10.3390/sym14010011 doi: 10.3390/sym14010011
![]() |
[19] |
M. Rabbani, M. Musarat, W. Alaloul, M. Rabbani, A. Maqsoom, S. Ayub, et al., A comparison between seasonal autoregressive integrated moving average (SARIMA) and exponential smoothing (ES) based on time series model for forecasting road accidents, Arabian J. Sci. Eng., 46 (2021), 11113–11138. https://doi.org/10.1007/s13369-021-05650-3 doi: 10.1007/s13369-021-05650-3
![]() |
[20] |
J. N. K. Liu, B. N. L. Li, T. S. Dillon, An improved naive Bayesian classifier technique coupled with a novel input solution method[rainfall prediction], IEEE Trans. Syst. Man Cybern. Part C Appl. Rev., 31 (2021), 249–256. https://doi.org/10.1109/5326.941848 doi: 10.1109/5326.941848
![]() |
[21] |
F. Wang, Z. Zhen, Z. Mi, H. Sun, S. Su, G. Yang, Solar irradiance feature extraction and support vector machines based weather status pattern recognition model for short-term photovoltaic power forecasting, Energy Build., 86 (2015), 427–438. https://doi.org/10.1016/j.enbuild.2014.10.002 doi: 10.1016/j.enbuild.2014.10.002
![]() |
[22] |
J. An, F. Yin, M. Wu, J. She, X. Chen, Multisource wind speed fusion method for short-term wind power prediction, IEEE Trans. Ind. Inf., 17 (2021), 5927–5937. https://doi.org/10.1109/TⅡ.2020.3006928 doi: 10.1109/TⅡ.2020.3006928
![]() |
[23] |
J. Sun, S. C. Gao, H. W. Dai, J. Cheng, M. Zhou, J. Wang, Bi-objective elite differential evolution algorithm for multivalued logic networks, IEEE Trans. Cybern., 50 (2020), 233–246. https://doi.org/10.1109/TCYB.2018.2868493 doi: 10.1109/TCYB.2018.2868493
![]() |
[24] |
S. C. Gao, Y. Yu, Y. Wang, J. Wang, J. Cheng, M. Zhou, Chaotic local search-based differential evolution algorithms for optimization, IEEE Trans. Syst. Man Cybern.: Syst., 51 (2021), 3954–3967. https://doi.org/10.1109/TSMC.2019.2956121 doi: 10.1109/TSMC.2019.2956121
![]() |
[25] |
D. B. Jia, K. Yanagisawa, M. Hasegawa, S. Hirobayashi, H. Tagoshi, T. Narikawa, et al., Time-frequency based non-harmonic analysis to reduce line noise impact for LIGO observation system, Astron. Comput., 25 (2018), 238–246. https://doi.org/10.1016/j.ascom.2018.10.003 doi: 10.1016/j.ascom.2018.10.003
![]() |
[26] | Y. Cheng, R. Wu, The research of aviation dangerous weather forecast for fog and haze based on BP neural network, in Proceedings of the 5th International Conference on Electrical Engineering and Automatic Control, Springer, 367 (2016), 877–883. https://doi.org/10.1007/978-3-662-48768-6_97 |
[27] |
X. M. Zhang, Y. Q. Zhou, H. J. Huang, Q. F. Luo, Enhanced salp search algorithm for optimization extreme learning machine and application to dew point temperature prediction, Int. J. Comput. Intell. Syst., 15 (2022). https://doi.org/10.1007/s44196-022-00160-y doi: 10.1007/s44196-022-00160-y
![]() |
[28] |
S. Gul, M. Khan, N. B. Yoma, S. W. Shah, Sheheryar, Enhancing the correlation between the quality and intelligibility objective metrics with the subjective scores by shallow feed forward neural network for time–frequency masking speech separation algorithms, Appl. Acoust., 188 (2022), 108539. https://doi.org/10.1016/j.apacoust.2021.108539 doi: 10.1016/j.apacoust.2021.108539
![]() |
[29] |
D. B. Jia, K. Yanagisawa, Y. Ono, K. Hirobayashi, M. Hasegawa, S. Hirobayashi, et al., Multiwindow nonharmonic analysis method for gravitational waves, IEEE Access, 6 (2018), 48645–48655. https://doi.org/10.1109/ACCESS.2018.2867494 doi: 10.1109/ACCESS.2018.2867494
![]() |
[30] |
B. R. Murlidar, H. Nguyen, J. Rostami, X. N. Bui, D. J. Armaghani, P. Ragam, et al., Prediction of flyrock distance induced by mine blasting using a novel Harris Hawks optimization-based multi-layer perceptron neural network, J. Rock Mech. Geotech. Eng., 13 (2021), 1413–1427. https://doi.org/10.1016/j.jrmge.2021.08.005 doi: 10.1016/j.jrmge.2021.08.005
![]() |
[31] |
J. J. Cheng, G. Y. Yuan, S. C. Gao, M. C. Zhou, C. Liu, H. Duan, et al., Accessibility analysis and modeling for IoV in an urban scene, IEEE Trans. Veh. Technol., 69 (2020), 4246–4256. https://doi.org/10.1109/TVT.2020.2970553 doi: 10.1109/TVT.2020.2970553
![]() |
[32] |
X. X. Qian, Y. R. Wang, S. C. Gao, Y. K. Todo, S. C. Gao, Mr2DNM: A novel mutual information-based dendritic neuron model, Comput. Intell. Neurosci., 2019 (2019). https://doi.org/10.1155/2019/7362931 doi: 10.1155/2019/7362931
![]() |
[33] |
M. Dong, H. Wu, H. Hu, R. Azzam, L. Zhang, Z. Zheng, et al., Deformation prediction of unstable slopes based on real-time monitoring and DeepAR model, Sensors, 21 (2021). https://doi.org/10.3390/s21010014 doi: 10.3390/s21010014
![]() |
[34] |
R. B. Jin, Z. H. Chen, K. Y. Wu, M. Wu, X. L. Li, R. Q. Yan, Bi-LSTM-based two-stream network for machine remaining useful life prediction, IEEE Trans. Instrum. Meas., 71 (2022), 1–10. https://doi.org/10.1109/TIM.2022.3167778 doi: 10.1109/TIM.2022.3167778
![]() |
[35] |
Q. Li, J. H. Tan, J. Wang, H. C. Chen, A multimodal event-driven lstm model for stock prediction using online news, IEEE Trans. Knowl. Data Eng., 33 (2021), 3323–3337. https://doi.org/10.1109/TKDE.2020.2968894 doi: 10.1109/TKDE.2020.2968894
![]() |
[36] |
F. G. Liu, Z. W. Zhang, R. L. Zhou, Automatic modulation recognition based on CNN and GRU, Tsinghua Sci. Technol., 27 (2022), 422–431. https://doi.org/10.26599/TST.2020.9010057 doi: 10.26599/TST.2020.9010057
![]() |
[37] |
X. Lin, F. R. Bi, X. Yang, X. Y. Bi, An echo state network with improved topology for time series prediction, IEEE Sens. J., 22 (2022), 5869–5878. https://doi.org/10.1109/JSEN.2022.3148742 doi: 10.1109/JSEN.2022.3148742
![]() |
[38] |
X. S. Yao, Y. N. Shao, S. Y. Fan, S. X. Cao, Echo state network with multiple delayed outputs for multiple delayed time series prediction, J. Franklin Inst. 359 (2022), 11089–11107. https://doi.org/10.1016/j.jfranklin.2022.09.059 doi: 10.1016/j.jfranklin.2022.09.059
![]() |
[39] |
W. Chen, J. Sun, S. Gao, J. J. Cheng, J. Wang, Y. Todo, Using a single dendritic neuron to forecast tourist arrivals to Japan, IEICE Trans. Inf. Syst., E100.D (2017), 190–202. https://doi.org/10.1587/transinf.2016EDP7152 doi: 10.1587/transinf.2016EDP7152
![]() |
[40] |
D. B. Jia, C. H. Li, Q. Liu, Q. Yu, X. Meng, Z. Zhong, et al., Application and evolution for neural network and signal processing in large-scale systems, Complexity, 2021 (2021). https://doi.org/10.1155/2021/6618833 doi: 10.1155/2021/6618833
![]() |
[41] |
S. Gao, M. Zhou, Y. Wang, J. Cheng, H. Yachi, J. Wang, Dendritic neuron model with effective learning algorithms for classification, approximation, and prediction, IEEE Trans. Neural Networks Learn. Syst., 30 (2019), 601–614. https://doi.org/10.1109/TNNLS.2018.2846646 doi: 10.1109/TNNLS.2018.2846646
![]() |
[42] |
M. Chaloupka, Historical trends, seasonality and spatial synchrony in green sea turtle egg production, Biol. Conserv., 101 (2001), 263–279. https://doi.org/10.1016/S0006-3207(00)00199-3 doi: 10.1016/S0006-3207(00)00199-3
![]() |
[43] |
T. Zhou, S. Gao, J. Wang, C. Chu, Y. Todo, Z. Tang, Financial time series prediction using a dendritic neuron model, Knowledge-Based Syst., 105 (2016), 214–224. https://doi.org/10.1016/j.knosys.2016.05.031 doi: 10.1016/j.knosys.2016.05.031
![]() |
[44] |
H. T. He, S. C. Gao, T. Jin, S. Sato, X. Y. Zhang, A seasonal-trend decomposition-based dendritic neuron model for financial time series prediction, Appl. Soft Comput., 108 (2021), 107488. https://doi.org/10.1016/j.asoc.2021.107488 doi: 10.1016/j.asoc.2021.107488
![]() |
[45] |
Z. J. Sha, L. Hu, Y. Todo, J. Ji, S. Gao, Z. Tang, A breast cancer classifier using a neuron model with dendritic nonlinearity, IEICE Trans. Inf. Syst., E98.D (2015), 1365–1376. https://doi.org/10.1587/transinf.2014EDP7418 doi: 10.1587/transinf.2014EDP7418
![]() |
[46] |
H. Li, X. T. Liu, D. B. Jia, Y. Y. Chen, P. F. Hou, H. N. Li, Research on chest radiography recognition model based on deep learning, Math. Biosci. Eng., 19 (2022), 11768–11781. https://doi.org/10.3934/mbe.2022548 doi: 10.3934/mbe.2022548
![]() |
[47] | J. Demšar, Statistical comparisons of classifiers over multiple data sets, J. Mach. Learn. Res., 7 (2006), 1–30. |
[48] |
Y. Cheng, W. N. Jia, R. H. Chi, A. Li, A clustering analysis method with high reliability based on Wilcoxon-Mann-Whitney testing, IEEE Access, 9 (2021), 19776–19787. https://doi.org/10.1109/ACCESS.2021.3053244 doi: 10.1109/ACCESS.2021.3053244
![]() |
1. | Dongbao Jia, Ming Cao, Wenbin Hu, Jing Sun, Hui Li, Yichen Wang, Weijie Zhou, Tiancheng Yin, Ran Qian, Multi-Objective Combinatorial Optimization Algorithm Based on Asynchronous Advantage Actor–Critic and Graph Transformer Networks, 2024, 13, 2079-9292, 3842, 10.3390/electronics13193842 | |
2. | Meizhen Deng, Yimeng Liu, Ling Chen, AI-driven innovation in ethnic clothing design: an intersection of machine learning and cultural heritage, 2023, 31, 2688-1594, 5793, 10.3934/era.2023295 | |
3. | Dongbao Jia, Ming Cao, Jing Sun, Feimeng Wang, Wei Xu, Yichen Wang, Interval Constrained Multi-Objective Optimization Scheduling Method for Island-Integrated Energy Systems Based on Meta-Learning and Enhanced Proximal Policy Optimization, 2024, 13, 2079-9292, 3579, 10.3390/electronics13173579 | |
4. | Leena Jenefa, Dr.Karuppasamy Ramanathan, Namita Mishra, Vishnu Vishnu, M. Jayakumar, S. Ashok, 2024, chapter 6, 9798369322840, 127, 10.4018/979-8-3693-2284-0.ch006 | |
5. | Pengfa He, Huaiyu Dong, Hailiang Liu, Cunli Zhang, 2024, A Monte Carlo Analysis of Condensation Risk from Sensor Error in Magnetic Resonance (MR) Cabinet, 979-8-3503-9028-5, 541, 10.1109/ISSET62871.2024.10779835 | |
6. | Dongbao Jia, Rui Ma, Weixiang Xu, Shiwei Zhao, Wenjun Ruan, Zhongxun Xu, Gravitational wave signal prediction technique based on advanced seasonal-trend decomposition using Loess, 2025, 42, 0264-9381, 065002, 10.1088/1361-6382/adb2d5 | |
7. | Haibo Li, Yongbo Yu, Zhenbo Zhao, Xiaokang Tang, A Time Series Short-Term Prediction Method Based on Multi-Granularity Event Matching and Alignment, 2024, 78, 1546-2226, 653, 10.32604/cmc.2023.046424 |
Weather data | Original Period | Chosen Sequence Length | Maximum Lyapunov Exponent |
Beijing's dew points (BJ) | 2010.01–2015.12 | 2187 | 0.6238 |
Shenyang's dew points (SY) | 2010.01–2015.12 | 2175 | 0.7034 |
Guangzhou's dew points (GZ) | 2010.01–2015.12 | 2183 | 0.1897 |
Shanghai's dew points (SY) | 2010.01–2015.12 | 2183 | 0.5258 |
Parameter | ks | θs | η | Parameter | ks | θs | η | Parameter | ks | θs | η |
Team 1 | 5 | 0 | 0.01 | Team 10 | 1 | 0 | 0.01 | Team 19 | 10 | 0 | 0.01 |
Team 2 | 5 | 0.5 | 0.01 | Team 11 | 1 | 0.5 | 0.01 | Team 20 | 10 | 0.5 | 0.01 |
Team 3 | 5 | 1 | 0.01 | Team 12 | 1 | 1 | 0.01 | Team 21 | 10 | 1 | 0.01 |
Team 4 | 5 | 0 | 0.05 | Team 13 | 1 | 0 | 0.05 | Team 22 | 10 | 0 | 0.05 |
Team 5 | 5 | 0 | 0.1 | Team 14 | 1 | 0 | 0.1 | Team 23 | 10 | 0 | 0.1 |
Team 6 | 5 | 0.5 | 0.05 | Team 15 | 1 | 0.5 | 0.05 | Team 24 | 10 | 0.5 | 0.05 |
Team 7 | 5 | 0.5 | 0.1 | Team 16 | 1 | 0.5 | 0.1 | Team 25 | 10 | 0.5 | 0.1 |
Team 8 | 5 | 1 | 0.05 | Team 17 | 1 | 1 | 0.05 | Team 26 | 10 | 1 | 0.05 |
Team 9 | 5 | 1 | 0.1 | Team 18 | 1 | 1 | 0.1 | Team 27 | 10 | 1 | 0.1 |
Team index | Ranks of parameters on | |||
BJ | SY | GZ | SH | |
1 | 15 | 18 | 6 | 14 |
2 | 2 | 10 | 13 | 12 |
3 | 14 | 16 | 12 | 23 |
4 | 25 | 8 | 9 | 13 |
5 | 10 | 13 | 7 | 19 |
6 | 3 | 15 | 15 | 8 |
7 | 5 | 9 | 14 | 6 |
8 | 12 | 14 | 23 | 24 |
9 | 11 | 11 | 19 | 21 |
10 | 26 | 21 | 11 | 10 |
11 | 9 | 6 | 24 | 5 |
12 | 6 | 5 | 10 | 2 |
13 | 21 | 22 | 16 | 15 |
14 | 16 | 12 | 21 | 18 |
15 | 7 | 1 | 1 | 9 |
16 | 8 | 2 | 4 | 4 |
17 | 4 | 3 | 3 | 3 |
18 | 1 | 4 | 5 | 1 |
19 | 13 | 17 | 18 | 7 |
20 | 18 | 23 | 20 | 17 |
21 | 23 | 27 | 25 | 27 |
22 | 19 | 7 | 8 | 22 |
23 | 17 | 19 | 2 | 11 |
24 | 24 | 20 | 17 | 16 |
25 | 20 | 25 | 26 | 26 |
26 | 22 | 26 | 22 | 20 |
27 | 27 | 24 | 27 | 25 |
Rank | Team Index | Rank | Team Index | Rank | Team Index |
1 | 12 | 10 | 19 | 19 | 14 |
2 | 7 | 11 | 9 | 20 | 23 |
3 | 17 | 12 | 5 | 21 | 26 |
4 | 13 | 13 | 21 | 22 | 15 |
5 | 10 | 14 | 18 | 23 | 11 |
6 | 8 | 15 | 3 | 24 | 22 |
7 | 6 | 16 | 4 | 25 | 25 |
8 | 20 | 17 | 2 | 26 | 24 |
9 | 16 | 18 | 1 | 27 | 27 |
Basic model | Using condition |
Elman | 10 hidden layers |
MLP | 15 hidden layers |
SVM | Radial basis function kernel |
DeepAR | GluonTS Toolbox |
DNM | —— |
DNM* | Simplified DNM model |
STLDNM | STL added |
STLDNM* | STL added |
LSTM | 100 hidden layers |
Data sets | MSE of | ||||||||
STLDNM* | STLDNM | DNM* | DNM | Elman | MLP | SVR | DeepAR | LSTM | |
BJ | 2.45E-01 | 4.79E+00 | 7.96E-01 | 4.74E+01 | 5.88E-01 | 3.40E-01 | 3.48E+00 | 3.49E+01 | 1.11E+00 |
SY | 2.65E-01 | 9.24E+00 | 1.49E+00 | 3.94E+01 | 4.71E-01 | 4.02E+00 | 3.20E+00 | 1.97E+01 | 1.09E+00 |
GZ | 5.42E-02 | 9.99E+00 | 6.65E-01 | 9.89E+00 | 1.07E-01 | 4.87E-01 | 1.69E-01 | 2.93E+02 | 4.53E-01 |
SH | 1.97E-01 | 1.12E+01 | 5.40E-01 | 1.70E+01 | 1.47E+00 | 1.90E+00 | 1.56E+00 | 1.45E+02 | 6.58E-01 |
Data sets | MAPE of | ||||||||
STLDNM* | STLDNM | DNM* | DNM | Elman | MLP | SVR | DeepAR | LSTM | |
BJ | 5.94E-06 | 4.42E-06 | 1.66E-05 | 3.90E-04 | 5.10E-06 | 4.68E-06 | 4.80E-06 | 1.53E-04 | 1.18E-05 |
SY | 8.31E-06 | 3.65E-05 | 1.30E-05 | 4.26E-04 | 1.14E-05 | 1.82E-07 | 8.75E-06 | 2.08E-04 | 7.73E-06 |
GZ | 8.10E-07 | 3.98E-05 | 1.15E-05 | 6.61E-06 | 6.98E-06 | 3.26E-06 | 1.37E-06 | 1.07E-03 | 4.03E-05 |
SH | 1.97E-06 | 2.13E-05 | 4.34E-06 | 4.95E-06 | 7.58E-06 | 6.72E-06 | 1.94E-06 | 7.94E-04 | 4.77E-06 |
Data sets | MAE of | ||||||||
STLDNM* | STLDNM | DNM* | DNM | Elman | MLP | SVR | DeepAR | LSTM | |
BJ | 3.03E-01 | 1.42E+00 | 7.62E-01 | 4.59E+00 | 5.46E-01 | 4.83E-01 | 1.33E+00 | 4.34E+00 | 7.69E-01 |
SY | 2.59E-01 | 1.99E+00 | 1.00E+00 | 3.53E+00 | 5.39E-01 | 1.70E+00 | 1.24E+00 | 3.49E+00 | 7.64E-01 |
GZ | 1.71E-01 | 1.99E+00 | 6.99E-01 | 1.77E+00 | 2.75E-01 | 5.29E-01 | 2.92E-01 | 1.58E+01 | 5.96E-01 |
SH | 2.26E-01 | 2.52E+00 | 6.15E-01 | 2.54E+00 | 9.38E-01 | 1.10E+00 | 8.70E-01 | 1.08E+01 | 5.93E-01 |
Model | MSE | Model | MAPE | Model | MAE |
STLDNM* | 1 | STLDNM* | 2 | STLDNM* | 1 |
STLDNM | 7 | STLDNM | 7 | STLDNM | 7 |
DNM* | 4 | DNM* | 6 | DNM* | 4 |
DNM | 8 | DNM | 8 | DNM | 8 |
Elman | 2 | Elman | 5 | Elman | 2 |
MLP | 5 | MLP | 3 | MLP | 5 |
SVR | 5 | SVR | 1 | SVR | 5 |
DeepAR | 9 | DeepAR | 9 | DeepAR | 9 |
LSTM | 3 | LSTM | 4 | LSTM | 3 |
Compared models | Exact p -values of | ||
MSE | MAPE | MAE | |
STLDNM* vs Elman | 1.312E-03 | 3.906E-03 | 9.155E-05 |
STLDNM* vs MLP | 3.052E-05 | ≥ 0.2 | 3.052E-05 |
STLDNM* vs SVR | 4.272E-04 | ≥ 0.2 | 6.104E-05 |
STLDNM* vs DeepAR | 3.052E-05 | 3.052E-05 | 3.052E-05 |
STLDNM* vs DNM | 3.052E-05 | 2.441E-04 | 3.052E-05 |
STLDNM* vs DNM* | 3.052E-05 | 3.357E-04 | 3.052E-05 |
STLDNM* vs STLDNM | 3.052E-05 | 6.104E-05 | 3.052E-05 |
STLDNM* vs LSTM | 3.052E-05 | 2.533E-03 | 3.052E-05 |
Model | Average time cost (seconds) | Rank |
STLDNM* | 2.003739 | 1 |
STLDNM | 7.709 | 5 |
DNM* | 2.132047 | 2 |
DNM | 14.33868 | 6 |
Elman | 6.26621 | 4 |
MLP | 4.766181 | 3 |
SVR | 113.448 | 8 |
DeepAR | Greater than 2 hours | 9 |
LSTM | 21.4106 | 7 |
Weather data | Original Period | Chosen Sequence Length | Maximum Lyapunov Exponent |
Beijing's dew points (BJ) | 2010.01–2015.12 | 2187 | 0.6238 |
Shenyang's dew points (SY) | 2010.01–2015.12 | 2175 | 0.7034 |
Guangzhou's dew points (GZ) | 2010.01–2015.12 | 2183 | 0.1897 |
Shanghai's dew points (SY) | 2010.01–2015.12 | 2183 | 0.5258 |
Parameter | ks | θs | η | Parameter | ks | θs | η | Parameter | ks | θs | η |
Team 1 | 5 | 0 | 0.01 | Team 10 | 1 | 0 | 0.01 | Team 19 | 10 | 0 | 0.01 |
Team 2 | 5 | 0.5 | 0.01 | Team 11 | 1 | 0.5 | 0.01 | Team 20 | 10 | 0.5 | 0.01 |
Team 3 | 5 | 1 | 0.01 | Team 12 | 1 | 1 | 0.01 | Team 21 | 10 | 1 | 0.01 |
Team 4 | 5 | 0 | 0.05 | Team 13 | 1 | 0 | 0.05 | Team 22 | 10 | 0 | 0.05 |
Team 5 | 5 | 0 | 0.1 | Team 14 | 1 | 0 | 0.1 | Team 23 | 10 | 0 | 0.1 |
Team 6 | 5 | 0.5 | 0.05 | Team 15 | 1 | 0.5 | 0.05 | Team 24 | 10 | 0.5 | 0.05 |
Team 7 | 5 | 0.5 | 0.1 | Team 16 | 1 | 0.5 | 0.1 | Team 25 | 10 | 0.5 | 0.1 |
Team 8 | 5 | 1 | 0.05 | Team 17 | 1 | 1 | 0.05 | Team 26 | 10 | 1 | 0.05 |
Team 9 | 5 | 1 | 0.1 | Team 18 | 1 | 1 | 0.1 | Team 27 | 10 | 1 | 0.1 |
Team index | Ranks of parameters on | |||
BJ | SY | GZ | SH | |
1 | 15 | 18 | 6 | 14 |
2 | 2 | 10 | 13 | 12 |
3 | 14 | 16 | 12 | 23 |
4 | 25 | 8 | 9 | 13 |
5 | 10 | 13 | 7 | 19 |
6 | 3 | 15 | 15 | 8 |
7 | 5 | 9 | 14 | 6 |
8 | 12 | 14 | 23 | 24 |
9 | 11 | 11 | 19 | 21 |
10 | 26 | 21 | 11 | 10 |
11 | 9 | 6 | 24 | 5 |
12 | 6 | 5 | 10 | 2 |
13 | 21 | 22 | 16 | 15 |
14 | 16 | 12 | 21 | 18 |
15 | 7 | 1 | 1 | 9 |
16 | 8 | 2 | 4 | 4 |
17 | 4 | 3 | 3 | 3 |
18 | 1 | 4 | 5 | 1 |
19 | 13 | 17 | 18 | 7 |
20 | 18 | 23 | 20 | 17 |
21 | 23 | 27 | 25 | 27 |
22 | 19 | 7 | 8 | 22 |
23 | 17 | 19 | 2 | 11 |
24 | 24 | 20 | 17 | 16 |
25 | 20 | 25 | 26 | 26 |
26 | 22 | 26 | 22 | 20 |
27 | 27 | 24 | 27 | 25 |
Rank | Team Index | Rank | Team Index | Rank | Team Index |
1 | 12 | 10 | 19 | 19 | 14 |
2 | 7 | 11 | 9 | 20 | 23 |
3 | 17 | 12 | 5 | 21 | 26 |
4 | 13 | 13 | 21 | 22 | 15 |
5 | 10 | 14 | 18 | 23 | 11 |
6 | 8 | 15 | 3 | 24 | 22 |
7 | 6 | 16 | 4 | 25 | 25 |
8 | 20 | 17 | 2 | 26 | 24 |
9 | 16 | 18 | 1 | 27 | 27 |
Basic model | Using condition |
Elman | 10 hidden layers |
MLP | 15 hidden layers |
SVM | Radial basis function kernel |
DeepAR | GluonTS Toolbox |
DNM | —— |
DNM* | Simplified DNM model |
STLDNM | STL added |
STLDNM* | STL added |
LSTM | 100 hidden layers |
Data sets | MSE of | ||||||||
STLDNM* | STLDNM | DNM* | DNM | Elman | MLP | SVR | DeepAR | LSTM | |
BJ | 2.45E-01 | 4.79E+00 | 7.96E-01 | 4.74E+01 | 5.88E-01 | 3.40E-01 | 3.48E+00 | 3.49E+01 | 1.11E+00 |
SY | 2.65E-01 | 9.24E+00 | 1.49E+00 | 3.94E+01 | 4.71E-01 | 4.02E+00 | 3.20E+00 | 1.97E+01 | 1.09E+00 |
GZ | 5.42E-02 | 9.99E+00 | 6.65E-01 | 9.89E+00 | 1.07E-01 | 4.87E-01 | 1.69E-01 | 2.93E+02 | 4.53E-01 |
SH | 1.97E-01 | 1.12E+01 | 5.40E-01 | 1.70E+01 | 1.47E+00 | 1.90E+00 | 1.56E+00 | 1.45E+02 | 6.58E-01 |
Data sets | MAPE of | ||||||||
STLDNM* | STLDNM | DNM* | DNM | Elman | MLP | SVR | DeepAR | LSTM | |
BJ | 5.94E-06 | 4.42E-06 | 1.66E-05 | 3.90E-04 | 5.10E-06 | 4.68E-06 | 4.80E-06 | 1.53E-04 | 1.18E-05 |
SY | 8.31E-06 | 3.65E-05 | 1.30E-05 | 4.26E-04 | 1.14E-05 | 1.82E-07 | 8.75E-06 | 2.08E-04 | 7.73E-06 |
GZ | 8.10E-07 | 3.98E-05 | 1.15E-05 | 6.61E-06 | 6.98E-06 | 3.26E-06 | 1.37E-06 | 1.07E-03 | 4.03E-05 |
SH | 1.97E-06 | 2.13E-05 | 4.34E-06 | 4.95E-06 | 7.58E-06 | 6.72E-06 | 1.94E-06 | 7.94E-04 | 4.77E-06 |
Data sets | MAE of | ||||||||
STLDNM* | STLDNM | DNM* | DNM | Elman | MLP | SVR | DeepAR | LSTM | |
BJ | 3.03E-01 | 1.42E+00 | 7.62E-01 | 4.59E+00 | 5.46E-01 | 4.83E-01 | 1.33E+00 | 4.34E+00 | 7.69E-01 |
SY | 2.59E-01 | 1.99E+00 | 1.00E+00 | 3.53E+00 | 5.39E-01 | 1.70E+00 | 1.24E+00 | 3.49E+00 | 7.64E-01 |
GZ | 1.71E-01 | 1.99E+00 | 6.99E-01 | 1.77E+00 | 2.75E-01 | 5.29E-01 | 2.92E-01 | 1.58E+01 | 5.96E-01 |
SH | 2.26E-01 | 2.52E+00 | 6.15E-01 | 2.54E+00 | 9.38E-01 | 1.10E+00 | 8.70E-01 | 1.08E+01 | 5.93E-01 |
Model | MSE | Model | MAPE | Model | MAE |
STLDNM* | 1 | STLDNM* | 2 | STLDNM* | 1 |
STLDNM | 7 | STLDNM | 7 | STLDNM | 7 |
DNM* | 4 | DNM* | 6 | DNM* | 4 |
DNM | 8 | DNM | 8 | DNM | 8 |
Elman | 2 | Elman | 5 | Elman | 2 |
MLP | 5 | MLP | 3 | MLP | 5 |
SVR | 5 | SVR | 1 | SVR | 5 |
DeepAR | 9 | DeepAR | 9 | DeepAR | 9 |
LSTM | 3 | LSTM | 4 | LSTM | 3 |
Compared models | Exact p -values of | ||
MSE | MAPE | MAE | |
STLDNM* vs Elman | 1.312E-03 | 3.906E-03 | 9.155E-05 |
STLDNM* vs MLP | 3.052E-05 | ≥ 0.2 | 3.052E-05 |
STLDNM* vs SVR | 4.272E-04 | ≥ 0.2 | 6.104E-05 |
STLDNM* vs DeepAR | 3.052E-05 | 3.052E-05 | 3.052E-05 |
STLDNM* vs DNM | 3.052E-05 | 2.441E-04 | 3.052E-05 |
STLDNM* vs DNM* | 3.052E-05 | 3.357E-04 | 3.052E-05 |
STLDNM* vs STLDNM | 3.052E-05 | 6.104E-05 | 3.052E-05 |
STLDNM* vs LSTM | 3.052E-05 | 2.533E-03 | 3.052E-05 |
Model | Average time cost (seconds) | Rank |
STLDNM* | 2.003739 | 1 |
STLDNM | 7.709 | 5 |
DNM* | 2.132047 | 2 |
DNM | 14.33868 | 6 |
Elman | 6.26621 | 4 |
MLP | 4.766181 | 3 |
SVR | 113.448 | 8 |
DeepAR | Greater than 2 hours | 9 |
LSTM | 21.4106 | 7 |